- 1Faculty of Engineering, Computing and Science, Swinburne University of Technology Sarawak Campus, Kuching, Malaysia
- 2Department of Biological Sciences and the Advanced Environmental Research Institute, University of North Texas, Denton, TX, United States
- 3Woods Hole Oceanographic Institution, Woods Hole, MA, United States
- 4Savannah River Ecology Laboratory, University of Georgia, Aiken, SC, United States
- 5Center for Reservoir and Aquatic Systems Research and Department of Biology, Baylor University, Waco, TX, United States
- 6Institute of Marine Sciences and Department of Earth, Marine, and Environmental Sciences, University of North Carolina at Chapel Hill, Morehead City, NC, United States
- 7School of the Environment, The University of Queensland, Brisbane, QLD, Australia
- 8CSIRO Environment, Brisbane, QLD, Australia
- 9Ecosystems and Global Change Group, School of the Environment, Trent University, Peterborough, ON, Canada
- 10State Key Laboratory of Lake Science and Environment, Nanjing Institute of Geography and Limnology, Chinese Academy of Sciences, Nanjing, China
- 11Interuniversity Research Group in Limnology (GRIL), University of Quebec at Montreal, Montreal, QC, Canada
- 12Molecular Eco-Geochemistry Laboratory, Department of Geological Sciences, The University of Alabama, Tuscaloosa, AL, United States
Dissolved organic matter (DOM) assemblages in freshwater rivers are formed from mixtures of simple to complex compounds that are highly variable across time and space. These mixtures largely form due to the environmental heterogeneity of river networks and the contribution of diverse allochthonous and autochthonous DOM sources. Most studies are, however, confined to local and regional scales, which precludes an understanding of how these mixtures arise at large, e.g., continental, spatial scales. The processes contributing to these mixtures are also difficult to study because of the complex interactions between various environmental factors and DOM. Here we propose the use of machine learning (ML) approaches to identify ecological processes contributing toward mixtures of DOM at a continental-scale. We related a dataset that characterized the molecular composition of DOM from river water and sediment with Fourier-transform ion cyclotron resonance mass spectrometry to explanatory physicochemical variables such as nutrient concentrations and stable water isotopes (2H and 18O). Using unsupervised ML, distinctive clusters for sediment and water samples were identified, with unique molecular compositions influenced by environmental factors like terrestrial input and microbial activity. Sediment clusters showed a higher proportion of protein-like and unclassified compounds than water clusters, while water clusters exhibited a more diversified chemical composition. We then applied a supervised ML approach, involving a two-stage use of SHapley Additive exPlanations (SHAP) values. In the first stage, SHAP values were obtained and used to identify key physicochemical variables. These parameters were employed to train models using both the default and subsequently tuned hyperparameters of the Histogram-based Gradient Boosting (HGB) algorithm. The supervised ML approach, using HGB and SHAP values, highlighted complex relationships between environmental factors and DOM diversity, in particular the existence of dams upstream, precipitation events, and other watershed characteristics were important in predicting higher chemical diversity in DOM. Our data-driven approach can now be used more generally to reveal the interplay between physical, chemical, and biological factors in determining the diversity of DOM in other ecosystems.
1 Introduction
The movement of water connects not only terrestrial and aquatic life but also fresh- and marine water subsidies, transporting, for example, large amounts of terrestrial carbon (C) in the form of particulate and dissolved organic matter (DOM) along the land-ocean aquatic continuum (Drake et al., 2018). During its journey, DOM provides nutrients and energy to the aquatic food web (Azam et al., 1983), undergoing many biotic and abiotic transformations depending on its intrinsic composition, as well as extrinsic constraints such as microbial community composition and environmental conditions (Berggren et al., 2022, Hu et al., 2022). Thus, the DOM pool represents a complex blend of numerous compounds with varied compositions and quantities (Catalán et al., 2021) arising from diverse sources, transformation processes, and environmental contexts (Cooper et al., 2022). DOM chemistry also reflects a combination of biogeochemical processes (Amon and Benner, 1996; Ward et al., 2017; Ferreira et al., 2020) occurring across terrestrial and aquatic ecosystems. Determining DOM molecular composition and its reactivity within and across watershed compartments are central pieces to disentangle its role in carbon and nutrient cycles and flux of gasses to the atmosphere in a changing world.
Although recent studies have shown distinct spatial patterns of DOM within and across streams (Riedel et al., 2016; Garayburu-Caruso et al., 2020; Stadler et al., 2023; Freeman et al., 2024), the intrinsic and/or extrinsic attributes driving such variations are not yet fully understood. Research has shown that the composition of DOM varies across different scales including in-stream compartments, positions in the river networks, and latitude zones (Jaffé et al., 2012; Roth et al., 2013; Hawkes et al., 2018). For example, distinct patterns of DOM molecules have been observed when comparing surface waters and hyporheic zones (Stegen et al., 2022) and in rivers with different sizes of upstream catchment areas (Danczak et al., 2023). Another study in US rivers showed that molecular richness in river sediment decreased with increasing latitude (Cui et al., 2024). Furthermore, the composition of DOM is shaped by its reactivity to photochemical and microbial transformations, as well as to solid-phase sequestration such as flocculation and adsorption (e.g., Lu et al., 2013; Wen et al., 2022). Currently, the processing rates of organic C degradation vary regionally and globally (Tiegs et al., 2019), likely arising from the differences in biotic (autotrophic production and heterotrophic microbial degradation) and abiotic (e.g., light) degradation of DOM across large spatial scales. Specifically, environmental factors such as temperature, precipitation, and solar irradiation, have been identified as important regulators of DOM compositions at both region and continental scales (Du et al., 2022, 2023). The variation in microbial community compositions, driven by environmental factors within and across stream ecosystems, also plays a role. The distinct capacities of different microbes for DOM synthesis and degradation can contribute to differences in DOM molecular composition (Amaral et al., 2016; Logue et al., 2016; D’Andrilli et al., 2019; Tanentzap et al., 2019; Wang et al., 2022).
Most of the aforementioned studies have characterized DOM and its association with physicochemical drivers by employing multivariate statistical methods like principal component analysis (PCA) and discriminant analysis (see for example Angst et al., 2016; Johnson et al., 2019 and Lynch et al., 2019). These methods, being primarily linear in nature, have limitations in accurately reflecting complex biogeochemical processes (e.g., varying stability of molecules under different biogeochemical conditions), resulting in a potential misinterpretation of meaningful information as noise. Machine learning (ML) approaches, on the other hand, are particularly useful in ecological studies with complex data where non-linear relationships and interactions between various environmental factors and parameters of interest such as DOM properties exist. Random Forest (RF), an ensemble learning-based ML algorithm proposed by Breiman (2001), has been shown to improve accuracy by integrating results from numerous decision trees, giving more weight to significant variables while minimizing the impact of noise. Additionally, RF can assess the importance of different variables that influence model accuracy, which is essential for understanding the distinctions between different samples. This approach offers a more effective way of handling complex molecular DOM data that can further improve interpretation of biotic and abiotic controls in diverse ecosystems. Other studies that showcase the nuances of identifying molecular DOM composition patterns include Spencer et al. (2007) on diurnal variability in riverine DOM composition, He et al. (2016) on the molecular diversity of riverine sediment organic matter, and Cuss et al. (2016) on classifying DOM using ML and fluorescence signatures. Artificial neural networks (ANNs) have also been applied to biogeochemical and ecological studies for their efficiency in revealing patterns and predicting outcomes. For instance, ANNs have been instrumental in identifying the patterns involved in the spatial and temporal variation of the abundance and composition of abiotic and biotic variables (Larsen et al., 2012; Broullón et al., 2020; Danczak et al., 2020).
Here, we use a ML approach to identify patterns and trends in the previously characterized molecular composition of continental-scale river and sediment DOM samples collected under the crowdsourced Worldwide Hydrobiogeochemical Observation Network for Dynamic River Systems (WHONDRS; see for example Barnard et al., 2022; Borton et al., 2022; Dwivedi et al., 2022; Goldman et al., 2022). The data set was created using Fourier Transform Ion Cyclotron Resonance Mass Spectrometry (FTICR-MS) which generates highly dimensional datasets that largely defy 2D or other linear approaches of data manipulation. Given the high dimensional complexity of these data, the use of ML methods may help resolve the drivers of DOM composition, reactivity, and chemical character across systems. We focus on molecular diversity (number of unique molecular formulae per sample, e.g., Danczak et al., 2023) and composition and address two main questions: (1) how do the different classes of DOM and their molecular attributes vary within and between sediment and surface waters? and (2) how do the relative contributions of biotic and abiotic variables (i.e., watershed characteristics, macronutrient ratios, oxidation state, sediment metabolism) drive variation in sediment and surface water DOM.
2 Materials and methods
To conduct this study, we analyzed previously published data from the WHONDRS Summer 2019 Sampling (S19S) campaign (Stegen et al., 2018) using unsupervised and supervised ML approaches to identify the environmental parameters influencing the diversity of DOM clusters (Figure 1).
