- Geography Department and Integrative Research Institute on Transformations of Human-Environment Systems (IRI THESys), Humboldt-Universität zu Berlin, Berlin, Germany
Against the background of a renewed interest in interdisciplinary water research, we begin this paper by diagnosing a need for deeper engagement at the epistemological and ontological level. We then analyse the ontological and epistemological commitments of three modeling examples: an academic human-flood model, a nutrient transfer decision support model and a policy facing water security model. These examples demonstrate how research practices are not neutral but intervene in the world by distributing agency unequally, providing naturalized and de-politicized explanations of the past and pre-configuring certain futures while foreclosing others. Lastly, we position hydrology's uncertainty tradition and its problematisation of choices in the research process as an entry point for reflexion on the contingencies of and ethical responsibility for research practices. This uncertainty tradition provides more common ground for collaboration between hydrologists and critical water researchers than previously acknowledged, while such collaboration would still thrive on confrontation. We conclude with a call for greater humility in water research, especially when using models, and practical suggestions for how researchers could uncover ontological and epistemological commitments and live up to the ethical responsibility they entail.
Introduction
Interdisciplinary collaborations in water research are not new, but a renewed interest has been instigated by the Scientific Decade 2013–2022 of the International Association of Hydrological Sciences (IAHS) on Change in Hydrology and Society—“Panta Rhei” (Montanari et al., 2013; McMillan et al., 2016). The key to successful collaboration is often seen in achieving an understanding of the priorities, approaches and concepts of the respective research fields (Carr et al., 2020). While such understanding is undoubtedly important, in this paper we argue that a deeper engagement with ontological and epistemological tensions will strengthen interdisciplinary collaboration (Krueger et al., 2016; Wesselink et al., 2017). Ontology and epistemology are important for understanding and shaping how research practices intervene in the world and with what consequences. With the term ontology we refer to theory of how the world is—or is becoming (Mol, 2002; Barad, 2007). With the term epistemology we refer to underlying assumptions about the nature of knowledge and appropriate ways of attaining or making that knowledge, which manifest in research methodologies. In this paper, we put an emphasis on models and the ontological and epistemological commitments they entail. Models are interesting because they are frequently focal points of interdisciplinary collaboration or controversy. They also bridge to the uncertainty tradition in hydrology and allow us to bring in philosophical discussions from this field (e.g., Beven, 2002, 2004, 2009; Baveye, 2004; Nearing et al., 2016, 2020). Our arguments, however, extend beyond models and apply to interdisciplinary and disciplinary research alike.
In water research, we see the ontological and epistemological fault line to lie between “generalising” traditions on the one hand and “interpretive” traditions on the other hand, not between social and natural science per se (Krueger et al., 2016). Different research traditions fall between these poles. They differ in the extent to which they consider abstraction from concrete observer situations to rules is possible (Figure 1). Generalizing traditions think underlying rules of the world or human behavior exist. Research seeks to uncover these rules, often quantitatively. With time, research is supposed to come closer to “the truth.” In this view, the researcher stands separate from the research object. Hydrology, economics and quantitative sociology have been historically more on the generalizing side. This makes economists, for example, “much more likely bed fellows for hydrologists than more qualitative social scientists” (Hall, 2019: 1777). Hall, however, foregrounds a quantitative-qualitative schism that for us does not go to the philosophical heart of the matter.
Interpretive traditions eschew general laws, theories or models. Instead, they interpret particularities that have led to events, often through case studies. Research focusses on everyday practices and how people give meaning to events and actions. In this view, the positionality of the researcher is part and parcel of method and interpretation. Anthropology, human geography and political science have been historically more on the interpretive side. Many water scholars working in these traditions self-identify as critical water researchers. “Critical” here refers to “critical theory” which foregrounds the contingency and plurality of knowledges, the role of power and differentiated vulnerabilities and access to water, and how these are reified by discursive and methodological framings (Wesselink et al., 2017; Rusca and Di Baldassarre, 2019; Venot et al., 2022). Critical theory is also interested in ontological questions related to water. More specifically, it is interested in the multiplicity of waters and relations with and around water, not only as different perceptions and ways of knowing water but as different ways of being-with-water (Linton, 2010; Yates et al., 2017).
Venot et al. (2022:1) summarize the troubles interpretive traditions have with generalizing traditions: “[Critical water] Researchers resist and challenge the pressures of commensuration and universalization (still commonly attached to much water-related science) because they have learned how the hegemony of some forms of knowledge has eclipsed or even violently erased others, in the process also disqualifying their bearers and allowing or justifying water dispossession.” This quote points to the higher status, and hence political power, that generalizing traditions in water research have historically enjoyed in society and science compared to interpretative traditions (Lave, 2016; Wesselink et al., 2017). Hence, much is at stake in trying to reconcile generalizing and interpretive traditions when social and natural sciences of water are supposed to work together. In particular, there is a risk that the social sciences are assigned a service role subordinate to the natural sciences (McMillan et al., 2016). For example, much of what anthropologists or human geographers hold dear in rich, place-based accounts of human-water relations gets lost when these accounts are simplified into variables or parameters of a model that seeks to generalize (Mostert, 2018). This subordination can happen in inter-personal collaborations as well as when hydrologists simply help themselves to social science methods and thereby uproot these methods from their ontological and epistemological foundations.
Wesselink et al. (2017) are optimistic about the role of narratives as a point of departure for collaboration because “the conscious reduction of rich narratives,” they argue, “surely has to be preferable to the automatic and a priori selection of knowledge from only those social science research areas that are compatible with quantitative modeling” (Wesselink et al., 2017:11, italics theirs). While this argument is clear as far as narratives as precursors for building socio-hydrological models are concerned, it remains unclear what benefits interpretive social sciences would get out of this mode of collaboration. Rusca and Di Baldassarre (2019) also challenge the alleged incompatibility between quantitative and qualitative research, which they loosely equate with generalizing and interpretive traditions. The authors argue that it is exactly the power of quantitative analysis that can be harnessed for critical water research. This resonates with arguments for mixed (quantitative-qualitative) methods by Massuel et al. (2018), for example. The arguments of Rusca and Di Baldassarre (2019) for the benefits of socio-hydrological modeling for interpretive water research, however, require greater scrutiny. We would argue that these benefits might be realized provided that underlying ontological and epistemological commitments are explicitly examined.
In the remainder of this paper, we analyse the ontological and epistemological commitments of three examples of interdisciplinary water research involving models (section Ontological and epistemological commitments in modeling water). We demonstrate how modeling practices intervene in the world and with what consequences. Even if we focus on modeling, our results speak to any research, modeling or otherwise, be it interdisciplinary or disciplinary. We then position hydrology's uncertainty tradition as an entry point for reflexion, arguing that uncertainty considerations have made hydrologists unwittingly more interpretivist than they might think (section Uncertainty as an entry point for reflexion). A similar point has been made in passing by Rusca and Di Baldassarre (2019). We conclude with a call for re-politicizing the debate around modeling and for greater humility in using models in the face of the ethical responsibility that ontological and epistemological commitments entail (section Conclusion). We suggest ways of questioning researchers' own commitments and how other disciplines might help in this task.
Ontological and epistemological commitments in modeling water
Melsen et al. (2018) argue that (socio-)hydrological models embed which processes are and which are not considered important, which scales and which uncertainties matter, and hence what the models can do, for whom and for what purposes. A groundwater model, for example, might be used to set abstraction limits which have differentiated economic effects on water users (Sanz et al., 2019). Flood risk models determine investments in flood risk management that also benefit some people more than others (Lane et al., 2011). Lane (2014) describes a flood risk model that excludes urban development as a risk factor, effectively creating a world where regulation automatically prevents urban development where it might increase flood risk.
