Dynamics Rationalize Proteolytic Susceptibility of the Major Birch Pollen Allergen Bet v 1
- Center for Molecular Biosciences Innsbruck, Institute of General, Inorganic and Theoretical Chemistry, University of Innsbruck, Innsbruck, Austria
Proteolytic susceptibility during endolysosomal degradation is decisive for allergic sensitization. In the major birch pollen allergen Bet v 1 most protease cleavage sites are located within its secondary structure elements, which are inherently inaccessible to proteases. The allergen thus must unfold locally, exposing the cleavage sites to become susceptible to proteolysis. Hence, allergen cleavage rates are presumed to be linked to their fold stability, i.e., unfolding probability. Yet, these locally unfolded structures have neither been captured in experiment nor simulation due to limitations in resolution and sampling time, respectively. Here, we perform classic and enhanced molecular dynamics (MD) simulations to quantify fold dynamics on extended timescales of Bet v 1a and two variants with higher and lower cleavage rates. Already at the nanosecond-timescale we observe a significantly higher flexibility for the destabilized variant compared to Bet v 1a and the proteolytically stabilized mutant. Estimating the thermodynamics and kinetics of local unfolding around an initial cleavage site, we find that the Bet v 1 variant with the highest cleavage rate also shows the highest probability for local unfolding. For the stabilized mutant on the other hand we only find minimal unfolding probability. These results strengthen the link between the conformational dynamics of allergen proteins and their stability during endolysosomal degradation. The presented approach further allows atomistic insights in the conformational ensemble of allergen proteins and provides probability estimates below experimental detection limits.
Introduction
With more than 30% of the population affected, immunoglobulin-E-mediated allergy is the most frequent immune disorder worldwide (Valenta, 2002; Valenta et al., 2010; Curin et al., 2018). Still, the determinants of what makes a protein an allergen are largely unknown (Scheurer et al., 2015; Verhoeckx et al., 2019). It has been demonstrated repeatedly that proteins with substantial similarity in sequence and structure are able to trigger an entirely different immune response (Mitropoulou et al., 2018; Eichhorn et al., 2019; Seutter von Loetzen et al., 2019; Tscheppe et al., 2020). Thus, the allergenic potential of a protein often cannot be rationalized, nor predicted, based on sequence or structural similarity to known allergens (Lu et al., 2018). However, one decisive feature biasing the immunologic response to a protein antigen is its proteolytic susceptibility (Toda et al., 2011; Apostolovic et al., 2016; Machado et al., 2016; Scheiblhofer et al., 2017; Wolf et al., 2018). Hence, reliable experimental and computational models of proteolytic susceptibility in the endosome represent promising tools to understand, and possibly even predict, what makes a protein an allergen.
In a study by Machado et al. (2016) point mutations were introduced to the major birch pollen allergen Bet v 1, which increased its proteolytic stability and also entirely modulated the resulting immune response. This trend was explained in the context of allergen processing and presentation (Freier et al., 2015; Machado et al., 2016; Scheiblhofer et al., 2017). Exogenous proteins enter antigen presenting cells, such as dendritic cells, via endocytosis. In the endosome, the protein is then cleaved into small peptide fragments by endolysosomal proteases, such as cathepsin S (Watts, 2001; Hsing and Rudensky, 2005; Conus and Simon, 2010; Egger et al., 2011; Freier et al., 2015). These fragments are then loaded onto major histocompatibility complex molecules type 2 (MHC2) and transported to the cell surface. The recognition of this MHC2-peptide complex by T-helper cells then determines the subsequent immune response (Watts, 2004; Valenta et al., 2010). Thus, proteolytic cleavage of the exogenous antigen is a crucial step in this mechanism and is discussed as the origin of the varying allergenic potential of the studied Bet v 1 variants (Thai et al., 2004; Machado et al., 2016; Wolf et al., 2018). Only antigens with an “optimal” stability are able to induce an allergic response on T-cell level. It has been postulated that proteins of low proteolytic stability are already cleaved in the early endosome, leading to a protective immune response. High proteolytic stability, on the other hand, leads to late degradation in the lysosome and thus fails to induce the required T cell polarization (Scheiblhofer et al., 2017). Consequently, targeted design of Bet v 1 variants with specific proteolytic susceptibilities is a promising approach in allergen-specific immunotherapy (Curin et al., 2018).
