- 1Department of Pharmacodynamics and Molecular Pharmacology, Faculty of Pharmacy, Collegium Medicum in Bydgoszcz, Nicolaus Copernicus University in Toruń, Toruń, Poland
- 2Department of Chemistry, University of Waterloo, Waterloo, ON, Canada
- 3Department of Chemistry, Middle East Technical University, Ankara, Turkey
- 4Laboratory of Aquatic Chemistry, School of Environmental Engineering, Technical University of Crete, Chania, Greece
- 5Institute of Marine Biology, Biotechnology and Aquaculture, Hellenic Centre for Marine Research, Heraklion, Greece
In marine ecosystems, sponges are ubiquitous sessile organisms that contain a wide range of specialized metabolites. These metabolites point to a diverse range of biochemical pathways. Some of these compounds are biomarkers that indicate the presence of bacteria that form symbiotic relationships with a host sponge (microsymbionts). Sponges hold considerable promise as bioindicators for seawater quality monitoring, as they are exposed to, and accumulate, significant levels of anthropogenic contamination in coastal areas. Solid-phase microextraction (SPME) is a low-invasive and non-exhaustive technique that combines sampling and extraction into a single step and offers the added benefit of biocompatible extraction phases. We used different types of SPME devices to extract exometabolites from sponges (genus: Sarcotragus) in situ. Following extraction, the samples were analyzed via GC- and LC-MS in order to verify the presence of compounds associated with quorum sensing, as well as to examine the metabolism of organic pollutants, such as monocyclic aromatic hydrocarbons (MAHs), polycyclic aromatic hydrocarbons (PAHs), pesticides, and other bioactive compounds in an untargeted format. As the results demonstrate, when the extracted metabolites are compared with the background controls, SPME offers a non-exhaustive approach that can be used in the field to discover novel metabolites deriving from complex holobionts such as marine sponges.
Introduction
Sponges are sessile filter feeders that are capable of turning over liters of water per day (Hentschel et al., 2006). They predominately feed on prokaryotic microorganisms, picoeukaryotes, nanoeukaryotes, and phytoplankton, with prokaryotes comprising the largest portion of their natural diet (Ribes et al., 1999). As water passes through the sponge, amoeboid archaeocytes in the sponge’s internal matrix capture and phagocytose the particulate organic matter. These sponge cells are highly efficient in the uptake of microorganisms, though they are generally perceived as not being very selective (Reiswig, 1971; Ribes et al., 1999). In addition to constituting an important part of a sponge’s diet, prokaryotic microorganisms are also found in their mesohyl matrix (Webster et al., 2001; Montalvo et al., 2005). These bacteria develop a symbiotic relationship with the sponge host, forming an ecological unit known as a “sponge holobiont” (Oulhen et al., 2016; Pita et al., 2018). The bacteria in these sponge holobionts can constitute of 40–60% of a sponge’s total biomass, their concentration exceeding that of the surrounding seawater by two to three orders of magnitude (Friedrich et al., 2001; Webster et al., 2001). The symbiotic bacteria can consist of host-specific bacteria from the phyla Acidobacteria, Chloroflexi, Actinobacteria, Poribacteria, and Alpha-, Gamma-, and Deltaproteobacteria (Wehrl et al., 2007). Along with these bacteria, archaea, unicellular algae, and facultative anaerobes also form symbiotic relationships with the sponge host (Taylor et al., 2007).
Sarcotragus foetidus (Porifera: Demospongiae) is a sponge species frequently found in shallow-water habitats in the Eastern Mediterranean Sea and the Aegean Sea (Pansini et al., 2000; Demir and Okus, 2007; Pavloudi et al., 2016; Gerovasileiou et al., 2018), including the vicinity of shallow hydrothermal vents (Pansini et al., 2000). This sponge species is known to host a number of symbiotic bacteria, such as heterotrophic bacteria and cyanobacteria (Konstantinou et al., 2018), as well as various species of fungal Penicillium, Cladosporium, and Fusarium (Altunok et al., 2015). A recent study found that three Mediterranean sponge species, Petrosia ficiformis, S. foetidus, and Aplysina aerophoba, contained higher levels of Guanine–Cytosine (GC) in their metagenome – including in the genome of the symbiont microorganisms – than were detected in the surrounding seawater’s metagenome (Horn et al., 2016). This finding indicates that the diversity of bacterial species and the composition of the microbiome within these sponges is distinct from that of the surrounding seawater. A detailed analysis of these symbionts’ defense systems against foreign genetic material, such as those related to viral defense, revealed that the sponge microbiome features enhanced Clustered Regularly Interspaced Short Palindromic Repeats (CRISPR) and Restriction Modification Systems (RMS) (Horn et al., 2016). These findings suggest that sponge microbiomes form defense systems against foreign DNA as a by-product of circulating water to maintain the symbiotic relationship between bacteria and the sponge host.
The diverse microbiota associated with sponges have significant importance in the host’s physiology and environmental chemistry due to their metabolic diversity. The microorganisms provide the host with secondary metabolites of important bioactivity, such as drugs that can overcome bacterial diseases to degradation metabolites of marine pollutants. The use of untargeted metabolomics profiling can provide insight into the complex metabolic variability within sponges and promote research toward the development of novel bioactive compounds with potential for pharmaceutical and industrial applications (Bauvais et al., 2017; Sauleau et al., 2017). In addition, this approach could complement traditional morphological and genetic approaches to classifying taxa (i.e., chemotaxonomy) (Ivanišević et al., 2011), as well as analyzing the sponge’s physiological state and its response to environmental stressors or fluctuations (Cachet et al., 2015; Reverter et al., 2018). Metabolomics studies on marine organisms have been primarily based on the direct extraction of metabolites using solvents, sampling, and solid-phase extraction (SPE), or by sampling the microorganism and then extracting bioactive metabolites in vitro. However, neither of these sample-preparation methods is optimal when it comes to sampling and extracting short-lived metabolites, as they require a metabolism quenching step (e.g., snap-freezing with liquid nitrogen) or the use of hot or cold organic solvents in order to completely quench metabolism. Moreover, these approaches cannot be applied immediately after sampling underwater, as this would lead to chemical modification or the dilution of the metabolites (Bojko et al., 2011).
The degradation of marine pollutants and their metabolites is particularly important, as some of the bacterial species that form symbiotic relationships with a sponge host are also known to metabolize marine pollutants. For instance, some bacteria can be evolved to be capable of metabolizing polycyclic aromatic hydrocarbons (PAHs) and monocyclic aromatic hydrocarbons (MAHs), two pollutants that are ubiquitous throughout the sea, into water-soluble products with smaller molecular weights. Oligotrophic bacteria have been discovered in certain sponge species (Arellano et al., 2013; Rubin-Blum et al., 2017), for example, bacteria belonging to the Cycloclasticus genus (Proteobacteria phylum), which are obligate marine PAH degraders that use PAHs as carbon and energy sources (Wang et al., 2018). This metabolic degradation also reduces the presence of PAHs in higher members of the food chain that utilize bacteria.
The feeding habits of sponges involve high filtration rates and the ability to ingest particles with sizes <50 μm, as well as the ability to capture pollutants efficiently, both in the dissolved and particulate phases. Past studies have shown that sponges effectively accumulate organic sea pollutants like PAHs, and can therefore be used as bioindicators for monitoring environmental stresses in marine settings. Indeed, symbiotic bacteria within the sponge holobiont are exposed to a larger amount of PAHs than other free-floating seawater bacteria; this makes them highly important microorganisms for the study of metabolomics pathways and metabolic products, as they can potentially provide new insights into the bioremediation of PAHs by marine bacteria (Space Scents, 2002; Demir and Okus, 2007; Kim et al., 2008). Hitherto, the study of metabolites and marine pollutants in sponges has been carried out by removing sections of the sponges and transferring them to the laboratory, where they are submitted to a multi-step treatment procedure before analysis. The development of a minimally invasive in situ and in vivo extraction method is of great interest, as it would eliminate the need to remove marine organisms from their habitat, and furthermore allow the elusive portion of the metabolome to be captured during its interactions with the living and non-living components of its ecosystem. This would be especially important when the analytical study of endangered marine species is aimed.
Solid-phase microextraction (SPME) simplifies the analysis of metabolites by combining sampling and extraction in one step and allowing for in situ sampling. In addition, SPME enables short-lived metabolites to be extracted on site in a non-exhaustive and low-invasive way (Pawliszyn, 2012a,b) while providing a balanced metabolite coverage through allowing both polar and non-polar metabolites get extracted efficiently (Bojko et al., 2014). Furthermore, SPME devices can be coated with biocompatible extraction phases specifically designed to restrict proteins, especially enzymes, which provides an additional advantage for metabolomics studies. Since enzymes are not extracted by the polymer coating, the metabolites will not be prone to quenching and chemical modifications during and after sampling (Bojko et al., 2011). A detailed review on the merits of the SPME technique in metabolomics studies has been published recently (Reyes-Garcés et al., 2018). In the present study, we test three different SPME devices for their ability to perform in situ analyte extraction on S. foetidus specimens. The sample analysis revealed the presence of a wide range of metabolites related to quorum sensing, mono- and polycyclic aromatic hydrocarbon degradation, and photosynthetic organisms, as well as a number of marine pollutants.
Materials and Equipment
The primary extraction phase used for the SPME fibers was a mixed-mode coating consisting of C18 and benzene sulfonic acid. The fibers were kindly provided by Supelco. ∼30 μm HLB (hydrophilic lipophilic balance) particles were purchased from Waters® (Milford, MA, United States). Divinylbenzene (DVB) and polyacrylonitrile (PAN) was purchased from Sigma-Aldrich (St. Louis, MO, United States). Polydimethyl siloxane (PDMS) was purchased from Dow (Midland, MI, United States). All solvents were purchased from Sigma-Aldrich (St. Louis, MO, United States).
For LC-MS analysis, A ThermoAcella autosampler-HPLC and an Exactive Orbitrap MS (Thermo Fisher Scientific, San Jose, CA, United States) were used to separate and detect the untargeted analytes, while chromatographic separations were performed using a Supelco Discovery pentafluorophenyl (PFP) HS F5 column (2.1 mm × 100 mm, 3 μm) (Supelco, Millipore-Sigma, Bellefonte, PA, United States).
For GC-MS analysis, Agilent 6890 gas chromatograph (GC) and a 5973 quadrupole mass spectrometer (MS). Chromatographic separation was performed by 30 m, HP-5 5% phenyl–methyl siloxane column (Agilent 19091J-433) with an I.D and thickness of 0.25 mm (Agilent Technologies Inc., Santa Clara, CA, United States).
Methods
Preparation of Thin Film Membranes, Blades, and Fibers
The TFME blades were prepared by using a flask-type sprayer to apply a slurry consisting of ∼30 μm HLB particles dispersed in PAN onto stainless-steel blades. This process has previously been described by Mirnaghi et al. (2011).