These are cases that can be described with Hacking (1999) as the world in the model “looping back” onto the world “out there” through the discourses and interventions afforded by the model1. A discourse after Foucault (2002) constrains what can be thought, said and done; when, where and by whom2. From the perspective of Science and Technology Studies (STS), what knowledge practices do in the world can be cast as an ontological question, following an understanding of the world not as pre-existing but as becoming. At a fundamental level, Barad (2007) develops this philosophical position as “agential realism” based on quantum physics. Interventions and discourses are also the ontological dimension that Mol (2002) describes with “enactment,” though her field of study were clinical practices. Through the practices of building and using models, certain worlds are enacted and not others.
These positions neither presume the world to be purely socially constructed, nor do they consider the world to exist completely external to human observers. Rather, humans and knowledge practices are conceptualized as intrinsic parts of the world. Knowledge practices have as much to do with the world researchers seek to make sense of as with the same researchers being part of that world. Crucially, following Mol (2002), Barad (2007) and others, knowledge practices entail an ethical responsibility toward the worlds enacted through those practices simply because methodologies are not externally given but involve choices (explicit or implicit). Since these choices matter, Lave (2016: 79) asks us “to choose the dynamics we allow to influence our work with careful attention to our broader intellectual, ecological, and political commitments. If we do not, others' commitments and priorities will continue to set our agendas.” Wesselink et al. (2017) discuss similar ethical questions under “axiology”—how and for what one values research. We now dissect three modeling examples to query their ontological and epistemological commitments and hence the worlds they enact.
Socio-hydrological models: Predictive tools or … what exactly?
We first turn to socio-hydrological models because they have been suggested as focal points for collaboration between more generalizing and more interpretive water research traditions (Wesselink et al., 2017; Rusca and Di Baldassarre, 2019). They have also been points of controversy. Clarifying the ontological and epistemological commitments of these models is important because they reveal what the collaborating researchers accept or relinquish in terms of their own philosophical positions, and hence what they might get out of the collaboration. The collaboration might turn on the epistemological question of whether socio-hydrological models are considered predictive tools (Srinivasan et al., 2017), or, if not, what their epistemological status is exactly. To discuss this question, we use different applications of the human-flood model by Di Baldassarre et al. (2013) and its more recent variant by Di Baldassarre et al. (2015) (Figure 2). We chose this model for its iconic status as the first model in the new field of socio-hydrology and because it has seen several applications that can be analyzed. The model was designed to represent the “levee effect,” according to which flood protection by levees leads to a false sense of security and precarious development of the floodplain so that the exposure to floods is actually increased once flooding does overtop the levees.
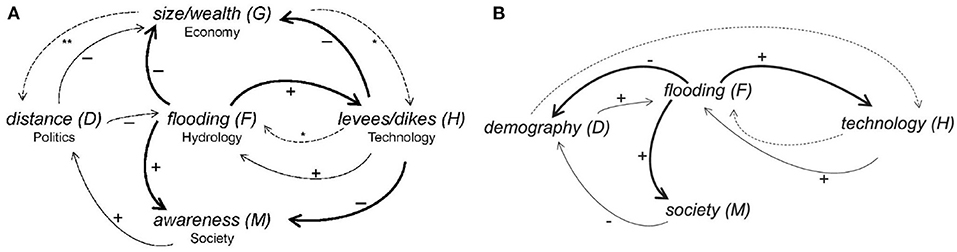
Figure 2. Descriptions of the socio-hydrological human-flood models of (A) Di Baldassarre et al. (2013) and (B) Di Baldassarre et al. (2015) (reproduced with permission).
From reading Di Baldassarre et al. (2013) we take that, among the five components in Figure 2A, hydrology and technology follow the realist ontology of engineering hydrology. Economy follows the neo-classical ontology of the benefit maximizing agent, though here approximating the economy with settlement size. Politics, in turn, is reduced ontologically to distance of settlements from the river. And society is reduced ontologically to awareness of flood disasters. The centrality of benefit maximization could be questioned, but here we focus on politics and society, which are obviously about much more than what is depicted in this model (as recognized by Di Baldassarre et al., 2013). Missing are at the very least peoples' differentiated exposure, vulnerability, response and adaptive capacity in relation to floods (Thaler, 2021). In the model variant of Di Baldassarre et al. (2015) (Figure 2B), the economy and politics components are merged into one—demography (in the flood plain)—which is what settlement size had been proxied with all along.
When parameterising the politics and society components, help might be sought from political and other social scientists. They might disagree, however, that the two components can be known through quantitative modeling (Wesselink et al., 2017). This is where the function of the model becomes decisive. If the function is prediction, then those political and social scientists might oppose the model (Troy et al., 2015). If the function is not prediction, then what is it (epistemologically)? Viglione et al. (2014: 11) offer the model as “a basis for discussion among researchers from different disciplines such as natural and social sciences.” This is more of a heuristic function, which entails an interesting shift in alliances for the hydrologists: the aforementioned political and social scientists would probably find the model as a heuristic more agreeable (Wesselink et al., 2017), while economists—perhaps providing the foundations of the economy component—might find this too weak an ambition.
The predictive ambition would also sit better with the behavioral psychologists whose concepts of risk cultures are brought into the model by Viglione et al. (2014). In this version of the model, collective memory is parameterised by memory loss rate, risk-taking attitude is parameterised by distance at which people would accept to live when they remember past floods, and trust is parameterised by proportion of psychological shock after flooding if levees are raised. Other social scientists, again, would find the treatment of these concepts too simplistic. Ridolfi et al. (2020), however, go even further by modeling ideal types of environmental risk perception and management stemming from cultural theory of risk. Despite acknowledging the simplifying assumptions of their version of the model, the authors offer another epistemological function of socio-hydrological models: testing social science theories empirically and thus contributing to social science as well as hydrology. However, no actual test is done in their study. And were it to be done, one needed to be clear about exactly what was being tested because only the four ideal types follow cultural theory of risk, not the underlying model structure.
The papers discussed so far dissociate themselves from prediction. However, they still find the model to “reproduce the typical patterns observed in many deltas and floodplain systems around the world” and “aim to test the validity of our assumptions by further exploring the socio-hydrology of floodplain systems” (Di Baldassarre et al., 2013: 3301). Viglione et al. (2014: 80) are happy to transfer lessons to “the real world.” Ridolfi et al. (2020: 14, italics ours) epitomize this predicament when they state on the one hand, “[a]n ideal type of a society is not a representation of reality, it is an analytical tool used to investigate differences between societies in their purest form,” and on the other hand, “ideal types can be used to construct theories, but they can also provide an explanation and interpretation of reality.”
Di Baldassarre et al. (2015) introduce the notion of stylized models after the notion of stylized facts from economics, meaning broad tendencies. The authors position their version of the model as a tool to explore plausible futures, contribute to theory development and inform empirical research (data to be collected). The lessons put forward for flood risk management, however, are rather circular: “flood-poor periods should also be of serious concern, particularly for societies relying on flood protection structures, as they tend to lower the memory of flooding and therefore increase societal vulnerability” (Di Baldassarre et al., 2015: 4779)—these are merely the assumptions put into the model in the first place.
Recent papers have begun to evaluate the model against data on flood-risk dynamics, which necessitates the operationalisation of the stylized variables in the model in terms of quantities that can be measured. Ciullo et al. (2017), for their Bangladesh case study, operationalise flood magnitude (W) with flooded area, flood damage (F) with economic loss, and floodplain population density (D) with Gross Domestic Product (GDP) (see Figure 2B for letters). For their Rome case study, the authors operationalise W with high-water level, D with population density, and flood protection level (H) with levee height.