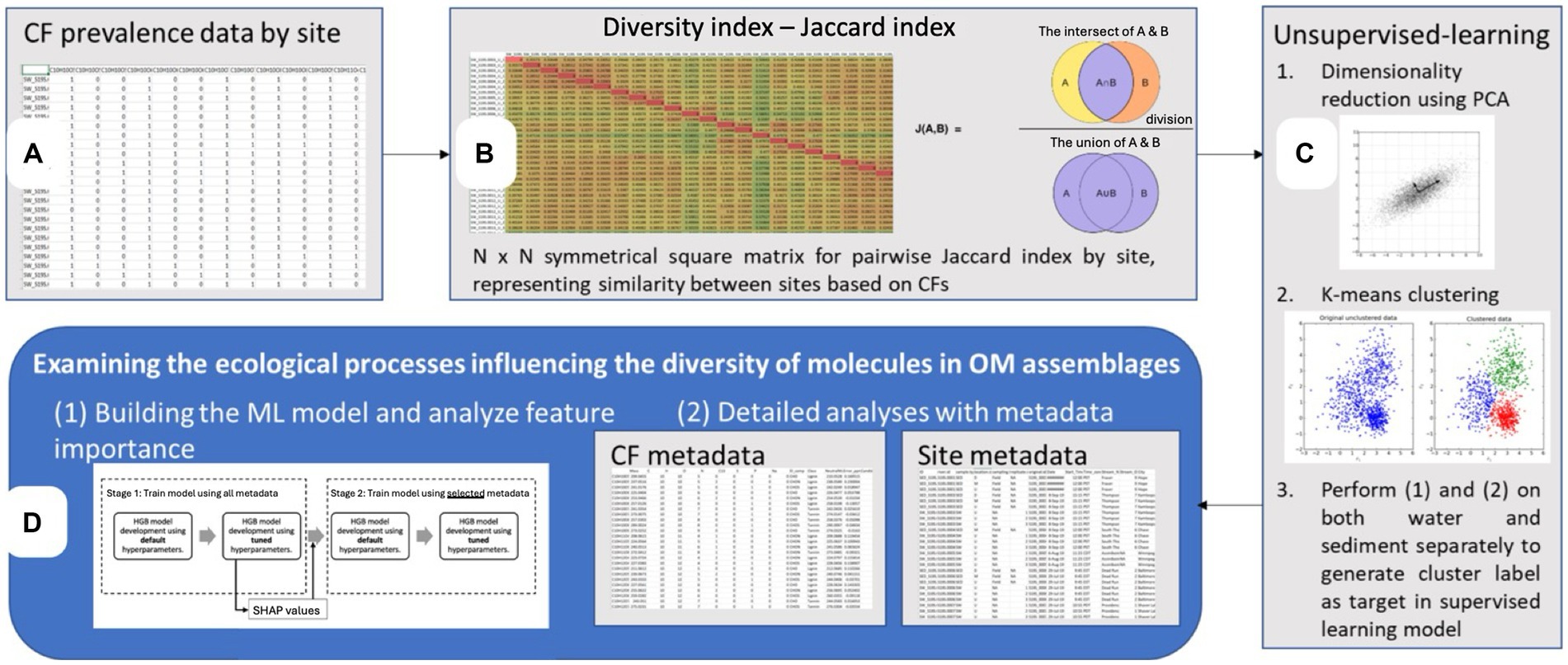
Figure 1. Overview of the multifaceted data analysis framework employed in this study [Steps (A–D)]. The framework begins with (A) the prevalence data for molecular (chemical) formulae (CF) across all sites, represented in a binary matrix format used to determine observed richness. Next (B) a diversity index based on the Jaccard similarity coefficient is calculated, yielding an N x N symmetrical matrix that captures the pairwise similarity between sites. Then (C) unsupervised machine learning techniques are applied, starting with dimensionality reduction via PCA (Principal Component Analysis) and followed by K-means clustering to identify inherent groupings in the data (water and sediment clusters). Lastly (D) a supervised machine learning approach, Histogram-based Gradient Boosting (HGB), is applied to obtain a deeper understanding of the environmental variables influencing molecular diversity of the dissolved organic matter (DOM) clusters.
2.1 Data sources
The samples we analyzed were collected and processed in 2019 as part of the WHONDRS consortium (Stegen et al., 2018), and the data were retrieved from publicly available data packages (Goldman et al., 2020; Toyoda et al., 2020). Full details on sample and metadata collection are provided in Garayburu-Caruso et al. (2020). In brief, during July and August 2019, 97 river corridor systems were sampled for surface water and sediment, along with metadata, climate, vegetation, and geospatial data. Surface water was collected in triplicate, filtered (0.22 μmSterivex), and stored in clean, pre-acidified amber VOA glass vials. Sediment samples were collected at sediment surface depths (1–3 cm) using a sterilized stainless-steel scoopula. Samples were rapidly shipped to the Pacific Northwest National Laboratory (PNNL, Richland, Washington United States) and surface water samples were frozen at −20°C upon arrival and sediments were sieved (<2 mm), subsampled, and stored at −20°C. A 12 Tesla Bruker SolariX FTICR-MS (mass resolving power was 220,000 at m/z 481.185) was used to collect ultrahigh-resolution mass spectra of DOM in each surface water and sediment sample (Garayburu-Caruso et al., 2020). The FTICR-MS was equipped with an Electrospray Ionization (ESI) source and operated in negative mode at a − 4.2 kV voltage. Data collection varied between surface water (0.05 s ion accumulation) and sediment (0.1 or 0.2 s ion accumulation), covering a m/z range of 100–900 at 4 M. The mass accuracy was less than 1 ppm for singly charged ions in the m/z 100–900 range (Garayburu-Caruso et al., 2020).
Surface water samples were analyzed for dissolved organic carbon (DOC) concentrations, stable water isotopes [oxygen (O) and hydrogen (H)], specific conductivity, total nitrogen (TN) concentrations, and concentrations of chloride (Cl−), sulfate (SO42−), nitrate (NO3−), nitrite (NO2−), and fluorine (F−); see Toyoda et al. (2020) for details of these measurements. Sediment samples were assessed for non-purgeable organic carbon as sediment, water extractable organic carbon (WEOC) concentrations, microbial respiration rates, and X-ray fluorescence; details can be found in Goldman et al. (2020). Additional information on WHONDRS and methods used can be found at https://whondrs.pnnl.gov. Some of the metadata for the continental United States sites are from the StreamCat database accessed through https://waterfolk.shinyapps.io/streamcat/ (Hill et al., 2016; Powers et al., 2023).
2.2 Fourier transform ion cyclotron resonance mass spectrometry data processing
The WHONDRS dataset has been discussed in other publications (i.e., Garayburu-Caruso et al., 2020) In the following, we provide a brief overview of the original data processing. Data were pre-processed (Garayburu-Caruso et al., 2020) using the BrukerDaltonik Data Analysis software (version 4.2), which allowed the conversion of raw spectra to a list of m/z values by applying a signal-to-noise ratio (S/N) of 7 and mass measurement error < 0.5 ppm. Peaks were then aligned, and molecular formulae assigned using Formularity software (Tolić et al., 2017). The initial assignments were post-processed using the R package ftmsRanalysis (Bramer et al., 2020), removing results outside of a high confidence m/z range (200–900) and/or with a 13C isotopic signature for further DOM characterization analysis. The ftmsRanalysis package calculates molecular formula properties and chemical classes (Kim et al., 2003; Koch and Dittmar, 2006; LaRowe and Van Cappellen, 2011). Molecular formulae were then classified into amino sugar-like, carbohydrate-like, condensed aromatic-like, lignin-likes, lipid-like, protein-like, tannin-like, and unsaturated hydrocarbon-like compounds using the assign_class() function (see Kim et al., 2003 for chemical class descriptions and elemental properties). Such chemical compound classes are determined based on the atomic O/C and H/C ratios from the assigned formulae, which have shown to be consistent with other analytical techniques (Kim et al., 2003).
Peak intensities were transformed into presence-absence data, with sediment samples from different river segments of the same river treated as replicates (Dorazio et al., 2011). Peaks that were assigned the same molecular formula due to minor mass differences were merged (0.5 ppm threshold). Only peaks with an assigned molecular formula and with an elemental combination of C1–130, H1–200, O1–50, N0–4, S0–2, and P0–1 were retained (Riedel and Dittmar, 2014). The Compound Identification Algorithm in Formularity was used with the following criteria: S/N > 7 and mass measurement error < 0.5 ppm. This algorithm takes into consideration the presence of C, H, O, N, S, and P and excludes other elements. Molecular formulae in the range of 0.3 ≥ H/C ≤ 2.2 and O/C ≤ 1.2 (Hawkes et al., 2020) and double bond equivalents minus oxygen ≤10 were considered reliable based on chemical feasibility (Herzsprung et al., 2014).
The molecular properties and chemical character of the molecular formulae were calculated, including their nominal oxidation state of C (NOSC) (unitless; Garayburu-Caruso et al., 2020), Gibbs Free Energy GFE (in kJ/mol C; according to LaRowe and Van Cappellen, 2011), double bond equivalent DBE (unitless; according to Koch and Dittmar, 2006), and degree of aromaticity AImod (unitless; according to Koch and Dittmar, 2006).
2.3 Machine-based learning examination of DOM composition
Our analysis focused on three categories: DOM data (matrix of assigned DOM molecular formulae), relevant environmental metadata, pertinent to biological and/or chemical DOM processes (including pH, water temperature, concentrations of Cl−, F−, and nitrate, isotopic composition, δ18O, δ2H, and mean annual temperature, MAT, among others), and DOM molecular properties and chemical character. Molecular formulae present in less than 10% of samples were categorized as “rare” and excluded.
The drivers of DOM molecular composition across diverse sites having similar characteristics can be difficult to interpret. To obtain a deeper understanding of differences and drivers of potentially small differences across the continental-scale dataset (Step A in Figure 1), we first reduced the dimensionality by applying molecular diversity indices (representing the composition of each sample) and counted observed richness as the number of unique molecular formulae per sample. Jaccard pairwise similarity coefficients were then calculated and used in Step B (Figure 1), resulting in a N x N engineered DOM dataset for both water (n = 265) and sediment (n = 239). Diversity metrics were calculated using the R package “vegan” (Oksanen et al., 2020) in the R environment (R Development Core Team, 2008). We then applied an unsupervised k-means clustering on the transformed data using PCA and the number of clusters decided by examining the distortion, inertias, and silhouette score for number of clusters ranging from 2 to 10 (Supplementary Figure S1). Each sample type was best characterized by 3 distinct clusters, referred to as Sed-0, Sed-1, Sed-2, Wat-0, Wat-1, and Wat-2 (see Figure 2 for clustering following PCA-k-means). The clustering is based on the Jaccard index (commonly used to determine how similar sample sets are), and likely represents similar ecological influences on DOM formation and diversity (Step C). After the removal of rare molecular formulae (present in less than 10% of samples) and unsupervised k-means clustering, we observed 4,936 molecular formulae in the 265 water samples and 4,053 molecular formulae in the 239 sediment samples with an overlap of 2,109 molecular formulae in both datasets. In total, 6,880 unique molecular formulae were found across water and sediment samples (Supplementary Table S1).
