- School of Civil Engineering, Vellore Institute of Technology, Vellore, India
Urban lakes provide crucial ecological, social, and economic benefits, but rapid urbanization and land use changes have significantly compromised their health and sustainability. This study investigates the interrelations among land use, temperature, and water quality in Vellore lakes (1997–2024) to address the sustainable development goals (SDG) 6.4 (water) and 13.3 (climate resilience). Satellite derived data revealed a direct correlation between a reduced water spread area (1.82%) and increasing LSTs, underscoring the adverse effects of urbanization. Notably, 64% of dissolved oxygen (DO) of the samples surpassed permissible limits. Hardness, electrical conductivity (EC), total dissolved solids (TDS) and DO emerge as the primary influencers of the Water Quality Index (WQI). It was validated by the XGBoost model. Based on WQI most of the lake water classified as “Poor” for drinking. Principle component analysis revealed 68% of the variance in water quality with main factors as LST, built-up, and vegetative cover. Furthermore, suggesting that implementing 30% green buffers, restoring 2–5% WSA, and adopting climate-smart urban planning could lower LST by 1.8°C. These science-driven strategies align with the SDGs and India’s Smart Cities Mission, offering a pathway to harmonize urbanization with ecological resilience and safeguard lake biodiversity under a changing climate.
1 Introduction
Water is the most valuable heritage of our planet. Water bodies or hydroscapes play a critical role in conserving diverse ecosystem services, including biodiversity preservation, climate modulation and regulation, carbon footprint sequestration, groundwater replenishment, water supply sustainability, precipitation patterns, river network vulnerability, sediment network, aquatic and terrestrial food chains (Paná et al., 2024; Jeppesen et al., 2009; Singhal et al., 2024; Abed-Elmdoust et al., 2016; Gao et al., 2022; Sarker et al., 2019). However, global trends such as human development, population expansion, migration and climate change pressurizing on quality and quantity of water resources, potentially influencing ecosystems (WHO, 2021, 2022). Similarly, temperature is an important factor for surfacescapes (lakes, tanks, and rivers) impacts aquatic ecosystem by affecting DO levels (DO and EPA, 2021), water quality, and evaporation rates (US Geological survey, 2018). Human-induced alterations to urban surfacescapes compromise environmental quality, climate change, and undermine the provision of essential ecosystem services for nearby communities (Patro et al., 2024). This explains how these environmental and anthropogenic factors affect water bodies.
Optimal temperature regulations and strategic land use planning are interdependent factors sensitive to human-induced changes in waterscapes, which are recognized as a global problem with significant consequences for the environment (Punja et al., 2024). Addressing water quality interlinkage requires expert intervention at all levels, from municipal level to global level. This is an important gap in monitoring developing countries, where the connection between water quality and surrounding land use can contribute to achieve multiple SDG (SDG 6, 3, 11, 12, 13; SDG, 2022) targets (Alcamo, 2019). With the increase in population, understanding how land use changes and temperature fluctuations induced by climate change affect water quality is essential. Water does not have an exact temperature range, but the seasonal fluctuations, lake morphometry, latitude, longitude, and surrounding landscape factors influence temperature of each lake (Fondriest Environmental, Inc, 2014). These changes in LULC and temperature directly impact hydrological sources across diverse landscapes, exacerbating non-point source pollution and ultimately degrading surface water quality through increased runoff, sedimentation, and nutrient loading (Umwali et al., 2021).
Two influential factors that significantly impact water quality are land use and temperature. Urbanization, characterized by increased built-up areas and reduced vegetation, alters the natural hydrological cycles, leading to greater runoff, flooding, and contamination rates that add nutrients into water bodies. Water quality in small (<0.5 hectares) or shallow (<2 m deep) lakes and reservoirs is sensitive to their immediate surroundings (Bettina et al., 2016; Singh et al., 2024), often resulting in non-point source pollution. At the same time, rising temperatures exacerbate these issues by accelerating evaporation, reducing DO levels, and promoting algal blooms, which further degrade water quality. Warmer temperatures and reduced inflow can lead to oxygen depletion in stratified reservoirs and lakes, creating favorable conditions ideal for naturally existing opportunistic waterborne pathogens (Weiskerger et al., 2019; Yaghouti et al., 2023). Recent research highlights that the combination of these factors creates complex systems and needs detailed study. While previous studies acknowledge the individual roles of land use and temperature in water quality deterioration, their synergistic consequences in rapidly urbanizing regions of the Global South unexplored. Understanding this interaction is crucial for developing effective water management strategies and mitigating the adverse effects of urbanization and climate change on water quality (CRDP, 2019).
This study addresses this gap by integrating LULC, LST, and water quality parameters in Vellore, India. Methodologically, combines satellite data (1997–2024), water quality index, machine learning, and multivariate statistics to analyse the interdependencies between satellite observations with ground-truth water chemistry. Examining the interdependency between these variables is essential to predict future trends and design mitigation strategies. Vellore, a rapidly urbanizing region in southern India. Vellore has been identified by the World Bank as one of the three cities in Tamil Nadu to be developed as a model city for advanced reforms in areas like revenue, grievance redressal, and systems for personnel, hospitals, and schools (Vellore and Smart City, 2016). The city’s proximity to Bengaluru and Chennai, along with its presence of a thriving leather industry, has significantly contributed to rapid growth. The city’s current land use reflects a balanced mix of residential, commercial, and institutional zones, and the city attracts a significant tourist presence, with an average footfall of 2.2 Lakh people growing at a rate of 3% annually (Vellore and Smart City, 2016). The city experiences a semi-arid climate, characterized by consistently high temperatures and relatively low rainfall. Understanding these dynamics is crucial not only for local water resource management but also for developing broader guidelines applicable to urban areas facing similar challenges.
Future models and research indicate that around 80% of the global population is projected to live under SSP (shared socioeconomic pathways) scenarios, facing significant multi-pollutant challenges, particularly in highly urbanized areas. River pollution in Southeast Asia is expected to be especially severe (Strokal et al., 2021). Global river basins are projected to face a threefold increase in water scarcity due to future pollution, emphasizing the growing environmental crisis (Wang et al., 2024). Studies have shown that increasing temperatures can lead to reduced surface water volumes, especially during summer months due to higher evaporation rates (Tong et al., 2023). Global lake warming is anticipated to continue unless significant reductions in greenhouse gas (GHG) emissions are achieved (Tong et al., 2023). In alignment with the 2030 agenda for sustainable development, addressing the impacts of temperature and land use on water quality is crucial to meeting SDG 6.4 (water use and scarcity; UN-Water, 2024) and SDG 13.3 (climate action; SDG, 2023) for progressive global targets (SDG, 2022; Jeffrey et al., 2024). The Environmental Protection Agency (EPA) quality criteria explains the importance of temperature layers and turnover in lakes, which are vital for sustaining aquatic species (EPA, 2021).
Previous studies have alerted the significant influence of land use and temperature on water quality, stressing the need to address these factors in future management plans to maintain ecological balance. Given the anticipated impacts of climate change and growing land-use pressures, addressing this issue is even more crucial (Murdoch et al., 2000). Consequently, numerous studies have investigated the relationship between water quality, land use, and temperature, concluding that significant relationship exists between these factors at different scales. The effects of climate change on water quality in North America (Lake trout; Guzzo et al., 2017), as well as Spain and United Kingdom with reduced surface water volumes documented (Figueras and Borrego, 2010; David, 1997; Kernan et al., 2010). Human intervention and drought have compromised high TDS, TSS, and temperature in Lake Mead’s, Las Vegas (Adjovu et al., 2023). Agricultural runoff and urban sprawl were identified as the primary drivers of water quality changes in the Suquía River Basin, Argentina, significantly impacting urban health (Paná et al., 2024). Similarly, Lake Muhazi is seasonally impacted by anthropogenic activities, particularly during the rainy season (Umwali et al., 2021), and historical changes in LSWTs and water quality in Dianchi Lake has a direct impact on water quality (Yang et al., 2018). Diverse water trajectories accumulate several contaminants due to urbanization affecting global water quality in the 21st century (Strokal et al., 2021). Natural water environments, including recreational waters, are changing due to climate change, with rising temperatures observed in many regions (WHO, 2021). Overall, these findings highlight the importance of considering land use and temperature in managing water bodies, especially where urban environment offer a realistic influence on the deterioration of water quality (Umwali et al., 2021). Addressing these factors is essential for protecting aquatic ecosystems and ensuring the health of our water resources from the perspective of climate change.
The objective of the research is to analyze the interlinkage of temperature and land use on water quality in urban lakes in Vellore. (1) To utilize satellite data to assess LULC and LST dynamics for the study area. (2) To evaluate the temporal trends in water surface area (WSA), LULC, and LST to identify drivers of water quality degradation. (3) To evaluate water quality parameters and predict WQI using the XGBoost model, correlating with physicochemical parameters. (4) To investigate the interrelations among water quality parameters, land use, and temperature variables using PCA analysis to understand their collective impact and recommend sustainable water management in urban alliances.
2 Materials and methods
2.1 Study area
Vellore is a key gateway into northeastern Tamil Nadu, India (Figure 1). The geographical coordinates of the region of interest aligned with latitudes 12° 15’ N and 13° 15’ N and longitudes 78° 20′E and 79° 50′E. Vellore spans a total land mass of approximately 741.18 km2, which is irregular in shape and has an elevation at an average of 283 meters. The weather in Vellore, characterized as tropical savanna climate, is one of the hottest and most humid in the region (Wladimir, 2018; Beck et al., 2018). During the summer, the temperatures reach up to 45°C, while the mean temperature drops to around 20°C in winter months. Vellore holds an annual rainfall of 1,034 mm, with nearly 90% occurring as rain and occasional drizzles, classified as a semi-arid region with frequent droughts and occasional floods (IMD, n.d.). Vellore’s current land use displays a mixture of urban, agricultural, institutional, health, and industrial sectors, along with a growing population density of approximately 2.3 times the expansion of built-up areas. The study area encompasses several urban lakes, including the prominent Palar River and its tributaries. This study considers five lakes in Vellore, each with unique characteristics. These lakes (Figure 2) are shallow, with long, thin, and varied structures, namely Sadupperi lake, Thorapadi Lake, Kalinzur Lake, Dharapadavedu, Lake and Kavannur Lake. These lakes are integral to the aquatic resources, water supply, agriculture, and parts of Vellore natural ecosystem also differ by land use types. Sadupperi lake, located on the outskirts of Vellore, has a catchment area of 69.54 sqkm. It receives runoff from surrounding urban areas and is connected to the Palar River. Thorapadi Lake, situated in the western part of Vellore, has a catchment area of 68.35 sqkm and is located adjacent to Sadupperi Lake and the Sadupperi dump yard. Kalinzur Lake (23.27 sq. km) and Dharapadavedu Lake (51.78 sq. km) are key urban lakes in Katpadi, primarily maintained in the urban landscape. Kavannur Lake, with a catchment area of 52.52sqkm, located in the western region of Vellore. It mainly receives agricultural runoff from surroundings and a prime connectivity to the Palar tributary. These lakes, often referred to seasonal lake-reservoirs or tanks (Eri in Tamil), are detailed in Table 1. They are characterized as non-system tanks in Vellore, typically standalone water bodies (TNUIFSL, 2006; Malarvizhi et al., 2022; Vellore CCBN, 2024). These lakes play a crucial role in maintaining Vellore’s environmental health and sustainability. An integrative review methodology (Whittemore and Knafl, 2005) was adopted to synthesize multi-disciplinary studies on land use, temperature, and water quality. This approach prioritized contextual gaps in identification of synergies between hydrological, climatic, and anthropogenic drivers, detailed methodological flow is depicted in Supplementary Figure S1.
