- 1Department of Atmospheric and Oceanic Science, University of Maryland, College Park, MD, United States
- 2National Aeronautics and Space Administration, Goddard Space Flight Center, Greenbelt, MD, United States
- 3European Centre for Medium-Range Weather Forecasts, Reading, United Kingdom
- 4Météo-France, Toulouse, France
- 5Nansen Environmental and Remote Sensing Center, Bergen, Norway
- 6Antarctic Climate and Ecosystems Cooperative Research Centre, Hobart, TAS, Australia
- 7Naval Research Laboratory, Monterey, CA, United States
- 8The University of Texas at Austin, Austin, TX, United States
- 9Naval Research Laboratory, Stennis Space Center, MS, United States
- 10King Abdullah University of Science and Technology, Thuwal, Saudi Arabia
- 11Euro-Mediterranean Center on Climate Change, Lecce, Italy
- 12Met Office, Exeter, United Kingdom
- 13University of California, Santa Cruz, Santa Cruz, CA, United States
- 14Commonwealth Scientific and Industrial Research Organisation, Canberra, ACT, Australia
- 15NATO Centre for Maritime Research and Experimentation, La Spezia, Italy
- 16Department of Atmospheric and Oceanic Science, University of Colorado, Boulder, Boulder, CO, United States
- 17Seoul National University, Seoul, South Korea
- 18Istituto di Scienze Marine, Consiglio Nazionale delle Ricerche, Rome, Italy
- 19JMA Meteorological Research Institute, Tsukuba, Japan
Developments in observing system technologies and ocean data assimilation (DA) are symbiotic. New observation types lead to new DA methods and new DA methods, such as coupled DA, can change the value of existing observations or indicate where new observations can have greater utility for monitoring and prediction. Practitioners of DA are encouraged to make better use of observations that are already available, for example, taking advantage of strongly coupled DA so that ocean observations can be used to improve atmospheric analyses and vice versa. Ocean reanalyses are useful for the analysis of climate as well as the initialization of operational long-range prediction models. There are many remaining challenges for ocean reanalyses due to biases and abrupt changes in the ocean-observing system throughout its history, the presence of biases and drifts in models, and the simplifying assumptions made in DA solution methods. From a governance point of view, more support is needed to bring the ocean-observing and DA communities together. For prediction applications, there is wide agreement that protocols are needed for rapid communication of ocean-observing data on numerical weather prediction (NWP) timescales. There is potential for new observation types to enhance the observing system by supporting prediction on multiple timescales, ranging from the typical timescale of NWP, covering hours to weeks, out to multiple decades. Better communication between DA and observation communities is encouraged in order to allow operational prediction centers the ability to provide guidance for the design of a sustained and adaptive observing network.
Introduction
Sustained high-quality observations are essential for improving our understanding of the ocean and its interactions with the atmosphere and the overall Earth system. An important tool to study the Earth system is the production of historically accurate four-dimensional reconstructions of quantities that characterize the ocean state (such as temperature, salinity, and currents). Mathematical methods from the field of Data Assimilation (DA) allow information provided from observations to be propagated in time and space to unobserved areas using the dynamical and physical constraints imposed by numerical models. When these methods are applied to form the aforementioned historical reconstructions, this procedure is called a retrospective analysis, or “reanalysis” (Kalnay et al., 1996; Dee et al., 2014). In addition to aiding in the study of the ocean itself, such reanalyses can also be used to initialize the ocean component of coupled Earth system models in order to produce long-term forecasts that may provide guidance from a few weeks out to a decade or longer (Meehl et al., 2014; Balmaseda, 2017). Here, we review the current state-of-the-art of DA applied to the ocean and collectively look forward over the next decade to make our own predictions about what kind of complementary in situ and satellite observations will be required to advance reanalysis and prediction, address end-user engagement, identify opportunities for integration, and connect to many of the themes of OceanObs’19.
Methodological Developments in Ocean Data Assimilation
Data assimilation is essentially an automation of the scientific method. A hypothesis is made and encoded in a numerical model. This model is then used to make predictions that can be tested against new observations. Prediction accuracy is then examined and provided as feedback to modify the model and methods, and the process repeats. The development and application of DA serves fundamental Earth science goals such as to: (1) fill gaps between sparse measurements to form a complete picture of the Earth system, (2) utilize the observing network to initialize forecast models, (3) characterize errors in the modeling and observing systems, and (4) identify areas of high uncertainty where observations can illuminate poorly understood phenomena, help target observing campaigns, and improve numerical models and forecasts. Here, we address the current state-of-the-art and limitations of ocean and coupled DA and propose paths forward.
Connecting Ocean Data Assimilation With Ocean Observing Efforts
Although the growing constellation of satellite observing platforms continues to provide a much more coherent view of the ocean surface, there are limitations that remain in the integrated ocean-observing system that prevent the accurate estimation of the full state of the ocean based on observations alone. In situ measurements are quite sparse, while small-scale processes important to air-sea interaction and the deep ocean remain largely unobserved. In order to acquire a complete picture of the ocean state while appropriately characterizing our uncertainty of this picture, the gaps in coverage must be bridged in space and time using rigorous mathematical methods. This is a primary activity of the DA community and requires close collaborations between theorists in academia and practitioners at operational centers.
Ocean DA has become routine practice at many operational prediction centers, both for ocean forecasting and for initializing coupled Earth system models (Edwards et al., 2015; Martin et al., 2015). The regular application of ocean DA either through operational forecasts or using retrospective analyses (reanalyses) is valuable for assessing the completeness and accuracy of the ocean-observing system. A variety of tools are available to assess the value of specific observing platforms, some that follow the methodologies of Observing System Experiments (OSEs) and Observing System Simulation Experiments (OSSEs), or Optimal Experimental Design (OED), while others are linked to the DA cycle itself, such as Forecast Sensitivity to Observation Impacts (FSOI) and estimating the effective degrees of freedom of the observing system (e.g., Oke et al., 2015a,b).
Advances in DA methods have been and will continue to be driven by new observing technologies. We mention two notable features of upcoming observing technologies that deserve attention. First, amongst recent and planned satellite missions are increasingly high-resolution datasets covering the ocean surface. The development of instrumentation such as VIIRS, SLSTR on board Sentinel-3 (A and B), and platforms such as the Surface Water and Ocean Topography (SWOT) mission indicate there will be large volumes of data available for assimilation.
At present, the fidelity of these data products is far higher than many operational ocean models are capable of resolving. Ocean DA faces a challenge due to computational limitations: there is a need to either increase the resolution of ocean models in order to take full advantage of new data sources using conventional DA approaches or design new methods to extract more information from these observations without resorting to high-resolution modeling (e.g., by using machine learning methods applied to high-resolution observations to produce dynamic parameterizations at the subgrid-scale – see for example Bolton and Zanna, 2019). The accurate specification of observation error correlations becomes more important as higher resolutions are used (e.g., Mazloff et al., 2018), making it more difficult to accurately assimilate new higher resolution observations.
Amongst in situ observing systems, there is a trend toward mobile and adaptive platforms and new DA methods will be needed to use the full breadth of information provided by these platforms. As technology improves, there is also an opportunity to explore potential feedback between operational ocean DA systems and observing system guidance in near real-time that redirects the observing system to increase sampling in areas where the forecasts have greatest sensitivity. Ocean-observing technologies in the form of gliders, autonomous underwater vehicles, high-frequency radars, profiling floats, drifters, tagged marine mammals (and other pelagic apex predators), and acoustic instruments continue to undergo rapid development, and data volumes from these platforms are growing rapidly, particularly in coastal regions. Quality assurance and quality control (QA/QC) protocols are necessary, especially for new types of observations. Operational centers should improve their capability to provide feedback in near real-time regarding the QC classifications of individual observations based on forecasts made using those observations.
From a fundamental standpoint, most of the approaches used for characterizing uncertainty in Ocean DA methods are predicated on the principles of Bayes’ theorem (Hoteit et al., 2018). A common assumption is that errors are Gaussian-distributed and that the time evolution of the errors is linear. As such, there are common limitations to all currently used DA methods and a primary goal for improving the accuracy and applicability of DA in the coming decade will be to relax these limiting constraints (see Martin et al., 2019; Moore et al., 2019, this issue). This has relevance to future ocean-observing system design, as it may change requirements on the observing system either to test and design new methods or to take advantage of new capabilities afforded by the methods.
In recent years, the Global Ocean Data Assimilation Experiment (GODAE) and its offshoot, GODAE OceanView (GOV) have been active in galvanizing ocean DA activities by providing a platform for promoting ocean DA and forging international collaborations (Bell et al., 2015). These activities will continue under the new guise of OceanPredict. Going forward, we recommend that this activity expand to further interface with the academic and operational ocean-observing, ocean modeling, and ocean DA communities.
The advent of coupled data assimilation
The components of the Earth system have traditionally been analyzed independently. However, modeling improvements and increases in computing power are now enabling the analysis of the Earth system as a whole (Saha et al., 2010, 2014; Lea et al., 2015). Observation-model synthesis activities that incorporate observational data into coupled Earth system models have led to the emergence of a new research area called Coupled Data Assimilation (CDA; Penny et al., 2017). While traditional methods have generally focused on a single scale of motion within any given DA system, an essential characteristic of CDA is the need to account for the multiple spatiotemporal scales present in the error dynamics of the coupled system. The most basic application of DA to coupled models has been the application of legacy DA systems to each component separately, which is called weakly coupled data assimilation (WCDA). In order to allow any observation to directly affect the analysis of multiple model components across their interface, the DA itself must also be coupled; this is called strongly coupled data assimilation (SCDA). For most modern DA methods, SCDA requires that the forecast error covariance matrix be produced for the coupled state. Efforts are underway to develop effective approaches for SCDA, though additional work is still needed to understand the complexities of this problem (Penny et al., 2019).
By isolating systematic errors in prediction systems, CDA may help identify new transformative directions in ocean-observing strategies targeted at eliminating these errors. Because CDA allows ocean observations to directly inform atmospheric state estimates and vice versa (Sluka et al., 2016; Sluka, 2018), the relevance of existing observations for state estimation and prediction must be clarified as the ocean-observing network evolves. CDA developments involve a necessary reevaluation of requirements for ocean-observing capabilities, either by reducing the presence of redundant information or by using such redundant information to calibrate multiple observing platforms. CDA can effectively leverage multidisciplinary, sustained, collocated observations, and may require more information in new geographic locations, or of new previously unmeasured quantities, to better understand the structure of the cross-domain error covariance. Over the next decade, those designing components of the Earth-observing system should pay close attention to developments in CDA.
Operational centers are now developing CDA methods for NWP and reanalysis applications that include components such as the ocean, sea ice, land, and atmosphere (Brassington et al., 2015). One of the original motivations for improving CDA methods was to ensure consistency between the different components of the Earth system. The use of coupled Earth system models for operational prediction provides the potential to produce forecasts that target multiple prediction timescales. At NWP timescales, the diurnal cycle has a large influence on coupled processes in the boundary layers of the atmosphere and ocean. Mesoscale interactions between sea surface temperature (SST) fronts and near-surface winds (Chelton and Xie, 2010) may have significance to winds throughout the troposphere. Potential sources of predictability for Subseasonal-to-Seasonal (S2S) timescales include establishing teleconnections associated with the Madden–Julian oscillation (MJO), the evolution of the El-Niño Southern Oscillation (ENSO), soil moisture, snow cover and sea ice, stratosphere–troposphere interactions, upper ocean conditions, and tropical-extratropical teleconnections (Vitart et al., 2015). At decadal prediction timescales, accounting for coupled oscillations such as the Atlantic Multidecadal Oscillation (AMO) and the Pacific Decadal Oscillation (PDO) (d’Orgeville and Peltier, 2007) may be of greater importance for CDA.
Beyond coupled atmosphere-ocean interactions, the application of CDA is also important to better understand other coupled processes in more detail. For example, DA in coupled ocean-sea ice models (Fenty and Heimbach, 2013; Bertino and Holland, 2017; Kimmritz et al., 2018) and coupled physical-biogeochemical models (Brasseur et al., 2009; Song et al., 2016; Verdy and Mazloff, 2017) at both regional and global scales are currently active areas of research, driven by improvements in remote-sensing observing platforms (e.g., sea ice concentration and thickness and ocean color) or new capabilities (e.g., biogeochemical Argo floats and airborne hyperspectral imagers). There have been few studies to date exploring DA applied to coupled land-ocean processes.
Focus on biological activity highlights the importance of physical variables often ignored in conventional ocean DA, such as upper-ocean vertical fluxes (Brasseur et al., 2009). Large-scale assimilation of marine biogeochemistry is limited by the lack of regular observations. The only routine observations with global coverage are satellite ocean color (Ford and Barciela, 2017). Existing DA efforts typically focus on generating products based purely on biogeochemical measurements independently of physical oceanographic measurements (e.g., Ciavatta et al., 2016; Gregg et al., 2017). As CDA begins to mature, it would be highly beneficial for the physical oceanographic reanalysis and ocean biogeochemical reanalysis efforts to start integrating with one another (Rosso et al., 2017). Early interest in moving in this direction has been indicated, for example, by Perruche et al. (2017) as part of the ERA-CLIM2 project. Regional ocean analyses are being used to predict Harmful Algal Blooms (HABs; Anderson et al., 2016), to understand economically important marine ecosystems (e.g., Schroeder et al., 2014, 2017) with a view to management, and to understand the migration habits of endangered marine species (e.g., Becker et al., 2016), and it is expected that these applications will be enhanced with CDA.