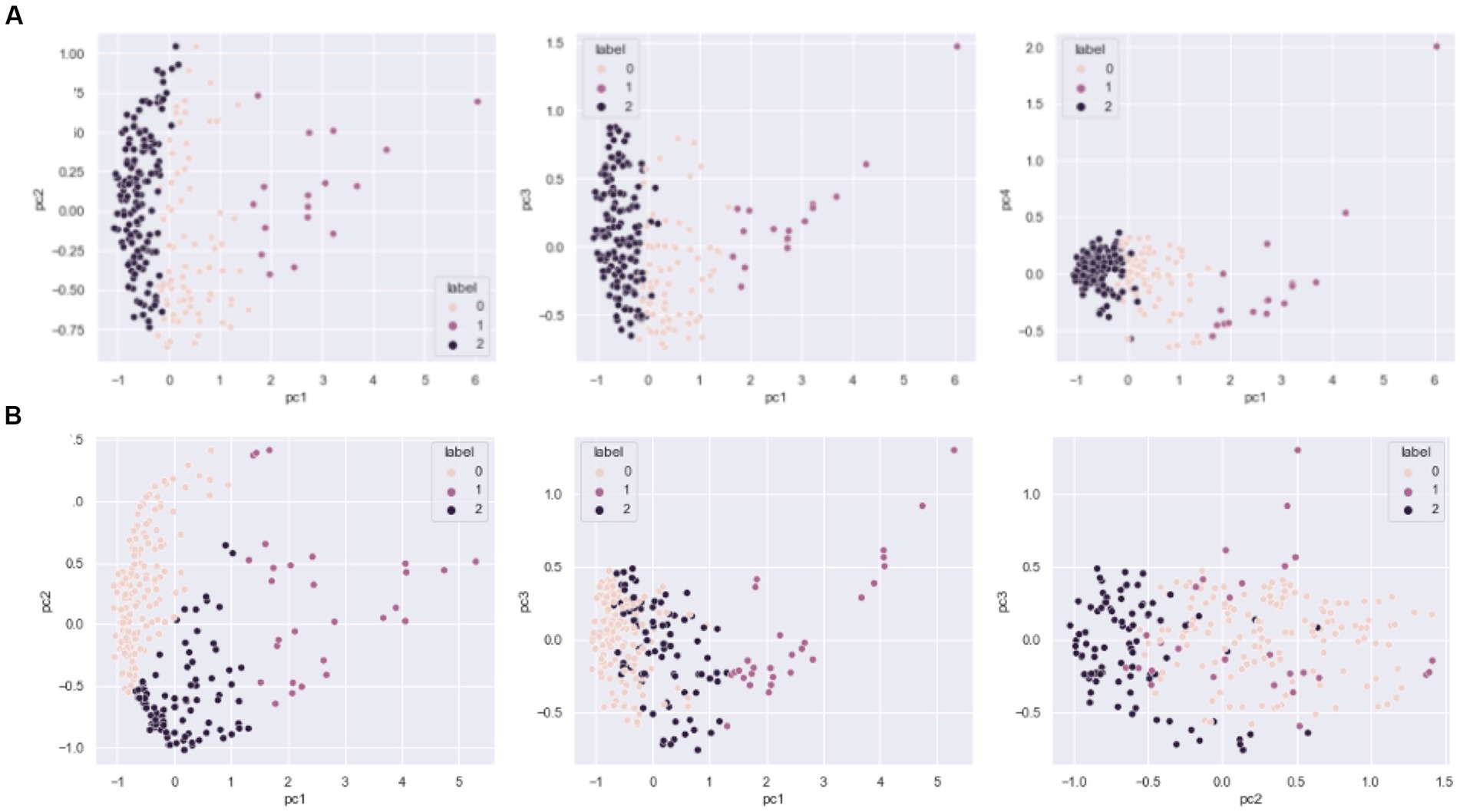
Figure 2. Principal component analysis (PCA)-based k-means clustering of the sediment (A) and water (B) samples. Different colors indicate the three different clusters found for the three principal components (PC1–PC3).
To obtain a better understanding of environmental influences driving the formation of the DOM clusters, we developed ML models. One-hot encoding was used for categorical data pre-processing. Class imbalances were addressed by generating additional samples for minority classes (clusters with lower sample numbers). Histogram-based Gradient Boosting (HGB) models were trained with the metadata to predict DOM cluster formations (Step D). Hyperparameter tuning was undertaken using the BayesSearchCV algorithm in the Scikit-Learn framework (Pedregosa et al., 2011). The hyperparameters (Supplementary Table S2) were selected to prevent model overfitting. SHapley Additive exPlanations (SHAP) values were computed for each set of metadata, ranked, and plotted. Features with positive SHAP values positively impact the prediction, while those with negative values have a negative impact. The magnitude is a measure of how strong the effect is. In each stage, two models were developed for each water and sediment sample. During the first stage of the model development, using all metadata, the models were developed using default and tuned hyperparameters. Metadata with high SHAP values computed from Stage 1 were selected for model training in Stage 2. Based on the SHAP values obtained in stage one, 13 metadata parameters were used to train the water model (13 for sediment) using HGB’s default hyperparameters and then the tuned hyperparameters. All models were evaluated for their performance using the 10-fold cross validation (CV), test accuracy score and accuracy score-based learning curve (Supplementary Table S3). Please see Supplementary Figure S2 (sediment) and Supplementary Figure S3 (water) for the accuracy-based learning curves for each HGB model and Supplementary Figures S4, S5 for an overview of SHAP values from each HGB model.
All data and codes are available at: https://github.com/WHONDRS-Crowdsourced-Manuscript-Effort/Topic4/tree/main.
3 Results and discussion
3.1 Unsupervised learning reveals diverse and distinct DOM clusters
Applying an unsupervised ML method resulted in highly distinguishable and unique clusters for the sample types - sediment and surface water. The 97 sampled systems and individual replicates (504 samples in total) were used as input for cluster analyses. For sediment samples, 76 samples were identified in the cluster 0 (Sed-0), 17 samples in cluster 1 (Sed-1) and the majority, 146 samples in cluster 2 (Sed-2). For surface water, 147 samples were clustered in the cluster 0 (Wat-0), 28 samples in the cluster 1 (Wat-1) and 90 in the cluster 2 (Wat-2). Concerning the prevalence of DOM molecular formulae, we found that most molecules identified in sediments were found across all clusters (70.1%), and some were exclusively present in Sed-0 and Sed-2 (29.9%), while a single unique formula was observed in Sed-2 and none were exclusively found in Sed-0 or Sed-1 (Supplementary Figure S6). Similar results were observed in water samples (Supplementary Figure S6), as an even higher number of molecular formulae were observed across the three clusters (80.5%), 17.7% in clusters Wat-0 and Wat-2, and just a few exclusively in Wat-0 only (0.45%) or in both Wat-0 and Wat-1 (1.4%). These results point toward homogenization in terms of shared molecular formulae across clusters, in both sediments and water habitats and potential variation in DOM signatures of individual samples within each cluster (which is considered below).
Differences in DOM molecular compositions of sediment and water samples belonging to the three identified clusters were expected given the potential influence of diverse environmental factors (e.g., terrestrial input, microbial activity, human activities, and runoff patterns) across the continental-scale dataset (Stegen et al., 2022). Using chemical compound classes determined by differences in atomic O/C and H/C ratios (Kim et al., 2003), we observed that, in general, samples of sediment clusters had a larger relative contribution of protein-like and unclassified chemical compound classes compared to water clusters. Sediment clusters 0, 1, and 2 had distinct compositions, with Sed-0 mostly comprised of lignin- and lipid-like compounds, Sed-1 was dominated by concentrated hydrocarbon-like (ConHC) and lignin-like compounds, and Sed-2 was dominated by lignin- and tannin-like compounds (Figure 3). The diversity in molecular composition potentially highlights different sources and processes affecting the clusters’ composition. Water clusters also had distinct compositions with Wat-0 being dominated by ConHC and tannin-like compounds, while that Wat-1 was dominated by amino sugar- and protein-like compounds, and Wat-2 by lignin-like compounds (Figure 3). Intriguingly, Sed-0 and Wat-1 contained the most diversified contribution of chemical compound classes. Molecular alpha diversity (considering observed richness) was significantly different (p < 0.01) across clusters, with diversity indices ranging from ~1,000 to 2,000 in sediment and slightly higher values between ~1,500 to 3,000 in water clusters (Figure 4).
