- 1Department of Biology, New Mexico State University, Las Cruces, NM, United States
- 2Department of Molecular, Cell, and Cancer Biology, University of Massachusetts Chan Medical School, Worcester, MA, United States
- 3Department of Microbiology and Immunology, Medical College of Wisconsin, Milwaukee, WI, United States
- 4Department of Quantitative Health Sciences, John A. Burns School of Medicine, University of Hawaii, Honolulu, HI, United States
Introduction: Anopheles gambiae is a primary malaria vector mosquito in Africa. RNA-seq based transcriptome analysis has been widely used to study gene expression underlying mosquito life traits such as development, reproduction, immunity, metabolism, and behavior. While it is widely appreciated that long non-coding RNAs (lncRNAs) are expressed ubiquitously in transcriptomes across metazoans, lncRNAs remain relatively underexplored in An. gambiae, including their identity, expression profiles, and biological functions. The lncRNA genes were poorly annotated in the current reference of the PEST genome of An. gambiae. In this study, a set of publicly available RNA-seq datasets was leveraged to identify lncRNAs across diverse contexts, including whole mosquitoes, mosquito cells and tissues (such as hemocytes, midguts, and salivary glands), as well as under various physiological conditions (e.g., sugar-feeding, blood-feeding, bacterial challenges, and Plasmodium infections).
Methods: A Transcript Discovery module implemented in the CLC genomics workbench was used to identify lncRNAs from selected published RNA-seq datasets.
Results: Across this pool of transcriptomes, 2684 unique lncRNA genes, comprising 4082 transcripts, were identified. Following their identification, these lncRNA genes were integrated into the mosquito transcriptome annotation, which served as a reference for analyzing both mRNAs and lncRNAs for transcriptional dynamics under various conditions. Unsurprisingly, and similar to what has been reported for mRNAs, lncRNAs exhibited context-dependent expression patterns. Co-expression networks constructed using weighted gene co-expression network analysis (WGCNA) highlighted the interconnections among lncRNAs and mRNAs, which provide potential functional networks in which these lncRNAs are involved. Furthermore, we identified polysome-associated lncRNAs within polysome-captured transcripts, suggesting that lncRNAs are likely involved in translation regulation and contribute to coding capacity for micropeptides. The analysis of a ChIP-seq dataset revealed a correlation between transcriptional activities of lncRNAs and observed epigenetic signatures.
Discussion: Overall, our study demonstrated that lncRNAs are transcribed alongside mRNAs in various biological contexts. The genome-wide annotation of lncRNA genes and integration into the PEST reference genome enable the simultaneous co-analysis of mRNA and lncRNA, which will enhance our understanding of their functions and shed light on their regulatory roles in An. gambiae biology.
1 Introduction
Upon a biological or environmental cue, the transcriptomic response directs the production of a proteome relevant to the biological condition. Transcriptomes represent the sum of transcripts of protein-coding genes and non-coding regulatory elements controlling gene expression. To date, transcriptomic studies have been focused primarily on protein-coding transcripts. However, in recent decades, the analysis of transcriptomic data has revealed a substantial number of polyadenylated transcripts devoid of protein-coding potential across various organisms (Claverie, 2005; Mattick, 2005; Carninci and Hayashizaki, 2007). The evolving landscape of long non-coding RNAs (lncRNAs) represents a complex domain, only beginning to be understood in terms of their functional capacity in diverse biological processes such as transcriptional regulation, chromatin remodeling, post-transcriptional regulation, and modulation of cellular signaling pathways (Borkiewicz et al., 2021; Mattick et al., 2023). LncRNAs have been identified in insects, including mosquitoes (Legeai and Derrien, 2015; Ahmad et al., 2021; Choudhary et al., 2021; Zafar et al., 2023). In the field of vector biology, functional genomic studies have been instrumental in elucidating the genetic basis for life traits relevant to vector competence. Recent attention has been devoted to the non-coding genome in vector mosquitoes, as highlighted in a recent review (Farley et al., 2021). For example, genome-wide identification of lncRNAs has been conducted in both Aedes aegypti (Etebari et al., 2016; Azlan et al., 2019) and Aedes albopictus (Azlan et al., 2021; Liu et al., 2022) under various biological contexts. There are 4155, 7609, and 1120 lncRNAs annotated in the reference genome of Ae. aegypti Liverpool, Ae. albopictus Foshan strain, and Anopheles stephensi Indian strain (UCISS2018), respectively (Vectorbase release 68). In Anopheles gambiae, lncRNAs have been documented in the transcriptomes from several developmental stages (Jenkins et al., 2015) and in the midgut following infection with Plasmodium berghei or Plasmodium falciparum (Padrón et al., 2014). However, lncRNA genes are poorly annotated in the reference PEST genome. In this study, we employed the transcript discovery module in the CLC Genomics Workbench to identify lncRNA transcripts across multiple transcriptomic scenarios from 12 An. gambiae and one An. coluzzii studies. These RNA-seq datasets represent diverse contexts, including distinct tissue types (e.g., whole body, midgut, salivary gland, and hemocytes), different diet types (sugar meal and blood meal), and immune challenges (bacterial and malaria infections). The identified lncRNAs were then integrated into the PEST genome annotation by indexing genomic coordinates for lncRNA genes. Selected transcriptomes were then mapped against the annotation to analyze the transcriptional abundance and dynamics for both mRNA and lncRNA transcripts simultaneously. Overall, capitalizing on published transcriptomes with diversity and complexity provides an unbiased and robust approach to generating a comprehensive catalog of potential lncRNAs in these conditions. This work demonstrates a rewarding example of leveraging datasets from existing studies to unveil biological novelty.
2 Materials and methods
2.1 Datasets used in this study
In the past decade, transcriptome interrogations have been widely used in mosquito research. Reutilization of published datasets in life sciences has become a powerful approach for making novel discoveries not addressed in the original publications (e.g., Sielemann et al., 2020). In this study, we selected 12 publicly available RNA-seq datasets from the NCBI domain, as outlined in Supplementary Table S1. The conditions of these RNA-seq studies include bacterial priming and challenge in the whole body or hemocytes; P. berghei and P. falciparum-infected midgut and salivary glands, cell lines upon 20 hydroxyecdysone treatment, and sugar- or blood-fed mosquitoes as well. The RNA-seq libraries were derived from polyA-enriched RNA and sequenced with Illumina RNA-seq protocol. These studies were well designed to characterize mRNA responses to the biological variable examined, but lncRNA was not included in the original design and analysis. In addition to the diverse research contexts, these datasets were selected due to the high data quality; the transcription patterns were properly validated using qRT-PCR in the original studies. Besides being used for lncRNA identification, selected datasets were used to demonstrate the transcriptional response of lncRNA and mRNA transcripts in the relevant contexts. We acknowledge the broad range of datasets in terms of sequencing libraries and sequencing platforms over a decade, which may have a technical bias for different datasets. Selected datasets were carefully chosen to demonstrate the lncRNAs in transcriptomes in the five exhibited cases (see Results).
2.2 LncRNA identification and annotation
We developed a pipeline for the detection of lncRNA transcripts from published transcriptomes by employing the module Large Gap Mapping (LGM) and Transcript Discovery in CLC Genomics Workbench (v.23.0.4). The first step of the pipeline performs read mapping against the Anopheles gambiae PEST reference genome (v.63) with mRNA annotation. The LGM parameters allow reads to span introns, enabling the recognition of transcripts that span splice junctions. The strandedness of a transcript is determined from the splice signatures of the mapping event. The expression level of lncRNA can be low; therefore, to increase sensitivity in detecting low-abundance lncRNAs, the RNA-seq reads were pooled from a given study for the mapping step. The resulting mappings from all datasets were merged into one by the track merging tool. The merged mapping results were used as input for the Transcript Discovery module to identify transcripts. Among the identified transcripts, annotated mRNA transcripts were separated based on the annotation of PEST reference, and the remaining transcripts were examined for protein-coding potentials using the Coding Potential Calculator (https://cpc.gao-lab.org/programs/run_cpc.jsp). A transcript is classified as a lncRNA if it exceeds 200 nucleotides in length and has no coding potential. The coordinates of predicted lncRNA transcripts were annotated in the genome. The mRNA and lncRNA annotations were used as reference in RNA-seq mapping to measure the transcriptional abundance of lncRNAs and mRNAs in RNA-seq samples. The salivary gland RNA-seq dataset from Pinheiro-Sliva (BioProject PRJEB8900) was derived from An. coluzzii. An. gambiae and An. coluzzii are very closely related species (Fontaine et al., 2015). The RNA-seq reads of this dataset were mapped against the An. gambiae PEST reference genome, so the mapped reads would be highly conserved between An. gambiae and An. coluzzii. The An. coluzzii-specific reads would be lost during mapping as a caveat.
