- 1Interactions Sol Plante Atmosphère, UMR1391, Institut National de Recherche Pour l'Agriculture, l'Alimentation et l'Environnement, Bordeaux Science Agro, Villenave d’Ornon, France
- 2Centre de Coopération Internationale en Recherche Agronomique pour le Développement, Centre National de la Recherche Scientifique, Interactions Sol Plante Atmosphère, Territoires, Environnement, Télédétection et Information Spatiale, University of Montpellier, AgroParisTech, Montpellier, France
- 3Géosciences Environnement Toulouse, UMR 5563, Université de Toulouse, Centre National de la Recherche Scientifique-IRD-OMP-Centre National d'Etudes Spatiales, Toulouse, France
- 4Centre National d'Etudes Spatiales, Toulouse, France
- 5INRIA Bordeaux Sud-Ouest, Geostat Team, Talence, France
- 6Laboratoire d'Etudes en Géophysique et Océanographie Spatiale, University of Toulouse, Centre National d'Etudes Spatiales/Centre National de la Recherche Scientifique/IRD/UT3, Toulouse, France
- 7Congo Basin Water Resources Research Center (CRREBaC) and the Regional School of Water, University of Kinshasa (UNIKIN), Kinshasa, Democratic Republic of Congo
- 8Department of Geology, Faculty of Sciences, University of Lubumbashi (UNILU), Lubumbashi, Democratic Republic of Congo
- 9VisioTerra, Champs-sur-Marne, France
Rivers play a crucial role in maintaining ecosystems and regional climates, while also providing essential water for irrigation and drinking. With the increasing impacts of climate change and human activities, rivers are becoming more prone to extreme events (droughts and floods) and induced erosion/deposition processes, making water-related risk management more challenging. The recent launch of the Surface Water and Ocean Topography (SWOT) mission, which focuses on continental surfaces with a spatial resolution of 100 m, has opened new avenues for applications in hydrology, hydrodynamics, and geomorphology. This study examines the initial results of the SWOT mission for sixteen rivers (ranging in width from 50 to 2,000 m) in the Cuvette Centrale of the Congo Basin, obtained in April 2023 during the fast sampling phase. The study assesses water surface elevations (WSE) and backscatter coefficient (σ0) measured from SWOT. Comparisons of WSE with data from other radar altimetry missions (Sentinel-3A and 3B, Jason-3, and Sentinel-6A) and GEDI data show high correlation coefficients of 0.977 (with a bias of 0.538 m) and 0.992 (with a bias of 1.011 m), respectively. The first maps of WSE slopes show realistic values, even in rivers less than 100 m wide, with steeper slopes upstream. Various WSE longitudinal profiles are retrieved with unprecedented spatial resolution, surpassing what other nadir altimetry missions have achieved. The σ0 values, between −10 and 20 dB on average, also appear consistent with other studies. These promising initial results pave the way for future studies on fluvial geomorphology dynamics and erosion/deposition processes from the new SWOT observations.
1 Introduction
Most of the Earth’s freshwater is found in glaciers and ice caps, groundwater and surface water. In the ‘surface water’ reservoir, the volume of freshwater originating from rivers represents only 0.006% of the total stock of freshwater on Earth (Gleick, 1993) but it plays an essential role in the water cycle (Wu et al., 2023). Rivers are also of fundamental ecological importance for the maintenance of ecosystems, the regional climate and the development of human societies. In particular, they provide the water needed for irrigation and freshwater for drinking (Boergens et al., 2017; Wu et al., 2023). Sometimes, they constitute transportation corridors for remote places (Tshimanga et al., 2022). They also play an essential role in hydrological and biogeochemical cycles (Yamazaki et al., 2019). In contrast to these essential aspects, river flooding causes the destruction of crops, settlements and infrastructure (Boergens et al., 2017). In addition, with climate change and increasing human activities, rivers are subject to unprecedented extreme events (floods and droughts) that are difficult to manage (Kreibich et al., 2022; Yang et al., 2021). It is therefore essential to study rivers in greater detail and to have a continuous spatio-temporal monitoring of water levels. However, over the last few decades, the number of in situ stations has sharply fallen especially in developing countries and moreover, access to them is often limited and their spatial coverage is unsuitable for regional/global monitoring (Group et al., 2001). To face this decline, Earth observations (EO) are increasingly used for monitoring surface water levels, extent and storage (Cretaux et al., 2023; Fassoni-Andrade et al., 2021; Papa et al., 2023; Papa and Frappart, 2021). Until recently, only radar altimetry among the different EO techniques was able to provide a temporally continuous monitoring of water levels, but spatially limited along the satellite groundtracks due to the nadir-looking configuration of the radar sensor (Abdalla et al., 2021; Cretaux et al., 2023). The Surface Water and Ocean Topography (SWOT) mission now permits an almost complete monitoring of inland water bodies at a spatial resolution of 100 m and a repeat cycle of 21 days (Fu et al., 2024). This unprecedented capability offers new perspectives for hydrology, hydrodynamics, and fluvial geomorphology applications such as the determination of river profiles due to the requirement of a maximum error of 1.7 cm/km for river slopes (Biancamaria et al., 2016).
The river profiles reflect both tectonic activity (i.e., upflift and subsidence which define the landscape) and morpho dynamics which is related to erosion and deposition processes modifying the topography (Seybold et al., 2021; Sinha and Parker, 1996; Whipple and Tucker, 1999). Generally concave, their slope is more pronounced in the upstream parts of the basin than downstream. Erosion of fluvial systems is governed by the total stream power which is function of the drainage area [a proxy of river discharge (Wobus et al., 2006)] and of the energy slope approximated by the water surface slope (Barker et al., 2008; Whipple and Tucker, 1999). While tectonics primarily drive river profile concavity, climate also significantly influences this characteristic (Chen et al., 2019).
EO-based observations of rivers profile and slope rely on the main following techniques: Global Positioning System (GPS)/Global Navigation Satellite Systems (GNSS) surveys, interpolations between the water levels derived from radar altimetry acquisitions, Synthetic Aperture Radar (SAR) Interferometry (InSAR). GPS/GNSS data surveys are generally based on the processing of data acquired by a mobile geodetic receiver that permits an accuracy of a few centimeters in the height estimates. This technique was used to estimate river profiles and slopes in large river basins such as the Amazon (Bourrel and Pouilly, 2004; Charriere et al., 2004; Callède et al., 2013; Medeiros Moreira, 2016), to generate dense surveys of river surface elevation along and across the river stream (Brasington et al., 2000), which can be used for validation of satellite estimates as in the case of the airborne campaign such as the ones made for preparing the SWOT mission (AirSWOT) (Altenau et al., 2017). Radar altimetry was also used to determine river profiles along large rivers such as Amazon, Congo, Brahmaputra and Mekong (Birkett et al., 2002; Boergens et al., 2017; Frappart et al., 2005; Jiang et al., 2017; Koblinsky et al., 1993; Leon et al., 2006). The major limitations of this technique are either the limited coverage of the radar altimetry groundtracks for most of the missions (from 80 km considering the 35-day repeat period of ERS/ENVISAT/SARAL to 315 km considering the ∼10-day repeat orbit from Topex-Poseidon/Jason-1,2,3/Sentinel-6 at the equator) or the low temporal resolution when using Cryosat-2 which has an equatorial cross-track separation of 7 km on its 369-day repeat orbit (see Frappart et al., 2017 for the orbital characteristics of the high accuracy radar altimetry missions) or using ICESat-2 (Scherer et al., 2023). Simultaneous SAR acquisitions at C-band from different incidence angles were achieved to generate the shuttle radar topography mission digital elevation model (SRTM DEM) using InSAR. The presence of winds and waves produces surface roughness that generates backscattering over rivers, and hence, water surface was derived. This information was used to derive reliable river profiles and slopes over rivers longer than 700 km over the whole Amazon Basin (LeFavour and Alsdorf, 2005). Although longitudinal profiles of water levels on different rivers have been carried out in all the studies mentioned above, spatial altimetry has limited spatial coverage, with too few data on rivers with strong hydrological dynamics.
In December 2022, the Surface Water Ocean Topography (SWOT) mission, the result of a cooperation between the Centre National d'Etudes Spatiales (CNES) and the National Aeronautics and Space Administration (NASA), was launched, and it is the first space mission dedicated to continental hydrology, including river monitoring. Unlike previous other radar altimetry missions such as the 10-day Jason-1/2/3/Sentinel-6A, 27-day Sentinel-3A/B and 35-day ERS-2/Envisat/Saral missions, this mission will provide coverage of all continental waters at a spatial and temporal resolution never before achieved. The aim of this study is to (1) explore the first results of the SWOT mission for retrieving hydrological parameters such as Water Surface Elevation (WSE), (2) compare SWOT data with other datasets, and (3) assess the contribution of this new mission compared with the other radar altimetry missions currently employed but not dedicated to continental hydrology.
2 Study site
The Congo Basin, located in equatorial Africa, is a transboundary basin that crosses nine countries: Zambia, Tanzania, Cameroon, Burundi, Rwanda, Republic of Congo, Democratic Republic of Congo, Angola and Central Africa Republic (Figure 1A; (Kitambo et al., 2022)). Its size and mean annual river flow of 3,687,000 km2 and 40,500 m3 s−1 respectively, makes it the second largest basin in the world after the Amazon (Alsdorf et al., 2016). The Congo Basin is mainly traversed by the 6,650 km-long Congo River, which rises in the southern Katanga Plateau in the southeastern Democratic Republic of Congo and flows into the Atlantic Ocean.
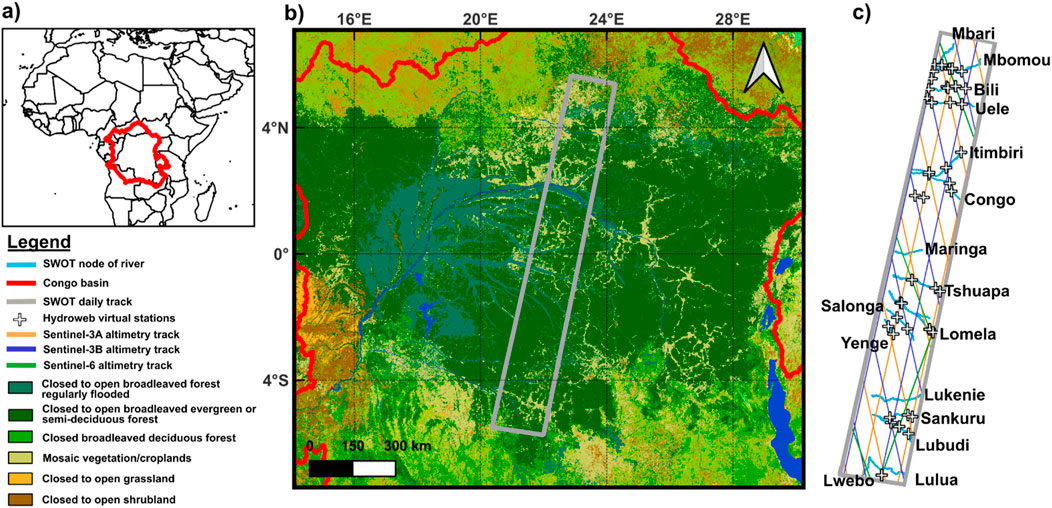
Figure 1. Location of the study site. (A) Location of the Congo Basin, (B) land cover over the Cuvette Centrale and (C) SWOT daily node track acquired on April 2023 over the Cuvette Centrale (rectangular gray frame).