To date, ECMWF has one of the more mature efforts developing a CDA system. An implicit coupling approach has been implemented in their CERA system, where the atmospheric 4D-Var and oceanic 3D-Var DA systems are synchronized using multiple outer iterations in the incremental variational formulation. This outer-loop coupling system is an approximation of a fully coupled 4D-Var system that tries to find an approximation to the same optimal solution by setting the coupled adjoint model and the cross-domain error covariance at the initial time of the assimilation window to zero (Laloyaux et al., 2018b). It takes between 6 and 12 h for the outer loop coupling to synchronize the coupled increments (Laloyaux et al., 2018a). This finding suggests that a long assimilation window (at least 12 h) is necessary for CERA to be an effective strategy for CDA. The outer-loop coupling employed by the CERA system could in principle be augmented by both the specification of the initial time coupled covariances and coupled adjoint. Such an approach could mitigate problems in cases where the coupled model is not able to synchronize the unbalanced increments that arise because the assimilation window is too short, the observations are inconsistent due to biases present in the observing platforms, or systematic modeling errors prevent agreement across the interface.
An Example Application of Cda: the Direct Assimilation of Satellite Radiances for Estimating Sst
The air-sea interface is one of the prime focus areas for early explorations of CDA. In addition to requiring a rethinking of DA algorithms and solution approaches, CDA affords the opportunity to improve the methods used to map the modeled state to a simulated “model equivalent” for each observation that can then be compared directly with observations. One of the most obvious places to start is improving the inputs provided to radiative transfer models. CDA provides a new capability to assimilate observed brightness temperature (BT) instead of relying on retrieval products such as proxy measurements for SST.
Current state-of-the-art coupled forecasting systems do not analyze interface states such as SST, sea surface salinity (SSS), or sea ice in a self-consistent manner. For example, many atmospheric and oceanic DA systems typically nudge toward SST retrieval products. However, this approach typically ignores caveats in the empirical methods used to convert satellite-measured radiances into SST retrieval data products (Donlon et al., 2007). Among the most serious are errors in model calibration at high latitudes as well as challenges in using skin SST estimates to constrain bulk temperature (Donlon et al., 2002). Diurnal variations of SST and near-surface cooling in the microlayer are processes that are well observed and studied (Kawai and Wada, 2007) but not very well represented in coupled atmosphere- ocean general circulation models (Brunke et al., 2008), in which reproducing SST variability remains a challenge (Lea et al., 2015).
There are numerous definitions for SST; for example, see Figure 1 of Donlon et al. (2007) or definitions established by the Group for High Resolution Sea Surface Temperature (GHRSST). Some of these definitions are conceptual (e.g., the interface SST) while others are derived from the method of measurement (e.g., infrared vs. microwave). Satellites have provided continuous infrared observations that sample in the upper 10–20 μm (the skin temperature) since the early 1980s and microwave observations (spatially less accurate than infrared, but insensitive to cloud cover and aerosols) that observe the upper few millimeters (the subskin temperature) since the late-1990s (Reynolds et al., 2007). In situ measurements of SST are sparser and typically comprised of top-level (1–2 m) moored buoys, drifting buoys (about 20–30 cm), and ship intake measurements (Castro et al., 2012; Legler et al., 2015) that are known to have large errors (Folland and Parker, 1995; Kennedy, 2014).
Satellite-based measurements of SST are inherently coupled due to influences from not only the sea surface but also the full atmospheric column above it. The measured SST is highly influenced by both atmosphere and ocean boundary layers as well as the strength of upward longwave radiation and turbulent heat flux exchanges. To avoid dealing with the complex calibration issues associated with satellite radiances, current prototype CDA systems typically rely on SST data products produced by specialists and assimilate either along-track (L2) SST estimates or gridded (L3 or L4) SST products such as Pathfinder (Casey et al., 2010), OSTIA (Stark et al., 2007), or ACSPO (Ignatov et al., 2016). See Martin et al. (2012) for a review of available L3 and L4 SST products. One of the main recommendations of a recent ECMWF workshop (Balmaseda et al., 2018) was to directly assimilate satellite radiances to constrain SST and sea ice, just as is done in NWP for atmospheric quantities. CDA offers an opportunity to treat the interfaces within the coupled model in a self-consistent manner, particularly when the forward model that is used to evaluate the “model equivalent” to the observation, H(x), depends on state information from multiple domains.
Both the NASA Global Modeling and Assimilation Office (GMAO) (Akella et al., 2017) and the National Oceanographic and Atmospheric Administration (NOAA) National Centers for Environmental Prediction (NCEP) Environmental Modeling Center (EMC) (Derber and Li, 2018) have already implemented methods to directly assimilate radiances in order to compute SST analyses. The NASA GMAO procedure followed Takaya et al. (2010) allows the SST diurnal cycle to be resolved in the model, which provides a near-surface temperature profile as a function of depth. Using the forecasted SST along with the forecasted atmospheric state as inputs to the radiative transfer model, the resulting forecast BT can be compared with observed BT. The difference between observed and forecasted BT is used by the DA method to form a consistent analysis of the combined atmospheric state and SST. In order to effectively constrain SST, observations that are sensitive to SST, such as infrared satellite radiance measurements onboard operational polar orbiting satellites, were added to the observing system (see Akella et al., 2017 for details and Gentemann and Akella, 2018 for a comparison/evaluation of their results with other diurnal-SST retrievals). The capability to assimilate satellite radiances in coupled forecasting systems has improved the predictability of the GMAO system, most notably near the surface. The BTs are atmospheric column-weighted measurements. Because infrared satellite measurements are sensitive to water vapor, improved resolution and assimilation of SST-sensitive BTs translated into improved observational innovation statistics for many satellite channels that contain information about tropospheric temperature and water vapor.
The advantages of combining infrared and microwave radiometric measurements of SST are already well established (Chelton and Wentz, 2005). A microwave satellite radiometer beyond the currently operational Global Precipitation Measurement – GPM Microwave Imager (Skofronick-Jackson et al., 2018) and Advanced Microwave Scanning Radiometer-2 (Kazumori et al., 2016) missions would provide the ability to maintain and further improve CDA at the air-sea interface. There is an immediate need to plan for a satellite salinity measurement mission beyond the 2020–2025 time frame (Durack et al., 2016; Vinogradova et al., 2017 this issue). Bearing in mind the collaborative nature of satellite missions, further coordination is needed for planning the next generation of NOAA satellites that follow the GOES-R, JPSS, DSCOVR, Jason-3, and COSMIC-2 missions (Volz et al., 2016).
Field campaigns and in situ measurements aid in the improvement of modeled near-surface temperature and salinity variations, and mixing processes. The existing network of drifting buoys [Figure 3 of Legler et al. (2015)] routinely reports near-surface (about 20 cm) measurements of SST, sea level pressure (SLP). The measured SLP is routinely assimilated into the NWP forecast models, and SST are used for calibration/validation of SST retrieval products. However, one cannot measure vertical SST variability and mixing with a single sensor (e.g., at 20 cm). Dedicated cruise campaigns such as those reported by Dong et al. (2017) suggest that adding one more temperature sensor and salinity sensor to the drifting buoy network can provide valuable measurements of SST/SSS near-surface variations. Such measurements would help with calibration and evaluation of observations as well as improve the representation of the diurnal cycle, the feedbacks between SST and surface salinity variations (Bellenger et al., 2017), and buoyancy-driven density variations in general.
Ocean and Coupled Earth System Reanalysis
An important application for DA is to develop historical reconstructions of the Earth system based on the observational record. Numerical models fulfill the basic large-scale equations of motion and satisfy conservation laws, but may have systematic errors. While this type of numerical modeling can provide insights into the mechanisms driving long-term variability (Haid et al., 2017), the systematic errors that arise can cause long-term drift in the modeled climate compared to the real Earth system. In contrast, statistical observational analyses (e.g., Abraham et al., 2013) can be applied to observed data to produce a full field reconstruction that closely agrees with the observational record. However, this approach does not typically ensure conservation laws are enforced, meaning there are known errors that are unaccounted for, and is not able to recover unobserved quantities. Retrospective analyses, or reanalyses, combine the advantages of both numerical modeling and statistical observation analyses to fulfill the conservation laws over discrete periods while also incorporating observed data and subsequently estimating unobserved quantities. Reanalyses can be used to study the evolution of the Earth’s climate during any time period for which we have an observational record. They are also useful for initializing “reforecasts” that can be used to calibrate bias-correction schemes for seasonal forecasts. Next, we document recent advances from the ocean reanalysis community and discuss unresolved challenges that require sustained activities for maximizing the utility of information content from observations, supporting data rescue, and advancing specific research and development requirements for reanalyses.
Advances and Unsolved Challenges in Producing Ocean Reanalyses
The original interest in developing ocean reanalyses arose largely from a desire to examine long-range climate-scale signals. Ocean reanalyses can be studied to enhance understanding of processes driving observed changes. They are also useful for studying recent changes in the climate for quantities that are difficult to observe continuously, such as transports (Mignac et al., 2018), or those that require consistent spatial data coverage at depth, such as ocean heat content (Balmaseda et al., 2013; Wunsch and Heimbach, 2014). To be able to draw robust conclusions, one must be confident that inhomogeneous time series or abrupt regime changes are caused by physically consistent processes – not artifacts associated with changes in the historical observing network. During much of the early history of ocean reanalysis development, there have been significant disagreements between estimates produced by different reanalysis approaches. This was due in large part to the scarcity of observational data, differences in model configurations, and discrepancies in DA methods.
However, due to advances in the ocean observing system, improvements in modeling, and advances in DA methods, ocean reanalysis products have been slowly converging.
To date, ocean reanalyses have been produced by many operational centers and research institutes (Carton and Giese, 2008; Sugiura et al., 2008; Xue et al., 2011; Chang et al., 2013; Wunsch and Heimbach, 2013; Blockley et al., 2014; Valdivieso et al., 2014; Köhl, 2015; Forget et al., 2015; Penny et al., 2015; Toyoda et al., 2016; Storto and Masina, 2016; Palmer et al., 2017; Zuo et al., 2017b). Balmaseda et al. (2015) provide a recent intercomparison study of about 20 reanalysis products. The extent to which reanalyses provide robust answers to questions about climate change and variability relies on many factors, including the fidelity of the numerical models, the accuracy of forcing fields, biases in observing platforms, uncertainties attributed to the observations and the background state (priors), and the sophistication of the DA schemes. Many of these considerations are highlighted in a recent study by Carton et al. (2019) comparing leading ocean reanalysis products (SODA3, ECCO4r3, and ORAS5).
Given the availability of ocean reanalysis products from multiple groups worldwide, we recommend that climate studies include the evaluation of as many products as possible to sample the range of uncertainty in the historical ocean state and disentangle possible inconsistencies that arise due to choices made in their construction. Uncertainties in ocean reanalysis state estimates result from accumulated errors from all system components (ocean model, boundary condition forcing, observations, and DA method). Uncertainty in ocean reanalyses as a whole can be studied using a multi-reanalysis ensemble approach (Balmaseda et al., 2015; Masina et al., 2017; Xue et al., 2017), which provides a way to not only investigate the accuracy of ocean reanalyses but also disentangle sources of uncertainty. A rough estimate can be achieved by comparing the consistency of the reanalyses (ensemble spread), interpreted as noise, with the natural variability (variance in time), interpreted as the signal.
Uncertainties in an individual system can also be assessed by accounting for errors explicitly in different system components, for example by using ensemble forecasts, by introducing stochastic perturbations in the model (Brankart et al., 2015), by estimating representativeness errors associated with observations in relation to the model resolution, and by estimating analysis/structure errors in forcing fields (Penny et al., 2015; Zuo et al., 2017a).
Surface forcing derived from atmospheric reanalyses induces systematic errors. Multi-forcing reanalyses may be performed to better estimate the impacts of these errors (Chaudhuri et al., 2013, 2016; Storto et al., 2016b; Carton et al., 2018; Yang et al., 2018). Recently, Zuo et al. (2017a) introduced a stochastic perturbation for the atmospheric forcing by taking into account both uncertainty from different atmospheric analysis data sets and uncertainty from the same analysis method with multiple ensemble members. Another method for adjusting uncertain atmospheric fields is by employing control methods, where adjustments to atmospheric surface forcing data are part of a formal inversion, assuming relatively accurate oceanic observations (e.g., Stammer et al., 2004; Liang and Yu, 2016). Uncertainty in initial conditions can also be evaluated using an ensemble approach, by performing several spin-up integrations with different DA system configurations (Zuo et al., 2018). Chevallier et al. (2017) showed that for coupled ocean-sea ice models driven by prescribed atmospheric forcing, part of the variability across ocean reanalyses is the result of differences in the atmospheric reanalyses used to force these systems, which is large in the polar regions (Lindsay et al., 2014). Part of the discrepancy in the atmospheric reanalyses is due to the treatment of the prescribed boundary conditions (e.g., sea ice), giving an example of a weaknesses in the “uncoupled” approach. Generally, both coupled climate models and ocean-ice models, driven by prescribed atmospheric forcing, cannot adequately represent the observed polar trends, whereas ocean reanalyses have proven quite adequate to capture these trends when observations are available to constrain the system (Chevallier et al., 2017; Uotila et al., 2018).
With the exception of smoother-based reanalyses generated by the Consortium for Estimating the Circulation and climate of the Ocean (ECCO; Wunsch and Heimbach, 2013; Köhl, 2015; Forget et al., 2015; Heimbach et al., 2019), most of the DA systems developed under GODAE and GODAE OceanView use some form of sequential DA (Martin et al., 2015). Some of the systems based on simplified assumptions about the forecast error characteristics suffer from problems with initialization, where the updates applied to the model at each assimilation step are not dynamically consistent. To date, many developers have attempted to minimize the negative impacts of these dynamical imbalances by ad hoc techniques such as nudging with incremental updates (Bloom et al., 1996). Some problems have been identified in the Equatorial region within a number of ocean reanalyses, in which the assimilation can induce spurious variability that has been damped by following several bias correction strategies (Waters et al., 2017).
An application of the 4D variational method in ocean DA has been developed with an emphasis on reconstructing the ocean on climate time scales (Stammer et al., 2016). Motivating these approaches were the goals of (i) using information contained in observations backward in time, (ii) enlarging the control space to include uncertain boundary conditions and model parameters, and (iii) deriving estimates with closed property budgets enforced by the equations of motion (e.g., Buckley et al., 2015; Piecuch et al., 2017). However, this approach also has potential limitations. For instance, increasing the control space also increases the dimension of the problem, which in turns makes the method very expensive for high-resolution global applications. There may also be challenges with relying on the accuracy of a linearization over long time windows.
