- 1Marine Research Division, AZTI, Sukarrieta, Spain
- 2Red Sea Research Center, King Abdullah University of Science and Technology, Thuwal, Saudi Arabia
Characterization of biodiversity has been extensively used to confidently monitor and assess environmental status. Yet, visual morphology, traditionally and widely used for species identification in coastal and marine ecosystem communities, is tedious and entails limitations. Metabarcoding coupled with high-throughput sequencing (HTS) represents an alternative to rapidly, accurately, and cost-effectively analyze thousands of environmental samples simultaneously, and this method is increasingly used to characterize the metazoan taxonomic composition of a wide variety of environments. However, a comprehensive study benchmarking visual and metabarcoding-based taxonomic inferences that validates this technique for environmental monitoring is still lacking. Here, we compare taxonomic inferences of benthic macroinvertebrate samples of known taxonomic composition obtained using alternative metabarcoding protocols based on a combination of different DNA sources, barcodes of the mitochondrial cytochrome oxidase I gene and amplification conditions. Our results highlight the influence of the metabarcoding protocol in the obtained taxonomic composition and suggest the better performance of an alternative 313 bp length barcode to the traditionally 658 bp length one used for metazoan metabarcoding. Additionally, we show that a biotic index inferred from the list of macroinvertebrate taxa obtained using DNA-based taxonomic assignments is comparable to that inferred using morphological identification. Thus, our analyses prove metabarcoding valid for environmental status assessment and will contribute to accelerating the implementation of this technique to regular monitoring programs.
Introduction
Environmental biomonitoring in coastal and marine ecosystems often relies on comprehensively, accurately, and repeatedly characterizing the benthic macroinvertebrate community (Yu et al., 2012). These organisms are considered a good indicator of ecosystem health and have demonstrated a rapid response to a range of natural and anthropogenic pressures (Johnston and Roberts, 2009). As a result, the macroinvertebrate community has been largely used to develop biotic indices (Diaz et al., 2004; Pinto et al., 2009; Borja et al., 2015), such as the AZTI's Marine Biotic Index (AMBI; Borja et al., 2000), used worldwide to assess the marine benthic status (Borja et al., 2015). Nevertheless, biomonitoring based upon benthic organisms has limitations because species identification requires extensive taxonomic expertise and it is time-consuming, expensive, and laborious (Yu et al., 2012; Wood et al., 2013; Aylagas et al., 2014). The rapid development of high-throughput sequencing (HTS) technologies represents a promising opportunity for easing the implementation of molecular approaches for biomonitoring programs (Bourlat et al., 2013; Dowle et al., 2015). In particular, DNA metabarcoding (Taberlet et al., 2012a) allows the rapid and cost-effective identification of the entire taxonomic composition of thousands of samples simultaneously (Zepeda Mendoza et al., 2015) and the ability to provide a more comprehensive community analysis than traditional assessments (Dafforn et al., 2014), which can enable the calculation of benthic indices in a much faster and accurate way compared to morphological methodologies.
Metabarcoding consists of simultaneously amplifying a standardized DNA fragment specific for a species (barcode) from the total DNA extracted from an environmental sample using conserved short DNA sequences flanking the barcode (primers; Hajibabaei, 2012; Cristescu, 2014). The obtained barcodes are then high-throughput sequenced and compared to a previously generated DNA sequence reference database from well-characterized species for taxonomic assignment (Taberlet et al., 2012a). In the case of animals, different barcodes such as portions of the small and large subunits of the nuclear ribosomal RNA (18S and 28S rRNA) genes (Machida and Knowlton, 2012) and of the mitochondrial cytochrome oxidase I (COI; Meusnier et al., 2008) and 16S rRNA genes (Sarri et al., 2014) have been proposed for metabarcoding. The COI gene is by far the most commonly used marker for metazoan metabarcoding (Ratnasingham and Hebert, 2013), for which thousands of reference sequences are available in public databases [the Barcode of Life Database (BOLD) contains >1,000,000 COI sequences belonging to animal species] and several amplification primers have been designed [more than 400 COI primers are published in the Consortium for the Barcode of Life (CBOL) primer database].
Several studies have used metabarcoding to characterize the metazoan taxonomic composition of aquatic environments (Porazinska et al., 2009; Chariton et al., 2010; Fonseca et al., 2014; Dell'Anno et al., 2015; Leray and Knowlton, 2015; Chain et al., 2016), and an increasing number of studies have directly applied the approach for environmental biomonitoring purposes (Ji et al., 2013; Dafforn et al., 2014; Pawlowski et al., 2014; Chariton et al., 2015; Gibson et al., 2015; Pochon et al., 2015; Zaiko et al., 2015). Initial studies inferring biotic indices from molecular data show the potential of metabarcoding for evaluating aquatic ecosystem quality (Lejzerowicz et al., 2015; Visco et al., 2015). However, before implementation of metabarcoding in regular biomonitoring programs, this approach needs to be benchmarked against morphological identification so that accurate taxonomic inferences and derived biotic indices can be ensured (Aylagas et al., 2014; Carugati et al., 2015). The accuracy of metabarcoding-based taxonomic inferences relies on the retrieval of a wide range of taxonomic groups from a given environmental sample using the appropriate barcode, primers, and amplification conditions (Deagle et al., 2014; Kress et al., 2015), and on the completeness of the reference database (Zepeda Mendoza et al., 2015). Some attempts have been performed to compare morphological vs. metabarcoding-based taxonomic inferences; yet, results are inconclusive as some studies do not apply both approaches to the same sample and/or have focused on a particular taxonomic group (Hajibabaei et al., 2012; Carew et al., 2013; Zhou et al., 2013; Gibson et al., 2014; Cowart et al., 2015; Zimmermann et al., 2015). A recent study (Gibson et al., 2015) has performed morphological and metabarcoding-based taxonomic identification on the same freshwater aquatic invertebrate samples, but limited their visual identifications to family level. Only two studies (Dowle et al., 2015; Elbrecht and Leese, 2015) have performed a robust benchmarking of metabarcoding using freshwater invertebrates and showed that this technique can be successfully applied to biodiversity assessment. In marine metazoans, all studies have focused only on plankton samples (Brown et al., 2015; Mohrbeck et al., 2015; Albaina et al., 2016). Thus, an exhaustive evaluation of metabarcoding for marine benthic metazoan taxonomic inferences is still lacking.
The use of extracellular DNA (the DNA released from cell lysis; Taberlet et al., 2012b) for biodiversity monitoring is increasingly applied to water (e.g., Ficetola et al., 2008; Foote et al., 2012; Thomsen et al., 2012; Kelly et al., 2014; Davy et al., 2015; Valentini et al., 2016), soil (Taberlet et al., 2012b), and sediment samples (Guardiola et al., 2015; Turner et al., 2015; Pearman et al., 2016). Constituting a significant fraction of the total DNA (Dell'Anno and Danovaro, 2005; Pietramellara et al., 2009; Torti et al., 2015), it is assumed that the taxonomic composition of the free DNA present in the environment reflects the biodiversity of the sample (Ficetola et al., 2008), which would simplify DNA extraction protocols (Pearman et al., 2016) and allow the detection of organisms that are even larger than the sample itself (Foote et al., 2012; Thomsen et al., 2012; Kelly et al., 2014; Davy et al., 2015). Thus, this method appears as a promising cost-effective alternative for macroinvertebrate diversity monitoring, but no robust evidence that the entire macroinvertebrate community can be detected using extracellular DNA exists so far.
The lack of a thorough comparison between morphological and metabarcoding-based taxonomic inferences of marine metazoa and of an evaluation of the use of metabarcoding for marine biotic index estimations prevents the application of metabarcoding in routine biomonitoring programs. Here, we benchmark alternative metabarcoding protocols based on a combination of different DNA sources (extracellular DNA and DNA extracted from previously isolated organisms), barcodes (short and long COI regions), and amplification conditions against benthic macroinvertebrate samples of known taxonomic composition. Additionally, we test the effect of the discrepancies between morphological and DNA-based taxonomic inferences in marine biomonitoring through the evaluation of the molecular based taxonomies performance when incorporated for the calculation of the AMBI and prove the suitability of molecular data based biotic indices to assess marine environmental status.
Methods
The experimental design followed to compare the performance of molecular and morphological based taxonomic inferences is summarized in Figure 1.