Within the central basin is a shallow depression of 1,176,000 km2, called the “Cuvette Centrale”, and extends from coordinates 3°S to 3°N in latitude, and from 16°E to 22°E in longitude (Betbeder et al., 2014; Becker et al., 2018; Frappart et al., 2021). The Cuvette Centrale plays an essential role in the carbon and hydrological cycles of the Congo basin (Betbeder et al., 2014; Kitambo et al., 2022; 2023). This area was formed during the Pliocene epoch by a filling in of sediments to form a shallow basin with very few variations in topography (average slope less than 7 cm/km between Kisangni and Kinshasa, (Devroey, 1959)) and slow-flowing rivers contributing to the formation of large wetlands (Bwangoy et al., 2010). This area is bordered by high plateaus and mountains.
The Cuvette Centrale has an equatorial climate, with average annual rainfall ranging from 1,800 to 2,200 mm/year and an annual evaporation rate of 1,050 mm/year (Bultot, 1971). The vast floodplain of the Cuvette Centrale is a complex hydrological system, with a large number of tributaries and swamps (Datok et al., 2022). The vast Cuvette Centrale floodplain contains the confluences of the main Congo River and two of its major tributaries, the Ubangi River in the north and the Sangha River in the south. Water levels at the outlet of the Congo Basin are subject to a bimodal flooding regime, with a main high-water peak in November-December, a secondary peak in April-May, a main low-water peak in August and a lower low-water peak in February-March. These high-water periods cause localized flooding along the rivers (Frappart et al., 2021c).
These floods are at the origin of a very diverse vegetation in the Cuvette Centrale (Figure 1B). Indeed, this area is mainly covered by forested wetland and is one of the largest swamp forests in the world (Alsdorf et al., 2016; Betbeder et al., 2014; Dargie et al., 2017). Several cover types have been distinguished in the Cuvette Centrale: closed to open broadleaved forest regularly flooded located along rivers, closed to open broadleaved evergreen or semi-deciduous forest, closed broadleaved deciduous forest, mosaic of vegetation/croplands, grassland and shrubland (Figure 1B).
More details on the Cuvette Centrale’s geological history, physiography, hydrology and vegetation are given in Alsdorf et al. (2016), Betbeder et al. (2014), Datok et al. (2022), and Kadima et al. (2011).
3 Materials and methods
3.1 Surface water ocean topography (SWOT)
3.1.1 Mission characteristics
The Surface Water Ocean Topography (SWOT) mission was developed by the Centre National d'Etudes Spatiales (CNES) and the National Aeronautics and Space Administration (NASA), with contributions from the Canadian Space Agency (CSA) and the United-Kingdom Space Agency (UKSA). The mission was launched on 15 December 2022. After its launch, the first 3 months were used for technical verification, followed by 3 months for calibration/validation, and then a minimum of 36 months for Earth mapping (Fu et al., 2024). Its main objectives are to measure both oceans and continental water surfaces, making it the first space mission developed for continental hydrology. SWOT measures water surface elevation, slope and water masks. Water surface elevation (noted WSE in the rest of the manuscript) is defined as the distance between the top of the water surface and a given reference surface (geoid, ellipsoid), which is different from the water depth corresponding to the distance between the water surface and the river bed (Biancamaria et al., 2016). The SWOT mission has the capability to meet or exceed the science requirements on rivers wider than 100 m and probably below this width limit (Fu et al., 2024).
Several instruments composed the payload of the SWOT mission. As other classical radar altimetry missions, a nadir altimeter Poséidon-3C working in Ku and C bands is installed and provides information in one dimension. However, the novelty of the SWOT mission is the Ka-band Radar Interferometer (KaRIn), which is a Synthetic Aperture Radar (SAR) interferometer using Ka band (35.75 GHz) with nadir incidence angles ranging from 0.6° to 3.9° (Fjørtoft et al., 2014). Two SAR antennas separated by a 10 m mast provide, for the first time, high-resolution 2D maps of spatial water heights, among others, on either side of the nadir. KaRIn operates in bistatic mode and gives images with pixel size of ∼6 m in the direction of the satellite orbit and from 10 m to 60 m in the range direction [see Fjørtoft et al. (2014) for more details]. These pixel sizes are given for images acquired in “radar projection” and multilooked, and thus are not corresponding to geolocated projection. Finally, the spatial resolution is about 22 m in the azimuth direction. SWOT will observe all continental surfaces every 21 days with revisit times depending on the location on Earth.
Summarized information on the SWOT mission are provided in Table 1 and in Fjørtoft et al. (2014) and Biancamaria et al. (2016).
3.1.2 Products
Various products are available free of charge from CNES and NASA at https://search.earthdata.nasa.gov/(last accessed on 21 March 2024) and https://hydroweb.next.theia-land.fr/(last accessed on 6 March 2024). Six products are currently available for continental surfaces:
• L2_HR_PIXC: Water Mask Pixel Cloud
• L2_HR_PIXCVec: Water Mask Pixel Cloud Auxiliary Data
• L2_HR_Raster: Raster NetCDF 100 or 250 m
• L2_HR_RiverSP: River Single Pass Shapefile (reaches and nodes)
• L2_HR_RiverAvg: River Single Pass Shapefile (reaches and nodes)
• L2_HR_LakeSP: Lake Vector Single Pass Shapefile
• L2_HR_LakeAvg: Lake Vector Cycle-Averaged Shapefile
At present, data acquired during the calibration/validation period in April-May-June 2023 are available, and data acquired since the satellite was placed in nominal orbit (since July 2023) are being processed and will be delivered shortly. Products from the calibration/validation phase correspond to version 1.1, and those obtained during the nominal acquisition phase are identified by version 2.0.
For our study, we used data acquired during the calibration/validation phase and therefore have daily data. We used the shapefile SWOT Level 2 River Single-Pass Vector Data Product, version 1.1, identified as “L2_HR_RiverSP”. The product is more precisely the version C PIB0 of KaRIn science data products. The WSE, slope and width variables are available in this product. On the Cuvette Centrale du Congo, 18 runs were obtained between 7 April 2023 and 25 April 2023. The track of the data obtained is shown in Figure 1C. Sixteen rivers were overflown by the SWOT mission in this area during the calibration/validation phase (see their names and locations in Figure 1C).
The product used contains records for each reach of the river covered by the granule concerned, and initially identified as river reaches in the SWOT River Database (SWORD), defined by the SWOT scientific group. The SWORD database combines several global river and satellite datasets to define the nodes and reaches that will make up the SWOT river vector data products (Altenau et al., 2021). Each reach (∼10 km) is divided into a number of nodes in the SWORD database. Reaches are defined in nodes which are spacing of approximately 200 m along the river concerned. For node products, basic hydrological attributes are measured by SWOT as WSE, river width and water surface area. Measurements in this Shapefile River product are defined as dots. Backscatter coefficient (noted as Sigma0, σ0) is also available in this product, which tells us about the type of soil.
3.1.3 Method
3.1.3.1 All data
SWOT “L2_HR_RiverSP” data (i.e., WSE, river width and backscatter coefficient) were filtered to remove outliers in every grid cell contained in each reach. To do this, the Z-score method was used, based on the following calculation (Equation 1) (Seo, 2006):
With:
X: value to test
For data not to be deleted, its z-score must be between −3 and 3, which corresponds to a confidence level of 99%.
3.1.3.2 Slope
Mean of SWE for each river were smoothed using a sliding average on 10 values using the following equation for each point x of the river (Equation 2):
With:
n: number of sampling
N: consecutive values used to calculate the slipping mean, here 10 values
Using the WSE smoothed data, slope of WSE have been calculated for each river using the following Equation 3:
With:
y: water surface elevation changes between two consecutive points (mm)
x: distance difference between the two consecutive points (km)
3.2 Data intercomparison
3.2.1 Radar altimetry-based time series of water levels
As no in situ water levels are available in the Cuvette Centrale during the acquisition time period of SWOT, time series of water levels derived from Sentinel-3A (since 2016, 27-day repeat period) and 3B (since 2018, 27-day repeat period), Sentinel-6A (since 2020, 10-day repeat period) and Jason-3 (since 2016, 10-day repeat period) over the Cuvette Centrale (i.e., virtual stations) were used to compare with SWOT and GEDI WSE. Altimetry tracks for Jason-3, Sentinel-3A and 3B, and Sentinel-6A are shown in Figure 1C.
The principle of radar altimetry is as follows: a radar altimeter emits an electromagnetic wave in the nadir direction and measures the time it takes for the wave to make the round trip. The distance between the satellite and the Earth’s surface (called altimeter Range R0) is derived to an accuracy of a few centimeters. Knowing the satellite’s altitude H relative to a reference ellipsoid and considering the various types of corrections to be made (mainly related to wave propagation in the atmosphere, and geophysical corrections), it is then possible to retrieve the water height according to the following equation (Chelton et al., 2001; Frappart et al., 2017; Normandin et al., 2018):
ΔRpropagation + ΔRgeophysical parameters are defined as following:
ΔRion represents the atmospheric refraction range correction caused by the ionosphere’s free electron content and its dielectric properties, while ΔRdry accounts for the correction due to the dry gas component of the troposphere. ΔRwet, on the other hand, represents the correction associated with the water vapor and cloud liquid water content in the troposphere.
With ΔRsolid Earth and ΔRpole are the corrections respectively accounting for crustal vertical motions due to the solid Earth and pole tides.
For each altimetry mission used, the data processing steps are the same. The input data used are L2 input files supplied in GDR/IGDR format by the space agencies CNES, ESA and NASA data centers. The retracking algorithm used here is OCOG. The processing consists of the following three steps: (1) extraction, selection and reading of the measurements for each input file, (2) calculation of the altimetric water heights along each track and cycle and (3) extraction of the data. All these steps are detailed in Frappart et al. (2015) and Normandin et al. (2018).
Over our study site, 45 virtual stations, defined as a cross section between altimetry tracks and rivers, were used to compare water surface elevations from the Hydrowebnext database and from the SWOT mission. Locations of these virtual stations are also presented in Figure 1C with white crosses. All these virtual stations are freely available at Hydrowebnext (https://hydroweb.next.theia-land.fr/). Virtual station is a time series of water surface elevations.
3.2.2 GEDI lidar data
3.2.2.1 GEDI mission characteristics
The NASA Global Ecosystem Dynamics Investigation (GEDI) installed aboard the International Space Station (ISS) is a full-waveform LiDAR instrument. Since April 2019, it has produced high-quality measurements of surface vertical structures. It is equipped with three 1,064 nm lasers. The acquired footprints along the eight tracks are separated by 600 m across the track, and 60 m along the track, with a footprint diameter of 25 m. The echoed waveforms are digitized to a maximum of 1,246 bins with a vertical resolution of 1 ns (15 cm) (Dubayah et al., 2020).
