- 1Earthwatch Europe, Oxford, United Kingdom
- 2Department of Civil and Environmental Engineering, Imperial College London, London, United Kingdom
- 3Department of Earth Sciences, Royal Holloway, University of London, Egham, United Kingdom
- 4Department of Biotechnology, Chemistry and Pharmacy, University of Siena, Consorzio Interuniversitario Nazionale per la Scienza e Tecnologia dei Materiali (INSTM), Siena, Italy
Urban green spaces are often promoted as nature-based solutions, thus helping to mitigate the negative effects of climate change. Estimating the potential environmental benefits provided by urban green space is difficult because of inconsistencies in management practices and their heterogeneous nature. Collecting data across such a spectrum of contexts at a large scale is costly and time consuming. In this study, we explore a novel integrated method for citizen scientists to assess the flood mitigation potential of urban green spaces. In three European cities, citizen scientists measured infiltration rate and associated soil characteristics in managed and unmanaged urban green spaces. The results show that simple citizen science-based measurements can indicate the infiltration potential (i.e., high vs. low) of soil at these sites. Infiltration rate was best predicted by measurements of soil compaction, soil color, air temperature, and level of insolation (i.e., high vs. low). These simple, fast methods can be repeated over time and space by citizen scientists to provide robust estimates of soil characteristics and the infiltration potential of soils that exist in similar temperate urban areas. A classification flow diagram was constructed and validated that allows citizen scientists to carry out such tests over a wider geographical region and at a higher frequency than would be available to research scientists alone. Most importantly, it allows citizens to take actions to improve infiltration in their local green space and support local flood resilience.
Introduction
By 2050 the global urban population is predicted to be twice that living in rural areas (United Nations, 2019). Whilst social and economic opportunities are driving the move to urban areas, there are clear environmental challenges to maintaining a healthy and habitable urban environment (Elmqvist et al., 2013). Many of these challenges are related to the loss of natural areas that provide ecosystem services e.g., water and air purification, temperature regulation, flood protection, and a range of important cultural services. One example is the loss or modification of former green space to gray infrastructure, resulting in increased impervious surfaces. This in turn increases urban run-off and transport of pollutants, resulting in increased flood risk and degraded water quality (Miller and Hutchins, 2017).
Climate change stands to amplify urban environmental challenges (Emilsson and Ode Sang, 2017), not least regarding urban hydrology. In Northern Europe, rain is predicted to fall more intensely, with the UK having seen a 10% increase in the number of days with widespread heavy rain in the past 30 years (Kendon et al., 2019). An increase in urban flooding across Europe, even under conservative warming projections, will impact soil and water quality (Alfieri et al., 2018), as well as overwhelming drainage systems that were designed for different land cover and climate conditions. For example, in London alone, 1.25 m people and 800,000 properties are at risk from tidal or surface water flooding (Future of London, 2016).
Nature-based solutions (NbS) such as urban green space offers an environmentally friendly method to mitigate urban flood risk (Keesstra et al., 2018). For example, urban trees provide multiple benefits within the hydrological cycle, such as intercepting rainfall with their canopy, absorbing water from the soil for their growth (McElrone et al., 2013) and aiding water infiltration through the modification of soil conditions (Li et al., 2014). In particular, infiltration is related to the magnitude of pluvial flooding as high infiltration arrests the celerity of the flood wave peak and reduces surface runoff (Horton, 1941). Infiltration rate is defined as the rate at which water can enter into soils and filter through subsequent layers (Pitt et al., 2008). It is regulated by soil characteristics such as porosity, bulk density, texture, and mineralogy (Lado and Ben-Hur, 2004; Yang and Zhang, 2011). Thus, infiltration rate can be used as an indicator of an area's ability to deal with heavy rainfall. However, knowledge of how soil and tree management practices affect infiltration of pluvial events and other ecosystem services is limited (Gaston et al., 2013). Moreover, given the wide range of park, garden, or street tree soil characteristics (e.g., compaction, water content, amount of organic matter), understanding the conditions of green spaces across the urban continuum is challenging.
Major urban areas, where gray infrastructure and population numbers are high, require special care and analysis for assigning green spaces to best facilitate their ecosystem services. Greater London, for example, has an estimated 21% canopy cover (Treeconomics, 2015), presenting a diversity of tree species located across a multitude of soil types. Therefore, identifying appropriate land management approaches for a specific location requires information on a fine scale that accounts for the substantial heterogeneity and patchwork make-up of urban green spaces (Gaston et al., 2013). Identifying areas of flood vulnerability or of high potential mitigation value is hampered by this heterogeneity, with data collection at the scale of individual trees and their microhabitat being difficult both logistically and financially (Toms and Newson, 2006; Hobbs and White, 2012). Information at a more detailed scale is key to understanding the capacity of urban green space to process heavy rain events and to be able to manage this NbS effectively.
Citizen science has proven to be an effective method for collecting environmental data. Citizen science involves the collection and/or analysis of data by the general public. Although a long-standing method, over the past decade the number and variety of citizen science projects have increased dramatically (Bonney et al., 2014). Simultaneous to increasing the scale of scientific data collection, the involvement of citizens in the scientific process fosters engagement and promotes education in local environmental issues, as well as opportunities for collaboration between stakeholders within a community (Thornhill et al., 2016; McKineley et al., 2017). Citizen scientists have been shown to enhance the geographical, temporal and contextual scope of data collection at the catchment and sub-catchment scale (Holck, 2007; Szabo et al., 2010; Belt and Krausman, 2012; Hadj-Hammou et al., 2017; Cunha et al., 2019). Citizen science also offers multiple pathways to the provision of real-time flooding data, integrated as part of early-warning systems for pluvial flooding (See, 2019; Pandeya et al., 2020).
Trees and their surrounding soil represent one of the few remaining natural amenities accessible to and managed by local citizens in urban areas, whether they are present in residents' own gardens, line city streets or adorn parks and green spaces. Thus, the activation and participation of citizen scientists to help determine soil conditions with respect to infiltration of high precipitation events might help to close the gap between the modeling and management of green spaces. Furthermore, the identification of sites with poor infiltration capacity could allow citizens to take simple actions in their own green space to improve their status (Soil Association, 2016).
Here we examine a range of simple citizen science methods to measure soil characteristics and assess soil water infiltration rate in urban areas, which could be used to enhance knowledge of pluvial flooding risk for a certain area. We validate these methods under different land management regimes across three European cities to identify if relatively quick and uncomplicated citizen scientist measurements can be used as a proxy for infiltration potential. The methods are coupled with a classification flow diagram that guides a citizen scientist through the process of investigating the flood mitigation capacity of their local urban green spaces. Although the classification flow diagram demonstrated in this study is most suited to the locations and tree species researched here, the workflow can serve as a blueprint to be extended to other contexts in the future.
Methods
Citizen Scientists
Over the course of the 2-year study, 520 citizen scientists were trained and assessed soil characteristics in three urban parks. Following a 1-h training session led by professional physical scientists on the background and methodologies of urban soils, trees, and infiltration processes, participant teams took measurements in urban parks (section Sites) on 2 consecutive days. The total sampling and measurement time was ~2 h per team per day. Over the course of 2 years (2018–2019), each location was revisited by different citizen scientists several times in spring, summer, and autumn (30 events in total).
Sites
The study sites were located in Birmingham, London, and Chantilly (France), cities which experience similar climatic conditions (Average annual temperature: 9, 11, and 11°C and average annual precipitation: 64.1, 57.5, and 60 mm in Birmingham, London, and Chantilly, respectively, Climate-Data, 2020). Here, mature (over 30 years old) Linden (Tilia spp) trees, one of the most common tree species found in urban environments, were selected in three urban parks, under which measurements were taken. Six Linden trees were located in Cannon Hill Park, Birmingham, a further six in Kew Gardens, London and three Linden trees were selected in Les Fontaines, Chantilly (France). Four sampling sites were identified, two sites due north and two sites due south of each tree (n = 60) (Supplementary Figure 1). These represented contrasting levels of direct solar radiation, whereby southern (less shaded) sites were exposed to more solar radiation during sampling periods (spring, summer, autumn). We refer to this as level of insolation, with southern sites exposed to high insolation and northern sites, low insolation.