The objective function for evaluation remains elusive when Ciullo et al. (2017: 884) note that “stylized models are not meant to fit the data as they aim to capture the essential dynamics and provide insights into possible behaviors of the overall system. Still, our aim is to diagnose the credibility of this modeling framework by evaluating the underlying conceptualization using real-world dynamics.” This connection to the “real world” enables lessons to be drawn for policy makers, but these are, again, rather circular. Sawada and Hanazaki (2020: 4778, 4787, italics ours), using Figure 2A, are more explicit in their objective function using a data assimilation setup, and are thus firmly in the business of “accurately reconstruct[ing] the historical human–flood interactions” and “predicting the future changes in sociohydrological processes.” The problem of measuring social awareness is relegated to the problem of formulating a measurement error model as if the concept of social awareness were a classical hydrological variable.
We conclude from the example of the human-flood model that a variety of epistemological commitments have been made by socio-hydrological models, ranging from predicting social-hydrological systems, over testing social science as well as hydrological theories, to heuristics for making sense of human-water relations. These commitments are not always clearly stated, but each affords specific collaborations with other natural and social scientists while troubling others. What we have paid little attention to so far are the ontological commitments of these models—the worlds they enact. These are equally, if not more, important. Thaler (2021) demonstrates how the parameterisation of the human-flood model (referring to the version in Figure 2B) entails assumptions about households' socio-economic status (affecting preparedness, level of physical damage and capacity to adapt) and its distribution on the floodplain. The model also entails commitments to particular social justice policies, including principles for distributing risk burdens. The model thus pre-configures certain futures while foreclosing others, a point we discuss with the next example.
Pre-configuring futures
Between 2008 and 2013 the first author was involved in several projects of participatory catchment management in the UK (Smith et al., 2015; Krueger et al., 2016). In these projects, decision support models were developed to inform stakeholder deliberations of the scale of aquatic pollution, respective responsibilities and possible mitigation strategies. There was thus an immediate policy relevance to these models. This will make it easier to illustrate how the world in the model loops back onto the world “out there” through direct interventions (or at least the planning thereof in the timeframes of the projects). We stress, however, that this looping effect works also in a less visible way with any model, be it motivated by fundamental or applied science, through the discourses embedded in and supported by the model. Whenever reference is made to the model (explicitly or implicitly), only certain things and not others can be thought, said and done, by different people, at different times and in different places. It is in this way—if not through direct intervention—that the model pre-configures (ontologically) certain futures while foreclosing others.
One of the aforementioned catchment management projects involved modeling nutrient transfers from land to water and their fate in the water courses. This task was accomplished with an export coefficient type model (Figure 3), calibrated with water quality data along the river network (Krueger, 2017). Initially, this model included the effects of sewage treatment works, household septic tanks, land use and livestock on nutrient transfer, as is common practice for these kinds of models. But the model did not include land management effects, only as a static background effect3. For the hydrologist involved, there was a good epistemological reason for neglecting land management effects because little data existed (from experiments or else) with which to parameterise these effects. A commitment to model parsimony (see section Uncertainty in hydrology) demanded not to overload the model with unconstrained parameters.
At one of the stakeholder meetings, however, members of the farming community stood up and rejected this model as a sensible basis for deliberating possible interventions. Land use were the last thing they would change. They would much rather like to see modeled the effects of less intrusive land management changes. After some controversy, which we do not retell here, the stakeholders eventually agreed on a model that included land management explicitly. Even if this meant that the range of effects of different land management practices could only be bracketed broadly following a government manual (Newell Price et al., 2011). A compromise on model parsimony was found by eliciting from the farming community and implementing in the model those practices they would consider taking up first instead of implementing all possible land management practices. The new model, too, will be incomplete in different ways. But it is compatible with the values brought to the table, including the epistemological values of the hydrologist, in a way that was acceptable to all stakeholders involved.
Our point with this example is that models pre-configure futures by enabling certain aspects to be manipulated in the world of the model and not others. In the example, the world in the model responded to land use changes but not to land management changes, thereby foreclosing futures where aquatic pollution is tackled by interventions that are less intrusive for the farm business. There was a good epistemological reason for doing this, at least for the hydrologist involved. Those farmers, by contrast, understandably demanded that less intrusive futures should remain on the table, which required a change to the model and a new compromise on model parsimony. Pre-configuring futures has a lot to do with agency: who can act in the world of the model and how (and maybe has a responsibility for doing so as in the case of pollution), and who cannot act and is thereby excluded from shaping the future. In the example's decision support setting the link to the world “out there” via the planning of interventions is easy to see, but we stress again that this looping effect works generally by way of discourses. We now turn to another policy relevant example.
The world in a model
Our last example is the water security model of Dadson et al. (2017), which gains policy relevance from being featured in the Report of the Global Water Partnership/OECD Task Force on Water Security and Sustainable Growth “Securing Water, Sustaining Growth” (Sadoff et al., 2015). The model simulates at the scale of nation-states the interaction of economic growth and investment in water-related infrastructure. The latter generates economic returns through productive investments while also increasing exposure to economic losses from water-related hazards that, in turn, are mitigated through protective investments (Figure 4A).
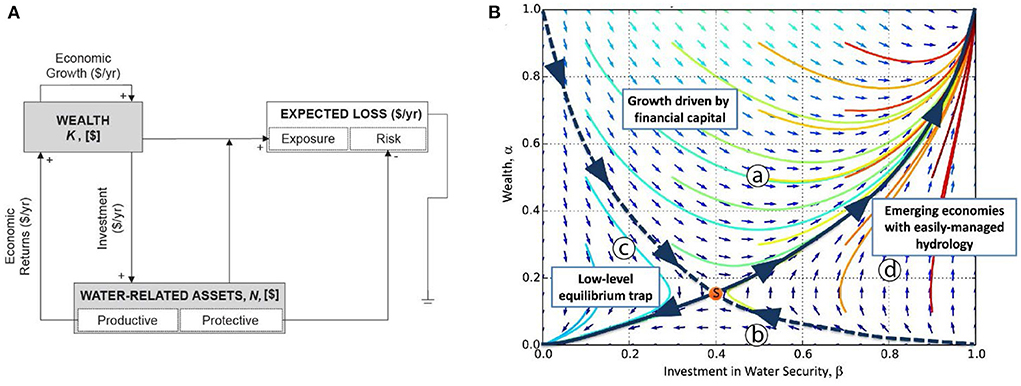
Figure 4. (A) Description of the water security model of Dadson et al. (2017) and (B) a resultant phase space (reproduced with permission).
The model is built on the premises that both an inadequate, unreliable supply of water and water-related hazards limit economic growth, and that infrastructure contributes to growth (Dadson et al., 2017). The model structure reflects the following assumptions: (i) a nation-state will invest in water-related infrastructure until the marginal investment cost balances the marginal benefits to productive sectors plus the marginal benefits from reducing water-related risks (diminishing marginal returns); (ii) growth occurs by capital accumulation and is proportional to the amount of existing capital.
Looking at a phase space of the model (Figure 4B), the authors equate the horizontal axis not only with investment in water-related infrastructure but also with a nation-state's “natural, hydrological endowment” (Dadson et al., 2017: 6426)4. This “endowment” gives water-rich countries a head start, so to speak, on their path to water security that reduces the need for investment in water-related infrastructure. The vertical axis represents general economic development. In this phase space, the authors identify two fixed points which are attractors: one at the bottom-left, called “poverty trap,” and on at the top-right, called “water security5.” In the two basins of attraction, where any combination of initial conditions leads to a trajectory toward the respective fixed point, the authors distinguish four realms (marked by letters a-d in Figure 4B).
