- 1Institute of Computational Science and Technology, Guangzhou University, Guangzhou, China
- 2School of Computer Science of Information Technology, Qiannan Normal University for Nationalities, Duyun, China
- 3Department of Computer and Information Science, Fordham University, New York, NY, United States
MicroRNAs (miRNAs) are a class of important non-coding RNAs, which play important roles in tumorigenesis and development by targeting oncogenes or tumor suppressor genes. One miRNA can regulate multiple genes, and one gene can be regulated by multiple miRNAs. To promote the clinical application of miRNAs, two fundamental questions should be answered: what's the regulatory mechanism of a miRNA to a gene, and which miRNAs are important for a specific type of cancer. In this study, we propose a miRNA influence capturing (miRNAInf) to decipher regulation relations of miRNAs on target genes and identify critical miRNAs in cancers in a systematic approach. With the pair-wise miRNA/gene expression profiles data, we consider the assigning problem of a miRNA on target genes and determine the regulatory mechanisms by computing the Pearson correlation coefficient between the expression changes of a miRNA and that of its target gene. Furthermore, we compute the relative local influence strength of a miRNA on its target gene. Finally, integrate the local influence strength and target gene's importance to determine the critical miRNAs involved in specific cancer. Results on breast, liver and prostate cancers show that positive regulations are as common as negative regulations. The top-ranked miRNAs show great potential as therapeutic targets driving cancer to a normal state, and they are demonstrated to be closely related to cancers based on biological functional analysis, drug sensitivity/resistance analysis and survival analysis. This study will be helpful for the discovery of critical miRNAs and development of miRNAs-based clinical therapeutics.
Introduction
MicroRNAs (miRNAs) are a class of small non-coding RNAs and have been proved to play important roles in regulating more than two thirds of human genes (Bandyopadhyay et al., 2010; Song et al., 2017). They usually regulate their target genes by binding to the complementary seed sequence at the 3′ untranslated region. The binding of miRNAs usually leads to the translation repression or degradation of the target mRNAs and ultimately affects the production of the corresponding proteins (Bartel, 2009; Fabian et al., 2010; Hata and Lieberman, 2015). For example, miR-21 was demonstrated to negatively regulate the expression of SAV1 (Sun et al., 2019) and Smad6 (Xu et al., 2016) in colorectal cancer. One single miRNA usually targets many genes and one gene might be regulated by multiple miRNAs. To decipher the relationships between miRNAs and their target genes and unveil miRNAs' biological functions, many miRNA targets databases, such as Targetscan (Agarwal et al., 2015), miRDB (Wong and Wang, 2015), miRanda (Betel et al., 2010), and mirTarbase (Chou et al., 2018), have been built based on various biological experiments and/or different computation methods.
The dysfunctions of miRNAs have been reported to be involved in the tumorigenesis of various cancers (Bartel, 2004; Gotte, 2010; Dela Cruz and Matushansky, 2011; Lovat et al., 2011; Liu W. et al., 2018; Xu et al., 2018). For this reason, miRNAs have become potential biomarkers in cancer diagnosis and treatment (Slack and Chinnaiyan, 2019). Furthermore, some miRNA-based therapeutics have entered into the clinical research, i.e., miR-16-based mimics in phase I clinical trial for treating advanced non-small cell lung cancer, and antimiRs targeted at miR-122 in phase II trial for treating hepatitis (Rupaimoole and Slack, 2017).
However, accumulating evidence indicates that miRNAs can also promote the expression of their target genes. For example, Vasudevan et al. found that miR396-3 could direct the AGO complex binding with the AU-rich elements to promote the translation of its target gene in Vasudevan et al. (2007). They further demonstrated that let-7 and synthetic miRcxcr4 could induce target mRNAs up-regulation on cell cycle arrest while repressing translation in proliferation cells (Vasudevan and Steitz, 2007). In addition to functioning in the cytoplasm, mature miRNAs are also found in the nucleus. Xiao et al. demonstrated that miR-24-1 in the nucleus can activate gene transcription by targeting their enhancers (Xiao et al., 2017). Up to now, more than 200 positive regulations of miRNAs on genes have been experimentally identified in the literature.
It becomes a fundamental problem to elucidate the regulatory relations between miRNAs and their target genes in systems biology. Specifically, we need to know which genes are positively regulated by one miRNA and which genes are negatively regulated by it. The answer to this problem will provide a foundation to study the critical roles of miRNAs in tumorigenesis. Recently, Tan et al. first investigated this problem based on the Pearson correlation coefficients between the expression of miRNAs and their target genes in pan-cancer datasets (Tan et al., 2019). Surprisingly, they found many positive correlated miRNA-gene pairs. This demonstrates that miRNAs could exert their important roles in various cancers by positively regulating many genes.
Another important issue is to determine the critical miRNAs potential to affect the overexpression or under-expression of cancer-related genes. The answer to this question will help to determine a few “level point” miRNAs for designing miRNA-based therapeutic strategies. Cui et al. combined the miRNA sequence features and miRNA disease spectrum width (DSW) to define the importance of miRNAs (Cui et al., 2019). However, this static definition could not reflect the different regulatory mechanisms between miRNAs and genes involved in specific cancer well.
In this paper, we propose a novel miRNA influence capturing (MiRNAInf) to decipher regulation relations of miRNAs on target genes and identify critical miRNAs in cancers in a systematic approach. We study miRNA-gene regulations by assuming that the expression of one gene is determined by its upstream miRNAs. We model the expression of a gene as a function of the expression of the miRNAs targeting it. Through the Taylor expansion, we employ the first partial derivative to a miRNA to denote its regulatory effect on the target gene. The first partial derivative is then approximated by the Pearson correlation coefficient of the expression change of a miRNA and that of its target gene between disease and normal control.
We finally define the global influence of a miRNA by combining its local influence strength in an individual cancer and the degree of its target gene in a PPI network. Our results on breast cancer, prostate adenocarcinoma and liver cancer datasets further demonstrate that positive regulations are as common as negative ones in miRNA-gene interactions. We also find that only a few miRNAs have significant influences on the cancer-related differentially expressed genes. The identified top miRNAs in the three datasets are not only highly correlated in a functional network, but also significantly enriched in some important functions such as inflammation, cell proliferation, apoptosis and cell cycle. It demonstrates that they are very likely to play essential roles coherently in tumorigenesis. More importantly, we find that the intervention of a few critical miRNAs may alleviate the abnormal expressions of most genes according to the regulatory effect and differential expression situation between miRNAs and their target genes. Besides, the identified important miRNAs influence patients' survival time of prognosis besides the sensitivity/resistance of some anti-cancer drugs. In sum, our study provides a systematic way to understand the key roles of miRNAs in cancers and to screen potential intervention miRNA biomarkers for future miRNA-based therapy and diagnosis in precision medicine.
Materials and Methods
Data Acquisition and Preprocessing
We collected three types of data: miRNA and gene expression data for three cancers, miRNA-gene interaction data, and protein-protein interaction (PPI) data from three different databases: TCGA, miRTarbase and STRING (Chou et al., 2016; Szklarczyk et al., 2017).