2.2 Field research: field sampling and sample analysis
Water sampling was conducted at specific locations based on a preliminary study. In this research, 25 monitoring sites were considered based on the data availability, accessibility, and full extents of lakes. Sampling was carried out monthly from August 2023 to August 2024. Prewashed, high-density, 1 L capacity polythene bottles were used to collect samples at different depths and preserved as per IS 3025 (BIS 1987, n.d.).
Physio-chemical parameters were measured in the field using handheld portable instruments to record pH, DO, TDS, and temperature to obtain real-time and with natural variability. Integrated sampling was followed, and the samples were preserved and analysed at the Environmental Laboratory, VIT, Vellore. The laboratory examination of physico-chemical characteristics includes electrode-based pH in samples, the Nephelometric Method (NTU) for measuring water cloudiness, and the EDTA titrimetric method for estimating calcium (Ca) and magnesium (Mg). Furthermore, bicarbonate (HCO3) and carbonate (CO3−2) by titration with H2SO4, using methyl orange and phenolphthalein as indicators. The AgNO3 (Mohr’s) technique for determining chloride (Cl) concentration. The double-beam UV spectrophotometer was used in determining nitrate (NO3), sulphate (SO2−₄), and fluoride (F) concentration (Loganathan and Sathiyamoorthy, 2024). Azide variant of the iodometric method (Winkler’s method) for determining dissolved oxygen (DO; DO and EPA, 2021), and biochemical oxygen demand. Tables 3 and 4 provide a statistical summary of water quality indicators and standards.
2.3 Calculation of water quality index
The WQI model is recognized techniques for assessing water quality to know the overall condition of water resources. By converting large amounts of water quality data into a single, interpretable index score (Uddin et al., 2023; Auddy et al., 2023; Kangabam et al., 2017). In this study, 14 parameters were selected based on their importance in water quality standards, with reference to the guidelines of the World Health organization (WHO; WHO, 2022; BIS: IS 10500, 2012) and the Indian Standard for drinking water quality (BIS). The calculation involved the following steps by assigning weightage (AW) ranging from 4 to 1 with reference to previous studies (Auddy et al., 2023; Kangabam et al., 2017; Supplementary Table S1). The relative weight was calculated based on Equation1.
RW = relative weightage, AW = assigned weightage for each parameter and n = number of parameters.
The quality rating scale (Qi) for each parameter is determined by comparing its concentration in the water sample to the corresponding standard value (WHO, 2022; BIS: IS 10500, 2012)(Equation 2).
From (Equation2) the pH and DO the quality rating calculated on basis as given below (Equation 3).
Here, Qi represents the quality rating for the parameter, Ci is the concentration of the parameter in the water sample, Si is the applicable standard for that parameter (Equations 1 and 4). Vi as the ideal value for pH = 8.5 and DO = 6.
The calculated WQI values were classified based on the categorization proposed by (Kangabam et al., 2017). To investigate potential sources of pollution in Vellore lakes, XGBoost was employed.
2.4 Satellite data collection
The data collection comprised various publicly accessible geographical datasets utilized for analysis. The primary data source was the US Geological Survey (USGS) platform, which provided GeoTIFF format for years 1997, 2010, 2020, and 2024. Data accuracy was ensured by selecting only imagery with 0% cloud cover. Table 2 presents the satellite datasets used in this study. Secondary data collection was obtained from Googe Earth Engine (GEE) TERRA dataset, from which LST variations were extracted. Additionally, Open Street map (Geofabrik) data for south zone utilized. The methodological flow is depicted in Supplementary Figure S1.
2.5 LULC analysis
The study collected 0% cloud cover Landsat scenes (path/row: 143/051) from the USGS EROS Center Open Server (Table 2). The LULC classification setup followed urban land use Level 1 training classes outlined in National Urban Information System India (NUIS) guidelines (NIUS, 2006), FAO guidelines (James et al., 1976; FAO, 2000). Land use training statistics for the study area and lakes WSA are presented in Supplementary Tables S2 and S3. LULC analysis was conducted in 2 stages. Stage 1 involved supervised image classification using a support vector machine (SVM) algorithm in ArcGIS 10.8.2 for the study area (Supplementary Table S2). Stage 2 extracted the land use of the lakes using SVM from Landsat 8 and 5 datasets for years 1997, 2010, 2020, and 2024, classifying the surrounding WSA into five classes: built-up areas, water bodies, flooded regions, barren lands, and vegetation (Supplementary Table S3).
2.6 Accuracy assessment
To evaluate the reliability of our LULC classification, an accuracy assessment was performed by comparing the classified results with Google reference data. A total of 50 random points for each WSA were selected to construct a confusion matrix, which was then used to calculate key metrics including overall accuracy and the kappa coefficient.
2.7 Analysis of LST
The study utilized the Google Earth Engine (GEE) to extract monthly mean LSTs using C6 daily Moderate Resolution Imaging Spectroradiometer (MODIS) terra satellite product MOD11A1 (MODIS; MODIS, 2020; GEE, 2022) for the Vellore (ROI) limits at a 1,000-meter grid scale. A cloud filtering algorithm (QA_PIXEL) was applied to remove the unwanted elements from the filtered images. The dataset was filtered for specific time periods (ee.Filter.date) to isolate data for the desired months and years. The ‘LST_Day_1km’ band was then used to calculate LST by averaging all daily images. Furthermore, the reduceRegion() to convert temperatures from Kelvin to degrees Celsius by using a scaling factor of 0.02 and 273.15, over 2001–2024.
The lake’s land use is articulated as WSA in the study. Additionally, LST of all lakes was estimated using thermal bands (Bands 6, 10, and 11) from the Landsat series for the years 1997, 2010, 2020, and 2024. The LST extraction for Landsat 8 and 5 differed by employing a map algebraic tool in ArcGIS 10.8.2 to convert spatial sensor thermal infrared raster data from Kelvin to Celsius. The split window algorithm is utilized to retrieve accurate LST values. A detailed description of this methodology, including the analysis techniques employed in this investigation, is detailed by (Manjunath and Jagadeesh, 2024).
2.8 Statistical and correlation analysis
Water quality evaluations were performed using Microsoft Excel. To predict water quality, various machine learning models employed Bootstrap Forest, Boosted Tree, Extreme Gradient Boosted Trees (XGBoost), and Neural Networks (Roberts and Damon, 2019). XGBoost was selected due to its high accuracy reported in several published studies (russ_wolfinger, 2020). Hence, an XGBoost add-in was considered for analysis, performing particularly well during both training and validation phases of WQI prediction. A multivariate correlation analysis was conducted to examine interactions among water quality parameters and to identify the constituent sources across all lakes. Further, Principal Component Analysis (PCA) was applied to assess temporal variations influencing water quality and to explore associations between land use, water quality parameters, LST, and temperature.
3 Results and discussion
3.1 LST evolution
MODIS-derived LST data were extracted from GEE for the study area covering from 2001 to 2024. The mean monthly LST for each year is presented in a nested bar chart (Figure 3). The average annual LST values varied initially, starting at 32.60°C and increasing linearly to 35.28°C, indicating a consistent increase of 2.68°C over the period. This translates to an annual average increase of 0.099°C per year. Figure 3 shows that the LST increase is more pronounced during summer months, typically from March to July. Within this period, the coldest year was 2001, and the hottest year is 2023. The highest LST values were recorded in March (37.06°C to 40.79°C), April (36.90°C to 40.95°C), and May (37.04°C to 40.87°C), with extended heat months observed near the borders of the defined months. A notable trend indicates LST reflecting an overall upward pattern. These findings suggest that urban expansion and associated land cover changes (Manjunath and Jagadeesh, 2024) contribute to the observed increase in LST, particularly in urban area experience higher LST compared to rural areas.
3.2 Land use analysis
The current land use map of Vellore, presented in Figure 4, shows the distribution of various land cover types. The overall accuracy of classification was 84% with a kappa coefficient of 0.76.
In Figure 4, waterbody (1.82%), built-up (28.26%), vegetation (18.76%), flooded area (1.20%), agriculture (30.25%), barren land (14.17%), and range land (13.79%) are identified land use percentages. These data highlight that agriculture dominates the landscape, while built-up areas account for nearly one-third of the urbanized space. The low percentage of WSA suggests that improved water management practices are needed.
The framework aims to balance urban expansion with environmental sustainability. In the specific case of Vellore, green spaces should ideally account for 10 to 30% of the urban area to ensure liveability and biodiversity, while waterbodies should be maintained at around 2–5% to support water management and ecological balance. These recommendations align with international guidelines and best practices for sustainable urban development (Syrbe and Chang, 2018; Rudd et al., 2018; IGUTP, 2015; UN habitate, 2010; NIUS, 2006).
3.3 Interaction between land use and LST in lakes WSA
The study area encompasses five primary lakes, namely Sadupperi, Thorapadi, Kalinzur, Dharapadavedu, and Kavannur, which serve as the focal points for this investigation. A temporal assessment of land use and LST from 1997 to 2024 reveals profound effects on the lakes WSA and local microclimates.
The land use in the Sadupperi lake area has undergone considerable changes between 1997 and 2024. Natural habitats, livelihood, and green stocks covered in the WSA have declined abruptly. Between 1997 and 2010, the average LST in Sadupperi WSA dropped by 2.735°C; however, an increase was observed from 2010 to 2024, reflecting a warming trend (Figure 5). The lake’s WSA has substantially decreased during this period, with notable encroachments in the northern and western parts, leading to rising local temperatures affecting the surrounding banks. Ongoing urban development is compromising the lake’s ecological integrity.
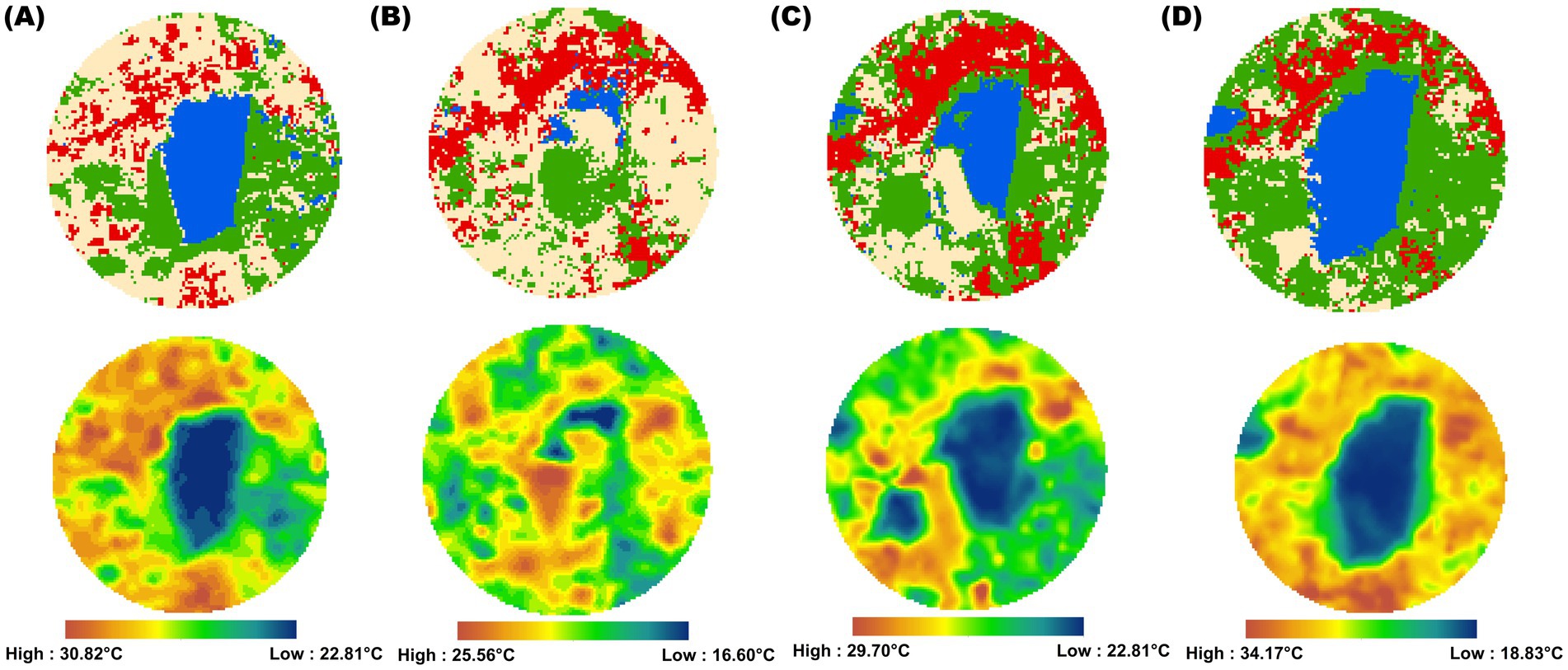
Figure 5. Temporal analysis of land use and LST in Sadupperi WSA for (A) 1997, (B) 2010, (C) 2020 and (D) 2024.