Sample Collection and Processing
Benthic samples were collected from 11 littoral stations (sampling depth ranging from 100 to 740 m) along the Basque Coast, Bay of Biscay (Supplementary Figure 1), during March 2013, using a van Veen grab (0.07–0.1 m2). At each location, after sediment homogenization, one subsample of sediment was taken from the surficial layer of the grab and stored in a sterile 15 ml falcon tube at −80 °C until extracellular DNA extraction (see below). In order to collect the benthic macroinvertebrate community (organism size >1 mm) present in each sample, the remaining sediment was sieved on site through a 1 mm size mesh, and the retained material preserved in 96% ethanol at 4 °C until processing (<6 months). Macroinvertebrate specimens were sorted and identified to the lowest possible taxonomic level based on morphology. Following taxonomic classification, each sample was divided into two identical subsamples by taking equal amount of tissue per taxa for each subsample. Tissues from one subsample were pooled and used for bulk DNA extraction. Each tissue of the second subsample was used for individual DNA extraction (see below).
Extracellular, Individual, and Bulk DNA Extraction
Extracellular DNA was extracted following an optimized protocol (Taberlet et al., 2012b). Briefly, 5 g of each sediment sample were mixed with 7.5 ml of saturated phosphate buffer and an equal volume of chloroform:isoamyl alcohol (IAA). After centrifugation for 5 min at 4,000 g, the aqueous phase was passed through a second round of chloroform:IAA purification and ethanol precipitated before elution of resulting DNA pellet in 100 μl Milli-Q water. For individual and bulk processing, total genomic DNA from each tissue and from the mix of tissues composing each sample, respectively, were extracted using the Wizard® Genomic DNA Purification kit (Promega, WI, USA) in a 125 μl of Milli-Q water final elution. The possible presence of PCR inhibitors in the bulk and extracellular DNA were removed using the Mobio PowerClean® DNA Clean-Up Kit. Genomic DNA integrity was assessed by electrophoresis, migrating about 100 ng of GelRed™-stained DNA on an agarose 1.0% gel, DNA purity was assessed using the Nanodrop® ND-1000 (Thermo Scientific) system and DNA concentration was determined with the Quant-iT dsDNA HS assay kit using a Qubit® 2.0 Fluorometer (Life Technologies). About 20 ng of each individually extracted DNA were used for DNA barcoding of single species (see details below). Subsequently, 5 μl of each individually extracted DNA at original concentration were pooled (hereafter referred as “pooled DNA”). Extracellular, bulk, and pooled DNA were used for PCR amplification and sequencing (see below).
Individual PCR Amplification and Sanger Sequencing
Individual DNA barcoding was performed for the species for which no COI barcode was available in public databases (see Table 1, Supplementary Material). The standard 658 bp COI barcode (folCOI) was targeted using the dgLCO1490 × dgHCO2198 primer pair (Meyer, 2003). Each individual DNA sample was amplified in a total volume reaction of 20 μl using 10 μl of Phusion® High-Fidelity PCR Master Mix (Thermo Scientific), 0.2 μl of each primer (10 μM), and 20 ng of genomic DNA. The thermocycling profile consisted of an initial 30 s denaturation step at 98 °C, followed by up to 35 cycles of 10 s at 98 °C, 30 s at 48 °C, and 45 s at 72 °C, and a final 5 min extension step at 72 °C. PCR products were considered positive when a clear single band of expected size was visualized on a 1.7% agarose gel. Samples with negative product were further amplified with the mlCOIintF × dgHCO2198 primer pair (Leray et al., 2013) targeting a 313 bp fragment of the COI gene (mlCOI). Negative samples were included with each PCR run as external control. PCR products were purified with ExoSAP-IT (Affymetrix) and Sanger sequenced.
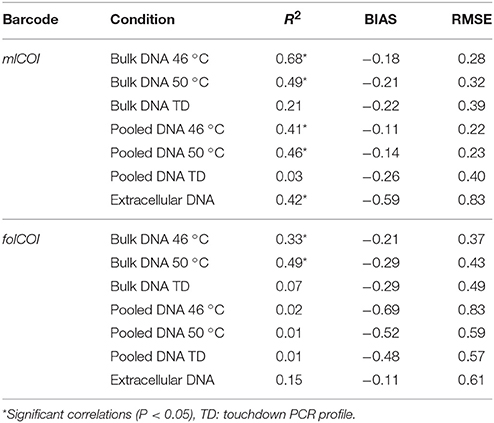
Table 1. Results from the regression model between traditional and molecularly inferred pa-AMBI values.
PCR Amplification for Library Preparation and Illumina Miseq Sequencing
Indexed paired-end libraries of pooled amplicons were prepared using two nested PCRs from the extracellular, bulk and pooled (mix of 5 μl of individually extracted DNA at original concentration) DNA obtained from each of the 11 collected samples. In parallel, three of the samples were processed per triplicate and considered independently in downstream analysis. For the first PCR, two universal primer pairs with overhang Illumina adapters were used to amplify two different length COI barcodes (the mlCOI and the folCOI). Three different PCR profiles were used to amplify each COI barcode from the bulk and pooled DNAs (46 and 50 °C annealing temperatures and a touchdown profile), whilst the extracellular DNA COI barcodes were amplified with 46 °C annealing temperature. PCRs were performed in a total volume of 20 μl using 10 μl of Phusion® High-Fidelity PCR Master Mix (Thermo Scientific), 0.5 μl of each primer (10 μM), and 2 μl of genomic DNA (5 ng/μl). The PCR conditions for the two different annealing temperatures consisted on an initial 30 s denaturation step at 98 °C, 27 cycles of 10 s at 98 °C, 30 s at 46 or 50 °C, and 45 s at 72 °C, and a final 5 min extension at 72 °C. For the touchdown profile the PCR conditions consisted on an initial 30 s denaturation step at 98 °C, 16 cycles of 10 s at 98 °C, 30 s at 62 °C (−1 °C per cycle), and 60 s at 72 °C, followed by 17 cycles at 46 °C annealing temperature, and a final 5 min extension at 72 °C (Leray et al., 2013). Negative controls were included with each PCR. Generated amplicons were purified with AMPure XP beads (Beckman Coulter), eluted in 50 μL MilliQ water and used as templates for the generation of the dual-indexed amplicons in the second PCR round following the “16S Metagenomic Sequencing Library Preparation” protocol (Illumina). Purified PCR products were quantified using the Quant-iT dsDNA HS assay kit using a Qubit® 2.0 Fluorometer (Life Technologies) and further normalized for all samples. Pools of 96 equal concentration amplicons were sequenced using the 2 × 300 paired-end on a MiSeq (Illumina).
DNA Barcode Reference Database
Trace files of Sanger sequences obtained from individual PCR amplifications were edited and trimmed to remove low quality bases (Q < 30) using SeqTrace 0.9.0 (Stucky, 2012) and checked for frame shifts using EXPASY (Gasteiger et al., 2003). COI sequences are available in “BCAS project” at BOLD (http://www.boldsystems.org) and in GenBank (accession numbers KT307619–KT307707). To generate our DNA reference database, we retrieved a total of 1,123,601 public COI aligned sequences from 96,641 different taxa from BOLD (October 2014), including the sequences generated in this study (COI RefSeq). After removing duplicates, a total of 505,033 sequences were kept and trimmed to the 658 bp Folmer COI fragment to generate the “BOLD database.” A smaller customized DNA reference database was generated using the 4231 sequences corresponding to species included in the AMBI list (see below; available at http://ambi.azti.es) extracted from the “BOLD database” to build the “AMBI database.” For the analyses of the folCOI reads, the 249 bp not sequenced internal fragment (see below) was removed from these two databases to construct the “BOLD gapped database” and the “AMBI gapped database.” The four resulting databases were formatted according to mothur (Schloss, 2009) standards.