Realm “a” is occupied by wealthy nation-states that can buffer any lack in “natural hydrological endowment” with financial capital, even if momentary declines of the economy have to be endured. Sadoff et al. (2015) equate this situation with the western USA in the early 20th century, e.g., the Colorado River basin (Dadson et al., 2017), and Israel in the mid-20th century. For realm “b” the authors see no correspondence in reality. These would be poor nation-states where no investment in water-related infrastructure would generate economic development, perhaps because large-scale commercial agriculture is not viable. In realm “c” the authors see nation-states whose “natural hydrological endowment” is so low that, despite the existence of financial capital, economic losses from water-related hazards eventually overwhelm the economy. Sadoff et al. (2015) see this situation in contemporary Niger and Chad. Dadson et al. (2017) see this situation in the Pakistani part of the Indus River basin. In realm “d” then are nation-states blessed by “easily-managed hydrology,” which can engage in unmitigated economic growth. This growth, however, generates infrastructure exposed to water-related hazards, which eventually require protective investments. Examples are the eastern USA in the mid-19th century and north-western European countries in the mid-18th century, e.g., in the Rhine River basin (Sadoff et al., 2015; Dadson et al., 2017).
The world “out there” is thus literally mapped onto the world in the model (a phase space). Economic growth and investment in water-related infrastructure provide at once naturalized explanations of the past as well as convenient paths to the future. The distribution of agency in those pasts and futures is again crucial. Especially disturbing is that some nation-states should find themselves poor or rich (some even “trapped”) purely as a result of “natural” hydrological causes. Not only does this narrative neglect all other causes—chiefly colonial history, especially in the real-world analogs identified by Sadoff et al. (2015)—it also assigns those countries no agency to change their situation (Loftus, 2015).
The policy recommendations that the authors derive from the model then are all too familiar. These are measures to shift a nation-state to the right in Figure 4B: municipal piped distribution systems, multi-purpose reservoirs, improved household sanitation and wastewater treatment (Dadson et al., 2017), water-saving technologies and desalination (Sadoff et al., 2015), complemented with insurance and reinsurance contracts and catastrophe bonds, and investments in human capital and institutions to manage infrastructure and allocate water (Dadson et al., 2017). Zeitoun et al. (2016) summarize the critique of such top-down interventions that hark back to the supply-side logic of large infrastructure projects of the past: large-scale infrastructure has hidden social, ecological and economic costs, might not fit with existing micro-scale infrastructure and is too inflexible to adapt to future conditions—hence gives a false sense of water security. Moreover, such top-down, often centralized initiatives reproduce colonial relations and reify technocratic approaches to water governance that hardly take into account local practices and uneven power relations (Alba et al., 2022).
The model also supports measures to shift a nation-state up in Figure 4B through the inflow of foreign capital, e.g., following the discovery of natural (mineral) resources (Sadoff et al., 2015; Dadson et al., 2017). Because such measures depend on external actors, they take even more agency away from state governments and local communities, often shifting the focus toward addressing international (neoliberal) agendas rather than local concerns. A notable example is the influence of Structural Adjustment Programs on institutional reforms and infrastructural investments in Sub-Saharan Africa (Alba and Bolding, 2016). What is more, if external investors are to be attracted by mineral resources, then this fuels colonial and violent extractivist logics (Shapiro and McNeish, 2021). Nevertheless, for Sadoff et al. (2015) and Dadson et al. (2017) an optimal investment programme is a mix of broad-based stimulation of national economic growth combined with directed investments in water infrastructure, institutions and information systems. This is simply the world one sees when economic growth and investment in water-related infrastructure are the only lenses to look at water security. The world in the model and the world “out there” blend through the kinds of historical explanations and policy recommendations attached to the model as one world lends support to the other. The function of the model in the Global Water Partnership/OECD report gives this issue all the more political charge.
Uncertainty as an entry point for reflexion
Having established the importance of ontological and epistemological commitments in water research involving models, which applies to interdisciplinary as well as disciplinary research with or without models, we now position the concept of uncertainty as an entry point for reflexion and collaboration. We query what hydrology's long-standing uncertainty practices tell us about the hydrological community's own thinking about the nature of the world and what can be known about it. In doing so we will challenge the historically generalizing outlook of hydrology and find hydrological practices to be closer to interpretive research traditions than is typically acknowledged.
Uncertainty in hydrology
Hydrology has a track-record in problematizing uncertainty in data and models. The uncertainty problem may be broken down into data uncertainty, model structural uncertainty and calibration uncertainty, which are now summarized in turn from a hydrological perspective. “Data uncertainty” is due to measurement error (often involving different component errors), the need for interpolation of measurements in space and time, and the scale dependence of measurements. The latter necessitates consideration of what instantaneous measurements at one location tell us about larger conceptual units of analysis such as spatial grid cells or catchments but also longer periods of time (McMillan et al., 2018). Nearing et al. (2016) challenge the notion of measurement error by arguing that measurement devices simply provide information and the potential for error lies not in this information per se but in the translation of the information to a conception of the physical system under study6. This translation might be called the “measurement model,” which would also include any interpolation or scaling.
“Model structural uncertainty” stems from the fact that more than one set of equations (model structure) is available to describe a hydrological system under study (Gupta et al., 2012). Several model structures correspond similarly well with the hydrological data at hand for that system, but none perfectly. This proliferation of model structures is due to the existence of multiple theories of hydrological processes, sometimes tied to specific spatial and temporal scales, as well as multiple translations of any one theory into operational models. Models built most faithfully from first principles are also the most complex and hence most plagued by the lack and scaling of data to parameterise, drive and test these models. At the same time, simpler models, ranging from statistical models to simplified physics-based models, whose complexity is tailored to the data and modeling purpose at hand, have a history of performing well for many hydrological tasks. Hence, “model parsimony” is an important consideration for hydrologists (compare example in section The world in a model). A parsimonious model is as complex as necessary but as simple as possible while maintaining some correspondence with hydrological theory.
Lastly, “calibration uncertainty” refers to the existence of several combinations of model parameter values that are more or less plausible. Models generally require calibration against independent data of some quantities the model predicts, e.g., runoff data in the case of a model predicting runoff from rainfall. The need for calibration arises because not every parameter in a model can be measured. This is so in practice due to resource constraints but also in principle because parameters are “effective parameters” at the scale of spatial and temporal model resolution that do not correspond to anything measurable, at least not without scaling (Beven, 2009, chapter 1.5). Since calibration data, too, are limited in scope and in their correspondence with the scale of model prediction, and any model is more or less wrong to begin with, there is calibration uncertainty. This is the same kind of uncertainty encountered in statistical regression.
These uncertainty considerations and how they are dealt with in research practice suggest that the hydrological community does not believe in one true representation of reality that they will eventually get to, contrary to what hydrology as a generalizing tradition would suggest. In hydrology this is framed as an epistemological problem, while an independently existing reality (ontology) is taken for granted (largely implicitly). Beven (2002: 2479, italics ours) summarizes the epistemological problem adamantly: “[A] formal environmental model can only ever be an approximation to the perceptual model of the complex processes governing the response to some forcing. […] [P]laces are unique in their characteristics and boundary conditions and their uniqueness is inevitably to some extent unknowable. […] [T]here will then always be the possibility of equifinality in model representations of a system, in that many different structures or parameter sets may give simulations that are acceptable representations of the observations available.” We now look a little closer at each of these three points.
With “perceptual model” Beven (2002) means a systematized qualitative understanding of the system under study, while a “formal model” is a representation of that understanding in the form of mathematical equations. A model development process is thus outlined, whose end point is the “procedural model,” i.e., the implementation of the equations in a computer programme (details in Beven, 2009). Beven's argument is that the perceptual model is as “realistic”—corresponding to an independently existing reality—as current understanding allows. Each step in the model development process, however, involves reductions of complexity and approximations (including spatial and temporal discretisation) that make the models progressively less realistic—though they still retain the possibility of being approximately realistic. The first quote above—“can only ever be” (Beven, 2002: 2479)—suggests that these are fundamental epistemological limitations of quantitative hydrological theory that will never be resolved.