Thorapadi’s WSA, located adjacent to Sadupperi lake, shows a significant temporal evolution of land use patterns (Figure 6). Notably, barren lands have been progressively been replaced by built-up areas along the east-to-south parts of WSA. The annual average LST stats in Thorapadi decreased by 2.335°C from 1997 to 2010, then increased by 2.525°C by 2024, indicating a severe warming trend. Urban encroachments around Thorapadi are replacing natural habitats with development activities, elevating the thermal signature of the region.
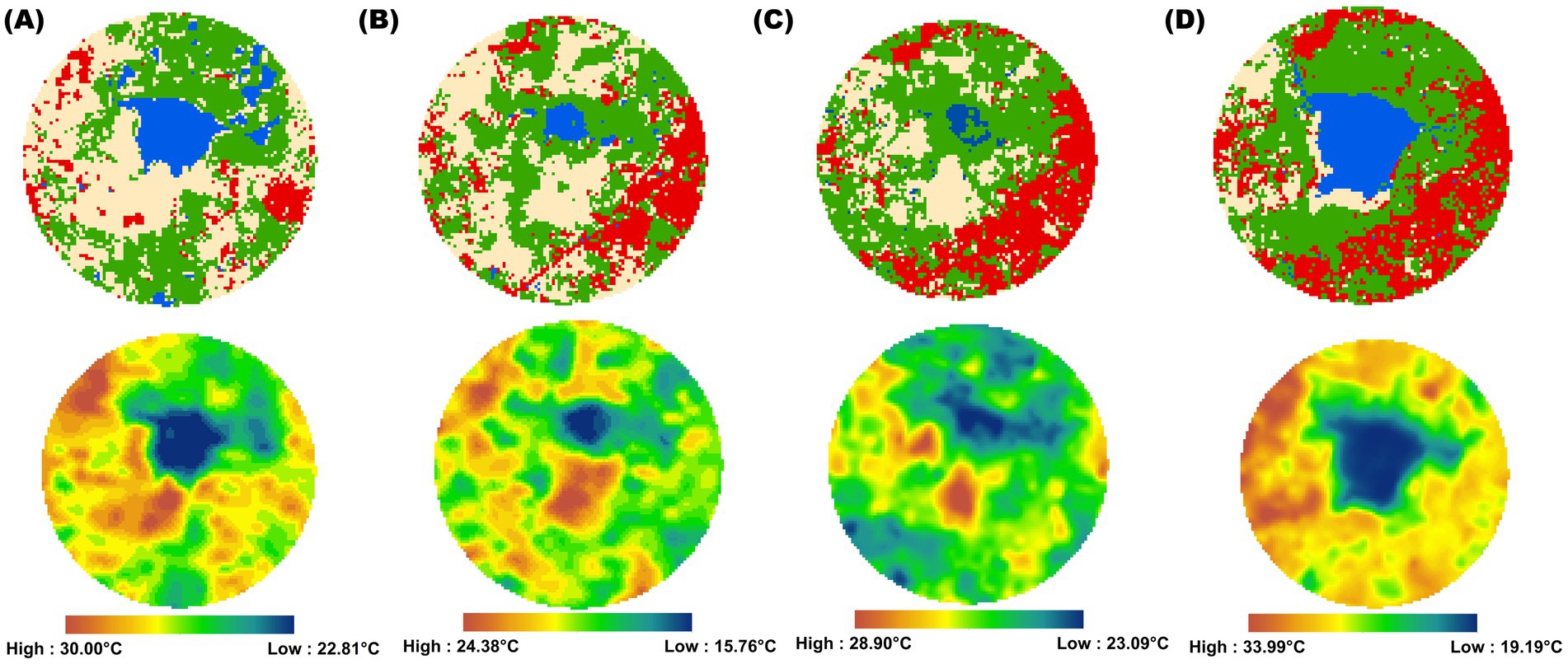
Figure 6. Temporal analysis of land use and LST in Thorapadi WSA for (A) 1997, (B) 2010, (C) 2020 and (D) 2024.
The WSAs of Kalinzur and Dharapadavedu are interconnected within the growing urban landscape of Katpadi. Figures 7, 8 illustrate the lakes experienced significant developmental pressure (northeast region to south sectors), with green and blue belts diminishing over time. Both the lakes saw reductions in LST between 1997 and 2010, followed by a sharp increase from 2010 to 2024. The average temperature change observed in Kalinzur (2.85°C) and Dharapadavedu (2.72°C) illustrates the magnitude of urban pressure on these water bodies.
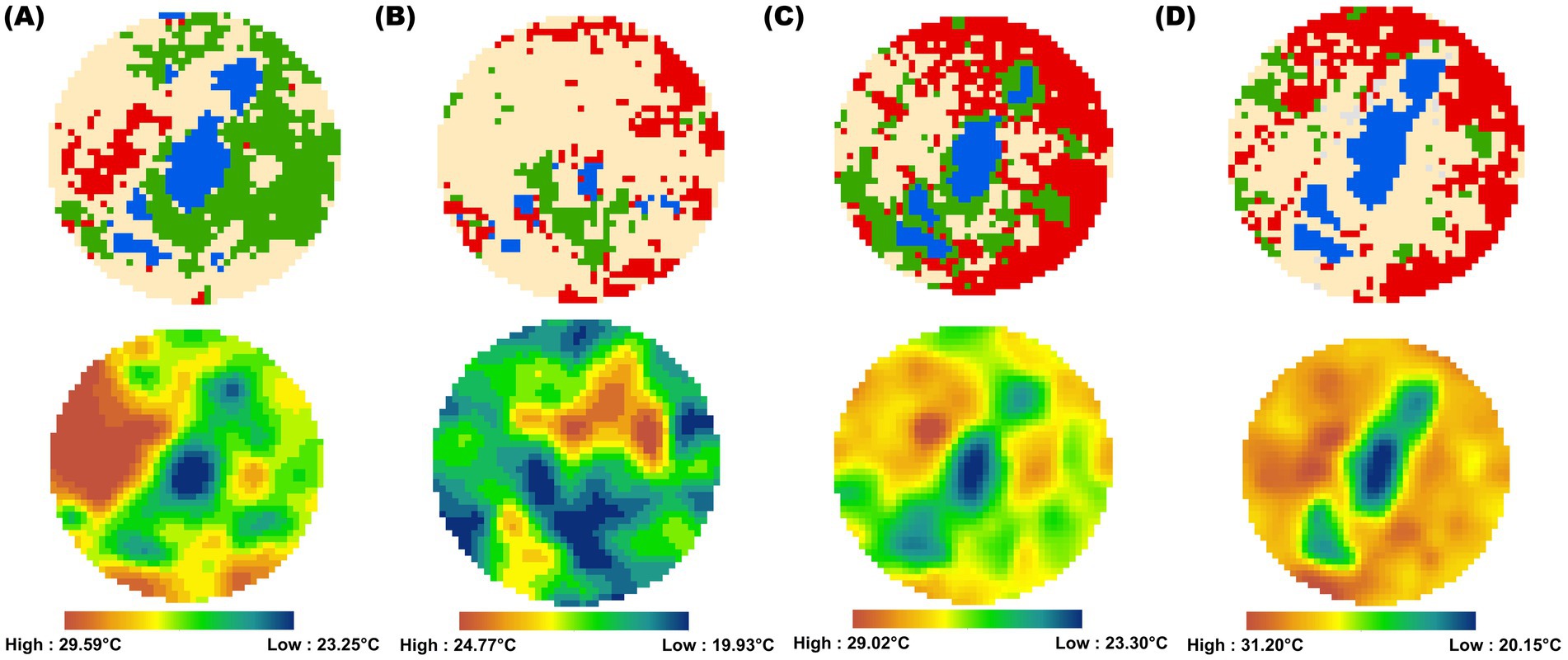
Figure 7. Temporal analysis of land use and LST in Kalinzur WSA for (A) 1997, (B) 2010, (C) 2020 and (D) 2024.
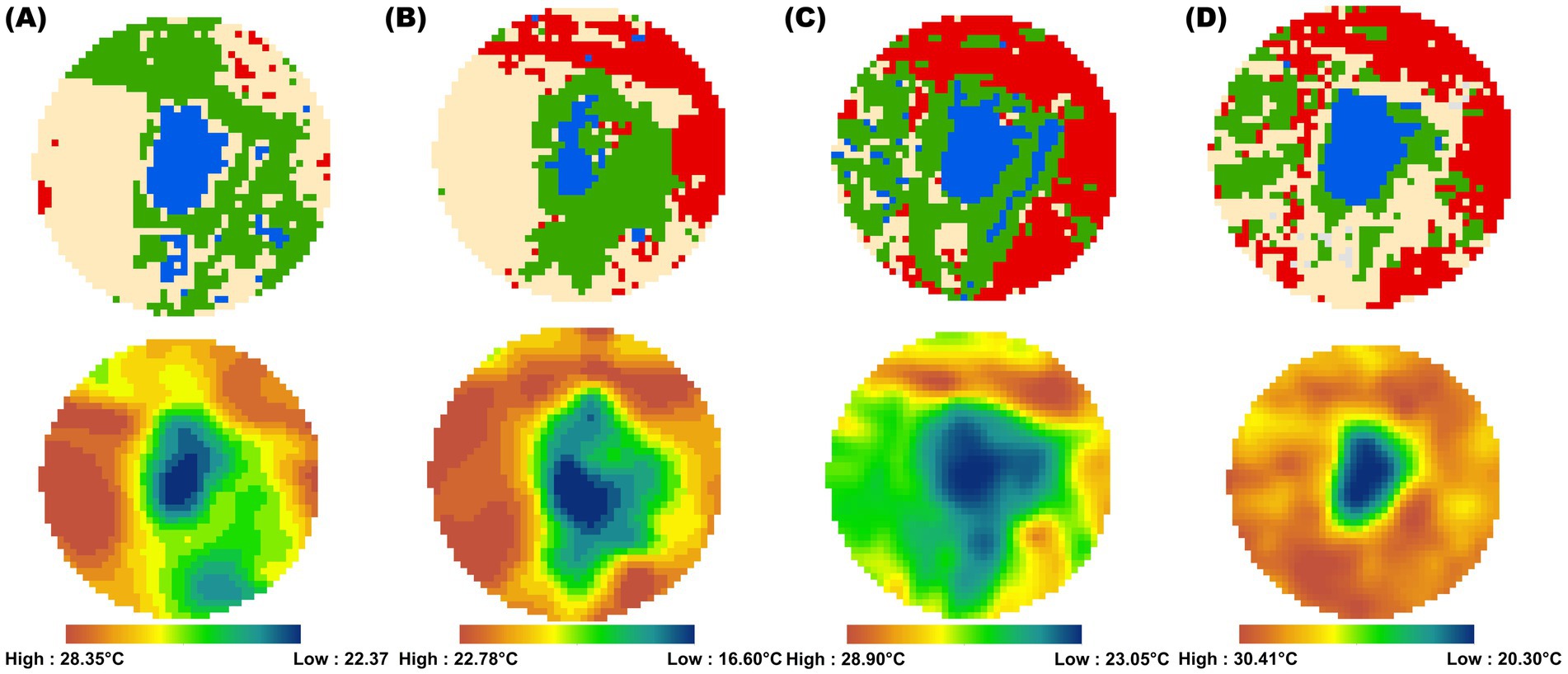
Figure 8. Temporal analysis of land use and LST in Dharapadavedu WSA for (A) 1997, (B) 2010, (C) 2020 and (D) 2024.