Amplicon Sequence Analysis
Demultiplexed reads were quality checked using FastQC (Andrews, 2010) and primer sequences removed using Trimmomatic 0.33 (Bolger et al., 2014). Since the mlCOI paired-end reads overlap in 237 bp and the folCOI paired-end reads do not overlap, different preprocessing steps are needed for each COI fragment. Forward and reverse mlCOI reads were merged using FLASH (Magoč and Salzberg, 2011) with a minimum and maximum overlap of, respectively, 20 bases below and above the expected overlapping region, and the resulting reads were trimmed using Trimmomatic at the first sliding window of 50 bp with an average quality score below 30. The folCOI forward and reverse reads were trimmed at 260 and 200 bp, respectively, based on the quality decrease after these positions observed on FastQC plots. Each pair of forward and reverse-complemented reverse read was pasted to create a 409 bp read that corresponds to the folCOI barcode without a 249 bp internal fragment. Further details on this new pipeline developed to analyze the universal 658 bp COI barcode which is too long for most HTS applications such as the Illumina MiSeq are detailed elsewhere (Aylagas and Rodríguez-Ezpeleta, 2016). Preprocessed reads from both barcodes were independently analyzed with mothur following the MiSeq standard operating procedure (Kozich et al., 2013). Briefly, sequences with ambiguous bases were discarded and the rest, aligned to the corresponding BOLD and AMBI reference databases. Only those mlCOI and folCOI reads aligning inside the barcode region and longer than 200 and 300 bp, respectively, were kept. After chimera removal using the de novo mode of UCHIME (Edgar et al., 2011), sequences were grouped into phylotypes according to the taxonomic assignments made based on the Wang method (Wang et al., 2007) using a bootstrap value of 90. The sequences that did not return any taxonomic assignment against the BOLD database were blasted against the NCBI non redundant database. Sequences have been deposited in the Dryad Digital Repository (http://dx.doi.org/10.5061/dryad.0sc0s).
Comparison of Morphological and Metabarcoding-Based Taxonomic Compositions
Only taxa representing at least 0.01% of the reads in one station were considered present in the taxonomic composition inferred from molecular data. An in-house script (Supplementary Figure 2) was used to calculate the degree of match between the molecular and morphologically inferred taxonomic compositions of each station. The detection success was normalized for each sample and transformed to percentage of matches (100% of matches means all taxa identified based on morphology have been detected using DNA-based approaches). Differences in mean values of the taxa detection percentages between DNA extraction methods, primers and PCR conditions were examined using a t-test at alpha = 0.05. Patterns of sample dissimilarity were visualized using non-metric multidimensional scaling (nMDS) based on taxa presence/absence and abundance using the Jaccard and Bray-Curtis indices, respectively, obtained using molecular approaches.
Comparison of Morphological and Metabarcoding-Based Biotic Indices
In order to compare morphological and metabarcoding-based biotic indices, we used AMBI, which is a status assessment index based on the pollution tolerances of the taxa present in a sample, with tolerance being expressed categorically into ecological groups (EGI, sensitive to pressure; EGII, indifferent; EGIII, tolerant; EGIV, opportunist of second order; and EGV, opportunist of first order). We calculated the presence/absence morphology-based AMBI (pa-AMBI) and the presence/absence genetics-based AMBI (pa-gAMBI; Aylagas et al., 2014) inferred through DNA metabarcoding of each sample, using the AMBI 5.0 software (http://ambi.azti.es). The relationships among pa-AMBI and pa-gAMBI values were examined using standardized major axis (SMA) estimation (Warton et al., 2006) using the software SMATR (Falster et al., 2003). In order to evaluate the performance of pa-gAMBI for each condition, root-mean-square error (RMSE) and bias were calculated (Walther and Moore, 2005).
Results
Morphological and Molecular Analysis
In total, 138 macroinvertebrate taxa belonging to nine different phyla were morphologically identified in the 11 stations. Representatives of two main phyla, Annelida, and Arthropoda, are present at all stations, with 94 and 21 taxa, respectively, whereas less represented phyla (Mollusca, Chaetognata, Cnidaria, Echinodermata, Nemertea, Nematoda, and Sipuncula) are absent from some stations and include less number of taxa (Supplementary Table 1). Individual DNA barcoding was successful on 61 and 24 of the 106 identified species with no COI barcode in public databases, for which new folCOI and mlCOI barcodes were generated, respectively, and included in the reference database. Despite this effort to increase the reference database, 21 species remain without barcode because amplification of both barcodes failed.
For each station, two condition combinations were tested for the extracellular DNA (two different barcodes) and six for the bulk and pooled DNAs (two different barcodes and three different PCR profiles). From the 238 samples analyzed, including triplicates performed on three of the stations, 14 had no PCR amplification (see Supplementary Table 2 for clarification on the number of samples produced for molecular analysis). The 224 remaining samples resulted in 16 million reads, from which about 56% passed quality filters and were used for taxonomic analysis (Supplementary Table 2). Of the total reads obtained from extracellular DNA, 71.5 and 73.4% could not be assigned to any metazoan phylum using the customized BOLD database and 24.9 and 25.6% were not assigned to Metazoa for mlCOI and folCOI, respectively. When blasted against NCBI, the reads obtained using mlCOI matched with bacteria (0.6%), non-metazoan eukaryotes (84%), metazoans (12.2%), or did not provide any match (3%), and the reads obtained using folCOI matched with bacteria (66.6%), non-metazoan eukaryotes (6%), metazoans (4.2%), archaea (0.05%), or did not provide any match (23.2%). The percentages of non-metazoan reads are much lower for bulk (0.03 and 0.04%) and pooled DNA (0.1 and 0.3%), and the proportion of Metazoa reads with no phylum assigned are lower for mlCOI (23.2 and 10.6% for bulk and pooled DNA, respectively) than for folCOI (29.94 and 31.6% for bulk and pooled DNA, respectively).
Comparison of Morphological and Molecular-Based Taxonomic Compositions
From the taxonomic inferences obtained using molecular approaches, only macroinvertebrates were considered for sample comparison (e.g., Chordata records were excluded for downstream analysis). The average percentage of recovered taxa (molecular taxonomy matches visual taxonomy) over all stations using different conditions is shown in Figure 2 (see Supplementary Figure 3 for percentage of recovered taxa considering only species level identification). Matches for taxonomic inferences based on metabarcoding of extracellular DNA are very low (3.4 and 3.1% for folCOI and mlCOI respectively), with only taxa from three phyla (Mollusca, Annelida, and Nemertea) retrieved (Supplementary Table 3). Results obtained between replicates from the same sample reveal similar taxonomic inferences. No significant differences were observed between the percentage of matches obtained using bulk and pooled DNA (p > 0.05). Interestingly, the mlCOI barcode outperforms the folCOI barcode (p < 0.05 for bulk and pooled DNA) and, within the mlCOI, the 46 and 50 °C annealing temperatures outperform the touchdown profile both for bulk and pooled DNA (p < 0.05). Overall, the best performing condition is the mlCOI barcode amplified using 46 °C annealing temperature, which results in a percentage of recovered taxa of 62.4% for all matches and of 76.3% for only matches at species level.
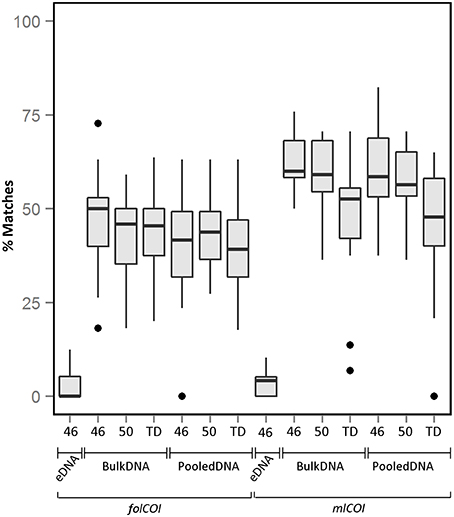
Figure 2. Boxplot showing the percentage of matches obtained between morphological and molecularly inferred taxonomic compositions over all stations. All matches using extracellular DNA (eDNA), bulk and pooled DNA approaches using different PCR conditions (46 or 50 °C annealing temperatures or TD: touchdown profile) for folCOI and mlCOI barcodes.
Using molecular approaches we were able to retrieve taxa that had not been morphologically identified. Representatives of Annelida (e.g., Tubificoides amplivasatus, Chloeia parva, and Mugga wahrbergi), Arthropoda (e.g., Scyllarus arctus and Limnoria sp.), Mollusca (e.g., Nucula nucleus, Galeomma turtoni, Thyasira ferruginea, and Entalina tetragona), and Echinodermata (e.g., Ophiura albida and Macrophiothrix sp.) were solely identified using DNA-based approaches. Moreover, we were able to find taxa belonging to two phyla that were not morphologically identified even at phylum level: two families (Triaenophoridae and Echinobothriidae) and one order (Acoeala) of Platyhelminthes and one family (Hemiasterellidae) of Porifera. As illustrated by the nMDS ordination plot of beta diversity (Figure 3), the greatest disparity in macroinvertebrate composition inferred using molecular taxonomy of each station was shown by the extracellular DNA approach.