The DVB-PDMS coating was prepared using the methodology previously described by Jiang and Pawlisyzn (Jiang and Pawliszyn, 2014). Briefly, the DVB-PDMS membranes were prepared by dispersing 0.2 g of DVB particles in 10 mL of hexane followed by vortex agitation. Next, 1.0 g of PDMS elastomer was added to the mixture, which was then subjected to sonication. In order to initiate crosslinking and condensation in the PDMS, 0.1 g of curing reagent of a catalyst was added to the mixture. Finally, the excess hexane was evaporated under N2 gas. Once the excess hexane had been removed, a thin layer of the final mixture was applied to the blades using the bar-coating method, and the crosslinking process was initiated and accelerated via thermal curing (as described in the aforementioned reference) (Jiang and Pawliszyn, 2014). Representative images for blades, membranes, and fibers are displayed in Supplementary Figure S1.
In situ SPME
The selected species, S. foetidus, is a ubiquitous sponge in Eastern Mediterranean coastal habitats. Its shape approximates a globular form and its body volume can often exceed 5 l. Its surface hosts an array of evenly distributed exhalant pores (oscula) which are well-defined, their openings reaching a diameter of 5 mm.
Sampled sponges were located in Souda Bay, which is the largest bay in the island of Crete, located on its northwest coast. The bay’s geographical orientation protects it from the cyclonic and anticyclonic eddies that characterize the island’s nearby seawater circulation patterns. The bay is home to a large civilian port that features a daily ferryboat service to and from Piraeus, and it also occasionally hosts large cruise ships. Souda Bay is also the site of the Crete Naval Base, which serves as a major naval base for the Hellenic Navy and NATO. In situ SPME was carried out via SCUBA diving at depths ranging from 2.4 to 3.3 m at a site in Souda Bay close to Marathi beach (35.5034°N/E24.1709°E) featuring natural populations of S. foetidus. Three sufficiently sized sponges separated by several meters of distance were selected as replicates for the experiment. Extractions were performed on different parts of each sponge’s body using three different SPME devices (membranes, blades, and fibers) in order to test each geometry’s relative efficacy for producing extraction profiles of various analytes. Figure 1 shows a Sarcotragus sponge during extraction using three different SPME devices. For the extraction protocols, the devices were separated into two groups: the “experimental group” and the “control group.” The experimental group extractions were performed by suspending the membranes several millimeters above exhalant pores (oscula) on the sponge’s surface. For extractions using blades, the devices were partially (approximately 2–4 mm) inserted into the openings of larger exhalant canals. Sampling via fibers was conducted by puncturing the tissue in order to penetrate into the mesohyl. Care was taken during this step to ensure that the coated surface was not sampling hollow cavities, such as the canals or subdermal lacunae characteristic of Irciniid sponges. For the “control group” extractions, replicates of each SPME device (membranes, needles and fibers) were left to float adjacent to the sea bottom several meters away from the sponges and any other macroorganism. Two to four replicates of each type of SPME device were used for each sponge in the “experimental group,” as well as in the “control group.” The extraction duration for each sample was 420 ± 60 s. Table 1 provides the codes for the individual SPME devices along with the depth of the individual sponges.
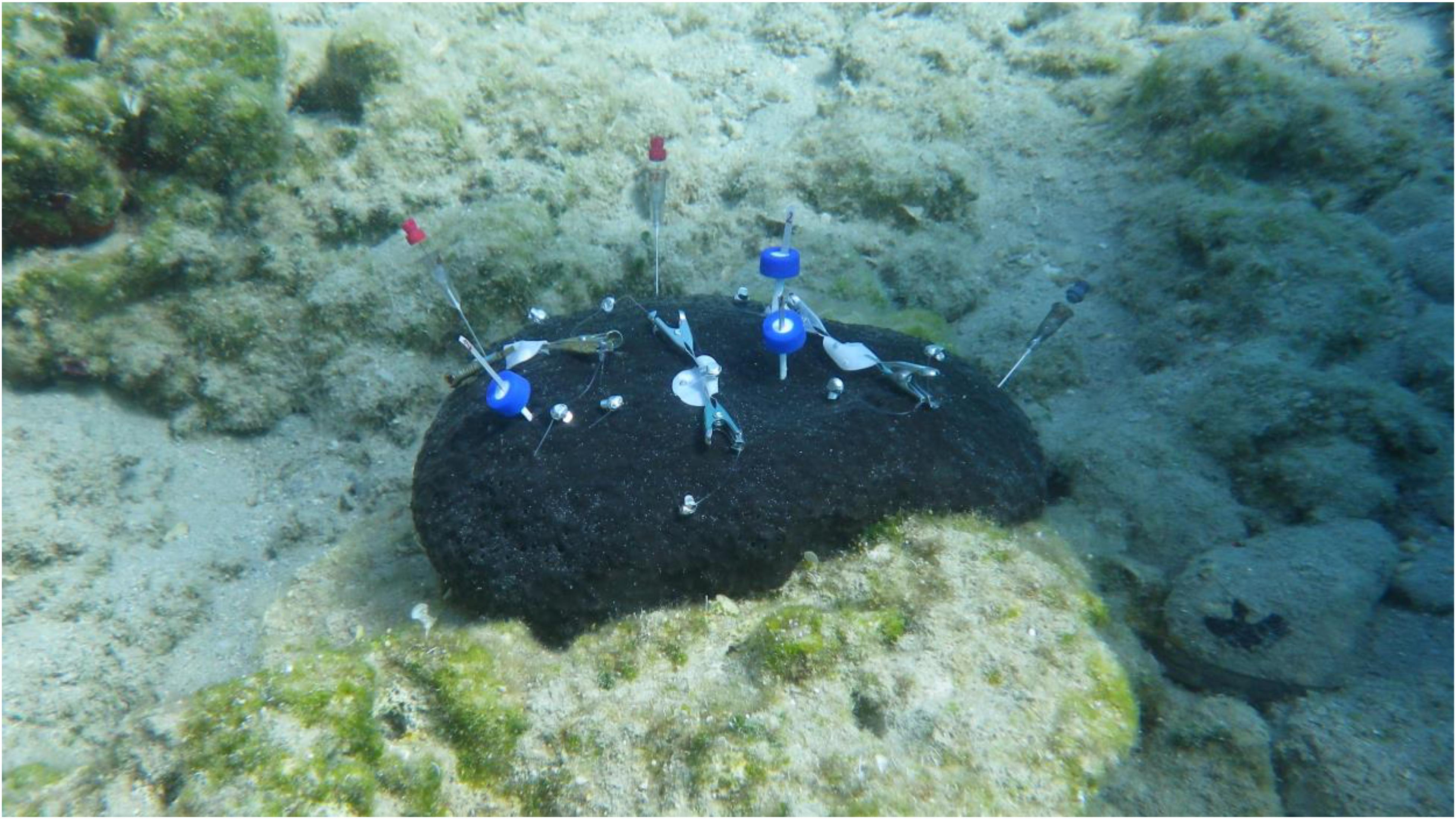
Figure 1. Individual Sarcotragus foetidus during in situ extraction with membrane, blade, and fiber SPME devices.
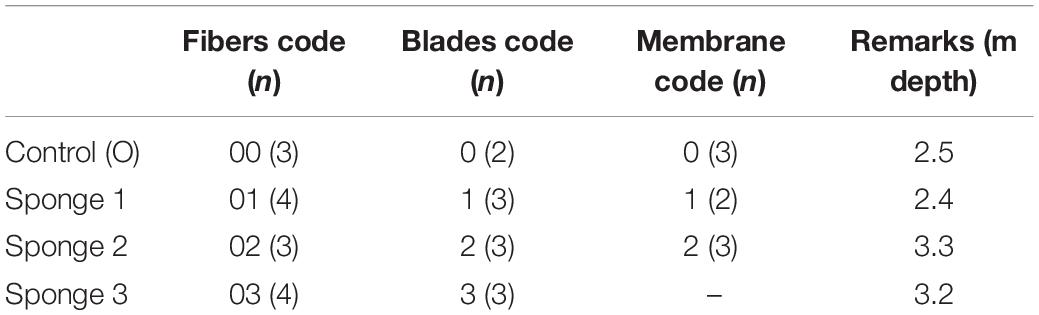
Table 1. Extraction durations and number of installed SPME devices (fibers, blades, and membranes) for each of the three replicate Sarcotragus foetidus specimens and the control group.
After the sampling, the SPME devices were stored in vials in dry ice and delivered to the laboratory in University of Waterloo. The membranes were cut into half to be desorbed for LC-MS and GC-MS analysis separately. For LC-MS experimental protocols, all devices were rinsed with HPLC-grade water for 10 s and then immersed into 300 μL of 80:20 acetonitrile (ACN):H2O for the desorption of the analytes. The desorption was carried out through 1000 rpm benchtop vortexing for 1 h. A scheme that represents this protocol is given in Supplementary Figure S2.
Analysis of Extracts via Mass Spectrometry
LC-MS Analysis
The LC-MS conditions were such that the mobile phase was pumped at a flow rate of 5 μL sec–1, and gradient elution was performed using a 2 component system consisting of Mobile Phase A, which consisted of a water: formic acid mixture (99.9:0.1, v:v), and mobile phase B, which consisted of a acetonitrile: formic acid mixture (99.9:0.1, v:v). The initial mobile phase conditions for the PFP column separation were 100% A from 0 to 180.0 s and decreased along a linear gradient to 10% A from 180 to 1500 s. After 1500 s, the mobile phase was placed on an isocratic hold at 10% A until 2040 s. The total run time was 2400 s per sample, including a 360 s column re-equilibration period.
Analysis was performed using positive electrospray ionization (ESI) via the PFP-HPLC method described above. The injection volume for all methods was 10 μL, and samples were stored at 4°C on the autosampler while awaiting injection. All samples were run in randomized order, and both instrument QCs and pooled QCs were run periodically to verify instrument performance. MS acquisition was performed with AGC = balanced (1 000 000 ions) and a 50 000 resolution at 2 Hz. The injection time onto the C-trap was 100 ms. Sheath gas (arbitrary units), auxiliary gas (arbitrary units), sweep gas (arbitrary units), ESI voltage (kV), capillary temperature (°C), and vaporizer temperature (°C) were (i) 30, 10, 5, 4.0 (−2.9 negative mode), 300, and 300, respectively, with an acquisition range of 100–1000 m/z for the positive and negative ESI reversed-phase methods. External instrument mass calibration was performed every 48 h to ensure that it was within 2 ppm for all ions.