The temporal analysis of Kavannur WSA from 1997 to 2024 is represented in Figure 9. Kavannur is located near the rural zones of Vellore along the banks of the Palar River. Agricultural activities dominate the landscape, with minimal build-up observed between 1997 and 2024. However, slight urban growth occurred in the northern region along the national highways post-2010, contributing to a moderate rise in LST. The southern profile of Kavannur WSA, influenced by Palar River with a flooded area, corresponds with higher LST. Between 2010 and 2024, the average LST in the WSA increased by 4.89°C. Agricultural areas maintained relatively stable LSTs (28.5–30.5°C), while urban areas exhibited higher LSTs (30–32°C). Unlike other WSAs, Kavannur shows less urbanization, although the increase in LST may be linked to the loss of green and blue spaces around the lakes and dry zone of Palar River, which impact the surrounding WSA.
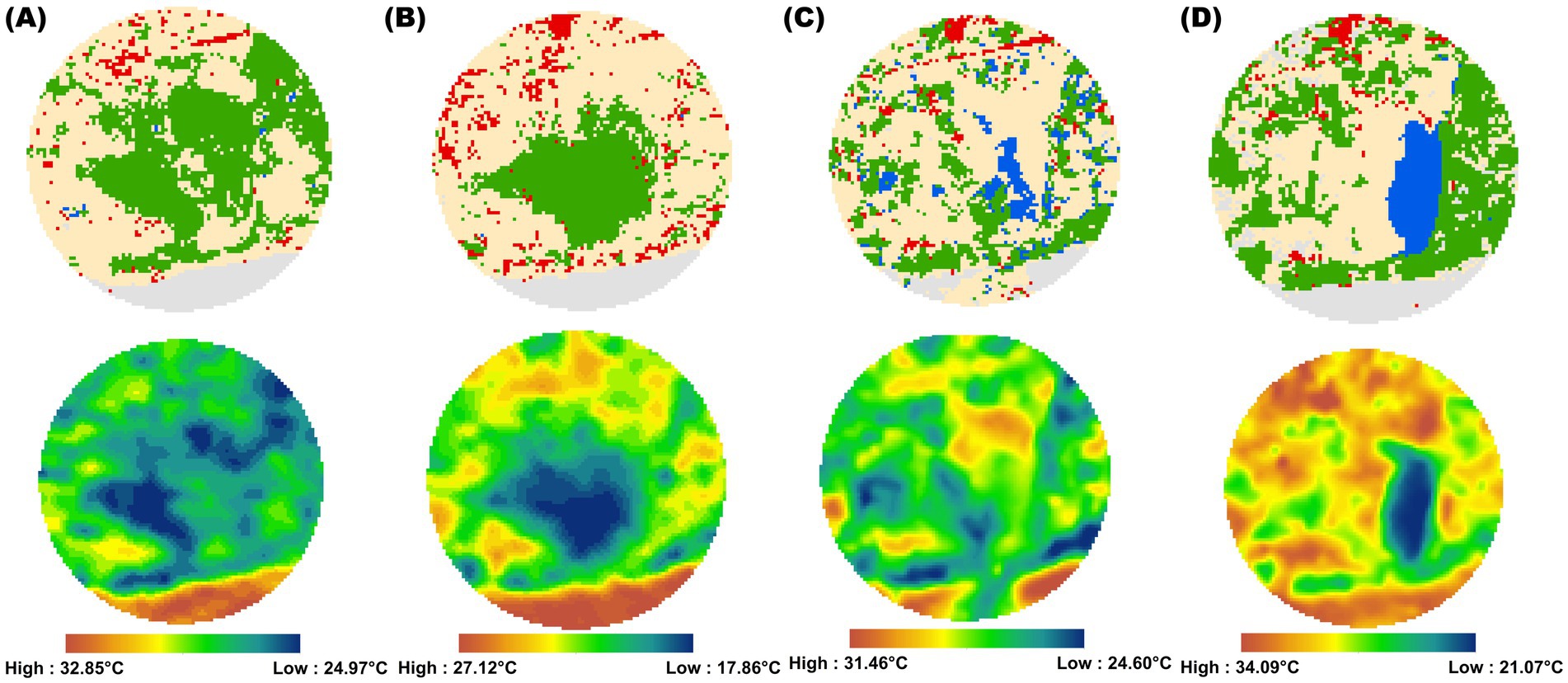
Figure 9. Temporal analysis of land use and LST in Kavannur WSA for (A) 1997, (B) 2010, (C) 2020 and (D) 2024.
3.4 Water quality parameters in the lakes
The water quality analysis was performed on 150 samples collected from Sadupperi, Thorapadi, Kalinzur, Dharapadavedu, and Kavannur Lakes between 2023 and 2024 (Table 3). The assessment revealed pH levels ranged from highly acidic (3.69) to highly alkaline (9.60), with a mean value of 7.69 and a coefficient of variation (CV) of 12.29%. This indicates overall neutral to slightly alkaline conditions across the lakes, although localized acidification, possibly from runoff. Total dissolved solids (TDS) concentrations varied significantly from 112 mg/L to 2,700 mg/L, suggesting contamination from agricultural runoff or sewage discharge. Hardness levels ranged from 210 to 1,200 mg/L, averaging 457.84 mg/L, with high concentrations of calcium (23.15–450 mg/L) and magnesium (3.81–274.50 mg/L), indicating potential leaching from calcareous soils or industrial effluents. Mean chloride and sulphate levels were 411.69 mg/L and 239.47 mg/L respectively, further pointing to sewage contamination and agricultural runoff, while significant variability in sulphate and nitrate levels underscores the impact of fertilizer runoff. DO levels ranged from 0.01 mg/L to 6.50 mg/L, suggesting a high rate of organic matter decomposition and inadequate aeration, often linked to untreated sewage. Biochemical Oxygen Demand (BOD) levels varied from 0.23 mg/L to 15.20 mg/L, indicating excessive microbial activity in some locations. Alkalinity ranged from 50 mg/L to 995 mg/L, revealing that some lakes possess buffering capacity against pH changes, although high alkalinity could also indicate contamination from domestic or agricultural sources.
In terms of water quality standards (Table 4), 64% of the samples lack sufficient DO levels. Additionally, 55.2% of samples overreached permissible limits for conductivity, 36% recorded BOD concentrations above the acceptable limit, 38.4% of turbidity, and fluoride levels were above safer limits. These findings underscore the influence of both natural and anthropogenic factors on lake water quality, with agricultural runoff, sewage discharge, and nearby small-scale industrial contamination being the likely primary sources of pollutants, which are influenced by surrounding land use practices.
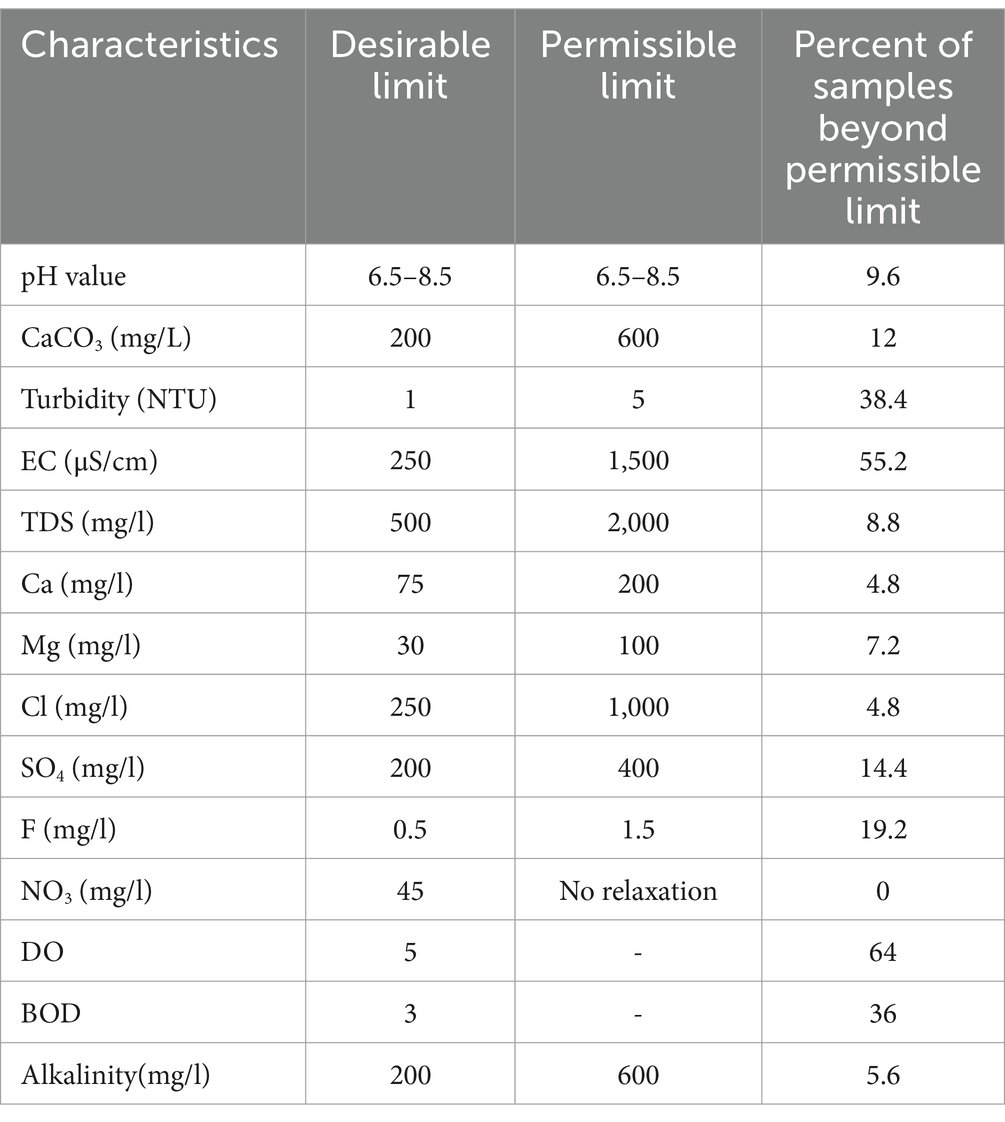
Table 4. Drinking water quality standards as per (CPCB, 2019; BIS: IS 10500, 2012; IITK, n.d.)