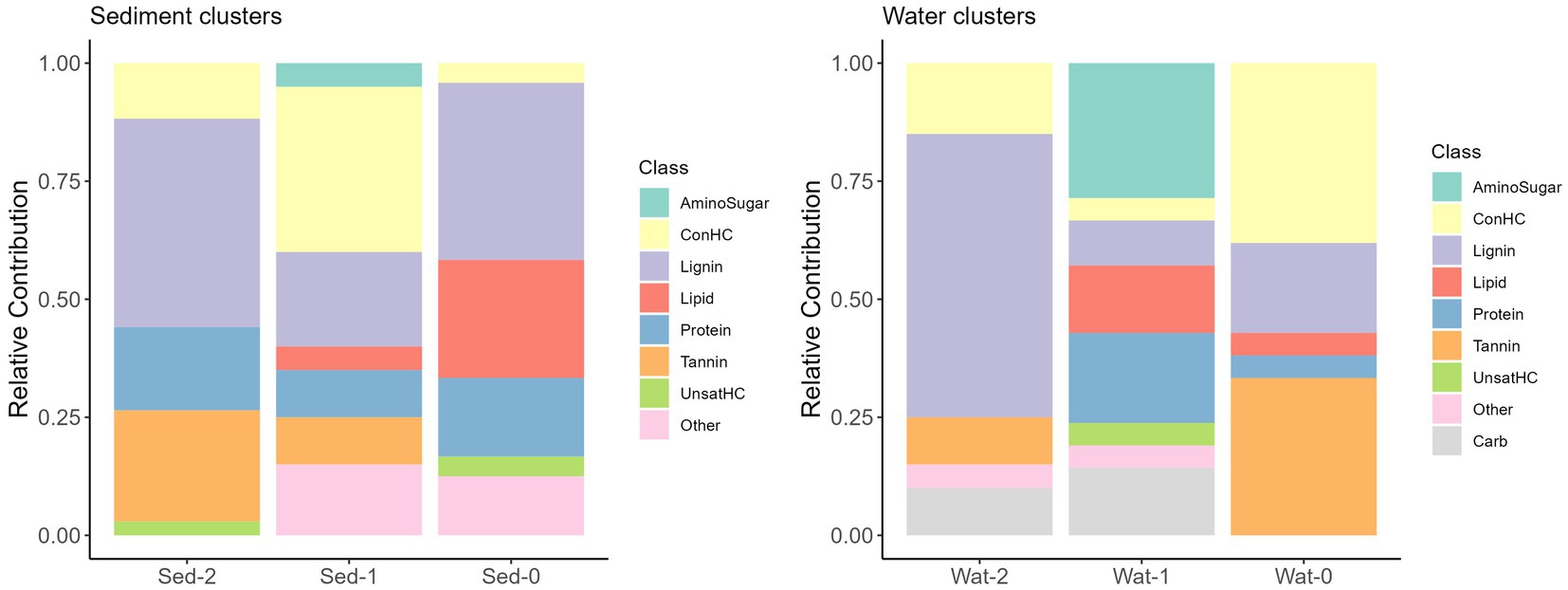
Figure 3. Overview of the relative contribution (%) of compound classes to the three sediment (left) and water (right) clusters. A heatmap of the key molecular formulae contributing to the cluster formation can be found in Supplementary Figure S7.
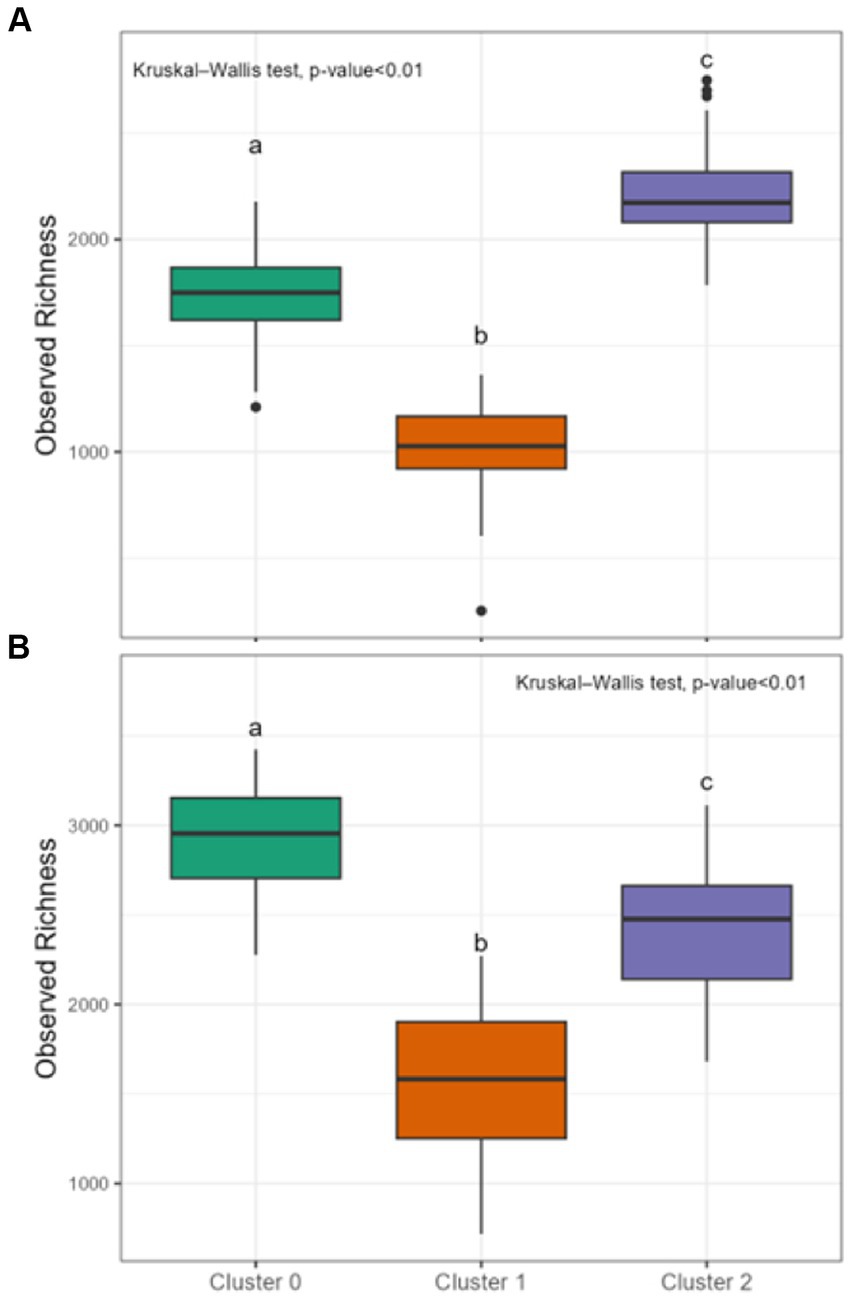
Figure 4. Diversity indices of sediment and water clusters. Observed richness for sediment clusters in (A) and for water clusters in (B). Lowercase letters a-c indicate significant differences between clusters based on Kruskal-Wallis followed by Dunn tests (p < 0.01). Each boxplot’s upper and lower hinges correspond to the first and third quartiles, respectively. The whiskers extend from the hinge to the largest and smallest value within 1.5 times the interquartile range. Data beyond whiskers are displayed as outlier points.
More information regarding the molecular composition of the clusters can be found in the Supplementary material (section 3). To summarize, the composition of sediment clusters indicates significant terrestrial inputs, particularly from vegetation. Sed-2 suggests influence from fresh plant debris due to its high CHO content. Nitrogen, sulfur, and phosphorus present in Sed-0 and Sed-1 point to microbial activity and human influences like agriculture and wastewater discharge. In water clusters, the abundance of CHO and lignin-like character in Wat-2 indicate terrestrial plant and soil inputs, hydrologic connectivity among soils and adjacent rivers, and the end-products of in-situ heterotrophic microbial degradation of DOM. Higher percentages of CHOS in Wat-0 may be attributed to biotic and abiotic sulfurization reactions under anoxic conditions or wastewater inputs. The abundance of lignin- and tannin-like character in Wat-0 and Wat-2 indicates natural and anthropogenic terrestrial sources from runoff and land use are also significant contributors. Phosphorus-containing formulae in Wat-1 and Wat-2 hints at nutrient cycling as a key process, potentially influenced by agricultural runoff (see Supplementary material section 3 for more details). This ML clustering approach revealed features like those obtained by optical fluorescence analyses (excitation emission matrices, e.g., Yamashita et al., 2008), and a methodological cross-validation in future studies could guide researchers toward more cost-effective methods to characterize DOM pools across spatial, temporal, and cross-boundaries scales.
The environmental parameters across sediment and water clusters showed distinct profiles for each cluster (see Supplementary Figures S8, S9). For the sediment clusters, only respiration rate and NPOC showed significant differences between the clusters (p-value of 0.01237 and 0, respectively; Supplementary Figure S8). In the sediment clusters, Sed-2 displayed significantly lower respiration rate, which could be related to the large contributions of lignin- and tannin-like classes–the large, structurally complex molecules that are considered more recalcitrant or resulting from microbial respiration. Sed-1 showed significantly higher NPOC concentrations. This cluster also contained more DOM having ConHC character, indicating the abundance of low-O containing DOM in the sediments, which may be related to in-situ processing or reflect the signature of previous processing in the water column before deposition. In contrast, the water clusters show a different pattern. For the water clusters, most variables showed significant differences, indicating that the clusters are highly distinct in terms of these environmental characteristics (Supplementary Figure S9). Wat-0 is characterized by greater distances from dams and gages and highest median number of days since precipitation, indicating DOM molecular composition sources in potentially more remote locations having drier climates. The dry condition corresponds to the higher proportions of condensed hydrocarbons in cluster 0, as fire can be a primary source for these compounds. Wat-2 is generally closer to the dam and gage with the least variability in distance, and experiences precipitation more frequently. This may be linked to higher proportions of lignin-like composition in Wat-2, since hydrological events predominantly facilitate the transfer of terrestrial DOM into aquatic ecosystems. Across both sediment and water clusters, the variability and median values suggest that each cluster experiences unique environmental conditions, with some being more prone to extremes and others displaying more homogeneity in their environmental parameters.
To determine whether the distribution of clusters is statistically significant with respect to latitude, longitude, or a combination of both, we performed point-biserial correlation and ANOVA (Analysis of Variance) tests. Like Cui et al. (2024), we observed a slight but statistically significant correlation between sediment cluster membership and latitude (Supplementary Table S4). While Sed-1 was positively related (p = 0.0029), Sed-2 showed a negative relationship with latitude (p = 0.0021). Sed-3 showed no significant correlation with latitude and all three clusters had no significant correlation with longitude. Water clusters displayed the opposite trend, no signification correlation with latitude but with longitude. This was, however, only true for Wat-0 which displayed a slight but statistically significant negative correlation with longitude (p = 0.091). This analysis suggests that while latitude and longitude do have some (weak) predictive power for cluster membership, they are not the main factors influencing it. More complex models and in-depth knowledge are required for a more accurate prediction of cluster membership.