GC-MS Analysis
Before GC-MS analysis, the DVB–PDMS membranes were cut into two equal parts. One half of the membrane was desorbed in solvent and subjected to LC-HRMS, while the other half was subjected to GC-MS using an Agilent 6890 gas chromatograph (GC) and a 5973 quadrupole mass spectrometer (MS). For GC-MS, the membrane was inserted into the thermal desorption unit (TDU) liner, where the analytes were thermally desorbed into GC by via TDU coupled to a cooled injection system (CIS) (GERSTEL GmbH, Mullheim, Germany). In this case, the CIS served three functions: first, as an interface between the column inlet and the TDU; second, as a cryo-focusing trap; and third, as a temperature-programed GC inlet.
Desorption of analytes from the membrane was performed with the TDU in splitless mode and the CIS in solvent vent mode, with a TDU transfer-line temperature of 300°C. Upon introducing a membrane into the TDU, the temperature was increased from 30 to 250°C at a rate of 1°C sec–1; the TDU was then held at 250°C for 180 s. in order to ensure that all of the extracted compounds were completely desorbed. As the TDU temperature increased, the analytes were desorbed from the membrane and cryo-focused in the CIS injector, which was maintained at −100°C during TDU desorption. The temperature was then increased to 280°C at a rate of 12°C s–1, where it was held for 600 s. After desorption, the membrane was retained at 220°C in the injector until the end of the chromatographic run.
Helium was used as carrier gas at a flow rate of 16.66 μL sec–1 in the GC column. The initial column temperature was set to 40°C and held for 1 min before being increased to 160°C at a rate of 0.08°C sec–1. Next, the column temperature was further increased to 200°C at a rate of 0.05°C sec–1 and then raised again to 250°C, where it was kept for 600 s. Finally, the temperature was increased to 300°C at 0.17°C sec–1. The MSD transfer-line temperature was set at 300°C, while the MS Quad and MS source temperatures were set at 150 and 230°C, respectively. The MS system was operated in electron ionization (EI) mode under 70 eV of ionization voltage, with ions being collected in the m/z 35–400 range.
Data Processing, Statistical Analysis, and Metabolite Identification
The LC-MS data was processed by first converting the raw data files to an mzXML format using the free Proteowizard software, MSconvert (Chambers et al., 2012). The software parameters were set as follows: mz level = 1 filter, 64-bit binary-encoding precision, and write index option. The converted files were then imported to MZmine 2 software for furthering peak filtering and detection (Olivon et al., 2017). After being imported, scan-by-scan filtering was performed on the data using a 5 data point Savitzky–Golay filter. A mass peak list was then generated using exact mass detection with an m/z range of 99–1000 m/z and a mass tolerance of 5.0 ppm. Once the mass list had been generated, chromatogram builder was used with a minimum peak height of 10 000 (arbitrary) and a minimum width of 1 s. The rebuilt chromatograms were then deconvoluted using a Savitzky–Golay filter with a minimum peak height of 10 000 (arbitrary) and peak-width setting of 1–60.0 s. The generated peak list was then filtered using an m/z-range of 99–1000, a retention time (RT) range of 48–2100 s, and peak width of 1–60 s. A compiled aligned peak-list table was then generated using 5 ppm, mass tolerance, 5% retention time tolerance, and weighting values of 10 and 20 (arbitrary) for RT and m/z, respectively. Finally, once the peaks associated with the instrument and sampler blanks had been manually removed, the peak list rows filter was again used on the compiled aligned peak list, only this time with the minimum peaks per row set to 3. This secondary filtering removed erroneous single detections, which was considered statistically prudent as there were always 5–6 replicates per sample. The processed-aligned peak list was then exported as a.csv file, which was then imported into SIMCA-14 multivariate data processing software. Principal component analysis (PCA) was then performed using Pareto scaling in order to determine significant features and to test the data fit for the various samples run. Partial least squares-discriminant analysis (PLS-DA) and orthogonal partial least squares-discriminant analysis (OPLS-DA) were further performed to show the effects of supervised group separation.
Next, additional statistical analysis was carried out using XCMS (Tautenhahn et al., 2012) to identify features that significantly differed between the experimental groups (i.e., each SPME device) and their corresponding control groups. m/z peaks with very low intensity and abnormal shapes were discarded. ANOVA was then used to conduct statistical significance tests on the data obtained from the experimental and control groups.
Chromatographic separation and tandem MS analysis were carried out on the QCs of each experimental sample and a single mixture of standard compounds (100 ppb each) in order to obtain information about the fragment patterns of the significantly different features of the two groups. This analysis was conducted using a high-resolution Exactive Thermo Orbitrap and an Q-Exactive Thermo Orbitrap MS (Thermo Fisher Scientific, San Jose, CA, United States) with the same column, methodology, and mobile phases described above. MS acquisition was performed through parallel reaction monitoring (PRM), with a resolution of 70 000, an isolation window of 1.6 m/z, normalized collision energies (dimensionless) of 20 and 40 eV, AGC = balanced (1 000 000 ions) at 2 Hz, and a C-trap injection time of 100 ms. The positive ESI reversed-phase methodology employed an acquisition range of 70–1000 m/z, and sheath gas (arbitrary units), auxiliary gas (arbitrary units), sweep gas (arbitrary units), capillary temperature (°C), and vaporizer temperature (°C) values of (i) 35, 5, 300, and 280, respectively. External instrument mass calibration was performed every 48 h to ensure it remained within 2 ppm for all ions. The PRM was carried out on a list of MS1 m/z values that corresponded to all m/z values of VIP > 1, which were determined by the SIMCA software.
To predict compounds through their fragmentation patterns, the fragmentation spectra (MS/MS, or MS2) corresponding to each parent ion’s (MS1) m/z on target-retention time points were exported as.prm files from MZmine. The exported.prm files were then imported to Sirius 4.0.1 software, which had been configured to mirror the settings for ESI mode and MS2 (20 and 40 eV, respectively). The computation of each feature was performed by Sirius with the settings, Orbitrap, 5 ppm, 10 candidates, and all PubChem formulas. The software’s predictions were only considered if at least three possible peaks were generated. Next, a CSI:FingerID search (Dührkop et al., 2015) was performed, and the results were compared with the MS1 METLIN annotations. Following this, the four highest peaks on the MS/MS spectra were input into the Fragment Similarity function of the METLIN database (Smith et al., 2005) in order to supplement the fragmentation pathway analysis. METLIN database fragment ion predictions that matched to the compound groups predicted by MS1 were chosen as our final annotation. If the METLIN database could not produce a sufficient fragment prediction, the molecular formula predicted by the Sirius software was used as our final annotation (Böcker et al., 2009; Dührkop and Böcker, 2015; Dührkop et al., 2015). Standards of compounds were assessed by comparing their fragmentation patterns to their specific retention time points. If the fragmentation patterns matched the time points, the compound was considered verified, and the spectra was not further analyzed through METLIN or Sirius. As can be seen in the Supporting Information, M denotes compounds identified by METLIN, and S denotes compounds identified by Sirius. Identified compounds were further searched on the KEGG database to further determine the biochemical pathways they are involved in Kanehisa (2006) and Kanehisa et al. (2017).
XCMS software was used to prepare the fold change in the peak areas of the experimental and control groups. Specifically, the average peak area of the experimental group’s m/z values was divided by the average peak area of the control group’s m/z values, with the resultant figure then being used to prepare a heat map. As seen in Supplementary Table S1, areas with higher intensities of red correspond to the induction of a compound, while areas with varying intensities of green correspond to the suppression of the compound (Supplementary Table S1).
As part of GC-MS analysis, the peaks in the chromatograms were identified and compared, particularly those peaks that were unique to each experimental group. The analytes associated with these unique peaks were then singled out and searched within the NIST database (Linstrom and Mallard, 2014). Supplementary Table S2 provides the percentage predictions for the top three identified compounds.
Results and Discussion
Three distinct SPME geometries were applied to different sections of sponges in situ and in vivo in order to test their ability to extract metabolites. Specifically (i) membranes were used to sample the seawater in close proximity to the sponge’s surface and its exhalant openings, where metabolites could be released into the environment; (ii) blades were used to sample water released by the sponge, which is rich in metabolic products; and (iii) fibers were used to sample the internal parts of the sponge’s undifferentiated mesohyl matrix. A schematic representation of the sponge and SPME devices is given in Figure 2.
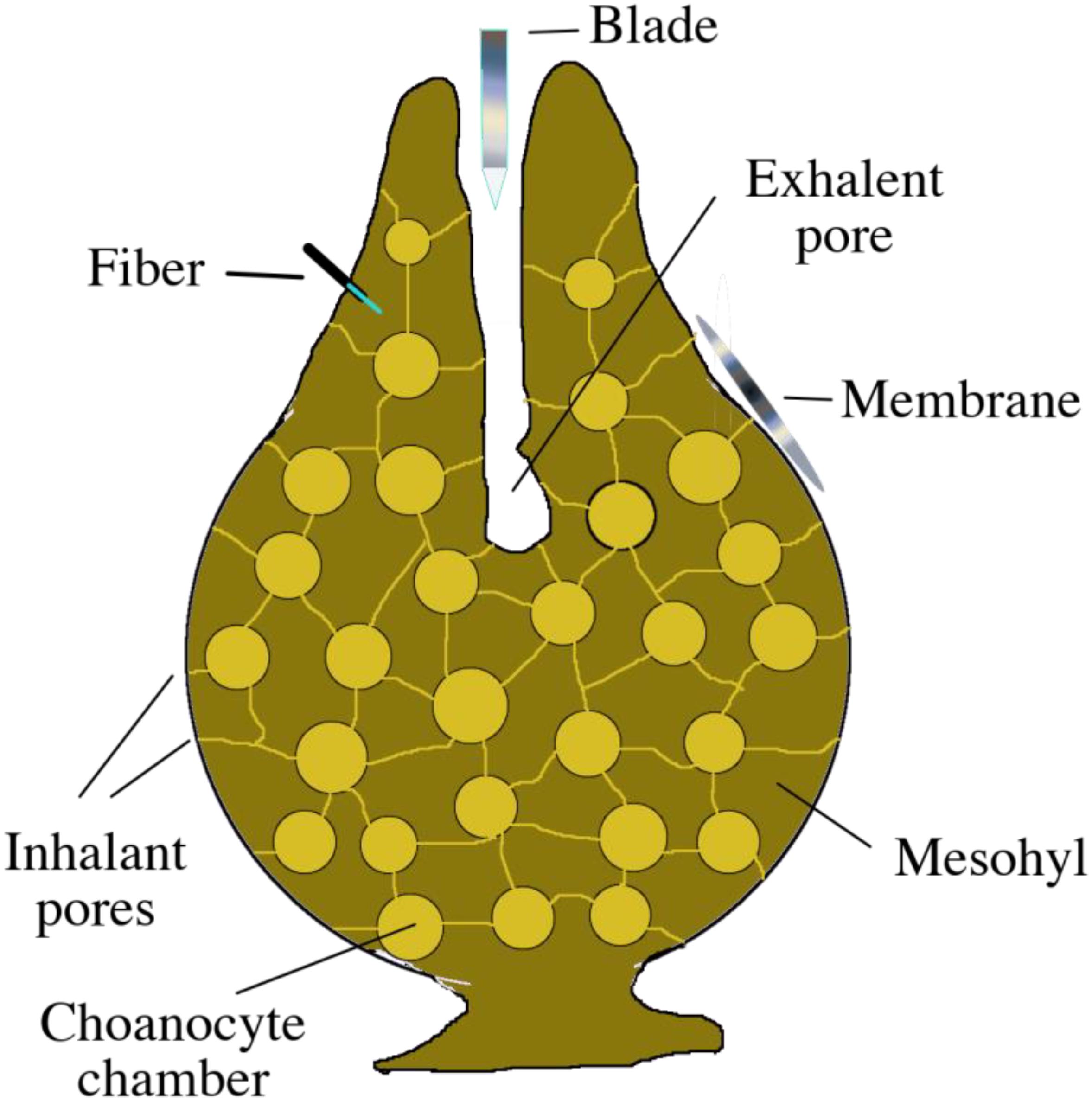
Figure 2. Basic schematic of a sponge’s anatomy, displaying the placement of the three different types of SPME devices during in situ extraction.