3.2.2.2 GEDI data product
Two GEDI data products were used in this study, the level 1B (L1B), and level 2A (L2A). These data products (L1 & L2) are available from the NASA Land Processes Distributed Active Archive Center (LP DAAC). In this study, from the release version V2 of the L1B data product (Dubayah et al., 2021b) and L2A data product (Dubayah et al., 2021a), we extracted the following variables derived from the processing algorithm a1 which demonstrated the best overall performance over water bodies (Fayad et al., 2022b): (1) the latitude, longitude, and elevation of the lowest mode (i.e., surface return); (2) the latitude, longitude, and elevation of the instrument; (3) the number of detected modes (num_detectedmodes); (4) the width of the Gaussian fit to the received waveform “rx_gwidth” (hereafter referred to as gwidth); (5) the amplitude of the smoothed waveforms lowest detected mode “zcross_amp” (hereafter referred to as amp); (6) the amplitude of each detected mode within the waveform “rx_modeamps”; (7) the mean and standard deviation of the background noise (mean and stddev). Next, the viewing angle (VA in degrees) and the signal-to-noise ratio (SNR in dB) were calculated for each GEDI shot (Fayad et al., 2022b; Nie et al., 2014). To calculate the SNR, the maximum amplitude within an acquired waveform, defined as the maximum of up to 19 possible values of rx_modeamps as well as the mean and standard deviation of the noise are used. The distance between the location of a GEDI shot and the location of the GEDI instrument projected at nadir onto the WGS84 reference ellipsoid and the altitude of GEDI instrument over the referenced ellipsoid at acquisition time of shot are used to calculate the viewing angle.
3.2.2.3 GEDI data filtering
The GEDI dataset comprises 44 acquisition dates, spanning from April 2019 until March 2023. Not all GEDI shots are useable since LiDAR returns can be strongly degraded due to the presence of clouds, water, and aerosols. Therefore, several filters were performed to remove non-viable shots:
• Since a footprint acquired over a water surface should only have a single return, as such, all acquired GEDI shots with a number of detected modes different from one (num_detectedmodes ≠ 1) were removed.
• After the application of the first filter, a second filter was applied that removed the shots having an elevation difference to the SRTM DEM of more than 50 m (Rodríguez et al., 2006).
• A third filter based on the median of absolute deviations (MAD) were used in order to minimize the potential impact of the residual outliers (Fayad et al., 2022a; Leys et al., 2013). The third filter is applied on each river by a 10 km transect. First, the median is calculated for each transect using all GEDI elevations (GEDI(i)) found on the transect (MT). Next, the absolute deviation from the median was calculated for each GEDI measurement along each transect (ADT(i) = abs(GEDIT(i) - MT)), followed by the median of absolute deviations (MADT = median(ADT(i)), and finally the standard deviations (stdT = 1.4826.MADT) were calculated. Only GEDI elevations within the range [MT - 2.stdT, MT + 2.stdT] were retained as in Frappart et al. (2024).
• Finally, a last filter is applied using the gwidth parameter. According to Fayad et al. (2022b), points with gwidth >25 have been removed.
On the sixteen rivers studied, 4,748 points were retained after applying the various filters for comparisons with other datasets.
The available GEDI elevations are relative to the WGS 84 ellipsoid. SWOT and Hydrowebnext elevations are referenced to EGM2008. In order to compare all our datasets, GEDI elevations needed to be referenced through the same vertical datum. Thus, the elevations provided by GEDI were converted to EGM2008 using EGM2008 provided by SWOT data.
3.2.3 IRIS database
ICESat-2 river surface slope (IRIS) database is composed of measures of water surface slope everytime the satellite ICESat-2’s orbit crosses a reach. Water surface slopes are derived from ICESat-2 ATL13 observations, detailed and validated in Scherer et al. (2023). The version used is Version v2.3 and is freely available at https://zenodo.org/records/7098114. The product provides the minimum, maximum and average slope derived with three different approaches (accross, along and combined per reach). Reaches used in this database are the same as those defined by the SWORD version v2 product for SWOT mission (Altenau et al., 2021).
3.2.4 Other datasets
A digital elevation model (DEM) was used in our study. The Forest And Buildings removed Copernicus Digital Elevation Model (FABDEM) is a DEM at one arc second (∼30 m) grid spacing. This global DEM has been developed using machine learning to remove buildings and forests from the Copernicus Digital Elevation Model (Hawker et al., 2022). This DEM is freely available at https://data.bris.ac.uk/data/dataset/s5hqmjcdj8yo2ibzi9b4ew3sn. Compared to former DEM, this one is better suited to applications that require accurate terrain heights, in particular flood simulation (Hawker et al., 2022).
4 Results
4.1 Filters
Daily SWOT data acquired from 6th to 24 April 2023 were used to derive river profiles in the Cuvette Centrale. The Z-score method was applied to remove outliers. Longitudinal profiles derived from SWOT WSE and sigma0 (σ0) before and after filtering are presented for 4 rivers (Figure 2). The selected profiles correspond to the most recurrent longitudinal profiles (x-axis is the distance from the outlet) of water level and backscattering coefficient on all the rivers analyzed (a total of sixteen rivers lie below the SWOT track, see Figure 1C for their locations). The different types of profile obtained are as follows: plateau, straight, with a steep peak and parabola. For each profile, the x-axis is corresponding to the distance from the outlet (km).
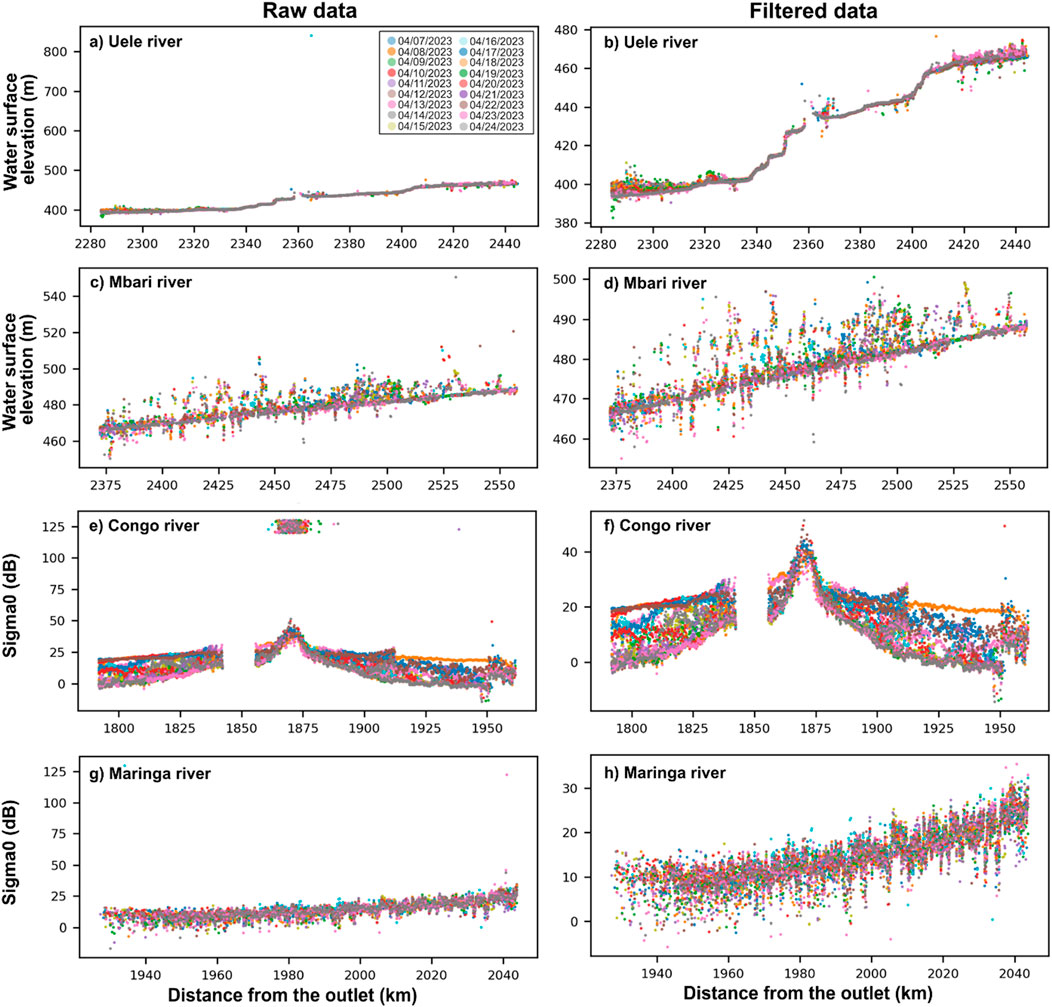
Figure 2. Examples of raw and filtered SWOT data: Uele River (A, B), Mbari River (C, D), Congo River (E, F) and Maringa River (G, H). For each example, data acquired during April 2023 is shown, from 6th to 24th.
In the example of the Uele river (Figures 2A, B), the filtering process produces a longitudinal profile of water heights, with water heights ranging from an average of 390 m at 2,280 km from the outlet to 470 m at 2,440 km. Different levels are visible with gentler slopes (0.110 m/km) around 2,280 to 2,330 km from the outlet, and at the end of the profile around 2,410 to 2,440 km from the outlet with a slope of 0.220 m/km. Rapid changes of altitude corresponding to cascades are observed, as between 2,350 and 2,352 km from the outlet with a slope of 5.5 m/km or from 2,395 to 2,408 km from the outlet with a slope of 1.2 m/km (Figure 2B). All the daily data shown in Figure 2B are not scattered (mean std ±0.88 m) and the mean profile is clearly visible.
In the case of the Mbari river (Figures 2C, D), filtering of the raw water level data gives a linear slope, with average water levels varying from 465 m to 486 m, between 2,375 and 2,555 km from the outlet. This gives an average slope of 0.12 m/km. After filtering the data (Figure 2D), they remain fairly scattered (479 ± 1.86 m).
For the Congo River, the σ0 values before filtering (Figure 2E) and after filtering (Figure 2F) are presented. The profile exhibits a peak of 35 ± 4.2 dB in the middle of section (1,870 km from the outlet), and decreasing values on each side. Despite a visible profile, the data are fairly scattered, with values oscillating between 0 and 22 ± 5.25 dB on each side of the peak.
Finally, for the Maringa river, filtering of the raw σ0 data (Figure 2G) produces a parabolic profile (Figure 2H) with data that are fairly scattered along the profile. σ0 values vary from 10 dB to 22 ± 2.06 dB on average (Figure 2H).