3.2 Supervised machine-based learning reveals influence of environmental parameters on the molecular richness of DOM clusters
To gain deeper insights into how the various environmental parameters influenced the formation of DOM clusters in water and sediment samples, we applied a supervised ML algorithm (Histogram-based Gradient Boosting, HGB) using SHAP (Shapley Additive exPlanations) values. Sediment and water clusters were the targets and all environmental variables used to train the model (see methods for more details). The HGB ML model consistently highlighted surface water isotopic composition δ18O (‰) and mean annual temperature (MAT; °C) as influential across both sediment and water sample types (Figure 5). Both water and sediment SHAP values, however, also show that certain features have more variable impacts than others on DOM cluster formation, as indicated by the spread of the SHAP values along the x-axis (Figure 5). For example, NPOC concentration and respiration rates for sediment clusters and stream order and variations in natural flow in water clusters appear to have highly variable impacts (Figure 5). These examples demonstrate the complex, non-linear relationships inherent in ecological data.
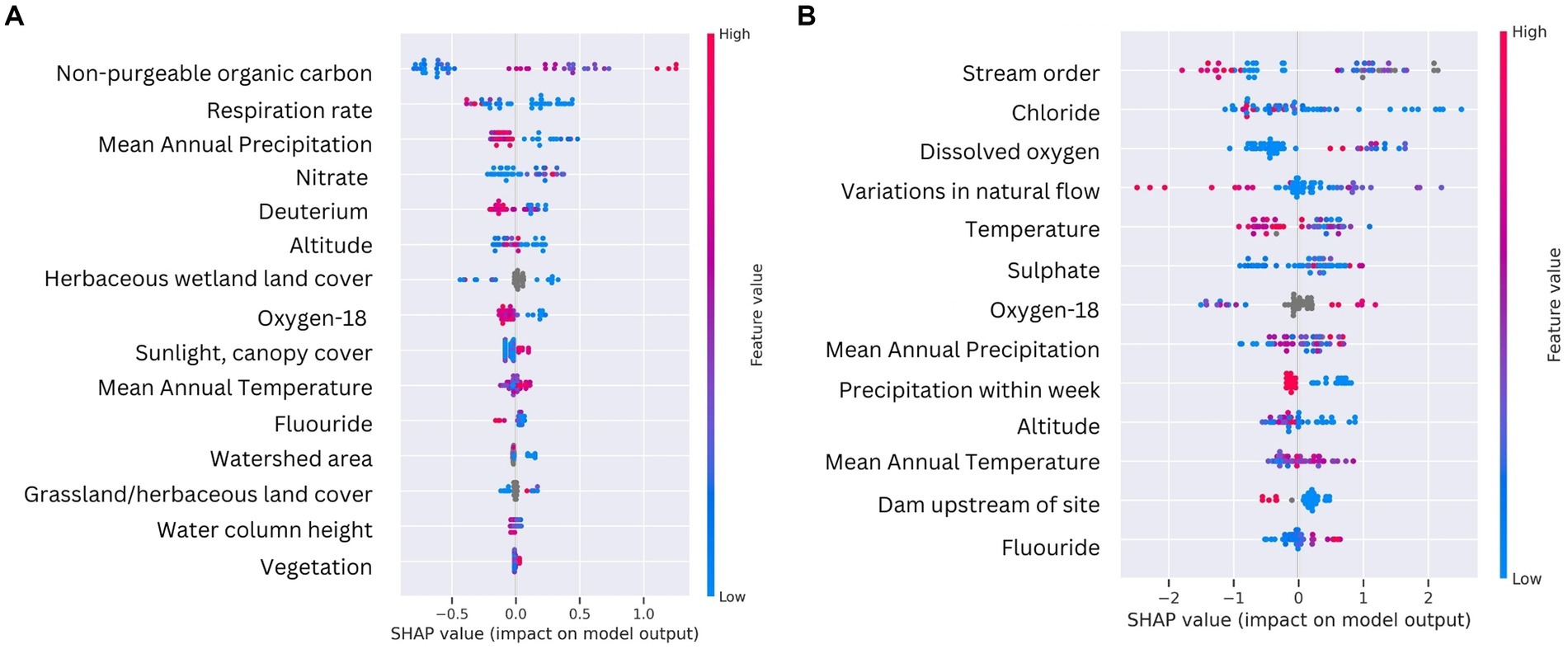
Figure 5. Summary plots for SHAP values of the two best performing HGB models for sediment (left) and water (right) sample types. Individual points represent samples. Features with positive SHAP values positively impact the prediction, while those with negative values have a negative impact. The magnitude is a measure of how strong the effect is. Features are arranged along the y axis based on their importance, which is given by the mean of their absolute SHAP values. The higher vertical position of the feature in the model, the greater importance it has on the model.
For sediment clusters, the SHAP values suggest that NPOC and nitrate concentrations help predict the DOM clusters. Features related to isotopic composition (δ18O and deuterium, ‰), and mean annual temperature (MAT; °C) were also highlighted as influential, which indicates the importance of geographic water source and thermodynamically favorable hydrological processes. Deuterium provides information about the role of precipitation, groundwater, and evaporation processes in continental waters, all of which may have different outcomes on the molecular composition of DOM (Baskaran et al., 2009). McDonough et al. (2022) discovered that the transformation of DOM in groundwater resulted in the elimination of oxidized DOM composition, along with an accumulation of both reduced photodegradable compounds and aerobically biodegradable compounds exhibiting a pronounced microbial signature. Ide et al. (2017) found significant variations in the number of DOM molecular formulae in rainwater, throughfall, soil water, groundwater, and stream water, with a linear correlation between DOM molecular diversity and the number of lignin-like molecules. Lignin-like composition was particularly high in groundwater samples. Sediment clusters 0 and 2 were characterized by more lignin-like composition (Figure 3) and could potentially be influenced by groundwater discharge.
Nitrate, respiration rate, mean annual precipitation, and grass percentage within 100-meter of the river all showed a negative influence on DOM cluster formation (Figure 5). The combination of respiration rate and water column depth could be interpreted as areas with deeper waters and more biological activity, as indicated by respiration rates, may harbor more diverse organic molecules within the sediment. Variables related to precipitation, in combination with nitrate, suggest that rainfall and higher nitrate concentrations may be associated with non-biomass building microbial processes leading to lower molecular richness in the sediment. Precipitation events have been shown to influence the amount and composition of DOM transported through river networks by mobilizing terrestrial DOM into the river water column and shifting flow paths to flushing upper, organic-rich soil horizons (Hong et al., 2012; Wagner et al., 2019). The negative influence of high nitrate concentrations could be due to conditions that are not conducive to molecular diversity, for example eutrophic conditions favoring the excessive production of algae-derived DOM. Elevated nitrate concentration is also commonly associated with agricultural influences, yet the impact on DOM can vary. Agricultural land use has been shown to increase microbially derived, protein-like DOM composition with decreased structural complexity (Wilson and Xenopoulos, 2008) and/or increase terrestrially derived, aromatic DOM composition (Shang et al., 2018; Ji et al., 2024). In comparing three types of riparian soils (forested, agricultural, and wetland soils) in headwater streams, Ji et al. (2024) found that agricultural soil DOM exhibited the lowest molecular richness, while agricultural particulate organic matter and DOM displayed highest molecular richness.
The water clusters had a broader range of SHAP values, suggesting that the model finds a greater variation in how the environmental parameters affect water DOM clusters. Physical and chemical parameters like MAT and inorganic ions (sulfate and chloride) have a substantial influence on the DOM clusters (Figure 5). Chloride has been associated with environmental conditions or events that foster a diverse array of organic molecules, such as increased groundwater discharge (Gue et al., 2018). Research conducted in coastal aquifers explored the molecular diversity of DOM in the subterranean estuary, revealing a unique ecohydrological interface where marine organic matter mixes with groundwater containing aged C from terrestrial sources (Waska et al., 2021). McDonough et al. (2021) used FTICR-MS to investigate the molecular composition and character of DOM in groundwater and reported that the molecular character of reactive DOM in groundwater differs from that of surface water. Fluoride had a positive impact on DOM clusters as well, particularly at higher values (Figure 5), supporting the potential role of groundwater discharge in DOM richness. Liu et al. (2015) explored how geochemical processes, including the role of DOM derived from rock weathering and biodegradation of organic matter, affect fluoride concentrations in groundwater. They showed that competitive adsorption of HCO3− and OH− with F− can lead to the release of F− from aquifer matrix into solution, increasing groundwater F− concentration. Overall, our ML approach can decipher environmental influences on DOM diversity that strongly agree with other published work.
The long-standing ecological conceptual model, the “River Continuum Concept,” has argued that stream order serves as a general predictor of DOM diversity, with the highest diversity appearing in low-order streams (Vannote et al., 1980). Evidence from empirical data, however, varies geographically and with anthropogenic influence. For instance, using FT-ICR MS, Mosher et al. (2015) showed 1st-order streams have the highest molecular formulae diversity and compound classes in a forested catchment, while Roebuck Jr et al. (2020) showed that the influence of stream order was outweighed by land use in regulating DOM compositions along a river continuum. Stream order had both negative (blue) and positive (red) influence on DOM clusters (Figure 5), which could indicate that DOM richness is increased in clusters consisting of samples taken in low-order streams and decreased in clusters made up by samples taken in higher-order streams.
Primary sources introducing flow variability showed a positive impact on DOM clusters (Figure 5). Such features could for example be dams, and the presence of upstream dams indeed showed a noticeable cluster of positive SHAP values, indicating that the presence of a dam upstream can be an important predictor for the model outcome. Dams showed a mix of positive and negative impacts, supporting our finding that Wat-2 is characterized by a close association to dams and gages as compared to Wat-0 and Wat-1 as being further away. Dams have been shown to affect the structure of DOM (Wang et al., 2021). In reservoirs created by dams, certain areas experience slower water flow compared to free-flowing river segments. This reduction in flow velocity alters the physical, chemical, and biological environment of the water, which in turn impacts the concentration and composition of DOM. Wang et al. (2021) showed that the reservoir area had relatively higher terrestrial input and increased abundance of recalcitrant DOM, a consequence of water intrusion from the main stem of the stream caused by the construction and operation of the reservoir. Dam constructions increase the residence time of DOM in the river (Hong et al., 2012) and Sun et al. (2017) noted that in slower flow areas of a mid-subtropical drinking water source reservoir, there was a higher content of certain DOM classes, supporting the notion that altered hydrodynamics can lead to variation in the DOM composition (Lynch et al., 2019). Non-anthropogenic organic debris dams in streams trap sediments and collect particulate organic matter, which again affects the concentration and composition of DOM in stream water (Bilby, 1981).