Each sample site was classified in terms of its management regime. Managed sites were defined as those having the majority of leaf litter and undergrowth vegetation cleared, typically these sample sites were located on amenity grass and had no or few nearby trees (n = 27). Meanwhile unmanaged sites were defined as having little to no human intervention in the removal of litter or undergrowth thus there was often a mix of herbaceous ground cover, vegetation litter and bare soil with other trees and vegetation close by (n = 26) (Supplementary Figure 2). Some sample sites (n = 7) were identified as intermediate (unmanaged but with a mown path or paved surface immediately nearby) and so were not included in the analyses.
Citizen Science Methods
At each sampling site, citizen scientists estimated soil color, compaction, texture, moisture content, and infiltration rate. Citizen scientists also collected surface and soil ring samples for subsequent laboratory analysis.
Participants assessed soil color using the Munsell soil color chart 7.5YR, identifying the color that most closely matched their soil sample. Munsell color co-ordinates were later converted to a numerical value (Supplementary Figure 3).
Compaction was measured on a patch of undisturbed soil, with the surface vegetation removed, using an Eijkelkamp pocket penetrometer. Participants measured the compaction of the topsoil by pushing the shaft (to a depth of 6.35 mm) of the pocket penetrometer with a constant force into the soil. The internal spring is calibrated such that participants read the compressive force required to insert the penetrometer to the nearest 0.25 kg/cm2.
Soil texture was characterized by participants following a standard soil handling protocol (Yolcubal et al., 2004). Following a decision diagram, participants classified their sample into specific categories of soil texture.
Soil moisture was obtained by measuring conductivity using a Delta-T Devices SM150T probe inserted into the topsoil. The raw data (conductivity) obtained was then converted to moisture content (Supplementary Material Equation 1). Participants took conductivity measurements in a radial pattern extending from the trunk of the study tree, which were averaged (n = 13) for the north and south sides, respectively.
Participants measured soil infiltration using a mini-disc infiltrometer (Decagon Devices, Inc). Both the upper chamber and lower water reservoir were filled with water, and the bottom elastomer with porous disk firmly replaced. The infiltrometer was placed on a flat surface with any vegetation removed to ensure good contact between the soil and infiltrometer. The suction was set to 1 cm and the water level read every 60 s (unless the infiltration rate was particularly fast, in which case participants recorded at shorter intervals of every 20–40 s). The initial volume of water was recorded followed by 10 additional readings (typically 10 min). The infiltration rate (Q) was calculated as follows (Equation 1):
where v is a multiplication factor to compensate for the frequency of readings taken (v = 3, 1.5, 1, and 0.5 for frequencies of 20, 40, 60, and 120 s, respectively), D is water depth in ml, and n is the number of readings taken.
In addition to the soil characteristics assessed by participants, local temperature, and precipitation data were obtained from the NOAA NCEP climate dataset (NOAA/OAR/ESRL PSL, https://psl.noaa.gov/). Hourly temperature and precipitation data were selected between 0600–1800 on each day of an event at the relevant location, and a daily average calculated.
Laboratory analyses of the soil samples collected by citizen scientists were conducted to assess volumetric water content at saturation, field capacity and sampling. This was used to help validate our citizen scientist collected measurements and eventual analysis of potential differences between sites.
Data Analysis
T-tests and Mann-Whitney tests were used to determine significance in the differences in means and medians of soil characteristics in areas with high and low infiltration and between the two land management types. ANOVAs and Kruskall-Wallis tests were used to compare differences in the measured soil characteristics between different city locations and seasons.
Logistic regression models were used to explore the relationship between infiltration rate and soil and local climate characteristics. Infiltration rate was transformed to a binomial value of low (<1.75 ml/min) or high infiltration rate (≥1.75 ml/min) as the dependent variable in the logistic models. This cut-off is the equivalent to a rainfall intensity of 6.6 mm/h, which qualifies as heavy rainfall according to the UK Met Office (McIntosh, 1963).
Independent variables (i.e., soil moisture, soil compaction, soil color, average daily temperature, low or high insolation, and soil texture) were included in the models following normalization. Soil texture was converted to an ordinal factor (Supplementary Table 1). All independent variables were standardized using a z score conversion (z = (x–μ)/σ) such that their different measurement scales were comparable in the model output. Data analysis was performed in R and using the RealStats extension in Microsoft Excel.
Results
Infiltration Rate
Infiltration rates varied according to location (Figure 1A) and the seasons (Figure 1A). Soils of the Chantilly park had the highest infiltration rates (mean infiltration rate 3.87 mL/min) compared to those in Birmingham (mean infiltration rate 3.29 mL/min) or London (mean infiltration rate 2.56 mL/min), (Welch t-test on log transformed infiltration rate: T = 26.2, df = 2, p < 0.001, Figure 1A). Summer infiltration (mean infiltration rate 5.29 mL/min) was higher than rates in spring (mean infiltration rate 2.03 mL/min) and autumn (average infiltration rate 2.40 mL/min), (Welch t-test on log transformed infiltration rate: T = 11.17, df = 2, p < 0.001, Figure 1B). Infiltration rates showed no significant difference between the managed and unmanaged sample sites (Welch t-test on log transformed infiltration rate: T = 0.23, df = 483, p = 0.8). Equally, when categorized as binary infiltration rates (high vs. low), these were also independent of management type (Fisher's exact test, two tails, p = 0.78).
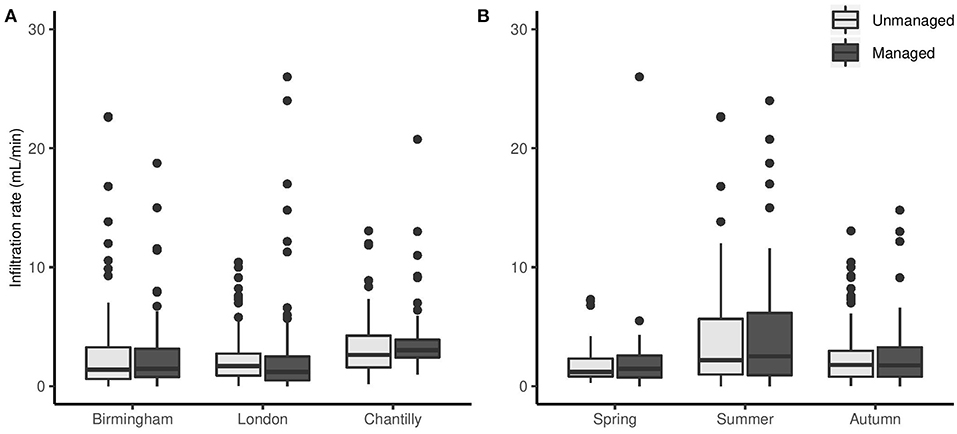
Figure 1. Boxplots of raw infiltration rate (mL/min) transformed to positive values, according to management regime (gray = unmanaged; black = managed), by (A) location of park where samples were taken, and (B) season. Central horizontal line in boxplot = median; box limits = first and third quartiles; whiskers = 1.5 times the interquartile range. Data beyond the whiskers plotted as outliers, with points >30 mL/min not shown.
Laboratory Analyses
The categorization of citizen scientist collected measurements of infiltration rate into either a low or high category was validated through laboratory analysis of soil samples. For the managed sites, volumetric water content at saturation showed significant differences between the low (mean: 54%) and high infiltration (mean: 52%) categories (Wilcox test = 3,868, p = 0.015). This was also confirmed in measurements of water content at field capacity (Wilcox = 4,025, p = 0.005, low infiltration mean: 53%, high infiltration mean: 51%), and sampling (T = 2.28, df = 157, p = 0.02, low infiltration water content at sampling mean: 27%, and high infiltration mean: 24%). For unmanaged sites, no significant difference was observed in the laboratory analyses of volumetric water content at saturation (T = −0.86, df = 144, p = 0.39), field capacity (Wilcox test = 2650.5, p = 0.89) or sampling (T = 0.21, df = 144, p = 0.83) between the low and high infiltration rate categories.