The WQI (Equation 5) values provide a comprehensive indication of the suitability of lake water for drinking and other purposes (Loganathan and Sathiyamoorthy, 2024). Table 5 presents the WQI scale for Vellore lakes. Sadupperi Lake has a WQI range from 23.45 to 74.28, indicating a relatively good water quality profile, with most samples classified as “Good.” In contrast, Thorapadi Lake shows that the WQI ranged from 20.59 to 69.14, with most of the samples classified as “Good,” and 16% “Poor” and 8% “Very Poor.” Kalinjur Lake exhibits a WQI ranging from 19.22 to 105.07, with significant fraction of samples are “Poor” or “Very Poor.” Dharapadavedu Lake presents moderate degradation, with WQI ranging from 37.29 to 115.34, samples distributed among “Good,” “Poor,” and “Unsuitable.” Kavannur Lake faces challenges from agricultural runoff, with WQI ranges from 39.51 to 115.51, as overall water quality rates “Very Poor” or “Unsuitable.”
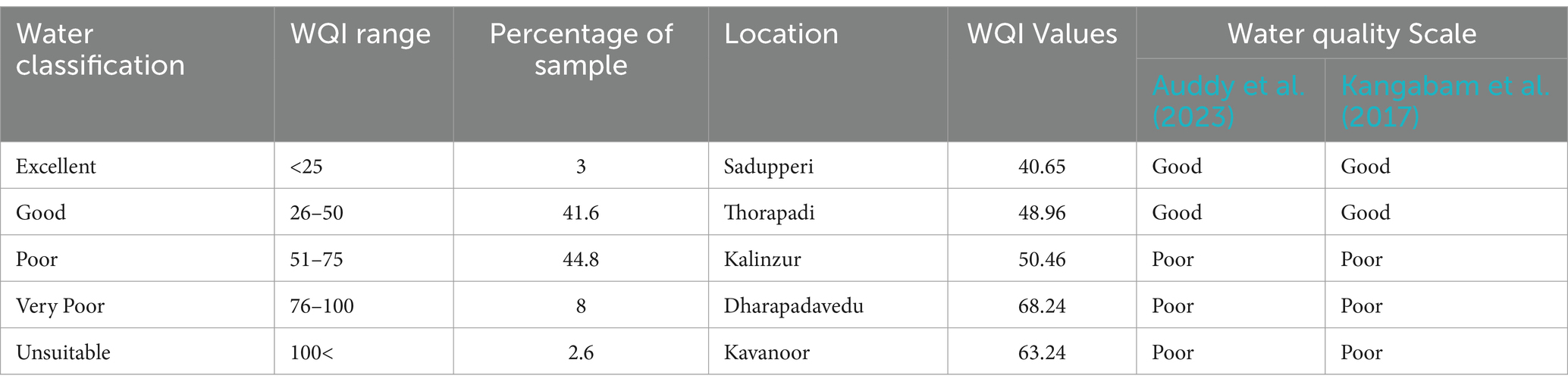
Table 5. Classification of lakes based on WQI (Kangabam et al., 2017).
The moderate range in water quality is observed mainly at Kavannur, Kalinzur, and Dharpadavedu lakes, primarily due to the runoff from surrounding urban and agricultural settlements. The results infer that water quality in some locations has declined significantly. Activities near these lakes, such as unauthorized sewage dumping, dump yards, biological waste, and river intervention, are reasons for the alarming deterioration of these water bodies. Consequently, the findings underscore the urgent need for sustainable urban planning, thermal regulation, nutrient management, and improved agricultural practices to protect and restore these vital water resources.
3.5 Water quality analysis using XGBoost
Comprehensive model screening applied to quantify the performance of five machine learning algorithms: Bootstrap Forest, Boosted Tree, Neural Networks, Generalized Regression Lasso, and XGBoost. These models were trained using 70% of the water quality data and validated on the remaining 30%, employing a 5-fold cross-validation method. Supplementary Table S4 presents the performance metrics of model screening (Figure 10), including R-square and mean root average squared error (RASE). Among the applied models, XGBoost emerged as the most suitable model for predicting WQI, displaying an exceptional R2 value of 0.992, indicating a strong correlation between the observed and predicted WQI values. Therefore, XGBoost was selected as the ideal model at handling complex data, making it most preferred method for WQI prediction over the other models.
The XGBoost model successfully predicted interaction WQI based on 14 key water quality parameters. The model performed exceptional performance on the training data set, with an R2 value of 0.99 (Figure 11A), indicating a near-perfect fit, and performed well on the validation data set by achieving an R2 value of 0.95 (Figure 11B), showcasing its accuracy in predicting WQI.
Feature importance analysis from the XGBoost model revealed that hardness, EC, TDS, chlorides, DO, and BOD are the most influential parameters significantly impacting the WQI (Supplementary Figure S2). Hardness is a key factor due to its high concentrations of calcium and magnesium ions, which can cause scaling and affect the usability of water for domestic and industrial purposes, as well as aquatic ecosystems. EC reflects the ion content and dissolved salts in water, which can originate from fertilizers, sewage, or industrial pollutants relating to water contamination. TDS represents the combined content of all inorganic and organic substances in the water, where high TDS can impair aquatic ecosystems and indicate contamination. Chlorides are commonly originated from domestic sewage, and agricultural runoff, affecting water salinity and quality. DO is a critical measure of water health, reflecting the availability of oxygen for aquatic organisms, with low DO levels often causing eutrophication and lower WQI. High BOD levels are directly linked to poor water quality, as they reflect the presence of untreated wastewater or runoff rich in organic pollutants, contributing to a lower WQI. This insight emphasizes the critical role these parameters play in assessing water quality and making them essential lake areas for water resource management and pollution control efforts.
The multivariate correlation matrices used to observe the interrelationship among all water quality parameters, with correlation coefficients ranging from +1 or −1, indicating strong and weak correlations (Webb, 2023). Sadupperi Lake (Figure 12A), located at the periphery of an urban zone. A significant positive correlation was observed between hardness and Ca (0.89) and Mg (0.64), as well as between TDS and chlorides (0.67). Turbidity exhibited a negative correlation with EC (−0.51), and WQI was strongly correlated with hardness (0.76) and turbidity (0.51). The Thorapadi lake (Figure 12B) water quality correlation matrix reveals an inverse relationship between pH and hardness, with positive correlations between turbidity and alkalinity (0.76) and between EC and chlorides (0.55). Interestingly, WQI showed a significant negative correlation with alkalinity (−0.67) and BOD (−0.99) and a positive correlation with calcium (0.90).
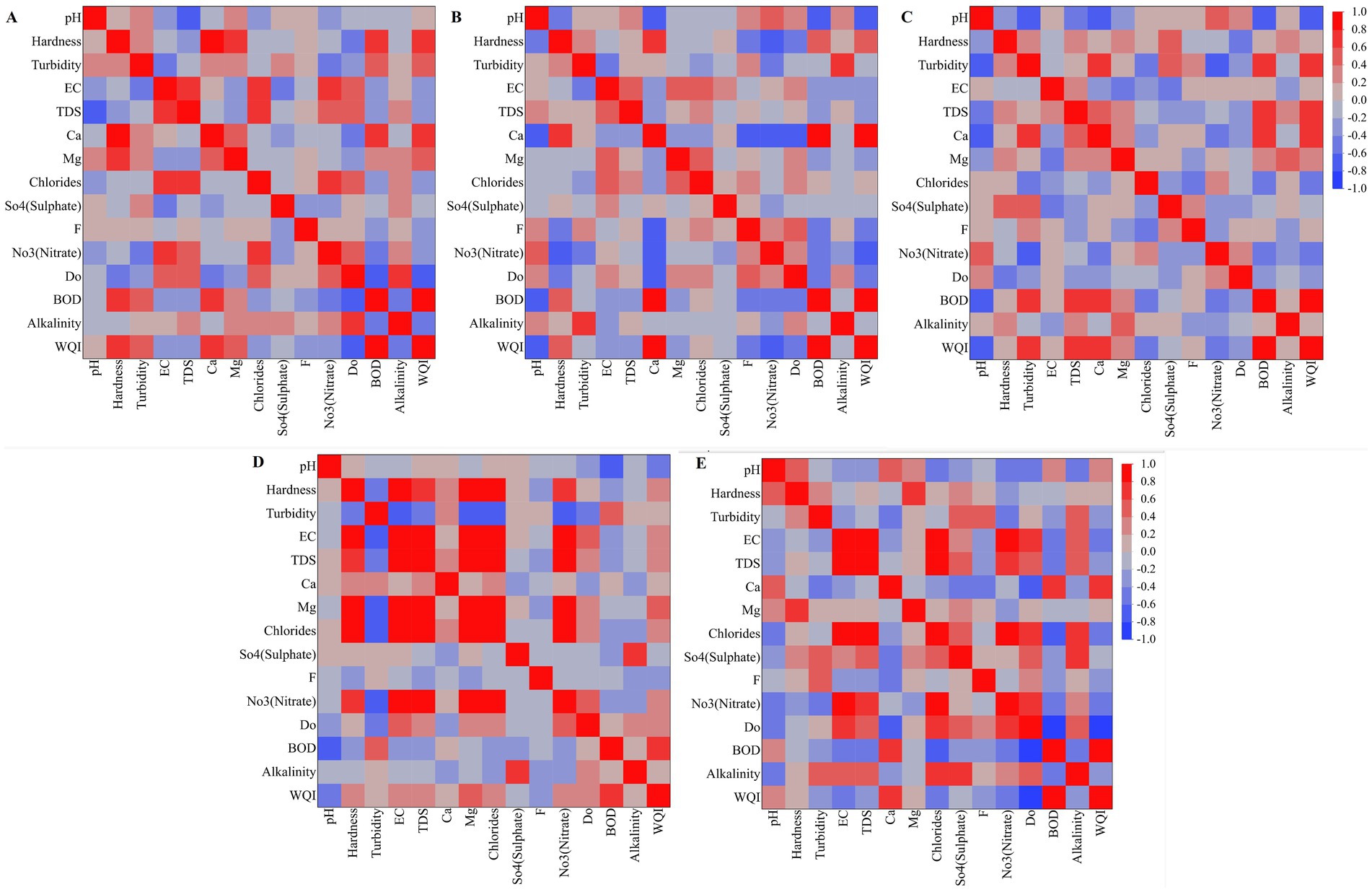
Figure 12. Multivariate interaction of water quality parameters (A) Sadupperi, (B) Thorapadi, (C) Kalinzur, (D) Dharapadavedu and (E) Kavanoor lakes.
The Kalinzur Lake (Figure 12C) is characterized by a strong correlation between turbidity and TDS (0.67), as well as moderate correlation with hardness (0.56) and EC (0.45), TDS and EC (0.51). BOD was very strongly negatively correlated with WQI (−0.99), reflecting that as BOD increases, water quality deteriorates. The Dharapadavedu Lake (Figure 12D) strong positive correlation between hardness and EC (0.83), TDS and EC (0.83), alkalinity and sulphates (0.66). A strong negative correlation is observed between turbidity and NO3 (−0.79), while WQI has a strong positive correlation with DO (0.69) and BOD (−0.78).
In Kavannur Lake, situated in the non-urban area with distinct multivariate relationships in Figure 12E. BOD had a strong positive correlation with alkalinity (0.97), EC and TDS (0.89), and chlorides (0.92), and significant negative correlations were observed between turbidity and Ca (−0.59), BOD and DO (−0.89), BOD and WQI (−0.97), WQI and NO3 (−0.56), and turbidity (−0.45). Hence BOD is strongly correlated with a decline in DO, potentially causing hypoxic or anoxic conditions that can harm aquatic life.