The Cancer Genome Atlas (TCGA) Data
We collected datasets with both miRNA and gene expression profiles for cancer samples and corresponding normal samples in this study. Three cancers with abundant gene expression and miRNA expression “pair datasets” were acquired from TCGA (http://tcga-data.nci.nih.gov/tcga/). They included 102 samples for breast cancer, 52 samples for prostate adenocarcinoma, and 49 samples for liver cancer.
MiRNA-Gene Interaction Data
Besides gene expression and miRNA expression data, we further downloaded miRNA-gene targets data from miRTarbase (Chou et al., 2016), a widely-used state-of-the-art database for miRNA-gene targets. miRTarbase includes 502,652 high quality experimentally validated miRNA-gene interactions between 2,599 miRNAs and 15,064 genes for the human species.
Protein-Protein Interaction (PPI) Data
The STRING database, which includes 10,048,286 interactions between the 19,576 proteins for human beings, integrates the experimentally validated and computationally predicted protein-protein interactions (Szklarczyk et al., 2017). In order to use the highly confident interactions, we selected the interactions with a combined score >150. The distribution of proteins' degrees indicates that a small number of proteins have interactions with hundreds of other nodes, while most proteins only have interactions with a few of other proteins, which satisfies power-law distribution (Please go to Figure S1 for details).
Methods
In this study, we propose a miRNAInf method to identify critical miRNAs involved in cancer as well as conducting comprehensive functional analysis for miRNAs. The flowchart of the proposed miRNAInf methods is illustrated in Figure 1. The proposed method consists of the three steps. First, we determine the significant differentially expressed miRNAs and genes based on their expression data from TCGA. Second, we compute the local influence strength of a miRNA to its target gene. Finally, we evaluate the global influence of a miRNA in a specific cancer by integrating the local influence strength and gene's importance.
Identify Differentially Expressed miRNAs and Genes
We conduct normalization for miRNA and gene expression data before identifying differentially expressed miRNAs and genes. For each miRNA epression sample, we apply the RPM (Reads Per Million) method for normalization as described in Equation (1) for its simplicity and efficiency (Faraldi et al., 2019).
where Eri means the read counts of miRNA i and Totalr indicates the total counts of all miRNAs in a specific sample. RPM normalizes all read counts with respect to the ratio between library size and a million number. Similarly, we normalize gene expression data as follows
where Egj means the read counts of gene j and Totalg indicates the total counts of all genes in a specific sample.
We then apply DESeq2 (Love et al., 2014) to identify differentially expressed genes (DEGs) and differentially expressed miRNAs (DEmiRs) by collecting all genes and miRNAs with p < 0.05 adjusted by FDR method in differential expression analysis of the normalized data. Finally, we get 546,457 and 313 DemiRs and 5,057, 3,665, 3,064 DEGs for breast, liver, and prostate cancer, respectively.
Compute miRNA Local Influence Strength on a Target Gene
The expression of a gene can be assumed as a function of the expression of the miRNAs targeting it. Given a gene j regulated by m miRNAs r1, r2, …ri, …rm, then its expression in disease state can be approximated by first-level Taylor expansion as
where is the expression value of gene j in normal state, Rj is the index set of the miRNAs regulating gene j, Δri = is the change of miRNA i between the disease state and normal state.
The gene expression change Δgj of gene j can be calculated by moving the gene expression in the normal state to the left side of Equation 3:
where the right side represents the sum of expression change of gene j induced by the perturbation of each miRNA i. The partial derivative actually reflects the influence strength of miRNA i on gene j. A given miRNA i may target many other genes, we assume that Δrij, the portion of the ith miRNA expression difference Δri between the disease and normal states, affecting gene j, is positively proportional to the expression change Δgj. Given the target gene index set Gi of miRNA i, we define Δrij as the product of the absolute change ratio of gene j and the change of miRNA i between the disease state and normal state Δri:
Based on this, we can calculate the Pearson correlation coefficient ρij between Δrij and Δgj. ρij > 0 indicates that miRNA i upregulate gene j, otherwise, it suppresses gene j. Then the partial derivative can be approximated by
where the coefficient kij can be assumed and approximated by a constant k for all miRNA-genes.
Given one miRNA i, it may have different influence strengths on each target gene. Thus, we compute its local influence strength Iij as follows
where the means the average difference between disease and normal tissues for all patients. The local influence strength Iij considers both the correlation coefficient ρij and the average difference . The higher correlation ρij between them, the larger its influence on gene j. Similarly, the larger average difference , the larger also its influence on gene j.
Evaluate the Global Influence of Each miRNA
In order to describe the importance of a miRNA in a specific disease, we consider both the number of its target genes and the importance of each gene. Here, we define the global influence of a miRNA i for the disease by weighting its local influence by the importance of its target genes:
where dj and dmax represent the degree of the gene j and the maximum degree in the PPI network, respectively. The importance of the gene j is modeled as the ratio of its degree between the maximum degree in the PPI: . The global influence of a miRNA i involves both the local influence strength and the importance of its target genes. The more targets regulated by miRNA i, the larger its global influence. Simultaneously, the larger the degrees of its target genes in PPI, the larger the global influence.
Results
Positive Regulations Are as Common as Negative Ones in Cancers
As mentioned before, it suggests that positive regulations also play important roles in cancers. We first study the distribution of the Pearson correlation coefficient ρij between the change of a miRNA and that of its target genes. Figure 2 shows the distribution of the number of |ρij|> 0.3 in breast cancer, liver cancer, and prostate cancer, respectively. First, the distribution of the number of ρij> 0.3 and that of ρij < −0.3 are very similar. Our observation further supports the results in Tan et al. (2019) that miRNAs exert both positive and negative regulations on their target genes. Second, most of the absolute ρij are smaller than or equal to 0.5, which indicates that most of the regulatory strengths are relatively weak because one miRNA may target even hundreds of genes; On the other hand, only a few absolute |ρij| are larger than 0.8, which indicates that several individual genes may be strongly regulated by very few miRNAs.
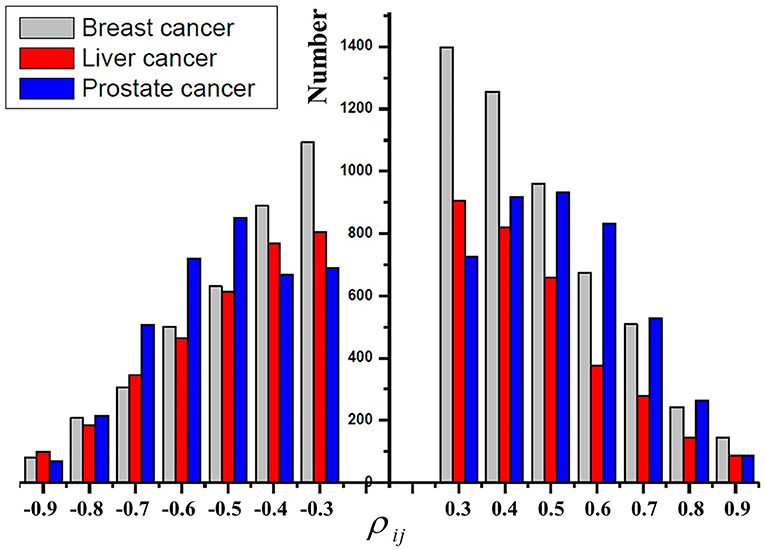
Figure 2. The distribution of the Pearson correlation coefficients ρij in breast cancer, liver cancer, and prostate cancer, respectively.