The results of our LC-MS runs were analyzed through SIMCA, with the results being used to plot PCA graphs. As the PCA graph in Figure 3 shows, while each SPME device had a different extraction profile, the replicates tended to be tightly clustered, which indicates good inter-device reproducibility. Furthermore, given the differences in surface area, solvent volumes used for desorption, and placement of the SPME devices, variations between the devices was to be expected. However, a secondary explanation for the variation in recovery rates is based on differences in the polymeric materials that constitute the thin-film coating, or the volume of extraction phase applied to each device.
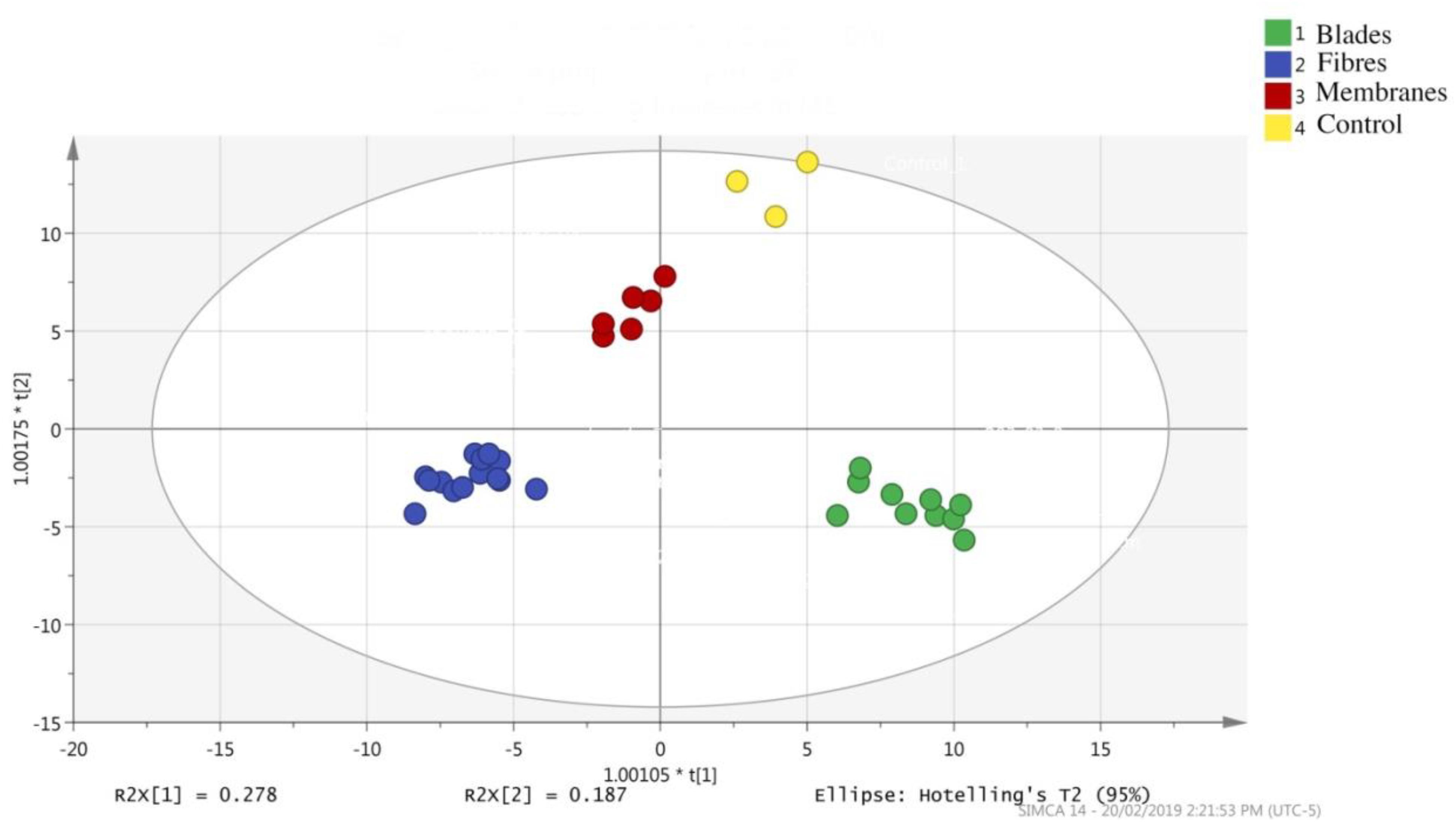
Figure 3. Principal component analysis plot showing the separation of the features obtained by three different SPME devices: blades, fibers, and membranes, as well as the control (water sample).
Solid-phase microextraction is a flexible technology that can be tailored to suit the application while still providing high sensitivity. For instance, SPME fibers are minimally invasive, which makes them ideal for sampling living tissue, as they do not harm the organism being sampled, in this case, a sponge. Similarly, thin-film (TF) devices can be used in a relatively low-invasive manner while still providing highly sensitive results; in this research, TF devices inserted into the inhalant pore enabled high-sensitivity sampling of compounds entering and exiting the sponge.
As shown in the PCA graphs in Figure 4 (membranes, blades, and fibers) and the OPLS-DA (membranes and blades) and PLS-DA graphs (fibers) in Supplementary Figure S3, the membrane- and blade-based SPME devices were clearly discriminated from the control groups when they were used for analyte extraction. However, the PCA graph does not show a clear separation of the experimental and control groups in the case of the fibers (Figure 4). In addition, while it was not possible to perform OPLS-DA using SIMCA, we were able to perform PLS-DA. As can be seen in Supplementary Figure S3, the resulting plot shows poor separation between the experimental and control groups, and a significantly lower number of different features. Indeed, the membranes were able to detect 168 significantly different features, while the blades were able to detect 57. In contrast, the fibers were only able to detect two significant features.
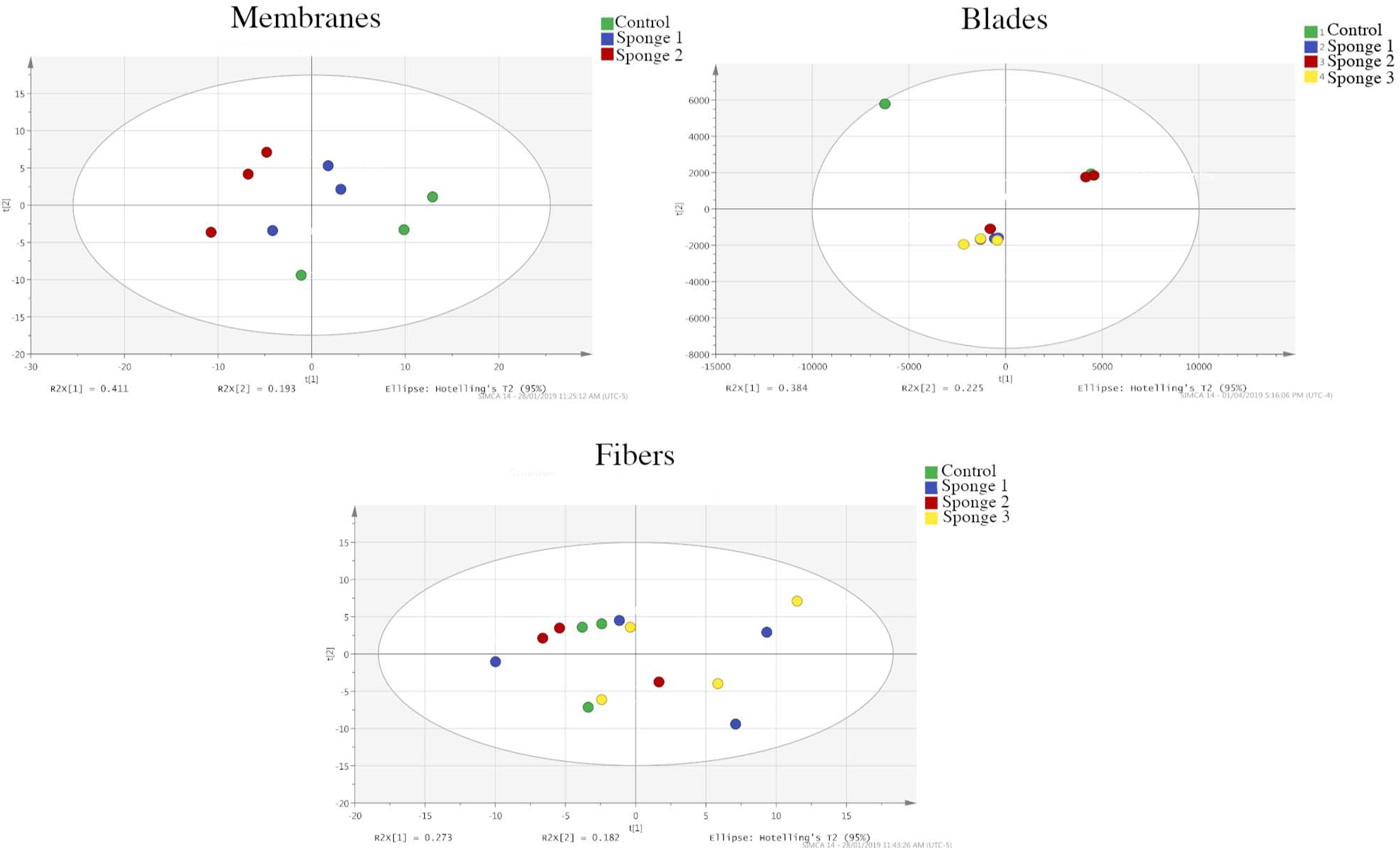
Figure 4. Principal component analysis plots showing the separation of the features of the analytes extracted via membranes, blades, and fibers in both the experimental and control groups. Membrane devices show clear separation, while the fiber-based devices show poor separation.