2.3 Validation of lncRNA using RT-PCR
Total RNA was isolated from naïve adult female mosquitoes using Trizol following the manual. The RNA samples were treated with DNase I-XT (NEB, Catalog #M0570) to remove residual genomic DNA. To make target-specific cDNA, the reverse primer was used for priming the cDNA synthesis in each target amplicon. PCR was conducted using the SuperScript III One-Step RT-PCR system (Invitrogen, Catalog # 12574-018). The primer sequences are given in Supplementary Table S2.
2.4 Quantification of transcriptional abundance of mRNA and lncRNA
Subsequently, the updated transcriptome annotation was used to quantify the transcriptional abundance of both mRNA and lncRNA transcripts using the RNA-seq analysis module in the CLC genomics workbench. The transcriptional abundance is represented by transcripts per million (TPM), and a false discovery rate (FDR)-adjusted p-value of <0.05 is used to determine differentially expressed transcripts in comparison. Principal Component Analysis (PCA) is a common unsupervised analysis method that reduces the overall dimensionality of multivariate datasets to a few dominant components (Wold et al., 1987). PCA was performed to visualize the expression patterns and relationships between different samples. The volcano plots were created to visualize differentially expressed transcripts. Both PCA and volcano plots were implemented by the RNA-seq analysis module within CLC genomics workbench.
2.5 LncRNA–mRNA network analysis using weighted gene co-expression networks analysis (WGCNA)
Weighted Gene Co-Expression Network Analysis (WGCNA) is a powerful tool to correlate genes (modules) in a complex transcriptional network (Langfelder and Horvath, 2008). WGCNA has been widely used in analyzing transcriptional patterns of mRNAs and lncRNAs to cluster lncRNAs with mRNAs in functional modules. These functional modules may reveal lncRNAs with potential biological significance in the contexts, which may provide clues for screening candidate lncRNAs to understand their roles in the contexts (Luo et al., 2019; Fan et al., 2022). To gain insights into the functional attributes of lncRNAs from the co-expressed mRNA transcripts under a given experimental condition, we used WGCNA to analyze the co-expression pattern of mRNA and lncRNA transcripts. The dataset from Kulkarni et al. (2021) was used to demonstrate this approach. The samples in the conditions of naive, injury, Enterobacter challenge, and Serratia challenge were used. Across these samples, transcripts with a TPM of less than 10 were excluded from the analysis. Initially, pairwise correlations were used to identify transcripts that show similar expression patterns. Then, we determined the appropriate soft-thresholding power and calculated a signed adjacent matrix. This matrix was then transformed into a topological overlap matrix. Subsequently, we identified modules with a minimum size of 50 transcripts. The function capacity of the modules was estimated by the transcripts within the module that have gene ontology (GO) annotations.
2.6 ChIP-seq data analysis
Gómez-Díaz et al. (2014) recognized the epigenetic signatures in the midgut from the ChIP-seq data on the transcriptional active marker (H3K27ac) and the transcriptional inactive marker H3K27me3. They also identified a correlation between these epigenetic signatures and the respective transcriptional patterns of mRNAs in the midgut. We used their annotated H3K27ac and H3K27me3 signatures and the midgut transcriptome data to examine if lncRNA expression is associated with the epigenetic signatures. The tracks of ChIP-seq H3K27ac reads, H3K27me3 reads, input control reads (without immunoprecipitation), and the midgut RNA-seq reads were aligned to visualize the transcript abundance near the H3K27ac and H3K27me3 peaks.
3 Results and discussion
3.1 Identification of putative lncRNAs using the transcript discovery approach
Similar to mRNA transcription, the transcription of lncRNA transcripts is context-dependent. To identify lncRNAs expressed under various transcriptomic scenarios, we utilized RNA-seq datasets from An. gambiae and An. coluzzii available in the public domain. These datasets were derived from different mosquito body/tissue types (whole body, gut, salivary gland, and hemocytes), following different meal types (sugar-fed and blood-fed), and following immune challenges posed by bacteria and malarial parasites (P. falciparum and P. berghei). The RNA-seq datasets used in this study can be found in Supplementary Table S1. To estimate the proportion of lncRNA reads in a transcriptome, we randomly selected 10 RNA-seq samples from different studies and mapped the reads against the annotated PEST genome reference. Notably, in the tested samples, 15–30% of the reads were mapped to intron and intergenic regions, indicating the presence of non-coding transcripts. Given this large fraction of mapping in non-coding regions, we employed the Transcript Discovery module in the CLC Genomics Workbench to identify lncRNA transcripts. This module facilitates the mapping of RNA-seq reads to a genomic reference with parameters allowing intron-spanning mapping. The resulting mappings were processed to infer novel transcripts. The predicted novel transcripts were examined for potential open reading frames. A transcript is defined as a lncRNA if it meets the following 3 criteria: the transcript is represented by a minimum of 10 reads across RNA-seq data sets examined, exceeds a length of 200 nucleotides, and lacks open reading frames (ORFs). Figure 1 illustrates the pipeline for lncRNA identification. The pipeline predicted 2684 unique lncRNA genes with 4082 transcripts. The predicted lncRNA transcripts are 200 – 8,672 nt in size; the number of transcripts per lncRNA gene ranges from 1-8 (97.2% of lncRNA genes have 1-3 transcripts), and the number of exons per lncRNA transcript is 1–10 (97.3% of transcripts have 1-3 exons). Overall, 59.4% of lncRNA transcripts are in the intergenic regions, 18.9% are located on the antisense strand within the gene boundaries, and 21.7% are located on the sense strands within the gene boundaries. The distribution of mRNA and lncRNA, along with their coordinates, is presented in Supplementary Table S3; Supplementary Figure S1. Figure 1 illustrates the lncRNA prediction pipeline, the locations of four representative lncRNAs, and seven RT-PCR validations from a cDNA sample of naive female adults at 3–5 days old. There is an EST database (expressed sequence tags, ESTdb, containing 153,332 sequences), in which the sequences represent the fragments of transcripts derived through single sequencing reactions conducted on randomly selected clones from various cDNA libraries from An. gambiae. Given their abundance, we predicted that lncRNA-derived fragments would be present in the ESTdb. Therefore, we ran reciprocal BLASTn by searching for the 4082 predicted lncRNA sequences against the EST sequences and by searching the 153,332 EST sequences against the 4082 lncRNA sequences. Using a threshold of bit score greater than 500, the BLAST revealed that 1529 EST sequences had lncRNA hits, and 611 lncRNAs had EST hits. A given EST sequence may have more than one lncRNA hit, and one lncRNA sequence may have more than one EST hit (Supplementary Table S4). LncRNA hits in the ESTdb provide additional evidence for lncRNA expression. We understand and acknowledge the caveats of the impracticality of validating lncRNAs within the contexts of the originally published studies, as this is outside the scope of the current work. An updated genome annotation was then created incorporating 4082 predicted lncRNA genes. This updated annotated reference was used to analyze mRNA and lncRNA transcripts in selected transcriptomes. See below.
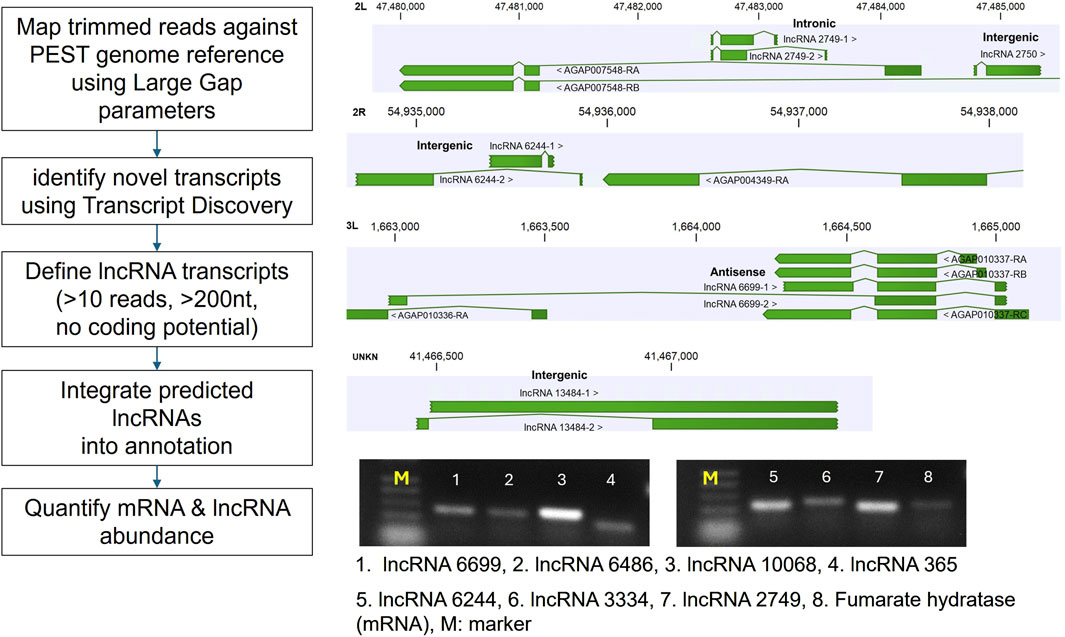
Figure 1. The pipeline of identifying lncRNAs from transcriptomic reads A. Pipeline of lncRNA discovery. Trimmed reads were mapped against the An. gambiae PEST reference genome with large gap parameters to identify transcripts without protein coding potentials. Identified lncRNAs were annotated and added to the PEST reference genome. The mRNA and lncRNA transcripts in the RNA-seq datasets were quantified against the PEST reference genome update to include lncRNA genes B. Genomic locations of 4 exemplary lncRNAs C. Validation of lncRNA using RT-PCR. The RNA sample from a pool of 10 naive adult females was used for target-specific RT-PCR. The gel images show the RT-PCR results for 7 lncRNAs and an mRNA control (AGAP001884, fumarate hydratase mRNA). M: molecular standard, 500 bp, 400 bp, 300 bp, 200 bp, 100 bp.