As an example, Figure 3 shows the scatter plots between the change of five miRNAs and that of their target gene KLF4, which regulates many critical physiologic and cellular processes (Wang et al., 2018). The X-axis of Figures 3A–E represents the expression change of a specific miRNA binding on target gene KLF4, X-axis of Figure 3F represents the total change of five miRNAs regulating KLF4, and the Y-axis of Figure 3 represents the expression change of KLF4. We can see that three miRNAs (hsa-miR-10b-5p, hsa-145-5p, and hsa-miR-335-5p) positively correlate with KLF4, while the other two miRNAs (hsa-32-5p and hsa-miR-7-5p) negatively correlate with it. This demonstrates that miRNAs targeting one gene may affect it differently. Their total expression changes positively correlate with that of KLF4 as shown in the last subplot of Figure 3. It indicates that some impacts of the negatively correlated miRNAs can be offset by those of dominant ones.
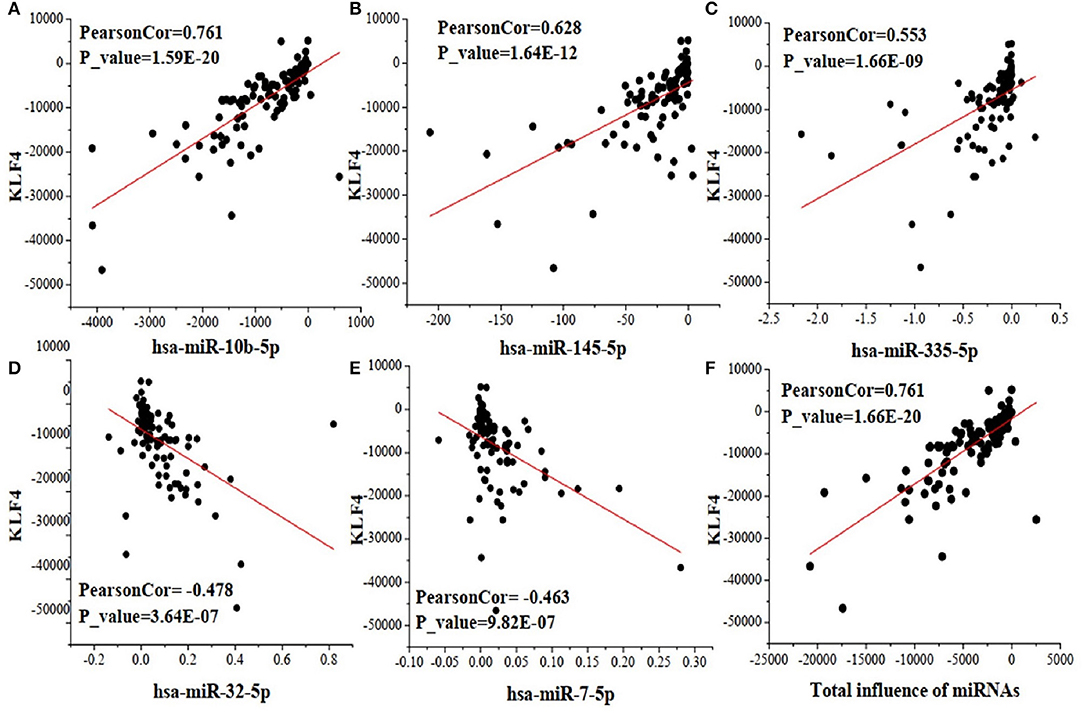
Figure 3. The scatter plots between the change of five miRNAs and that of their target gene KLF4. (A) the correlation between hsa-miR-10b-5p and KLF4; (B) the correlation between hsa-miR-145-5p and KLF4; (C) the correlation between hsa-miR-335-5p and KLF4; (D) the correlation between hsa-miR-32-5p and KLF4; (E) the correlation between hsa-miR-7-5p and KLF4; (F) the correlation of total influence of the five miRNAs and their target gene KLF4.
miRNAs Regulate Their Target Genes in a Complex Way
In this section, we select a portion of miRNAs and their target genes and display their local influence relation in a bipartite graph in Figure 4. We can see that one miRNA may promote the expression of some genes while repressing that of the others. On the other hand, one gene may be upregulated by some miRNAs while being downregulated by other miRNAs. Furthermore, one miRNA may have a larger influence (wide lines) on some genes while having relatively smaller influence (thin lines) on the others. The observations demonstrate that miRNAs interact with their target genes in a complex way. The inference of these complex interactions forms the basis for us to understand the detailed roles of each miRNA on a specific gene. For example, PIK3CA is regulated by hsa-miR-10b-5p (Influence strength, 0.9509), hsa-miR-335-5p (Influence strength, 2.83E-4), hsa-miR-17-5p (Influence strength,-7.75E-4), hsa-miR-19a-3p (Influence strength,-7.47E-5), and hsa-miR-155-5p (Influence strength,-7.29E-4). Then its expression is thus mainly upregulated by hsa-miR-10b-5p.