Overall, the analysis of water quality across Sadupperi, Thorapadi (semi-urban), Kalinzur, Dharapadavedu (urban area), and Kavannur (non-urban) lakes reveals natural and anthropogenic factors shaped by land use are influencing water quality. Semi-urban lakes like Sadupperi and Thorapadi face moderate pollution levels from urban runoff, which primarily affects hardness, TDS, and organic pollution (BOD), and aligns with the findings of Sadupperi lake (Raghul and Porchelvan, 2025). Urbanized lakes (Kalinjur and Dharapadavedu) exhibit more severe degradation due to organic loads and urban runoff (including influences from nearby railway dump yards). In contrast, non-urban Kavannur Lake experiences distinct pollution patterns, primarily from biological waste, agricultural runoff, with BOD and nutrient loading being the primary threats. The strong correlation between BOD and declining DO indicates that organic matter from agricultural activity and nearby river sources is significantly impairing the lake’s water quality.
3.6 Principle component analysis
PCA was conducted to identify the key factors influencing water quality in the lakes by examining the complex interactions between climatic factors, land use, and water quality parameters (Figure 13). This analysis extracted five principal components, namely PC1, PC2, PC3, PC4, and PC5, with eigenvalues greater than 1. Collectively, these components explain 81.03–90.83% of the total variance in the data, providing valuable insights into the underlying factors influencing lake water quality. The variance of each lake explained is summarized in Table 6. The remaining variance attributed to random variability or minor patterns.
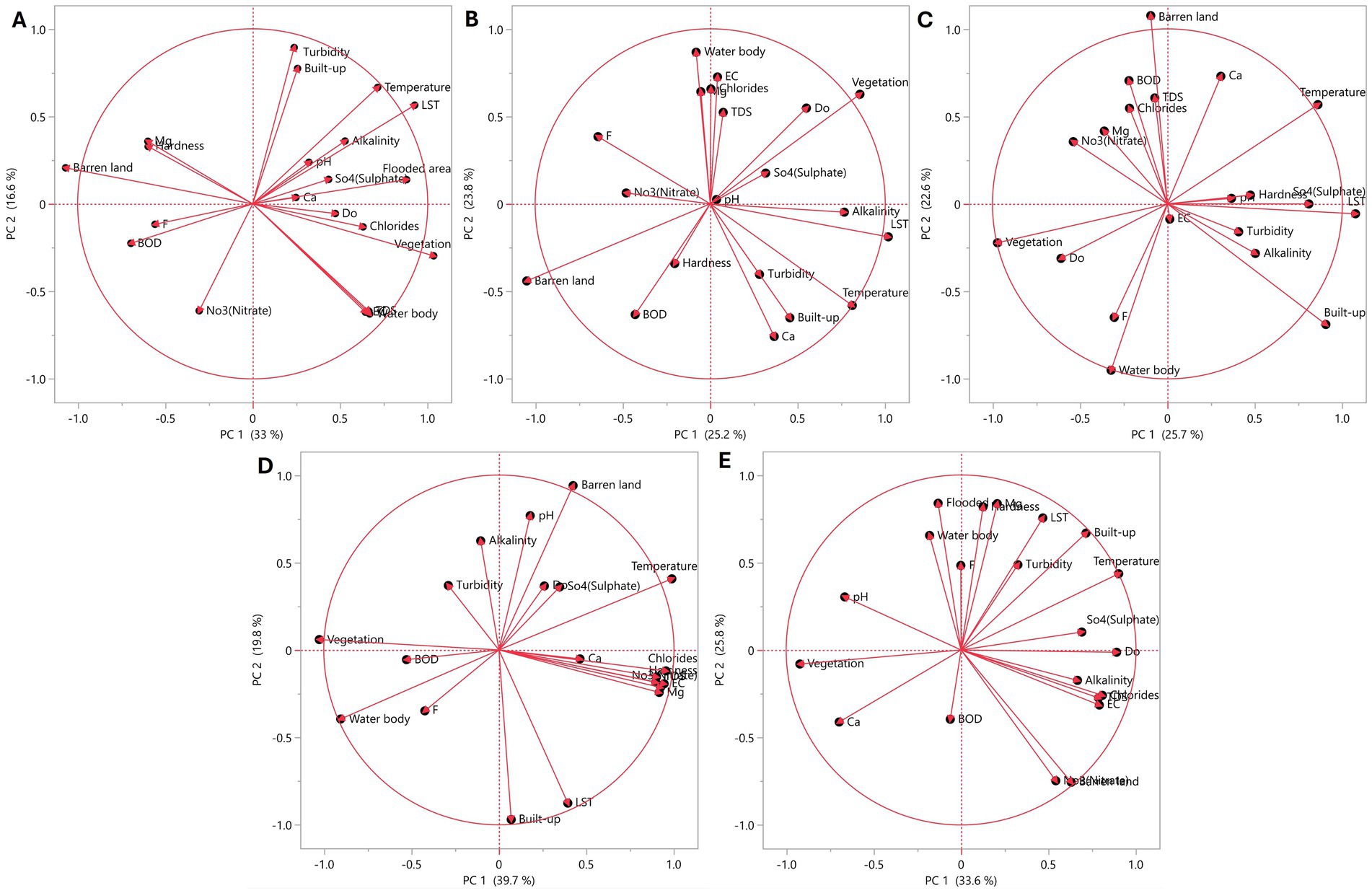
Figure 13. Principle component analysis of (A) Sadupperi, (B) Thorapadi, (C) Kalinzur, (D) Dharapadavedu, and (E) Kavanoor Lakes.
PC1 emerged as the most influential component, accounting for 33–39.7% of the variance. It was predominantly characterized by high loadings of pH, hardness, alkalinity, DO, and temperature, indicating a strong interrelationship among these variables. These chemical properties are likely influenced by similar environmental and anthropogenic factors, which suggest PC1 captures the core chemical dynamics affecting lake ecosystems.
PC2 accounted for 16.648 and 25.8% of the variance and was dominated by the impact of LST, temperature, built-up, barren land, and vegetation on water quality. The inclusion of these variables (PC2) indicates land use patterns and temperature significantly influence water quality, with a strong influence of urbanization (built-up areas) suggesting that land cover changes are contributing to water temperature and nutrient dynamics.
On the other hand, PC3 accounted for 14.953–16.6% of the variance, and was dominated by nitrate and fluoride. This component represents the nutrient cycling processes and provides insight into eutrophication, which negatively impacts aquatic life. However, PC4 (8.17–9.98% variance) was associated with variables such as turbidity, DO, BOD, and alkalinity. PC5, accounting for 6.24–7.45% of the variance, like variables of PC4, highlights the importance of the degree of organic pollution (contamination) in water quality.
The PCA results indicate similarities in component structures across lakes, with variations in the loadings and percentages of variance. The loadings and the percentage of variance explained differ from lake. This suggests that while common factors influence water quality, their relative importance varies among the lakes. These findings support previous research highlighting the interconnection of lake ecosystems (Locke, 2024; Paná et al., 2024). Overall, the PCA analysis underscores the interconnection of water quality, temperature factors, land use, nutrient cycling, and organic pollution. While physical and chemical parameters explain a significant portion of the variance in water quality, the considerable influence of land use patterns highlights the need for integrated management approaches that address both urban development and preservation of natural vegetation. The observed nutrient cycling in lakes such as Thorapadi and Kalnizur raises concerns about the potential eutrophication, necessitating proactive monitoring of nutrient levels, especially nitrate and fluoride. Additionally, lakes like Kavannur should prioritize the management of organic pollutants to maintain healthy water ecosystems. The dominant variables and their relationships with Sadupperi lake (Figure 14), illustrates the importance of land use, temperature, and water quality variables. Similar variable patterns in other lakes included in the Supplementary Figure S3.
4 Discussion
Figure 3 illustrates the decadal LST pattern across the study area, revealing an accelerated rate of urbanization. These urbanization impacts have resulted in increased temperatures in the lake surrounding WSA and urban regions, adversely affecting aquatic ecosystems and surrounding biodiversity. Understanding ecotopes diversity is essential for sustainable ecosystem management and biodiversity preservation (Verma et al., 2023). Numerous studies confirm that climate change influences water temperature and quality in surface waters by affecting DO and TDS, which are essential for ecological health (Ducharne, 2008). According to guidelines from ANSI, AWWA, and GCDWQ, temperature plays a crucial role in water quality by affecting TDS and turbidity levels at higher temperatures. Additionally, temperature correlates with pH, DO, and BOD levels (metabolic demand in aquatic species), which aligns with observed results in lake water quality assessments (Canada.ca, 2009; Health Canada, 2021, 2024; AWWA, 2021). The Vellore, with landlocked geography and recent precipitation changes contribute to high temperatures, with the lake experiencing surface water temperatures up to 30°C (86°F) during summer months, which is significantly impact water quality. To maintain ecological stability, it is essential to monitor lakes and maintain water temperature within an optimal range between 15°C and 25°C (59°F–77°F), as recommended by various water quality guidelines (AWWA, 2021; Health Canada, 2021). Similar to the research findings, (Arya et al., 2025) integrated the significance of temperature and vegetation in impacting public health, particularly in children, emphasizing the need for environmental management.
The PCA results explained the important insights into the interactions between land use, water quality, and climatic factors (LST and temperature) across the lakes. These correlations reinforce the influence of specific land uses and climatic variables on water quality. Vegetation cover consistently correlated with improved water quality across the lakes. For instance, high positive correlations were observed between vegetation and parameters such as pH (Sadupperi: 0.91; Thorapadi: 0.79) and DO (Sadupperi: 0.22; Thorapadi: 0.79), indicating that vegetation has high correlation to oxygenation, likely by reducing surface runoff. In Thorapadi Lake, the negative correlation between vegetation and BOD (−0.76) suggests that vegetative cover helps lower organic pollution. In Kavannur Lake, strong correlations between vegetation and DO (0.93) as well as alkalinity (0.52) suggest that vegetative cover enhances water quality and regulates temperature. These findings highlight the importance of maintaining and restoring vegetative buffers around lakes, particularly in urbanized and semi-urbanized areas.
Built-up and barren land were found to be negatively impacting water quality, primarily by increasing turbidity, BOD, and temperature, while decreasing DO. In Sadupperi Lake, built-up areas correlated strongly with turbidity (0.71) and BOD (0.68), signifying the detrimental effects due to urban runoff. Similarly, Thorapadi Lake showed a strong relationship between built-up areas and turbidity (0.75) and BOD (0.68), along with reduced DO (−0.22), illustrating urbanization contribution to deteriorating water quality. In the case of Kalnizur and Dharapadavedu Lake, barren land and built-up areas were associated with BOD (0.88) while negatively correlating with DO, suggesting that barren areas exacerbate pollution through enhanced erosion and sedimentation. Implementing green infrastructure and maintaining blue patches could mitigate the adverse effects of urbanization on lake surroundings.
The correlation between LST and temperature was consistently strong across lakes (Sadupperi: 0.96; Thorapadi: 0.91; Kalnizur: 0.78; Dharapadavedu: 0.86), indicating a close association. Increased temperature and LST were linked to a reduction in DO as a critical impact on aquatic health. For instance, in Sadupperi Lake, temperature and LST were negatively correlated with DO (−0.87). Similar patterns were observed in Thorapadi Lake (−0.63), Kalinzur Lake (−0.83), Kavannur Lake (−0.29), and Dharapadavedu lake (−0.36). In more natural (cloud water) areas, options for managing water quality are limited. However, direct interventions such as using chemicals to treat water or removing invasive species, and depth of water can help mitigate the impacts of climate stress (Martina et al., 2023; Malhi et al., 2020). Efforts to manage temperature through thermal regulation strategies, such as expanding vegetative cover and urban heat islands, are crucial for improving water quality and sustaining aquatic ecosystems.