Differences in soil characteristics, as collected by citizen scientists, between the managed and unmanaged sites were also examined. Significant differences in soil color (T = 8.15, df = 426, p < 0.001), soil compaction (Wilcox test = 9,465, p < 0.001) and soil texture (X2 = 12.71, df = 1, p < 0.001) were found, with a higher soil color (i.e., darker) for unmanaged soil, higher compaction for managed soils and a higher soil texture (i.e., more aerated) assignment for unmanaged soils. Binary soil infiltration (Fisher's exact test, two tails, p = 0.78) and soil moisture (T = 0.37, df = 464, p = 0.7) did not show significant differences between land managements.
Logistic Regression Models
An initial binary logistic regression model aggregating both management regimes (managed and unmanaged sites) provided an estimate of high and low infiltration (X2 = 29.43, p < 0.001, n = 490; accuracy (percentage of correct predictions by the model) = 0.64, AUC ROC = 0.61). Initially, the model included footfall intensity as a ranked factor according to the observed level of foot traffic each location received. However, this factor was subsequently removed from the model (and subsequent separated managed and unmanaged models), as it was highly correlated with compaction (rs = 0.94, n = 6, p < 0.05), to avoid collinearity. Air temperature and soil moisture showed some correlation (r = −0.44, n = 488), although this was considered moderate (Schober et al., 2018); therefore, we retained both factors in the model as they could provide additional information.
Given the relatively low accuracy and specificity/selectivity of the aggregated model and the clear differences between soil characteristics of the managed and unmanaged sites, separate models were developed. The utility of simple observational data (managed vs. unmanaged) in citizen science has been well-documented elsewhere (Champion et al., 2018; Thornhill et al., 2018; Pohle et al., 2019).
Managed Model
The model for managed sites demonstrated increased accuracy compared to the aggregated model, with a specificity/selectivity indicating a fair model (Accuracy = 0.69, ROC AUC = 0.72) (Bradley, 1997; Fawcett, 2006). The model distinguished between high and low infiltration rates (χ2 = 38.08, p < 0.001, n = 255) based on the suite of measurements made by the citizen scientists (Figure 2).
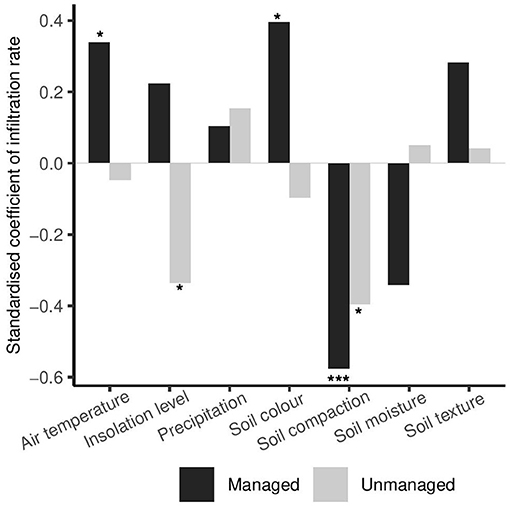
Figure 2. Standardized coefficients (z score: z = (x–μ)/σ) of binary infiltration rate in the managed (black) and unmanaged (gray) logistic regression models in response to each of the model's independent variables: average daily air temperature, location of sample site relative to level of insolation, average daily precipitation, soil color, soil compaction, average soil moisture, and soil texture. Positive standardized coefficients signify a positive relationship; negative coefficients denote a negative relationship between the independent variable and the likelihood of high infiltration rates (≥1.75 mL/min). Asterisks denote variables that were significant predictors of high infiltration rate in each model (*<0.05, ***<0.001; Table 1).
In particular, the measurements of soil color, soil compaction, and average air temperature were the most important for managed sites (Table 1, Figure 2). The model indicated that low soil compaction, high soil color (i.e., darker; related to organic matter content), and higher average air temperature were associated with high infiltration rates. Soil moisture and soil texture were not found to be significant factors (p = 0.06 for both parameters; Table 1), while their coefficients suggested that lower soil moisture and sandier soil textures were associated with higher infiltration at the managed sites (Table 1).
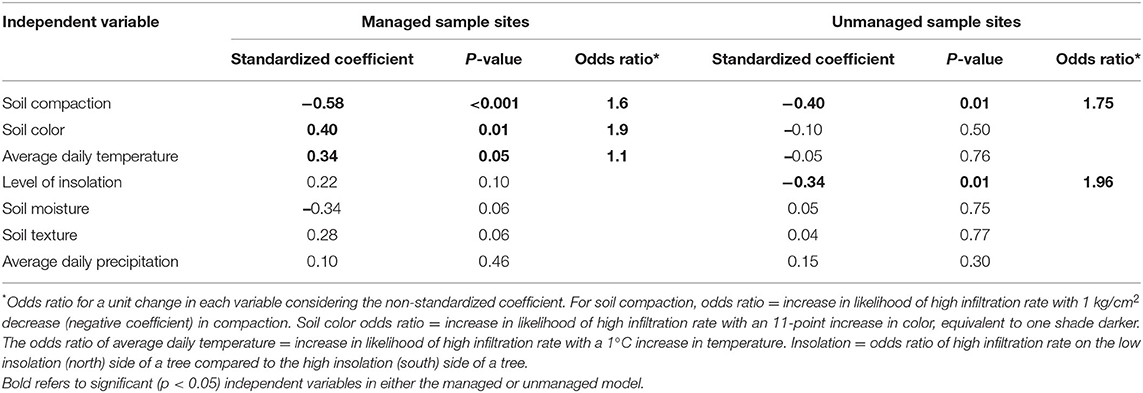
Table 1. Comparison of all variables in each of the logistic regression models, showing standardized coefficients (z score: z = (x–μ)/σ) for predicting high infiltration rate (≥1.75 mL/min) and their significance level.
Unmanaged Model
For unmanaged sites, the logistic model had lower-than-desired accuracy (Accuracy = 0.60, ROC AUC = 0.65), but remained significant (χ2 = 17.57, p < 0.01, n = 235) and had a higher AUC than that obtained in the aggregated model. In this model, soil compaction and location with respect to insolation were the most important variables (Table 1). Low soil compaction and sites with low insolation (i.e., samples to the north side of trees) were associated with high infiltration rates (Figure 2).
Soil Characteristics Influencing Binary Infiltration Rate
In both models, soil compaction was the most significant variable with the highest standardized coefficient (Table 1). As compaction was negatively correlated with infiltration rate, a 1 kg/cm2 decrease in soil compaction nearly doubled the odds that the site would have a high infiltration rate (1.6 and 1.75 times at managed and unmanaged sites, respectively), all else being equal (Table 1). Our measurements confirmed that soil was more compacted at the managed sample sites (Wilcox test = 9,465, p < 0.001, Figure 3A), a pattern maintained throughout seasons and across locations (Figures 3A,B).