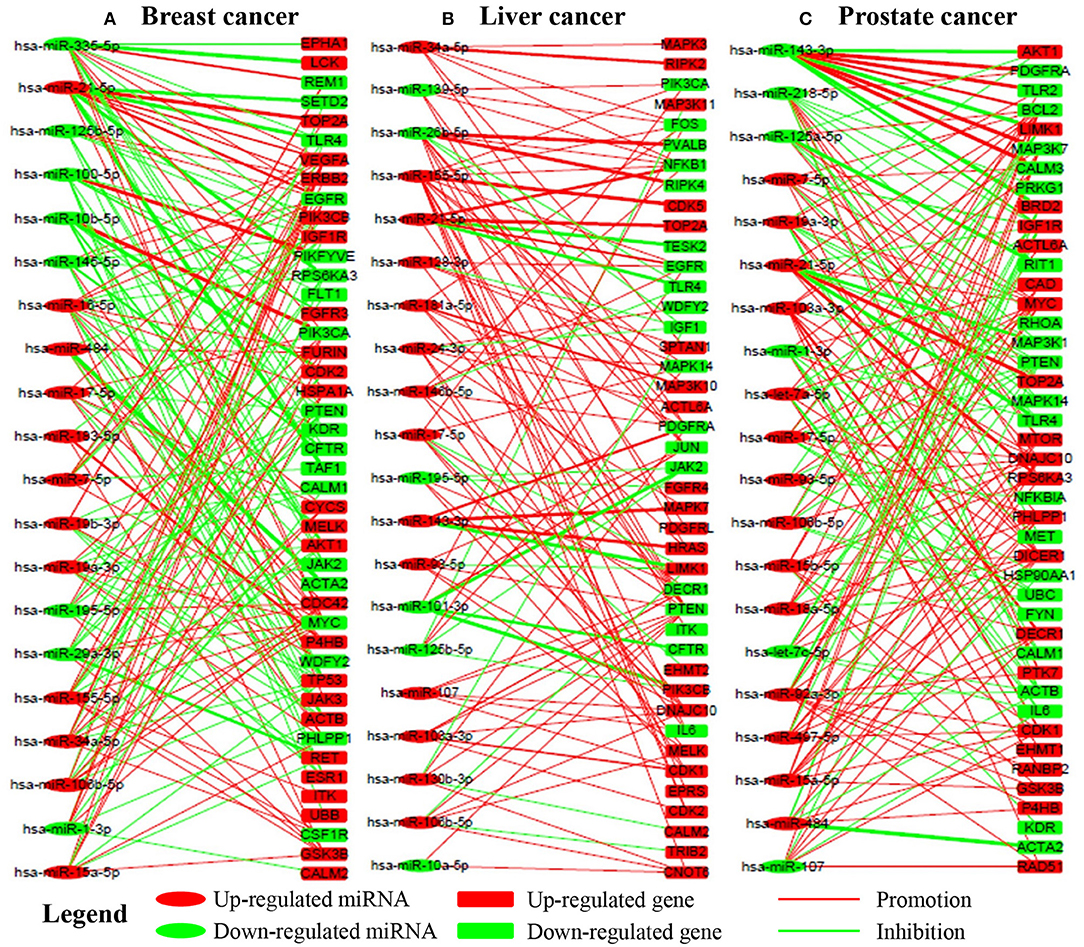
Figure 4. The regualtory networks between miRNAs and genes in breast, liver, and prostate cancer, respectively. Red and green lines indicate upregualtion and downregualtion, respectively. Line width indicates the absoulte value of the local influence strength. Red and green nodes indicate overexpression and underexpression, respectively. (A–C) are the subnetworks for breast, liver and prostate cancer respectively.
Only a Few miRNAs Have Significant Global Influences on Cancers
From the perspective of systems biology, we are more interested in the most critical miRNAs, i.e., dominant miRNAs that have the greatest influence on the whole regulatory network. Identifying the dominant miRNAs will answer the key question: which miRNAs are regulators of the most cancer-related genes?
Figure 5 illustrates the global influence of the top-ranked 20 miRNAs in breast, liver and prostate cancers, respectively. We can see that some miRNAs appear in all the three cancers whereas others may only show in one or two cancers. It suggests that some miRNAs play a common important role in many cancers while others are more related to specific cancers. Moreover, there are only a few miRNAs whose global influences are extremely larger than those of the others. This indicates their dysfunction may have very crucial impacts on the development of cancers. For instance, miR-21 ranked as the top one in the three cancers, has been confirmed highly involved in cancer proliferation and metastasis (Liu H. et al., 2018; Wang et al., 2019). On the other hand, we find that the most influenced genes by the dysfunctional miRNAs are highly related with cancers, such as CDK2, TP53, HRAS, NFKB1 (Carroll et al., 2000; Normanno et al., 2009; Xu et al., 2016).
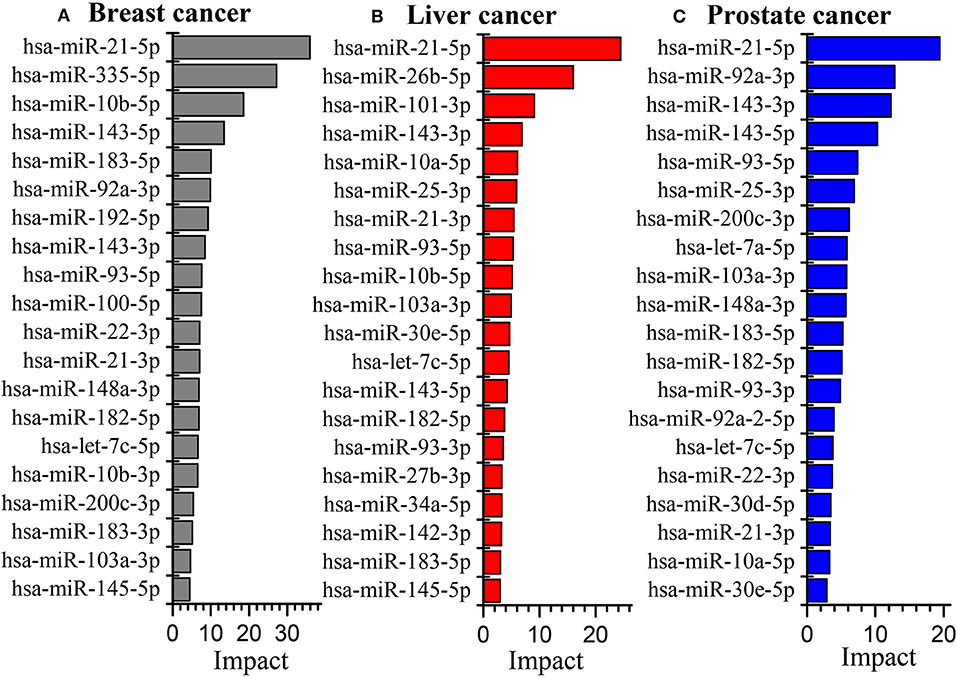
Figure 5. The global influence of the top 20 miRNAs in breast cancer (A), liver cancer (B) and prostate cancer (C) datasets, respectively.
Functional Analysis of the Critical miRNAs
Intervention of a Few Critical miRNAs May Help to Alleviate the Abnormal Expression of Most Cancer-Related Genes
Because one miRNA may regulate multiple downstream genes and the intervention on it may have different effects on the expression of its target genes. From the perspective of miRNA-based therapy, it is very crucial to figure out how the intervention of one miRNA may affect the abnormal expression of their target genes. If a miRNA promotes the expression of a gene and they are both overexpressed or under-expressed, then the intervention of the miRNA will exert a positive effect on the target gene to alleviate its abnormal expression; If a miRNA represses the expression of a gene and they have an opposite abnormal expression situation, then the intervention of the miRNA will also exert a positive effect on the target gene to alleviate the abnormal expression. Conversely, if a miRNA promotes the expression of a gene and they have the opposite abnormal expression situation, then the intervention of the miRNA will exert a negative effect on the target gene to deteriorate its abnormal expression. On the other hand, if a miRNA represses the expression of a gene and they are both overexpressed/under-expressed, then the intervention of the miRNA will also exert a negative effect on the target gene to deteriorate its abnormal expression.
Figure 6 shows the subnetwork of hsa-miR-21-5p in the three cancers, when intervention on hsa-miR-21-5p, the left-hand genes are those being positively affected while the right-hand ones negatively affected genes. This reveals that the intervention of one miRNA may have complex effects on cancer-related genes. Specifically, the abnormal expression of some genes can be alleviated while the other may be further deteriorated.