Overall, the common trends reveal that vegetation consistently improves water quality, enhancing oxygenation and reducing organic pollution, whereas built-up areas and barren land degrade water quality by increasing turbidity, BOD, and temperature while decreasing DO (Figure 14). Temperature and LST are strongly correlated and negatively impact DO and aquatic health. The findings suggest that changes in land use and temperature patterns can have cascading effects on lake hydrology, water quality, and ecosystem functioning, necessitating a holistic approach that integrates hydro-ecological principles into lake management and conservation strategies. Figure 15 briefly illustrates the study’s key finding.
4.1 Environmental and climate implications
Earth’s climate is governed by energy balance and radiative processes that shape temperature patterns across different scales (Sarker, 2022). Present study shows that rapid urbanization and increasing LST in Vellore directly degrade urban WSA’s, findings align with global observations where impervious surfaces amplify non-point source pollution (Umwali et al., 2021). PCA analysis further reveals that LST, and built-up areas account for 68% of WQI variability, emphasizing the importance of land-use planning in protecting aquatic ecosystems. Preserving vegetative buffers around lakes and strict zoning regulations for industrial/residential expansion could mitigate runoff contamination, aligning with SDG 6 targets.
Furthermore, a strong correlation between LST and water temperature (r = 0.82) indicates that urban heat islands exacerbate climate-driven warming in lakes, leading to accelerated evaporation, reduced water availability during dry seasons, and the formation of hypoxia zones that promote algal blooms and pathogens (Weiskerger et al., 2019). Water vapor, a natural greenhouse gas, contribute to heat trapping while land-lake wind patterns complicate regional climatic variability (Sarker, 2022). Integrating LST trends into climate models is crucial for predicting hydrological impacts under future warming scenarios, supporting SDG 13. The multidisciplinary governance frameworks combining urban planning, climate resilience, and water management inform policymakers to consider climatic and anthropogenic factors.
However, study also is limited by reliance on satellite and ground-based observations, with the relationships between parameters established solely through correlation matrices. Future studies should prioritize collecting periodic site data from various lakes and exploring advanced statistical or machine learning models to better capture the interactions between temperature and water quality with high resolution constraints and expanded ground-truthing efforts to further refine these interrelationships.
5 Conclusion
This study investigated the interdependence of temperature and land use on water quality, focusing on the impact of these factors on Vellore lakes. Over the past three decades, the region has experienced significant land use changes, with built-up areas increasing to 28.26% and water bodies reduced up to 1.82%, resulting in a rise in LST by 2.68°C. The continuous decreasing WSA in all lakes, exacerbated by encroachments and land use changes, has led to microclimatic imbalances. Water quality analysis revealed that 64% of DO samples exceeded permissible limits, accompanied by EC, high turbidity, and nutrient pollution from urban runoff and agricultural effluents.
Advanced machine learning models such as XGBoost outperformed traditional approaches in terms of accuracy and reliability in predicting water quality indices. Moreover, WQI distinguished between lakes in various urban settings and showed semi-urbanized lakes (Sadupperi and Thorapadi) maintained ‘good and average’ water quality, whereas more urbanized lakes (Kalinzur and Dharapadavedu) were classified as ‘poor,’ with Kavannur lake also classified as ‘poor and unsuitable.’ Multivariate analysis and PCA highlighted the importance of DO, BOD, and turbidity in relation to temperature factors, land use, nutrient cycling, and organic pollution. The PCA further underscored the critical role of vegetation cover in mitigating the impact of oxygenation and organic pollution across studied lakes.
The findings emphasize the indispensable role of green spaces and water bodies in mitigating thermal stress and preserving ecological stability. Restorative strategies such as enhancing vegetation cover by 10–30%, increasing waterbody area by 2–5%, and implementing sustainable urban planning measures (urban forestry, hydro ecological strategies, and cooling materials) are recommended. This research emphasizes the need for sustainable water management practices, prioritizing polishing land use practices under the Smart Cities Mission. By integrating land use planning, temperature mitigation, and water quality enhancement, the current investigation offers a transformative pathway toward sustainable urban lake management. It underscores the need for proactive, science-driven policies that harmonize urban growth with environmental stewardship, ensuring that urban lakes remain resilient ecosystems that support both biodiversity and human well-being.
Data availability statement
The datasets presented in this study can be found in online repositories. The names of the repository/repositories and accession number(s) can be found in the article/Supplementary material.
Author contributions
DM: Writing – review & editing, Writing – original draft, Conceptualization, Software, Resources, Investigation, Visualization, Methodology, Validation, Formal analysis, Data curation. PJ: Visualization, Investigation, Validation, Supervision, Writing – review & editing.
Funding
The author(s) declare that no financial support was received for the research and/or publication of this article.
Acknowledgments
The authors thank Vellore Institute of Technology, Vellore for providing research facilities for conducting this research work.
Conflict of interest
The authors declare that the research was conducted in the absence of any commercial or financial relationships that could be construed as a potential conflict of interest.
Generative AI statement
The author(s) declare that no Gen AI was used in the creation of this manuscript.
Publisher’s note
All claims expressed in this article are solely those of the authors and do not necessarily represent those of their affiliated organizations, or those of the publisher, the editors and the reviewers. Any product that may be evaluated in this article, or claim that may be made by its manufacturer, is not guaranteed or endorsed by the publisher.
Supplementary material
The Supplementary material for this article can be found online at: https://www.frontiersin.org/articles/10.3389/frwa.2025.1598238/full#supplementary-material
References
Abed-Elmdoust, A., Miri, M. A., and Singh, A. (2016). Reorganization of river networks under changing spatiotemporal precipitation patterns: an optimal channel network approach. Water Resour. Res. 52, 8845–8860. doi: 10.1002/2015WR018391
Adjovu, G. E., Stephen, H., and Ahmad, S. (2023). Spatial and temporal dynamics of key water quality parameters in a thermal stratified lake ecosystem: the case study of Lake Mead. Earth 4, 461–502. doi: 10.3390/earth4030025
Alcamo, J. (2019). Water quality and its interlinkages with the sustainable development goals. Curr. Opin. Environ. Sustain. 36, 126–140. doi: 10.1016/j.cosust.2018.11.005
Arya, P. K., Sur, K., Kundu, T., Dhote, S., and Singh, S. K. (2025). Unveiling predictive factors for household-level stunting in India: a machine learning approach using NFHS-5 and satellite-driven data. Nutrition 132:112674. doi: 10.1016/j.nut.2024.112674
Auddy, N., Rai, A. K., Chatterjee, S., Pobi, K., Dutta, S., and Nayek, S. (2023). Trophic classification and assessment of Lake health using indexing approach and geostatistical methods for sustainable Management of Water Resources. Water Pract. Technol. 18, 967–980. doi: 10.2166/wpt.2023.039
AWWA (2021). M71 climate action plans - adaptive management strategies for utilities, first edition. 1st Edn. United states of America: American Water Works Association.
Beck, H. E., Zimmermann, N. E., McVicar, T. R., Vergopolan, N., Berg, A., and Wood, E. F. (2018). Present and future Köppen-Geiger climate classification maps at 1-km resolution. Sci. Data 5:180214. doi: 10.1038/sdata.2018.214
Bettina, Rickert, Ingrid, Chorus, and Oliver, Schmoll. (2016). “Protecting surface water for health: identifying, assessing and managing drinking-water quality risks in surface-water catchments.” World Health Organization. 2016. Available online at: https://iris.who.int/bitstream/handle/10665/246196/9789241510554-eng.pdf?sequence=1 or https://www.who.int/publications/i/item/9789241510554#:~:text=Protecting%20surface%20water%20for%20health%20embraces%20the%20challenge,health%2C%20which%20ultimately%20contributes%20to%20public%20health%20protection (Accessed October 20, 2024).
BIS 1987. (n.d.). “IS 3025–1 (1987): Methods of sampling and test (physical and chemical) for water and wastewater part 1—sampling.”
Canada.ca (2009). Guidance on the temperature aspects of drinking water.” Governament of Canada, Health, Canada. Available online at: https://www.canada.ca/en/health-canada/services/publications/healthy-living/guidelines-canadian-drinking-water-quality-guideline-technical-document-temperature.html?wbdisable=true#wb-cont (Accessed October 25, 2024).
CPCB (2019). “Water quality standards.” Central Pollution Control Board,. Ministry of Environment, Forest and Climate Change, Government of India. 2019.
CRDP. (2019). “Water quality across regional stream networks: the influence of land cover and land use, climate, and biogeochemical processing on spatiotemporal variance.” U.S. Geological Survey. Available online at: https://www.usgs.gov/programs/climate-research-and-development-program/science/water-quality-across-regional-stream#overview (Accessed April 17, 2019).
David, W. Schindler. (1997). “Widespread effects of climatic warming on freshwater ecosystems in North America.” Wiley Library. doi: 10.1002/(SICI)1099-1085(19970630)11:8%3C1043::AID-HYP517%3E3.0.CO;2-5 (Accessed July 5, 2024).
DO and EPA. (2021). “Dissolved Oxygen.” https://www.epa.gov/awma/dissolved-oxygen-parameter-factsheet.
Ducharne, A. (2008). Hydrology and earth system sciences importance of stream temperature to climate change impact on water quality. Hydrol. Earth Syst. Sci. 12, 797–810. doi: 10.5194/hess-12-797-2008
FAO (2000). Antonio Di Gregorio. Land cover classification system (Lccs).” Nairobi, Kenia. Available online at: https://www.fao.org/3/x0596e/x0596e00.htm (Accessed February 10, 2024).
Figueras, M. J., and Borrego, J. J. (2010). New Perspectives in monitoring drinking water microbial quality. Int. J. Environ. Res. Public Health 7, 4179–4202. doi: 10.3390/ijerph7124179
Fondriest Environmental, Inc. (2014). “Water temperature, "fundamentals of Environmental measurements.” Fondriest Environmental Learning Centre. 2014. Available online at: https://www.fondriest.com/environmental-measurements/parameters/water-quality/water-temperature/ (Accessed October 25, 2024).
Gao, Y., Sarker, S., Sarker, T., and Leta, O. T. (2022). Analyzing the critical locations in response of constructed and planned dams on the Mekong River basin for Environmental integrity. Environ. Res. Commun. 4:9459. doi: 10.1088/2515-7620/ac9459
GEE. (2022). “MODIS/Terra surface reflectance daily L2G global 250m SIN grid V006.” Google Earth Engine Data Catalog. Available online at: https://developers.google.com/earth-engine/datasets/catalog/MODIS_061_MOD11A1 (Accessed October 10, 2024).
Guzzo, M. M., Blanchfield, P. J., and Rennie, M. D. (2017). Behavioral responses to annual temperature variation Alter the dominant energy pathway, growth, and condition of a cold-water predator, vol. 114, 2921 doi: 10.5281/zenodo.832921
Health Canada (2021). GUIDANCE ON THE. Water and air quality bureau, healthy environments and consumer safety branch, Health Canada, Ottawa, Ontario. (Catalogue No H144-92/2021E-PDF).
Health Canada. (2024). “Guidelines for Canadian drinking water quality summary tables.” Available online at: https://www.canada.ca/en/health-canada/services/environmental-workplace-health/reports-publications/water-quality.html (Accessed April 28, 2024).
IGUTP (2015). “International guidelines on urban and territorial planning.” Available online at: www.unhabitat.org (Accessed April 28, 2024).
IITK. (n.d.). “Drinking water quality standards,.” Indian Institute of Technology Kanpur. Available online at: https://iitk.ac.in/iwd/wq/drinkingwater.html (Accessed October 10, 2024).
James, R., Anderson, Ernest E, and Hardy, John T., Roach, Richard, and Witmer, E.. (1976). “A land use and land cover classification system for use with remote sensor data.”