There could be two possible reasons for this result: The first potential explanation is related with the surface area. Since blades and membranes have much larger surface areas, they naturally contain a greater volume of extraction phase, which allows them to extract more analytes than a fiber. The second possible reason is related to the area of application. Namely, the fibers were inserted directly into the sponge’s tissue, which has a comparatively lower level of water flow than the areas sampled by the blades and membranes. As such, the fibers would have been exposed to less material than the blades or the membranes. It is also possible that the fibers were unable to extract a significant volume of metabolites due to them being bound to the biological matrix in the sponge and, thus, being low in free concentrations. The range of detected analytes was dominated by those in the ambient seawater and the seawater that passed through the sponge, with the number of analytes coming from the sponge’s tissue being minute. Since the blades and membranes were constantly subjected to the water flow maintained by the sponge, they would have been exposed to a much larger range of compounds.
The supervised analysis (OPLS-DA and PLS-DA) plots displayed similar results to the PCA plots, with membranes and blades showing clear separation and fibers not doing so (Supplementary Figure S3).
Identification of the Extracted Compounds
Supplementary Table S1 displays the information for the extracted compounds, which were identified using the m/z of their parent ions and fragmentation patterns. The significantly different features (m/z peaks) were tentatively identified using the free online database, METLIN (Smith et al., 2005), taking 5 ppm accuracy as the maximum limit in m/z error. However, in most cases, the high-accuracy m/z values corresponded to tens of possible identified compounds or metabolites. Previous researchers have attempted to tackle this problem by predicting the retention times of the analytes via liquid chromatography (Cao et al., 2015; Bruderer et al., 2017). Unfortunately, these predicted retention times are unsuitable for some metabolomics studies, as the logP values of some compounds may not be included in databases, or the predicted logP values of different compounds may be highly similar. This makes it harder to annotate the analytes because their retention times in the LC column may be very similar. It is possible that all of the analytes extracted during this research were derived from the sponge cells, symbiotic microorganisms, or other possible compounds, including toxicants and pollutants within the seawater passing through the sponge matrix. For this reason, predicting the metabolites by cross-referencing free online databases would not have been the correct approach, as it may have led to many false-positive identifications.
When it comes to confirming compounds, the only current option for untargeted metabolomics is to synthesize or isolate the predicted compounds. However, considering the immense number of possible metabolites predicted by free online databases, purchasing compounds or isolating them from biological sources would be very costly and potentially time-consuming. One method of minimizing the number of tentatively predicted compounds, and by extension, the risk of false-positive identifications, would be through fragmenting the parent ions (MS/MS) and comparing the m/z values of daughter ions with those reported on free online databases, such as METLIN. However, processing the data through this method has two disadvantages. The first disadvantage is that the MS1 data are from experiments conducted all over the world using very different instruments, whereas the MS/MS (MS2) data on free online databases are low and mostly predicted in silico. The second disadvantage is that most of the MS/MS data on these databases are produced using Q-TOF instruments. This is potentially problematic because the design of the collision cell and the ion detection mechanism differ between Q-Exactive and Q-TOF instruments. As such, the collision energies produced by the fragmentation of the parent ion would be variable, resulting in different relative intensities for daughter (fragment) ions (Tang et al., 2016).
Given the limited usefulness of online databases for correctly determining compounds, we further investigated the MS/MS spectra using Sirius software. This software, which was designed by bioinformaticians at the University of Jena, determines the molecular formula of analytes by analyzing daughter ions that correspond to possible molecular formulas after certain losses, such as H2O or CO (Dührkop and Böcker, 2015). To further assist with the identification of molecules, Sirius uses CSI:FingerID software, which uses fragmentation tree computation and machine learning to search for molecular structures in public databases such as KEGG and PubChem (Dührkop et al., 2015). Since PubChem includes a database of previously characterized chemical compounds – rather than all possible chemical formulas – we elected to use it to search our predicted molecular formulas. During this stage, we searched for compounds predicted by the METLIN database rather than simply relying on the top molecular identification provided by CSI:FingerID. If the CSI:FingerID list included one or more compounds listed in the METLIN database, we finalized our identification and recorded the compound name. If the METLIN database failed to return any predictions corresponding to the formula identified by Sirius, we displayed the formula that best matched the fragmentation pattern and the m/z value of the parent ion. Supplementary Figure S4 provides LC-MS spectra for some of the Sirius-annotated features (that correspond to m/z values 137.0599, 149.06, 251.1641, and 340.2846), as well as the in silico predicted fragment ions and fragmentation pathways. All of the compounds discussed in the section “Results and Discussion” were identified using this methodology.
An online search of the KEGG database (Kanehisa, 2006; Kanehisa et al., 2017) was performed once the identification stage was completed to determine whether specific biochemical pathways for these metabolites had been reported.
Extracted Analytes Display Compounds Related With Microbial Quorum Sensing
The presence of symbiotic bacteria in Sarcotragus and other Irciniid sponge species has been well documented (Hardoim and Costa, 2014; Horn et al., 2016; Saurav et al., 2016; Thomas et al., 2016). Since bacteria are also food sources for these filter-feeding metazoans, they rely on signaling activity to coordinate their cooperative activities in order to maintain their symbiotic relationship with the sponges, thus ensuring their survival. Signaling activity among bacteria, which is broadly referred to as “quorum sensing,” involves the release of autoinducers, the activation of signal transduction cascades, and the induction of gene expression regulation (Churchill and Chen, 2011). Quorum sensing plays an important role in the development of bacterial biofilm and how attractive these biofilms are to higher-order marine organisms (Huang et al., 2007). The first remarkable finding from the analysis of the extracted analytes was the presence of acyl-homoserine lactones. Acyl-homoserine lactones (ACLs) are signaling molecules generally related to cell-to-cell communication and quorum sensing among Gram-negative bacteria. These autoinducing chemical compounds coordinate diverse, often cooperative activities, such as bioluminescence, biofilm formation, and exoenzyme secretion (Schaefer et al., 2008; Schuster et al., 2013). The presence of ACL-related quorum-sensing activity among Alpha- and Gammaproteobacteria, which are microsymbionts of sponge species, has been previously reported (Taylor et al., 2004; Schupp et al., 2005). ACL-related quorum sensing is a key component in initiating the transfer of bacteria from a free-living state to a host-associated, symbiotic state (Parsek and Greenberg, 2000; Majerczyk et al., 2013; Schuster et al., 2013).
The extractions performed by placing the blades in the sponge’s exhalent pores would be likely to collect signaling molecules associated with quorum sensing, as bacteria routinely release these molecules into their surroundings. It is also possible that the surrounding water that was continuously pumped by these individual sponges contained the quorum-sensing compounds, however, the fold-increase in the intensity of the m/z peaks we acquired through high-resolution LC-MS was always higher in our experimental groups, compared to the controls. One of the analytes extracted via thin-film blades was N-3-oxo-hexadec-11(Z)-enoyl-L-Homoserine lactone (m/z 352.2467, [M + H]+), which is a C16 homoserine lactone derivative. Long-chain AHLs are common in Sarcotragus sp., and prior research has shown that they are often released by Paracoccus sp., which are known to have flexible associations, for example, either being free-living or host-associated (Saurav et al., 2016).
Two probable identifications of AHLs were N-pentadecanoyl-L-Homoserine lactone (m/z 326.2685, [M + H]+) and N-hexadecanoyl-L-Homoserine lactone (m/z 340.2846, [M + H]+). These AHLs possess varying carbon chains, and prior reports have noted their synthesis by Burkholderia sp. (Proteobacteria) and Pseudomonas sp. Furthermore, previous studies have found that Burkholderia sp. is the dominant microbial species in the metagenome of the endemic marine sponge, Arerosclera brasiliensis (Trindade-Silva et al., 2012). Our findings were consistent with this prior research, as our extractions also showed a biomarker for the presence of Burkholderia sp. among the microbiome.
Metabolism of MAHs and PAHs by Symbiotic Bacteria
Monocyclic aromatic hydrocarbons, such as benzene, toluene, ethyl benzene, and xylene (BTEX), and PAHs, such as naphthalene, phenanthrene, and pyrene, are compounds possessing aromatic rings that are commonly released as industrial and urban waste into marine ecosystems, causing ecotoxicity (Weissenfels et al., 1990; Oberoi et al., 2015). Although MAHs and PAHs tend to mostly be present in shallow waters (coastal water, estuaries, etc.) (Fernandes et al., 1997; Baumard et al., 1998), aromatic hydrocarbons such as polybrominated and polychlorinated biphenyls can reach the deepest parts of the ocean after adsorbing to particulate matter (Jamieson et al., 2017). Fortunately, the microorganisms of these ecosystems possess the ability to either degrade or utilize these aromatic hydrocarbon pollutants. Bacterial metabolic pathways are one of the predominant ways in which these compounds are degraded, particularly pathways related to bacteria from the genus Sphingomonas, Micrococcus, Streptomyces, Bacillus, Pseudomonas, and Burkholderia (Desai et al., 2008; Jegan et al., 2010; Lin et al., 2010; Andreolli et al., 2011; Oberoi et al., 2015).
Among the notable annotated GC-MS analytes (Supplementary Table S2) extracted via the thin-film membranes were those related to the metabolism of MAHs. Biphenyl, which is a metabolite related to the degradation of dioxins, was extracted from sponge #1, but was not detected by the devices in the control group. Similarly, propyl benzene, which is a degradation product of toluene (as reported by the KEGG database), was extracted from sponge #2 in significantly higher amounts than in the control (Supplementary Figure S5, peak areas of ion chromatograms). One reason for the presence of these compounds among our analytes may have to do with the fact that many species of sponges are known to contain MAH- and PAH-utilizing bacteria, such as Cycloclasticus pugetii and Burkholderia sp. (Rehmann and Daugulis, 2006, 2007; Kim et al., 2011) These results further support our finding that Sarcotragus sp. forms a symbiotic relationship with Burkholderia sp.
The most notable metabolite detected during LC-MS analysis of the SPME blades was 3-isochromanone (or one of its isomers) (m/z 149.06, [M + H]+), which is a metabolite associated with PAH degradation. 3-isochromanone is a metabolite related to fluorene or fluorene-related compounds (Mrozik et al., 2003), and it is also present as one of the metabolic compounds of Pseudomonas sp., Sphingomonas sp., and Burkholderia sp. (Weissenfels et al., 1990; Wattiau et al., 2001; Andreolli et al., 2011) Toluate (or vinycathecol) (m/z 137.0599, [M + H]+), which is an intermediate product of xylene (or styrene) degradation, was also extracted using the thin film membranes.