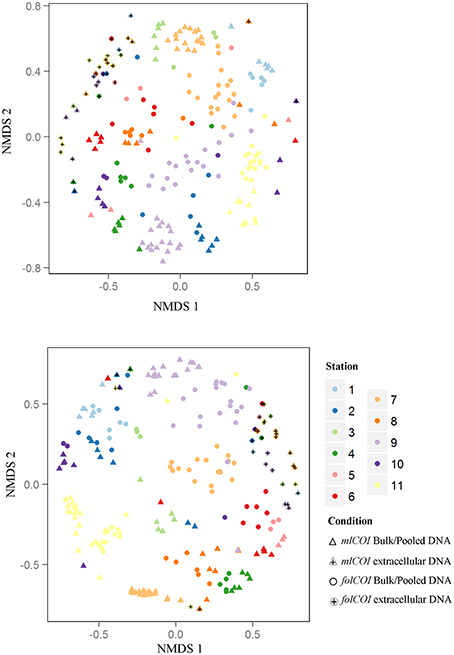
Figure 3. Non-metric multidimensional scaling (nMDS) plots. (Top) Jaccard (presence-absence) and (Bottom) Bray-Curtis (abundance) dissimilarities for 32 samples of extracellular DNA and 192 samples of bulk or pooled DNA approaches, from 11 littoral stations for the two barcodes (mlCOI and folCOI).
Comparison of Morphological and Metabarcoding-Based Biotic Indices
The correlation between pa-AMBI and pa-gAMBI values obtained from the taxonomic composition inferences using the AMBI database is shown in Figure 4. The pa-AMBI values that best correlate with pa-gAMBI values are those obtained using bulk and pooled DNA approaches at 46 or 50 °C annealing temperatures obtained with mlCOI (Table 1). Generally, pa-gAMBI values tend to score lower than pa-AMBI values (negative bias over all stations). This tendency can be also observed in the variation of the percentage of taxa found belonging to each ecological group obtained using morphological and molecular taxonomic identifications (Supplementary Figure 4). The non-detection of taxa belonging to tolerant and opportunistic ecological groups (III, IV, and V) when using folCOI, especially for pooled DNA method, leads to poor correlations between pa-AMBI and pa-gAMBI values.
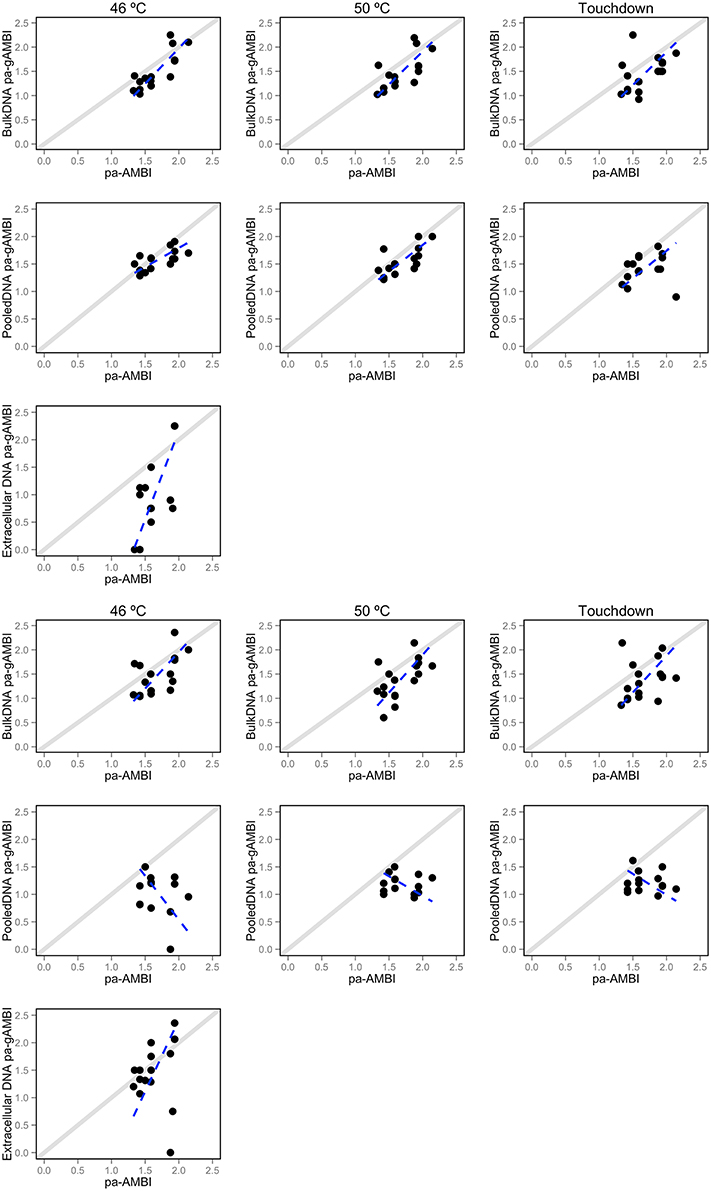
Figure 4. Relationship between pa-AMBI and pa-gAMBI values. For each DNA-based approach (extracellular, bulk and pooled DNA) and PCR condition (46 or 50 °C annealing temperatures or Touchdown profile) displayed separately for each barcode—mlCOI (top 3 rows) and folCOI (bottom 3 rows). Each dot shows the relationship between the pa-AMBI (x-axis) and pa-gAMBI value (y-axis) for each station. The dotted lines represent the results of model II regression and the diagonal showing perfect correlation between the two observations is depicted.
Discussion
Effect of PCR-Based Analysis Biases on Taxonomic Inferences
Finding the primer pair and PCR conditions that most accurately recover the organisms present in an environmental sample is crucial for a successful application of metabarcoding to biomonitoring. Several studies analyzing the same samples with morphological and molecular taxonomy have been performed so far to benchmark COI based metabarcoding in animals, all focusing exclusively on freshwater or terrestrial macroinvertebrates (Hajibabaei et al., 2012; Carew et al., 2013; Gibson et al., 2014; Dowle et al., 2015; Elbrecht and Leese, 2015) or carried out under morphological identifications limited to high taxonomic levels (Gibson et al., 2015). Thus, studies on marine benthic communities that prove the suitability of DNA-based approaches for environmental biomonitoring are lacking. Using samples of known taxonomic composition, we show that an alternative barcode that targets a shorter region of the COI gene outperforms the 658 bp region that is commonly used for metabarcoding metazoans (Carew et al., 2013; Ji et al., 2013; Dowle et al., 2015; Elbrecht and Leese, 2015; Zaiko et al., 2015). Our data corroborate previous studies unveiling the lack of universality in the COI primers, which is translated to biases during PCR step (Pochon et al., 2013; Deagle et al., 2014). However, the increased performance of the short region, previously demonstrated for individual barcoding on marine metazoans (Leray et al., 2013) and metabarcoding in insects (Brandon-Mong et al., 2015) proves that the mlCOI barcode retrieves a high proportion of the morphologically identified taxa. This fact also corroborates the preferred use of small barcodes for metabarcoding, which provide pair-end overlaps on Illumina sequencing and good taxonomic resolution for species identification (Meusnier et al., 2008). Additionally, the folCOI barcode returns more reads with no match and metazoan reads not assigned to any specific phylum, which could be attributed to the fact that longer barcodes can accumulate more errors during the PCR and sequencing processes (Schirmer et al., 2015).