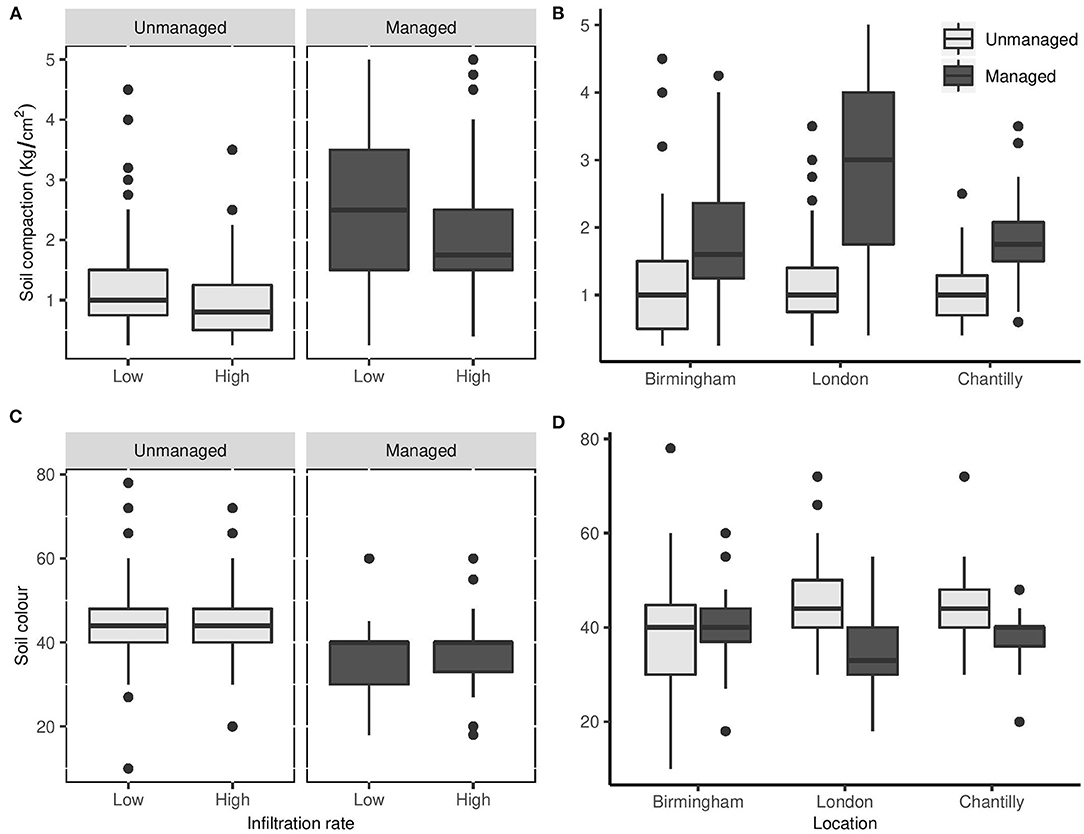
Figure 3. Soil compaction (kg/cm2) according to (A) management type: unmanaged (gray) and managed (black) in relation to infiltration rate categorized (binary) as low (<1.75 mL/min), or high (≥1.75 mL/min), (B) location of the park where sampling took place. Soil color (higher number = darker) in relation to (C) management type: unmanaged (gray) and managed (black) in relation to infiltration rate categorized (binary) as low (<1.75 ml/min) or high (≥1.75 ml/min), (D) location of the park where sampling took place. Boxplots as per Figure 2.
Soil color was a predictor of infiltration rate in the managed model only (Table 1), where darker soil was linked to high infiltration rates. Soil was generally darker at the unmanaged sites (T = 8.15, df = 426, p < 0.001, Figure 3C) and showed a higher variance overall (F = 1.87, df = 234, 254, p < 0.001). This pattern was most evident at the Chantilly and London sites, but not in Birmingham, where the discrepancy between management type was very small (Two-way ANOVA: F2 = 24.05, p < 0.001, Figure 3D). Soil color in the unmanaged samples was more heterogeneous and exhibited a greater change between seasons compared to the managed sites (Two-way ANOVA: F2 = 4.03, p < 0.02), where lighter colors were evident during the summer.
Daily air temperature was an important factor at the managed sites for predicting infiltration rate. The positive role of air temperature on infiltration was confirmed by the significant difference between managed sites classified as low and high infiltration rate (Wilcox = 6940.5, p = 0.04). There was no significant difference in air temperature comparing unmanaged sites with low and high infiltration (Wilcox = 7102.5, p = 0.7).
The degree of insolation, determined by the location of the sample site with respect to the tree canopy (north vs. south), was an important factor in the unmanaged sites (Figure 4). Southern samples were exposed to more solar radiation, as such the average daytime surface temperature of the southern sites was expected to be higher (Jim, 2015). For managed sites, there was a significant difference in air temperature between sites with different infiltration levels (Wilcox = 6940.5, p = 0.04); but these different infiltration levels were independent of the level of insolation (X2 = 1.69, df = 1, p = 1.9, Figure 4). On the other hand, at unmanaged sites high and low infiltration rate was dependent on the level of insolation (X2 = 5.96, df = 1, p = 0.01, Figure 4) but there was no difference in median air temperature (Wilcox = 7102.5, p = 0.7) between sites with different infiltration levels.
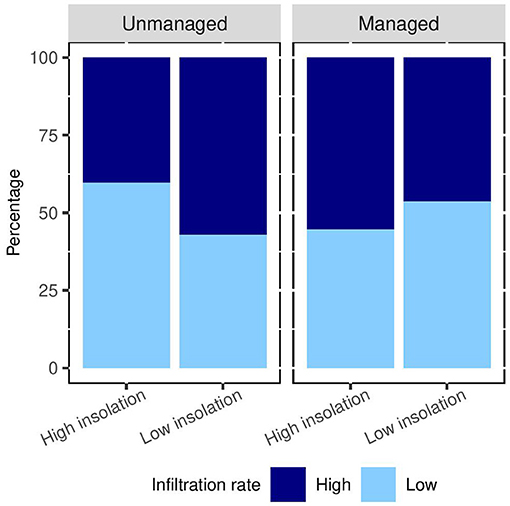
Figure 4. Percentage of samples falling in the low (light blue, <1.75 mL/min) or high (dark blue, ≥1.75 mL/min) infiltration rate categories on the low insolation (north) or high insolation (south) side of the sampled trees.
The model indicated that in unmanaged areas, soil samples that were exposed to less insolation were 1.9 times more likely to have high infiltration rates than those that were exposed to more insolation, all else being equal (Table 1; Figure 4). This was not associated with differences in organic content or soil moisture. In fact, low and high levels of insolation had no explanatory power at unmanaged sites in relation to soil color (Wilcox test = 7,363, p = 0.32), compaction (Wilcox = 6,419, p = 0.41), texture (Wilcox = 6,865, p = 0.97), or moisture (Wilcox = 6,754, p = 0.86).
Discussion
Our results indicate that, using easy to perform measurements (i.e., soil compaction, soil color, air temperature, and level of insolation), citizen scientists can categorize whether an urban green space containing trees (e.g., their garden or local park) had sufficient infiltration capacity to reduce the impact of a heavy rainfall event.
Soil Characteristics
Infiltration rates measured directly with mini-disk infiltrometers by citizen scientists showed significant variation based on the location and timing of sampling (Figure 1), attesting to the heterogeneous nature of urban environments and alluding to the complexity of using an infiltrometer with little prior experience. Soil conditions, location and sampling season of each site were important characteristics with respect to infiltration.
Less compacted soil equates to higher soil porosity and therefore higher infiltration rate (Pitt et al., 2008; Yang and Zhang, 2011; Elliot et al., 2018). Compacted soils have fewer voids that are essential to the movement of water, gases and plant roots, which together influence soil structure and therefore impact root growth and the efficiency of fertilizer, critical for a healthy soil. The more compact nature of the managed sites (Figure 3A) in our study could be related to the lack of leaf litter and their higher footfall and the application of machinery for vegetation and litter maintenance (Yang and Zhang, 2011; Elliot et al., 2018). This was particularly evident at the London managed site (Figure 3B), located in Kew Gardens, which experiences a high throughput of visitors, 2.36 million in 2018–19 (Kew Royal Botanic Gardens, 2019), leading to high soil compaction.
Darker soils are often associated with higher organic matter content (Fitzpatrick, 1986; Galvao and Vitorello, 1998). In many studies, soils with high organic matter content have a higher infiltration rate than soils with lower organic matter contents (Boyle et al., 1989; Chen et al., 2014; Wang et al., 2018); while other studies have found no influence (Phillips et al., 2019). Our citizen scientists identified a darker soil color in unmanaged sites compared to managed sites (Figure 3C), where in the latter organic leaf litter and clippings were regularly removed. However, darker soils were not a predictive factor of infiltration at unmanaged sites (Table 1, Figure 2). At managed sites that have low litter input, the color of the surface soil is more static (Tóth et al., 2007). Links between soil color, organic matter content, and infiltration are further influenced by a range of other variables including soil bulk density, soil texture, and root paths (Bartens et al., 2008), which are more complex to measure on a large scale.