The findings of the present research demonstrate the metabolism of MAH and PAH by bacteria through identifying the presence of metabolites related to these compounds among the analytes detected via LC-MS and GC-MS analysis (Supplementary Tables S1, S2).
Metabolites Related With Photosynthetic Organisms
Our method was also able to detect a selection of metabolites that may play important roles in the metabolism of photosynthetic organisms, such as methyl cinnamate (m/z 163.0755, [M + H]+), dihydroconiferyl alcohol (m/z 183.1017, [M + H]+), tecostanine (m/z 184.1697, [M + H]+), apiole (m/z 223.0966, [M + H]+), and 12-hydroxyjasmonic acid (m/z 227.1279, [M + H]+). For example, methyl cinnamate is an active compound found in many plant-based essential oils (Viña and Murillo, 2003; Chen Y.Y. et al., 2012). Similarly, apiole is also common in essential oils and has been found to have anti-cancer properties against colon-cancer cells (Wei et al., 2012). At present, it is unknown whether these compounds are produced by photosynthetic microorganisms, such as cyanobacteria, which could potentially be associated with sponges. However, the presence of some of these compounds, such as methyl cinnamate (Supplementary Table S1), in our samples can be possibly attributed to their uptake from the surrounding seawater and subsequent release.
Secondary Metabolites of Sponges With Diverse Bioactivities
Sponges are a great source of secondary metabolites related to terpene and terpenoid, such as monoterpenoids, diterpenoids, and sesquiterpenoids (Faulkner, 2001). These sponge-, or sponge-holobiont sourced terpenoids could have diverse bioactivities, such as cytotoxicity, or antimicrobial, antiviral, anti-cancer, and anti-inflammatory features (Ebada et al., 2010; Gordaliza, 2010; Chen W. et al., 2012). As demonstrated in Supplementary Table S1, terpenoids comprised a very large portion of the compounds extracted via SPME. Sarcotragus sp. is a source of terpenoids with cytotoxic and antimicrobial activities (Wang et al., 2008). Moreover, this species has also been found to contain terpenes with cytotoxic effects against cancer cells (Liu et al., 2001) and possible antitumor activities, which is a finding that should be confirmed through further study (Abed et al., 2011). In addition, other sponge-derived terpenoids could possess anti-inflammatory properties. For example, the sesquiterpene-quinones of the Dysidea cf. cristagalli species could potentially be harnessed by humans for their potential anti-inflammatory effects (McNamara et al., 2005).
The extractions also revealed the presence of metabolite irone (m/z 207.1745, [M + H]+), which could be an exometabolite of fungi using sponges as hosts (Zhao et al., 2010). Abscisic alcohol (m/z 251.1641, [M + H]+) is an intermediate metabolite in the synthesis of abscisic acid (ABA), which is produced by sponges in response to heat stress (Bassaganya-Riera et al., 2010). Although ABA concentrations were higher in the control samples, this result may have been due to the sponges releasing this compound in high concentrations prior to SPME sampling. Triterpene glycosides, which are known secondary metabolites of the Caribbean reef sponge, Erylus formossus, perform a defensive function, protecting their host sponge species from predatory fishes. As such, their related terpene glycosides could be important metabolites with defensive properties against animals (Kubanek et al., 2000). The unidentified functions of some of the terpenes and terpenoids listed in Supplementary Table S1 could possibly be related to defensive roles or indicate medicinal properties, which should further be examined in future studies.
Accumulation of Pesticides and Drugs in the Marine Environment
As we went through our annotated list of compounds, we observed that the extracted analytes also contained pesticides and drugs, with some of these compounds being present in higher amounts in the sponge experimental groups than the controls. This finding was likely due to the fact that sponges are constantly pumping and taking in large amounts of surrounding seawater, thus accumulating pollutants (Gentric et al., 2016). Among the drugs and bioactive organic chemicals that were extracted, those with potential therapeutic applications included ligustilide (a phthalide), 3-Dimethylallyl-4-hydroxybenzaldehyde (m/z 191.1066, [M + H]+), S-carboxymethyl-L-cysteine (m/z 202.0137, [M + Na]+), primidone (m/z 219.112, [M + H]+), cimbuterol (m/z 234.162, [M + H]+), piroglirida (m/z 271.1973, [M + H]+), N-pelargonoyl dopamine (m/z 294.2093, [M + H]+), isopropyl dodecylphosphonofluoridate (IDFP) (m/z 295.2197, [M + H]+), and impulsin (m/z 300.2896, [M + H]+). 2,4-xylidine (m/z 122.0965, [M + H]+) is a carcinogenic compound that was detected in higher concentrations among our control groups.
Conclusion
Sponges are organisms that are ubiquitous in coastal marine habitats. These organisms are well-known for hosting and forming symbiotic relationships with an array of bacterial genera, with the resulting sponge holobionts displaying a wide range of biomarkers that can be involved in several biochemical pathways. SPME is a non-exhaustive method that uses biocompatible materials, which prevent bioactivity in the sponge or its microbiome. As such, SPME is a highly suitable approach for extracting short-lived metabolites in situ. This work documents the capacity of SPME to extract a wide spectrum of metabolites from the S. foetidus holobiont. The extracted metabolites were largely related to functions such as quorum sensing, mono- and polycyclic aromatic hydrocarbon degradation, and the retention of other pollutants by the sponges. This in situ SPME approach, with its non-invasive and biocompatible properties, provides advantages that cannot be offered by other sampling methods. Most notable among these advantages is its ability to enable sampling without affecting endangered or threatened organisms, such as rare or endangered sponge species. This is significant, as it enables researchers to directly monitor how sponges respond to environmental fluctuations and stressors without endangering the well-being of the organism.
Data Availability Statement
This manuscript contains previously unpublished data. The name of the repository and accession number(s) are not available.
Author Contributions
EP, TD, and JP conceived the study. BB, BO, and EB curated the data. BB and BO formally analyzed the data and wrote the original draft. BB, EB, and EP investigated the results. EP and TD developed the methodology. TD and JP administered the project and supervised the study.
Funding
This work was supported by the Natural Sciences and Engineering Research Council of Canada Discovery Grant RGPIN-2019-03904.
Conflict of Interest
The authors declare that the research was conducted in the absence of any commercial or financial relationships that could be construed as a potential conflict of interest.
Acknowledgments
The authors would like to acknowledge Krzysztof Gorynski, Evangelina Yiantzi, and Sofia Lendor for the technical support provided during the experimental part of this work and Supelco for providing the mixed mode SPME fibers. BB, EB, and EP would like to acknowledge the COST Action CA 16215 for the opportunity of collaboration.
Supplementary Material
The Supplementary Material for this article can be found online at: https://www.frontiersin.org/articles/10.3389/fmars.2019.00632/full#supplementary-material
References
Abed, C., Legrave, N., Dufies, M., Robert, G., Guérineau, V., Vacelet, J., et al. (2011). A new hydroxylated nonaprenylhydroquinone from the mediterranean marine sponge Sarcotragus spinosulus. Mar. Drugs 9, 1210–1219. doi: 10.3390/md9071210
Altunok, M., Özkaya, F. C., Engin, S., Tanrikul, T. T., Aydinlik, Ş., and Ulukaya, E. (2015). In vitro antibacterial activity of sponge-associated fungi against bacterial aquaculture pathogens. Fresenius Environ. Bull. 24, 2158–2166.
Andreolli, M., Lampis, S., Zenaro, E., Salkinoja-Salonen, M., and Vallini, G. (2011). Burkholderia fungorum DBT1: a promising bacterial strain for bioremediation of PAHs-contaminated soils. FEMS Microbiol. Lett. 319, 11–18. doi: 10.1111/j.1574-6968.2011.02259.x
Arellano, S. M., Lee, O. O., Lafi, F. F., Yang, J., Wang, Y., Young, C. M., et al. (2013). Deep sequencing of myxilla (Ectyomyxilla) methanophila, an epibiotic sponge on cold-seep tubeworms, reveals methylotrophic, thiotrophic, and putative hydrocarbon-degrading microbial associations. Microb. Ecol. 65, 450–461. doi: 10.1007/s00248-012-0130-y
Bassaganya-Riera, J., Skoneczka, J., Kingston, D., Krishnan, A., Misyak, S., Guri, A., et al. (2010). Mechanisms of action and medicinal applications of abscisic acid. Curr. Med. Chem. 17, 467–478. doi: 10.2174/092986710790226110
Baumard, P., Budzinski, H., and Garrigues, P. (1998). Polycyclic aromatic hydrocarbons in sediments and mussels of the western mediterranean sea. Environ. Toxicol. Chem. 17, 765–776.
Bauvais, C., Bonneau, N., Blond, A., Pérez, T., Bourguet-Kondracki, M. L., and Zirah, S. (2017). Furanoterpene diversity and variability in the marine sponge Spongia officinalis, from untargeted LC-MS/MS metabolomic profiling to furanolactam derivatives. Metabolites 7:E27. doi: 10.3390/metabo7020027
Böcker, S., Letzel, M. C., Lipták, Z., and Pervukhin, A. (2009). SIRIUS: decomposing isotope patterns for metabolite identification. Bioinformatics 25, 218–224. doi: 10.1093/bioinformatics/btn603
Bojko, B., Cudjoe, E., Pawliszyn, J., and Wasowicz, M. (2011). Solid-phase microextraction. How far are we from clinical practice? Trends Anal. Chem. 30, 1505–1512. doi: 10.1016/j.trac.2011.07.008
Bojko, B., Reyes-Garce′s, N., Bessonneau, V., Goryn′ski, K., Mousavi, F., Souza Silva, E. A., et al. (2014). Solid-phase microextraction in metabolomics. Trends Anal. Chem. 61, 168–180. doi: 10.1016/j.trac.2014.07.005
Bruderer, T., Varesio, E., and Hopfgartner, G. (2017). The use of LC predicted retention times to extend metabolites identification with SWATH data acquisition. J. Chromatogr. B Anal. Technol. Biomed. Life Sci. 1071, 3–10. doi: 10.1016/j.jchromb.2017.07.016
Cachet, N., Genta-Jouve, G., Ivanisevic, J., Chevaldonné, P., Sinniger, F., Culioli, G., et al. (2015). Metabolomic profiling reveals deep chemical divergence between two morphotypes of the zoanthid Parazoanthus axinellae. Sci. Rep. 5:8282. doi: 10.1038/srep08282
Cao, M., Fraser, K., Huege, J., Featonby, T., Rasmussen, S., and Jones, C. (2015). Predicting retention time in hydrophilic interaction liquid chromatography mass spectrometry and its use for peak annotation in metabolomics. Metabolomics 11, 696–706. doi: 10.1007/s11306-014-0727-x
Chambers, M. C., Maclean, B., Burke, R., Amodei, D., Ruderman, D. L., Neumann, S., et al. (2012). A cross-platform toolkit for mass spectrometry and proteomics. Nat. Biotechnol. 30, 918–920. doi: 10.1038/nbt.2377
Chen, W., Li, Y., and Guo, Y. (2012). Terpenoids of Sinularia soft corals: chemistry and bioactivity. Acta Pharm. Sin. B 2, 227–237. doi: 10.1016/j.apsb.2012.04.004
Chen, Y. Y., Lee, M. H., Hsu, C. C., Wei, C. L., and Tsai, Y. C. (2012). Methyl cinnamate inhibits adipocyte differentiation via activation of the CaMKK2-AMPK pathway in 3T3-L1 preadipocytes. J. Agric. Food Chem. 60, 955–963. doi: 10.1021/jf203981x
Churchill, M. E. A., and Chen, L. (2011). Structural basis of acyl-homoserine lactone-dependent signaling. Chem. Rev. 111, 68–85. doi: 10.1021/cr1000817
Demir, V., and Okus, E. (2007). Marine Sponges from Datça-Bozburun Peninsua-a Specially Protected Area in the South Eastern Aegean Sea (Turkey). Available at: www.tcpdf.org (accessed January 10, 2019).