Jeffrey, D. Sachs, Guillaume, Lafortune, and Grayson, Fuller. (2024). “Sustainable development report 2024 includes the sdg index and dashboards.”
Jeppesen, E., Kronvang, B., Meerhoff, M., Søndergaard, M., Hansen, K. M., Andersen, H. E., et al. (2009). Climate change effects on runoff, catchment phosphorus loading and Lake ecological state, and potential adaptations. J. Environ. Qual. 38, 1930–1941. doi: 10.2134/jeq2008.0113
Kangabam, R. D., Bhoominathan, S. D., Kanagaraj, S., and Govindaraju, M. (2017). Development of a water quality index (WQI) for the Loktak Lake in India. Appl Water Sci 7, 2907–2918. doi: 10.1007/s13201-017-0579-4
Kernan, M., Battarbee, R. W., and Moss, B. (2010). Climate change impacts on freshwater ecosystems. Hoboken, UK: Wiley Blackwell.
Locke, K. A. (2024). Impacts of land use/land cover on water quality: A contemporary review for researchers and policymakers. Water Quality Research Journal: IWA Publishing.
Loganathan, S., and Sathiyamoorthy, M. (2024). Groundwater quality assessment for drinking, irrigation purposes and fuzzy logic-based water quality index for industrial suitability in Walajapet taluk, Ranipet District, Tamil Nadu, India. Aqua Water Infrastruct. Ecosyst. Soc. 73, 1766–1787. doi: 10.2166/aqua.2024.411
Malarvizhi, K., Kumar, S., and Porchelvan, V. (2022). Urban sprawl modelling and prediction using regression and seasonal ARIMA: a case study for Vellore, India. Model. Earth Syst. Environ. 8, 1597–1615. doi: 10.1007/s40808-021-01170-z
Malhi, Y., Franklin, J., Seddon, N., Solan, M., Turner, M. G., Field, C. B., et al. (2020). Climate change and ecosystems: threats, opportunities and solutions. Philos. Trans. R. Soc. B Biol. Sci. 375:20190104. doi: 10.1098/rstb.2019.0104
Manjunath, D. R., and Jagadeesh, P. (2024). Dynamics of urban development patterns on thermal distributions and their implications on water spread areas of Vellore, Tamil Nadu, India. Front. Sustain. Cities 6:1462092. doi: 10.3389/frsc.2024.1462092
Martina, A. C., Mukherji, A., Araos, M., and Balasubramanya, S. (2023). “Water” in Climate change 2022 – Impacts, adaptation and vulnerability. eds. B. E. J. Cisneros and Z. Kundzewicz (Cambridge, UK and New York, USA: Cambridge University Press), 551–712.
MODIS. (2020). “MOD11A1 V061.” MODIS/Terra Land Surface Temperature/Emissivity Daily L3 Global 1 Km SIN Grid. Available online at: https://lpdaac.usgs.gov/products/mod11a1v061/ (Accessed October 10, 2024).
Murdoch, P. S., Baron, J. S., and Miller, T. L. (2000). Potential effects of climate change on surface-water quality in North America 1. J. Am. Water Resour. Assoc. 36, 347–366. doi: 10.1111/j.1752-1688.2000.tb04273.x
NIUS. (2006). “National Urban Information System (NUIS) scheme guidelines for Implementation Town & Country Planning Organisation Government of India Ministry of Urban Development.”
Paná, S., Victoria Marinelli, M., Bonansea, M., Ferral, A., Valente, D., Valdez, V. C., et al. (2024). The multiscale Nexus among land use-land cover changes and water quality in the Suquía River basin, a semi-arid region of Argentina. Sci. Rep. 14:4670. doi: 10.1038/s41598-024-53604-0
Patro, K. S. K., Yadav, V. K., Dadarao, W. S., and Sharma, A. (2024). Temporal dynamics of land use land cover (LULC) changes and assessment of Environmental and climatic parameters using remote sensing and GIS: a case study in Limboti reservoir, Loha taluka, Maharashtra, India. Indian J. Fisheries 71:3. doi: 10.21077/ijf.2024.71.3.136129-06
Punja, V., Pathak, V., Shukla, S. P., and Tripathi, R. P. (2024). The impact of land use/land cover change on water quality in the middle ganga region: a review. Top. Catal. 67, 1024–1037. doi: 10.1007/s11244-024-01996-8
Raghul, M., and Porchelvan, P. (2025). An approach for monitoring chlorophyll-a and turbidity levels in Saduperi and Kaveripakkam Lakes located in and around Vellore region, Tamil Nadu, India, using spectral Unmixing technique. J. Indian Soc. Remote Sens. 53, 711–730. doi: 10.1007/s12524-024-02019-5
Roberts,, and Damon,. (2019). “Neural networks with JMP: building neural networks to determine body fat percentages based on various health measures.” Medium. Available online at: https://medium.com/swlh/neural-networks-with-jmp-7a945ebceb2c (Accessed October 4, 2019).
Rudd, Andrew, Simon, David, Cardama, Maruxa, Birch, Eugénie L, and Revi, Aromar. (2018). “Chapter 9: The UN, the urban sustainable development goal, and the new urban agenda.”
russ_wolfinger. (2020). “XGBoost add-in for JMP pro.” JMP User Community. Available online athttps://community.jmp.com/t5/JMP-Add-Ins/XGBoost-Add-In-for-JMP-Pro/ta-p/319383 (Accessed October 8, 2020).
Sarker, S. (2022). Fundamentals of climatology for engineers: lecture note. Eng. Multidiscip. Digit. Publish. Instit. 3, 573–595. doi: 10.3390/eng3040040
Sarker, S., Veremyev, A., Boginski, V., and Singh, A. (2019). Critical nodes in river networks. Sci. Rep. 9:11178. doi: 10.1038/s41598-019-47292-4
SDG. (2023). “Sustainable development high-level political forum on sustainable development, convened under the auspices of the economic and social council progress towards the sustainable development goals.”
Singh, M. C., Singh, J., and Sur, K. (2024). Spatio–temporal water quality assessment of Chohal and Damsal dams located in Kandi region of Punjab, India by geospatial technique and on-site investigation. Environ. Earth Sci. 83:51. doi: 10.1007/s12665-023-11354-8
Singhal, A., Jaseem, M., Divya, S. S., Prajapati, P., Singh, A., and Jha, S. K. (2024). Identifying potential locations of hydrologic monitoring stations based on topographical and hydrological information. Water Resour. Manag. 38, 369–384. doi: 10.1007/s11269-023-03675-x
Strokal, M., Bai, Z., Franssen, W., Hofstra, N., Koelmans, A. A., Ludwig, F., et al. (2021). Urbanization: an increasing source of multiple pollutants to Rivers in the 21st century. NPJ Urban Sustain. 1:24. doi: 10.1038/s42949-021-00026-w
Syrbe, Ralf-Uwe, and Chang, Jiang. (2018). “Options and challenges for implementing green spaces in urban development.” PP. 105–73.
Tong, Y., Feng, L., Wang, X., Pi, X., Wang, X., and Iestyn Woolway, R. (2023). Global Lakes are warming slower than surface air temperature due to accelerated evaporation. Nat. Water 1, 929–940. doi: 10.1038/s44221-023-00148-8
Uddin, M. G., Stephen, N., Azizur, R., and Agnieszka, I. O. (2023). Performance analysis of the water quality index model for predicting water state using machine learning techniques. Process. Saf. Environ. Prot. 169, 808–828. doi: 10.1016/j.psep.2022.11.073
Umwali, E. D., Kurban, A., Isabwe, A., Mind’je, R., Azadi, H., Guo, Z., et al. (2021). Spatio-seasonal variation of water quality influenced by land use and land cover in Lake Muhazi. Sci. Rep. 11:17376. doi: 10.1038/s41598-021-96633-9
UN habitate. (2010). Planning sustainable cities un-habitat practices and perspectives. Available online at: www.unhabitat.orgACKNOWLEDGEMENTS (Accessed May 28, 2024).
UN-Water. (2024). “Integrated monitoring initiative for SDG 6, Indicator 6.4.1: change in water use efficiency over time.” United nation water. Available online at: https://www.unwater.org/our-work/integrated-monitoring-initiative-sdg-6/indicator-641-change-water-use-efficiency-over-time (Accessed October 20, 2024).
US Geological survey. (2018). “Temperature and water.” Water Science School, USGS. Available online at: https://www.usgs.gov/special-topics/water-science-school/science/temperature-and-water (Accessed October 20, 2024).
Vellore and Smart City. (2016). “The Smart City challenge stage 2: Smart Vellore - Vellore Smart City proposal.”
Vellore CCBN. (2024). “City corporate cum business plan for Katpadi town panchayat final Report community consulting India private limited 1 project overview.”
Verma, V. K., Sur, K., and Prakash, C. (2023). Ecotope-based diversity monitoring of wetland using infused machine learning technique. Water Conserv. Sci. Eng. 8:38. doi: 10.1007/s41101-023-00212-0
Wang, M., Bodirsky, B. L., Rijneveld, R., Beier, F., Bak, M. P., Batool, M., et al. (2024). A triple increase in Global River basins with water scarcity due to future pollution. Nat. Commun. 15:880. doi: 10.1038/s41467-024-44947-3
Webb, Rachel L. (2023). “Mostly harmless statistics.” Available online at: https://LibreTexts.org (Accessed May 28, 2024).
Weiskerger, C. J., Brandão, J., Ahmed, W., Aslan, A., Avolio, L., Badgley, B. D., et al. (2019). Impacts of a changing earth on microbial dynamics and human health risks in the continuum between beach water and sand. Water Res. 162, 456–470. doi: 10.1016/j.watres.2019.07.006
Whittemore, Robin, and Knafl, Kathleen. (2005). “The integrative review: updated methodology.” J. Adv. Nurs. 52, 546–553, doi: 10.1111/j.1365-2648.2005.03621.x
WHO. (2021). “Guidelines on recreational water quality.” Coastal and fresh waters, World Health Organization. Available online at: https://www.who.int/publications/i/item/9789240031302 (Accessed October 10, 2024).
Wladimir, K. (2018). “Köppen climate classification.” in Available online at: https://en.Wikipedia.Org/Wiki/K%C3%B6ppen_climate_classification. Wikipedia the free encyclopedia (Accessed August 5, 2024).
Yaghouti, M., Heidarzadeh, N., Ulloa, H. N., and Nakhaei, N. (2023). The impacts of climate change on thermal stratification and dissolved oxygen in the temperate, dimictic Mississippi Lake, Ontario. Eco. Inform. 75:102087. doi: 10.1016/j.ecoinf.2023.102087
Keywords: sustainable urban development, SDG 6.4 and 13.3, smart cities, climate change, principal component analysis, XGBoost
Citation: Manjunath DR and Jagadeesh P (2025) Interdependence of temperature and land use on water quality in urban lakes, Vellore, Tamil Nadu, India. Front. Water. 7:1598238. doi: 10.3389/frwa.2025.1598238
Edited by:
Soumendra Bhanja, Oak Ridge National Laboratory (DOE), United StatesReviewed by:
Shiblu Sarker, Virginia Department of Conservation and Recreation, United StatesKoyel Sur, Punjab Remote Sensing Centre (PRSC), India
Copyright © 2025 Manjunath and Jagadeesh. This is an open-access article distributed under the terms of the Creative Commons Attribution License (CC BY). The use, distribution or reproduction in other forums is permitted, provided the original author(s) and the copyright owner(s) are credited and that the original publication in this journal is cited, in accordance with accepted academic practice. No use, distribution or reproduction is permitted which does not comply with these terms.
*Correspondence: P. Jagadeesh, cC5qYWdhZGVlc2hAdml0LmFjLmlu