As observed for sediment clusters, recent precipitation events also had a cluster of high positive SHAP values at lower feature values (Figure 5) which suggests that rainfall events have a significant impact on water DOM clusters. DOM increases in streams during heavy rainstorms and snowmelt, mainly due to storm flow flushing through upper, organic matter-rich soil horizons (Kaiser and Guggenberger, 2005).
Recent research at the basin level, such as the study by Danczak et al. (2023) and Cui et al. (2024), have documented a correlation between watershed attributes and the chemical diversity of DOM in water. This research revealed that in the Yakima River, DOM chemical diversity expands with the growth of the watershed area and fluctuates with different types of land cover. While the extent to which findings from specific sites can be generalized to larger areas remains uncertain, our analysis on a continental scale hints at the possibility that connections between DOM diversity and watershed traits might be widespread. A better understanding of the watershed characteristics driving DOM beta diversity and richness could be instrumental in forecasting the chemical diversity of riverine DOM across extensive geographical regions.
4 Summary and conclusions
Our limited capacities to unravel biogeochemical processes in lotic ecosystems worldwide at different spatial and temporal scales, combined with a poor knowledge on complex interactions between abiotic and biotic drivers, result in an urgent need to develop new strategies and tools to study DOM. Such new approaches may allow us to identify the major patterns governing ecological processes, so we can predict how they might be affected in a changing world. Here, we applied unsupervised and supervised ML approaches to analyze the diversity and molecular composition of continental-scale river and sediment DOM samples of the WHONDRS database. We then assessed the potential influence of environmental parameters on their molecular diversity. This data-driven approach provided a mechanism to identify common DOM clusters and the key environmental conditions that generate these groups of compounds. While both sediment and water samples shared some common influential features, we found clear differences in the range and nature of the most influential parameters. Supervised ML revealed that features like dams, precipitation events, and watershed characteristics had significant impacts on the DOM composition and diversity, particularly in water samples. The study also underscored the complex and non-linear relationships inherent in ecological data, highlighting the need for advanced analytical methods like ML to understand non-linear correlations in large data sets and bridge relationship gaps across carbon cycling scientists in diverse ecological communities.
Data availability statement
The original contributions presented in the study are included in the article/Supplementary material, further inquiries can be directed to the corresponding author.
Author contributions
MM: Conceptualization, Data curation, Funding acquisition, Methodology, Project administration, Software, Supervision, Writing – original draft, Writing – review & editing. JD: Supervision, Data curation, Formal analysis, Investigation, Visualization, Writing – original draft, Writing – review & editing. VS: Data curation, Investigation, Methodology, Validation, Writing – review & editing. RB: Data curation, Formal analysis, Writing – original draft, Writing – review & editing. MB: Data curation, Investigation, Methodology, Resources, Writing – original draft, Writing – review & editing. ML: Software, Validation, Data curation, Formal analysis, Investigation, Methodology, Visualization, Writing – review & editing. FR: Software, Data curation, Formal analysis, Investigation, Methodology, Validation, Visualization, Writing – review & editing. AT: Supervision, Conceptualization, Data curation, Formal analysis, Investigation, Methodology, Validation, Visualization, Writing – original draft, Writing – review & editing. JW: Conceptualization, Investigation, Methodology, Validation, Writing – review & editing. MiM: Methodology, Validation, Visualization, Writing – review & editing, Data curation, Formal analysis, Investigation. YL: Investigation, Validation, Writing – review & editing.
Funding
The author(s) declare financial support was received for the research, authorship, and/or publication of this article. This research was supported by the United States Department of Energy, Office of Science, Office of Biological and Environmental Research, and Environmental System Science (ESS) Program. This contribution originates from the River Corridor Scientific Focus Area (SFA) project at Pacific Northwest National Laboratory (PNNL). PNNL is operated by Battelle Memorial Institute for the United States Department of Energy under Contract No. DE-AC05-76RL01830. This material is based upon work supported by the Department of Energy Office of Environmental Management under Award Number DE-EM0005228 to the University of Georgia Research Foundation for co-author RB.
Acknowledgments
Data used in this manuscript and for future manuscripts in this collection are from the WHONDRS’ 2019 crowdsourced global sampling campaign and we thank those that participated in the design and implementation of that effort. We also thank organizers and participants of the crowdsourced workshop (Borton et al., 2022) where initial scientific questions and hypotheses were developed.
Conflict of interest
The authors declare that the research was conducted in the absence of any commercial or financial relationships that could be construed as a potential conflict of interest.
The author(s) declared that they were an editorial board member of Frontiers, at the time of submission. This had no impact on the peer review process and the final decision.
Publisher’s note
All claims expressed in this article are solely those of the authors and do not necessarily represent those of their affiliated organizations, or those of the publisher, the editors and the reviewers. Any product that may be evaluated in this article, or claim that may be made by its manufacturer, is not guaranteed or endorsed by the publisher.
Supplementary material
The Supplementary material for this article can be found online at: https://www.frontiersin.org/articles/10.3389/frwa.2024.1379284/full#supplementary-material
References
Amaral, V., Graeber, D., Calliari, D., and Alonso, C. (2016). Strong linkages between DOM optical properties and main clades of aquatic bacteria. Limnol. Oceanogr. 61, 906–918. doi: 10.1002/lno.10258
Amon, R. M. W., and Benner, R. (1996). Photochemical and microbial consumption of dissolved organic carbon and dissolved oxygen in the Amazon River system. Geochim. Cosmochim. Acta 60, 1783–1792. doi: 10.1016/0016-7037(96)00055-5
Angst, G., John, S., Mueller, C. W., Kögel-Knabner, I., and Rethemeyer, J. (2016). Tracing the sources and spatial distribution of organic carbon in subsoils using a multi-biomarker approach. Sci. Rep. 6:29478. doi: 10.1038/srep29478
Azam, F., Fenchel, T., Field, J. G., Gray, J. S., Meyer-Reil, L.-A., Thingstad, F., et al. (1983). The ecological role of water-column microbes in the sea. Mar. Ecol. Prog. Ser. 10, 257–263. doi: 10.3354/meps010257
Barnard, M. A., Emani, S. R., Fortner, S. K., Haygood, L., Sun, Q., White-Newsome, J. L., et al. (2022). GeoHealth perspectives on integrated, coordinated, open, networked (ICON) science. Earth Space Sci. 9:e2021EA002157. doi: 10.1029/2021EA002157
Baskaran, S., Ransley, T., Brodie, R. S., and Baker, P. (2009). Investigating groundwater–river interactions using environmental tracers. Aust. J. Earth Sci. 56, 13–19. doi: 10.1080/08120090802541887
Berggren, M., François, G., Magdalena, B., Ishi, B., Anne, D., Jeffrey, A. M., et al. (2022). Unified understanding of intrinsic and extrinsic controls of dissolved organic carbon reactivity in aquatic ecosystems. Ecology. 103:e3763. doi: 10.1002/ecy.3763
Bilby, R. E. (1981). Role of organic debris dams in regulating the export of dissolved and particulate matter from a forested watershed. Ecology 62, 1234–1243. doi: 10.2307/1937288
Borton, M. A., Collins, S. M., Graham, E. B., Garayburu-Caruso, V. A., Goldman, A. E., de Melo, M., et al. (2022). It takes a village: using a crowdsourced approach to investigate organic matter composition in global rivers through the lens of ecological theory. Front. Water 4:453. doi: 10.3389/frwa.2022.870453
Bramer, L. M., White, A. M., Stratton, K. G., Thompson, A. M., Claborne, D., Hofmockel, K., et al. (2020). ftmsRanalysis: an R package for exploratory data analysis and interactive visualization of FT-MS data. PLoS Comput. Biol. 16:e1007654. doi: 10.1371/journal.pcbi.1007654
Broullón, D., Pérez, F. F., Velo, A., Hoppema, M., Olsen, A., Takahashi, T., et al. (2020). A global monthly climatology of oceanic total dissolved inorganic carbon: a neural network approach. Earth Syst. Sci. Data 12, 1725–1743. doi: 10.5194/essd-12-1725-2020
Catalán, N., Pastor, A., Borrego, C. M., Casas-Ruiz, J. P., Hawkes, J. A., Gutiérrez, C., et al. (2021). The relevance of environment vs. composition on dissolved organic matter degradation in freshwaters. Limnol. Oceanogr. 66, 306–320. doi: 10.1002/lno.11606
Cooper, W. T., Chanton, J. C., D’Andrilli, J., Hodgkins, S. B., Podgorski, D. C., Stenson, A. C., et al. (2022). A history of molecular level analysis of natural organic matter by FTICR mass spectrometry and the paradigm shift in organic geochemistry. Mass Spectrom. Rev. 41, 215–239. doi: 10.1002/mas.21663