The second quote—“unknowable” (Beven, 2002: 2479)—points to equally fundamental limitations in applying the aforementioned theory to particular places. Beven (2002: 2467, italics ours) specifies: “Clearly, for many environmental systems, the perceived complexities are such that all the boundary conditions, auxiliary conditions and system characteristics cannot be knowable given current measurement technologies.” First of all, every place of model application, e.g., hydrological plot or catchment, is unique. This is an ontological argument, which is supported by the variability in hydrological responses between plots that may otherwise be considered replicates in an experimental setting (Seeger, 2007). Second, measurement capabilities are—and according to the quote above always will be—such that even foundational principles like conservation of mass and energy cannot be demonstrated unequivocally. This is an epistemological argument. The consequence is that models, when applied to a particular place, require calibration against limited data on boundary and auxiliary conditions. This ties them to context. Especially since the parameters calibrated are effective parameters at the scale of model discretisation, model parameterisations cannot be transferred easily between places.
The consequence of calibrating effective parameters of imperfect models with limited data leads to Beven's equifinality problem of several parameterisations—and indeed model structures—yielding simulations that fit the data at hand similarly well. The third of the initial quotes above—“always” (Beven, 2002: 2479)—suggests that this is again a fundamental epistemological issue. Beven (2009) recites all passages discussed here 7 years later, and the way we have seen his diagnosis being received in hydrological publications and at conferences ever since suggests that the hydrological community largely agrees. For example, Nearing et al. (2020: 1) consent that “most hydrologists recognize that no model, measurement, or prediction is true in a strict sense,” even if this recognition conflicts with the way those hydrologists use error distributions or standard methods for model evaluation due to a failure to explicate underlying epistemologies. Nearing et al. (2016: 1670) go as far as stating “we cannot arrive at generalities.”
Choices
In the absence of generalities, we do not need to go as far as relativism [Baveye, 2004, in response to Beven (2002)] to recognize that practicing hydrology in the face of uncertainty involves making choices. Baveye (2004) calls these choices “observer dependencies” that come with working at spatially and temporally heterogeneous observational scales. One of his examples is soil volumetric water content, for which different observers may consider different volumes of soil and thus may end up with different values for the same soil at the same location. Another issue is that measurements are often not replicable, either because they are intrusive, i.e., alter the system, or because replicates are infeasible for financial or other practical reasons. When it comes to choices in modeling, Beven (2004), in response to Baveye (2004), speaks of “operator dependencies”: The choice of model structure, including the selection of processes and boundary conditions and their representation; the choice of feasible values or prior distributions for effective parameters, including any calibration period, and allowing for scale, non-linearity and heterogeneity effects; the choice of data model in relation to available measurements and, again, associated scale and heterogeneity effects.
How modeling choices lead to different model outputs is well documented in hydrology. Recent examples include: Mendoza et al. (2016), who show that the choice of model structure, calibration data and objective function can affect how climate change impacts are portrayed; Melsen et al. (2019), who demonstrate that modeling choices can have a significant impact on the timing, volumes and extremes of floods and droughts; and Borona et al. (2021), who show the same for the choice of climate data product when calculating drought indices. There is a relatively recent movement in hydrology toward documenting modeling choices to increase the reproducibility of modeling studies, e.g., by following standardized workflows (Hutton et al., 2016).
Choices may be “subjective” (Baveye, 2004; Beven, 2004; Melsen et al., 2019)—in the sense of not-objective—but they are not arbitrary (Krueger et al., 2012). Choices follow certain logics of science as long as we recognize science as a socio-material practice (Lane, 2014; Krueger et al., 2016; Melsen et al., 2018). Hamilton et al. (2022) argue that usefulness, reliability and feasibility for a given context guide modeling choices. Lane (2014) traces the genealogy of Manning's formula of average open channel velocity through historical and contemporary papers and practitioner guidelines. Linton and Krueger (2020), also on the basis of papers, policy documents and guidelines, describe the contingencies in establishing the phosphorus standard of British rivers in the context of the EU Water Framework Directive. Sanz et al. (2019) self-document how choices were made during the development of a groundwater model. The important point is that these choices could be made differently and that they have consequences in the world. Choices help enact a certain world at the expense of others (Mol, 2002). We have discussed three examples in section Ontological and epistemological commitments in modeling water. Since choices have consequences, scientists have an ethical responsibility for the choices they make (Barad, 2007). We elaborate this responsibility in section Conclusion.
When we talk of choices, we do not mean only choices that are made in a conscious effort. Most of the time, we would argue, the choices are rather “ways of doing things” that are learned during one's upbringing as a researcher in a particular scientific tradition. Addor and Melsen (2019) show based on a bibliometric study that the choice of model structure in hydrology follows a legacy effect. Research groups tend to favor one particular model over others due to practicality, convenience, experience and habit (compare Babel et al., 2019). The authors explain this effect by the communities of practice that have formed around these models, often initially through training and support initiatives, which help to ease model application. Other reasons given are a siloed and fragmented mode of model development in hydrology and a certain resistance to change in general. Both these reasons are arguably favored by the current system of academic competition. Melsen (2022) probed the motivations for modeling choices further by interviewing hydrological modelers. In her results, reasons to do with the research team were dominant next to individual reasons. Previous experience in the team was the single most prevalent reason for modeling choices that was cited, whereby the reliance on the team decreased with career stage. Melsen interprets these findings with an alignment of modeling philosophies at the recruitment stage of young researchers.
The importance of organizational attachment in shaping choices in hydrology is corroborated by the survey by Horton et al. (2022). The authors also find adequacy of model choice for a problem at hand (e.g., landscape features, perceived processes) to be important, on top of technical reasons like code availability and computational constraints. We find the resurgence of model adequacy important here—as a way of the “nature” being modeled making itself known alongside the aforementioned social factors. The world “kicking back” in the words of Barad (2007). In the same way that models are not pure representations of nature, they are not purely social constructs (as foregrounded by Melsen, 2022) either. Not forgetting technicalities, Lane (2014: 942, italics ours) summarizes: “the hydrological knowledge that results from this practice cannot be understood if it is divorced from the networks within which it is produced, that is, an assemblage of elements that are material (e.g., conservation of fluid mass, flood defenses), technical (e.g., state of knowledge, computational power), regulatory (e.g., defined modeling procedures) and human (e.g., ability to improvise, perception).”
Common ground with interpretive social sciences
We suggest that the uncertainty considerations discussed in the previous section have made hydrologists unwittingly more interpretivist than they might think (Figure 5). The notion of equifinality—that an observed phenomenon can be explained by several model structures and parameterisations—suggests the absence of general laws, theories or models. The uniqueness of place requires hydrologists to interpret particularities. Every application of a model is effectively a case study. And the need for choices puts the focus on how these choices are made in practice, including the positionality of the researcher. This said, hydrologists talking of uncertainty suggests a clinging on to the remnants of positivism in hydrology as a generalizing tradition. For something or someone to be uncertain there must be the idea of certainty, at least as an ideal state. But from realizing uncertainty it seems a small step to deeper reflexions on just these philosophical underpinnings (as indeed was the case for Beven, 2002, 2004, 2009; Baveye, 2004; Nearing et al., 2016, 2020). Meanwhile, for interpretivist social scientists the concept of uncertainty might be enough to recognize common ground as a basis for articulating differences and still work together.
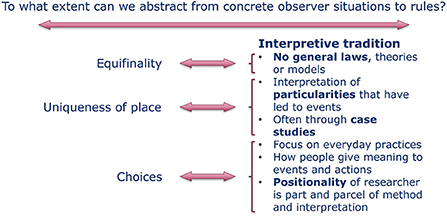
Figure 5. Common ground between uncertainty considerations in hydrology and the interpretive scientific tradition (own elaboration).