The effect of the PCR annealing temperature has been shown to affect retrieved taxonomic composition in bacterial and archaeal metabarcoding using the 16S rRNA gene (Sipos et al., 2007; Lee et al., 2012; Pinto and Raskin, 2012). Here, we show that the use of inappropriate PCR conditions can also affect the final taxonomic assignment in metazoan metabarcoding analyses. Our results show that a constant low annealing temperature (46 or 50 °C) provides more accurate taxonomic inferences compared to the touchdown profile, which contrasts with previous studies (Hansen et al., 1998; Simpson et al., 2000; Leray et al., 2013). Moreover, it is well-established that the more PCR cycles, the more spurious sequences and chimera are formed during PCR (Haas et al., 2011), which could explain the lower taxa detection rate when using the touchdown profile (which includes five more cycles). Further, the nature of the organisms and their size may bias DNA extraction (i.e., hard shells or chitin exoskeleton can prevent cell lysis and DNA from small organisms can be less effectively extracted). Here, we have ensured that DNA from all organisms is present in the pooled sample by pooling individually extracted DNAs, and show that the results of the pooled DNA and bulk extracted DNA are comparable.
The Use of Extracellular DNA for Biodiversity Estimations
The extracellular DNA-based metabarcoding for biodiversity assessments has the potential of detecting big-size organisms in small samples, which facilitates sampling strategies and could resulting in a more cost-effective approach for environmental biomonitoring (Taberlet et al., 2012b; Thomsen et al., 2012; Thomsen and Willerslev, 2015). Several studies have used extracellular DNA from the water column to detect vertebrates (Ficetola et al., 2008; Thomsen et al., 2012; Valentini et al., 2016) freshwater macroinvertebrates (Goldberg et al., 2013; Mächler et al., 2014) and benthic eukaryotes (Guardiola et al., 2015; Pearman et al., 2016). Yet, so far, this approach has not been proved valid for biodiversity assessment as no comparison with samples of known taxonomic composition has been performed. To our knowledge, only one attempt exists to detect the whole freshwater benthic macroinvertebrate community from extracellular DNA extracted from samples of known composition (Hajibabaei et al., 2012), but the authors used the preservative ethanol as controlled environment containing the free DNA rather than natural scenarios. In our analyses, only a small proportion of the taxa identified using morphological methods are retrieved using extracellular DNA present in the sediment. Indeed, even considering the taxa not identified through morphological taxonomy, the extracellular DNA-based analyses only identify 30 macroinvertebrate taxa over all stations, which is much lower than the total diversity inferred from morphology and from DNA extracted from the isolated organisms. Therefore, the striking differences obtained between morphological and extracellular DNA metabarcoding based taxonomic inferences suggest that further studies are needed before using sediment extracellular DNA as a suitable source for macroinvertebrate biodiversity assessment; yet, more experiments testing the effect of sediment sample size, DNA degradation scenarios, or DNA extraction protocols are required, as it is possible that sampling more deeply in the sediment, or using the water column provides better results, and/or that the optimal DNA extraction procedure has not been employed (Corinaldesi et al., 2005).
Effect Misinterpreting Community Composition in Environmental Biomonitoring
Environmental biomonitoring programs rely on the detection of a wide range of taxonomic groups, which are usually amplified using universal primers (Leray et al., 2013). The abovementioned biases inherent to PCR-based analyses can lead to greater recovery of sequences of some species and the exclusion of others (Elbrecht and Leese, 2015; Piñol et al., 2015). Thus, it is important to see whether in samples containing species from numerous phyla, metabarcoding is also able to retrieve a high proportion of taxa that suffices for environmental monitoring. In general, we show a high percentage of recovery using bulk DNA among the nine different phyla identified using morphological approach. However, in our metabarcoding analyses, some taxa identified using morphological methodologies remain undetected using both short and long COI barcodes, whereas others appear only using metabarcoding. The species exclusively detected using metabarcoding represent potential cryptic species (e.g., Tyasira flexuosa/Thyasira ferruginea and Ophiura texturata/Ophiura albida) or unable to be classified based on morphological characters. Further, some additional identified taxa [i.e., two phyla detected from extracellular DNA (Platyhelminthes and Porifera)] may either represent organisms which had been missed by taxonomy based on morphology and metabarcoding from previously isolated organisms due to their small size (<1 mm) or detected due to the fact that the free DNA has been transported from other localities (Roussel et al., 2015).
Consequences of the misinterpretation of the taxonomic composition could result in erroneous biodiversity assessment, which may impede the implementation of DNA metabarcoding in regular biomonitoring programs (Chariton et al., 2015; Cowart et al., 2015; Lejzerowicz et al., 2015; Zaiko et al., 2015). In particular, calculation of biotic indices based on pollution tolerances assigned to the taxa retrieved from the sample (Maurer et al., 1999; Borja et al., 2000) may be affected by the approach used for taxonomic assignment. We show that, despite using the metabarcoding conditions that most accurately detect the morphologically identified taxa, some differences between both approaches are observed. Yet, in general, pa-gAMBI values obtained from metabarcoding analyses provide significant presence-absence community estimations and can be used for calculating biotic indices.
Conclusions
Representing a promising opportunity to overcome the time-consuming and high cost of traditional methodologies for species identification, it is anticipated that DNA metabarcoding will be routinely used in biomonitoring programs in the near future. Yet, the application of this technique to regular biomonitoring programs requires benchmarking and standardization. Here, we demonstrate through an exhaustive study design that, using the appropriate conditions, metabarcoding presents a great potential to characterize biodiversity and to provide accurate biotic indices. Thus, our findings will contribute to accelerating the implementation of metabarcoding for environmental status assessment.
Additional Information
Accession codes: All Sanger and Illumina generated sequences have been deposited in GenBank (accession numbers KT307619–KT307707) and DRYAD (http://dx.doi.org/10.5061/dryad.0sc0s).
Author Contributions
Conceived and designed the study: EA, AB, and NRE. Performed the experiments: EA. Contributed reagents/materials: XI. Analyzed the data: EA and NRE. Interpreted the data and wrote the paper: EA, AB, and NRE. All authors reviewed the manuscript.
Funding
This manuscript is a result of the DEVOTES (DEVelopment Of innovative Tools for understanding marine biodiversity and assessing good Environmental Status—http://www.devotes-project.eu) project funded by the European Union (7th Framework Program “The Ocean of Tomorrow” Theme, grant agreement no. 308392) and the Basque Water Agency (URA) through a Convention with AZTI. EA is supported by the “Fundación Centros Tecnológicos” through an “Iñaki Goenaga” doctoral grant.
Conflict of Interest Statement
The authors declare that the research was conducted in the absence of any commercial or financial relationships that could be construed as a potential conflict of interest.
Acknowledgments
We thank Iñaki Mendibil and Craig T. Michell for technical assistance, Iñigo Muxika, Jon Corell, and Germán Rodríguez for discussions and Vega Asensio (www.norarte.es) for preparing Figure 1. The specimen taxonomic identification was done by experts from the Cultural Society INSUB. This paper is contribution number 770 from AZTI (Marine Research Division).
Supplementary Material
The Supplementary Material for this article can be found online at: http://journal.frontiersin.org/article/10.3389/fmars.2016.00096
References
Albaina, A., Aguirre, M., Abad, D., Santos, M., and Estonba, A. (2016). 18S rRNA V9 metabarcoding for diet characterization: a critical evaluation with two sympatric zooplanktivorous fish species. Ecol. Evol. 6, 1809–1824. doi: 10.1002/ece3.1986
Andrews, S. (2010). FastQC: A Quality Control Tool for High Throughput Sequence Data. Available online at: http://www.bioinformatics.babraham.ac.uk/projects/fastqc
Aylagas, E., Borja, A., and Rodríguez-Ezpeleta, N. (2014). Environmental status assessment using DNA metabarcoding: towards a genetics based Marine Biotic Index (gAMBI). PLoS ONE 9:e90529. doi: 10.1371/journal.pone.0090529
Aylagas, E., and Rodríguez-Ezpeleta, N. (2016). “Analysis of Illumina MiSeq amplicon reads: application to benthic indices for environmental monitoring,” in Marine Genomics Methods and Protocols, Methods in Molecular Biology, ed S. J. Bourlat (New York, NY: Springer), 1452.