Cui, Y., Wen, S., Stegen, J. C., Hu, A., and Wang, J. (2024). Chemodiversity of riverine dissolved organic matter: effects of local environments and watershed characteristics. Water Res. 250:121054. doi: 10.1016/j.watres.2023.121054
Cuss, C. W., McConnell, S. M., and Guéguen, C. (2016). Combining parallel factor analysis and machine learning for the classification of dissolved organic matter according to source using fluorescence signatures. Chemosphere 155, 283–291. doi: 10.1016/j.chemosphere.2016.04.061
D’Andrilli, J., Junker, J. R., Smith, H. J., Scholl, E. A., and Foreman, C. M. (2019). DOM composition alters ecosystem function during microbial processing of isolated sources. Biogeochemistry 142, 281–298. doi: 10.1007/s10533-018-00534-5
Danczak, R. E., Chu, R. K., Fansler, S. J., Goldman, A. E., Graham, E. B., Tfaily, M. M., et al. (2020). Using metacommunity ecology to understand environmental metabolomes. Nat. Commun. 11:6369. doi: 10.1038/s41467-020-19989-y
Danczak, R. E., Garayburu-Caruso, V. A., Renteria, L., McKever, S. A., Otenburg, O. C., Grieger, S. R., et al. (2023). Riverine organic matter functional diversity increases with catchment size. Front. Water 5:7108. doi: 10.3389/frwa.2023.1087108
Dorazio, R. M., Gotelli, N. J., and Ellison, A. M. (2011). Modern methods of estimating biodiversity from presence-absence surveys. Biodiversity loss in a changing planet. 277–302. doi: 10.5772/23881
Drake, T. W., Raymond, P. A., and Spencer, R. G. M. (2018). Terrestrial carbon inputs to inland waters: a current synthesis of estimates and uncertainty. Limnol. Oceanogr. Lett. 3, 132–142. doi: 10.1002/lol2.10055
Du, Y., Chen, F., Zhang, Y., He, H., Wen, S., Huang, X., et al. (2023). Human activity coupled with climate change strengthens the role of lakes as an active pipe of dissolved organic matter. Earth's Future 11:e2022EF003412. doi: 10.1029/2022EF003412
Du, Y., Luo, C., Chen, F., Zhang, Q., Zhou, Y., Jang, K.-S., et al. (2022). Water depth and transparency drive the quantity and quality of organic matter in sediments of Alpine Lakes on the Tibetan plateau. Limnol. Oceanogr. 67, 1959–1975. doi: 10.1002/lno.12180
Dwivedi, D., Santos, A. L. D., Barnard, M. A., Crimmins, T. M., Malhotra, A., Rod, K. A., et al. (2022). Biogeosciences perspectives on integrated, coordinated, open, networked (ICON) science. Earth Space Sci. 9:2119. doi: 10.1029/2021EA002119
Ferreira, V., Elosegi, A., Tiegs, S. D., von Schiller, D., Young, R., Tiegs, S., et al. (2020). Organic matter decomposition and ecosystem metabolism as tools to assess the functional integrity of streams and rivers–a systematic review. Water 12:3523. doi: 10.3390/w12123523
Freeman, E. C., Emilson, E. J. S., Dittmar, T., Braga, L. P. P., Emilson, C. E., Goldhammer, T., et al. (2024). Universal microbial reworking of dissolved organic matter along environmental gradients. Nat. Commun. 15:187. doi: 10.1038/s41467-023-44431-4
Garayburu-Caruso, V. A., Danczak, R. E., Stegen, J. C., Renteria, L., Mccall, M., Goldman, A. E., et al. (2020). Using community science to reveal the global chemogeography of river metabolomes. Meta 10:20518. doi: 10.3390/metabo10120518
Goldman, A. E., Arnon, S., Bar-Zeev, E., Chu, R. K., Danczak, R. E., Daly, R. A., et al. (2020). WHONDRS summer 2019 sampling campaign: global river corridor sediment FTICR-MS, dissolved organic carbon, aerobic respiration, elemental composition, grain size, total nitrogen and organic carbon content, bacterial abundance, and stable isotopes (v8).
Goldman, A. E., Emani, S. R., Pérez-Angel, L. C., Rodríguez-Ramos, J. A., and Stegen, J. C. (2022). Integrated, coordinated, open, and networked (ICON) science to advance the geosciences: introduction and synthesis of a special collection of commentary articles. Earth Space Sci. 9:2099. doi: 10.1029/2021ea002099
Gue, A., Grasby, S., and Mayer, B. (2018). Influence of saline groundwater discharge on river water chemistry in the Athabasca oil sands region – a chloride stable isotope and mass balance approach. Appl. Geochem. 89, 75–85. doi: 10.1016/J.APGEOCHEM.2017.10.004
Hawkes, J. A., D’Andrilli, J., Agar, J. N., Barrow, M. P., Berg, S. M., Catalán, N., et al. (2020). An international laboratory comparison of dissolved organic matter composition by high resolution mass spectrometry: are we getting the same answer? Limnol. Oceanogr. Methods 18, 235–258. doi: 10.1002/lom3.10364
Hawkes, J. A., Radoman, N., Bergquist, J., Wallin, M. B., Tranvik, L. J., and Löfgren, S. (2018). Regional diversity of complex dissolved organic matter across forested hemiboreal headwater streams. Sci. Rep. 8:16060. doi: 10.1038/s41598-018-34272-3
He, W., Chen, M., Park, J.-E., and Hur, J. (2016). Molecular diversity of riverine alkaline-extractable sediment organic matter and its linkages with spectral indicators and molecular size distributions. Water Res. 100, 222–231. doi: 10.1016/j.watres.2016.05.023
Herzsprung, P., Hertkorn, N., Von Tumpling, W., Harir, M., Friese, K., and Schmitt-Kopplin, P. (2014). Understanding molecular formula assignment of Fourier transform ion cyclotron resonance mass spectrometry data of natural organic matter from a chemical point of view. Anal. Bioanal. Chem. 406, 7977–7987. doi: 10.1007/s00216-014-8249-y
Hill, R. A., Weber, M. H., Leibowitz, S. G., Olsen, A. R., and Thornbrugh, D. J. (2016). The stream-catchment (StreamCat) dataset: a database of watershed metrics for the conterminous United States. J. Am. Water Resour. Assoc. 52, 120–128. doi: 10.1111/1752-1688.12372
Hong, H., Yang, L., Guo, W., Wang, F., and Yu, X. (2012). Characterization of dissolved organic matter under contrasting hydrologic regimes in a subtropical watershed using PARAFAC model. Biogeochemistry 109, 163–174. doi: 10.1007/s10533-011-9617-8
Hu, A., Choi, M., Tanentzap, A. J., Liu, J., Jang, K. S., Lennon, J. T., et al. (2022). Ecological networks of dissolved organic matter and microorganisms under global change. Nat. Commun. 13:3600. doi: 10.1038/s41467-022-31251-1
Ide, J., Ohashi, M., Takahashi, K., Sugiyama, Y., Piirainen, S., Kortelainen, P., et al. (2017). Spatial variations in the molecular diversity of dissolved organic matter in water moving through a boreal forest in eastern Finland. Sci. Rep. 7:42102. doi: 10.1038/srep42102
Jaffé, R., Yamashita, Y., Maie, N., Cooper, W. T., Dittmar, T., Dodds, W. K., et al. (2012). Dissolved organic matter in headwater streams: compositional variability across climatic regions of North America. Geochim. Cosmochim. Acta 94, 95–108. doi: 10.1016/j.gca.2012.06.031
Ji, H., Wang, H., Wu, Z., Wang, D., Wang, X., Fu, P., et al. (2024). Source, composition and molecular diversity of dissolved and particulate organic matter varied with riparian land use in tropical coastal headstreams. Sci. Total Environ. 908:168577. doi: 10.1016/j.scitotenv.2023.168577
Johnson, J. J., Olin, J. A., and Polito, M. J. (2019). A multi-biomarker approach supports the use of compound-specific stable isotope analysis of amino acids to quantify basal carbon source use in a salt marsh consumer. Rapid Commun. Mass Spectrom. 33, 1781–1791. doi: 10.1002/rcm.8538
Kaiser, K., and Guggenberger, G. (2005). Storm flow flushing in a structured soil changes the composition of dissolved organic matter leached into the subsoil. Geoderma 127, 177–187. doi: 10.1016/j.geoderma.2004.12.009
Kim, S., Kramer, R. W., and Hatcher, P. G. (2003). Graphical method for analysis of ultrahigh-resolution broadband mass spectra of natural organic matter, the van Krevelen diagram. Anal. Chem. 75, 5336–5344. doi: 10.1021/ac034415p
Koch, B. P., and Dittmar, T. (2006). From mass to structure: an aromaticity index for high-resolution mass data of natural organic matter. Rapid Commun. Mass Spectrom. 20, 926–932. doi: 10.1002/rcm.2386
LaRowe, D. E., and Van Cappellen, P. (2011). Degradation of natural organic matter: a thermodynamic analysis. Geoch. Cosmoch. Acta. 75, 2030–2042. doi: 10.1016/j.gca.2011.01.020
Larsen, P. E., Field, D., and Gilbert, J. A. (2012). Predicting bacterial community assemblages using an artificial neural network approach. Nat. Methods 9, 621–625. doi: 10.1038/nmeth.1975
Liu, H., Guo, H., Yang, L., Wu, L., Li, F., Li, S., et al. (2015). Occurrence and formation of high fluoride groundwater in the Hengshui area of the North China plain. Environ. Earth Sci. 74, 2329–2340. doi: 10.1007/s12665-015-4225-x
Logue, J. B., Stedmon, C. A., Kellerman, A. M., Nielsen, N. J., Andersson, A. F., Laudon, H., et al. (2016). Experimental insights into the importance of aquatic bacterial community composition to the degradation of dissolved organic matter. ISME J. 10, 533–545. doi: 10.1038/ismej.2015.131
Lu, Y. H., Bauer, J. E., Canuel, E. A., Yamashita, Y., Chambers, R. M., and Jaffé, R. (2013). Photochemical and microbial alteration of dissolved organic matter in temperate headwater streams associated with different land use. J. Geophys. Res. Biogeosci. 118, 566–580. doi: 10.1002/jgrg.20048
Lynch, L. M., Sutfin, N. A., Fegel, T. S., Boot, C. M., Covino, T. P., and Wallenstein, M. D. (2019). River channel connectivity shifts metabolite composition and dissolved organic matter chemistry. Nat. Commun. 10:459. doi: 10.1038/s41467-019-08406-8
McDonough, L. K., Andersen, M. S., Behnke, M. I., Rutlidge, H., Oudone, P., Meredith, K., et al. (2022). A new conceptual framework for the transformation of groundwater dissolved organic matter. Nat. Commun. 13:2153. doi: 10.1038/s41467-022-29711-9
McDonough, L., Behnke, M., Spencer, R., Marjo, C., Andersen, M., Meredith, K., et al. (2021). Molecular insights into the unique degradation trajectory of natural dissolved organic matter from surface to groundwater. Copernicus Meetings.