Interestingly, none of the mutations introduced by Machado et al. (2016) were located in the initial cleavage sites. Hence, the recognition pattern of the protease remains unchanged. Still, the cleavage rates already differ substantially after introduction of a single point mutation, i.e., the exchange of aspartate 69 with isoleucine (D69I). Additionally, the initial cleavage sites are mostly located within stable secondary structure elements, e.g., the main initial cleavage site of Bet v 1 is found in the first alpha helix between residues A16 and I23 (Egger et al., 2011; Freier et al., 2015). Yet, for the involved proteases it is sterically impossible to bind the allergen in this conformation (Madala et al., 2010). Thus, the allergen inevitably has to unfold locally to become susceptible to proteolysis and lead to the observed cleavage pattern.
It is well-established that proteins in solution fluctuate between diverse conformational states of varying probability, including (partially) unfolded states (Karplus and Weaver, 1976; Henzler-Wildman and Kern, 2007; Boehr et al., 2009; Dror et al., 2012; Latorraca et al., 2017). We thus surmise that the equilibrium between folded and partially unfolded conformational states of a protein, is linked to its proteolytic susceptibility. In line with this concept, increased cleavage rates indicate a shift of the ensemble toward partially unfolded conformations, whereas a stabilizing mutation decreases the probability of local unfolding (Freier et al., 2015). Substantial efforts have led to a multitude of structural models of allergen proteins with a Bet v 1-like fold from X-ray crystallography as well as NMR structure refinements (Gajhede et al., 1996; Schweimer et al., 1999; Aalberse, 2000; Ahammer et al., 2017; Moraes et al., 2018; Jacob et al., 2019). However, despite the utmost importance of local unfolding for proteolytic cleavage, no structural models depicting partially unfolded conformations have yet been solved. This might indicate a low probability of the partially unfolded state within the solution ensemble, below the detection limit of X-ray crystallography and NMR structure refinements (Hart et al., 2016). However, using dynamic NMR experiments, including backbone amide 15N relaxation dispersion and hydrogen-deuterium exchange experiments, in combination with MD simulations we were able to capture a relation between conformational dynamics and varying cleavage rates of Bet v 1 variants (Grutsch et al., 2017). While, increased flexibility was observed around initial cleavage sites, the obtained data did not allow for a structural model of a partially unfolded state.
As described above, Bet v 1 is among the most extensively studied allergen proteins in experiment, computational models and clinical studies (Spangfort et al., 2003; Bollen et al., 2010; Berkner et al., 2014; Garrido-Arandia et al., 2014; Grutsch et al., 2014; Kitzmuller et al., 2015; Kamenik et al., 2016; Moingeon et al., 2016; Biedermann et al., 2019). With an estimated 100 million of individuals suffering from birch pollen allergy, it is also one of the clinically most relevant allergens (Hartl et al., 2004). Most intriguingly for the present study, the degradation patterns of Bet v 1 and a multitude of its mutants have been studied with a degradome assay (Egger et al., 2011; Machado et al., 2016). This assay was specifically designed to mimic endolysosomal degradation of allergen proteins and allows for a time-resolved quantification of proteolytic susceptibility. Thereby, detailed information on location and quantity of the initial scissile sites became accessible (Egger et al., 2011). Several studies have been published focusing on the proteolytic susceptibility and fold stability of Bet v 1 variants (Pree et al., 2007; Husslik et al., 2015; Apostolovic et al., 2016; Ferrari et al., 2016; Machado et al., 2016; Pekar et al., 2018). In addition to variations in sequence also environmental factors, such as nitration or ligand binding, have been shown to impact proteolytic susceptibility as well as the immunogenicity of Bet v 1 and allergen proteins in general (Mogensen et al., 2002; Ackaert et al., 2014; Asam et al., 2014; Grutsch et al., 2014; von Loetzen et al., 2014, 2015; Foo et al., 2019; Soh et al., 2019).