Bolger, A. M., Lohse, M., and Usadel, B. (2014). Trimmomatic: a flexible trimmer for Illumina Sequence Data. Bioinformatics 30, 2114–2120. doi: 10.1093/bioinformatics/btu170
Borja, A., Franco, J., and Perez, V. (2000). A marine biotic index to establish the ecological quality of soft-bottom benthos within European estuarine and coastal environments. Mar. Pollut. Bull. 40, 12. doi: 10.1016/S0025-326X(00)00061-8
Borja, Á., Marín, S. L., Muxika, I., Pino, L., and Rodriguez, J. G. (2015). Is there a possibility of ranking benthic quality assessment indices to select the most responsive to different human pressures? Mar. Pollut. Bull. 97, 85–94. doi: 10.1016/j.marpolbul.2015.06.030
Bourlat, S. J., Borja, A., Gilbert, J., Taylor, M. I., Davies, N., Weisberg, S. B., et al. (2013). Genomics in marine monitoring: new opportunities for assessing marine health status. Mar. Pollut. Bull. 74, 19–31. doi: 10.1016/j.marpolbul.2013.05.042
Brandon-Mong, G. J., Gan, H. M., Sing, K. W., Lee, P. S., Lim, P. E., and Wilson, J. J. (2015). DNA metabarcoding of insects and allies: an evaluation of primers and pipelines. Bull. Entomol. Res. 105, 717–727. doi: 10.1017/S0007485315000681
Brown, E. A., Chain, F. J., Crease, T. J., MacIsaac, H. J., and Cristescu, M. E. (2015). Divergence thresholds and divergent biodiversity estimates: can metabarcoding reliably describe zooplankton communities? Ecol. Evol. 5, 2234–2251. doi: 10.1002/ece3.1485
Carew, M. E., Pettigrove, V. J., Metzeling, L., and Hoffmann, A. A. (2013). Environmental monitoring using next generation sequencing: rapid identification of macroinvertebrate bioindicator species. Front. Zool. 10:45. doi: 10.1186/1742-9994-10-45
Carugati, L., Corinaldesi, C., Dell'Anno, A., and Danovaro, R. (2015). Metagenetic tools for the census of marine meiofaunal biodiversity: an overview. Mar. Genomics 24, 11–20. doi: 10.1016/j.margen.2015.04.010
Chain, F. J. J., Brown, E. A., MacIsaac, H. J., and Cristescu, M. E. (2016). Metabarcoding reveals strong spatial structure and temporal turnover of zooplankton communities among marine and freshwater ports. Divers Distrib. 22, 493–504. doi: 10.1111/ddi.12427
Chariton, A. A., Court, L. N., Hartley, D. M., Colloff, M. J., and Hardy, C. M. (2010). Ecological assessment of estuarine sediments by pyrosequencing eukaryotic ribosomal DNA. Front. Ecol. Environ. 8:5. doi: 10.1890/090115
Chariton, A. A., Stephenson, S., Morgan, M. J., Steven, A. D., Colloff, M. J., Court, L. N., et al. (2015). Metabarcoding of benthic eukaryote communities predicts the ecological condition of estuaries. Environ. Pollut. 203, 165–174. doi: 10.1016/j.envpol.2015.03.047
Corinaldesi, C., Danovaro, R., and Dell'Anno, A. (2005). Simultaneous recovery of extracellular and intracellular DNA suitable for molecular studies from marine sediments. Appl. Environ. Microbiol. 71, 46–50. doi: 10.1128/AEM.71.1.46-50.2005
Cowart, D. A., Pinheiro, M., Mouchel, O., Maguer, M., Grall, J., Miné, J., et al. (2015). Metabarcoding is powerful yet still blind: a comparative analysis of morphological and molecular surveys of seagrass communities. PLoS ONE 10:e0117562. doi: 10.1371/journal.pone.0117562
Cristescu, M. E. (2014). From barcoding single individuals to metabarcoding biological communities: towards an integrative approach to the study of global biodiversity. Trends Ecol. Evol. 29, 566–571. doi: 10.1016/j.tree.2014.08.001
Dafforn, K. A., Baird, D. J., Chariton, A. A., Sun, M. Y., Brown, M. V., Simpson, S. L., et al. (2014). Faster, higher and stronger? The pros and cons ofmolecular faunal data for assessing ecosystem condition. Adv. Ecol. Res. 51, 1–40.
Davy, C. M., Kidd, A. G., and Wilson, C. C. (2015). Development and validation of Environmental DNA (eDNA) markers for detection of freshwater turtles. PLoS ONE 10:e0130965. doi: 10.1371/journal.pone.0130965
Deagle, B. E., Jarman, S. N., Coissac, E., Pompanon, F., and Taberlet, P. (2014). DNA metabarcoding and the cytochrome c oxidase subunit I marker: not a perfect match. Biol. Lett. 10:20140562. doi: 10.1098/rsbl.2014.0562
Dell'Anno, A., Carugati, L., Corinaldesi, C., Riccioni, G., and Danovaro, R. (2015). Unveiling the biodiversity of deep-sea nematodes through metabarcoding: are we ready to bypass the classical taxonomy? PLoS ONE 10:e0144928. doi: 10.1371/journal.pone.0144928
Dell'Anno, A., and Danovaro, R. (2005). Extracellular DNA plays a key role in deep-sea ecosystem functioning. Science 309, 2179–2179. doi: 10.1126/science.1117475
Diaz, R. J., Solan, M., and Valente, R. M. (2004). A review of approaches for classifying benthic habitats and evaluating habitat quality. J. Environ. Manage. 73, 165–181. doi: 10.1016/j.jenvman.2004.06.004
Dowle, E. J., Pochon, X., Banks, J., Shearer, K., and Wood, S. A. (2015). Targeted gene enrichment and high throughput sequencing for environmental biomonitoring: a case study using freshwater macroinvertebrates. Mol. Ecol. Resour. doi: 10.1111/1755-0998.12488. [Epub ahead of print].
Edgar, R. C., Haas, B. J., Clemente, J. C., Quince, C., and Knight, R. (2011). UCHIME improves sensitivity and speed of chimera detection. Bioinformatics 27, 2194–2200. doi: 10.1093/bioinformatics/btr381
Elbrecht, V., and Leese, F. (2015). Can DNA-based ecosystem assessments quantify species abundance? Testing primer bias and biomass–sequence relationships with an innovative metabarcoding protocol. PLoS ONE 10:e0130324. doi: 10.1371/journal.pone.0130324
Falster, D., Warton, D., and Wright, I. (2003). SMATR: Standardised Major Axis Tests And Routines. Available online at: http://www.bio.mq.edu.au/ecology/SMATR
Ficetola, G. F., Miaud, C., Pompanon, F., and Taberlet, P. (2008). Species detection using environmental DNA from water samples. Biol. Lett. 4, 423–425. doi: 10.1098/rsbl.2008.0118
Fonseca, V. G., Carvalho, G. R., Nichols, B., Quince, C., Johnson, H. F., Neill, S. P., et al. (2014). Metagenetic analysis of patterns of distribution and diversity of marine meiobenthic eukaryotes. Glob. Ecol. Biogeogr. 23, 1293–1302. doi: 10.1111/geb.12223
Foote, A. D., Thomsen, P. F., Sveegaard, S., Wahlbert, M., Kielgast, J., Kyhn, L. A., et al. (2012). Investigating the potential use of environmental DNA (eDNA) for genetic monitoring of marine mammals. PLoS ONE 7:e41781. doi: 10.1371/journal.pone.0041781
Gasteiger, E., Gattiker, A., Hoogland, C., Ivanyi, I., Appel, R. D., and Bairoch, A. (2003). ExPASy: the proteomics server for in-depth protein knowledge and analysis. Nucleic Acids Res. 31, 3784–3788. doi: 10.1093/nar/gkg563
Gibson, J., Shokralla, S., Curry, C., Baird, D. J., Monk, W. A., King, I., et al. (2015). Large-scale biomonitoring of remote and threatened ecosystems via high-throughput sequencing. PLoS ONE 10:e0138432. doi: 10.1371/journal.pone.0138432
Gibson, J., Shokralla, S., Porter, T. M., King, I., van Konynenburg, S., Janzen, D. H., et al. (2014). Simultaneous assessment of the macrobiome and microbiome in a bulk sample of tropical arthropods through DNA metasystematics. Proc. Natl. Acad. Sci. U.S.A. 111, 8007–8012. doi: 10.1073/pnas.1406468111