Other difficulties are connected with the irregular observing network. This often causes spurious variability in reanalysis products, especially in multi-decadal reanalyses covering historical periods with highly varying observing systems ranging from the sparse pre-satellite era to present. This has been the subject of many investigations aiming to include bias-correction schemes within the reanalysis (Balmaseda et al., 2007; Lea et al., 2008; Storto et al., 2016a). However, this creates the additional challenge of estimating these biases while having only a limited number of “anchoring” (i.e., unbiased) observations. Before the deployment of the Argo network, the sampling of observations used by ocean reanalyses is generally sparse, which has implications for the reliability of quantities such as global ocean heat content before the 2000s. However, several studies showed that beginning in the early 1980s, the observing system is able to reasonably constrain the global ocean heat content (Storto et al., 2016b). There is growing interest amongst the ocean reanalysis community in the deep Argo program (Zilberman, 2017), with the hope that this will gradually fill the gap in knowledge of the ocean state below 2000 m and allow the deep ocean warming contribution to be assessed with greater precision. Care is also being taken in ocean reanalyses to synergistically exploit a large number of data sources (altimetry, gravimetry, Argo, tide-gauges, etc.) to create a reliable representation of freshwater and mass balances. Data used for evaluation, not necessarily assimilated (e.g., buoys, drifters, tide-gauges, RAPID and OSNAP arrays, SAMOC and SOCCOM programs, ADCP data, etc.) are also crucial for assessing uncertainty in reanalyses and improving process representation in models.
Within historical data records, the accuracy of the observations assimilated is often unknown or underestimated due to lack of metadata. This also prevents effective bias-correction procedures from being implemented and may lead to the erroneous specification of instrumental errors. For the historical ocean subsurface temperature record, the situation is improving through an internationally coordinated community effort (Domingues and Palmer, 2015)1, focusing on recovery of data and metadata, development of intelligent metadata, coordinated quality control (automated and expert), and assignment of uncertainties. Their overall goal is to produce a long-term climate quality global ocean subsurface database that can be used with greater confidence by the ocean reanalysis community and other users. The first interim IQuOD database product is available from The IQuOD Team (2018).
Reanalyses will continue to extend further backward in time to cover longer historical periods, following the trend set by Compo et al. (2011) and ECMWF’s ERA-20C, and later followed by comparable century-long ocean reanalyses (Giese et al., 2016; Yang et al., 2017) and the coupled reanalysis CERA-20C (Laloyaux et al., 2018a). This will require improved methods to handle sparse observations, discontinuities in the observation network, and correction of large-scale biases, as well as continuous efforts on data rescue. With the recent emergence of coupled Earth system reanalyses, non- oceanic data will also play an important role, particularly in time periods where ocean observations are extremely sparse or non-existent. Ocean background errors are expected to evolve significantly during the reanalysis period due to the ever-changing observing network. The development of time dependent background error covariance estimates has proved beneficial (Penny et al., 2015; Penny, 2017; Yang et al., 2017). The full introduction of flow-dependent background errors involves estimating the ocean background error covariances from the ensemble and developing methods to deal with sampling limitations. Such ensemble-based error covariance information can account for anisotropic and inhomogeneous correlations that are difficult to estimate with traditional methods. An Ensemble of Data Assimilation systems (EDA) showed some benefits in the atmosphere by dynamically changing the weight given to the background depending on the observation density (Poli et al., 2013) and such methods may be useful for the ocean as well.
In addition to climate investigations, ocean reanalyses using higher resolution eddy-permitting models have a long history among the members of the Global Ocean Data Assimilation Experiment (GODAE) and the follow-on GODAE OceanView. The production of high-resolution ocean reanalyses started naturally as a historical extension of operational analysis experiments, with a series of products disseminated by Mercator Ocean (Ferry et al., 2007, 2010; Garric et al., 2018), by CSIRO and the Bureau of Meteorology (Bluelink) (Oke et al., 2005, 2008, 2013), by NERSC (Sakov et al., 2012), and by JMA and JAMSTEC (Usui et al., 2017). These products have proved instructive for global and regional investigations of ocean variability (Schiller and Oke, 2015; Feng et al., 2016), ocean processes (Oke and Griffin, 2011), and for studies of the ocean-observing system (Lea et al., 2013; Fujii et al., 2015). Going forward, it is expected that the resolution of ocean reanalyses will increase to allow representation of eddy dynamics and to fully include mesoscale and coastal ocean dynamics. This requires the improvement of small-scale ocean dynamics in models and the development of DA methods that are capable of assimilating rapidly changing, strongly non-linear, and non-Gaussian observational constraints.
Advances and Unsolved Challenges in Producing Coupled Reanalyses
Coupled model integrations with prescribed radiative forcing have been the backbone of the coordinated experiments for the World Climate Research Programme (WCRP) Coupled Model Intercomparison Project (CMIP) that were designed for contributing to the Intergovernmental Panel on Climate Change (IPCC). Century-long coupled reanalyses go a step further by assimilating information about the actual observed state of the Earth system, without deteriorating the model representation of low-frequency variability and change. While this is a tremendous challenge, it is essential in order to advance our understanding of climate variability and change and to identify the broader impacts on global communities.
Key benefits expected from a coupled reanalysis are: a more consistent treatment of the interfaces between different model components, better use of observations near these interfaces, and improved representation of global budgets of conserved quantities. In principle, the use of a coupled model as the forecast component within a DA system makes it possible to fully account for ocean-atmosphere, ocean- ice-atmosphere, and land-atmosphere feedbacks. This can only be achieved, however, if the assimilation of near-surface observations respects the consistency at the boundaries as imposed by the model and if the modeled dynamics at the boundary are consistent with observations.
In the 20th-century coupled reanalysis (CERA-20C) produced at ECMWF, the ocean and the atmosphere communicate hourly through air-sea coupling at the outer-loop level of the variational method. In this system, changes in the state of the atmosphere indirectly impact the ocean properties, and vice-versa, and both systems adjust to each other during each analysis cycle. There is a more consistent energy balance in CERA-20C, with the net heat fluxes at the air-sea interface (0.15 ± 1.1 W/m2) and ocean temperature increments (−0.11 ± 1.9 W/m2) averaging close to zero over the century, compared to the forced ocean reanalysis ORA-20C (−1.62 ± 1.89 W/m2 and 1.66 ± 2.32 W/m2). However, given that the SST in the ocean component of CERA-20C was nudged toward an external data product, this suggests that there is further room for improvement. While midlatitude storms, heat waves, or cold-air outbreaks are often well-represented in regions with dense observational coverage, this is not always the case for tropical cyclones, which are difficult to model and not well-constrained by observations. CERA-20C struggles to correctly represent several tropical cyclones at the beginning of the 20th century (Laloyaux et al., 2018a). More work is needed to quality control observations from the International Best Track Archive for Climate Stewardship (IBTrACS). This is expected to improve the ability of historical reanalyses to facilitate the study of weather extremes. Based on the development of CERA at ECMWF, which implements the Copernicus Climate Change Service2 on behalf of the European Union, there are ambitions to produce a moderate-resolution global coupled centennial reanalysis by 2022, allowing a better representation of long-term trends in the climate system.
Beyond CERA-20C, ECMWF’s reanalysis portfolio has recently been extended to include CERA-SAT (Schepers et al., 2018), a pilot reanalysis for coupled DA using the full modern atmospheric and ocean-observing systems. CERA-SAT was produced using ECMWF’s CERA coupled assimilation system and constitutes a 10-member EDA available for a 9-year period from January 1, 2008 to December 31, 2016. CERA-SAT serves as a proof-of-concept for CDA in the context of modern NWP-observing systems. Preliminary assessments have shown ocean-atmosphere coupling to be beneficial in tropical regions, while degradation is evident in the extra tropics, when comparing the coupled CERA-SAT system using SST nudged to OSTIA to an atmosphere-only reanalysis of the same setup but forced with OSTIA SST.
Centers that routinely produce reanalyses are often also engaged in other activities (for instance, operational prediction, mission support, and ocean monitoring). In order to carry out all of these missions, and to successfully transition the currently in-production uncoupled reanalyses to future coupled reanalyses requires careful planning for appropriate computational and storage resources. We highly recommend that funding agencies plan for such upcoming future needs in order to dedicate sufficient resources to support, within the next decade, not only coupled ocean-atmosphere reanalyses but also the inclusion of additional components, such as atmospheric constituents, chemistry, and ocean biogeochemistry. Such efforts are underway in the United States as detailed in NOAA’s strategic implementation plan (SIPv4, 2017), which is a partnership among NASA, NOAA, the Department of Defense (DoD), and the Joint Center for Satellite Data Assimilation (JCSDA) and contributing external and international agencies.
Using Ocean Observations to Improve Prediction
We next describe the existing observing system and gaps in observational coverage and recommend designs of observational and modeling experiments to evaluate the impact of ocean observations on forecast skill. The advances and enhanced spatial and temporal resolution obtained over the last 10 years in both satellite and in situ observations have enabled the use of DA to constrain coupled Earth system models for the first time to a realistic representation of the large-scale upper ocean thermal structure (upper 1000 m). However, there are still components of the coupled system that remain unconstrained. For example, the lack of air-sea flux measurements with global coverage poses a challenge to constraining the atmosphere-ocean exchanges without adequate observational sampling. This type of observing network should be enhanced in the future as they are not only crucial in the context of CDA and its applications to S2S and decadal prediction but also for the evaluation of climate simulations. In particular, we recommend the development of air-sea-flux-observing satellite missions.
We emphasize the need for continuous long observational records to enhance prediction capabilities. Ocean reanalysis systems naturally extend to the initialization of seasonal, interannual, and decadal prediction systems, where the role of subsurface ocean initialization has been recognized as crucial (Balmaseda et al., 2009). S2S and decadal forecasting typically rely on the existence of reforecasts covering several decades in order to calibrate the model output and for skill assessment. These reforecasts are initialized by ocean or coupled reanalyses (Balmaseda, 2017). The length of the reforecast record adds value to the forecast. For this purpose, sustained data rescue activities are recommended as well as maintaining stability of the existing observing system. Recently, ocean reanalyses used to initialize seasonal prediction systems (reforecasts and near real-time reanalyses) have become publicly available via the EU-funded Copernicus Programme and are being used to evaluate subsequent forecasts (Juricke et al., 2018).
Measurements from observing platforms such as satellites, moored surface and subsurface buoys, drifters, floats, dedicated manned and unmanned vehicles, research ships, and vessels of opportunity are collected and distributed with various time lags. Operational predictions rely on observational platforms equipped with the capability for distribution in real-time or near real-time. Some observation types are used primarily as independent measurements for evaluation because they cannot be assimilated due to time delays or other technical complications. These include ocean current profilers, satellite-derived ocean surface currents, and a suite of biogeochemical observations such as carbon, oxygen, nutrients, ocean color, and phytoplankton.
Overall, a lack of uniformity in data management infrastructures imposes problems for the effective and efficient use of the global observing system in prediction efforts. These issues include, but are not limited to, delayed and duplicate data receipts, versioning issues, missing data and metadata, and non-documented data processing procedures. In order to advance the deployment of effective ocean-observing systems, modern data management infrastructures are needed such that all activities along the data flow pipeline, from data collection through assembly and preservation, are more automated and fault-tolerant and progressively advance the systems toward interoperability. Building strong collaboration amongst the observing networks, data managers, and decadal forecasting centers will lead to improved access and uptake of data and to efficiencies that will eventually lead to improvements both in the observing networks and the decadal prediction system.
The future ocean observational requirements for the decadal prediction system include sustained and reliable data streams that have global sampling and are continuous in time, subject to regular quality control and calibration procedures, and encompass several spatial and temporal scales (e.g., National Academies of Sciences, Engineering, and Medicine [NASEM], 2017). To this end, there is great value to centralized data centers that collate observations from individual observing platforms in order to provide timely access to data and a consistent data format for ease of integration into DA systems.
Prediction at Subseasonal to Seasonal Timescales
Many operational prediction centers are currently undertaking a transition from atmospheric NWP on a time range of 0–2 weeks to seamless forecasts that bridge the gap between medium-range weather and seasonal forecasts. This transition is driven by a growing consensus that coupled Earth system modeling benefits forecasts on a wide range of timescales (Hoskins, 2013; Vitart et al., 2017). The new focus on prediction with coupled models is highlighted in efforts such as forecasting the onset of monsoons, characterizing teleconnections of the MJO, and providing advance warning for extreme weather events (Vitart and Robertson, 2018).
Subseasonal prediction, focusing on the period transitioning from NWP to seasonal timescales, stands to gain considerably from combining the higher model resolutions of NWP with the coupled modeling approach of seasonal prediction. The MJO is the dominant mode of intraseasonal variability in the tropics and is considered a major source of predictability on the subseasonal time scale (Waliser, 2011). With respect to the ocean, anomalies in SST affect air-sea heat fluxes and affect atmospheric circulation (Woolnough et al., 2007).
Vitart et al. (2014) indicated significant gains in prediction skill after a decade of producing operational forecasts at ECMWF, pointing to an average gain of about 1 day of MJO prediction skill per year and improved ability to predict the North Atlantic Oscillation (NAO) and sudden stratospheric warmings (SSW). Skill scores improve with increased horizontal resolution and the addition of new modeling components such as a dynamic sea ice model. The introduction of new modeling components also presents the opportunity to assimilate new observational data not previously utilized for sub-seasonal prediction. Zampieri et al. (2018) indicate high potential for sea ice prediction in the sub-seasonal timescales, especially for late summer forecasts, and advocate the need to reduce systematic seasonally dependent model biases and develop advanced DA capabilities to constrain sea ice extent and sea ice thickness.