4.2 Intercomparison of water surface elevation (WSE)
The water surface elevations obtained from the SWOT mission were compared with the time series of water levels obtained by other radar altimetry missions (Sentinel-3A and 3B, Sentinel-6A and Jason-3) from Hydrowebnext website (Figure 3A) and GEDI data (Figure 3C). Comparisons between WSE from Hydrowebnext and GEDI are shown in Figure 3B. A total of 40 comparisons were performed between water surface elevations estimated the same day for both datasets (Figure 3A) between SWOT and Hydrowebnext. A R2 determination coefficient of 0.977 was obtained, with a Root Mean Square Error (RMSE) of 0.02 m and a mean bias of 0.538 m (comparison SWOT- Hydrowebnext). For GEDI intercomparison, 56 comparisons were done, with a R2 of 1, a RMSE of 0.003 m and a mean bias of 0.069 m (comparison GEDI - Hydrowebnext). At last, comparisons of the mean WSE from SWOT and GEDI were done in Figure 3C with 3,316 points. A R2 of 0.992 was calculated with a RMSE of 0.002 m and a bias of 1.011 m (comparison SWOT-GEDI). The spatial distribution of the comparison between SWOT and GEDI is shown in Figure 4.
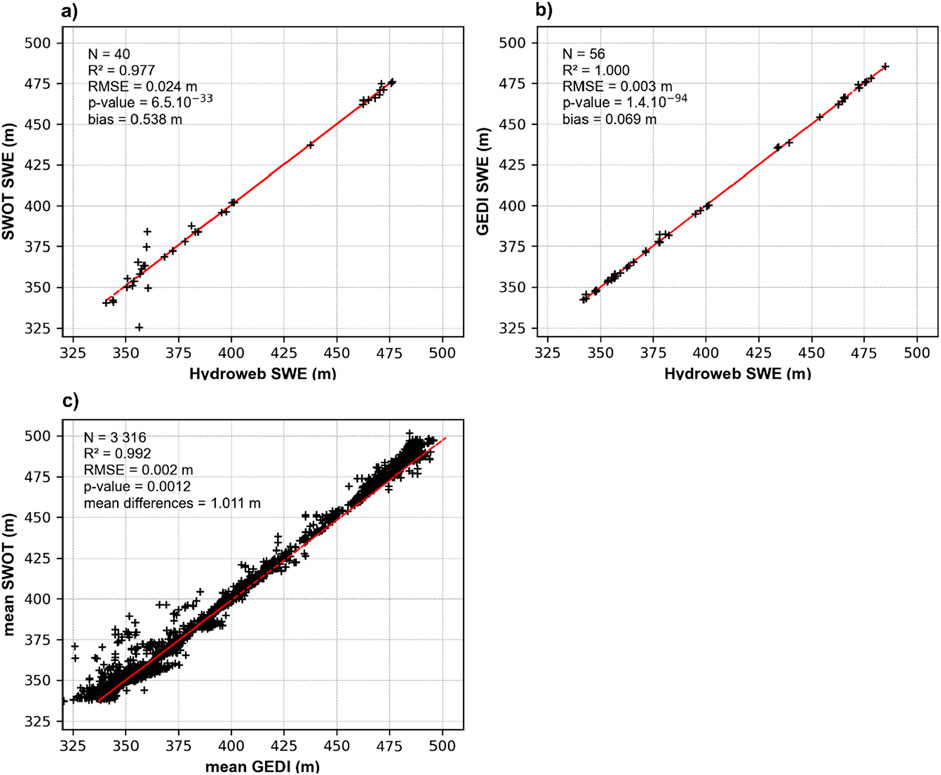
Figure 3. Comparison of water surface elevations (WSE, in m) over all rivers of the study area, (A) SWOT and nadir radar altimetry from Hydrowebnext website, (B) GEDI and nadir radar altimetry from Hydrowebnext website and (C) SWOT and GEDI. The number of samples (N), the determination coefficient (R2), the Root Mean Square Error (RMSE, in m), the p-value and the bias or mean differences (in m) are displayed on the figure.
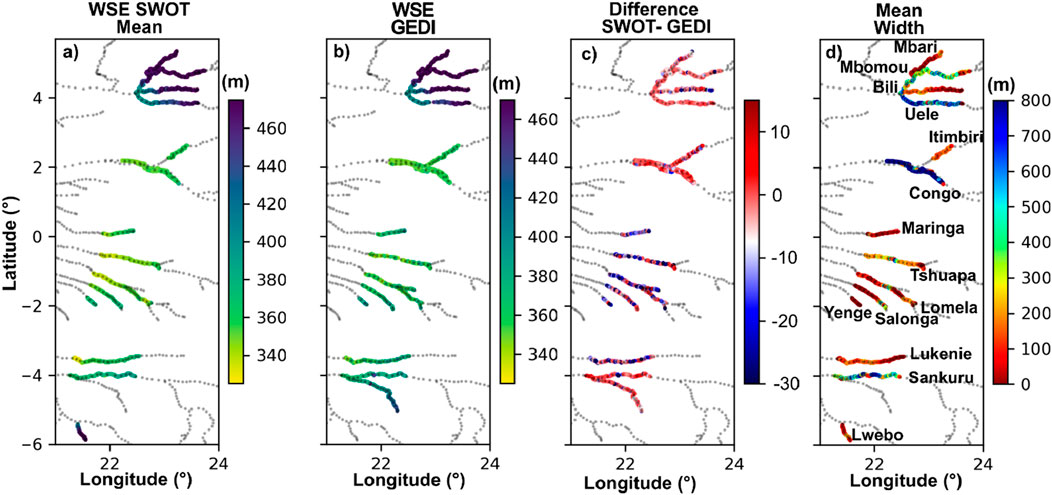
Figure 4. Comparison of water surface elevations (WSE) over the study area for (A) SWOT (in m), (B) GEDI (in m), (C) difference between SWOT and GEDI (in m) and (D) mean river width (in m).
4.3 Different cases of WSE and σ0
After filtering and intercomparing the SWOT WSE data on the sixteen rivers in Congo’s Cuvette Centrale, the WSE (in m), σ0 (in dB) and river width (in m) profiles were analyzed. For reasons of clarity, only four rivers which are representative of the results over the whole Cuvette, were selected: Itimbiri, Tshuapa, Sankuru and Lwebo. Their locations are shown in Figure 1C, Itimbiri being the most northerly and Lwebo the most southerly. These 4 rivers were selected because the profiles obtained were not previously shown in our study (Figure 2). In Figure 3, the digital elevation model FABDEM is shown with the black longitudinal profile line selected to present the filtered profiles of WSE, σ0 and river width.
For the Itimbiri River (Figures 5A–D), Figure 5B shows the WSE over a longitudinal profile of about 90 km, with a variation of 5 m (slope of 0.055 m/km). The WSE data are scattered (3.63 ± 1.95 m), but some dates show a linear profile (04/10/2023 and 04/08/2023). In parallel, σ0 values range between −2 and 10 ± 4.84 dB with a decrease observed upstream of the longitudinal profile (Figure 5C). For this longitudinal profile, the width of the Itimbiri river varies from approximately 80–280 m.
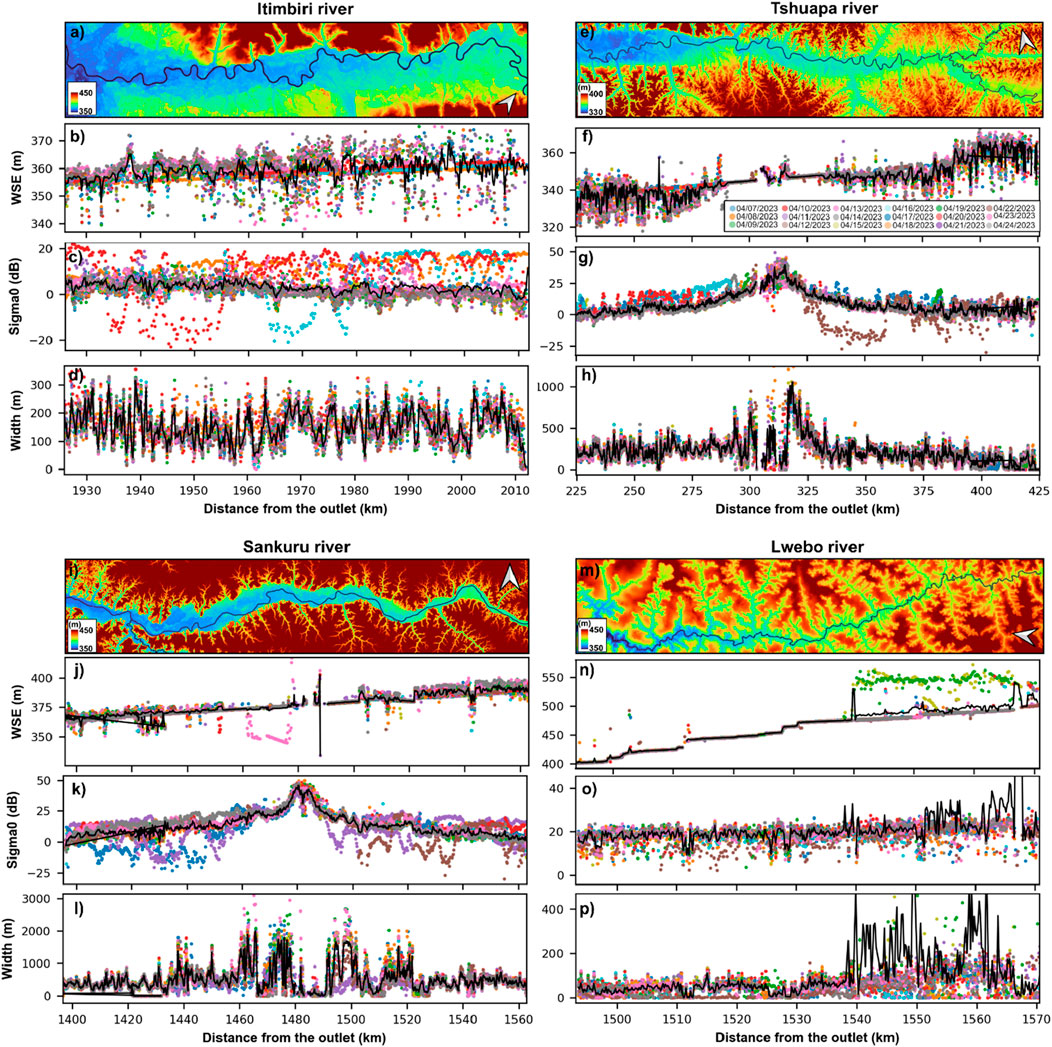
Figure 5. Sigma0 (dB), Water Surface Elevation (WSE) and width (m) profiles after data filtering for four selected rivers: Itimbiri (A–D), Tshuapa (E–H), Saknuru (I–L) and Lwebo (M–P). For each river, four figures are displayed: FABDEM with the selected longitudinal profile in black, WSE (m) along the longitudinal profile presented previously, Sigma0 (dB) along the longitudinal profile presented previously, the width of the river along the profile. Black lines represent the averaged values for each parameter.
The Tshuapa river presents a completely different profile for WSE, σ0 and width (Figures 5E–H). For WSE (Figure 5F), the profile slopes from upstream to downstream, with values ranging from 330 m to 360 ± 2.24 m on average. The data are relatively scattered along the average profile shown in black (Figure 5F). Between 280 and 340 km, the data are not very scattered (345 ± 0.97 m). σ0 values show a peak around 315 km with values of 30 ± 4 dB. On either side of the peak, σ0 values decrease (from 30 to 0 ± 3 dB) with distance and reach around 0 dB. Finally, at the same distance (315 km from the outlet) as the peak observed for σ0, the width of the Itimbiri river appears to be higher, with values around 700 m. The WSE values (345.5 ± 1 m) showed little dispersion.