3.2 Quantification of transcriptional abundance of mRNA and lncRNA transcripts
Both lncRNAs and mRNAs are constitutive transcripts in transcriptomes. Integrated analysis of mRNA and lncRNA transcripts in a given transcriptome will help to reveal the functional contexts where lncRNAs are expressed and provide insights into the potential functional association of lncRNAs (Luo et al., 2019; Xu et al., 2019; Zhang et al., 2020; Mattick et al., 2023; Yu et al., 2023). The updated transcriptome annotation, which incorporated the 4082 predicted lncRNA transcripts, was used as a reference for mapping RNA-seq reads to quantify the abundance of both mRNA and lncRNA transcripts. We analyzed published datasets from two independent studies. The dataset from Kulkarni et al. (2021) has six transcriptomes representing gene expression responses to different bacterial priming regimes and bacterial challenges (Kulkarni et al., 2021) and the dataset from (Yan et al., 2022) including 12 transcriptomes from circulating hemocytes (CH), heart and periosteal hemocytes (HPH) and control abdominal body (A) under conditions of naïve, injury, E. coli, and S. aureus challenges. Transcript abundance was measured in TPM.
In naïve mosquitoes with native or primed microbiota, their transcriptomes were overall similar but displayed discernable distinctions, as shown in the PCA analysis of transcriptional abundance (TPM) for both mRNAs and lncRNAs (Figures 2A, B). Upon injury or infection (Enterobacter or Serratia challenges), respective transcriptomes show distinct expression patterns, clearly separated from the naive transcriptomes with different priming regimes. In the mRNA plot (Figure 2A), the three components (PC1, PC2, and PC3) captured 59.4% of the variance, whereas in the lncRNA plot (Figure 2B), the three components explained only 32.5% of the variance. The remaining 67.5% of variance was split into more components, with each explaining only a small portion of variance, suggesting many diverse features in the transcriptomes. The observations suggest mosquitoes differentiate between priming regimes and bacterial species for challenge, leading to distinct transcriptomic responses for both mRNAs and lncRNAs. Notably, lncRNAs exhibited greater diversity than mRNAs under the same conditions.
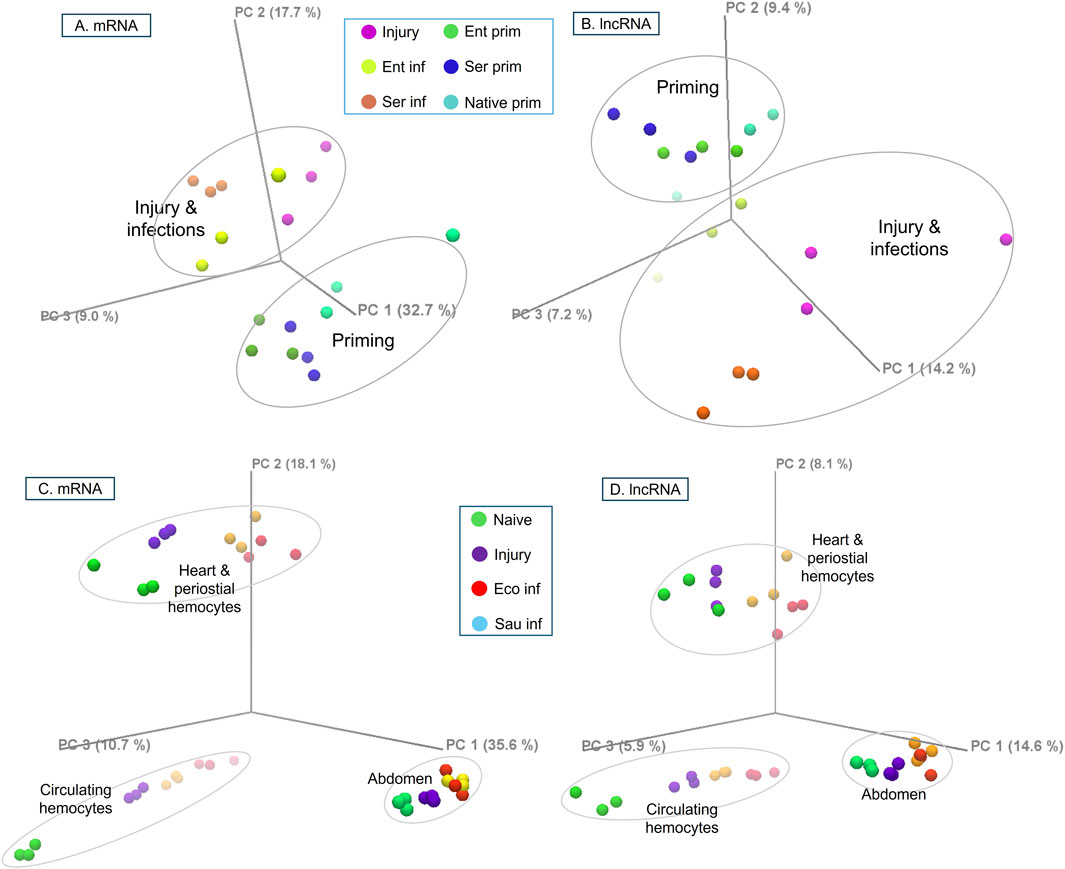
Figure 2. The PCA plots of TPM for mRNA and lncRNA transcripts in different conditions. The RNA-seq reads were mapped against mRNA or lncRNA annotation, and expression patterns were plotted in three principal components, PC1, PC2, and PC3. (A, B) mRNA and lncRNA expression of whole mosquitoes upon bacterial priming and challenge. (C, D) mRNA and lncRNA expression of circulating hemocytes, heart and periosteal hemocytes, and abdominal cells upon bacterial challenges. Injury: sterile H2O injection, Ent: Enterobacter sp., Ser: Serratia sp., Eco: E. coli, Sau: S. aureus.
In Yan et al., (2022), upon different conditions (i.e., naïve, injury, E. coli or S. aureus challenge), CH, HPH, and abdominal cells (AC) displayed distinct transcriptomic responses. Across these conditions, 8419 mRNAs and 357 lncRNAs were detected with TPM>1 in at least one condition. The difference between cell types was larger than the difference due to treatments. Both mRNAs and lncRNAs showed this pattern with a remarkable similarity (Figures 2C, D). In response to the treatments, the abdominal transcriptomes clustered more tightly. In contrast, CH and HPH transcriptomes exhibited greater variation, suggesting that the two types of hemocytes have discrete functional assignments and play distinct roles in the responses. The first three principal components (PC1-3) of the mRNA data captured 64.4% of the total variance. In contrast, the PC1-3 of the lncRNAs explained only 28.6% of the variance, suggesting that lncRNAs have a more complex transcriptional diversity than mRNAs.
We further analyzed the differentially expressed (DE) transcripts in different cell types, specifically AC, CH, and HPH. Figure 3 illustrates the Venn diagrams of DE transcripts (TPM>10 in at least one condition), which exhibit at least a 2-fold difference in expression and are supported by a false discovery rate (FDR) of p < 0.05. Under naive conditions, pairwise comparisons revealed 2818 DE mRNAs and 78 DE lncRNAs between CH and HPH hemocytes. Additionally, we observed 3045 – 3862 DE mRNAs and 96 – 114 DE lncRNAs between abdominal cells and hemocytes (Figures 3A, B). Upon infections, CH and HPH cells exhibited context-specific sets of DE mRNA and lncRNA transcripts. We also identified a core group of 1372 DE mRNAs and 80 DE lncRNAs that were shared across all comparisons (Figures 3C, D). When comparing the core DE mRNAs between CH and HPH, CH showed enrichment in DE mRNA transcripts associated with energy metabolism (genes involved in glycolysis, TCA, and mitochondrial ATP production) and immune responses (Rel1, CEC2, 4 CLIPBs, 6 LRIMs, 5 PPOs). On the other hand, HPH displayed enrichment in DE mRNAs across various functional categories, including 4 transcripts of ABCC transporters, 7 transcripts in the cuticular proteins family, 16 transcripts of cytochrome P450 (CYP) genes, 12 transcripts in the GPCR, galanin/allatostatin family, and a few transcripts of immune-related genes (Rel1, lysozyme, and 2 TEP genes).