Zhu et al. (2018) showed that MJO forecast skill can be improved in the NCEP Global Ensemble Forecast System (GEFS) from an average of 12.5 days (control) to nearly 22 days by (1) adding stochastic physical perturbations, (2) considering ocean impacts by using a two-tiered sea surface temperature approach (combing an analysis product with a forecast of SST from a coupled model), and (3) applying a new scale-aware convection scheme to improve the model physics for tropical convection. They also showed improved ensemble mean anomaly correlation of 500-hPa geopotential height in the extratropics over weeks 3 and 4.
El-Niño Southern Oscillation is an inherently coupled phenomenon and one of the most studied sources of interannual variability in the climate system (Wu et al., 2009). Though mostly associated with the tropical Pacific, ENSO variability impacts the global climate (Timmermann et al., 2018). Changes in SST are an indicator of changes in ocean heat storage and transport and these oceanic processes further interact with changing atmospheric momentum and heat fluxes. Prediction skill for the SSTs associated with ENSO have improved over time. At ECMWF, for example, the skill in predicting SST anomalies in the NINO3.4 region has consistently improved as the DA system evolved starting from the S1 system in 1997. If subsurface ocean and satellite altimeter observations are withheld from the analysis, there is a severe degradation in skill comparable to 15 years of progress in seasonal forecasting (Figure 1).
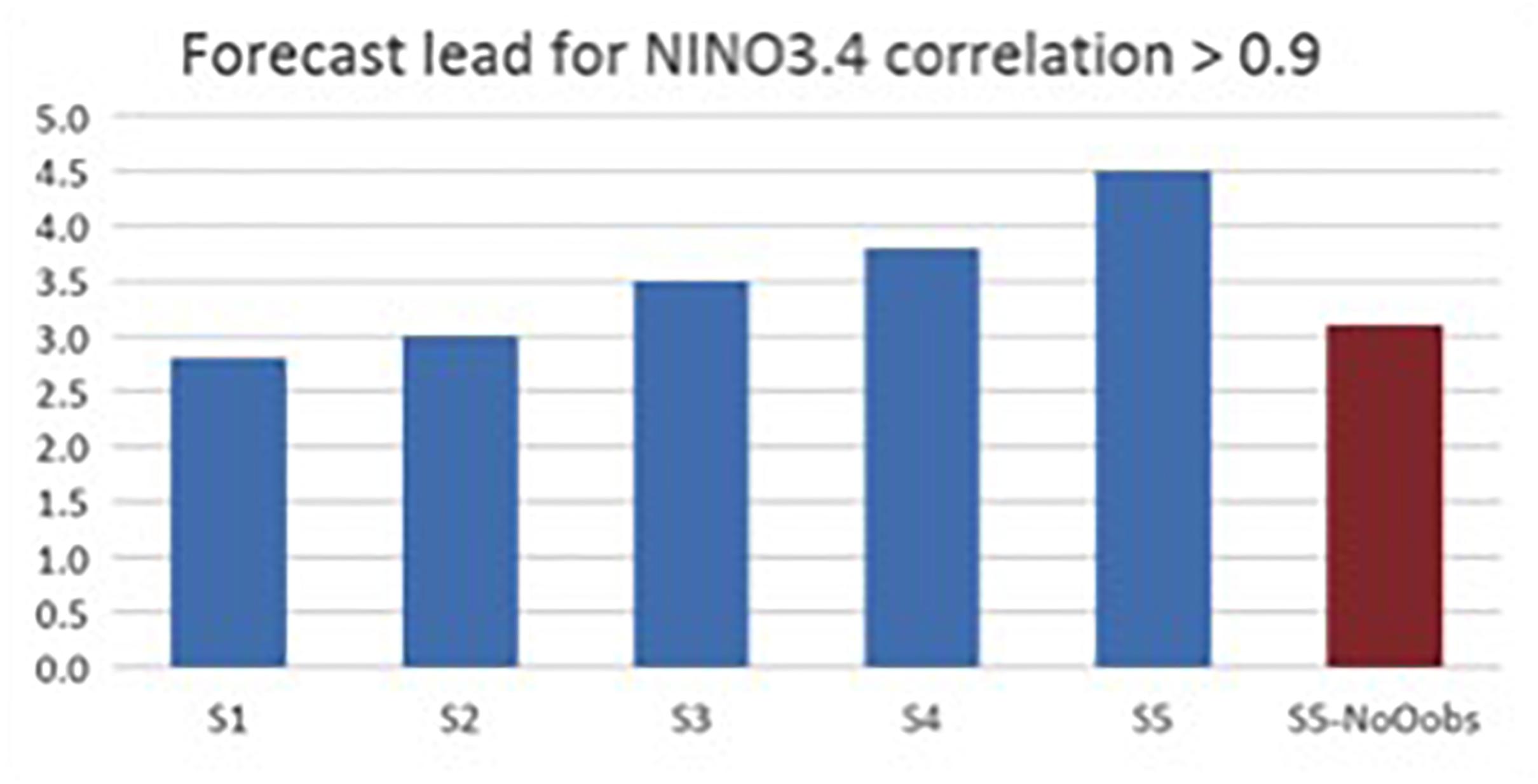
Figure 1. Lead time in months at which NINO3.4 SST anomaly correlation drops below 0.9 over the period 1987–2002. Results are given for five generations of seasonal forecasting systems at ECMWF (S1–S5). A version of SEAS5 without ocean data assimilation (SEAS5-NoOobs) is given for reference from Stockdale et al. (2018).
The original motivation for the Tropical Atmosphere-Ocean (TAO) array and Triangle Trans-Ocean Buoy Network (TRITON) was the 1982–1983 ENSO event (McPhaden, 1995; Ando and Kuroda, 2002). These moorings have provided surface meteorological observations, ocean temperatures in the upper 500 m, salinity and current measurements at selected moorings, and have played a key role in better understanding the ENSO phenomenon and advancing seasonal forecast systems in the decades since their implementation (McPhaden et al., 2010). To support S2S prediction, new observing systems must account for processes occurring over a much broader range of timescales.
Innovative observing technology in the sub-surface layer and at the air-sea interface can help to improve understanding of coupled interactions critical for S2S prediction. Self-sailing boats currently exist that can autonomously gather ocean and atmospheric observations over large areas of the ocean surface. Such technologies have the potential to precipitously drop costs of collecting observations of quantities such as wind, temperature, humidity, salinity, dissolved oxygen, and fluorescence near the ocean surface. These technologies are promising for constraining surface flux estimates in CDA, leading to improved modeling of air-sea interaction and improved initialization of coupled model forecasts. S2S forecasts for high latitudes and midlatitudes can be improved with more numerous and accurate ocean and sea-ice observations in data-sparse regions.
A redesign of the TAO/TRITON array is currently underway by the Tropical Pacific Observing System (TPOS-2020) working group3 that is largely influenced by the volume of new complementary data provided by a number of new observing platforms. TPOS-2020 currently plans a “backbone” design that will support and supplement the broader observing network, including satellite measurements. The TPOS-2020 design is likely to include measurements of the air-sea interface with a vertical and temporal resolution not possible from remote-sensing platforms. Complementary observations include satellite measurements of quantities such as sea level, SST, SSS, wind stress, and precipitation (Mason et al., 2010; National Academies of Sciences, Engineering,, and Medicine [NASEM], 2018, Chp. 2) as well as the in situ Argo profiling float program (see Legler et al., 2015 for a comprehensive review of operational observing systems).
The tropical Atlantic and Indian Oceans are also locations of strong air-sea interaction, exhibiting their own local dominant modes of interannual variability, such as the Indian Ocean Dipole (Saji et al., 1999; Webster et al., 1999) and the Atlantic Niño (Wang, 2005), both of which can modify the timing and expression of ENSO. To track the evolving state of these oceans the TAO mooring design has gradually been extended to the Prediction and Research Moored Array in the Tropical Atlantic (PIRATA) and more recently the Research Moored Array for African-Asian-Australian Monsoon Analysis and Prediction (RAMA) in the tropical Indian Ocean.
The requirements of an observing system change in the Arctic, where the Argo float network is limited due to seasonal ice cover and strong stratification and where satellite remote sensing is limited by heavy cloud cover. These environmental challenges, along with increasing recognition of the importance of seasonal changes in Arctic and their impact on weather systems, has led to rapid development of new instrument types. As regular data from these new instruments become available, evaluation of their impact on S2S forecasts will be needed.
Prediction centers have been slow to incorporate SSS data in ocean DA systems (Maes et al., 2014), though there have been some indications of potential benefits for upper ocean processes that could impact S2S and decadal prediction. Hackert et al. (2011, 2014), Zhu et al. (2014), Tranchant et al. (2018), and Martin et al. (2019) indicated that improved salinity estimates have the potential to improve ENSO forecasts. Though, to date, the impacts shown have been somewhat minor. A number of other studies showed positive impacts due to the assimilation of SSS in controlled experiments, including improved upper ocean salinity (Vernieres et al., 2014), improved surface currents, mixed-layer depth, and barrier layer thickness (Chakraborty et al. (2014, 2015), and improved temporal variability of the vertical distribution of salinity in areas with large freshwater input (Seelanki et al., 2018). Still, the low temporal frequency of the data, large uncertainty estimates attributed to instantaneous observations, and large platform-specific biases (Bao et al., 2019), make the assimilation of SSS a continuing challenge. A next-generation technology that could produce SSS observations with the frequency, accuracy, and coverage of SST observations would be a high-impact capability.
Observing system experiments conducted with real-time forecasting systems have found utility in assimilating sea level observations from multiple altimeters (Lea et al., 2014; Oke et al., 2015a,b). For example, Lea et al. (2014) showed that withholding Jason-2 data resulted in a 4% increase in the global RMS SSH innovations, while withholding all altimeter data resulted in a 16% increase of the global RMS SSH innovations. Verrier et al. (2017) conducted observing system simulation experiments with an eddy-permitting model (1/4-degree horizontal resolution) and found that forecasts of sea level and ocean currents are continually improved when incrementally increasing the number of satellite altimeters from one to two (∼30% error reduction) and from two to three (∼10% additional error reduction). They also note that when assimilating several altimeters, the analysis can resolve western boundary current scales closer to 100 km, versus the native model’s capability to resolve scales around 100–200 km.
Further evaluating observing system impacts on ocean analyses and S2S forecasts will contribute to an ongoing discussion in the design of new oceanic observing systems, such as TPOS-2020 and AtlantOS4. Additionally, new and upcoming satellite missions such as the Surface Water and Ocean Topography (SWOT) will provide higher-fidelity SSH observations than ever before. Coordination between international groups such as CLIVAR and GODAE OceanView is needed for significant progress to be made with international observing efforts (Fuiji, 2019). These international efforts, together with Global Ocean Observing System (GOOS) and its expert panels focusing on physics and biogeochemistry need to work together to build an observing system that recognizes user priorities.
Prediction at Decadal Climate Timescales
Interest in the viability of decadal forecasts is driven by a recognition that these timescales are of increasing importance to decision makers both for governmental policy and private industry (Meehl et al., 2009; Kirtman et al., 2013). Decadal prediction can encompass timescales between several years to a few tens of years, with relevant processes interwoven with those relevant to both S2S forecasts and long- term climate projections. In the extratropics, for example, distinct climate variability has been associated with annual changes in the storm tracks and associated meteorological conditions over the North Pacific and North Atlantic, such as the Pacific Decadal Oscillation (PDO) and the North Atlantic Oscillation (NAO) (Scaife et al., 2014). Decadal prediction is dependent on our ability to forecast not only internal variability of the Earth’s climate system, such as the large-scale climate modes (ENSO, NAO, and PDO), but also how these modes will change under the influence of changes in external forcing, such as arising from human activity. The World Climate Research Program (WCRP) has recognized near-term climate prediction as one of its grand challenges. Despite this recognition, the extent to which decadal climate predictions are able to provide reliable and useful information to users remains uncertain (Meehl et al., 2014).
A sufficiently well observed ocean is crucial for the development of useful decadal predictions (Smith et al., 2012). In order to predict the evolution of natural climate variability, coupled models must be initialized with observations informing the current state of the climate system.
Predictability over these timescales will rely principally on accurately forecasting the slower modes of the coupled climate system, which are highly dependent on long-timescale ocean dynamics. Thus, decadal prediction systems will rely ever more heavily on a sustained ocean-observing system to initialize and verify predictions, similarly to what happened for NWP systems. Sparseness, non-uniformity, and secular changes in the ocean observing system represent a challenge for the initialization and evaluation of a decadal prediction system. Therefore, key factors enabling improved climate prediction skill are the availability of consistent surface and subsurface ocean observations over sufficiently long time spans, improved understanding of processes involved with ocean-atmosphere coupling, and the ability to track the climate modes of variability that determine predictability on a given spatiotemporal scale.
During the last decade, satellites and autonomous in situ platforms have driven a step change in our ability to observe the ocean in near real-time. The use of remotely sensed and autonomous in situ platforms has revolutionized the ocean observing system, and the fast, technological advance on platforms and sensors will continue to improve the system (Figure 2). The next decade will expand upon these advances with new sensors and platforms, coupled with advances in telecommunications.
Decadal prediction systems generally assimilate or relax to SST analysis products. However, an increasing number of systems are also including interior ocean observations, such as temperature and salinity profiles, and sea ice (Doblas-Reyes et al., 2011; O’Kane et al., 2018). Decadal prediction systems, as they focus on seasonal to longer timescales, rely on both real-time data and delayed-mode quality assurance and quality control data (QA/QC) for model initialization and evaluation. Coupled decadal prediction systems often use atmospheric states sampled either from reanalyses or operational products to initialize the atmosphere. However, this practice may need to be revisited and potentially replaced with more sophisticated methods such as CDA. For example, comparison of these products with the sparsely available ocean surface meteorological flux buoys consistently show significant differences both globally and regionally, indicating imbalances in the surface energy and freshwater fluxes at the air- sea interface (Yu, 2019). Maintaining and extending surface flux buoys is vital to understanding the source of these inconsistencies, to improving coupled models, and to evaluating decadal prediction systems.