Mosher, J. J., Kaplan, L. A., Podgorski, D. C., McKenna, A. M., and Marshall, A. G. (2015). Longitudinal shifts in dissolved organic matter chemogeography and chemodiversity within headwater streams: a river continuum reprise. Biogeochemistry 124, 371–385. doi: 10.1007/s10533-015-0103-6
Oksanen, J., Blanchet, F. G., Friendly, M., Kindt, R., Legendre, P., McGlinn, D., et al. (2020). Vegan: Community Ecology Package. R package version 2.5–7. Available at: https://github.com/vegandevs/vegan (Accessed January 26, 2024).
Pedregosa, F., Varoquaux, G., Gramfort, A., Michel, V., Thirion, B., Grisel, O., et al. (2011). Scikit-learn: machine learning in Python. J. Mach. Learn. Res. 12, 2825–2830.
Powers, S. M., Barnard, M. A., Macleod, M. S., Miller, L. A., and Wagner, N. D. (2023). Spatially intensive patterns of water clarity in reservoirs determined rapidly with sensor-equipped boats and satellites. J. Geophys. Res. Biogeosci. 128:7650. doi: 10.1029/2023jg007650
R Development Core Team. (2008). R: A language and environment for statistical computing. R Foundation for Statistical Computing, Vienna, Austria. Available at: http://www.R-project.org (Accessed January 26, 2024).
Riedel, T., and Dittmar, T. (2014). A method detection limit for the analysis of natural organic matter via Fourier transform ion cyclotron resonance mass spectrometry. Anal. Chem. 86, 8376–8382. doi: 10.1021/ac501946m
Riedel, T., Zark, M., Vähätalo, A. V., Niggemann, J., Spencer, R. G. M., Hernes, P. J., et al. (2016). Molecular signatures of biogeochemical transformations in dissolved organic matter from ten world rivers. Front. Earth Sci. 4:85. doi: 10.3389/feart.2016.00085
Roebuck, J. A. Jr., Seidel, M., Dittmar, T., and Jaffé, R. (2020). Controls of land use and the river continuum concept on dissolved organic matter composition in an anthropogenically disturbed subtropical watershed. Environ. Sci. Technol. 54, 195–206. doi: 10.1021/acs.est.9b04605
Roth, V.-N., Dittmar, T., Gaupp, R., and Gleixner, G. (2013). Latitude and pH driven trends in the molecular composition of DOM across a north south transect along the Yenisei River. Geochim. Cosmochim. Acta 123, 93–105. doi: 10.1016/j.gca.2013.09.002
Shang, P., Lu, Y., Du, Y., Jaffé, R., Findlay, R. H., and Wynn, A. (2018). Climatic and watershed controls of dissolved organic matter variation in streams across a gradient of agricultural land use. Sci. Total Environ. 612, 1442–1453. doi: 10.1016/j.scitotenv.2017.08.322
Spencer, R. G. M., Pellerin, B. A., Bergamaschi, B. A., Downing, B. D., Kraus, T. E. C., Smart, D. R., et al. (2007). Diurnal variability in riverine dissolved organic matter composition determined by in situ optical measurement in the San Joaquin River (California, USA). Hydrol. Process. 21, 3181–3189. doi: 10.1002/hyp.6887
Stadler, M., Barnard, M. A., Bice, K., de Melo, M. L., Dwivedi, D., Freeman, E. C., et al. (2023). Applying the core-satellite species concept: characteristics of rare and common riverine dissolved organic matter. Front. Water 5:6042. doi: 10.3389/frwa.2023.1156042
Stegen, J. C., Fansler, S. J., Tfaily, M. M., Garayburu-Caruso, V. A., Goldman, A. E., Danczak, R. E., et al. (2022). Organic matter transformations are disconnected between surface water and the hyporheic zone. Biogeosciences 19, 3099–3110. doi: 10.5194/bg-19-3099-2022
Stegen, J. C., Goldman, A. E., Blackburn, S. E., Chu, R. K., Danczak, R. E., Garayburu-Caruso, V. A., et al. (2018). WHONDRS surface water sampling for metabolite biogeography (geochemistry and aligned FTICR-MS).
Sun, Q., Jiang, J., Zheng, Y., Wang, F., Wu, C., and Xie, R.-R. (2017). Effect of a dam on the optical properties of different-sized fractions of dissolved organic matter in a mid-subtropical drinking water source reservoir. Sci. Total Environ. 598, 704–712. doi: 10.1016/j.scitotenv.2017.04.175
Tanentzap, A. J., Fitch, A., Orland, C., Emilson, E. J. S., Yakimovich, K. M., Osterholz, H., et al. (2019). Chemical and microbial diversity covary in fresh water to influence ecosystem functioning. Proc. Natl. Acad. Sci. USA 116, 24689–24695. doi: 10.1073/pnas.1904896116
Tiegs, S. D., Costello, D. M., Isken, M. W., Woodward, G., McIntyre, P. B., Gessner, M. O., et al. (2019). Global patterns and drivers of ecosystem functioning in rivers and riparian zones. Sci. Adv. 5:eaav0486. doi: 10.1126/sciadv.aav0486
Tolić, N., Liu, Y., Liyu, A., Shen, Y., Tfaily, M. M., Kujawinski, E. B., et al. (2017). Formularity: software for automated formula assignment of natural and other organic matter from ultrahigh-resolution mass spectra. Analyt. Chem. 89, 12659–12665. doi: 10.1021/acs.analchem.7b03318
Toyoda, J. G., Goldman, A. E., Arnon, S., Bar-Zeev, E., Chu, R. K., Danczak, R. E., et al. (2020). WHONDRS summer 2019 sampling campaign: global river corridor surface water FTICR-MS, NPOC, TN, anions, stable isotopes, bacterial abundance, and dissolved inorganic carbon (v6).
Vannote, R. L., Minshall, G. W., Cummins, K. W., Sedell, J. R., and Cushing, C. E. (1980). The river continuum concept. Can. J. Fish. Aquat. Sci. 37, 130–137. doi: 10.1139/f80-017
Wagner, S., Fair, J. H., Matt, S., Hosen, J. D., Raymond, P., Saiers, J., et al. (2019). Molecular hysteresis: hydrologically driven changes in riverine dissolved organic matter chemistry during a storm event. J. Geophys. Res. Biogeosci. 124, 759–774. doi: 10.1029/2018jg004817
Wang, K., Pang, Y., He, C., Li, P., Xiao, S., Shi, Q., et al. (2021). Three gorges reservoir construction induced dissolved organic matter chemistry variation between the reservoir and non-reservoir areas along the Xiangxi tributary. Sci. Total Environ. 784:147095. doi: 10.1016/j.scitotenv.2021.147095
Wang, Y., Xie, R., Shen, Y., Cai, R., He, C., Chen, Q., et al. (2022). Linking microbial population succession and DOM molecular changes in Synechococcus-derived organic matter addition incubation. Microbiol. Spectr. 10:e0230821. doi: 10.1128/spectrum.02308-21
Ward, N. D., Bianchi, T. S., Medeiros, P. M., Seidel, M., Richey, J. E., Keil, R. G., et al. (2017). Where carbon goes when water flows: carbon cycling across the aquatic continuum. Front. Mar. Sci. 4:7. doi: 10.3389/fmars.2017.00007
Waska, H., Simon, H., Ahmerkamp, S., Greskowiak, J., Ahrens, J., Seibert, S. L., et al. (2021). Molecular traits of dissolved organic matter in the subterranean estuary of a high-energy beach: indications of sources and sinks. Front. Mar. Sci. 8:7083. doi: 10.3389/fmars.2021.607083
Wen, S., Lu, Y., Luo, C., An, S., Dai, J., Liu, Z., et al. (2022). Adsorption of humic acids to lake sediments: Compositional fractionation, inhibitory effect of phosphate, and implications for lake eutrophication. J. Hazard. Mater. 433. doi: 10.1016/j.jhazmat.2022.128791
Wilson, H. F., and Xenopoulos, M. A. (2008). Effects of agricultural land use on the composition of fluvial dissolved organic matter. Nat. Geosci. 2, 37–41. doi: 10.1038/ngeo391
Keywords: DOM, river networks, FTICR-MS, molecular composition, random forest, cluster analysis, ecosystem properties, unsupervised machine learning
Citation: Müller M, D’Andrilli J, Silverman V, Bier RL, Barnard MA, Lee MCM, Richard F, Tanentzap AJ, Wang J, de Melo M and Lu Y (2024) Machine-learning based approach to examine ecological processes influencing the diversity of riverine dissolved organic matter composition. Front. Water. 6:1379284. doi: 10.3389/frwa.2024.1379284
Edited by:
Shai Arnon, Ben-Gurion University of the Negev, IsraelReviewed by:
Alain Isabwe, University of Michigan, United StatesSusanne Stadler, Federal Institute For Geosciences and Natural Resources, Germany
Copyright © 2024 Müller, D’Andrilli, Silverman, Bier, Barnard, Lee, Richard, Tanentzap, Wang, de Melo and Lu. This is an open-access article distributed under the terms of the Creative Commons Attribution License (CC BY). The use, distribution or reproduction in other forums is permitted, provided the original author(s) and the copyright owner(s) are credited and that the original publication in this journal is cited, in accordance with accepted academic practice. No use, distribution or reproduction is permitted which does not comply with these terms.
*Correspondence: Moritz Müller, bW11ZWxsZXJAc3dpbmJ1cm5lLmVkdS5teQ==