Rusca and Di Baldassarre (2019) come to a similar conclusion, but via integration of different research methods and outputs that are considered complementary, whereby ontological and epistemological differences are bracketed. This seems to work for the cases the authors cite, but when integration requires one to relinquish one's position on how (if at all) to generalize from the particular then we suggest that an agonistic mode of collaboration is more fruitful (Krueger et al., 2016). Agonism here means using the confrontation of ontological and epistemological positions as a point of reflexion on how one's methods and corresponding outputs are situated in a specific history, context and scientific tradition. We here refer to the concept of situated knowledges of Haraway (1988), which brings not only the partiality and political charge of methods and outputs into view but also holds the potential for doing research differently.
Conclusion
Uncertainty considerations in hydrology provide much common ground with the interpretive social sciences. Realizing this is a useful entry point for clarifying ontological and epistemological commonalities and differences between social and natural science researchers and re-considering alliances toward a collaboration at eye level. We have illustrated such an entry point with the iconic example of the socio-hydrological human-flood model. Clarifying ontological and epistemological commitments then provides a point of reflexion on how knowledge practices intervene in the world, as we have shown with our own case of the nutrient transfer model. From there follows a critique of all too simple world making practices, which we exercised with the example of the water security model from the Global Water Partnership/OECD report.
Knowledge practices are not externally given but involve choices. These choices matter as they enact a world that could be different. Whether these choices are made explicitly or are inscribed in research traditions, researchers have an ethical responsibility toward the worlds they enact (Mol, 2002; Barad, 2007). This responsibility breaks with the traditional idea of the neutrality of science. In fact, it is the very image of the neutral scientist that often naturalizes those choices and obscures their political charge (Knappe et al., 2019). Living up to this responsibility, we believe it is crucial to interrogate what concepts, data and models do in the world, and importantly what they leave out. For example: Who has and has not got agency in a model? Which actions are possible and which are foreclosed? Which understandings of water are included and which are not? A structured way into such a reflexion would be to look out for the junctures in a research process. For example, when building a model, where are things done in a way that could be different, e.g., using a particular model structure. Researchers can then ask what compels them to do things a certain way and what consequences it would have to do them differently. One might ask for example what a differently gendered world in the model would look like (Packett et al., 2020). Or one might explicitly contrast different ontological commitments in a research project (Venot and Jensen, 2022). Constructing counter-factuals like these is a powerful tool for exploring the contingencies of research practices.
However, finding the junctures in a research process that make a difference requires unpacking a thick layer of entrenched practices and power structures that are too often taken for granted by research traditions. Interviews with modelers, for example, will then only probe the surface as they will elicit rationalized interpretations of the research process and any choices made. A deeper analysis would require ethnographic methods like participant observation—“following modelers around” in laboratory studies tradition. This mode of engagement, in turn, requires domain knowledge, for instance knowledge of computing languages and modeling tools. This is one reason why we believe hydrologists should collaborate more with interpretive social scientists and vice versa: to create opportunities to question knowledge practices jointly. Finding junctures in a research process also implies taking seriously the existence of multiple water ontologies and their politics (Yates et al., 2017; Venot and Jensen, 2022). An intervention of indigenous actors, for example, would help unsettle dominant Western worldviews. The agonistic engagement of different ontologies and epistemologies can be a catalyst for conducting research that is more aware of and hopefully more caring toward its world making potential.
Jasanoff (2021: 6) argues that there are “unresolved divisions about the forms of representation and deliberation that are suited to making judgments about the collective good in distant and unknown futures.” We expect that these controversies will come to light when engaging in the kinds of reflexions outlined in this paper. A re-politicization of this debate, e.g., through critical transdisciplinary science (Krueger et al., 2016; Knappe et al., 2019), seems urgently needed. In the face of the crisis of techno-managerial interventions and the science that underpins them, Jasanoff (2021) asks for more humble ways of knowing. Grounded in ethics, politics and law, such knowledge practices should prepare for a radical reimagining of social-ecological relations that is arguably needed to face the challenges of our time. “Humility,” Jasanoff (2007: 33) says, “instructs us to think harder about how to reframe problems so that their ethical dimensions are brought to light, which new facts to seek and when to resist asking science for clarification.” The last part is important: We should not be afraid to resist certain knowledge practices—such as certain types of models—when they enact a world that we do not wish to live in.
Data availability statement
The original contributions presented in the study are included in the article/supplementary material, further inquiries can be directed to the corresponding author.
Author contributions
All authors listed have made a substantial, direct, and intellectual contribution to the work and approved it for publication.
Funding
This work has benefitted from funding by the Volkswagen Foundation through grant no. 96955 (Water Security for Whom?). The article processing charge was funded by the Deutsche Forschungsgemeinschaft (DFG, German Research Foundation)−491192747 and the Open Access Publication Fund of Humboldt-Universität zu Berlin.
Acknowledgments
Our arguments have been sharpened in numerous discussions with Krystin Unverzagt, Anja Klein and Joerg Niewoehner (all IRI THESys). Four anonymous reviews helped in focussing the article.
Conflict of interest
The authors declare that the research was conducted in the absence of any commercial or financial relationships that could be construed as a potential conflict of interest.
Publisher's note
All claims expressed in this article are solely those of the authors and do not necessarily represent those of their affiliated organizations, or those of the publisher, the editors and the reviewers. Any product that may be evaluated in this article, or claim that may be made by its manufacturer, is not guaranteed or endorsed by the publisher.
Footnotes
1. ^We distinguish the “world in the model” from the “world out there” that humans have some sensory experience of and interact with while being part of that world. The world in the model and the world “out there” are made to align in various ways that we analyse in this paper. We use the singular “world” here even though a model affords enactments of different worlds, e.g. at different stages of model conception, development and use. Similarly, as the scholarship on ontologies of water reminds us, there are multiple ontologies of the world constantly coexisting, sometimes more cooperatively and sometimes more conflictual. From this perspective, the plural “worlds” might be more apt.
2. ^We note that Ian Hacking, who coined the term “looping effects,” has hesitated to apply these effects to the material world. He has applied it to people. People respond to the way they are “known,” e.g. through medical diagnosis, and this changes (ontologically) their way to be a person. We nevertheless consider looping a useful metaphor of how knowledge practices intervene in the world but feel compelled to qualify that this is done via discourse as well as direct intervention by, again, people.
3. ^Land use in this context refers to agricultural crops or other vegetation types grown in the catchment, while land management refers to all aspects of agricultural or environmental management of that land use, such as tillage, fertilization, buffer strips etc.
4. ^A phase space of a system dynamics model is a space spanned by the model variables [in our example economic growth (“wealth”) and investment in water-related infrastructure (“investment in water security”)]. All possible states of the system (combinations of values of the variables) are therefore represented in this phase space. One can trace possible trajectories of the system through a phase space as done in Figure 4B. Different choices of model parameters lead to different phase spaces.
5. ^An attractor in a phase space is a portion of the space that the system tends to evolve toward given certain starting conditions. Attractors can have multiple geometric shapes; in our example they are fixed points. In general, not all fixed points are attractors.
6. ^Nearing et al. (2016: 1668) define information as “the property of a signal [e.g. a data stream] that effects a change in our state of belief about some hypothesis [e.g. coded in form of a model]”.