The heterogeneous nature of the in situ ocean-observing system requires comprehensive metadata, sophisticated data integration, and organized interpretation activity in order to realize the maximum benefit of the observations. Effective data management requires a strong collaborative effort across activities including observation collection, metadata and data assembly using community accepted standards, QA/QC, data publication that enables local and interoperable access, and secure archiving that guarantees long-term preservation of collected data.
Statistics of the innovations generated within the DA procedure can be evaluated to identify broad biases in the differences between model and observations. For example, one can identify regions where there are large innovations due to the assimilation of daily, satellite SSH and SST anomalies.
Significant impacts are often found in the dynamically active regions such as the high-latitude oceans, boundary currents, and along the Equator. Further, with appropriate DA methods, regions of large model biases can be accurately estimated and reduced via direct assimilation of observations (Evensen, 2003). On longer timescales, the sparser in situ observing network can provide similar guidance for correcting long-timescale model biases.
There remain many unanswered questions on the fundamental nature and drivers of ocean variability. Decadal prediction depends on the presence of “oscillations” that have the potential to remain coherent on multi-year to multi-decadal time scales. To the extent that such slowly evolving dynamical regimes exist (e.g., along which climate anomalies propagate), it is important that the DA system is capable of maintaining these lower frequency signals. It is also critical to understand how these anomalous ocean signals are influenced by the ocean-atmosphere boundary. Improved dynamical understanding of the ocean, sea ice, and atmosphere, and their coupled interfaces and teleconnections, will lead to more reliable and skillful multi-year to decadal climate forecasts.
There is a need for full-depth observations that provide measurements able to resolve the dominant temporal and spatial scales of variability of the ocean. We encourage continuing to leverage the sustained ocean-observing infrastructure for short-term intensive process study campaigns that target key knowledge gaps such as air-sea-land and ice coupling. When such process studies are conducted, greater interaction with the DA community before, during, and after the campaigns could help to identify observations that may be good candidates for transitioning into the sustained observing system. To this end, we encourage stronger collaboration between the communities developing near-term forecasting and ocean observing platforms to aid model development and observational design.
Conclusion
The ocean-observing system plays an important role in developing historical reconstructions of the ocean and initializing forecasts of the coupled Earth system at all timescales. The ideal observational sampling strategy will continue to evolve as we improve our understanding of the spatial and temporal scales of ocean variability and as technological observing capabilities improve. An ongoing challenge for the reanalysis and prediction communities will be to maintain close collaboration with the ocean-observing community that is developing the next-generation ocean-observing systems. This collaboration should occur at all stages, including the design, implementation, and decision making that determines sustained observations. The ocean DA community should provide programmatic guidance to the ocean-observing community regarding what types of observations would be most useful when established in a sustained observing network to best support ocean monitoring and prediction at various timescales. This problem requires the solution of a complicated optimization problem that is defined by a stated goal (e.g., to maximize the skill of a forecast), while taking into account the limitations of the forecast model, of each observing platform, and of the DA method itself. So far, this is not a mainstream activity and further coordination is needed in the coming decade to make observing system design a key application of ocean DA and CDA.
We anticipate a continuing race between the physical scales resolved by modeling and observing systems. DA systems must be able to constrain increasingly high-resolution numerical models at physical scales supported by the observation network. This poses dual challenges to make better use of a sparse observing system that will become increasingly coarse, in a relative sense, as model resolutions increase, as well as the need to incorporate as much information as possible from high-resolution satellite observing systems. With upcoming satellite missions, the satellite-based ocean-observing system may at times evolve to support much higher resolutions of observed data products than a state-of-the-art operational forecast model can support. While a precise DA strategy should be developed for such scenarios, we also encourage coordination to take place between the modeling and prediction centers and the teams developing plans for future satellite observing missions in order to ensure prioritization of those missions that have maximum impact on prediction skill.
To support CDA developments for operational applications, we recommend that a high priority be placed on ensuring consistency between atmosphere and ocean data governance bodies (e.g., WMO and Copernicus Climate Change Service and Copernicus Marine Environment Monitoring Service). At present, many ocean observations risk missing the cut-off times associated with the timelines of operational NWP. Improved infrastructure is needed to support research and operations, including real-time transmission of observed data and real-time feedback from users regarding the quality control of those data relative to other observing sources. For operational coupled Earth system approaches, used for reanalyses and prediction, it is crucial to enhance the consistency between the atmospheric and the ocean-observing systems, not only in terms of timeliness and infrastructure but also in terms of funding support and sustainability. The European Environment Agency State of Play Report (The European Environment Agency [EEA], 2017) pointed out that the ocean-observing system lacks prospects for long term funding. About 70% of data in the GOOS is funded by time-limited research projects (in contrast to 25% for atmospheric observations). In situ ocean observations are based on infrastructures mainly supported by national agencies, and in recent years the number of observation sites and platforms have gone through periods of decline. They also emphasized that more coordination is needed between funding agencies, operators, and users of ocean observations internationally. In addition, the EEA State of Play report emphasized the lack of biogeochemical and deep (2000 m and deeper) ocean observations.
Finally, the combination of increases in computing power and availability of observations has enabled the development of ensemble coupled DA systems. Ensemble-based approaches have the ability to identify and track the largest growing disturbances within the system. These growing disturbances represent regions of high variance where potential predictability of the system resides. The identification of these growing disturbances provides information about regions of the ocean where observations are likely to have the largest impact on the evolving coupled system and likely lead to useful predictions at all scales. Emerging CDA methods, enabled by coupled Earth system modeling, provide a great opportunity for increased collaboration across communities and rapid advances in scientific understanding over the next decade.
Author Contributions
All authors contributed to a group writing of this review manuscript. SP was the lead coordinator and writer. AM and MM (ocean data assimilation), SP (coupled data assimilation), SA (CDA of radiance data for SST), AS (ocean reanalysis), AS (S2S prediction), and BS (decadal prediction) were section leads for each subsection of the manuscript.
Funding
SP was supported by the National Oceanographic and Atmospheric Administration (NOAA) via the National Environmental Satellite, Data, and Information Service (NESDIS), and the Next Generation Global Prediction System (NGGPS) Research to Operations (R2O) program, and the Climate Prediction Office (CPO). BS, TO’K, PS, TM, and CC were supported by CSIROs Decadal Climate Forecasting Project. PH is supported in part by NASA #NNH15ZDA001N-MAP and NSF-OPP #1750035.
Conflict of Interest Statement
The authors declare that the research was conducted in the absence of any commercial or financial relationships that could be construed as a potential conflict of interest.
The Reviewer DM declares an ongoing collaboration on a project with the Author, PH, as a contribution to the collaboration on OceanObs19. The peer review was handled under the close supervision of the Chief Editors to ensure an objective process.
Footnotes
References
Abraham, J. P., Baringer, M., Bindoff, N. L., Boyer, T., Cheng, L. J., Church, J. A., et al. (2013). A review of global ocean temperature observations: implications for ocean heat content estimates and climate change. Rev. Geophys. 51, 450–483. doi: 10.1002/rog.20022
Akella, S., Todling, R., and Suarez, M. (2017). Assimilation for skin SST in the NASA GEOS atmospheric data assimilation system. Q. J. R. Meteorol. Soc. 143, 1032–1046. doi: 10.1002/qj.2988
Anderson, C. R., Kudela, R. M., Kahru, M., Chao, Y., Rosenfeld, L. K., Bahr, F. L., et al. (2016). Initial skill assessment of the California harmful algae risk mapping (C- HARM) system. Harmful Algae 59, 1–18. doi: 10.1016/j.hal.2016.08.006
Ando, K., and Kuroda, Y. (2002). Two modes of salinity and temperature variation in the surface layer of the pacific warm pool. J. Oceanogr. 58, 599–609.
Balmaseda, M. A. (2017). Data assimilation for Initialization of seasonal forecasts. The sea: the science of ocean prediction. J. Mar. Res. 75, 331–359. doi: 10.1371/journal.pcbi.1004239
Balmaseda, M. A., Alves, O., Arribas, A., Awaji, T., Behringer, D., Ferry, N., et al. (2009). Ocean initialization for seasonal forecasts. Oceanography 22, 154–159. doi: 10.5670/oceanog.2009.73
Balmaseda, M. A., de Rosnay, P., Hersbach, H., Keeley, S., McNally, T., and Tietsche, S. (2018). Workshop on Observations and Analysis of Sea-Surface Temperature and Sea Ice for NWP and Climate Applications. Reading: ECMWF.
Balmaseda, M. A., Dee, D., Vidard, A., and Anderson, D. L. (2007). A multivariate treatment of bias for sequential data assimilation: application to the tropical oceans. Q. J. R. Meteorol. Soc. 133, 167–179. doi: 10.1002/qj.12
Balmaseda, M. A., Hernandez, F., Storto, A., Palmer, M. D., Alves, O., Shi, L., et al. (2015). The ocean reanalyses intercomparison project (ORA-IP). J. Operat. Oceanogr. 8, s80–s97. doi: 10.1080/1755876X.2015.1022329
Balmaseda, M. A., Trenberth, K. E., and Källén, E. (2013). Distinctive climate signals in reanalysis of global ocean heat content. Geophys. Res. Lett. 40, 1754–1759. doi: 10.1002/grl.50382
Bao, S., Wang, H., Zhang, R., Yan, H., and Chen, J. (2019). Comparison of satellite-derived sea surface salinity products from SMOS, Aquarius, and SMAP. J. Geophys. Res. 124, 1932–1944. doi: 10.1029/2019JC014937
Becker, E. A., Forney, K. A., Fiedler, P. C., Barlow, J., Chivers, S. J., Edwards, C. A., et al. (2016). Moving towards dynamic ocean management: how well do modeled ocean products predict species distributions? Remote Sens. 8:149. doi: 10.3390/rs8020149
Bell, M. J., Schiller, A., Le Traon, P. Y., Smith, N. R., Dombrowsky, E., and Wilmer-Becker, K. (2015). An introduction to GODAE oceanview. J. Operat. Oceanogr. 8, s2–s11. doi: 10.1080/1755876X.2015.1022041
Bellenger, H., Drushka, K., Asher, W., and Reverdin, G. (2017). Extension of the prognostic model of sea surface temperature to rain-induced cool and fresh lenses. J. Geophys. Res. 122, 484–507. doi: 10.1002/2016jc012429
Bertino, L., and Holland, M. M. (2017). Coupled ice-ocean modeling and predictions. J. Mar. Res. 75, 839–875. doi: 10.1357/002224017823524017
Blockley, E. W., Martin, M. J., McLaren, A. J., Ryan, A. G., Waters, J., Lea, D. J., et al. (2014). Recent development of the Met Office operational ocean forecasting system: an overview and assessment of the new Global FOAM forecasts. Geosci. Model. Dev. 7, 2613–2638. doi: 10.5194/gmd-7-2613-2014
Bloom, S. C., Takacs, L. L., da Silva, A. M., and Ledvina, D. (1996). Data assimilation using incremental analysis updates. Mon. Wea. Rev. 124, 1256–1271. doi: 10.1175/1520-0493(1996)124<1256:dauiau>2.0.co;2
Bolton, T., and Zanna, L. (2019). Applications of deep learning to ocean data inference and subgrid parameterization. JAMES 11, 376–399. doi: 10.1029/2018ms001472
Brankart, J.-M., Candille, G., Garnier, F., Calone, C., Melet, A., Bouttier, P.-A., et al. (2015). A generic approach to explicit simulation of uncertainty in the NEMO ocean model. Geosci. Model Dev. 8, 1285–1297. doi: 10.5194/gmd-8-1285-2015
Brasseur, P., Gruber, N., Barciela, R., Brander, K., Hobday, A. J., Huret, M., et al. (2009). Integrating biogeochemistry and ecology into ocean data assimilation systems. Oceanography 22, 206–215. doi: 10.5670/oceanog.2009.80
Brassington, G. B., Martin, M. J., Tolman, H. L., Akella, S., Balmeseda, M., Chambers, C. R. S., et al. (2015). Progress and challenges in short- to medium-range coupled prediction. J. Operat. Oceanogr. 8, s239–s258. doi: 10.1080/1755876X.2015.1049875
Brunke, M. A., Zeng, X., Misra, V., and Beljaars, A. (2008). Integration of a prognostic sea surface skin temperature scheme into weather and climate models. J. Geophys. Res. 113:D21117.