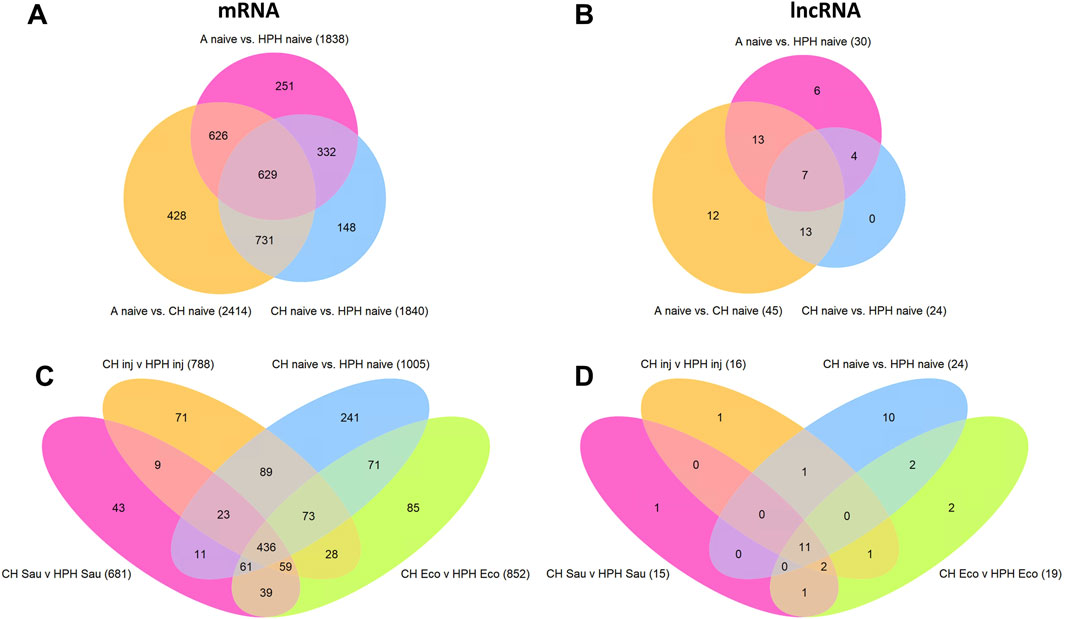
Figure 3. Venn diagrams of differentially expressed mRNA and lncRNA transcripts. (A, B), DE mRNA and lncRNA transcripts in three comparisons between naive abdominal cells (A), circulating hemocytes (CH), and heart and periosteal hemocytes (HPH). (C, D), DE mRNA and lncRNA transcripts in four comparisons between CH and HPH cells in conditions of naive, injury, E. coli, or S. aureus challenges. The comparisons include transcripts with TMP >10 in at least one condition, a minimum absolute fold change >2, and an FDR-adjusted p-value <0.05.
Overall, the profiles of pairwise DE transcripts and the shared core DE transcripts indicate that transcriptional regulation of lncRNAs, as well as mRNAs, is cell-type specific and condition-dependent. These findings align with the observations of lncRNAs documented in other organisms (Luo et al., 2019; Xu et al., 2019; Zhang et al., 2020; Mattick et al., 2023; Yu et al., 2023).
3.3 Co-expression network of lncRNAs and mRNAs
Next, we applied Weighted Correlation Network Analysis (WGCNA) to predict co-expressed networks of lncRNAs and mRNAs. To demonstrate the process, we utilized the dataset of transcriptional response to bacterial challenges (Kulkarni et al., 2021). WGCNA performed transcript clustering and generated modules consisting of co-expressed transcripts. The clustering dendrogram and corresponding modules are depicted in Figure 4A. In the comparison of injury versus naïve transcriptomes, WGCNA identified 8 modules. For the Enterobacter challenge versus injury transcriptomes, 11 modules were identified, while the Serratia challenge versus injury transcriptomes yielded 18 modules (Supplementary Table S5). Each module contained a mixture of mRNA and lncRNA transcripts, and the proportions of mRNAs and lncRNAs were highly correlated, as the proportion of mRNAs increased or decreased in a module, the proportion of lncRNAs tended to follow suit. The transcriptional responses to Enterobacter and Serratia infections exhibited distinct clustering/module patterns. For instance, in the transcriptome responding to Enterobacter infection, module turquoise comprised 7887 transcripts (6880 mRNA and 1007 lncRNA transcripts). However, in response to Serratia infection, these transcripts were spread across all 18 modules. In the transcriptome upon Serratia infection, the dominant module turquoise contained 3412 transcripts; these transcripts were spread across 11 modules in the transcriptome responding to Enterobacter. This indicates that different Gram-negative bacteria induce different transcriptomic networks. This observed transcriptional pattern aligns well with the PCA analysis presented in Figure 2.
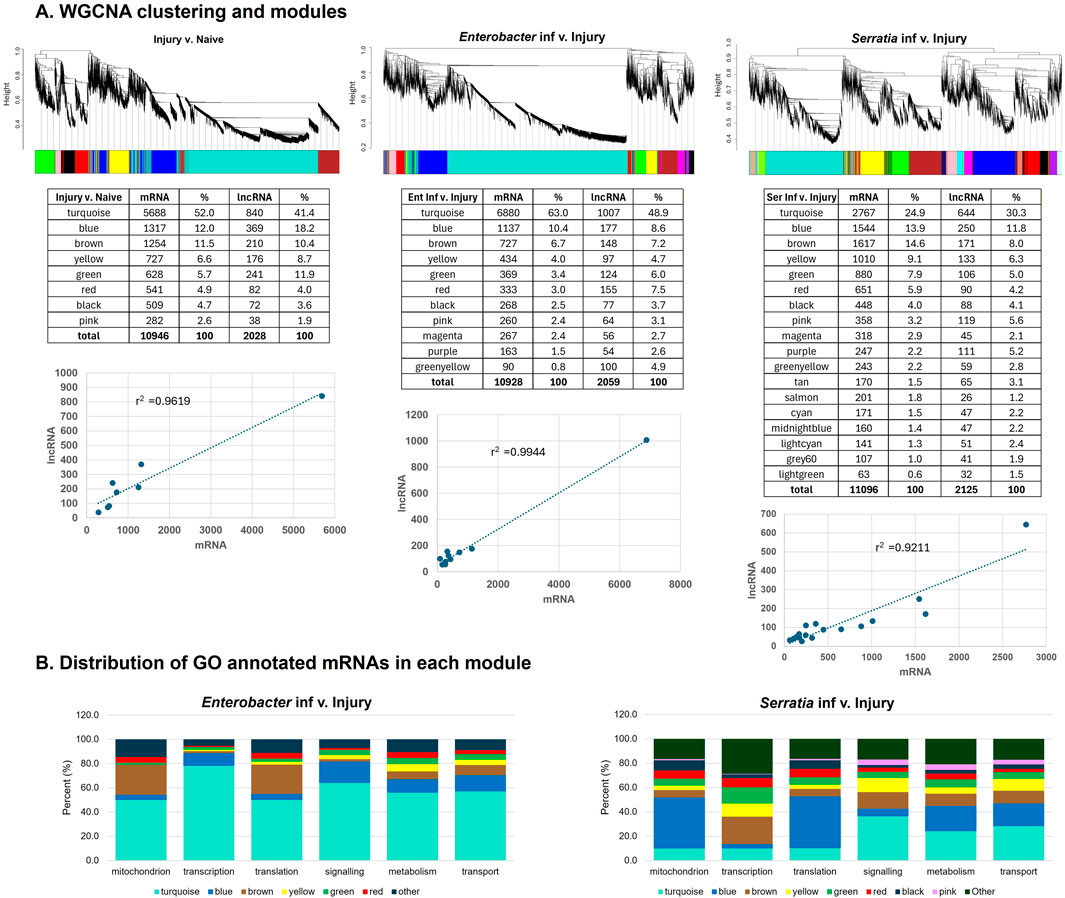
Figure 4. Cluster dendrogram and gene modules identified by WGCNA. (A) Hierarchical clustering and colored modules of co-expression of mRNAs and lncRNAs upon injury, Enterobacter and Serratia challenges. The number of mRNA and lncRNA transcripts in each module was presented in the tables under the respective cluster dendrogram. The correlation of mRNA and lncRNA counts among modules was plotted. (B) Distribution of modules in each of the 6 functional categories of GO annotation.