Soil moisture and texture measured by citizen scientists had limited influence on predicting infiltration, and only at the managed sites. We had expected that soil moisture, in particular, would have played a more important role, as high antecedent soil moisture reduces infiltration rates (Tromble et al., 1974; Pitt et al., 2008). The comparison with the laboratory analyses tends to support this (mean water content at sampling for low infiltration rate was 27% and for high infiltration rate was 24%, T = 2.28, df = 157, p < 0.02). Soil texture trends were somewhat unclear, even though soils with high clay or silt content tend toward lower infiltration (Yang and Zhang, 2011). The uncertainty of the relationship between soil texture and infiltration may be related to the complexity of the citizen science soil texture method itself, rather than the parameter and should be analyzed separately.
The effect of the orientation of the soil samples with respect to the trees influenced infiltration in unmanaged areas. Typically, shaded soil exposed to less insolation has higher water content, carbon, nitrogen and phosphorus concentrations, compared to more exposed conditions (Akpo et al., 2005). This favors decomposition of available leaf litter, with positive impacts on infiltration (Anthelme and Dangles, 2012). In fact, an increased likelihood of high infiltration rate was found on the northern sides of trees, with lower insolation, in the unmanaged sites (Figure 4).
On the other hand, in the managed sites, overall air temperature played a stronger role on infiltration than level of insolation. One explanation for this could be the lower tree density in managed sites, as infiltration rate has been shown to be sensitive to temperature in some conditions (Jaynes, 1990). Meanwhile, the lack of influence of air temperature in the unmanaged sites could be the result of soil insulation by leaf litter (Xiong and Nilsson, 1997), although more detailed temperature measurements, specifically at the soil surface, would be needed for confirmation. Together this indicates the importance of fine-scale measurements, as microhabitat differences can influence soil conditions.
Citizen Scientists' Role
Based on these results, a classification flow diagram was built to allow citizen scientists to differentiate sites that have high or low infiltration potential (Figure 5). Construction of this classification flow diagram had two objectives: first, to allow citizens (and planners) to better understand the conditions of their local park or garden with respect to intense rain events; and secondly, to inform management actions to improve flood protection aspects of local green spaces. The classification flow diagram was built based on data from parks with trees (Tilia spp) in European temperate areas; thus, its application is defined to areas with similar climatic conditions, soil types and in proximity to similar tree types. Additional data could support the extension of our classification flow diagram for use at other locations in the future.
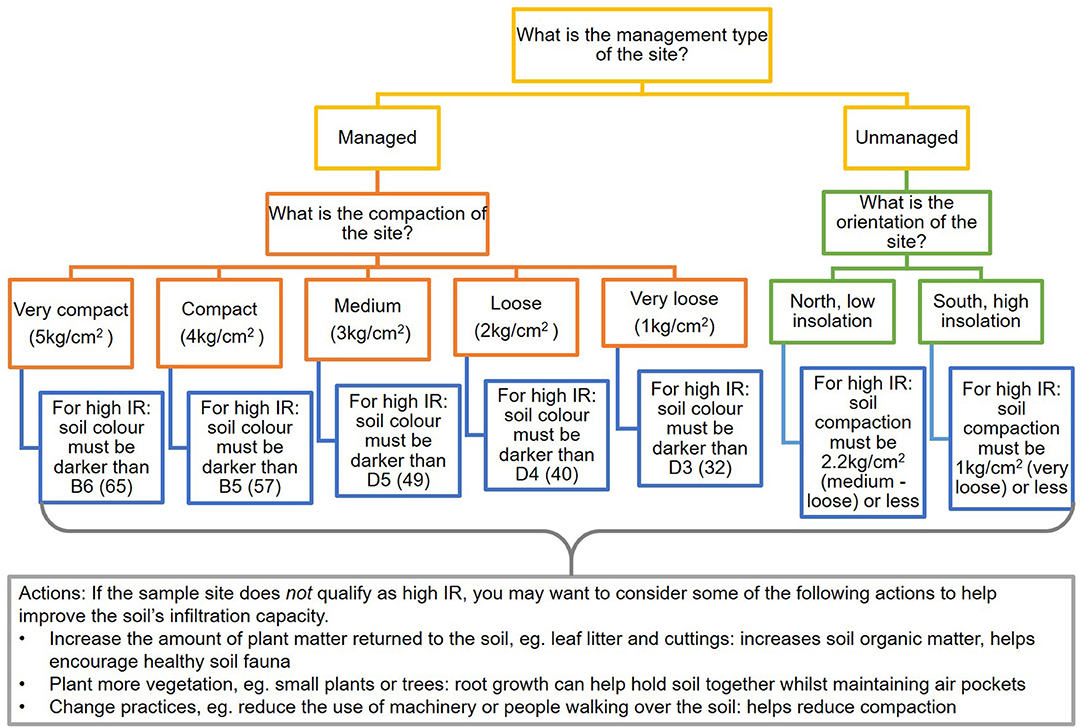
Figure 5. Classification flow diagram for citizen scientists to estimate the infiltration potential of their local urban green space, and suggested actions to take to improve infiltration potential if samples cannot be classified as high infiltration rate (Soil Association, 2016). IR = Infiltration rate.
The classification flow diagram begins by identifying the management type of the green space (managed sites: the majority of leaf litter and undergrowth vegetation cleared; vs. unmanaged sites: little to no human intervention, Supplementary Figure 1), and then by presenting the initial factor to be measured (compaction for managed; level of insolation for unmanaged). A threshold value of the second parameter was then defined to allow for the identification of high- and low-infiltration soils. For the managed sites, air temperature was also an important factor. The figures shown in this example diagram are specific to a site at 11°C, the annual mean temperature for both London and Paris (Climate-Data, 2020). The mean annual temperature for Birmingham is 9°C; therefore, the associated minimum colors required to estimate high infiltration rate are shown in Table 2.
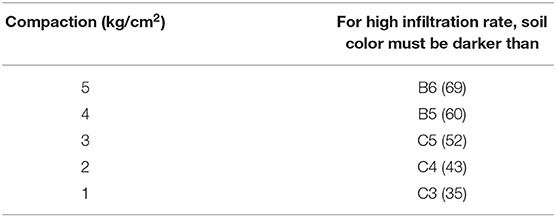
Table 2. Minimum soil colors for classifying high infiltration soils when sampling at 9°C, the mean annual temperature of Birmingham.
Data omitted for the initial building of the model because of their poor match were input into the classification flow diagram in a validation exercise. The classification flow diagram was able to predict high and low infiltration rate correctly in many cases (Table 3), despite the limited sample size (n = 7) and the input data being originally discounted due to their unsuitability for the defined management categories. The unmanaged branch of the flow diagram performed less well, resulting from the poorer-fitting unmanaged model, suggesting the influence of additional factors. More data would help to improve the applicability of the classification flow diagram to a wider range of locations and climates. However, particularly in the case of the managed sample sites, the classification flow diagram can immediately be utilized by citizen scientists in areas similar to those used here.
For example, a citizen scientist may be interested in measuring the infiltration capacity of the soil in their garden. As they might mow their lawn and clear the resulting litter, they would consider this site as managed, and thus follow the left-hand branch of the diagram. The citizen scientist would first estimate soil compaction. If the soil were loose (i.e., compaction <2 kg/cm2), the individual would next evaluate soil color. If soil color were then darker than D4 on the Munsell color chart, the site should be considered to have high infiltration rate. Repeat measurements (>3) in close proximity would allow for greater information on site variability. If sampling suggested that the soil had low infiltration capacity, the lower gray box in Figure 5 provides suggested actions to be taken to improve the infiltration capacity of the urban green space (Soil Association, 2016).