Buckley, M. W., Ponte, R. M., Forget, G., and Heimbach, P. (2015). Determining the origins of advective heat transport convergence variability in the North Atlantic. J. Clim. 28, 3943–3956. doi: 10.1175/JCLI-D-14-00579.1
Carton, J. A., Chepurin, G. A., Chen, L., and Grodsky, S. A. (2018). Improved global net surface heat flux. J. Geophys. Res. 123, 3144–3163. doi: 10.1002/2017JC013137
Carton, J. A., and Giese, B. S. (2008). A reanalysis of ocean climate using simple ocean data assimilation (SODA). Mon. Weather Rev. 136, 2999–3017. doi: 10.1175/2007mwr1978.1
Carton, J. A., Penny, S. G., and Kalnay, E. (2019). Temperature and Salinity Variability in the SODA3, ECCO4r3, and ORAS5 Ocean Reanalyses, 1993–2015. J. Clim. 32, 2277–2293. doi: 10.1175/JCLI-D-18-0605.1
Casey, K. S., Brandon, T. B., Cornillon, P., and Evans, R. (2010). in The Past, Present and Future of the AVHRR Pathfinder SST Program. Oceanography from Space, eds V. Barale, J. F. R. Gower, and L. Alberotanza (Berlin: Springer). doi: 10.1175/jcli-d-18-0605.1
Castro, S. L., Wick, G. A., and Emery, W. J. (2012). Evaluation of the relative performance of sea surface temperature measurements from different types of drifting and moored buoys using satellite7 derived reference products. J. Geophys. Res. 117:C02029. doi: 10.1029/2011JC007472
Chakraborty, A., Sharma, R., Kumar, R., and Ba, S. (2014). A SEEK filter assimilation of sea surface salinity from Aquarius in an OGCM: implication for surface dynamics and thermohaline structure. J. Geophys. Res. 119, 4777–4796. doi: 10.1002/2014JC009984
Chakraborty, A., Sharma, R., Kumar, R., and Basu, S. (2015). Joint assimilation of Aquarius-derived sea surface salinity and AVHRR-derived sea surface temperature in an ocean general circulation model using SEEK filter: implication for mixed layer depth and barrier layer thickness. J. Geophys. Res. 120, 6927–6942. doi: 10.1002/2015JC010934
Chang, Y.-S., Zhang, S., Rosati, A., Delworth, T. L., and Stern, W. F. (2013). An assessment of oceanic variability for 1960–2010 from the GFDL ensemble coupled data assimilation. Clim. Dyn. 40, 775–803. doi: 10.1007/s00382-012-1412-2
Chaudhuri, A. H., Ponte, R. M., and Forget, G. (2016). Impact of uncertainties in atmospheric boundary conditions on ocean model solutions. Ocean Model. 100, 96–108. doi: 10.1016/j.ocemod.2016.02.003
Chaudhuri, A. H., Ponte, R. M., Forget, G., and Heimbach, P. (2013). A comparison of atmospheric reanalysis surface products over the ocean and implications for uncertainties in air–sea boundary forcing. J. Clim. 26, 153–170. doi: 10.1175/JCLI-D-12-00090.1
Chelton, D. B., and Wentz, F. J. (2005). Global microwave satellite observations of sea surface temperature for numerical weather prediction and climate research. Bull. Am. Meteorol. Soc. 86, 1097–1116. doi: 10.1175/bams-86-8-1097
Chelton, D. B., and Xie, S.-P. (2010). Coupled ocean-atmosphere interaction at oceanic mesoscales. Oceanography 23, 52–69. doi: 10.5670/oceanog.2010.05
Chevallier, M., Smith, G. C., Dupont, F., Lemieux, J.-F., Forget, G., Fujii, Y., et al. (2017). Intercomparison of the Arctic sea ice cover in global ocean-sea ice reanalyses from the ORA-IP project. Clim. Dyn. 49, 1107–1136. doi: 10.1007/s00382-016-2985-y
Ciavatta, S., Kay, S., Saux-Picart, S., Butenschön, M., and Allen, J. I. (2016). Decadal reanalysis of biogeochemical indicators and fluxes in the North West European shelf-sea ecosystem. J. Geophys. Res. Oceans 121, 1824–1845. doi: 10.1002/2015jc011496
Compo, G. P., Whitaker, J. S., Sardeshmukh, P. D., Matsui, N., Allan, R. J., Yin, X., et al. (2011). The twentieth century reanalysis project. Quart. J. Roy. Meteor. Soc. 137, 1–28. doi: 10.1002/qj.776
Dee, D. P., Balmaseda, M., Balsamo, G., Engelen, R., Simmons, A. J., and Thépaut, J.-N. (2014). Toward a consistent reanalysis of the climate system. Bull. Am. Meteorol. Soc. 95, 1235–1248. doi: 10.1175/bams-d-13-00043.1
Derber, J., and Li, X. (2018). Assimilating SST With an Atmospheric DA System. Available at: https://www.ecmwf.int/sites/default/files/elibrary/2018/17984-assimilating-sst-atmospheric-da-system.pdf (accessed January 2018).
Doblas-Reyes, F. J., Balmaseda, M. A., Weisheimer, A., and Palmer, T. N. (2011). Decadal climate prediction with the European centre for medium-range weather forecasts coupled forecast system: impact of ocean observations. J. Geophys. Res. 116:D19111. doi: 10.1029/2010JD015394
Domingues, C. M., and Palmer, M. D. (2015). The IQuOD initiative: towards an international quality- controlled ocean database. CLIVAR Exchanges 67, 38–40.
Dong, S., Volkov, D., Goni, G., Lumpkin, R., and Foltz, G. R. (2017). Near7surface salinity and temperature structure observed with dual-sensor drifters in the subtropical South Pacific. J. Geophys. Res. 122, 5952–5969. doi: 10.1002/2017jc012894
Donlon, C., Robinson, I., Casey, K. S., and Vazquez-Cuervo, J. (2007). The global ocean data assimilation experiment high-resolution sea surface temperature pilot project. Bull. Am. Meteorol. Soc. 88, 1197–1214. doi: 10.1175/bams-88-8-1197
Donlon, C. J., Minnett, P. J., Gentemann, C., and Nightingale, T. J. (2002). Toward improved validation of satellite sea surface skin temperature measurements for climate research. J. Clim. 15, 353–369. doi: 10.1175/1520-0442(2002)015<0353:tivoss>2.0.co;2
d’Orgeville, M., and Peltier, W. R. (2007). On the pacific decadal oscillation and the atlantic multidecadal oscillation: might they be related? Geophys. Res. Let. 34:L23705. doi: 10.1029/2007GL031584
Durack, P. J., Lee, T., Vinogradova, N. T., and Stammer, D. (2016). Keeping the lights on for global ocean salinity observation. Nat. Clim. Change 6, 228–231. doi: 10.1038/nclimate2946
Edwards, C. A., Moore, A. M., Hoteit, I., and Cornuelle, B. D. (2015). Regional ocean data assimilation. Annu. Rev. Mar. Sci. 7, 6.1–6.22.
Evensen, G. (2003). The Ensemble Kalman filter: theoretical formulation and practical implementation. Ocean Dyn. 53, 343–367. doi: 10.1007/s10236-003-0036-9
Feng, M., Zhang, X., Oke, P., Monselesan, D., Chamberlain, M., Matear, R., et al. (2016). Invigorating ocean boundary current systems around Australia during 1979-2014 – as simulated in a near-global eddy-resolving ocean model. J. Geophys. Res. 121, 3395–3408. doi: 10.1002/2016JC011842
Fenty, I., and Heimbach, P. (2013). Coupled sea ice–ocean-state estimation in the labrador sea and baffin bay. J. Phys. Oceanogr. 43, 884–904. doi: 10.1175/JPO-D-12-065.1
Ferry, N., Parent, L., Garric, G., Barnier, B., Jourdain, N. C., and the Mercator Team. (2010). Mercator global eddy permitting ocean reanalysis GLORYS1V1: description and results. Mercator. Newslett. 36, 15–27.
Ferry, N., Rémy, E., Brasseur, P., and Maes, C. (2007). The Mercator global ocean operational analysis system: assessment and validation of an 11-year reanalysis. J. Mar. Syst. 65, 540–560. doi: 10.1016/j.jmarsys.2005.08.004
Folland, C. K., and Parker, D. E. (1995). Correction of instrumental biases in historical sea surface temperature data. Q. J. R. Meteorol. Soc. 121, 319–367. doi: 10.1002/qj.49712152206
Ford, D., and Barciela, R. (2017). Global marine biogeochemical reanalyses assimilating two different sets of merged ocean colour products. Remote Sens. Environ. 203, 40–54. doi: 10.1016/j.rse.2017.03.040
Forget, G., Campin, J. M., Heimbach, P., Hill, C. N., Ponte, R. M., and Wunsch, C. (2015). ECCO version 4: an integrated framework for non-linear inverse modeling and global ocean state estimation. Geosci. Model Dev. 8, 3071–3104. doi: 10.5194/gmd-8-3071-2015
Fujii, Y., Cummings, J., Xue, Y., Schiller, A., Lee, T., Balmaseda, M. A., et al. (2015). Evaluation of the tropical pacific observing system from the ocean data assimilation perspective. Q. J. R. Meteorol. Soc. 141, 2481–2496. doi: 10.1002/qj.2579
Fuiji, Y. (2019). Observing system evaluation based on ocean data assimilation and prediction systems: on-going challenges and future vision for designing/supporting ocean observational networks. Front. Mar. Sci. (accepted).
Garric, G., Parent, L., Greiner, E., Drévillon, M., Hamon, M., Lellouche, J., et al. (2018). “Performance and quality assessment of the global ocean eddy-permitting physical reanalysis GLORYS2V4. Operational oceanography serving sustainable marine development,” in Proceedings of the Eight EuroGOOS International Conference, Bergen.
Gentemann, C. L., and Akella, S. (2018). Evaluation of NASA GEOS7ADAS modeled diurnal warming through comparisons to SEVIRI and AMSR2 SST observations. J. Geophys. Res. 123, 1364–1375. doi: 10.1002/2017JC013186
Giese, B. S., Seidel, H. F., Compo, G. P., and Sardeshmukh, P. D. (2016). An ensemble of ocean reanalyses for 1815–2013 with sparse observational input. J. Geophys. Res. Oceans 121, 6891–6910. doi: 10.1002/2016JC012079
Gregg, W. W., Rousseaux, C. S., and Franz, B. A. (2017). Global trends in ocean phytoplankton: a new assessment using revised ocean colour data. Remote Sens. Lett. 8, 1102–1111. doi: 10.1080/2150704X.2017.1354263
Hackert, E., Ballabrera-Poy, J., Busalacchi, A. J., Zhang, R.-H., and Murtugudde, R. (2011). Impact of sea surface salinity assimilation on coupled forecasts in the tropical Pacific. J. Geophys. Res. Oceans 116:C05009. doi: 10.1029/2010JC006708
Hackert, E., Busalacchi, A. J., and Ballabrera-Poy, J. (2014). Impact of Aquarius sea surface salinity observations on coupled forecasts for the tropical Indo-Pacific Ocean. J. Geophys. Res. 119, 4045–4067. doi: 10.1002/2013JC009697
Haid, V., Iovino, D., and Masina, S. (2017). Impacts of freshwater changes on Antarctic sea ice in an eddy-permitting sea-ice–ocean model. Cryosphere 11, 1387–1402. doi: 10.5194/tc-11-1387-2017
Heimbach, P., Fukumori, I., Hill, C. N., Ponte, R. M., Stammer, D., Wunsch, C., et al. (2019). Putting it all together: adding value to the global ocean and climate observing systems with complete self-consistent ocean state and parameter estimates. Front. Mar. Sci. 6:55. doi: 10.3389/fmars.2019.00055
Hoskins, B. (2013). The potential for skill across the range of the seamless weather7climate prediction problem: a stimulus for our science. Q. J. R. Meteorol. Soc. 139, 573–584. doi: 10.1002/qj.1991
Hoteit, I., Luo, X., Bocquet, M., Kohl, A., and Eit-El-Fquih, B. (2018). “Data assimilation in oceanography: current status and new directions,” in New Frontiers in Operational Oceanography, eds E. Chassignet, A. Pascual, J. Tintore, and J. Verron (Scotts Valley, CA: CreateSpace Independent Publishing Platform).
Ignatov, A., Zhou, X., Petrenko, B., Liang, X., Kihai, Y., Dash, P., et al. (2016). AVHRR GAC SST reanalysis version 1 (RAN1). Remote Sens. 8:315. doi: 10.3390/rs8040315
Juricke, S., MacLeod, D., Weisheimer, A., Zanna, L., and Palmer, T. (2018). “Seasonal to annual ocean forecasting skill and the role of model and observational uncertainty,” in Proceedings 20th conference EGU General Assembly, EGU2018, Vienna.
Kalnay, E., Kanamitsu, M., Kistler, R., Collins, W., Deaven, D., Gandin, L., et al. (1996). The NCEP/NCAR 40- year reanalysis project. Bull. Amer. Meteor. Soc. 77, 437–471.
Kawai, Y., and Wada, A. (2007). Diurnal sea surface temperature variation and its impact on the atmosphere and ocean: a review. J. Oceanogr. 63, 721–744. doi: 10.1007/s10872-007-0063-0
Kazumori, M., Geer, A. J., and English, S. J. (2016). Effects of all7sky assimilation of GCOM7 W/AMSR2 radiances in the ECMWF numerical weather prediction system. Q. J. R. Meteorol. Soc. 142, 721–737. doi: 10.1002/qj.2669
Kennedy, J. J. (2014). A review of uncertainty in in situ measurements and data sets of sea surface temperature. Rev. Geophys. 52, 1–32. doi: 10.1002/2013RG000434
Kimmritz, M., Counillon, F., Bitz, C. M., Massonnet, F., Bethke, I., and Gao, Y. (2018). Optimising assimilation of sea ice concentration in an Earth system model with a multicategory sea ice model. Tellus 2018:1435945.
Kirtman, B., Power, S. B., Adedoyin, J. A., Boer, G. J., Bojariu, R., Camilloni, I., et al. (2013). in Near-term Climate Change: Projections and Predictability. Climate Change 2013: The Physical Science Basis. Contribution of Working Group I to the Fifth Assessment Report of the Intergovernmental Panel on Climate Change, eds T. F. Stocker, D. Qin, G.-K. Plattner, M. Tignor, S. K. Allen, J. Boschung, et al. (Cambridge: Cambridge University Press).