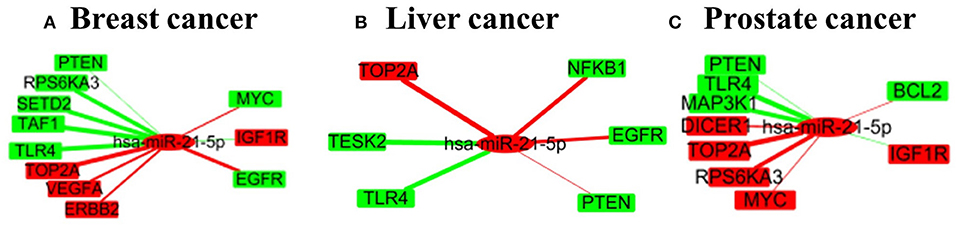
Figure 6. A small subnetwork of miRNA-21-5p and its target genes in three cancers. (A–C) are for the subnetworks in breast cancer, liver cancer and prostate cancer respectively.
Based on the regulation relations and the abnormal expression situations, we summarize the number of positively and the negatively affected miRNA-gene pairs of the top five miRNAs in Table 1. The “+”/“-” symbols in Table 1 represent the number of positively/negatively affected miRNA-gene pairs after intervention. We have the following observations from the table. First, we can see that the interventions of the five miRNAs may affect about 1,000 of abnormal miRNA-gene pairs which indicate they regulate many downstream genes. Second, the number of positively affected genes is extremely larger than that of the negatively affected ones. Third, the absolute relative influence strengths of most of the positively affected pairs are larger than 0.5. The observations indicate that the intervention of the top five miRNAs may significantly drive the cancer-related genes to the normal levels. Therefore, they are potential invention biomarkers for miRNA-based therapy.
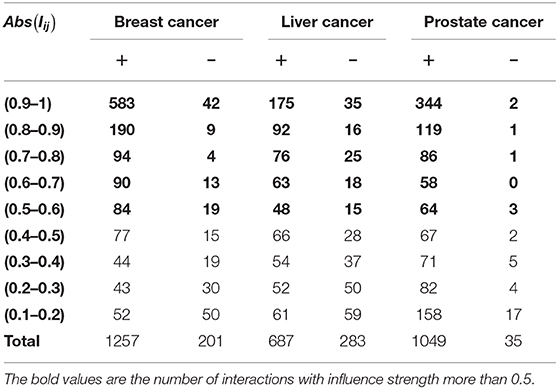
Table 1. The number of positive and negative effect interactions between the top 5 miRNAs and their targets in three cancers, respectively.
Most of the Critical miRNAs Involve in Some Important Biological Functions
We conduct functional analysis for top-ranked miRNAs by integrating the co-expression similarity, co-GO similarity, co-literature similarity, and co-similar disease similarity by using miRNA functional analysis tool MISIM (Li et al., 2019). There are 14, 15, and 13 miRNAs respectively annotated by MISIM in the top 20 important miRNAs of the three cancer datasets.
Figure 7A shows the function similarity network of the top 20 miRNAs, where red color lines denote the correlation coefficients larger than 0.5. It suggests most of the miRNAs are highly correlated in their biological functions. Figure 7B shows the top 10 enriched biological functions (FDR<7.0E-02) of the miRNAs. These biological functions, such as inflammation, cell proliferation, apoptosis, and cell cycle, have been verified closely related to different cancers (Evan and Vousden, 2001; Taniguchi and Karin, 2018; Xu et al., 2020). This indicates the critical miRNAs might interact in a highly coherent way to drive the biological system from normal to disease state in the three cancers.
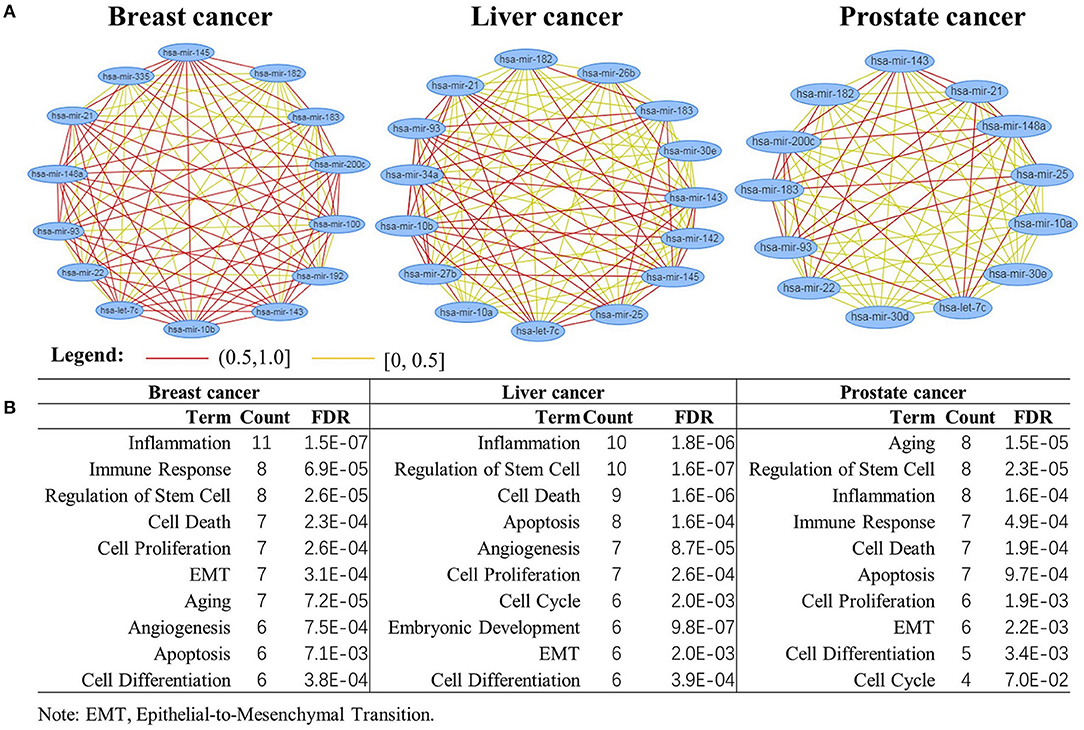
Figure 7. Biological function analysis of the top 20 miRNAs. (A) miRNAs function enrichment analysis; (B) functional similarity network analysis of the miRNAs.
As the overlapped critical miRNA in three cancers, hsa-mir-21 was reported to involved in various cancers such as colorectal cancer, breast cancer and lung cancer (Xu et al., 2016; Liu W. et al., 2018). It has been reported that overexpression of miR-21 could promote the cellular proliferation, colony formation, invasion and also inhibit cell death in a wide variety of cancerous cells by regulation of various targets including PTEN, TPM1, and PDCD4 (Najjary et al., 2020).
Some Critical miRNAs Also Impact the Resistance/Sensitivity of Drugs
Some non-coding RNAs (ncRNAs) especially miRNAs could promote sensitivity or produce resistance of drugs by regulating their target genes. To evaluate the impacts of the critical miRNAs on the resistance/sensitivity of drugs, we submit the top 20 miRNAs to two state-of-the-art miRNA-drug interaction databases: ncDR (Dai et al., 2017) and mTD (Chen et al., 2017). The two databases include 1,056, 384, and 127 records for miRNA-drug interactions in breast, liver and prostate cancers respectively as well as curating a lot of resistance/sensitivity related ncRNAs.