References
Addor, N., and Melsen, L. A. (2019). Legacy, rather than adequacy, drives the selection of hydrological models. Water Resour. Res. 55, 378–390. doi: 10.1029/2018WR022958
Alba, R., and Bolding, A. (2016). IWRM avant la lettre? Four key episodes in the policy articulation of IWRM in downstream Mozambique. Water Alternat. 9, 549–568. doi: 10.2307/j.ctvh8r2qk.12
Alba, R., Kooy, M., and Bruns, A. (2022). Conflicts, cooperation and experimentation: analysing the politics of urban water through Accra's heterogeneous water supply infrastructure. Environ. Plan. E Nat. Space 5, 250–271. doi: 10.1177/2514848620975342
Babel, L., Vinck, D., and Karssenberg, D. (2019). Decision-making in model construction: unveiling habits. Environ. Model. Softw. 120, 104490. doi: 10.1016/j.envsoft.2019.07.015
Barad, K. (2007). Meeting the Universe Halfway: Quantum Physics and the Entanglement of Matter and Meaning. Durham: Duke University Press. doi: 10.2307/j.ctv12101zq
Baveye, P. (2004). The emergence of a new kind of relativism in environmental modelling: a commentary. Proc. R. Soc. Lond. Ser. A Math. Phys. Eng. Sci. 460, 2141–2146. doi: 10.1098/rspa.2003.1256
Beven, K. (2002). Towards a coherent philosophy for modelling the environment. Proc. R. Soc. Lond. Ser. A Math. Phys. Eng. Sci. 458, 2465–2484. doi: 10.1098/rspa.2002.0986
Beven, K. (2004). Reply to “The emergence of a new kind of relativism in environmental modelling: a commentary” by Philippe Baveye. Proc. R. Soc. Lond. Ser. A Math. Phys. Eng. Sci. 460, 2147–2151. doi: 10.1098/rspa.2003.1257
Borona, P., Busch, F., Krueger, T., and Rufin, P. (2021). Uncertainty in drought identification due to data choices, and the value of triangulation. Water 13, 3611. doi: 10.3390/w13243611
Carr, G., Barendrecht, M. H., Debevec, L., Kuil, L., and Blöschl, G. (2020). People and water: understanding integrated systems needs integrated approaches. J. Water Supply Res. Technol. Aqua 69, 819–832. doi: 10.2166/aqua.2020.055
Ciullo, A., Viglione, A., Castellarin, A., Crisci, M., and Di Baldassarre, G. (2017). Socio-hydrological modelling of flood-risk dynamics: comparing the resilience of green and technological systems. Hydrol. Sci. J. 62, 880–891. doi: 10.1080/02626667.2016.1273527
Dadson, S., Hall, J. W., Garrick, D., Sadoff, C., Grey, D., and Whittington, D. (2017). Water security, risk, and economic growth: insights from a dynamical systems model. Water Resour. Res. 53, 6425–6438. doi: 10.1002/2017WR020640
Di Baldassarre, G., Viglione, A., Carr, G., Kuil, L., Salinas, J. L., and Blöschl, G. (2013). Socio-hydrology: conceptualising human-flood interactions. Hydrol. Earth Syst. Sci. 17, 3295–3303. doi: 10.5194/hess-17-3295-2013
Di Baldassarre, G., Viglione, A., Carr, G., Kuil, L., Yan, K., Brandimarte, L., et al. (2015). Debates—perspectives on socio-hydrology: capturing feedbacks between physical and social processes. Water Resour. Res. 51, 4770–4781. doi: 10.1002/2014WR016416
Gupta, H. V., Clark, M. P., Vrugt, J. A., Abramowitz, G., and Ye, M. (2012). Towards a comprehensive assessment of model structural adequacy. Water Resour. Res. 48, 11044. doi: 10.1029/2011WR011044
Hall, J. W. (2019). Socio-hydrology in perspective—Circa 2018. Water Resour. Res. 55, 1776–1777. doi: 10.1029/2019WR024870
Hamilton, S. H., Pollino, C. A., Stratford, D. S., Fu, B., and Jakeman, A. J. (2022). Fit-for-purpose environmental modeling: targeting the intersection of usability, reliability and feasibility. Environ. Model. Softw. 148, 105278. doi: 10.1016/j.envsoft.2021.105278
Haraway, D. (1988). Situated knowledges: the science question in feminism and the privilege of partial perspective. Femin. Stud. 14, 575–599. doi: 10.2307/3178066
Horton, P., Schaefli, B., and Kauzlaric, M. (2022). Why do we have so many different hydrological models? A review based on the case of Switzerland. WIREs Water 9, e1574. doi: 10.1002/wat2.1574
Hutton, C., Wagener, T., Freer, J., Han, D., Duffy, C., and Arheimer, B. (2016). Most computational hydrology is not reproducible, so is it really science? Water Resour. Res. 52, 7548–7555. doi: 10.1002/2016WR019285
Jasanoff, S. (2021). Humility in the anthropocene. Globalizations 18, 839–853. doi: 10.1080/14747731.2020.1859743
Knappe, H., Holfelder, A.-K., Löw Beer, D., and Nanz, P. (2019). The politics of making and unmaking (sustainable) futures: introduction to the special feature. Sustain. Sci. 14, 891–898. doi: 10.1007/s11625-019-00704-w
Krueger, T. (2017). Bayesian inference of uncertainty in freshwater quality caused by low-resolution monitoring. Water Res. 115, 138–148. doi: 10.1016/j.watres.2017.02.061
Krueger, T., Maynard, C., Carr, G., Bruns, A., Mueller, E. N., and Lane, S. (2016). A transdisciplinary account of water research. Wiley Interdiscip. Rev. Water 3, 369–389. doi: 10.1002/wat2.1132
Krueger, T., Page, T., Hubacek, K., Smith, L., and Hiscock, K. (2012). The role of expert opinion in environmental modelling. Environ. Model. Softw. 36, 4–18. doi: 10.1016/j.envsoft.2012.01.011
Lane, S. N. (2014). Acting, predicting and intervening in a socio-hydrological world. Hydrol. Earth Syst. Sci. 18, 927–952. doi: 10.5194/hess-18-927-2014
Lane, S. N., Landstroem, C., and Whatmore, S. J. (2011). Imagining flood futures: risk assessment and management in practice. Philos. Trans. R. Soc. A Math. Phys. Eng. Sci. 369, 1784–1806. doi: 10.1098/rsta.2010.0346
Lave, R. (2016). Stream restoration and the surprisingly social dynamics of science. WIREs Water 3, 75–81. doi: 10.1002/wat2.1115
Linton, J., and Krueger, T. (2020). The ontological fallacy of the water framework directive: implications and alternatives. Water Altern. 13, 513–533. Available online at: https://www.water-alternatives.org/index.php/alldoc/articles/volume-13/issue-3-1/591-a13-3-9
Loftus, A. (2015). Water (in)security: securing the right to water. Geogr. J. 181, 350–356. doi: 10.1111/geoj.12079
Massuel, S., Riaux, J., Molle, F., Kuper, M., Ogilvie, A., Collard, A.-L., et al. (2018). Inspiring a broader socio-hydrological negotiation approach with interdisciplinary field-based experience. Water Resour. Res. 54, 2510–2522. doi: 10.1002/2017WR021691
McMillan, H., Montanari, A., Cudennec, C., Savenjie, H., Kreibich, H., Krueger, T., et al. (2016). Panta Rhei 2013–2015: global perspectives on hydrology, society and change. Hydrol. Sci. J. 61, 1174–1191. doi: 10.1080/02626667.2016.1159308
McMillan, H. K., Westerberg, I. K., and Krueger, T. (2018). Hydrological data uncertainty and its implications. Wiley Interdiscip. Rev. Water 5, e1319. doi: 10.1002/wat2.1319
Melsen, L. A. (2022). It takes a village to run a model—the social practices of hydrological modeling. Water Resour. Res. 58, e2021WR030600. doi: 10.1029/2021WR030600
Melsen, L. A., Teuling, A. J., Torfs, P. J. J. F., Zappa, M., Mizukami, N., Mendoza, P. A., et al. (2019). Subjective modeling decisions can significantly impact the simulation of flood and drought events. J. Hydrol. 568, 1093–1104. doi: 10.1016/j.jhydrol.2018.11.046