To gain insights into the potential functions of modules, we examined the gene ontology (GO) annotations for mRNAs. Approximately 70% of mRNA transcripts responsive to Enterobacter infection (9066 out of 12987) have GO annotations, while 9464 out of 13221 (71.6%) mRNA transcripts responded to Serratia infection have GO annotations. Based on their GO assignments, we categorized the mRNAs into six functional categories: mitochondria, transcription, translation/ribosome, signaling, transport, and metabolism. Upon the Enterobacter challenge, the dominant module turquoise contained mRNAs from all six categories (Figure 4B). For the Serratia infection, mRNAs from module blue were dispersed across the categories of translation, mitochondria, metabolism, and transport. In contrast, the module turquoise consisted of mRNAs from the categories of signaling, metabolism, and transport. These patterns suggest that transcriptional networks include genes from diverse functional categories. The co-expression of lncRNAs and mRNAs within these modules suggests potential functional connections, including regulatory interactions between them in carrying out these functions. The correlation provides insights into the possible roles of lncRNAs based on the known functions of mRNAs within the same module. WGCNA-based integrated analysis of mRNAs and lncRNAs in transcriptomes has been widely used for various lncRNA studies (Luo et al., 2019; Zhang et al., 2021; Almeida et al., 2023).
3.4 The mRNA and lncRNA transcripts in the midgut and salivary glands post Plasmodium falciparum infection
To compare the expression of mRNAs and lncRNAs between the midgut and salivary glands, we utilized five datasets, which include the midgut transcriptome datasets from the naïve midguts and salivary glands of 6- to 8-day-old adult An. gambiae Kisumu strain (Padrón et al., 2014; Kulkarni et al., 2021), and the P. falciparum Pf3D7-and Pf7G8-infected midgut 1 dpi of the An. gambiae L3-5 strain, the P. berghei infected midgut 1 dpi of the An. gambiae G3 strain (Padrón et al., 2014), the sugar-fed naïve midgut of An. gambiae G3 strain (Hixson et al., 2022) and the Pb-infected salivary glands 18-19 dpi of An. coluzzii (Pinheiro-Silva et al., 2015). In Ruiz et al. (2019), An. gambiae Kisumu strain was infected with Pf3D7. The midgut transcriptome (Pf-MG) was obtained from the midgut 7 days post-infection (7 dpi) when the oocysts were encapsulated, and the salivary gland transcriptome (Pf-SG) was derived from the salivary glands 14 dpi, corresponding to the sporozoites invasion. There were three replicates for each tissue type, which enabled a statistical comparison. First, we compared the mRNAs between MG and SG and identified those that were prominently expressed in either tissue (Supplementary Table S6). The Pf-MG transcriptome was enriched with mRNAs coding for protein families of aminopeptidases, carboxypeptidases, ribosomal proteins, chymotrypsins, trypsins, galectins, cytochrome P450s, aquaporins, ABC transporters, sugar transporters, and solute carrier proteins. In contrast, the Pf-SG transcriptome showed an enrichment of mRNAs encoding protein families like D7, salivary gland proteins, cytochrome P450, ABC transporters, and C-type lectins. Additionally, tissue-specific transcription factors were found in both transcriptomes. These findings were consistent with the original analyses. The TPMs from the five datasets are presented in Supplementary Table S6.
Notably, immune gene sets displayed distinct patterns between the Pf-infected midgut and salivary glands. Antimicrobial peptide (AMP) genes Def1, CEC1, CEC2, CecB, and Gam1 were abundantly expressed in both tissues, but the abundance was higher in the salivary glands. Caudal, a midgut-specific transcription factor that acts as a Rel2 antagonist (Clayton et al., 2013), is predominantly expressed in the midgut. Between the Pf-MG and Pf-SG transcriptomes, Cad exhibited 146.5-fold higher expression in Pf-MG than in Pf-SG, while Rel2 displayed 2.3-fold higher expression in Pf-SG compared to Pf-MG (Supplementary Table S6). Strikingly, families of leucine-rich repeat proteins (LRIMs), complement-like thioester-containing proteins (TEPs), prophenoloxidases (PPOs), C-type lectins (CTLs), CLIP serine proteases, and serpins (SRPNs) were expressed at higher TPM in Pf-SG than in Pf-MG. It appears that under naive conditions, the basal expression level of these immune genes was constitutively higher in SG than in MG (Supplementary Figure S2A), and Pf or Pb infection could transcriptionally affect some of these mRNAs in MG or SG (Supplementary Figures S2B, SC). The constitutive expression of these immune genes in SG was corroborated by the findings from a previous study reported by Scarpassa et al., in which the SG transcriptomes were profiled for five wild-captured Amazonian anophelines: An. darlingi, An. braziliensis, An. marajora, An. nuneztovari, and An. triannulatus. Putative proteins were predicted and annotated in the study (Scarpassa et al., 2019). From these annotated peptide sequences of An. darlingi, An. braziliensis, An. marajora, and An. triannulatus, the members of TEPs, LRIMs, CLIPs, and SRPNs were recognized. This transcriptional pattern of the immune genes suggests a few implications: (i) The differential expression of immune genes between MG 7 dpi and SG 14 dpi likely corresponds to the fact that the immune activities at 7 dpi have subsided in the midgut because with encapsulated oocysts that are immune-quiet, whereas the immune responses are highly active in the salivary glands at 14 dpi with ongoing sporozoite invasion; (ii) In the midgut, Caudal negatively regulates the immune gene transcription mediated by the Imd pathway transcription factor, Rel2, which may contribute to the microbial homeostasis in the midgut, as being suggested in (Clayton et al., 2013); (iii) SG is an active immune organ/tissue with a set of constitutively expressed immune genes. Intriguingly, an anatophysiological connection exists between the salivary glands and the gut. Lou et al. showed that during probing and blood feeding, the salivary gland proteins were depleted but detected by an anti-SG antibody in the midgut post a blood meal (Luo et al., 2000). It is possible that the SG-produced immune proteins, such as TEPs, LRIMs, and CLIPs, etc., remain functional after they enter the midgut during blood feeding, which complements the Caudal controlled low production of these immune proteins in the midgut. Further investigations are needed to test this hypothesis.
We then analyzed differentially expressed mRNAs and lncRNAs in the dataset from Ruiz et al. (2019). Using the criteria of TPM >10 in at least one sample, FDR p-value <0.05, and absolute fold change >2, we identified 2591/7217 (35.9%) mRNAs and 153/501 (30.5%) lncRNAs that were differentially expressed between the Pf-MG and Pf-SG transcriptomes. The volcano plots are presented in Figure 5.
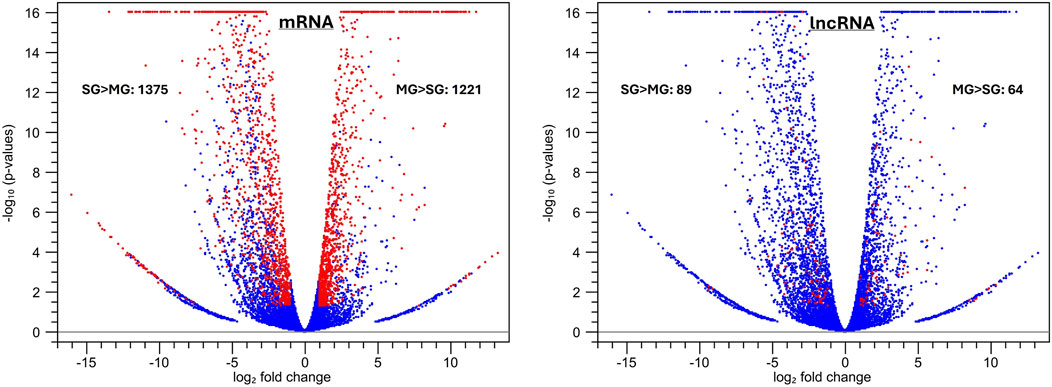
Figure 5. Volcano plots of differentially expressed mRNA and lncRNA transcripts between the Pf-MG and the Pf-SG transcriptomes from the dataset (Ruiz et al., 2019). The differentially expressed transcripts are highlighted in red using the filtering criteria: TPM >10 in at least one sample, FDR p-value <0.05, and absolute fold change >2 between MG and SG. A total of 2591 DE mRNAs and 153 DE lncRNAs were identified, represented by red dots in the graph.
Our analysis recognized lncRNAs in the midgut and salivary transcriptome (Supplementary Table S6); some are expressed differently between the two tissues with a P. falciparum infection (Figure 5). This data represents another example of context-dependent lncRNA expression in transcriptomes. The roles of lncRNAs in the context require further investigation to elucidate.