We find that there are 10, 6, and 4 miRNAs impacting drug resistance/sensitivity cases in breast cancer, liver and prostate cancer, respectively, among the top 20 miRNAs. The main reason for the small number of miRNAs in the liver and prostate cancers lies in that there are relatively fewer records about them in the two databases. Figure 8 shows their abnormal expression and corresponding influence on drug sensitivity/resistance. On one hand, one miRNA may influence multiple drugs with different effects. For example, the over-expression of hsa-mir-182 in breast cancer could induce drug resistance to both Olaparib and Cisplatin while promoting the sensitivity of Tamoxifen. On the other hand, some miRNAs may promote a drug sensitivity while others induce its resistance. The complex effects of these miRNAs on cancer-related drugs not only further demonstrate their importance in cancer development, but also provide a new insight for accurate drug selection.
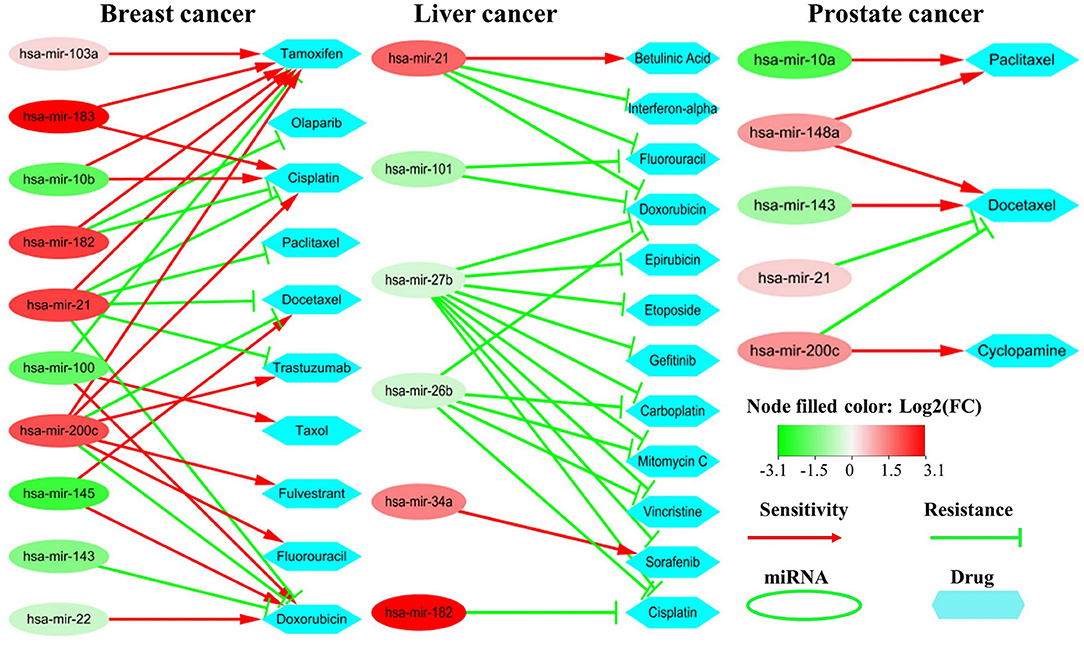
Figure 8. Drug sensitivity/resistance analysis of the top 20 miRNAs. The red (or green) oval represents the miRNA is up-regulated (or down-regulated) in cancer samples. The red line denotes promoting drug sensitivity while the green line denotes inducing drug resistance.
The Expression of Critical miRNAs Is Highly Related to the Survival Time of Prognosis
We also find that critical miRNAs can influence the survival time of prognosis seriously. Figure 9 shows the Kaplan–Meier curves of the top three miRNAs in breast, liver and prostate cancers, respectively. Most of them are significantly correlated to the overall survival time in both breast and liver cancers except for prostate cancer. One major reason is that most prostate tumors are slow-growing and many of them are not lethal. Furthermore, some important correlations between the miRNAs are supported by wet-lab experiments. For example, Yan et al. demonstrated that overexpression of miR-21 was associated with human breast cancer poor prognosis (Yan et al., 2008). Ji et al. showed that liver cancer patients with low miR-26 expression had shorter overall survival time (Ji et al., 2009, 2013). These observations indicate that the identified critical miRNAs may also serve as potential biomarkers for the survival time of prognosis.
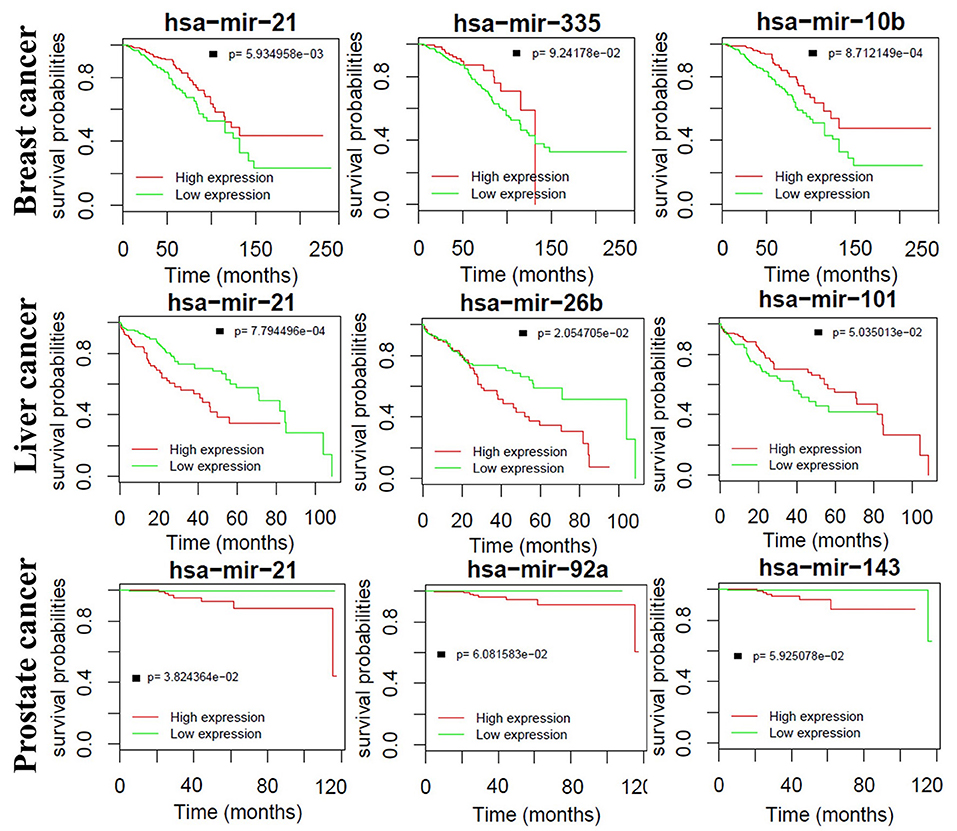
Figure 9. Kaplan–Meier curves of the top three miRNAs in breast, liver and prostate cancers, respectively.