Desai, A. M., Autenrieth, R. L., Dimitriou-Christidis, P., and McDonald, T. J. (2008). Biodegradation kinetics of select polycyclic aromatic hydrocarbon (PAH) mixtures by Sphingomonas paucimobilis EPA505. Biodegradation 19, 223–233. doi: 10.1007/s10532-007-9129-9123
Dührkop, K., and Böcker, S. (2015). “Fragmentation trees reloaded,” in Research in Computational Molecular Biology. RECOMB 2015. Lecture Notes in Computer Science, Vol. 9029, ed. T. Przytycka (Cham: Springer).
Dührkop, K., Shen, H., Meusel, M., Rousu, J., and Böcker, S. (2015). Searching molecular structure databases with tandem mass spectra using CSI:FingerID. Proc. Natl. Acad. Sci. U.S.A. 112, 12580–12585. doi: 10.1073/pnas.1509788112
Ebada, S. S., Lin, W. H., and Proksch, P. (2010). Bioactive sesterterpenes and triterpenes from marine sponges: occurrence and pharmacological significance. Mar. Drugs 8, 313–346. doi: 10.3390/md8020313
Faulkner, D. J. (2001). Marine natural products (1999). Nat. Prod. Rep. 18, 1–49. doi: 10.1039/b006897g
Fernandes, M. B., Sicre, M. A., Boireau, A., and Tronczynski, J. (1997). Polyaromatic hydrocarbon (PAH) distributions in the Seine River and its estuary. Mar. Pollut. Bull. 34, 857–867. doi: 10.1016/S0025-326X(97)00063-65
Friedrich, A. B., Fischer, I., Proksch, P., Hacker, J., and Hentschel, U. (2001). Temporal variation of the microbial community associated with the mediterranean sponge Aplysina aerophoba. FEMS Microbiol. Ecol. 38, 105–113. doi: 10.1016/S0168-6496(01)00185-184
Gentric, C., Rehel, K., Dufour, A., and Sauleau, P. (2016). Bioaccumulation of metallic trace elements and organic pollutants in marine sponges from the South Brittany Coast, France. J. Environ. Sci. Health A Tox. Hazard. Subst. Environ. Eng. 51, 213–219. doi: 10.1080/10934529.2015.1094327
Gerovasileiou, V., Dailianis, T., Sini, M., Otero, M. D. M., Numa, C., Katsanevakis, S., et al. (2018). Assessing the regional conservation status of sponges (Porifera): the case of the Aegean ecoregion. Mediterr. Mar. Sci. 18, 43–52. doi: 10.12681/mms.14461
Gordaliza, M. (2010). Cytotoxic terpene quinones from marine sponges. Mar. Drugs 8, 2849–2870. doi: 10.3390/md8122849
Hardoim, C. C. P., and Costa, R. (2014). Temporal dynamics of prokaryotic communities in the marine sponge Sarcotragus spinosulus. Mol. Ecol. 23, 3097–3112. doi: 10.1111/mec.12789
Hentschel, U., Usher, K. M., and Taylor, M. W. (2006). Marine sponges as microbial fermenters. FEMS Microbiol. Ecol. 55, 167–177. doi: 10.1111/j.1574-6941.2005.00046.x
Horn, H., Slaby, B. M., Jahn, M. T., Bayer, K., Moitinho-Silva, L., Förster, F., et al. (2016). An Enrichment of CRISPR and other defense-related features in marine sponge-associated microbial metagenomes. Front. Microbiol. 7:1751. doi: 10.3389/fmicb.2016.01751
Huang, Y. L., Dobretsov, S., Ki, J. S., Yang, L. H., and Qian, P. Y. (2007). Presence of acyl-homoserine lactone in subtidal biofilm and the implication in larval behavioral response in the polychaete Hydroides elegans. Microb. Ecol. 54, 384–392. doi: 10.1007/s00248-007-9210-9219
Ivanišević, J., Thomas, O. P., Lejeusne, C., Chevaldonné, P., and Pérez, T. (2011). Metabolic fingerprinting as an indicator of biodiversity: towards understanding inter-specific relationships among Homoscleromorpha sponges. Metabolomics 7, 289–304. doi: 10.1007/s11306-010-0239-232
Jamieson, A. J., Malkocs, T., Piertney, S. B., Fujii, T., and Zhang, Z. (2017). Bioaccumulation of persistent organic pollutants in the deepest ocean fauna. Nat. Ecol. Evol. 1:51. doi: 10.1038/s41559-016-0051
Jegan, J., Vijayaraghavan, K., Senthilkumar, R., and Velan, M. (2010). Naphthalene degradation kinetics of micrococcus sp., isolated from activated sludge. Clean Soil Air Water 38, 837–842. doi: 10.1002/clen.200900148
Jiang, R., and Pawliszyn, J. (2014). Preparation of a particle-loaded membrane for trace gas sampling. Anal. Chem. 861, 403–410. doi: 10.1021/ac4035339
Kanehisa, M. (2006). From genomics to chemical genomics: new developments in KEGG. Nucleic Acids Res. 34, D354–D357. doi: 10.1093/nar/gkj102
Kanehisa, M., Furumichi, M., Tanabe, M., Sato, Y., and Morishima, K. (2017). KEGG: new perspectives on genomes, pathways, diseases and drugs. Nucleic Acids Res. 45, D353–D361. doi: 10.1093/nar/gkw1092
Kim, M., Bae, S. S., Seol, M., Lee, J. H., and Oh, Y. S. (2008). Monitoring nutrient impact on bacterial community composition during bioremediation of anoxic PAH-contaminated sediment. J. Microbiol. 46:615. doi: 10.1007/s12275-008-0097-z
Kim, Y. M., Murugesan, K., Schmidt, S., Bokare, V., Jeon, J. R., Kim, E. J., et al. (2011). Triclosan susceptibility and co-metabolism - A comparison for three aerobic pollutant-degrading bacteria. Bioresour. Technol. 102, 2206–2212. doi: 10.1016/j.biortech.2010.10.009
Konstantinou, D., Gerovasileiou, V., Voultsiadou, E., and Gkelis, S. (2018). Sponges-cyanobacteria associations: global diversity overview and new data from the eastern mediterranean. PLoS One 13:e0195001. doi: 10.1371/journal.pone.0195001
Kubanek, J., Pawlik, J. R., Eve, T. M., and Fenical, W. (2000). Triterpene glycosides defend the Caribbean reef sponge Erylus formosus from predatory fishes. Mar. Ecol. Prog. Ser. 207, 69–77. doi: 10.3354/meps207069
Lin, C., Gan, L., and Chen, Z. L. (2010). Biodegradation of naphthalene by strain Bacillus fusiformis (BFN). J. Hazard. Mater. 182, 771–777. doi: 10.1016/j.jhazmat.2010.06.101
Linstrom, P. J., and Mallard, W. G. (2014). NIST Chemistry WebBook, NIST Standard Reference Database Number 69. Gaithersburg, MD: NIST.
Liu, Y., Bae, B. H., Alam, N., Hong, J., Sim, C. J., Lee, C. O., et al. (2001). New cytotoxic sesterterpenes from the sponge Sarcotragus species. J. Nat. Prod. 65, 1307–1314. doi: 10.1021/np0101494
Majerczyk, C., Kinman, L., Han, T., Bunt, R., and Greenberg, E. P. (2013). Virulence of Burkholderia mallei quorum-sensing mutants. Infect. Immun. 81, 1471–1478. doi: 10.1128/IAI.00048-13
McNamara, C. E., Larsen, L., Perry, N. B., Harper, J. L., Berridge, M. V., Chia, E. W., et al. (2005). Anti-inflammatory sesquiterpehe-quinones from the New Zealand sponge Dysidea cf. cristagalli. J. Nat. Prod. 68, 1431–1433. doi: 10.1021/np050171n
Mirnaghi, F. S., Chen, Y., Sidisky, L. M., and Pawliszyn, J. (2011). Optimization of the coating procedure for a high-throughput 96-blade solid phase microextraction system coupled with LC-MS/MS for analysis of complex samples. Anal. Chem. 83, 6018–6025. doi: 10.1021/ac2010185
Montalvo, N. F., Mohamed, N. M., Enticknap, J. J., and Hill, R. T. (2005). Novel actinobacteria from marine sponges. Antonie van Leeuwenhoek 87, 29–36. doi: 10.1007/s10482-004-6536-x
Mrozik, A., Piotrowska-Seget, Z., and £abużek, S. (2003). Bacterial degradation and bioremediation of polycyclic aromatic hydrocarbons. Polish J. Environ. Stud. 12, 15–25.