In the example of the Sankuru river, the WSE profile is not very noisy (376 ± 2.16 m), and a difference of 20 m is measured between 1,400 and 1,560 km from the outlet, i.e., the equivalent of 160 km (Figure 5I). The average slope obtained is 0.125 m/km. The values of σ0 (Figure 5J) show a profile similar to that observed for the Itimbiri river (Figure 5H) with a peak around 1,480 km with values reaching 45 ± 5 dB. This peak in σ0 does not seem to be associated with a peak in the width of the river (Figure 5L). On average, the width of the river is around 473 ± 118 m, with values reaching 2,000 m (Figure 5L).
Finally, in the case of the Lwebo river, the WSE profile varies from 400 to 500 ± 7.47 m from 1,493 to 1,570 km from the outlet, and the WSEs increase per level (Figure 5M). σ0 values are scattered and range from 5 to 20 ± 6.63 dB (Figure 5O). Finally, the width of the Lwebo river varies from 0 to 200 m with an average of 98 ± 133 m (Figure 5P).
These initial results show that the SWOT mission can measure the parameters (WSE, σ0) on different rivers, with very different widths and profiles. Moreover, rivers with widths of less than 100 m, as in the case of the Lwebo river, could also be retrieved.
4.4 Mean of parameters (WSE, σ0)
Figure 6 shows the mean obtained for the parameters WSE (Figure 6A), σ0 (Figure 6B) and river width (Figure 6C). Figures 6D, E show the standard deviations obtained for WSE and σ0, respectively. Means and standard deviations were calculated on the daily data set used in April 2023, with 18 acquisitions. The mean values of WSE vary between 330 and 470 ± 10 m, and the slopes are well depicted for each river. The steepest slopes are obtained for the rivers located in the northern part, the Uele (0.39 m/km), and its tributaries Mbomou (0.42 m/km) and Bili (0.47 m/km) (Figures 6A, C for their locations). Mean values of σ0 values vary between 0 and 50 ± 10 dB on average over April 2023 (Figure 6B), with maximum values observed in the center of the study area. Finally, the standard deviations of the WSE are highest for the widest rivers, which are the Congo (353 ± 5 m), Uele (429 ± 2 m), Mbomou (462 ± 3.5 m) and Sankuru (477 ± 2.5 m). The same applies to σ0, with the highest values in the widest rivers (Figure 6E).
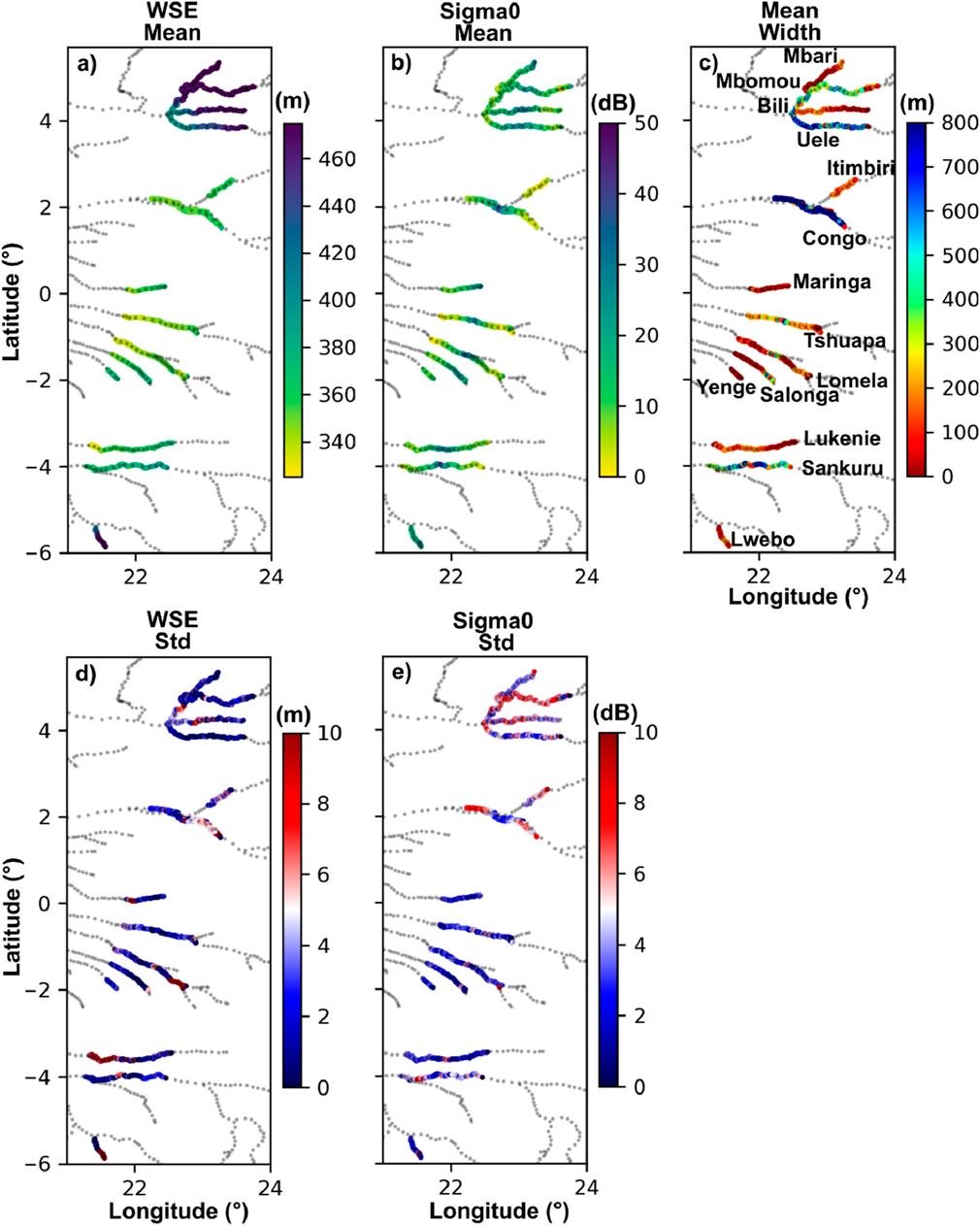
Figure 6. (A) Mean of Surface Water Extent (WSE, in m), (B) mean of Sigma0 (in dB), (C) mean river width (in m), (D) standard deviation of WSE (in m) and (E) standard deviation of Sigma0 (in dB) over April 2023 and over all rivers covered by SWOT data.
5 Discussion
5.1 SWOT data quality (WSE, σ0 and slope)
Firstly, the quality of the WSEs derived from daily SWOT data acquired in April 2023 was compared with the water levels derived from other altimetry missions (Sentinel-3A and 3B, Jason-3 and Sentinel-6). The comparison between these two WSE datasets shows a correlation coefficient R equal to 0.977, RMSE equal to 0.024 m and a bias equal to 0.538 m. This comparison shows very good agreement between the two datasets.
Comparison of the WSE data for SWOT data averaged over the month of April 2023 with GEDI data averaged over 2019–2023 gives very good results, with R2 = 0.99, RMSE = 0.002 m and mean differences = 1.011 m. Here, as the comparison between WSE from SWOT and GEDI is done between data not acquired at same time, we consider mean difference and not biases. The mean differences map between WSE from GEDI and SWOT data is shown in Figure 7. High positive or negative mean differences (<-10 m and >10 m) are found on rivers with the smallest widths, such as the Mbari, Lomela and Tshuappa rivers, or on the upstream part of the widest rivers (case of the Itimbiri and Sankuru rivers, Figure 7). The possible reasons for these high mean differences are: (1) comparison of average GEDI and average SWOT points acquired at a different time in the rivers’ hydrological cycle, (2) geolocation problems with GEDI data and (3) difficulty of the SWOT sensor to measure WSE in the narrowest rivers, (3) the lower quality of the GEDI data compared to other radar and lidar altimeters (Frappart et al., 2021a).
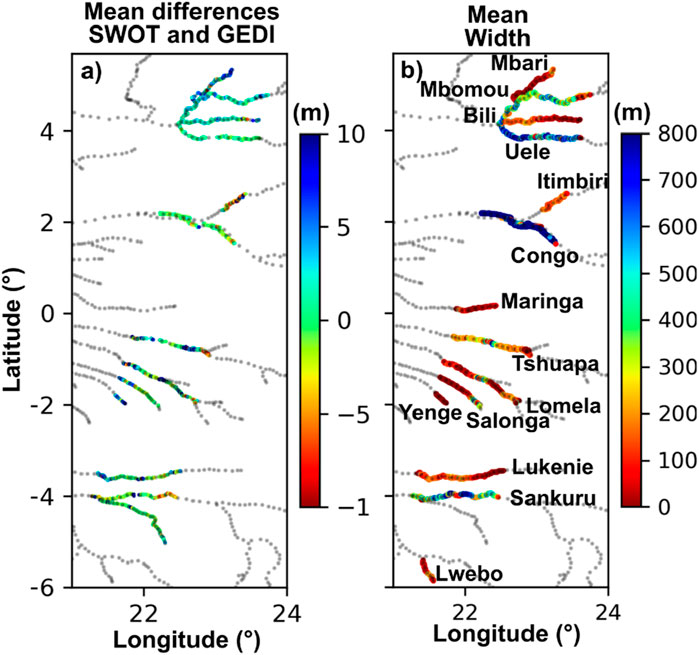
Figure 7. (A) Mean differences between SWOT and GEDI Water Surface Elevations (WSE, in m) and (B) mean river width (in m) with their names.
Concerning point (1), the rivers in our study area have a water level that varies from 2 to 4 m on average (Mbari: 4 m, Lomela: 3 m, Tshuapa: 2 m, Itimbiri: 2 m and Sankuru: 3 m) at the transition from dry to wet periods. So, even if a GEDI point and a SWOT point were acquired at a different time in the hydrological cycle, the highest mean differences (>10 m or < -10 m) cannot be explained by this hydrological cycle, whose water level variations are at most 4 m. Concerning point (2) on the horizontal geolocation problems of GEDI points, Xu et al. (2023) showed that this error was on average between 3.04 and 65.03 m. So, in a river whose width is less than 100 and is bordered by forest along its banks, the closest GEDI point to the selected SWOT point may in fact correspond to a tree. Finally, the third and last plausible reason may be due to the acquisition of SWOT data. Indeed, in the example of the Lwebo river, whose longitudinal WSE profile is shown in Figure 5N, σ0 profile in Figure 5O, width profile in Figure 5P, this river has a plateau WSE profile with steep rises (localized at 1,500, 1,503, 1,515, 1,527, 1,532, and 1,550 km from the outlet, Figure 5N). Upstream of this profile (1,540 km from the outlet), we note a greater dispersion of WSE compared to the mean longitudinal profile (Figure 5N). This observation is accompanied by a greater dispersion in terms of river width (Figure 5P). When the river width becomes too narrow (<100 m), the WSE measurements acquired by the KaRIn sensor on board SWOT show more dispersion. The same applies to the Itimbiri River (Figures 5B, C), where the maximum width is 300 m. WSE are highly dispersed along the all river. Thus, as the river width decreases sharply, the data acquired by the KaRIn sensor appear more scattered. These initial observations by SWOT show that this new mission is capable of measuring WSE in rivers, but there are limitations for rivers with widths of less than 100 m, for which a higher scatter in the observations may be seen.