Köhl, A. (2015). Evaluation of the GECCO2 ocean synthesis: transports of volume, heat, and freshwater in the Atlantic. Q. J. R. Meteorol. Soc. 141, 166–181. doi: 10.1002/qj.2347
Laloyaux, P., de Boisseson, E., Balmaseda, M., Bidlot, J. R., Broennimann, S., Buizza, R., et al. (2018a). CERA-20C: a coupled reanalysis of the twentieth century. J. Adv. Model. Earth Syst. 10, 1172–1195. doi: 10.1029/2018MS001273
Laloyaux, P., Frolov, S., Ménétrier, B., and Bonavita, M. (2018b). Implicit and explicit cross correlations in coupled data assimilation. Q. J. R. Meteorol. Soc. 144, 1851–1863. doi: 10.1002/qj.3373
Lea, D. J., Drecourt, J., Haines, K., and Martin, M. J. (2008). Ocean altimeter assimilation with observational and model-bias correction. Q. J. R. Meteorol. Soc. 134, 1761–1774. doi: 10.1002/qj.320
Lea, D. J., Martin, M. J., King, R. R., Hines, A., Walters, D., and Thurlow, M. (2015). Assessing a new coupled data assimilation system based on the Met Office coupled atmosphere–land–ocean–sea ice model. Mon. Weather Rev. 143, 4678–4694. doi: 10.1175/MWR-D-15-0174.1
Lea, D. J., Martin, M. J., and Oke, P. R. (2013). Demonstrating the complementarity of observations in an operational ocean forecasting system. Q. J. R. Meteorol. Soc. 140, 2037–2049. doi: 10.1002/qj.2281
Lea, D. J., Martin, M. J., and Oke, P. R. (2014). Demonstrating the complementarity of observations in an operational ocean forecasting system. Q. J. R. Meteorol. Soc. 140, 2037–2049. doi: 10.1002/qj.2281
Legler, D. M., Freeland, H. J., Lumpkin, R., Ball, G., McPhaden, M. J., North, S., et al. (2015). The current status of the real-time in situ global ocean observing system for operational oceanography. J. Operat. Oceanogr. 8, 189–200. doi: 10.1080/1755876X.2015.1049883
Liang, X., and Yu, L. (2016). Variations of the global net air–sea heat flux during the “Hiatus” period (2001–10). J. Clim. 29, 3647–3660. doi: 10.1175/JCLI-D-15-0626.1
Lindsay, R., Wensnahan, M., Schweiger, A., and Zhang, J. (2014). Evaluation of seven different atmospheric reanalysis products in the Arctic. J. Clim. 27, 2588–2606. doi: 10.1175/jcli-d-13-00014.1
Maes, C., Reul, N., Behringer, D., and O’Kane, T. J. (2014). The salinity signature of the equatorial Pacific cold tongue as revealed by the satellite SMOS mission. Geosci. Lett. 1:17.
Martin, M., Dash, P., Ignatov, A., Banzon, V., Beggs, H., Brasnett, B., et al. (2012). Group for high resolution sea surface temperature (GHRSST) analysis fields inter-comparisons. Part 1: a GHRSST multi-product ensemble (GMPE). Deep Sea Res. Part II: Top. Stud. Oceanogr. 77, 21–30. doi: 10.1016/j.dsr2.2012.04.013
Martin, M. J., Balmaseda, M., Bertino, L., Brasseur, P., and Brassington, G. (2015). Status and future of data assimilation in operational oceanography. J. Operat. Oceanogr. 8, s28–s48. doi: 10.1080/1755876X.2015.1022055
Martin, M. J., King, R. R., While, J., and Aguiar, A. B. (2019). Assimilating satellite sea-surface salinity data from SMOS, Aquarius and SMAP into a global ocean forecasting system. Q. J. R. Meteorol. Soc. 145, 705–726. doi: 10.1002/qj.3461
Masina, S., Storto, A., Ferry, N., Valdivieso, M., Haines, K., Balmaseda, M., et al. (2017). An ensemble of eddy-permitting global ocean reanalyses from the MyOcean project. Clim. Dyn. 49, 813–841. doi: 10.1007/s00382-015-2728-5
Mason, P. J., Zillman, J. W., Simmons, A., Lindstrom, E. J., Harrison, D. E., Dolman, H., et al. (2010). Implementation Plan for the Global Observing System for Climate in Support of the UNFCCC (2010 Update). Geneva: WMO.
Mazloff, M. R., Cornuelle, B. D., Gille, S. T., and Verdy, A. (2018). Correlation lengths for estimating the large-scale carbon and heat content of the southern ocean. J. Geophys. Res. 123, 883–901. doi: 10.1002/2017jc013408
McPhaden, M. J. (1995). The tropical atmosphere ocean (TAO) array is completed. Bull. Am. Meteorol. Soc. 76, 739–741.
McPhaden, M. J., Busalacchi, A. J., and Anderson, D. L. T. (2010). A TOGA retrospective. Oceanography 23, 86–103. doi: 10.5670/oceanog.2010.26
Meehl, G. A., Goddard, L., Boer, G., Burgman, R., Branstator, G., Cassou, C., et al. (2014). Decadal climate prediction: an update from the trenches. Bull. Amer. Meteorol. Soc. 95, 243–267.
Meehl, G. A., Goddard, L., Murphy, J., Stouffer, R. J., Boer, G., Danabasoglu, G., et al. (2009). Decadal prediction: can it be skillful? Bull. Am. Meteorol. Soc. 90, 1467–1485.
Mignac, D., Ferreira, D., and Haines, K. (2018). South Atlantic meridional transports from NEMO-based simulations and reanalyses. Ocean Sci. 14, 53–68. doi: 10.5194/os-14-53-2018
Moore, A. M., Martin, M. J., Akella, S. R., Arango, H. G., Balmaseda, M. A. A., Bertino, L., et al. (2019). Synthesis of ocean observations using data assimilation for operational, real-time and reanalysis systems: a more complete picture of the state of the ocean. Front. Mar. Sci. 6. doi: 10.3389/fmars.2019.00090
National Academies of Sciences, Engineering,, and Medicine [NASEM] (2018). Thriving on Our Changing Planet: A Decadal Strategy for Earth Observation from Space. Washington, DC: The National Academies Press.
National Academies of Sciences, Engineering, and Medicine [NASEM] (2017). Sustaining Ocean Observations to Understand Future Changes in Earth’s Climate. Washington, DC: The National Academies Press.
O’Kane, T. J., Sandery, P. A., Monselesan, D. P., Sakov, P., Chamberlain, M. A., Matear, R. J., et al. (2018). Coupled data assimilation and ensemble initialization with application to multi-year ENSO prediction. J. Clim. 32, 997–1024.
Oke, P. R., Brassington, G. B., Griffin, D. A., and Schiller, A. (2008). The bluelink ocean data assimilation system (BODAS). Ocean Model. 20, 46–70. doi: 10.1016/j.ocemod.2007.11.002
Oke, P. R., and Griffin, D. A. (2011). The cold-core eddy and strong upwelling off the coast of New South Wales in early 2007. Deep Sea Res. 58, 574–591. doi: 10.1016/j.dsr2.2010.06.006
Oke, P. R., Larnicol, G., Fujii, Y., Smith, G. C., Lea, D. J., Guinehut, S., et al. (2015a). Assessing the impact of observations on ocean forecasts and reanalyses: part 1, global studies. J. Oper. Oceanogr. 8, s49–s62. doi: 10.1080/1755876x.2015.1022067
Oke, P. R., Larnicol, G., Jones, E. M., Kourafalou, V., Sperrevik, A. K., Carse, F., et al. (2015b). Assessing the impact of observations on ocean forecasts and reanalyses: part 2, regional applications. J. Oper. Oceanogr. 8, s63–s79. doi: 10.1080/1755876x.2015.1022080
Oke, P. R., Sakov, P., Cahill, M. L., Dunn, J. R., Fiedler, R., Griffin, D. A., et al. (2013). Towards a dynamically balanced eddy-resolving ocean reanalysis: BRAN3. Ocean Modelling 67, 52–70. doi: 10.1016/j.ocemod.2013.03.008
Oke, P. R., Schiller, A., Griffin, G. A., and Brassington, G. B. (2005). Ensemble data assimilation for an eddy- resolving ocean model. Q. J. R. Meteorol. Soc. 131, 3301–3311. doi: 10.1256/qj.05.95
Palmer, M. D., Roberts, C. D., Balmaseda, M., Chang, Y.-S., Chepurin, G., Ferry, N., et al. (2017). Ocean heat content variability and change in an ensemble of ocean reanalyses. Clim. Dyn. 49, 909–930. doi: 10.1007/s00382-015-2801-0
Penny, S. G. (2017). Mathematical foundations of hybrid data assimilation from a synchronization perspective. Chaos 27:126801. doi: 10.1063/1.5001819
Penny, S. G., Akella, S., Alves, O., Bishop, C., Buehner, M., Chevallier, M., et al. (2017). Coupled Data Assimilation for Integrated Earth System Analysis and Prediction: Goals, Challenges and Recommendations. Geneva: World Meteorological Organization.
Penny, S. G., Bach, E., Bhargava, K., Chang, C.-C., Da, C., Sun, L., et al. (2019). Strongly coupled data assimilation in multiscale media: experiments using a quasi-geostrophic coupled model. J. Adv. Model Earth Syst. 11. doi: 10.1029/2019MS001652
Penny, S. S., Behringer, D. W., Carton, J. A., and Kalnay, E. (2015). A hybrid global ocean data assimilation system at NCEP. Mon. Weather Rev. 143, 4660–4677. doi: 10.1063/1.5001819
Perruche, C., Albert, A., and Drillet, Y. (2017). “PISCES biogeochemical reanalysis,” in Proceedings of the ERA-CLIM2 3rd General Assembly, Vienna.
Piecuch, C. G., Ponte, R. M., Little, C. M., Buckley, M. W., and Fukumori, I. (2017). Mechanisms underlying recent decadal changes in subpolar North Atlantic Ocean heat content. J. Geophys. Res. 122, 7181–7197. doi: 10.1002/2017JC012845
Poli, P., Hersbach, H., Tan, D. G. H., Dee, D. P., Thépaut, J.-N., Simmons, A., et al. (2013). The Data Assimilation System and Initial Performance Evaluation of the ECMWF Pilot Reanalysis of the 20th-Century Assimilating Surface Observations Only (ERA-20C). Reading: ECMWF.
Reynolds, R. W., Smith, T. M., Liu, C., and Chelton, D. B. (2007). Daily high-resolution-blended analyses for sea surface temperature. J. Clim. 20, 5473–5496. doi: 10.1175/2007jcli1824.1
Rosso, I., Mazloff, M. R., Verdy, A., and Talley, L. D. (2017). Space and time variability of the Southern Ocean carbon budget. J. Geophys. Res. 122, 7407–7432. doi: 10.1002/2016JC012646
Saha, S., Moorthi, S., Pan, H.-L., Wu, X., Wang, J., Nadiga, S., et al. (2010). The NCEP climate forecast system reanalysis. Bull. Amer. Meteor. Soc. 91, 1015–1057. doi: 10.1175/2010BAMS3001.1
Saha, S., Moorthi, S., Wu, X., Wang, J., Nadiga, S., Tripp, P., et al. (2014). The NCEP climate forecast system version 2 journal of climate. J. Clim. 27, 2185–2208. doi: 10.1175/JCLI-D-12-00823.1
Saji, N., Goswami, H. B. N., Vinayachandran, P. N., and Yamagata, T. (1999). A dipole mode in the tropical indian ocean. Nature 401, 360–363. doi: 10.1038/43854
Sakov, P., Counillon, F., Bertino, L., Lister, K. A., Oke, P. R., and Korablev, A. (2012). TOPAZ4 an ocean-sea ice data assimilation system for the North Atlantic and Arctic. Ocean Sci. 8, 633–656. doi: 10.5194/os-8-633-2012
Scaife, A. A., Arribas, A., Blockley, E., Brookshaw, A., Clark, R. T., Dunstone, N., et al. (2014). Skillful long-range prediction of European and North American winters. Geophys. Res. Lett. 41, 2514–2519. doi: 10.1002/2014GL059637
Schepers, D., de Boisséson, E., Eresmaa, R., Lupu, C., and de Rosnay, P. (2018). CERA-SAT: a coupled satellite-era reanalysis. ECMWF Newslett. 155, 32–37. doi: 10.21957/sp619ds74g
Schiller, A., and Oke, P. R. (2015). Dynamics of ocean surface mixed layer variability in the Indian Ocean. J. Geophys. Res. 120, 4162–4186. doi: 10.1002/2014JC010538
Schroeder, I. D., Santora, J. A., Bograd, S. J., Hazen, E. L., Sakuma, K. M., Moore, A. M., et al. (2017). Source water variability as a driver of rockfish recruitment in the California Current ecosystem. Can. J. Fish. Aquat. Sci. 27, R1206–R1208.
Schroeder, I. D., Santora, J. A., Moore, A. M., Edwards, C. A., Fiechter, J., Hazen, E., et al. (2014). Application of a data-assimilative regional ocean modeling system for assessing California Current System ocean conditions, krill, and juvenile rockfish interannual variability. Geophys. Res. Lett. 41, 5942–5950. doi: 10.1002/2014gl061045
Seelanki, V., Sreenivas, P., and Prasad, K. V. S. R. (2018). Impact of Aquarius sea-surface salinity assimilation in improving the ocean analysis over Indian Ocean. Mar. Geodesy 41, 144–158. doi: 10.1080/01490419.2017.1422817
SIPv4 (2017). Strategic Implementation Plan (SIP) for Evolution of NGGPS to a National Unified Modeling System Version 4. Available at: https://www.weather.gov/media/sti/nggps/Presentations%202017/Implementation/SIP-FY18-20- v4.pdf (accessed December 5, 2017).
Skofronick-Jackson, G., Kirschbaum, D., Petersen, W., and Huffman, G. (2018). The global precipitation measurement (GPM) mission’s scientific achievements and societal contributions: reviewing four years of advanced rain and snow observations. Q. J. R. Meteorol. Soc. 144, 27–48. doi: 10.1002/qj.3313
Sluka, T. C. (2018). Strongly Coupled Ocean-Atmosphere Data Assimilation With the Local Ensemble Transform Kalman Filter. Ph.D. Dissertation, University of Maryland, Maryland.