Goldberg, C. S., Sepulveda, A., Ray, A., Baumgardt, J., and Waits, L. P. (2013). Environmental DNA as a new method for early detection of New Zealand mudsnails (Potamopyrgus antipodarum). Freshwater Sci. 32, 792–800. doi: 10.1899/13-046.1
Guardiola, M., Uriz, M. J., Taberlet, P., Coissac, E., Wangensteen, O. S., and Turon, X. (2015). Deep-Sea, deep-sequencing: metabarcoding extracellular DNA from sediments of marine canyons. PLoS ONE 10:e0139633. doi: 10.1371/journal.pone.0139633
Haas, B. J., Gevers, D., Earl, A. M., Feldgarden, M., Ward, D. V., Giannoukos, G., et al. (2011). Chimeric 16S rRNA sequence formation and detection in Sanger and 454-pyrosequenced PCR amplicons. Genome Res. 21, 494–504. doi: 10.1101/gr.112730.110
Hajibabaei, M. (2012). The golden age of DNA metasystematics. Trends Genet. 28, 535–537. doi: 10.1016/j.tig.2012.08.001
Hajibabaei, M., Spall, L. J., Shodralla, S., and Konynenburg, S. (2012). Assessing biodiversity of a freshwater benthic macroinvertebrate community through non-destructive environmental barcoding of DNA from preservative ethanol. BMC Ecol. 12:28. doi: 10.1186/1472-6785-12-28
Hansen, M. C., Tolker-Nielsen, T., Givskov, M., and Molin, S. (1998). Biased 16S rDNA PCR amplification caused by interference from DNA flanking the template region. FEMS Microbiol. Ecol. 26, 141–149. doi: 10.1111/j.1574-6941.1998.tb00500.x
Ji, Y., Ashton, L., Pedley, S. M., Edwards, D. P., Tang, Y., Nakamura, A., et al. (2013). Reliable, verifiable and efficient monitoring of biodiversity via metabarcoding. Ecol. Lett. 16, 1245–1257. doi: 10.1111/ele.12162
Johnston, E. L., and Roberts, D. A. (2009). Contaminants reduce the richness and evenness of marine communities: a review and meta-analysis. Environ. Pollut. 157, 1745–1752. doi: 10.1016/j.envpol.2009.02.017
Kelly, R. P., Port, J. A., Yamahara, K. M., and Crowder, L. B. (2014). Using environmental DNA to census marine fishes in a large mesocosm. PLoS ONE 9:e86175. doi: 10.1371/journal.pone.0086175
Kozich, J. J., Westcott, S. L., Baxter, N. T., Highlander, S. K., and Schloss, P. D. (2013). Development of a dual-index sequencing strategy and curation pipeline for analyzing amplicon sequence data on the MiSeq Illumina sequencing platform. Appl. Environ. Microbiol. 79, 5112–5120. doi: 10.1128/AEM.01043-13
Kress, W. J., García-Robledo, C., Uriarte, M., and Erickson, D. L. (2015). DNA barcodes for ecology, evolution, and conservation. Trends Ecol. Evol. 30, 25–35. doi: 10.1016/j.tree.2014.10.008
Lee, C. K., Herbold, C. W., Polson, S. W., Wommack, K. E., Williamson, S. J., McDonald, I. R., et al. (2012). Groundtruthing next-gen sequencing for microbial ecology-biases and errors in community structure estimates from PCR amplicon pyrosequencing. PLoS ONE 7:e44224. doi: 10.1371/journal.pone.0044224
Lejzerowicz, F., Esling, P., Pillet, L., Wilding, T. A., Black, K. D., and Pawlowski, J. (2015). High-throughput sequencing and morphology perform equally well for benthic monitoring of marine ecosystems. Sci. Rep. 5:13932. doi: 10.1038/srep13932
Leray, M., and Knowlton, N. (2015). DNA barcoding and metabarcoding of standardized samples reveal patterns of marine benthic diversity. Proc. Natl. Acad. Sci. U.S.A. 112, 2076–2081. doi: 10.1073/pnas.1424997112
Leray, M., Yang, Y. J., Meyer, P. C., Mills, C. S., Agudelo, N., Ranwez, V., et al. (2013). New versatile primer set targeting a short fragment of the mitochondrial COI region for metabarcoding metazoan diversity: application for characterizing coral reef fish gut contents. Front. Zool. 10:34. doi: 10.1186/1742-9994-10-34
Machida, J. R., and Knowlton, N. (2012). PCR Primers for metazoan nuclear 18S and 28S ribosomal DNA sequences. PLoS ONE 7:e46180. doi: 10.1371/journal.pone.0046180
Mächler, E., Deiner, K., Steinmann, P., and Altermatt, F. (2014). Utility of environmental DNA for monitoring rare and indicator macroinvertebrate species. Freshwater Sci. 33, 1174–1183. doi: 10.1086/678128
Magoč, T., and Salzberg, S., L. (2011). FLASH: fast length adjustment of short reads to improve genome assemblies. Bioinformatics 27, 2957–2963. doi: 10.1093/bioinformatics/btr507
Maurer, D., Nguyen, H., Robertson, G., and Gerlinger, T. (1999). The Infaunal Trophic Index (ITI): its suitability for marine environmental monitoring. Ecol. Appl. 9, 14. doi: 10.1890/1051-0761(1999)009[0699:TITIII]2.0.CO;2
Meusnier, I., Singer, G. A., Landry, J. F., Hickey, D. A., Hebert, P. D., and Hajibabaei, M. (2008). A universal DNA mini-barcode for biodiversity analysis. BMC Genomics 9:214. doi: 10.1186/1471-2164-9-214
Meyer, P. C. (2003). Molecular systematics of cowries (Gastropoda: Cypraeidae) and diversification patterns in the tropics. Biol. J. Linn. Soc. 79, 401–459. doi: 10.1046/j.1095-8312.2003.00197.x
Mohrbeck, I., Raupach, M. J., Martínez Arbizu, P., Knebelsberger, T., and Laakmann, S. (2015). High-throughput sequencing-the key to rapid biodiversity assessment of marine metazoa? PLoS ONE 10:e0140342. doi: 10.1371/journal.pone.0140342
Pawlowski, J., Esling, P., Lejzerowicz, F., Cedhagen, T., and Wilding, T. A. (2014). Environmental monitoring through protist next-generation sequencing metabarcoding: assessing the impact of fish farming on benthic foraminifera communities. Mol. Ecol. Resour. 14, 1129–1140. doi: 10.1111/1755-0998.12261
Pearman, J. K., Irigoien, X., and Carvalho, S. (2016). Extracellular DNA amplicon sequencing reveals high levels of benthic eukaryotic diversity in the central Red Sea. Mar. Genomics 26, 29–39. doi: 10.1016/j.margen.2015.10.008
Pietramellara, G., Ascher, J., Borgogni, F., Ceccherini, M. T., Guerri, G., and Nannipieri, P. (2009). Extracellular DNA in soil and sediment: fate and ecological relevance. Biol. Fertil. Soils 45, 219–235. doi: 10.1007/s00374-008-0345-8
Piñol, J., Mir, G., Gomez-Polo, P., and Agustí, N. (2015). Universal and blocking primer mismatches limit the use of high-throughput DNA sequencing for the quantitative metabarcoding of arthropods. Mol. Ecol. Resour. 15, 819–830. doi: 10.1111/1755-099
Pinto, A. J., and Raskin, L. (2012). PCR biases distort bacterial and archaeal community structure in pyrosequencing datasets. PLoS ONE 7:e43093. doi: 10.1371/journal.pone.0043093
Pinto, R., Patrício, J., Baeta, A., Fath, B. D., Neto, J. M., and Marques, J. C. (2009). Review and evaluation of estuarine biotic indices to assess benthic condition. Ecol. Indic. 9, 1–25. doi: 10.1016/j.ecolind.2008.01.005
Pochon, X., Bott, N. J., Smith, K. F., and Wood, S. A. (2013). Evaluating detection limits of next-generation sequencing for the surveillance and monitoring of international marine pests. PLoS ONE 8:e73935. doi: 10.1371/journal.pone.0073935
Pochon, X., Wood, S. A., Keeley, N. B., Lejzerowicz, F., Esling, P., Drew, J., et al. (2015). Accurate assessment of the impact of salmon farming on benthic sediment enrichment using foraminiferal metabarcoding. Mar. Pollut. Bull. 100, 370–382. doi: 10.1016/j.marpolbul.2015.08.022