Here, we analyze the fold dynamics of the Bet v 1.0101 (Bet v 1a) wild-type protein and two variants with varying stability in the context of proteolytic susceptibility. We study the isoform Bet v 1.0102 (Bet v 1d) as destabilized variant. Differing in seven amino acids, Bet v 1d shares a sequence identity of 95.6% with the wild-type protein Bet v 1a (Wagner et al., 2008). Only one mutation is found in the vicinity of the major initial cleavage site (F30V). However, this exchange cannot explain the considerable difference found for the cleavage rate at this site (Egger et al., 2011; Freier et al., 2015). Even more fascinating is the above-mentioned point-mutant Bet v 1a-D69I. This mutation is located far from the cleavage site, both in sequence and in structure. Nevertheless, the decrease of the degradation rate compared to the wild-type protein is substantial (Machado et al., 2016). In an effort to quantify distinctions in structural fluctuations within the native state ensemble we perform classic molecular dynamics (MD) simulations. We thereby compare the stability of the three Bet v 1 variants (Bet v 1a, Bet v 1d, and Bet v 1a-D69I) based on dynamics observed at the nano- to microsecond timescale. We characterize the dynamics of the native state ensemble at pH 5, mimicking the acidic conditions in the endolysosomal antigen-processing compartment, with classic MD (cMD) simulations. In a previous study on Bet v 1 variants described above, we have applied a similar workflow, combined with NMR relaxation experiments to study a broach range of dynamics. With the use of NMR relaxation dispersion measurements, we found the initial cleavage site in Bet v 1 d to be more dynamic than in Bet v 1a, particularly on the millisecond timescale. Yet, all previous calculations were adjusted to the experimental conditions and thus protonation states resembling pH 7 were applied (Machado et al., 2016; Grutsch et al., 2017). In this study however, we aim at modeling ensembles of Bet v 1 variants, as they are expected to occur at pH 5. This decrease in pH substantially alters the protonation states, and thus H-bond networks as well as coulomb interactions within each structure (Hofer et al., 2019). Hence, the presented results are not directly comparable to previously published findings. Additionally, we present the first approach to capture the partial unfolding in allergen proteins and estimate the associated thermodynamics and kinetics. It is expected that the conformational rearrangements within the entire fold, which are inherently linked to the partial unfolding of the initial cleavage site, are extensive. Furthermore, as described above, we expect that the probability of the partially unfolded state of the studied Bet v 1 variants is low compared to the folded state at the applied conditions (37°C, 1 bar, pH 5). Thus, it is highly unlikely to observe this process on timescales accessible to classic MD simulations. Hence, we employ an enhanced sampling technique, well-tempered metadynamics MD (MDMD) (Barducci et al., 2008; Laio and Gervasio, 2008; Leone et al., 2010), to drive the transition from a folded to a locally unfolded conformational state.
The applied workflows allow us on the one hand to study motions and interactions within the folded state, where barriers are low and dynamics on the ns- to μs-timescale can be monitored (Bowman, 2016). On the other hand, we also profile the slow motions resulting in partial unfolding of the Bet v 1 variants in atomistic detail. By calculating thermodynamic and kinetic properties from both approaches we rationalize the experimentally determined differences in proteolytic susceptibility.
Methods
Simulation Setup
The starting structures for the wild-type protein, Bet v 1a, and its isoform Bet v 1d were prepared from the respective available crystal structures [PDB codes 4A88 (Kofler et al., 2012) and 1FM4, respectively (Marković-Housley et al., 2003)] with the program MOE (Molecular Operating Environment) (Labute, 2009; MOE, 2019). All ions, crystallization agents and water molecules were removed. The D69I point mutant was created with the program MOE via single point mutation, followed by a short backbone relaxation, since no experimental structures were available.
The LEaP module of the AmberTools19 program suite (Case et al., 2018) was used to add missing hydrogens and create topology and coordinate files. The AMBER ff14SB force field (Maier et al., 2015) was used for all simulations. The protein was solvated in TIP3P water (Jorgensen et al., 1983) with a cubic box maintaining a minimum wall distance of 10 Å. Prior to production, all systems were equilibrated according to an elaborate protocol previously developed in our group (Wallnoefer et al., 2010).
All simulations were performed with the GPU implementation of the pmemd module of AMBER 18 (Salomon-Ferrer et al., 2013). To keep the target temperature of 310 K, the Langevin thermostat (Adelman and Doll, 1976) was used with a collision frequency of 2 ps−1. Furthermore, we used the Berendsen barostat with a relaxation time of 2 ps to maintain atmospheric pressure (Berendsen et al., 1984). The SHAKE algorithm was used to constrain all bonds involving hydrogens and allow the use of a 2 fs time step (Ryckaert et al., 1977) for the simulation. Long range electrostatics were treated with the particle-mesh Ewald method (PME) and a non-bonded cutoff of 8 Å was used (Darden et al., 1993).