Sluka, T. C., Penny, S. G., Kalnay, E., and Miyoshi, T. (2016). Assimilating atmospheric observations into the ocean using strongly coupled ensemble data assimilation. Geophys. Res. Lett. 43, 752–759. doi: 10.1002/2015GL067238
Smith, D. M., Scaife, A. A., and Kirtman, B. (2012). What is the current state of scientific knowledge with regard to seasonal and decadal forecasting. Environ. Res. Lett. 7:015602. doi: 10.1088/1748-9326/7/1/015602
Song, H., Edwards, C., Moore, A. M., and Fiechter, J. (2016). Data assimilation in a coupled physical- biogeochemical model of the California Current System using an incremental lognormal 4- dimensional variational approach: part 3, Assimilation in a realistic context using satellite and in situ observations. Ocean Model. 106, 159–172. doi: 10.1016/j.ocemod.2016.06.005
Stammer, D., Balmaseda, M., Heimbach, P., Köhl, A., and Weaver, A. (2016). Ocean data assimilation in support of climate applications: status and perspectives. Ann. Rev. Mar. Sci. 8, 491–518. doi: 10.1146/annurev-marine-122414-034113
Stammer, D., Ueyoshi, K., Köhl, A., Large, W. G., Josey, S. A., and Wunsch, C. (2004). Estimating air-sea fluxes of heat, freshwater, and momentum through global ocean data assimilation. J. Geophys. Res. 109, C05023. doi: 10.1029/2003JC002082
Stark, J. D., Donlon, C. J., Martin, M. J., and McCulloch, M. E. (2007). “OSTIA: an operational, high resolution, real time, global sea surface temperature analysis system,” in Proceedings of the Oceans 07 Conference Marine Challenges: Coastline to Deep Sea, (Aberdeen: IEEE).
Stockdale, T. N., Balmaseda, M. A., Johnson, S., Ferranti, L., Molteni, F., and Magnusson, L. (2018). SEAS5 and the Future Evolution of the Long-Range Forecasting System. Reading: ECMWF Technical Memorandum.
Storto, A., and Masina, S. (2016). C-GLORSv5: an improved multipurpose global ocean eddy-permitting physical reanalysis. Earth Syst. Sci. Data 8, 679–696. doi: 10.5194/essd-8-679-2016
Storto, A., Masina, S., and Navarra, A. (2016a). Evaluation of the CMCC eddy7permitting global ocean physical reanalysis system (C7GLORS, 1982–2012) and its assimilation components. Q. J. R. Meteorol. Soc. 142, 738–758. doi: 10.1002/qj.2673
Storto, A., Yang, C., and Masina, S. (2016b). Sensitivity of global ocean heat content from reanalyses to the atmospheric reanalysis forcing: a comparative study. Geophys. Res. Lett. 43, 5261–5270. doi: 10.1002/2016GL068605
Sugiura, N., Awaji, T., Masuda, S., Mochizuki, T., Toyoda, T., Miyama, T., et al. (2008). Development of a four-dimensional variational coupled data assimilation system for enhanced analysis and prediction of seasonal to interannual climate variations. J Geophys. Res. 113:C10017. doi: 10.1029/2008JC004741
Takaya, Y., Bidlot, J. R., Beljaars, A., and Janssen, P. A. (2010). Refinements to a prognostic scheme of skin sea surface temperature. J. Geophys. Res. 115:C06009.
The European Environment Agency [EEA] (2017). State of Play ReportEEA/IDM/15/026/. (LOT1). Available at: https://insitu.copernicus.eu/library/reports/state-of-play-report-observations-december-2017-2 (accessed November 28, 2017).
The IQuOD Team (2018). International Quality Controlled Ocean Database (IQuOD) version 0.1 - aggregated and community quality controlled ocean profile data 1772-2018 (NCEI Accession 0170893). Version 1.1. Silver Spring: NOAA.
Timmermann, A., An, S. I., Kug, J. S., Jin, F. F., Cai, W., Capotondi, A., et al. (2018). El Niño–Southern Oscillation complexity. Nature 559, 535–545.
Toyoda, T., Fujii, Y., Yasuda, T., Usui, N., Ogawa, K., Kuragano, T., et al. (2016). Data assimilation of sea ice concentration into a global ocean-sea ice model with corrections for atmospheric forcing and ocean temperature fields. J. Oceanogr. 72, 235–262. doi: 10.1007/s10872-015-0326-0
Tranchant, B., Remy, E., Greiner, E., and Legalloudec, O. (2018). Data assimilation of SMOS observations into the Mercator Ocean operational system: focus on the Nino 2015 event. Ocean Sci. Discuss. 15, 543–563. doi: 10.5194/os-2018-113
Uotila, P., Goosse, H., Haines, K., Chevallier, M., Barthelemy, A., Bricaud, C., et al. (2018). An assessment of ten ocean reanalyses in the polar regions. Clim. Dyn. 52, 1613–1650. doi: 10.1007/s00382-018-4242-z
Usui, N., Wakamatsu, T., Tanaka, Y., Hirose, N., Toyoda, T., Nishikawa, S., et al. (2017). Four- dimensional variational ocean reanalysis: a 30-year high-resolution dataset in the western North Pacific (FORA-WNP30). J. Oceanogr. 73, 205–233. doi: 10.1007/s10872-016-0398-5
Valdivieso, M., Haines, K., Zuo, H., and Lea, D. (2014). Freshwater and heat transports from global ocean synthesis. J. Geophys. Res. Oceans 119, 394–409. doi: 10.1002/2013JC009357
Verdy, A., and Mazloff, M. R. (2017). A data assimilating model for estimating Southern Ocean biogeochemistry. J. Geophys. Res. 122, 6968–6988. doi: 10.1002/2016JC012650
Vernieres, G., Kovach, R., Keppenne, C., Akella, S., Brucker, L., and Dinnat, E. (2014). The impact of the assimilation of Aquarius sea surface salinity data in the GEOS Ocean Data Assimilation System. J. Geophys. Res. 119, 6974–6987. doi: 10.1002/2014JC010006
Verrier, S., Le Traon, P.-Y., and Remy, E. (2017). Assessing the impact of multiple altimeter missions and Argo in a global eddy-permitting data assimilation system. Ocean Sci. 13, 1077–1092. doi: 10.5194/os-13-1077-2017
Vinogradova, N. T., Lee, T. H., Boutin, J., Drushka, K., Fournier, S., Sabia, R., et al. (2019). Satellite salinity observing system: recent discoveries and the way forward. Front. Mar. Sci. (in press). doi: 10.3389/fmars.2019.00243
Vitart, F., Ardilouze, C., Bonet, A., Brookshaw, A., Chen, M., Codorean, C., et al. (2017). The Subseasonal to Seasonal (S2S) Prediction Project Database. Bull. Amer. Meteor. Soc. 98, 163–173. doi: 10.1175/BAMS-D-16-0017.1
Vitart, F., Balsamo, G., Buizza, R., Ferranti, L., Keeley, S., Magnusson, L., et al. (2014). “Sub-seasonal predictions,” in Paper Presented ECMWF Technical Memorandum, Special Topic Paper on Sub- Seasonal Predictions Presented at the 43rd ECMWF Scientific Advisory Committee, Reading.
Vitart, F., and Robertson, A., and the S2S steering group (2015). Sub-Seasonal to Seasonal Prediction: Linking Weather and Climate. Seamless Prediction of the Earth System: From Minutes to Months. Geneva: WMO.
Vitart, F., and Robertson, A. W. (2018). The sub-seasonal to seasonal prediction project (S2S) and the prediction of extreme events. Clim. Atmos. Sci. 1:3. doi: 10.3390/ijerph13020206
Volz, S., Maier, M., and Di Pietro, D. (2016). “The NOAA Satellite Observing System Architecture study,” in Proceedings of the 2016 IEEE International Geoscience and Remote Sensing Symposium (IGARSS), Beijing.
Waliser, D. E. (2011). in Predictability and Forecasting. Intraseasonal Variability of the Atmosphere-Ocean Climate System, eds W. K. M. Lau and D. E. Waliser (Heidelberg: Springer).
Wang, C. (2005). “ENSO, Atlantic climate variability, and the Walker and Hadley circulations,” in The Hadley Circulation: Present, Past, and Future, eds H. F. Diaz and R. S. Bradley (Dordrecht: Kluwer Academic Publishers), 173–202. doi: 10.1007/978-1-4020-2944-8_7
Waters, J., Bell, M. J., Martin, M. J., and Lea, D. J. (2017). Reducing ocean model imbalances in the equatorial region caused by data assimilation. Q. J. R. Meteorol. Soc. 143, 195–208. doi: 10.1002/qj.2912
Webster, P. J., Moore, A. M., Loschnigg, J. P., and Leben, R. P. (1999). Coupled ocean–atmosphere dynamics in the Indian Ocean during 1997–98. Lett. Nat. 401, 356–360. doi: 10.1038/43848
Woolnough, S. J., Vitart, F., and Balmaseda, M. A. (2007). The role of the ocean in the Madden-Julian oscillation: implications for the MJO prediction. Q. J. R. Meteorol. Soc. 133, 117–128. doi: 10.1002/qj.4
Wu, R., Kirtman, B. P., and van den Dool, H. (2009). An analysis of ENSO prediction skill in the CFS retrospective forecasts. J. Clim. 22, 1801–1818. doi: 10.1175/2008JCLI2565.1
Wunsch, C., and Heimbach, P. (2013). “Dynamically and kinematically consistent global ocean circulation and ice state estimates,” in Ocean Circulation and Climate - A 21st Century Perspective”, International Geophysics Series, Vol. 103, eds G. Sielder, J. Church, S. Griffes, J. Gould, and J. Church (Cambridge: Academic Press).
Wunsch, C., and Heimbach, P. (2014). Bidecadal thermal changes in the Abyssal Ocean. J. Phys. Oceanogr. 44, 2013–2030. doi: 10.1175/JPO-D-13-096.1
Xue, Y., Huang, B., Hu, Z. Z., Kumar, A., Wen, C., Behringer, D., et al. (2011). An assessment of oceanic variability in the NCEP climate forecast system reanalysis. Clim. Dyn. 37, 2511–2539. doi: 10.1007/s00382-010-0954-4
Xue, Y., Wen, C., Kumar, A., Balmaseda, M., Fujii, Y., Alves, O., et al. (2017). A real-time ocean reanalyses intercomparison project in the context of tropical pacific observing system and ENSO monitoring. Clim. Dyn. 49, 3647–3672. doi: 10.1007/s00382-017-3535-y
Yang, C., Masina, S., and Storto, A. (2017). Historical ocean reanalyses (1900–2010) using different data assimilation strategies. Q. J. R. Meteorol. Soc. 143, 479–493. doi: 10.1002/qj.2936
Yang, C., Storto, A., and Masina, S. (2018). Quantifying the effects of observational constraints and uncertainty in atmospheric forcing on historical ocean reanalyses. Clim. Dyn. 52, 3321–3342. doi: 10.1007/s00382-018-4331-z
Yu, L. (2019). Global air-sea fluxes of heat fresh water, and momentum: energy budget closure and unanswered questions. Ann. Rev. Mar. Sci. 11, 227–248. doi: 10.1146/annurev-marine-010816-060704
Zampieri, L., Goessling, H. F., and Jung, T. (2018). Bright prospects for Arctic sea ice prediction on subseasonal time scales. Geophys. Res. Lett. 45, 9731–9738. doi: 10.1029/2018GL079394
Zhu, J., Huang, B., Zhang, R.-H., Hu, Z.-Z., Kumar, A., Balmaseda, M. A., et al. (2014). Salinity anomaly as a trigger for ENSO events. Nat. Sci. Rep. 4:6821. doi: 10.1038/srep06821
Zhu, Y., Zhou, X., Li, W., Hou, D., Melhauser, C., Sinsky, E., et al. (2018). Toward the improvement of subseasonal prediction in the National Centers for environmental prediction global ensemble forecast system. J. Geophys. Res. 123, 6732–6745. doi: 10.1029/2018JD028506
Zilberman, N. V. (2017). Deep Argo: sampling the total ocean volume in state of the climate in 2016. Bull. Am. Meteorol. Soc. 98, S73–S74. doi: 10.1175/2017BAMSStateoftheClimate.1
Zuo, H., Balmaseda, M. A., Boisseson, E., and Hirahara, S. (2017a). A New Ensemble Generation Scheme for Ocean Reanalysis. Reading: ECMWF.
Zuo, H., Balmaseda, M. A., and Mogensen, K. (2017b). The new eddy-permitting ORAP5 ocean reanalysis: description, evaluation and uncertainties in climate signals. Clim. Dyn. 49, 791–811. doi: 10.1007/s00382-015-2675-1
Keywords: data assimilation, reanalysis, coupled data assimilation, S2S prediction, decadal prediction, ocean observation network, ocean data assimilation, ocean reanalysis
Citation: Penny SG, Akella S, Balmaseda MA, Browne P, Carton JA, Chevallier M, Counillon F, Domingues C, Frolov S, Heimbach P, Hogan P, Hoteit I, Iovino D, Laloyaux P, Martin MJ, Masina S, Moore AM, de Rosnay P, Schepers D, Sloyan BM, Storto A, Subramanian A, Nam S, Vitart F, Yang C, Fujii Y, Zuo H, O’Kane T, Sandery P, Moore T and Chapman CC (2019) Observational Needs for Improving Ocean and Coupled Reanalysis, S2S Prediction, and Decadal Prediction. Front. Mar. Sci. 6:391. doi: 10.3389/fmars.2019.00391
Received: 01 November 2018; Accepted: 24 June 2019;
Published: 11 July 2019.
Edited by:
Sabrina Speich, École Normale Supérieure, FranceReviewed by:
Feiyu Lu, Princeton University, United StatesDimitris Menemenlis, NASA Jet Propulsion Laboratory (JPL), United States
Copyright © 2019 Penny, Akella, Balmaseda, Browne, Carton, Chevallier, Counillon, Domingues, Frolov, Heimbach, Hogan, Hoteit, Iovino, Laloyaux, Martin, Masina, Moore, de Rosnay, Schepers, Sloyan, Storto, Subramanian, Nam, Vitart, Yang, Fujii, Zuo, O’Kane, Sandery, Moore and Chapman. This is an open-access article distributed under the terms of the Creative Commons Attribution License (CC BY). The use, distribution or reproduction in other forums is permitted, provided the original author(s) and the copyright owner(s) are credited and that the original publication in this journal is cited, in accordance with accepted academic practice. No use, distribution or reproduction is permitted which does not comply with these terms.
*Correspondence: Stephen G. Penny, U3RldmUuUGVubnlAbm9hYS5nb3Y=