Conclusion
MiRNAs have been reported as a kind of important non-coding regulators influencing the expression of more than 60% genes. In this paper, we proposed a novel miRNA influence capturing (miRNAInf) method to characterize the regulatory mechanism of miRNA on their target genes as well as identify critical miRNAs that have dominantly important impacts on target genes. Out results from the breast, prostate and liver cancer datasets further verify that miRNAs may either upregulate or downregulate their target genes instead of mainly repressing them. We identified some critical miRNAs involved in the three cancers by constructing a miRNA-gene regulatory network. Our biological functional analysis shows that those critical miRNAs are not only highly correlated with each other but also involved in many important biological functions such as apoptosis, proliferation, etc. Furthermore, miRNA-gene interaction analysis reveals that the intervention of only a few top crucial miRNAs may potentially alleviate the abnormal expressions of many genes and push the cancer system to a normal situation. It suggests that the identified crucial miRNAs may serve as potential biomarkers for miRNA-based therapy as well as diagnosis. In addition, we find some critical miRNAs may influence the sensitivity/resistance of drugs as well as the survival time of prognosis. Our study provides a strong foundation to support the combination of miRNA-based therapy and cancer drugs to improve the treatment effect in precision medicine. To the best of our knowledge, this study first provides a systematic approach to decipher the roles of miRNAs in the diagnosis and prognosis of complex diseases and will inspire future studies in this field.
Data Availability Statement
All datasets generated for this study are included in the article/Supplementary Material. The source codes for this study can be found at https://github.com/xupeng2017/MiRNAInf.
Author Contributions
WL and PX designed the methods. PX wrote the codes. WL, PX, QW, JY, YR, GF, ZK, XS, and HH analyzed the data. WL, HH, and PX wrote the manuscript. All authors read and approved the final manuscript.
Funding
This work was funded in part by the National Key R & D Program of China (Grant No. 2019YFA0706402), National Natural Science Foundation of China (grant nos. 61572367, 61972107, and 61972109), Specialized Fund for Science and Technology Platform and Talent Team Project of Guizhou Province (No. QianKeHePingTaiRenCai [2016]5609), Key Supported Disciplines of Guizhou Province Computer Application Technology (No. QianXueWeiHeZi ZDXK [2016]20).
Conflict of Interest
The authors declare that the research was conducted in the absence of any commercial or financial relationships that could be construed as a potential conflict of interest.
Acknowledgments
We would like to thank the reviewers for their valuable comments and suggestions.
Supplementary Material
The Supplementary Material for this article can be found online at: https://www.frontiersin.org/articles/10.3389/fgene.2020.00278/full#supplementary-material
References
Agarwal, V., Bell, G. W., Nam, J.-W., and Bartel, D. P. (2015). Predicting effective microRNA target sites in mammalian mRNAs. Elife 4:e05005. doi: 10.7554/eLife.05005.028
Bandyopadhyay, S., Mitra, R., Maulik, U., and Zhang, M. Q. (2010). Development of the human cancer microRNA network. Silence 1:6. doi: 10.1186/1758-907X-1-6
Bartel, D. P. (2004). MicroRNAs: genomics, biogenesis, mechanism, and function. Cell 116, 281–297. doi: 10.1016/S0092-8674(04)00045-5
Bartel, D. P. (2009). MicroRNAs: target recognition and regulatory functions. Cell 136, 215–233. doi: 10.1016/j.cell.2009.01.002
Betel, D., Koppal, A., Agius, P., Sander, C., and Leslie, C. (2010). Comprehensive modeling of microRNA targets predicts functional non-conserved and non-canonical sites. Genome Biol. 11:R90. doi: 10.1186/gb-2010-11-8-r90
Carroll, J. S., Prall, O. W., Musgrove, E. A., and Sutherland, R. L. (2000). A pure estrogen antagonist inhibits cyclin E-Cdk2 activity in MCF-7 breast cancer cells and induces accumulation of p130-E2F4 complexes characteristic of quiescence. J. Biol. Chem. 275, 38221–38229. doi: 10.1074/jbc.M004424200
Chen, X., Xie, W.-B., Xiao, P.-P., Zhao, X.-M., and Yan, H. (2017). mTD: a database of microRNAs affecting therapeutic effects of drugs. J. Genet. Genomics. 44, 269–271. doi: 10.1016/j.jgg.2017.04.003
Chou, C. H., Chang, N. W., Shrestha, S., Hsu, S. D., Lin, Y. L., Lee, W. H., et al. (2016). miRTarBase 2016: updates to the experimentally validated miRNA-target interactions database. Nucleic Acids Res. 44, D239–D247. doi: 10.1093/nar/gkv1258
Chou, C. H., Shrestha, S., Yang, C. D., Chang, N. W., Lin, Y. L., Liao, K. W., et al. (2018). miRTarBase update 2018: a resource for experimentally validated microRNA-target interactions. Nucleic Acids Res. 46, D296–D302. doi: 10.1093/nar/gkx1067
Cui, C., Shi, B., Shi, J., Zhou, Y., and Cui, Q. (2019). Defining the importance score of human microRNAs and mutants using their single nucleotide random forest regression and sequence data. Adv. Theory Simul. 2:1900083. doi: 10.1002/adts.201900083
Dai, E., Yang, F., Wang, J., Zhou, X., Song, Q., An, W., et al. (2017). ncDR: a comprehensive resource of non-coding RNAs involved in drug resistance. Bioinformatics 33, 4010–4011. doi: 10.1093/bioinformatics/btx523
Dela Cruz, F., and Matushansky, I. (2011). MicroRNAs in chromosomal translocation-associated solid tumors: learning from sarcomas. Discov. Med. 12, 307–317.
Evan, G. I., and Vousden, K. H. (2001). Proliferation, cell cycle, and apoptosis in cancer. Nature 411, 342–348. doi: 10.1038/35077213
Fabian, M. R., Sonenberg, N., and Filipowicz, W. (2010). Regulation of mRNA translation and stability by microRNAs. Annu. Rev. Biochem. 79, 351–379. doi: 10.1146/annurev-biochem-060308-103103
Faraldi, M., Gomarasca, M., Sansoni, V., Perego, S., Banfi, G., and Lombardi, G. (2019). Normalization strategies differently affect circulating miRNA profile associated with the training status. Sci. Rep. 9:1584. doi: 10.1038/s41598-019-38505-x
Hata, A., and Lieberman, J. (2015). Dysregulation of microRNA biogenesis and gene silencing in cancer. Sci. Signal. 8:re3. doi: 10.1126/scisignal.2005825
Ji, J., Shi, J., Budhu, A., Yu, Z., Forgues, M., Roessler, S., et al. (2009). MicroRNA expression, survival, and response to interferon in liver cancer. N. Engl. J. Med. 361, 1437–1447. doi: 10.1056/NEJMoa0901282
Ji, J., Yu, L., Yu, Z., Forgues, M., Uenishi, T., Kubo, S., et al. (2013). Development of a miR-26 companion diagnostic test for adjuvant interferon-alpha therapy in hepatocellular carcinoma. Int. J. Biol. Sci. 9, 303–312. doi: 10.7150/ijbs.6214
Li, J., Zhang, S., Wan, Y., Zhao, Y., Shi, J., Zhou, Y., et al. (2019). MISIM v2.0: a web server for inferring microRNA functional similarity based on microRNA-disease associations. Nucleic Acids Res. 47, W536–W541. doi: 10.1093/nar/gkz328
Liu, H., Cheng, L., Cao, D., and Zhang, H. (2018). Suppression of miR-21 expression inhibits cell proliferation and migration of liver cancer cells by targeting Phosphatase and Tensin Homolog (PTEN). Med. Sci. Monit. 24, 3571–3577. doi: 10.12659/MSM.907038
Liu, W., Cui, Z., and Zan, X. (2018). Identifying cancer-related microRNAs based on subpathways. IET Syst. Biol. 12, 273–278. doi: 10.1049/iet-syb.2018.5025
Lovat, F., Valeri, N., and Croce, C. M. (2011). MicroRNAs in the pathogenesis of cancer. Semin. Oncol. 38, 724–733. doi: 10.1053/j.seminoncol.2011.08.006
Love, M. I., Huber, W., and Anders, S. (2014). Moderated estimation of fold change and dispersion for RNA-seq data with DESeq2. Genome Biol. 15:550. doi: 10.1186/s13059-014-0550-8
Najjary, S., Mohammadzadeh, R., Mokhtarzadeh, A., Mohammadi, A., Kojabad, A. B., and Baradaran, B. (2020). Role of miR-21 as an authentic oncogene in mediating drug resistance in breast cancer. Gene 738:144453. doi: 10.1016/j.gene.2020.144453.