3.5 Polysome-associated lncRNAs
Polysome-associated long non-coding RNAs have garnered significant interest in recent years due to their pervasive presence, ability to code for noncanonical small ORFs (microproteins), and potential functional roles in various cellular processes (Chen et al., 2020; Li et al., 2020; Dangelmaier and Lal, 2021; Bonilauri and Dallagiovanna, 2022; Han et al., 2022; Bonilauri et al., 2024). In a prior investigation of mosquito transcriptomes, Mead et al. analyzed cellular and polysomal transcripts in the midgut of mosquitoes after they were fed blood meals infected with P. falciparum (IBM) or uninfected normal blood meal control (NBM) (Mead et al., 2012). In this study, we used the dataset to compare the dynamics of lncRNA transcripts in the polysomal fraction (PS) and non-polysomal fraction (NP) between the IBM and NBM samples. To determine the extent of transcript association with polysomes, we calculated the polysomal portion in the total cellular transcripts as PL = PS/(PS + NP) for both the IBM and NBM samples. Like mRNAs, lncRNAs also had a significant portion in the polysomal portion. With a cutoff TPM ≥5, there were 314 lncRNAs in the unfractionated transcriptome; 251 (79.9%) were associated with polysomes. Compared with the NBM transcriptome, IBM elevated the polysomal portion of mRNAs, which was consistent with the original analysis in Mead et al. (2012). Intriguingly, lncRNAs exhibited the same trend of polysome association as mRNAs upon the P. falciparum infection (Table 1). Regarding coding potential, 9 PS lncRNAs with a length range of 958–1257 nt had putative CDS for small peptides of 71–112 amino acids. These results suggest that some polysomal lncRNAs may be involved in translation regulation, and others may possess coding potential for small peptides. A recent study shows that lncRNAs are translated during human neuronal differentiation (Douka et al., 2021). Another study found that lncRNAs are commonly associated with the ribosome in a human cell line, suggesting that the ribosome may be a default destination and degradation site for most lncRNAs (Carlevaro-Fita et al., 2016). While the exact functions of lncRNAs at ribosomes are still debated, their association with polysomes indicates potential roles in modulating translation efficiency or RNA stability.
3.6 Epigenetic signature and transcriptional abundance of lncRNAs nearby
Many lncRNAs have promoters and transcribed regions associated with active chromatin signatures, as seen in Drosophila (Chen et al., 2016), and some lncRNAs may also influence chromatin architecture (Nickerson and Momen-Heravi, 2024). Using ChIP-seq, Gómez-Díaz et al. (2014) profiled the midgut (MG) epigenome for two key histone modifications: H3K27ac (associated with active promoters and enhancers) and H3K27me3 (associated with repressed regions). They also conducted RNA-seq on midguts from 6- to 8-day-old females. Their study recognized 6639 H3K27ac peaks and 12,939 H3K27me3 peaks in the naive midgut epigenome (Gómez-Díaz et al., 2014). These two epigenetic signatures are associated with the high and low expression levels of mRNAs in the midgut, respectively (Gómez-Díaz et al., 2014). We used their datasets to examine the transcriptional abundance of lncRNAs near these histone modifications. First, we mapped the midgut RNA-seq set against our mRNA-lncRNA annotation to measure the expression level (TPM) of transcripts and then identified the histone peaks that intersect with the lncRNAs or their promoters (defined as regions located 200 bp upstream of transcription start sites (TSSs), the same criteria Gómez-Díaz et al. used in their study). According to these criteria, 307 H3K27ac peaks intersect with 243 lncRNA genes, 237 of 243 (97.5%) lncRNAs were expressed with the TPM range of 0.1–562.1 with a mean of 8.0, and 365 H3K27me3 peaks intersect with 248 lncRNA genes, and 168 of 248 (67.7%) lncRNAs were expressed with the TPM range of 0.1–14.6 with a mean of 0.9. This indicates more expressed lncRNAs were associated with the H3K27ac peaks than with the H3K27me3 peaks (Chi-Square = 75.38, p < 0.00001). This pattern suggests that lncRNA expression is also associated with both epigenetic signatures in the midgut transcriptome. Figure 6 presents four genomic regions with annotated histone modification peaks [from Gómez-Díaz et al. (2014)] and nearby lncRNA and/or mRNA transcripts, along with the ChIP-seq reads mapping tracks for H3K27ac, H3K27me3, and the input background control, MG RNA-seq reads mapping tracks and respective TPM values. For instance, H3K27ac peak 1767 is associated with AGAP001548 mRNA and lncRNA 3352, both are expressed abundantly (Figure 6A), while in the region where H3K27me3 peaks 760, 315, and 202 are located, AGAP013210 and lncRNA 14014 are both expressed in a very low level with TPM less than 0.5 (Figure 6D). Our analysis suggests possible epigenetic control of lncRNA expression in mosquitoes. It has been shown that the interactions between lncRNAs and epigenetic modifications are mutually shaped by both sides, i.e., lncRNA expression can be controlled epigenetically, and epigenetic modification can be regulated by certain lncRNAs (Mangiavacchi et al., 2023). This is an unexplored area in mosquito research. It would be interesting to see investigations in the future.
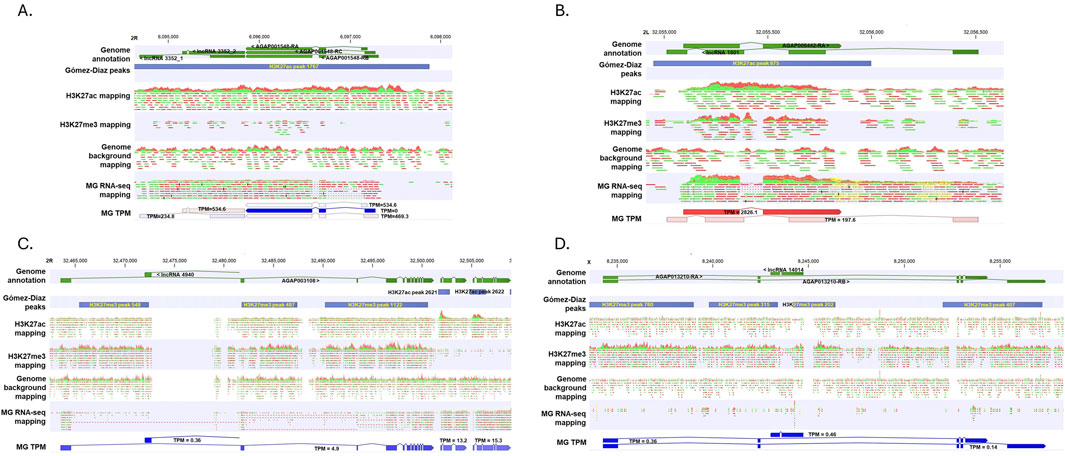
Figure 6. Representative genomic regions where active mark H3K27ac and repressive mark H3K27me3 and nearby genes are located. Genome annotation, annotated ChIP-seq peaks, ChIP reads mapping tracks, MG RNA-seq reads mapping and transcript TPM were aligned. Gómez-Díaz peaks were derived from the original study (Gómez-Díaz et al., 2014). (A-D) represent 4 genomic regions. See the main text for details.
Enhancers are crucial DNA regulatory elements that play a significant role in initiating transcription by recruiting transcription factors (Ray-Jones and Spivakov, 2021). Active enhancers can be transcribed into enhancer RNAs (eRNAs), which are a part of lncRNAs. Recently, Holm et al. identified 3288 active genomic enhancers from wild-caught specimens of An. coluzzii from Burkina Faso using Self-Transcribing Active Regulatory Region sequencing (STARR-seq) (Holm et al., 2021). According to the enhancer coordinates, we located 278 lncRNAs on the 5′ side of enhancers and 270 lncRNAs on the 3′ side of enhancers, with a distance between 0 and 5,000 nt (Supplementary Table S7). This distribution suggests possible correlations between lncRNAs and enhancers; either lncRNA expression is regulated by enhancers, or certain lncRNAs represent eRNAs of co-localized enhancers. The cataloged lncRNAs described here are unlikely to include many eRNAs as eRNAs are not frequently polyadenylated.
3.7 Summary Remarks
The identification of lncRNAs from transcriptomes has been widely applied across various organisms (Muret et al., 2017; Kern et al., 2018; Bennett et al., 2021), including An. gambiae (Padrón et al., 2014; Jenkins et al., 2015). In our study, we employed a transcript discovery tool to identify lncRNAs from the selected transcriptomes derived from whole mosquitoes, midgut, salivary glands, hemocytes under different conditions (e.g., sugar-meal, blood-meal, bacterial infection, or Plasmodium infection). The predicted lncRNAs were integrated into the transcript annotation, and this updated annotation was used for transcriptome analysis encompassing both mRNAs and lncRNAs, as depicted in Figure 1. As exemplified in Figure 2, the transcription patterns of lncRNAs and mRNAs exhibited similar principal component analysis (PCA) patterns. This similarity was observed in both whole mosquito transcriptomes upon bacterial challenges and hemocyte transcriptomes upon bacterial challenges, suggesting context-dependent co-expression of mRNA and lncRNA. Further validation came from the Weighted Gene Co-expression Network Analysis (WGCNA), which revealed that transcriptional networks comprised both mRNAs and lncRNAs (Figure 4). Comparing transcriptomes from midguts and salivary glands revealed previously overlooked patterns. Notably, many immune gene families were expressed more abundantly in the salivary glands than in the midguts. Additionally, tissue-specific expressions of lncRNAs were identified in the comparison between the midgut and salivary glands (Figure 5). Furthermore, by mapping polysome-associated transcripts, we discovered that lncRNAs can engage with translational machinery (Table 1). Finally, we examined the genomic regions with epigenetic modifications of H3K27ac and H3K27me3, along with the expression levels of nearby lncRNAs in a midgut dataset (Figure 6). Conclusively, our analyses demonstrate that lncRNAs are actively expressed in all transcriptomes, and their composition and transcriptional regulation are context-dependent. We recommend including lncRNAs in reference for comprehensive transcriptome analyses. The lncRNA annotation also provides a valuable resource for further investigations into the functions of lncRNAs across various life traits in An. gambiae.