Measuring infiltration rate with an infiltrometer requires more training than is required to estimate soil color, compaction, tree orientation associated to level of insolation, and temperature measurements. Properly setting up and using an infiltrometer can be complex (Kosmala et al., 2016), requires calculations for interpretation post-hoc, and takes a minimum of 10 min per measurement. Conversely, measuring several different simple soil parameters to estimate soil infiltration indirectly is simpler and more engaging, offering a learning opportunity that encourages survey completion and commitment to repeated sampling (i.e., retention: Ryan et al., 2001). As an example, a site assessment with three repeat measurements could be completed in 20 min or less (Table 4). However, it is worth noting that compaction measurements, although simple in terms of methodology, typically requires the use of equipment which can be associated with a cost (in this study a Pocket Penetrometer was used, costing in the region of £90). Therefore, we suggest two options which could account for this. Firstly, a targeted sampling campaign in a particular area of interest liaised via a central authority or organization could loan equipment such as pocket penetrometers to citizen scientist groups, as the Drinkable Rivers project does for water quality (see https://drinkablerivers.org/). Compared to minidisc infiltrometers, penetrometers are easier to use, requiring minimal amounts of training, offering more robust results, less expensive and more easily shipped or posted to citizen scientists, making them suitable for this type of loan set-up. Alternatively, or as a complementary solution, citizen scientists could use simpler homemade penetrometers, such as from a knitting needle and spool of thread (Science Buddies Staff, 2020), a pointed stick or even using your finger (Davidson, 1965). Both options provide a low-cost means to test relative soil compaction by a citizen scientist.
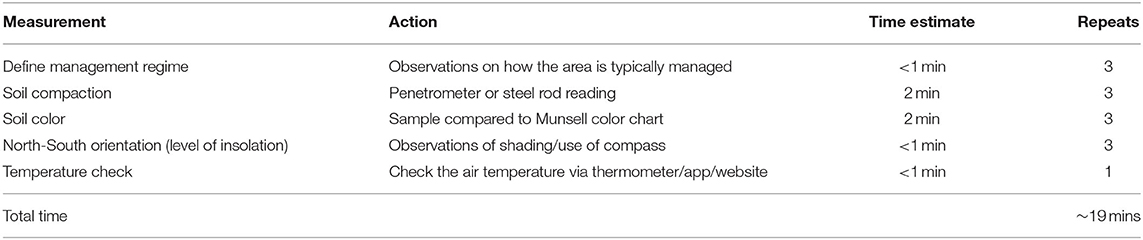
Table 4. Estimates of citizen scientist measurement times required to complete the classification flow diagram (Figure 5), assuming three repeat measurements within the sampled green space.
Furthermore, compaction and soil organic content data might indicate which factors are leading to low infiltration rate and thus provide opportunities for mitigation actions to improve infiltration, empowering the citizen scientist to take action based on their measurements. Citizen science has greatest impact when there is a potential call for action (van Noordwijk et al., 2021) and when that action is simple, engaging, and complies with local social norms (Rare The Behavioural Insights Team, 2019). The opportunity to modify local conditions to improve resilience to pluvial flooding represents a strong potential motivation for continued participation in this kind of citizen science activity.
While this study only looked at soil around trees in urban parks, 29% of the total urban land area in Great Britain is residential gardens (ONS, 2019). In some cities, up to 40% of green space is located on private property, where homeowners have direct control over soil and tree management (Davies et al., 2009; Gaston et al., 2013). Therefore, local knowledge regarding a neighborhood capacity to deal with heavy rain events lies within domestic gardens (Cameron et al., 2012). Participation in citizens' own gardens lends itself to ease of access, and to repeated measurements over time (Toms and Newson, 2006; Williams et al., 2016), throughout all seasons. Furthermore, homeowners and park managers have multiple options for improving soil compaction and organic matter content, including soil aeration, and changing use practices such as vegetation and leaf litter removal practices (Soil Association, 2016).
The information resulting from these measurements is coarse, yet it could enhance the efficient prioritization of resources to improve the utility of green spaces as NbS for flood protection. In particular, if in the future this method were to be used at scale, data collection input via a GIS based survey or app, it would allow for other valuable factors such geo-locating sample points to be easily captured. This would help to build a spatial picture of the flood mitigation potential of urban green spaces, thus achieving an overview of the overall risk of an area. The integration of citizen science-collected data into early warning systems for pluvial flooding is growing (See, 2019); therefore, datasets such as those presented here could, in the meantime, feed into and complement these resources.
Others have demonstrated the value of citizen scientists in collecting soil data over a large area (Bone et al., 2012). More generally, participating in citizen science empowers local residents as they are positively contributing to local decision-making and policy (McKineley et al., 2017). Moreover, citizens also have the potential to learn from the experience at a scale greater than their own survey spot, which translates to enhanced environmental awareness (Hobbs and White, 2012; Geoghegan et al., 2016).
Conclusions and Future Outlook
Considering the increasing importance of climate change on urban environments, the development of simple methods for citizen scientists to assess the capacity of their local green areas to mitigate pluvial flooding could provide valuable new knowledge to local planners, park managers and to the citizen scientists themselves. Through replication over a wide area and range of management types at a high frequency, an overview of the status of soil in terms of protection from pluvial flooding could be developed. This would allow for a better understanding of how different park and garden management regimes function under different climates. These data could be used to identify priority areas at risk of flooding and to help develop more complex models at validation sites. The participation of citizen scientists has additional benefits in being able to generate information from hitherto restricted private green space in domestic gardens, as well as reducing costs and labor inputs, and empowering communities to better manage their local environment.
To achieve this, future sampling should incorporate a greater number of sample sites to account for a greater geographical spread and represent different climatic regions and underlying soil types. Further sampling could also investigate soils from different types of green space, for example including residential gardens as well as urban parks, and consider the effect of different tree species on soil infiltration. The inclusion of greater numbers of citizen scientists would allow for these proposed increases in sampling effort, while reaching a wider audience, who could in turn benefit from the educational and engagement opportunities of citizen science.
Data Availability Statement
The raw data supporting the conclusions of this article will be made available by the authors, without undue reservation.
Author Contributions
MC, SL, and WB contributed to conception and design of the study. BP performed the statistical analysis and wrote the first draft of the manuscript. SL wrote sections of the manuscript. All authors contributed to manuscript revision, read, and approved the submitted version.
Funding
Funding was provided by HSBC under the HSBC Water Programme.
Conflict of Interest
The authors declare that the research was conducted in the absence of any commercial or financial relationships that could be construed as a potential conflict of interest.
Acknowledgments
We gratefully acknowledge the citizen scientists who participated in the Sustainable Training Programme (STP) and Sustainable Leadership Programme (SLP) events and collected data. We also acknowledge the park owners that allowed the data to be collected (Kew Gardens in London, Cannon Hill in Birmingham, and Les Fontaines in Chantilly, France) and their keepers and rangers for facilitating the process. To the scientists of our partner research institutes: Imperial College London (ICL), University of Reading, National Research Institute for Agriculture, Food and Environment, France (INRAE), and French National Centre for Scientific Research (CNRS), who trained and supported the citizen scientists; and the facilitators and co-ordinators who made the events possible. ICL who conducted laboratory analyses on soil samples collected during the events.