With regard to the σ0 values of the Itimbiri (Figure 5C), Tshuappa (Figure 5G), Sankuru (Figure 5K) and Lwebo (Figure 5O) rivers, different profiles are observed. A decrease is visible for Itimbiri (values ranging from −2 to 10 ± 4.84 dB on average), a stagnation for Lwebo (values ranging from 5 to 20 ± 6.63 dB), and a peak in the middle of the river equal to 30 ± 4 dB for Tshuappa (located 315 km from the outlet) and equal to 45 ± 5 dB for Sankuru (located 1,480 km from the outlet). The two peaks observed for the Tshuapa and Sankuru rivers represent the acquisition of data at the nadir of the KaRIn sensor on board the SWOT satellite. At this point, the index angle is 0°, so the σ0 values are higher and lower on each side as the measurement moves away from the nadir. These results are consistent with those of the study by Rudant et al. (2019), where σ0 values were calculated as a function of incidence angle. The lower the angle of incidence, the higher the σ0 values, and the curve of σ0 values as a function of angle of incidence has an exponential decrease, whatever the type of surface considered (Rudant et al., 2019). This kind of profile characterized by a peak in σ0 values is only visible on the Tshuapa, Sankuru and Congo rivers since these are long transects (200, 160 and 190 km, respectively), unlike the Itimbiri and Lwebo rivers which are tributaries with shorter lengths (80 km for both rivers). When we look at the average σ0 values over the whole area (Figure 6B), we see this type of profile with a clearly visible peak for the main river branches with high σ0 values, which shows the nadir of the SWOT satellite over this area. Finally, the average values obtained for the profiles (Figure 5) and for the zone as a whole (Figure 6) range from −5–30 dB. Only a few studies have looked at Ka-band σ0 values over different soil types (Frappart et al., 2021b; 2015). The average values of σ0, obtained by the AltiKa altimeter operating in Ka-band on board the Saral satellite, vary between 4 and 20 dB over the flooded forests of Amazonia (located at the same latitudes as our area and whose soil type most closely looks like the Congo Central Cuvette, (Frappart et al., 2021b),). In the study by Frappart et al. (2015), σ0 values measured by the same sensor over the Congo rainforest range from 10 to 21 dB on average. In Fayne et al. (2024), σ0 values at Ka-band over open water have values between −8–16 dB, with a strong decrease when the incidence angle is increasing.
Water surface slopes from SWOT have been compared to IRIS ICESat-2 database over the study area (Figure 8). Three rivers with different profiles have been selected: Sankuru characterized by small slope changes (Figures 8A, B), Uele with two steep changes (Figures 8C, D) and Mbomou with a small slope upstream and a very steep change downstream (Figures 8E, F). For each river, SWOT WSE profile with smoothed data in red is shown on the left panel, and the slopes calculated from SWOT and provided by the IRIS database are presented on the right panel. Whatever the river studied, the spatial sampling of the IRIS database (one point every ∼8 km on average) is much lower than the one of SWOT data (100 m resampled at 1 km to smooth the river slopes) for the various river profiles. As a result, high slopes are not detected by the IRIS database, particularly for the Sankuru (distance from the outlet 1,430, 1,505, 1,525 and 1,540 km) and Mbomou (2,325 km from the outlet) rivers. For each longitudinal slope profile, the IRIS database provides at best around thirty points, compared with 1,000 points with the SWOT data. The SWOT data are able to detect smaller-scale changes in slope compared to IRIS data.
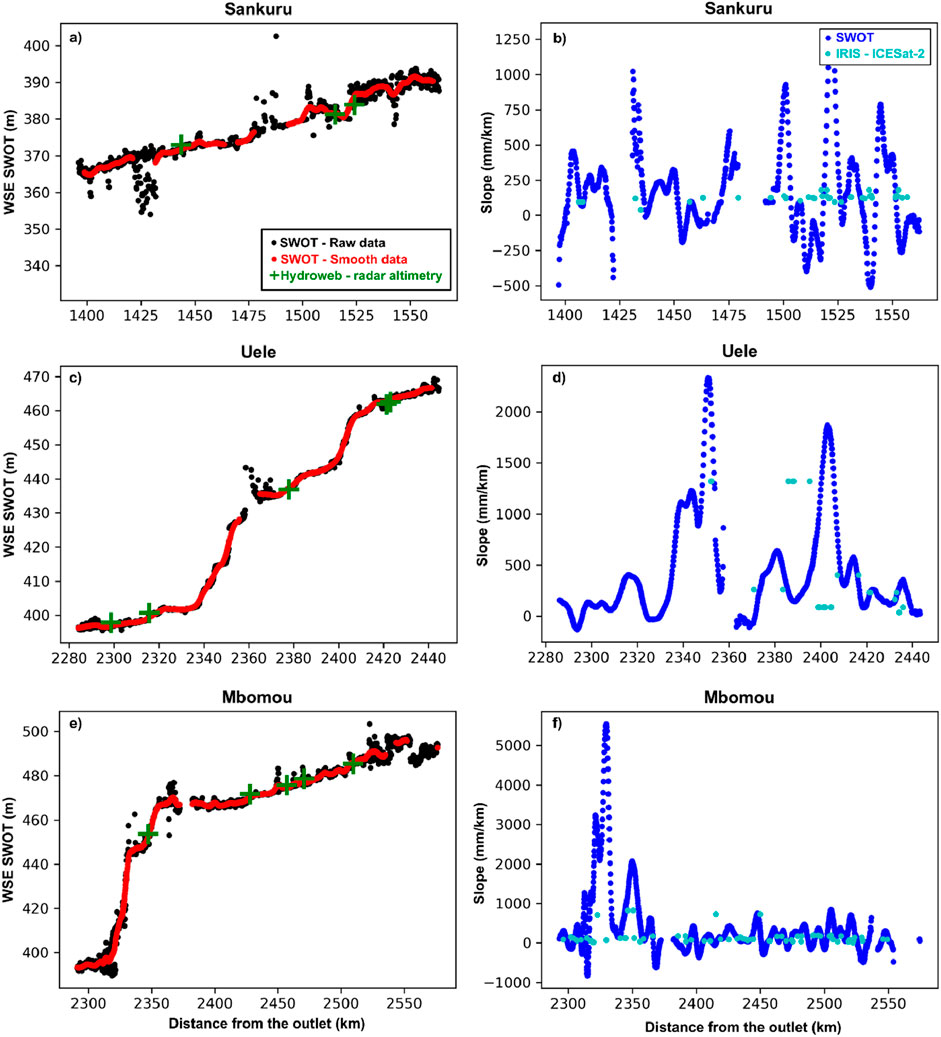
Figure 8. (A) WSE (m) from SWOT (raw data - black dots - and smoothed data - red dots) and Hydroweb radar altimetry data (green crosses) for (A) Sankuru, (C) Uele and (E) Mbomou rivers, slopes (mm/km) from SWOT (blue dots) and IRIS (turquoise) database for (B) Sankuru, (D) Uele and (F) Mbomou rivers.
5.2 River longitudinal profiles
The type of river profile is a key indicator of the morpho dynamic evolution the river has undergone over the course of its history (Boulton et al., 2013; Boulton, 2020). In our study, of the sixteen rivers analyzed in the central Congo basin, several types of longitudinal WSE profiles were observed, such as concave profiles and profiles with slope breaks.
The shape of the profiles and the various breaks give an indication of the progression of tectonically-generated, lithologically-dependent regressive incision. Generally speaking, the longitudinal profile of a river will tend towards a concave shape upstream, with higher elevations, which will allow the transport of solid loads and the incision of the riverbed downstream (Larue, 2014; Seybold et al., 2021). Most of the rivers observed here have this profile, which is the result of a dynamic equilibrium between erosion/deposition processes. In contrast, profiles with rectilinear segments and slope breaks are mostly caused either by a lithological contact or by uplift upstream of an active fault, or by a lowering of the base level that will generate regressive erosion (Bishop et al., 2005).
Longitudinal slope profiles with such precision, since the data provide a WSE every 200 m (when taking the shapefile product of rivers), unlike other altimetry missions, which obtain at best 4 points on the same profile (see green crosses on Figures 8A, C, E). This data will be an extremely important and promising alternative for fields of application such as hydrodynamics, hydrology and geomorphology, particularly in areas with little in situ data or no human access.
6 Conclusion and perspectives
Water surface elevations (WSE) in rivers in Congo’s Central Cuvette have been observed for the first time, with the first daily results from the calibration phase of the SWOT mission. Dedicated to continental hydrology, this mission will make it possible to study all the rivers and lakes on the globe. In our study, we focused on the shapefile product for rivers, enabling us to study, among other things, WSE water heights and σ0 on sixteen rivers with different widths, ranging from 50 m to 2,000 m.
First, SWOT data acquired over 18 runs in April 2023 were filtered to remove outliers. An intercomparison of WSE from SWOT was done, using WSE derived from other nadir altimetry (Sentinel-3A and 3B, Jason-3 and Sentinel-6A) from Hydrowebnext and GEDI data. These initial results are very encouraging, with a R2 correlation coefficient of 0.977 and a bias of 0.538 m calculated between the SWOT data and the Hydrowebnext database, and a correlation of 0.992 and a mean difference of 1.011 m between the SWOT and the GEDI data.
Over all sixteen rivers studied, the WSE profiles appear to be consistent with realistic slopes, higher upstream than downstream. Finally, by analyzing the longitudinal profiles of the WSE, we note that two types of profile stand out: concave and rectilinear with slope breaks. Although other altimetry missions have been able to study river slope profiles in previous studies, these were not as accurate as those obtained by the SWOT mission today. These different profiles are a key indicator of the morpho dynamic evolution the river has undergone over the course of its history. Finally, the average σ0 values obtained for the sixteen rivers are consistent with previous studies.
This work, which presents the first results obtained on the rivers using SWOT data, could be continued in the future to study seasonal variations in slopes over the course of a year with a precision never before obtained using other nadir altimetry. It will therefore be possible to study the hydrological dynamics of different rivers around the world. All this data is easy to access and free of charge. Thus, this type of data could prove very useful, in part, for hydrological and hydrodynamic models, for geomorphologists, and for navigation.
Data availability statement
The original contributions presented in the study are included in the article/supplementary material, further inquiries can be directed to the corresponding author.
Author contributions
CN: Conceptualization, Data curation, Formal Analysis, Funding acquisition, Investigation, Methodology, Project administration, Resources, Software, Supervision, Validation, Visualization, Writing–original draft, Writing–review and editing. FF: Conceptualization, Funding acquisition, Investigation, Project administration, Supervision, Writing–review and editing. NB: Methodology, Validation, Writing–review and editing. LB: Funding acquisition, Methodology, Supervision, Validation, Writing–review and editing. SL: Writing–review and editing. BY: Writing–review and editing. BK: Writing–review and editing. FP: Validation, Writing–review and editing. SR: Writing–review and editing. J-PW: Supervision, Writing–review and editing.