Data availability statement
The datasets presented in this study can be found in online repositories. The names of the repository/repositories and accession number(s) can be found in the article/Supplementary Material.
Ethics statement
The manuscript presents research on animals that do not require ethical approval for their study.
Author contributions
JX: Conceptualization, Data curation, Formal Analysis, Funding acquisition, Methodology, Project administration, Resources, Writing – original draft, Writing – review and editing. KH: Conceptualization, Data curation, Formal Analysis, Writing – review and editing. MR: Formal Analysis, Funding acquisition, Writing – review and editing. VK: Formal Analysis, Writing – review and editing.
Funding
The author(s) declare that financial support was received for the research and/or publication of this article. This work was partially supported by the Biology Department, New Mexico State University to JX MR was supported by the National Institutes of Health, NIAID #AI145999.
Acknowledgments
This study utilized publicly available data from the NCBI database. The authors appreciate the original research and published research articles. The authors thank Tathagata Debnath, a graduate student in the Computer Science Department of New Mexico State University, for his assistance in the data analysis in the early stage of this study.
Conflict of interest
The authors declare that the research was conducted in the absence of any commercial or financial relationships that could be construed as a potential conflict of interest.
Generative AI statement
The author(s) declare that no Generative AI was used in the creation of this manuscript.
Publisher’s note
All claims expressed in this article are solely those of the authors and do not necessarily represent those of their affiliated organizations, or those of the publisher, the editors and the reviewers. Any product that may be evaluated in this article, or claim that may be made by its manufacturer, is not guaranteed or endorsed by the publisher.
Supplementary material
The Supplementary Material for this article can be found online at: https://www.frontiersin.org/articles/10.3389/frnar.2025.1555885/full#supplementary-material
References
Ahmad, P., Bensaoud, C., Mekki, I., Rehman, M. U., and Kotsyfakis, M. (2021). Long non-coding RNAs and t
Almeida, M. C., Felix, J., de, S., Lopes, M. F., da, S., de Athayde, F. R. F., Troiano, J. A., Scaramele, G.N. F., et al. (2023). Co-expression analysis of lncRNA and mRNA suggests a role for ncRNA-mediated regulation of host-parasite interactions in primary skin lesions of patients with American tegumentary leishmaniasis. Acta Tropica 245, 106966. doi:10.1016/j.actatropica.2023.106966
Azlan, A., Obeidat, S. M., Theva Das, K., Yunus, M. A., and Azzam, G. (2021). Genome-wide identification of Aedes albopictus long noncoding RNAs and their association with dengue and Zika virus infection. PLoS Negl. Trop. Dis. 15, e0008351. doi:10.1371/journal.pntd.0008351
Azlan, A., Obeidat, S. M., Yunus, M. A., and Azzam, G. (2019). Systematic identification and characterization of Aedes aegypti long noncoding RNAs (lncRNAs). Sci. Rep. 9, 12147. doi:10.1038/s41598-019-47506-9
Bennett, M., Ulitsky, I., Alloza, I., Vandenbroeck, K., Miscianinov, V., Mahmoud, A. D., et al. (2021). Novel transcript discovery expands the repertoire of pathologically-associated, long non-coding RNAs in vascular smooth muscle cells. Int. J. Mol. Sci. 22, 1484. doi:10.3390/ijms22031484
Bonilauri, B., and Dallagiovanna, B. (2022). Microproteins in skeletal muscle: hidden keys in muscle physiology. J. Cachexia Sarcopenia Muscle 13, 100–113. doi:10.1002/jcsm.12866
Bonilauri, B., Ribeiro, A. L., Spangenberg, L., and Dallagiovanna, B. (2024). Unveiling polysomal long non-coding RNA expression on the first day of adipogenesis and osteogenesis in human adipose-derived stem cells. Int. J. Mol. Sci. 25, 2013. doi:10.3390/ijms25042013
Borkiewicz, L., Kalafut, J., Dudziak, K., Przybyszewska-Podstawka, A., and Telejko, I. (2021). Decoding LncRNAs. Cancers (Basel) 13, 2643. doi:10.3390/cancers13112643
Carlevaro-Fita, J., Rahim, A., Guigó, R., Vardy, L. A., and Johnson, R. (2016). Cytoplasmic long noncoding RNAs are frequently bound to and degraded at ribosomes in human cells. RNA 22, 867–882. doi:10.1261/rna.053561.115
Carninci, P., and Hayashizaki, Y. (2007). Noncoding RNA transcription beyond annotated genes. Curr. Opin. Genet. and Dev. 17, 139–144. doi:10.1016/j.gde.2007.02.008
Chen, J., Brunner, A. D., Cogan, J. Z., Nunez, J. K., Fields, A. P., Adamson, B., et al. (2020). Pervasive functional translation of noncanonical human open reading frames. Science 367, 1140–1146. doi:10.1126/science.aay0262
Chen, M. J., Chen, L. K., Lai, Y. S., Lin, Y. Y., Wu, D. C., Tung, Y. A., et al. (2016). Integrating RNA-seq and ChIP-seq data to characterize long non-coding RNAs in Drosophila melanogaster. BMC Genomics 17, 220. doi:10.1186/s12864-016-2457-0
Choudhary, C., Sharma, S., Meghwanshi, K. K., Patel, S., Mehta, P., Shukla, N., et al. (2021). Long non-coding RNAs in insects. Anim. (Basel) 11, 1118. doi:10.3390/ani11041118
Claverie, J. M. (2005). Fewer genes, more noncoding RNA. Science 309, 1529–1530. doi:10.1126/science.1116800
Clayton, A. M., Cirimotich, C. M., Dong, Y., and Dimopoulos, G. (2013). Caudal is a negative regulator of the Anopheles IMD pathway that controls resistance to Plasmodium falciparum infection. Dev. and Comp. Immunol. 39, 323–332. doi:10.1016/j.dci.2012.10.009
Dangelmaier, E. A., and Lal, A. (2021). Experimental validation of the noncoding potential for lncRNAs. Methods Mol. Biol. 2348, 221–230. doi:10.1007/978-1-0716-1581-2_15
Douka, K., Birds, I., Wang, D., Kosteletos, A., Clayton, S., Byford, A., et al. (2021). Cytoplasmic long noncoding RNAs are differentially regulated and translated during human neuronal differentiation. RNA 27, 1082–1101. doi:10.1261/rna.078782.121
Etebari, K., Asad, S., Zhang, G., and Asgari, S. (2016). Identification of Aedes aegypti long intergenic non-coding RNAs and their association with wolbachia and dengue virus infection. PLoS Negl. Trop. Dis. 10, e0005069. doi:10.1371/journal.pntd.0005069
Fan, C., Xiong, F., Tang, Y., Li, P., Zhu, K., Mo, Y., et al. (2022). Construction of a lncRNA–mRNA Co-expression network for nasopharyngeal carcinoma. Front. Oncol. 12, 809760. doi:10.3389/fonc.2022.809760
Farley, E. J., Eggleston, H., and Riehle, M. M. (2021). Filtering the junk: assigning function to the mosquito non-coding genome. Insects 12, 186. doi:10.3390/insects12020186
Fontaine, M. C., Pease, J. B., Steele, A., Waterhouse, R. M., Neafsey, D. E., Sharakhov, I. V., et al. (2015). Mosquito genomics. Extensive introgression in a malaria vector species complex revealed by phylogenomics. Science 347, 1258524. doi:10.1126/science.1258524
Gómez-Díaz, E., Rivero, A., Chandre, F., and Corces, V. G. (2014). Insights into the epigenomic landscape of the human malaria vector Anopheles gambiae. Front. Genet. 5, 277. doi:10.3389/fgene.2014.00277
Han, C., Sun, L., Pan, Q., Sun, Y., Wang, W., and Chen, Y. (2022). Polysome profiling followed by quantitative PCR for identifying potential micropeptide encoding long non-coding RNAs in suspension cell lines. Star. Protoc. 3, 101037. doi:10.1016/j.xpro.2021.101037
Hixson, B., Bing, X. L., Yang, X., Bonfini, A., Nagy, P., and Buchon, N. (2022). A transcriptomic atlas of Aedes aegypti reveals detailed functional organization of major body parts and gut regional specializations in sugar-fed and blood-fed adult females. Elife 11, e76132. doi:10.7554/eLife.76132