Oberoi, A. S., Philip, L., and Bhallamudi, S. M. (2015). Biodegradation of various aromatic compounds by enriched bacterial cultures: part a–monocyclic and polycyclic aromatic hydrocarbons. Appl. Biochem. Biotechnol. 176, 1870–1888. doi: 10.1007/s12010-015-1684-1
Olivon, F., Grelier, G., Roussi, F., Litaudon, M., and Touboul, D. (2017). MZmine 2 data-preprocessing to enhance molecular networking reliability. Anal. Chem. 89, 7836–7840. doi: 10.1021/acs.analchem.7b01563
Oulhen, N., Schulz, B. J., and Carrier, T. J. (2016). English translation of Heinrich Anton de Bary’s 1878 speech, ‘Die Erscheinung der Symbiose’ (‘De la symbiose’). Symbiosis 69, 131–139. doi: 10.1007/s13199-016-0409-8
Pansini, M., Morri, C., and Bianchi, C. N. (2000). The sponge community of a subtidal area with hydrothermal vents: Milos Island. Aegean Sea Estuar. Coast. Shelf Sci. 51, 627–635. doi: 10.1006/ecss.2000.0674
Parsek, M. R., and Greenberg, E. P. (2000). Acyl-homoserine lactone quorum sensing in Gram-negative bacteria: a signaling mechanism involved in associations with higher organisms. Proc. Natl. Acad. Sci. U.S.A. 97, 8789–8793. doi: 10.1073/pnas.97.16.8789
Pavloudi, C., Christodoulou, M., and Mavidis, M. (2016). Macrofaunal assemblages associated with the sponge Sarcotragus foetidus Schmidt, 1862 (Porifera: Demospongiae) at the coasts of Cyprus and Greece. Biodivers. Data J. 4, e8210. doi: 10.3897/BDJ.4.e8210
Pawliszyn, J. (ed.) (2012b). “Theory of solid-phase microextraction,” in Handbook of Solid Phase Microextraction, (Amsterdam: Elsevier), doi: 10.1016/B978-0-12-416017-0.00002-4
Pita, L., Rix, L., Slaby, B. M., Franke, A., and Hentschel, U. (2018). The sponge holobiont in a changing ocean: from microbes to ecosystems. Microbiome 6:46. doi: 10.1186/s40168-018-0428-1
Rehmann, L., and Daugulis, A. J. (2006). Biphenyl degradation kinetics by Burkholderia xenovorans LB400 in two-phase partitioning bioreactors. Chemosphere 63, 972–979. doi: 10.1016/j.chemosphere.2005.08.076
Rehmann, L., and Daugulis, A. J. (2007). Biodegradation of biphenyl in a solid-liquid two-phase partitioning bioreactor. Biochem. Eng. J. 36, 195–201. doi: 10.1016/j.bej.2007.02.016
Reiswig, H. M. (1971). Particle feeding in natural populations of three marine demosponges. Biol. Bull. 141, 568–591. doi: 10.2307/1540270
Reverter, M., Tribalat, M.-A., Pérez, T., and Thomas, P. O. (2018). Metabolome variability for two Mediterranean sponge species of the genus Haliclona: specificity, time, and space. Metabolomics 14:114. doi: 10.1007/s11306-018-1401-5
Reyes-Garcés, N., Gionfriddo, E., Gómez-Ríos, G. A., Alam, M. N., Boyacl, E., Bojko, B., et al. (2018). Advances in solid phase microextraction and perspective on future directions. Anal. Chem. 90, 302–360. doi: 10.1021/acs.analchem.7b04502
Ribes, M., Coma, R., and Gili, J. M. (1999). Natural diet and grazing rate of the temperate sponge Dysidea avara (Demospongiae, Dendroceratida) throughout an annual cycle. Mar. Ecol. Prog. Ser. 176, 179–190. doi: 10.3354/meps176179
Rubin-Blum, M., Antony, C. P., Borowski, C., Sayavedra, L., Pape, T., Sahling, H., et al. (2017). Short-chain alkanes fuel mussel and sponge Cycloclasticus symbionts from deep-sea gas and oil seeps. Nat. Microbiol. 2:17093. doi: 10.1038/nmicrobiol.2017.93
Sauleau, P., Moriou, C., and Al Mourabit, A. (2017). Metabolomics approach to chemical diversity of the Mediterranean marine sponge Agelas oroides. Nat. Prod. Res. 31, 1625–1632. doi: 10.1080/14786419.2017.1285298
Saurav, K., Burgsdorf, I., Teta, R., Esposito, G., Bar-Shalom, R., Costantino, V., et al. (2016). Isolation of marine Paracoccus sp. Ss63 from the sponge Sarcotragus sp. and characterization of its quorum-sensing chemical-signaling molecules by LC-MS/MS analysis. Isr. J. Chem. 56, 330–340. doi: 10.1002/ijch.201600003
Schaefer, A. L., Greenberg, E. P., Oliver, C. M., Oda, Y., Huang, J. J., Bittan-Banin, G., et al. (2008). A new class of homoserine lactone quorum-sensing signals. Nature 454, 595–599. doi: 10.1038/nature07088
Schupp, P. J., Charlton, T. S., Taylor, M. W., Kjelleberg, S., and Steinberg, P. D. (2005). Use of solid-phase extraction to enable enhanced detection of acyl homoserine lactones (AHLs) in environmental samples. Anal. Bioanal. Chem. 383, 132–137. doi: 10.1007/s00216-005-3387-x
Schuster, M., Joseph Sexton, D., Diggle, S. P., and Peter Greenberg, E. (2013). Acyl-homoserine lactone quorum sensing: from evolution to application. Annu. Rev. Microbiol. 67, 43–63. doi: 10.1146/annurev-micro-092412-155635
Smith, C. A., O’Maille, G., Want, E. J., Qin, C., Trauger, S. A., Brandon, T. R., et al. (2005). METLIN: a metabolite mass spectral database. Ther. drug Monit. 27, 747–751. doi: 10.1097/01.ftd.0000179845.53213.39
Space Scents (2002). Space Scents. Available at: https://science.nasa.gov/science-news/science-at-nasa/2002/18dec_scents (accessed July 01, 2019).
Tang, H., Wang, X., Xu, L., Ran, X., Li, X., Chen, L., et al. (2016). Establishment of local searching methods for orbitrap-based high throughput metabolomics analysis. Talanta 156–157, 163–171. doi: 10.1016/j.talanta.2016.04.051
Tautenhahn, R., Patti, G. J., Rinehart, D., and Siuzdak, G. (2012). XCMS online: a web-based platform to process untargeted metabolomic data. Anal. Chem. 84, 5035–5039. doi: 10.1021/ac300698c
Taylor, M. W., Radax, R., Steger, D., and Wagner, M. (2007). Sponge-associated microorganisms: evolution, ecology, and biotechnological potential. Microbiol. Mol. Biol. Rev. 71, 295–347. doi: 10.1128/MMBR.00040-6
Taylor, M. W., Schupp, P. J., Baillie, H. J., Charlton, T. S., De Nys, R., Kjelleberg, S., et al. (2004). Evidence for acyl homoserine lactone signal production in bacteria associated with marine sponges. Appl. Environ. Microbiol. 70, 4387–4389. doi: 10.1128/AEM.70.7.4387-4389.2004
Thomas, T., Moitinho-Silva, L., Lurgi, M., Björk, J. R., Easson, C., Astudillo-García, C., et al. (2016). Diversity, structure and convergent evolution of the global sponge microbiome. Nat. Commun. 7:11870. doi: 10.1038/ncomms11870
Trindade-Silva, A. E., Rua, C., Silva, G. G. Z., Dutilh, B. E., Moreira, A. P. B., Edwards, R. A., et al. (2012). Taxonomic and functional microbial signatures of the endemic marine sponge arenosclera brasiliensis. PLoS One 7:e39905. doi: 10.1371/journal.pone.0039905
Viña, A., and Murillo, E. (2003). Essential oil composition from twelve varieties of basil (Ocimum spp.) grown in Colombia. J. Braz. Chem. Soc. 14, 744–749. doi: 10.1590/S0103-50532003000500008
Wang, N., Song, J., Kyoung, H. J., Lee, H. S., Li, X., Oh, K. B., et al. (2008). Sesterterpenoids from the sponge Sarcotragus sp. J. Nat. Prod. 71, 551–557. doi: 10.1021/np0780147
Wang, W., Wang, L., and Shao, Z. (2018). Polycyclic aromatic hydrocarbon (PAH) degradation pathways of the obligate marine PAH degrader Cycloclasticus sp. strain P1. Appl. Environ. Microbiol. 84:e01261-18. doi: 10.1128/AEM.01261-18
Wattiau, P., Bastiaens, L., Van Herwijnen, R., Daal, L., Parsons, J. R., Renard, M. E., et al. (2001). Fluorene degradation by Sphingomonas sp. LB126 proceeds through protocatechuic acid: a genetic analysis. Res. Microbiol. 152, 861–872. doi: 10.1016/S0923-2508(01)01269-4
Webster, N. S., Wilson, K. J., Blackall, L. L., and Hill, R. T. (2001). Phylogenetic diversity of bacteria associated with the marine sponge Rhopaloeides odorabile. Appl. Environ. Microbiol. 67, 434–444. doi: 10.1128/AEM.67.1.434-444.2001
Wehrl, M., Steinert, M., and Hentschel, U. (2007). Bacterial uptake by the marine sponge Aplysina aerophoba. Microb. Ecol. 53, 355–365. doi: 10.1007/s00248-006-9090-4
Wei, P.-L., Tu, S.-H., Lien, H.-M., Chen, L.-C., Chen, C.-S., Wu, C.-H., et al. (2012). The in vivo antitumor effects on human COLO 205 cancer cells of the 4,7-dimethoxy-5-(2-propen-1-yl)-1,3-benzodioxole (apiole) derivative of 5-substituted 4,7-dimethoxy-5-methyl-l,3-benzodioxole (SY-1) isolated from the fruiting body of Antrodia camphorate. J. Cancer Res. Ther. 8, 532–536. doi: 10.4103/0973-1482.106529
Weissenfels, W. D., Beyer, M., and Klein, J. (1990). Degradation of phenanthrene, fluorene and fluoranthene by pure bacterial cultures. Appl. Microbiol. Biotechnol. 32, 479–484. doi: 10.1007/BF00903787
Keywords: SPME, Porifera, microsymbionts, metabolomics, metabolite identification, LC-HRMS, GC-MS, high-resolution mass spectrometry
Citation: Bojko B, Onat B, Boyaci E, Psillakis E, Dailianis T and Pawliszyn J (2019) Application of in situ Solid-Phase Microextraction on Mediterranean Sponges for Untargeted Exometabolome Screening and Environmental Monitoring. Front. Mar. Sci. 6:632. doi: 10.3389/fmars.2019.00632
Received: 01 July 2019; Accepted: 26 September 2019;
Published: 11 October 2019.
Edited by:
Andrew Stanley Mount, Clemson University, United StatesReviewed by:
Valeria Costantino, University of Naples Federico II, ItalyDonald J. Gerhart, Duke University, United States
Copyright © 2019 Bojko, Onat, Boyaci, Psillakis, Dailianis and Pawliszyn. This is an open-access article distributed under the terms of the Creative Commons Attribution License (CC BY). The use, distribution or reproduction in other forums is permitted, provided the original author(s) and the copyright owner(s) are credited and that the original publication in this journal is cited, in accordance with accepted academic practice. No use, distribution or reproduction is permitted which does not comply with these terms.
*Correspondence: Thanos Dailianis, dGhhbm9zZEBoY21yLmdy; Janusz Pawliszyn, amFudXN6QHV3YXRlcmxvby5jYQ==
†These authors have contributed equally to this work