Normanno, N., Tejpar, S., Morgillo, F., de Luca, A., van Cutsem, E., and Ciardiello, F. (2009). Implications for KRAS status and EGFR-targeted therapies in metastatic CRC. Nat. Rev. Clin. Oncol. 6, 519–527. doi: 10.1038/nrclinonc.2009.111
Rupaimoole, R., and Slack, F. J. (2017). MicroRNA therapeutics: towards a new era for the management of cancer and other diseases. Nat. Rev. Drug Discov. 16, 203–222. doi: 10.1038/nrd.2016.246
Slack, F. J., and Chinnaiyan, A. M. (2019). The role of non-coding RNAs in oncology. Cell 179, 1033–1055. doi: 10.1016/j.cell.2019.10.017
Song, J., Hu, Y., Li, J., Zheng, H., Wang, J., Guo, L., et al. (2017). Different microRNA profiles reveal the diverse outcomes induced by EV71 and CA16 infection in human umbilical vein endothelial cells using high-throughput sequencing. PLoS ONE 12:e0177657. doi: 10.1371/journal.pone.0177657
Sun, B. Z., Zhang, J. Z., Liu, M. H., and Guan, L. Y. (2019). Alkannin inhibits proliferation, migration and invasion of hepatocellular carcinoma cells via regulation of miR-92a. Biomed. Pharmacotherapy 114:108782. doi: 10.1016/j.biopha.2019.108782
Szklarczyk, D., Morris, J. H., Cook, H., Kuhn, M., Wyder, S., Simonovic, M., et al. (2017). The STRING database in 2017: quality-controlled protein-protein association networks, made broadly accessible. Nucleic Acids Res. 45, D362–D368. doi: 10.1093/nar/gkw937
Tan, H., Huang, S., Zhang, Z., Qian, X., Sun, P., and Zhou, X. (2019). Pan-cancer analysis on microRNA-associated gene activation. EBioMedicine 43, 82–97. doi: 10.1016/j.ebiom.2019.03.082
Taniguchi, K., and Karin, M. (2018). NF-κB, inflammation, immunity and cancer: coming of age. Nat. Rev. Immunol. 18, 309–324. doi: 10.1038/nri.2017.142
Vasudevan, S., and Steitz, J. A. (2007). AU-rich-element-mediated upregulation of translation by FXR1 and Argonaute 2. Cell 128, 1105–1118. doi: 10.1016/j.cell.2007.01.038
Vasudevan, S., Tong, Y., and Steitz, J. A. (2007). Switching from repression to activation: microRNAs can up-regulate translation. Science 318, 1931–1934. doi: 10.1126/science.1149460
Wang, H., Tan, Z., Hu, H., Liu, H., Wu, T., Zheng, C., et al. (2019). microRNA-21 promotes breast cancer proliferation and metastasis by targeting LZTFL1. BMC Cancer 19:738. doi: 10.1186/s12885-019-5951-3
Wang, L., Shen, F., Stroehlein, J. R., and Wei, D. (2018). Context-dependent functions of KLF4 in cancers: could alternative splicing isoforms be the key? Cancer Lett. 438, 10–16. doi: 10.1016/j.canlet.2018.09.005
Wong, N., and Wang, X. (2015). miRDB: an online resource for microRNA target prediction and functional annotations. Nucleic Acids Res. 43, D146–D152. doi: 10.1093/nar/gku1104
Xiao, M., Li, J., Li, W., Wang, Y., Wu, F., Xi, Y., et al. (2017). MicroRNAs activate gene transcription epigenetically as an enhancer trigger. RNA Biol. 14, 1326–1334. doi: 10.1080/15476286.2015.1112487
Xu, P., Wang, J., Sun, B., and Xiao, Z. (2018). Integrated analysis of miRNA and mRNA expression data identifies multiple miRNAs regulatory networks for the tumorigenesis of colorectal cancer. Gene 659, 44–51. doi: 10.1016/j.gene.2018.03.050
Xu, P., Zhao, G., Kou, Z., Fang, G., and Liu, W. (2020). Classification of cancers based on a comprehensive pathway activity inferred by genes and their interactions. IEEE Access 8, 30515–30521. doi: 10.1109/access.2020.2973220
Xu, P., Zhu, Y., Sun, B., and Xiao, Z. (2016). Colorectal cancer characterization and therapeutic target prediction based on microRNA expression profile. Sci. Rep. 6:20616. doi: 10.1038/srep20616
Keywords: miRNA, influence, cancer, target gene, regulation relation
Citation: Xu P, Wu Q, Yu J, Rao Y, Kou Z, Fang G, Shi X, Liu W and Han H (2020) A Systematic Way to Infer the Regulation Relations of miRNAs on Target Genes and Critical miRNAs in Cancers. Front. Genet. 11:278. doi: 10.3389/fgene.2020.00278
Received: 14 January 2020; Accepted: 09 March 2020;
Published: 31 March 2020.
Edited by:
Meng Zhou, Wenzhou Medical University, ChinaReviewed by:
Guimin Qin, Xidian University, ChinaXuequn Shang, Northwestern Polytechnical University, China
Copyright © 2020 Xu, Wu, Yu, Rao, Kou, Fang, Shi, Liu and Han. This is an open-access article distributed under the terms of the Creative Commons Attribution License (CC BY). The use, distribution or reproduction in other forums is permitted, provided the original author(s) and the copyright owner(s) are credited and that the original publication in this journal is cited, in accordance with accepted academic practice. No use, distribution or reproduction is permitted which does not comply with these terms.
*Correspondence: Wenbin Liu, wbliu6910@126.com; Henry Han, xhan9@fordham.edu