Holm, I., Nardini, L., Pain, A., Bischoff, E., Anderson, C. E., Zongo, S., et al. (2021). Comprehensive genomic discovery of non-coding transcriptional enhancers in the african malaria vector Anopheles coluzzii. Front. Genet. 12, 785934. doi:10.3389/fgene.2021.785934
Jenkins, A. M., Waterhouse, R. M., and Muskavitch, M. A. (2015). Long non-coding RNA discovery across the genus anopheles reveals conserved secondary structures within and beyond the Gambiae complex. BMC Genomics 16, 337. doi:10.1186/s12864-015-1507-3
Kern, C., Wang, Y., Chitwood, J., Korf, I., Delany, M., Cheng, H., et al. (2018). Genome-wide identification of tissue-specific long non-coding RNA in three farm animal species. BMC Genomics 19, 684. doi:10.1186/s12864-018-5037-7
Kulkarni, A., Pandey, A., Trainor, P., Carlisle, S., Chhilar, J. S., Yu, W., et al. (2021). Trained immunity in Anopheles gambiae: antibacterial immunity is enhanced by priming via sugar meal supplemented with a single gut symbiotic bacterial strain. Front. Microbiol. 12, 649213. doi:10.3389/fmicb.2021.649213
Langfelder, P., and Horvath, S. (2008). WGCNA: an R package for weighted correlation network analysis. BMC Bioinforma. 9, 559. doi:10.1186/1471-2105-9-559
Legeai, F., and Derrien, T. (2015). Identification of long non-coding RNAs in insects genomes. Curr. Opin. Insect Sci. 7, 37–44. doi:10.1016/j.cois.2015.01.003
Li, X. L., Pongor, L., Tang, W., Das, S., Muys, B. R., Jones, M. F., et al. (2020). A small protein encoded by a putative lncRNA regulates apoptosis and tumorigenicity in human colorectal cancer cells. Elife 9, e53734. doi:10.7554/eLife.53734
Liu, W., Cheng, P., Zhang, K., Gong, M., Zhang, Z., and Zhang, R. (2022). Systematic identification and characterization of long noncoding RNAs (lncRNAs) during Aedes albopictus development. PLoS Negl. Trop. Dis. 16, e0010245. doi:10.1371/journal.pntd.0010245
Luo, E., Matsuoka, H., Yoshida, S., Iwai, K., Arai, M., and Ishii, A. (2000). Changes in salivary proteins during feeding and detection of salivary proteins in the midgut after feeding in a malaria vector mosquito, Anopheles stephensi (Diptera: Culicidae). Med. Entomology Zoology 51, 13–20. doi:10.7601/mez.51.13
Luo, M., Wang, L., Yin, H., Zhu, W., Fu, J., and Dong, Z. (2019). Integrated analysis of long non-coding RNA and mRNA expression in different colored skin of koi carp. BMC Genomics 20, 515. doi:10.1186/s12864-019-5894-8
Mangiavacchi, A., Morelli, G., and Orlando, V. (2023). Behind the scenes: how RNA orchestrates the epigenetic regulation of gene expression. Front. Cell Dev. Biol. 11, 1123975. doi:10.3389/fcell.2023.1123975
Mattick, J. S. (2005). The functional genomics of noncoding RNA. Science 309, 1527–1528. doi:10.1126/science.1117806
Mattick, J. S., Amaral, P. P., Carninci, P., Carpenter, S., Chang, H. Y., Chen, L. L., et al. (2023). Long non-coding RNAs: definitions, functions, challenges and recommendations. Nat. Rev. Mol. Cell Biol. 24, 430–447. doi:10.1038/s41580-022-00566-8
Mead, E. A., Li, M., Tu, Z., and Zhu, J. (2012). Translational regulation of Anopheles gambiae mRNAs in the midgut during Plasmodium falciparum infection. BMC Genomics 13, 366. doi:10.1186/1471-2164-13-366
Muret, K., Klopp, C., Wucher, V., Esquerre, D., Legeai, F., Lecerf, F., et al. (2017). Long noncoding RNA repertoire in chicken liver and adipose tissue. Genet. Sel. Evol. 49, 6. doi:10.1186/s12711-016-0275-0
Nickerson, J. A., and Momen-Heravi, F. (2024). Long non-coding RNAs: roles in cellular stress responses and epigenetic mechanisms regulating chromatin. Nucleus 15, 2350180. doi:10.1080/19491034.2024.2350180
Padrón, A., Molina-Cruz, A., Quinones, M., Ribeiro, J. M., Ramphul, U., Rodrigues, J., et al. (2014). In depth annotation of the Anopheles gambiae mosquito midgut transcriptome. BMC Genomics 15, 636. doi:10.1186/1471-2164-15-636
Pinheiro-Silva, R., Borges, L., Coelho, L. P., Cabezas-Cruz, A., Valdés, J. J., do Rosário, V., et al. (2015). Gene expression changes in the salivary glands of Anopheles coluzzii elicited by Plasmodium berghei infection. Parasites and Vectors 8, 485. doi:10.1186/s13071-015-1079-8
Ray-Jones, H., and Spivakov, M. (2021). Transcriptional enhancers and their communication with gene promoters. Cell Mol. Life Sci. 78, 6453–6485. doi:10.1007/s00018-021-03903-w
Ruiz, J. L., Yerbanga, R. S., Lefèvre, T., Ouedraogo, J. B., Corces, V. G., and Gómez-Díaz, E. (2019). Chromatin changes in Anopheles gambiae induced by Plasmodium falciparum infection. Epigenetics and Chromatin 12 (5), 5. doi:10.1186/s13072-018-0250-9
Scarpassa, V. M., Debat, H. J., Alencar, R. B., Saraiva, J. F., Calvo, E., Arca, B., et al. (2019). An insight into the sialotranscriptome and virome of Amazonian anophelines. BMC Genomics 20, 166. doi:10.1186/s12864-019-5545-0
Sielemann, K., Hafner, A., and Pucker, B. (2020). The reuse of public datasets in the life sciences: potential risks and rewards. PeerJ 8, e9954. doi:10.7717/peerj.9954
Wold, S., Esbensen, K., and Geladi, P. (1987). Principal component analysis. Chemom. Intelligent Laboratory Syst. 2, 37–52. doi:10.1016/0169-7439(87)80084-9
Xu, Q., Liu, X., Chao, Z., Wang, K., Wang, J., Tang, Q., et al. (2019). Transcriptomic analysis of coding genes and non-coding RNAs reveals complex regulatory networks underlying the black back and white belly coat phenotype in Chinese wuzhishan pigs. Genes (Basel) 10, 201. doi:10.3390/genes10030201
Yan, Y., Sigle, L. T., Rinker, D. C., Esté1vez-Lao, T. Y., Capra, J. A., and Hillyer, J. F. (2022). The immune deficiency and c-Jun N-terminal kinase pathways drive the functional integration of the immune and circulatory systems of mosquitoes. Open Biol, 12(9), 19220111. doi:10.1098/rsob.220111
Yu, S., Wang, G., Liao, J., Shen, X., and Chen, J. (2023). Integrated analysis of long non-coding RNAs and mRNA expression profiles identified potential interactions regulating melanogenesis in chicken skin. Br. Poult. Sci. 64, 19–25. doi:10.1080/00071668.2022.2113506
Zafar, J., Huang, J., Xu, X., and Jin, F. (2023). Recent Advances and Future Potential of Long Non-Coding RNAs in Insects. Int J Mol Sci, 24(3), 1457–1470. doi:10.3390/ijms24032605
Zhang, S., You, L., Xu, Q., Ou, J., Wu, D., Yuan, X., et al. (2020). Distinct long non-coding RNA and mRNA expression profiles in the hippocampus of an attention deficit hyperactivity disorder model in spontaneously hypertensive rats and control wistar Kyoto rats. Brain Res. Bull. 161, 177–196. doi:10.1016/j.brainresbull.2020.03.015
Keywords: long noncoding RNA (lncRNA), RNA-Seq, transcriptome, mosquitoes, Anopheles gambiae
Citation: Xu J, Hu K, Riehle MM and Khadka VS (2025) Identification of long noncoding RNAs (lncRNAs) and co-transcriptional analysis of mRNAs and lncRNAs in transcriptomes of Anopheles gambiae. Front. RNA Res. 3:1555885. doi: 10.3389/frnar.2025.1555885
Received: 05 January 2025; Accepted: 27 March 2025;
Published: 15 April 2025.
Edited by:
Kaushlendra Tripathi, University of Alabama at Birmingham, United StatesReviewed by:
Scott Barbee, University of Denver, United StatesMohammad Kashif, National Institute of Allergy and Infectious Diseases (NIH), United States
Copyright © 2025 Xu, Hu, Riehle and Khadka. This is an open-access article distributed under the terms of the Creative Commons Attribution License (CC BY). The use, distribution or reproduction in other forums is permitted, provided the original author(s) and the copyright owner(s) are credited and that the original publication in this journal is cited, in accordance with accepted academic practice. No use, distribution or reproduction is permitted which does not comply with these terms.
*Correspondence: Jiannong Xu, anh1QG5tc3UuZWR1; Kai Hu, a2FpLmh1QHVtYXNzbWVkLmVkdQ==; Michelle M. Riehle, bXJpZWhsZUBtY3cuZWR1