Funding
The author(s) declare that financial support was received for the research, authorship, and/or publication of this article. CN is funded by a CNES post-doctoral grant. This study was also funded by the CNES SWOT grants SWHYM, WHYGHGS and DYBANGO.
Conflict of interest
The authors declare that the research was conducted in the absence of any commercial or financial relationships that could be construed as a potential conflict of interest.
The author(s) declared that they were an editorial board member of Frontiers, at the time of submission. This had no impact on the peer review process and the final decision.
Publisher’s note
All claims expressed in this article are solely those of the authors and do not necessarily represent those of their affiliated organizations, or those of the publisher, the editors and the reviewers. Any product that may be evaluated in this article, or claim that may be made by its manufacturer, is not guaranteed or endorsed by the publisher.
References
Abdalla, S., Abdeh Kolahchi, A., Ablain, M., Adusumilli, S., Aich Bhowmick, S., Alou-Font, E., et al. (2021). Altimetry for the future: building on 25 years of progress. Adv. Space Res. 68, 319–363. doi:10.1016/j.asr.2021.01.022
Alsdorf, D., Beighley, E., Laraque, A., Lee, H., Tshimanga, R., O’Loughlin, F., et al. (2016). Opportunities for hydrologic research in the Congo Basin. Rev. Geophys. 54, 378–409. doi:10.1002/2016RG000517
Altenau, E. H., Pavelsky, T. M., Durand, M. T., Yang, X., Frasson, R.P. de M., and Bendezu, L. (2021). The surface water and Ocean Topography (SWOT) mission River Database (SWORD): a global river network for satellite data products. Water Resour. Res. 57, e2021WR030054. doi:10.1029/2021WR030054
Altenau, E. H., Pavelsky, T. M., Moller, D., Lion, C., Pitcher, L. H., Allen, G. H., et al. (2017). AirSWOT measurements of river water surface elevation and slope: tanana River, AK. Geophys. Res. Lett. 44, 181–189. doi:10.1002/2016GL071577
Becker, M., Papa, F., Frappart, F., Alsdorf, D., Calmant, S., da Silva, J. S., et al. (2018). Satellite-based estimates of surface water dynamics in the Congo River Basin. Interna. J. Appli. Earth Obser. Geoinfor. 66, 196–209. doi:10.1016/j.jag.2017.11.015
Barker, D. M., Lawler, D. M., Knight, D. W., Morris, D. G., Davies, H. N., and Stewart, E. J. (2008). Longitudinal distributions of river flood power: the combined automated flood, elevation and stream power (CAFES) methodology. EARTH Surf. Process. LANDFORMS 34, 280–290. doi:10.1002/esp.1723
Betbeder, J., Gond, V., Frappart, F., Baghdadi, N. N., Briant, G., and Bartholome, E. (2014). Mapping of central Africa forested wetlands using remote sensing. IEEE J. Sel. Top. Appl. Earth Observations Remote Sens. 7, 531–542. doi:10.1109/JSTARS.2013.2269733
Biancamaria, S., Lettenmaier, D. P., and Pavelsky, T. M. (2016). “The SWOT mission and its capabilities for land hydrology,” in Remote sensing and water Resources. Editors A. Cazenave, N. Champollion, J. Benveniste, and J. Chen (Cham: Springer International Publishing), 117–147. doi:10.1007/978-3-319-32449-4_6
Birkett, C. M., Mertes, L. A. K., Dunne, T., Costa, M. H., and Jasinski, M. J. (2002). Surface water dynamics in the Amazon Basin: application of satellite radar altimetry. J. Geophys. Res. 107. doi:10.1029/2001JD000609
Bishop, P., Hoey, T. B., Jansen, J. D., and Artza, I. L. (2005). Knickpoint recession rate and catchment area: the case of uplifted rivers in Eastern Scotland. Earth Surf. Process. Landf. 30, 767–778. doi:10.1002/esp.1191
Boergens, E., Nielsen, K., Andersen, O. B., Dettmering, D., and Seitz, F. (2017). River levels derived with CryoSat-2 SAR data classification—a case study in the Mekong River basin. Remote Sens. 9, 1238. doi:10.3390/rs9121238
Boulton, S., Stokes, M., and Mather, A., 2013. Transient fluvial incision as an indicator of active faulting and surface uplift in the Moroccan High Atlas. EGU2013-5481.
Boulton, S. J. (2020). Geomorphic response to differential uplift: river long profiles and knickpoints from guadalcanal and makira (Solomon Islands). Front. Earth Sci. 8. doi:10.3389/feart.2020.00010
Bourrel, L., and Pouilly, M. (2004). Hidrología y dinámica fluvial del Río Mamoré. Diversidad biológica en la llanura de inundación del río Mamoré. Centro de Ecología Simón I. Patiño, 95–116.
Brasington, J., Rumsby, B. T., and McVey, R. A. (2000). Monitoring and modelling morphological change in a braided gravel-bed river using high resolution GPS-based survey. Earth Surf. Process. Landforms 25, 973–990. doi:10.1002/1096-9837(200008)25:9<973::AID-ESP111>3.0.CO;2-Y
Bwangoy, J.-R. B., Hansen, M. C., Roy, D. P., Grandi, G. D., and Justice, C. O. (2010). Wetland mapping in the Congo Basin using optical and radar remotely sensed data and derived topographical indices. Remote Sens. Environ. 114, 73–86. doi:10.1016/j.rse.2009.08.004
Callède, J., Moreira, D., and Calmant, S. (2013). Détermination de l'altitude du Zéro des stations hydrométriques en Amazonie brésilienne. Application aux lignes d'eau des Rios Negro, Solimões et Amazone. Solimões Amaz. rseau 26, 153–171. doi:10.7202/1016065ar
Charriere, M., Bourrel, L., Gautier, E., and Pouilly, M. (2004). “División geomorfológica del río Mamoré. Diversidad biológica en la llanura de inundación del Río Mamoré,” in Importancia ecológica de la dinámica fluvial, Fundacíon Simón I. Patiño. Editors M. Pouilly, S. G. Beck, M. Moraes, and C. Ibañez (La Paz), 77–94.
Chelton, D. B., Ries, J. C., Haines, B. J., Fu, L.-L., and Callahan, P. S. (2001). “Chapter 1 satellite altimetry,” in International geophysics (Elsevier), 1–ii. doi:10.1016/S0074-6142(01)80146-7
Chen, S.-A., Michaelides, K., Grieve, S. W. D., and Singer, M. B. (2019). Aridity is expressed in river topography globally. Nature 573, 573–577. doi:10.1038/s41586-019-1558-8
Cretaux, J.-F., Calmant, S., Papa, F., Frappart, F., Paris, A., and Berge-Nguyen, M. (2023). Inland surface waters quantity monitored from remote sensing. Surv. Geophys 44, 1519–1552. doi:10.1007/s10712-023-09803-x
Dargie, G. C., Lewis, S. L., Lawson, I. T., Mitchard, E. T. A., Page, S. E., Bocko, Y. E., et al. (2017). Age, extent and carbon storage of the central Congo Basin peatland complex. Nature 542, 86–90. doi:10.1038/nature21048
Datok, P., Fabre, C., Sauvage, S., N’kaya, G. D. M., Paris, A., Santos, V. D., et al. (2022). “Investigating the role of the cuvette Centrale in the hydrology of the Congo river basin,” in Congo basin hydrology, climate, and biogeochemistry (American Geophysical Union AGU), 247–273. doi:10.1002/9781119657002.ch14
Devroey, E.-J. (1959). Annuaire hydrologique du Congo belge et du Ruanda-Urundi. 1958. Annuaire hydrologique du Congo belge et du Ruanda-Urundi. 11.