Porazinska, D. L., Giblin-Davis, R. M., Faller, L., Farmerie, W., Kanzaki, N., Morris, K., et al. (2009). Evaluating high-throughput sequencing as a method for metagenomic analysis of nematode diversity. Mol. Ecol. Resour. 9, 1439–1450. doi: 10.1111/j.1755-0998.2009.02611.x
Ratnasingham, S., and Hebert, P. D. (2013). A DNA-based registry for all animal species: the barcode index number (BIN) system. PLoS ONE 8:e66213. doi: 10.1371/journal.pone.0066213
Roussel, J.-M., Paillisson, J.-M., Tréguier, A., Petit, E., and Cadotte, M. (2015). The downside of eDNA as a survey tool in water bodies. J. Appl. Ecol. 52, 823–826. doi: 10.1111/1365-2664.12428
Sarri, C., Stamatis, C., Sarafidou, T., Galara, I., Godosopoulos, V., Kolovos, M., et al. (2014). A new set of 16S rRNA universal primers for identification of animal species. Food Control 43, 35–41. doi: 10.1016/j.foodcont.2014.02.036
Schirmer, M., Ijaz, U. Z., D'Amore, R., Hall, N., Sloan, W. T., and Quince, C. (2015). Insight into biases and sequencing errors for amplicon sequencing with the Illumina MiSeq platform. Nucleic Acids Res. 43, e37. doi: 10.1093/nar/gku1341
Schloss, P. D. (2009). Introducing mothur: open-source, platform-independent, community-supported software for describing and comparing microbial communities. Appl. Environ. Microbiol. 75, 7537–7541. doi: 10.1128/AEM.01541-09
Simpson, J. M., McCracken, V. J., Gaskins, H. R., and Mackie, R. I. (2000). Denaturing gradient gel electrophoresis analysis of 16S ribosomal DNA amplicons to monitor changes in fecal bacterial populations of weaning pigs after introduction of lactobacillus reuteri strain MM53. Appl. Environ. Microbiol. 66, 4705–4714. doi: 10.1128/AEM.66.11.4705-4714.2000
Sipos, R., Székely, A. J., Palatinszky, M., Révész, S., Marialigeti, K., and Nikolausz, M. (2007). Effect of primer mismatch, annealing temperature and PCR cycle number on 16S rRNA gene-targetting bacterial community analysis. FEMS Microbiol. Ecol. 60, 341–350. doi: 10.1111/j.1574-6941.2007.00283.x
Stucky, B. J. (2012). SeqTrace: a graphical tool for rapidly processing DNA sequencing chromatograms. J. Biomol. Tech. 23, 90–93. doi: 10.7171/jbt.12-2303-004
Taberlet, P., Coissac, E., Pompanon, F., Brochmann, C., and Willerslev, E. (2012a). Towards next-generation biodiversity assessment using DNA metabarcoding. Mol. Ecol. 21, 2045–2050. doi: 10.1111/j.1365-294X.2012.05470.x
Taberlet, P., Prud'Homme, S. M., Campione, E., Roy, J., Miquel, C., Shehzad, W., et al. (2012b). Soil sampling and isolation of extracellular DNA from large amount of starting material suitable for metabarcoding studies. Mol. Ecol. 21, 1816–1820. doi: 10.1111/j.1365-294X.2011.05317.x
Thomsen, P. F., Kielgast, J., Iversen, L. L., Møller, P. R., Rasmussen, M., and Willerslev, E. (2012). Detection of a diverse marine fish fauna using environmental DNA from seawater samples. PLoS ONE 7:e41732. doi: 10.1371/journal.pone.0041732
Thomsen, P. F., and Willerslev, E. (2015). Environmental DNA – An emerging tool in conservation for monitoring past and present biodiversity. Biol. Conserv. 183, 4–18. doi: 10.1016/j.biocon.2014.11.019
Torti, A., Lever, M. A., and Jørgensen, B. B. (2015). Origin, dynamics, and implications of extracellular DNA pools in marine sediments. Mar. Genomics 24, 185–196. doi: 10.1016/j.margen.2015.08.007
Turner, C. R., Uy, K. L., and Everhart, R. C. (2015). Fish environmental DNA is more concentrated in aquatic sediments than surface water. Biol. Conserv. 183, 93–102. doi: 10.1016/j.biocon.2014.11.017
Valentini, A., Taberlet, P., Miaud, C., Civade, R., Herder, J., Thomsen, P. F., et al. (2016). Next-generation monitoring of aquatic biodiversity using environmental DNA metabarcoding. Mol. Ecol. 25, 929–942. doi: 10.1111/mec.13428
Visco, J. A., Apothéloz-Perret-Gentil, L., Cordonier, A., Esling, P., Pillet, L., and Pawlowski, J. (2015). Environmental monitoring: inferring the diatom index from next-generation sequencing data. Environ. Sci. Technol. 49, 7597–7605. doi: 10.1021/es506158m
Walther, B. A., and Moore, J. L. (2005). The concepts of bias, precision and accuracy, and their use in testing the performance of species richness estimators, with a literature review of estimator performance. Ecography 28, 815–829. doi: 10.1111/j.2005.0906-7590.04112.x
Wang, Q., Garrity, G. M., Tiedje, J. M., and Cole, J. R. (2007). Naive Bayesian classifier for rapid assignment of rRNA sequences into the new bacterial taxonomy. Appl. Environ. Microbiol. 73, 5261–5267. doi: 10.1128/AEM.00062-07
Warton, D. I., Wright, I. J., Falster, D. S., and Westoby, M. (2006). Bivariate line-fitting methods for allometry. Biol. Rev. 81, 259–291. doi: 10.1017/S1464793106007007
Wood, S. A., Smith, K. F., Banks, J. C., Tremblay, L. A., Rhodes, L., Mountfort, D., et al. (2013). Molecular genetic tools for environmental monitoring of New Zealand's aquatic habitats, past, present and the future. New Zeal. J. Mar. Fresh 47, 90–119. doi: 10.1080/00288330.2012.745885
Yu, D. W., Ji, Y., Emerson, B. C., Wang, X., Ye, C., Yang, C., et al. (2012). Biodiversity soup: metabarcoding of arthropods for rapid biodiversity assessment and biomonitoring. Methods Ecol. Evol. 3, 613–623. doi: 10.1111/j.2041-210X.2012.00198.x
Zaiko, A., Samuiloviene, A., Ardura, A., and Garcia-Vazquez, E. (2015). Metabarcoding approach for nonindigenous species surveillance in marine coastal waters. Mar. Pollut. Bull. 100, 53–59. doi: 10.1016/j.marpolbul.2015.09.030
Zepeda Mendoza, M. L., Sicheritz-Pontén, T., and Gilbert, M. T. P. (2015). Environmental genes and genomes: understanding the differences and challenges in the approaches and software for their analyses. Brief. Bioinform. doi: 10.1093/bib/bbv001. [Epub ahead of print].
Zhou, X., Li, Y., Liu, S., Yang, Q., Su, X., Zhou, L., et al. (2013). Ultra-deep sequencing enables high-fidelity recovery of biodiversity for bulk arthropod samples without PCR amplification. Gigascience 2:4. doi: 10.1186/2047-217X-2-4
Keywords: Illumina MiSeq, COI barcodes, extracellular DNA, AMBI, biotic indices, macroinvertebrates
Citation: Aylagas E, Borja A, Irigoien X and Rodriguez-Ezpeleta N (2016) Benchmarking DNA Metabarcoding for Biodiversity-Based Monitoring and Assessment. Front. Mar. Sci. 3:96. doi: 10.3389/fmars.2016.00096
Received: 15 April 2016; Accepted: 30 May 2016;
Published: 10 June 2016.
Edited by:
Michael Elliott, University of Hull, UKReviewed by:
Katherine Dafforn, University of New South Wales, AustraliaJosé Lino Vieira De Oliveira Costa, Centro de Oceanografia, Portugal
Copyright © 2016 Aylagas, Borja, Irigoien and Rodríguez-Ezpeleta. This is an open-access article distributed under the terms of the Creative Commons Attribution License (CC BY). The use, distribution or reproduction in other forums is permitted, provided the original author(s) or licensor are credited and that the original publication in this journal is cited, in accordance with accepted academic practice. No use, distribution or reproduction is permitted which does not comply with these terms.
*Correspondence: Naiara Rodríguez-Ezpeleta, bnJvZHJpZ3VlekBhenRpLmVz