Supplementary Material
The Supplementary Material for this article can be found online at: https://www.frontiersin.org/articles/10.3389/frwa.2021.654493/full#supplementary-material
References
Akpo, L. E., Goudiaby, V. A., and Le Houerou, H. N. (2005). Tree shade effects on soils and environmental factors in a savannah of Senegal. West Afr. J. Appl. Ecol. 7:45647. doi: 10.4314/wajae.v7i1.45647
Alfieri, L., Dottori, F., Betts, R., Salamon, P., and Feyen, L. (2018). Multi-model projections of river flood risk in europe under global warming. Climate 6:6. doi: 10.3390/cli6010006
Anthelme, F., and Dangles, O. (2012). Plant-plant interactions in tropical alpine environments. Perspect. Plant Ecol. Evol. Syst. 14, 363–372. doi: 10.1016/j.ppees.2012.05.002
Bartens, J., Day, S. D., Harris, J. R., Dove, J. E., and Wynn, T. M. (2008). Can urban tree roots improve infiltration through compacted subsoils for stormwater management? J. Environ. Qual. 37, 2048–2057. doi: 10.2134/jeq2008.0117
Belt, J. J., and Krausman, P. R. (2012). Evaluating population estimates of mountain goats based on citizen science. Wildl. Soc. Bull. 36, 264–276. doi: 10.1002/wsb.139
Bone, J., Archer, M., Barraclough, D., Eggleton, P., Flight, D., Head, M., et al. (2012). Public participation in soil surveys: lessons from a pilot study in England. Environ. Sci. Technol. 46, 3687–3696. doi: 10.1021/es203880p
Bonney, R., Shirk, J. L., Phillips, T. B., Wiggins, A., Ballard, H. L., Miller-Rushing, A. J., et al. (2014). Next steps for citizen science. Science 343, 1436–1437. doi: 10.1126/science.1251554
Boyle, M., Frankenberger, W. T., and Stolzy, L. H. (1989). The influence of organic matter on soil aggregation and water infiltration. J. Product. Agric. 2, 290–299. doi: 10.2134/jpa1989.0290
Bradley, A. P. (1997). The use of the area under the ROC curve in the evaluation of machine learning algorithms. Pattern Recogn. 30, 1145–1159. doi: 10.1016/S0031-3203(96)00142-2
Cameron, R. W., Blanusa, T., Taylor, J. E., Salisbury, A., Halstead, A. J., Henricot, B., et al. (2012). The domestic garden – its contribution to urban green infrastructure. Urban Forest. Urban Green. 11, 129–137. doi: 10.1016/j.ufug.2012.01.002
Champion, C., Hobday, A. J., Tracey, S. R., and Pecl, G. T. (2018). Rapid shifts in distribution and high-latitude persistence of oceanographic habitat revealed using citizen science data from a climate change hotspot. Glob. Chang. Biol. 24, 5440–5453. doi: 10.1111/gcb.14398
Chen, Y. J., Day, S. D., Wick, A. F., and McGuire, K. J. (2014). Influence of urban land development and subsequent soil rehabilitation on soil aggregates, carbon and hydraulic conductivity. Sci. Tot. Environ. 494, 329–336. doi: 10.1016/j.scitotenv.2014.06.099
Climate-Data (2020). Climate: Europe. Available online at: https://en.climate-data.org/europe/ (accessed December 1, 2020).
Cunha, D. G. F., Magri, R. A. F., Tromboni, F., Ranieri, V. E. L., Fendrich, A. N., Campanhão, L. M. B., et al. (2019). Landscape patterns influence nutrient concentrations in aquatic systems: citizen science data from Brazil and Mexico. Freshwater Sci. 38, 365–378. doi: 10.1086/703396
Davidson, D. T. (1965). “Penetrometer measurements,” in Methods of Soil Analysis, Part 1. Physical and Mineralogical Properties, Including Statistics of Measurement and Sampling, ed C.A. Black (Madison, WI: American Society of Agronomy), 472–484. doi: 10.2134/agronmonogr9.1.c37
Davies, Z. A., Fuller, R., Loram, A., Irvine, K., Sims, V., et al. (2009). A national scale inventory of resource provision for biodiversity within domestic gardens. Biol. Conserv. 142, 761–771. doi: 10.1016/j.biocon.2008.12.016
Elliot, R. M., Adkins, E. R., Culligan, P. J., and Palmer, M. I. (2018). Stormwater infiltration capacity of street tree pits: quantifying the influence of different design and management strategies in New York City. Ecol. Eng. 111, 157–166. doi: 10.1016/j.ecoleng.2017.12.003
Elmqvist, T., Fragkias, M., Goodness, J., Güneralp, B., Marcotullio, P. J., McDonald, R. I., et al. (2013). Urbanisation, Biodiversity and Ecosystem Services: Challenges and Opportunities: A Global Assessment. Cham: Springer Nature, 755. doi: 10.1007/978-94-007-7088-1
Emilsson, T., and Ode Sang, Å. (2017). “Impacts of climate change on urban areas and nature-based solutions for adaptation,” in Nature-Based Solutions to Climate Change Adaptation in Urban Areas. Theory and Practice of Urban Sustainability Transitions, ed N. Kabisch, H. Korn, J. Stadler, and A. Bonn (Cham: Springer), 15–27. doi: 10.1007/978-3-319-56091-5_2
Fawcett, T. (2006). An introduction to ROC analysis. Pattern Recognit. Lett. 27, 861–874. doi: 10.1016/j.patrec.2005.10.010
Galvao, L., and Vitorello, I. (1998). Role of organic matter in obliterating the effects of iron on spectral reflectance and colour of Brazilian tropical soils. Int. J. Remote Sens. 19, 1969–1979. doi: 10.1080/014311698215090
Gaston, K. J., Avila-Jimenez, M. L., and Edmondson, J. L. (2013). Managing urban ecosystems for goods and services. J. Appl. Ecol. 50, 830–840. doi: 10.1111/1365-2664.12087
Geoghegan, H., Dyke, A., Pateman, R., West, S., and Everett, G. (2016). Understanding Motivations for Citizen Science. Final report on behalf of the UKEOF. University of Reading, Stockholm Environment Institute (University of York) and University of the West of England.
Hadj-Hammou, J., Loiselle, S., Ophof, D., and Thornhill, I. (2017). Getting the full picture: assessing the complementarity of citizen science and agency monitoring data. PLoS ONE 12:0188507. doi: 10.1371/journal.pone.0188507
Hobbs, S. J., and White, P. C. (2012). Motivations and barriers in relation to community participation in biodiversity recording. J. Nat. Conserv. 20, 364–373. doi: 10.1016/j.jnc.2012.08.002
Holck, M. H. (2007). Participatory forest monitoring: an assessment of the accuracy of simple cost–effective methods. Biodivers. Conserv. 17, 2023–2036. doi: 10.1007/s10531-007-9273-4
Horton, R. E. (1941). An approach towards a physical interpretation of infiltration-capacity. Soil Sci. Soc. Am. J. 5, 399–417. doi: 10.2136/sssaj1941.036159950005000C0075x
Jaynes, D. B. (1990). Temperature variations effect on field-measured infiltration. Soil Sci. Soc. Am. J. 54, 305–312. doi: 10.2136/sssaj1990.03615995005400020002x
Jim, C. Y. (2015). Thermal performance of climber greenwalls: effects of solar irradiance and orientation. Appl. Energy 154, 631–643. doi: 10.1016/j.apenergy.2015.05.077
Keesstra, S., Nunes, J., Novara, A., Finger, D., Avelar, D., Kalantari, Z., et al. (2018). The superior effect of nature based solutions in land management for enhancing ecosystem services. Sci. Total Environ. 610–611, 997–1009. doi: 10.1016/j.scitotenv.2017.08.077
Kendon, M., McCarthy, M., Jevrejeva, S., Matthews, A., and Legg, T. (2019). State of the UK Climate 2018. Int. J. Climatol. 39, 1–54. doi: 10.1002/joc.6213
Kew Royal Botanic Gardens (2019). Annual Reports and Accounts. UK: APS Group on behalf of the Controller of Her Majesty's Stationary Office.