Melsen, L. A., Vos, J., and Boelens, R. (2018). What is the role of the model in socio-hydrology? Discussion of “Prediction in a socio-hydrological world”. Hydrol. Sci. J. 63, 1435–1443. doi: 10.1080/02626667.2018.1499025
Mendoza, P. A., Clark, M. P., Mizukami, N., Gutmann, E. D., Arnold, J. R., Brekke, L. D., et al. (2016). How do hydrologic modeling decisions affect the portrayal of climate change impacts? Hydrol. Process. 30, 1071–1095. doi: 10.1002/hyp.10684
Mol, A. (2002). The Body Multiple: Ontology in Medical Practice. Durham: Duke University Press. doi: 10.1215/9780822384151
Montanari, A., Young, G., Savenije, H. H. G., Hughes, D., Wagener, T., Ren, L. L., et al. (2013). “Panta Rhei—everything flows”: change in hydrology and society—the IAHS scientific decade 2013–2022. Hydrol. Sci. J. 58, 1256–1275. doi: 10.1080/02626667.2013.809088
Mostert, E. (2018). An alternative approach for socio-hydrology: case study research. Hydrol. Earth Syst. Sci. 22, 317–329. doi: 10.5194/hess-22-317-2018
Nearing, G. S., Ruddell, B. L., Bennett, A. R., Prieto, C., and Gupta, H. V. (2020). Does information theory provide a new paradigm for earth science? Hypothesis testing. Water Resour. Res. 56, e2019WR024918. doi: 10.1029/2019WR024918
Nearing, G. S., Tian, Y., Gupta, H. V., Clark, M. P., Harrison, K. W., and Weijs, S. V. (2016). A philosophical basis for hydrological uncertainty. Hydrol. Sci. J. 61, 1666–1678. doi: 10.1080/02626667.2016.1183009
Newell Price, J. P., Harris, D., Taylor, M., Williams, J. R., Anthony, S. G., Duethmann, D., et al. (2011). “An Inventory of Mitigation Methods and Guide to their Effects on Diffuse Water Pollution, Greenhouse Gas Emissions and Ammonia Emissions from Agriculture: User Guide”. (Prepared as part of Defra Project WQ0106). Available online at: https://repository.rothamsted.ac.uk/item/98583/an-inventory-of-mitigation-methods-and-guide-to-their-effects-on-diffuse-water-pollution-greenhouse-gas-emissions-and-ammonia-emissions-from-agriculture
Packett, E., Grigg, N. J., Wu, J., Cuddy, S. M., Wallbrink, P. J., and Jakeman, A. J. (2020). Mainstreaming gender into water management modelling processes. Environ. Model. Softw. 127, 104683. doi: 10.1016/j.envsoft.2020.104683
Ridolfi, E., Albrecht, F., and Di Baldassarre, G. (2020). Exploring the role of risk perception in influencing flood losses over time. Hydrol. Sci. J. 65, 12–20. doi: 10.1080/02626667.2019.1677907
Rusca, M., and Di Baldassarre, G. (2019). Interdisciplinary critical geographies of water: capturing the mutual shaping of society and hydrological flows. Water 11, 1973. doi: 10.3390/w11101973
Sadoff, C. W., Hall, J. W., Grey, D., Aerts, J. C. J. H., Ait-Kadi, M., Brown, C., et al. (2015). Securing Water, Sustaining Growth: Report of the GWP/OECD Task Force on Water Security and Sustainable Growth. Oxford: University of Oxford.
Sanz, D., Vos, J., Rambags, F., Hoogesteger, J., Cassiraga, E., and Gómez-Alday, J. J. (2019). The social construction and consequences of groundwater modelling: insight from the Mancha Oriental aquifer, Spain. Int. J. Water Resour. Dev. 35, 808–829. doi: 10.1080/07900627.2018.1495619
Sawada, Y., and Hanazaki, R. (2020). Socio-hydrological data assimilation: analyzing human–flood interactions by model–data integration. Hydrol. Earth Syst. Sci. 24, 4777–4791. doi: 10.5194/hess-24-4777-2020
Seeger, M. (2007). Uncertainty of factors determining runoff and erosion processes as quantified by rainfall simulations. Catena 71, 56–67. doi: 10.1016/j.catena.2006.10.005
Shapiro, J., and McNeish, J.-A., (eds.). (2021). Our Extractive Age: Expressions of Violence and Resistance. London: Routledge. doi: 10.4324/9781003127611
Smith, L., Hiscock, K., Porter, K., Krueger, T., and Benson, D. (2015). “Getting informed: Tools and approaches for assessment, planning and management,” in Catchment and River Basin Management: Integrating Science and Governance, eds. L. Smith, K. Porter, K. Hiscock, M. J. Porter, and D. Benson (Oxon: Routledge), 222–238. doi: 10.4324/9780203129159
Srinivasan, V., Sanderson, M., Garcia, M., Konar, M., Blöschl, G., and Sivapalan, M. (2017). Prediction in a socio-hydrological world. Hydrol. Sci. J. 62, 338–345. doi: 10.1080/02626667.2016.1253844
Thaler, T. (2021). Social justice in socio-hydrology—how we can integrate the two different perspectives. Hydrol. Sci. J. 66, 1503–1512. doi: 10.1080/02626667.2021.1950916
Troy, T. J., Konar, M., Srinivasan, V., and Thompson, S. (2015). Moving sociohydrology forward: a synthesis across studies. Hydrol. Earth Syst. Sci. 19, 3667–3679. doi: 10.5194/hess-19-3667-2015
Venot, J.-P., and Jensen, C. B. (2022). A multiplicity of prek(s): enacting a socionatural mosaic in the Cambodian upper Mekong delta. Environ. Plan. E Nat. Space 5, 1446–1465. doi: 10.1177/25148486211026835
Venot, J.-P., Vos, J., Molle, F., Zwarteveen, M., Veldwisch, G. J., Kuper, M., et al. (2022). A bridge over troubled waters. Nat. Sustain. 5, 92–92. doi: 10.1038/s41893-021-00835-y
Viglione, A., Di Baldassarre, G., Brandimarte, L., Kuil, L., Carr, G., Salinas, J. L., et al. (2014). Insights from socio-hydrology modelling on dealing with flood risk: roles of collective memory, risk-taking attitude and trust. J. Hydrol. 518, 71–82. doi: 10.1016/j.jhydrol.2014.01.018
Wesselink, A., Kooy, M., and Warner, J. (2017). Socio-hydrology and hydrosocial analysis: toward dialogues across disciplines. WIREs Water 4, e1196. doi: 10.1002/wat2.1196
Yates, J. S., Harris, L. M., and Wilson, N. J. (2017). Multiple ontologies of water: politics, conflict and implications for governance. Environ. Plan. D Soc. Space 35, 797–815. doi: 10.1177/0263775817700395
Keywords: Panta Rhei, Science and Technology Studies, choice, enactment, agonism, critical, model, political
Citation: Krueger T and Alba R (2022) Ontological and epistemological commitments in interdisciplinary water research: Uncertainty as an entry point for reflexion. Front. Water 4:1038322. doi: 10.3389/frwa.2022.1038322
Received: 06 September 2022; Accepted: 01 November 2022;
Published: 17 November 2022.
Edited by:
Melissa Haeffner, Portland State University, United StatesReviewed by:
Luk J. M. Peeters, CSIRO Land and Water, AustraliaShervan Gharari, University of Saskatchewan, Canada
Bihu Suchetana, Indian Institute of Technology Roorkee, India
Rebecca Lave, Indiana University, United States
Copyright © 2022 Krueger and Alba. This is an open-access article distributed under the terms of the Creative Commons Attribution License (CC BY). The use, distribution or reproduction in other forums is permitted, provided the original author(s) and the copyright owner(s) are credited and that the original publication in this journal is cited, in accordance with accepted academic practice. No use, distribution or reproduction is permitted which does not comply with these terms.
*Correspondence: Tobias Krueger, dG9iaWFzLmtydWVnZXJAaHUtYmVybGluLmRl