Dubayah, R., Blair, J. B., Goetz, S., Fatoyinbo, L., Hansen, M., Healey, S., et al. (2020). The global ecosystem dynamics investigation: high-resolution laser ranging of the earth’s forests and topography. Sci. Remote Sens. 1, 100002. doi:10.1016/j.srs.2020.100002
Dubayah, R., Hofton, M., Blair, J., Armston, J., Tang, H., and Luthcke, S. (2021a). GEDI L2A elevation and height metrics data global footprint level V002. doi:10.5067/GEDI/GEDI02_A.002
Dubayah, R., Luthcke, S., Blair, J., Hofton, M., Armston, J., and Tang, H. (2021b). GEDI L1B geolocated waveform data global footprint level V002. doi:10.5067/GEDI/GEDI01_B.002
Fassoni Andrade, A. C., Fleischmann, A. S., Papa, F., Paiva, R. C. D. de, Wongchuig, S., Melack, J. M., et al. (2021). Amazon hydrology from space: scientific advances and future challenges. Rev. Geophys. 59, e2020RG000728. doi:10.1029/2020RG000728
Fayad, I., Baghdadi, N., Bailly, J.-S., Frappart, F., and Pantaleoni Reluy, N. (2022a). Correcting GEDI water level estimates for inland waterbodies using machine learning. Remote Sens. 14, 2361. doi:10.3390/rs14102361
Fayad, I., Baghdadi, N., and Frappart, F. (2022b). Comparative Analysis of GEDI’s elevation accuracy from the first and second data product releases over inland waterbodies. Remote Sens. 14, 340. doi:10.3390/rs14020340
Fayne, J. V., Smith, L. C., Liao, T. H., Pitcher, L. H., Denbina, M., Chen, A. C., et al. (2024). Characterizing near-nadir and low incidence ka-band SAR backscatter from wet surfaces and diverse land covers. IEEE J. Sel. Top. Appl. Earth Observations Remote Sens. 17, 985–1006. doi:10.1109/jstars.2023.3317502
Fjørtoft, R., Gaudin, J.-M., Pourthié, N., Lalaurie, J.-C., Mallet, A., Nouvel, J.-F., et al. (2014). KaRIn on SWOT: characteristics of near-nadir ka-band interferometric SAR imagery. IEEE Trans. Geoscience Remote Sens. 52, 2172–2185. doi:10.1109/TGRS.2013.2258402
Frappart, F., Blarel, F., Fayad, I., Bergé-Nguyen, M., Crétaux, J.-F., Shu, S., et al. (2021a). Evaluation of the performances of radar and lidar altimetry missions for water level retrievals in mountainous environment: the case of the Swiss lakes. Remote Sens. 13, 2196. doi:10.3390/rs13112196
Frappart, F., Blarel, F., Papa, F., Prigent, C., Mougin, E., Paillou, P., et al. (2021b). Backscattering signatures at Ka, Ku, C and S bands from low resolution radar altimetry over land. Adv. Space Res. 25 Years Prog. Radar Altimetry 68, 989–1012. doi:10.1016/j.asr.2020.06.043
Frappart, F., Blumstein, D., Cazenave, A., Ramillien, G., Birol, F., Morrow, R., et al. (2017). “Satellite altimetry: principles and applications in earth sciences,” in Wiley encyclopedia of electrical and electronics engineering (Hoboken, NJ, USA: John Wiley & Sons, Inc.), 1–25. doi:10.1002/047134608X.W1125.pub2
Frappart, F., Fatras, C., Mougin, E., Marieu, V., Diepkilé, A. T., Blarel, F., et al. (2015). Radar altimetry backscattering signatures at Ka, Ku, C, and S bands over west Africa. Phys. Chem. Earth, Parts A/B/C, Emerg. Sci. Appl. Microw. remote Sens. data 83–84, 96–110. doi:10.1016/j.pce.2015.05.001
Frappart, F., Seyler, F., Martinez, J.-M., León, J. G., and Cazenave, A. (2005). Floodplain water storage in the Negro River basin estimated from microwave remote sensing of inundation area and water levels. Remote Sens. Environ. 99, 387–399. doi:10.1016/j.rse.2005.08.016
Frappart, F., Tong Minh, D. H., Baghdadi, N., Crétaux, J.-F., Fayad, I., and Bergé-Nguyen, M. (2024). Improving mean water lake surface elevation estimates using dense lidar measurements from the GEDI satellite mission. Remote Sens. Appl. Soc. Environ. 35, 101213. doi:10.1016/j.rsase.2024.101213
Frappart, F., Zeiger, P., Betbeder, J., Gond, V., Bellot, R., Baghdadi, N., et al. (2021c). Automatic detection of inland water bodies along altimetry tracks for estimating surface water storage variations in the Congo Basin. Remote Sens. 13, 3804. doi:10.3390/rs13193804
Frappart, F., Zeiger, P., Betbeder, J., Gond, V., Bellot, R., Baghdadi, N., et al. (2021). Automatic detection of inland water bodies along altimetry tracks for estimating surface water storage variations in the Congo Basin. Remote Sen. 13 (19), 3804. doi:10.3390/rs13193804
Fu, L.-L., Pavelsky, T., Cretaux, J.-F., Morrow, R., Farrar, J. T., Vaze, P., et al. (2024). The surface water and Ocean Topography mission: a breakthrough in radar remote sensing of the ocean and land surface water. Geophys. Res. Lett. 51, e2023GL107652. doi:10.1029/2023GL107652
Group, T. A. H., Vörösmarty, C., Askew, A., Grabs, W., Barry, R. G., Birkett, C., et al. (2001). Global water data: a newly endangered species. Eos, Trans. Am. Geophys. Union 82, 54–58. doi:10.1029/01EO00031
Hawker, L., Uhe, P., Paulo, L., Sosa, J., Savage, J., Sampson, C., et al. (2022). A 30 m global map of elevation with forests and buildings removed. Environ. Res. Lett. 17, 024016. doi:10.1088/1748-9326/ac4d4f
Jiang, L., Schneider, R., Andersen, O. B., and Bauer-Gottwein, P. (2017). CryoSat-2 altimetry applications over rivers and lakes. Water 9, 211. doi:10.3390/w9030211
Kadima, E., Delvaux, D., Sebagenzi, S. N., Tack, L., and Kabeya, S. M. (2011). Structure and geological history of the Congo Basin: an integrated interpretation of gravity, magnetic and reflection seismic data. Basin Res. 23 (5), 499–527. doi:10.1111/j.1365-2117.2011.00500.x
Kitambo, B., Papa, F., Paris, A., Tshimanga, R. M., Calmant, S., Fleischmann, A. S., et al. (2022). A combined use of in situ and satellite-derived observations to characterize surface hydrology and its variability in the Congo River basin. Hydrology Earth Syst. Sci. 26, 1857–1882. doi:10.5194/hess-26-1857-2022
Kitambo, B. M., Papa, F., Paris, A., Tshimanga, R. M., Frappart, F., Calmant, S., et al. (2023). A long-term monthly surface water storage dataset for the Congo basin from 1992 to 2015. Earth Syst. Sci. Data 15, 2957–2982. doi:10.5194/essd-15-2957-2023
Koblinsky, C. J., Clarke, R. T., Brenner, A. C., and Frey, H. (1993). Measurement of river level variations with satellite altimetry. Water Resour. Res. 29, 1839–1848. doi:10.1029/93WR00542
Kreibich, H., Van Loon, A. F., Schröter, K., Ward, P. J., Mazzoleni, M., Sairam, N., et al. (2022). The challenge of unprecedented floods and droughts in risk management. Nature 608, 80–86. doi:10.1038/s41586-022-04917-5
Larue, J.-P. (2014). “Profils longitudinaux et ruptures de pente: enseignements géomorphologiques en Bretagne du sud,” in Physio-Géo. Géographie physique et environnement, 49–65. doi:10.4000/physio-geo.3798
LeFavour, G., and Alsdorf, D. (2005). Water slope and discharge in the Amazon River estimated using the shuttle radar topography mission digital elevation model. Geophys. Res. Lett. 32. doi:10.1029/2005GL023836
Leon, J. G., Calmant, S., Seyler, F., Bonnet, M.-P., Cauhopé, M., Frappart, F., et al. (2006). Rating curves and estimation of average water depth at the upper Negro River based on satellite altimeter data and modeled discharges. J. Hydrology, ICWRER - Symposium Dresden, Ger. 328, 481–496. doi:10.1016/j.jhydrol.2005.12.006
Leys, C., Ley, C., Klein, O., Bernard, P., and Licata, L. (2013). Detecting outliers: do not use standard deviation around the mean, use absolute deviation around the median. J. Exp. Soc. Psychol. 49, 764–766. doi:10.1016/j.jesp.2013.03.013
Medeiros Moreira, D. (2016). Apport des données de géodésie spatiale pour l'étude du bassin hydrologique amazonien (Doctoral dissertation, Toulouse 3).
Nie, S., Wang, C., Li, G., Pan, F., Xi, X., and Luo, S. (2014). Signal-to-noise ratio–based quality assessment method for ICESat/GLAS waveform data. OE 53, 103104. doi:10.1117/1.OE.53.10.103104
Normandin, C., Frappart, F., Diepkilé, A. T., Marieu, V., Mougin, E., Blarel, F., et al. (2018). Evolution of the performances of radar altimetry missions from ERS-2 to sentinel-3A over the inner Niger delta. Remote Sens. 10, 833. doi:10.3390/rs10060833
Papa, F., Crétaux, J.-F., Grippa, M., Robert, E., Trigg, M., Tshimanga, R. M., et al. (2023). Water resources in Africa under global change: monitoring surface waters from space. Surv. Geophys 44, 43–93. doi:10.1007/s10712-022-09700-9
Papa, F., and Frappart, F. (2021). Surface water storage in rivers and wetlands derived from satellite observations: a review of current advances and future opportunities for hydrological sciences. Remote Sens. 13, 4162. doi:10.3390/rs13204162
Rodríguez, E., Morris, C. S., and Belz, J. E. (2006). A global assessment of the SRTM performance. Photogramm. Eng. remote Sens. 72, 249–260. doi:10.14358/PERS.72.3.249
Rudant, J.-P., Frison, P.-L., and Paris-Est, U. (2019). Teledetection Radar: De L’image D’intensite Initiale Au Choix Du Mode De Calibration Des Coefficients De Diffusion.
Scherer, D., Schwatke, C., Dettmering, D., and Seitz, F. (2023). ICESat-2 river surface slope (IRIS): a global reach-scale water surface slope dataset. Sci. Data 10 (1), 359. doi:10.1038/s41597-023-02215-x
Seo, S. (2006). A review and comparison of methods for detecting outliers in univariate data sets. Available at: http://d-scholarship.pitt.edu/7948/(Accessed March 6, 24).
Seybold, H., Berghuijs, W. R., Prancevic, J. P., and Kirchner, J. W. (2021). Global dominance of tectonics over climate in shaping river longitudinal profiles. Nat. Geosci. 14, 503–507. doi:10.1038/s41561-021-00720-5
Sinha, S. K., and Parker, G. (1996). Causes of concavity in longitudinal profiles of rivers. Water Resour. Res. 32, 1417–1428. doi:10.1029/95WR03819
Tshimanga R. M., N'kaya G. D. M., and Alsdorf D. (2022). Congo basin hydrology, climate, and biogeochemistry: a Foundation for the future (John Wiley & Sons), 269.
Whipple, K. X., and Tucker, G. E. (1999). Dynamics of the stream-power river incision model: implications for height limits of mountain ranges, landscape response timescales, and research needs. J. Geophys. Res. Solid Earth 104, 17661–17674. doi:10.1029/1999JB900120
Wobus, C., Whipple, K. X., Kirby, E., Snyder, N., Johnson, J., Spyropolou, K., et al. (2006). “Tectonics from topography: procedures, promise, and pitfalls,” in Tectonics, climate, and landscape evolution. Editors S. D. Willett, N. Hovius, M. T. Brandon, and D. M. Fisher (Geological Society of America), 0. doi:10.1130/2006.2398(04
Wu, Q., Ke, L., Wang, J., Pavelsky, T. M., Allen, G. H., Sheng, Y., et al. (2023). Satellites reveal hotspots of global river extent change. Nat. Commun. 14, 1587. doi:10.1038/s41467-023-37061-3
Xu, Y., Ding, S., Chen, P., Tang, H., Ren, H., and Huang, H. (2023). Horizontal geolocation error evaluation and correction on full-waveform LiDAR footprints via waveform matching. Remote Sens. 15, 776. doi:10.3390/rs15030776
Yamazaki, D., Ikeshima, D., Sosa, J., Bates, P. D., Allen, G. H., and Pavelsky, T. M. (2019). MERIT hydro: a high-resolution global hydrography map based on latest topography dataset. Water Resour. Res. 55, 5053–5073. doi:10.1029/2019WR024873
Keywords: SWOT mission, rivers, water surface elevation, backscattering coefficient, Cuvette Centrale, Congo basin
Citation: Normandin C, Frappart F, Baghdadi N, Bourrel L, Peña Luque S, Ygorra B, Kitambo B, Papa F, Riazanoff S and Wigneron J-P (2024) First results of the surface water ocean topography (SWOT) observations to rivers elevation profiles in the Cuvette Centrale of the Congo Basin. Front. Remote Sens. 5:1466695. doi: 10.3389/frsen.2024.1466695
Received: 18 July 2024; Accepted: 27 September 2024;
Published: 10 October 2024.
Edited by:
Jiangyuan Zeng, Chinese Academy of Sciences (CAS), ChinaCopyright © 2024 Normandin, Frappart, Baghdadi, Bourrel, Peña Luque, Ygorra, Kitambo, Papa, Riazanoff and Wigneron. This is an open-access article distributed under the terms of the Creative Commons Attribution License (CC BY). The use, distribution or reproduction in other forums is permitted, provided the original author(s) and the copyright owner(s) are credited and that the original publication in this journal is cited, in accordance with accepted academic practice. No use, distribution or reproduction is permitted which does not comply with these terms.
*Correspondence: Cassandra Normandin, Y2Fzc2FuZHJhLm5vcm1hbmRpbkBpbnJhZS5mcg==, Y2Fzc2FuZHJhLm5vcm1hbmRpbkBnbWFpbC5jb20=