Kosmala, M., Wiggins, A., Swanson, A., and Simmons, B. (2016). Assessing data quality in citizen science. Front. Ecol. Environ. 14:1436. doi: 10.1002/fee.1436
Lado, M., and Ben-Hur, M. (2004). Soil mineralogy effects on seal formation, runoff and soil loss. Appl. Clay Sci. 24, 209–224. doi: 10.1016/j.clay.2003.03.002
Li, X., Niu, J., and Xie, B. (2014). The effect of leaf litter cover on surface runoff and soil erosion in Northern China. PLoS ONE 9:e0107789. doi: 10.1371/journal.pone.0107789
McElrone, A. J., Choat, B., Gambetta, G. A., and Brodersen, C. R. (2013). Water uptake and transport in vascular plants. Nat. Educ. Knowled. 4:6. Available online at: https://www.nature.com/scitable/knowledge/library/water-uptake-and-transport-in-vascular-plants-103016037/
McKineley, D. C., Miller-Rushing, A. J., Ballard, H. L., Bonney, R., Brown, H., Cook-Patton, S. C., et al. (2017). Citizen science can improve conservation science, natural resource management, and environmental protection. Biol. Conserv. 208, 15–28. doi: 10.1016/j.biocon.2016.05.015
Miller, J. D., and Hutchins, M. (2017). The impacts of urbanisation and climate change on urban flooding and urban water quality: a review of the evidence concerning the United Kingdom. J. Hydrol. 12, 345–362. doi: 10.1016/j.ejrh.2017.06.006
ONS (2019). UK natural capital: urban accounts. Natural capital accounts containing information about green space in urban areas. Office for National Statistics. Available online at: https://www.ons.gov.uk/economy/environmentalaccounts/bulletins/uknaturalcapital/urbanaccounts (accessed January 13, 2021).
Pandeya, B., Uprety, M., Paul, J. D., Sharma, R. R., Dugar, S., and Buytaert, W. (2020). Mitigating flood risk using low-cost sensors and citizen science: a proof-of-concept study from western Nepal. J. Flood Risk Manage. 14:e12675. doi: 10.1111/jfr3.12675
Phillips, T. H., Baker, M. E., Lauter, K., Yesilonis, I., and Pavao-Zuckerman, M. A. (2019). The capacity of urban forest patches to infiltrate stormwater is influenced by soil physical properties and soil moisture. J. Environ. Manage. 246, 11–18. doi: 10.1016/j.jenvman.2019.05.127
Pitt, R., Chen, S.-E., Clark, S. E., Swenson, J., and Ong, C. K. (2008). Compaction's impacts on urban storm-water infiltration. J. Irrigat. Drain. Eng. 134, 652–658. doi: 10.1061/(ASCE)0733-9437(2008)134:5(652)
Pohle, I., Helliwell, R., Aube, C., Gibbs, S., Spencer, M., and Spezia, L. (2019). Citizen science evidence from the past century shows that Scottish rivers are warming. Sci. Total Environ. 659, 53–65. doi: 10.1016/j.scitotenv.2018.12.325
Rare and The Behavioural Insights Team (2019). Behavior Change For Nature: A Behavioral Science Toolkit for Practitioners. Arlington, VA: Rare.
Ryan, R. L., Kaplan, R., and Grese, R. E. (2001). Predicting volunteer commitment in environmental stewardship programmes. J. Environ. Plan. Manage. 44, 629–648. doi: 10.1080/09640560120079948
Schober, P., Boer, C., and Schwarte, L. A. (2018). Correlation coefficients: appropriate use and interpretation. Anaesth. Anal. 126, 1763–1768. doi: 10.1213/ANE.0000000000002864
Science Buddies Staff (2020). Soil Compaction. Available online at: https://www.sciencebuddies.org/science-fair-projects/project-ideas/geo_p010/geology/soil-compaction#summary (accessed March 3, 2021).
See, L. (2019). A review of citizen science and crowdsourcing in applications of pluvial flooding. Front. Earth Sci. 7:44. doi: 10.3389/feart.2019.00044
Soil Association (2016). Seven Ways to Save Our Soils. Available online at: https://www.soilassociation.org/media/4672/7-ways-to-save-our-soils-2016.pdf (accessed September 16, 2020).
Szabo, J. K., Vesk, P. A., Baxter, P. W. J., and Possingham, H. P. (2010). Regional avian species declines estimated from volunteer-collected long-term data using list length analysis. Ecol. Appl. 20, 2157–2169. doi: 10.1890/09-0877.1
Thornhill, I., Chautard, A., and Loiselle, S. (2018). Monitoring biological and chemical trends in temperate still waters using citizen science. Water 10:839. doi: 10.3390/w10070839
Thornhill, I., Loiselle, S., Lind, K., and Ophof, D. (2016). The citizen science opportunity for researchers and agencies. Bioscience 66, 720–721. doi: 10.1093/biosci/biw089
Toms, T. P., and Newson, S. E. (2006). Volunteer surveys as a means of inferring trends in garden mammal populations. Mamm. Rev. 36, 309–317. doi: 10.1111/j.1365-2907.2006.00094.x
Tóth, J. A., Lajtha, K., Kotroczó, Z., Krakomperger, Z., Caldwell, B., Bowden, R., et al. (2007). The effect of climate change on soil organic matter decomposition. Acta Silvatica Lignaria Hungarica 3, 75–85. Available online at: http://aslh.nyme.hu/fileadmin/dokumentumok/fmk/acta_silvatica/cikkek/Vol03-2007/06_toth_et_al_2007p.pdf
Tromble, J. M., Renard, K. G., and Thatcher, A. P. (1974). Infiltration for three rangeland soil-vegetation complexes. J. Range Manage. 27, 318–321. doi: 10.2307/3896834
United Nations (2019). World Urbanization Prospects: The 2018 Revision. New York, NY: United Nations.
van Noordwijk, C. G. E., Bishop, I., Staunton-Lamb, S., Oldfield, A., Loiselle, L., Geoghegan, H., et al. (2021). “Creating positive environmental impact through citizen science,” in The Science of Citizen Science, eds K. Vohland, A. Land, L. Ceccaroni, R. Lemmens, J. Perello, M. Ponti, et al. (Cham: Springer), 373–395. doi: 10.1007/978-3-030-58278-4_19
Wang, P., Zheng, H., Ren, Z., Zhang, D., Zhai, C., Mao, Z., et al. (2018). Effects of Urbanisation, soil property and vegetation configuration on soil infiltration of urban forest in Changchun, Northeast China. Chin. Geogr. Sci. 28, 482–494. doi: 10.1007/s11769-018-0953-7
Williams, R. L., Stafford, R., and Goodenough, A. E. (2016). Biodiversity in urban gardens: assessing the accuracy of citizen science data on garden hedgehogs. Urban Ecosyst. 18, 819–833. doi: 10.1007/s11252-014-0431-7
Xiong, S., and Nilsson, C. (1997). Dynamics of leaf litter accumulation and its effects on riparian vegetation: a review. Bot. Rev. 63, 240–261. doi: 10.1007/BF02857951
Yang, J.-L., and Zhang, G.-L. (2011). Water infiltration in urban soils and its effects. J. Soils Sediments 11, 751–761. doi: 10.1007/s11368-011-0356-1
Yolcubal, I., Brusseau, M. L., Artiola, J. F., Wierenga, P., and Wilson, L. G. (2004). “Environmental physical properties and processes,” in Environmental Monitoring and Characterisation, eds J. F. Artiola, I. L. Pepper, and M. L. Brusseau (Waltham, MA: Academic Press), 207–239. doi: 10.1016/B978-012064477-3/50014-X
Keywords: citizen science, nature-based solutions, infiltration rate, pluvial flooding, urban trees, green space
Citation: Pudifoot B, Cárdenas ML, Buytaert W, Paul JD, Narraway CL and Loiselle S (2021) When It Rains, It Pours: Integrating Citizen Science Methods to Understand Resilience of Urban Green Spaces. Front. Water 3:654493. doi: 10.3389/frwa.2021.654493
Received: 16 January 2021; Accepted: 16 March 2021;
Published: 15 April 2021.
Edited by:
Sven Schade, European Commission, ItalyReviewed by:
Federica Marando, Joint Research Centre, ItalyBeth Hall, Purdue University, United States
Copyright © 2021 Pudifoot, Cárdenas, Buytaert, Paul, Narraway and Loiselle. This is an open-access article distributed under the terms of the Creative Commons Attribution License (CC BY). The use, distribution or reproduction in other forums is permitted, provided the original author(s) and the copyright owner(s) are credited and that the original publication in this journal is cited, in accordance with accepted academic practice. No use, distribution or reproduction is permitted which does not comply with these terms.
*Correspondence: Bethany Pudifoot, YnB1ZGlmb290QGVhcnRod2F0Y2gub3JnLnVr