We used well-tempered metadynamics MD (MDMD) (Barducci et al., 2008) as implemented in GROMACS version 2016.4 (Abraham et al., 2015), i.e., plumed 2.4.0 (Tribello et al., 2014), to achieve a transition from the folded to partially unfolded states. The underlying idea of MDMD is to fill up potential energy minima with a time-dependent Gaussian bias potential, which consequently lowers the energetic barriers along a selected reaction coordinate. Thereby, the conformational space of a protein is sampled much more efficiently and slow motions become accessible (Laio and Parrinello, 2006; Laio and Gervasio, 2008). In well-tempered MDMD additionally the height of the Gaussians is adaptively changed (Barducci et al., 2008). After extensive testing, the distance between the Cαs of the residues flanking the initial cleavage site, i.e., A16 and I23, was identified as suitable reaction coordinate resulting in the most efficient sampling of the local unfolding process. Gaussian functions were deposited every 1,000 steps with a height of 10.0 kcal/mol, a width of 0.35 and biasfactor of 30. To capture a diverse set of unfolding pathways we performed ten independent 10 ns well-tempered MDMD simulations for each system. For each run we assigned new starting velocities (see Supplementary Figure 1A).
The introduction of a bias potential renders the calculation of accurate thermodynamics and especially kinetic properties rather challenging (Stelzl et al., 2018). We evaluated the energetics of the captured unfolding pathways by reweighting the reaction coordinate as described by Tiwary and Parrinello (2015). However, as depicted in Supplementary Figure 2, the individual free energy curves of each system vary substantially. We surmise that this behavior stems from the varying unfolding pathways captured in the individual simulations. Due to the large differences between the runs for each system we were not able to perform reliable comparisons between systems. While the straight-forward approach of boosting the end-to-end distance of the cleavage site led to a highly efficient sampling of the unfolding process, this reaction coordinate fails to reflect this complex process in terms of reweighted free energies. We circumvent these limitations by seeding a multitude of cMD simulations along the captured transition pathway. To do so, we clustered the captured ensembles based on Cα positions using an average-linkage hierarchical clustering algorithm with a distance cutoff of 4 Å. The resulting representative structure of each cluster was then used as a starting structure for a cMD simulation of 500 ns length applying the same setup as described above (see Supplementary Figure 1B). We then combined the cMD simulations of each Bet v 1 variant by constructing a Markov state model (MSM) (Prinz et al., 2011). The construction of the MSM allows to quantify thermodynamic and kinetic properties of each ensemble without the intrinsic bias resulting from the seeding process (Bowman et al., 2013; Kohlhoff et al., 2014). Similar workflows have already been proven to be extremely efficient and highly reliable (Noe et al., 2009; Nedialkova et al., 2014; Biswas et al., 2018; Fernandez-Quintero et al., 2018; Sun et al., 2018; Zimmerman et al., 2018).
Analysis
The programs cpptraj and pytraj from the AmberTools19 suite, combined with in-house python scripts were used for analysis. The number of native contacts was calculated using mdtraj as described by Best et al. (2013). Here, we used each N and O in the backbone with a distance below 3 Å to define a native contact. We performed principle component analysis (PCA) based the distances between backbone N and O in helix 1 and helix 2 to compare structural variances. For the construction of the PCA space we combined the trajectories from all Bet v 1 variants and then projected the individual structural data of each variant onto the two largest eigenvectors (PC1 and PC2). PCA identifies the motions which account for the largest structural variance. Time-lagged independent component analysis (tICA), on the other hand, is a dimensionality-reduction technique which detects the slowest-relaxing degrees of freedom (Molgedey and Schuster, 1994; Chodera and Noe, 2014; Schwantes et al., 2016). The tICA space thus facilitates a kinetic clustering, which is prerequisite for building MSMs (Bowman et al., 2013; Shukla et al., 2015). We employed the same input features for tICA as described for PCA. We applied the implemented k-means clustering algorithm to discretize the tICA space into 100 microstates. A lag time of 10 ns was chosen, since the estimated slowest timescales are approximately independent of the lag time at that point (Pande et al., 2010; Bowman et al., 2013) (see Supplementary Figure 2). Subsequently Perron cluster cluster analysis (PCCA+) was performed to achieve an intuitive coarse-grained representation of the Bayesian MSM (Röblitz and Weber, 2013). To estimate the reliability of the calculated models we performed typical MSM validations, i.e., plotting the implied timescales as a function of lag-time as well as a Chapman-Kolmogorov test (Supplementary Figure 3). Furthermore, we show uncertainty estimates for the presented models (Supplementary Tables 1, 2) as well as the state probabilities as a function of lag time (Supplementary Figure 5). PCA, tICA and MSM analyses as well as plotting of the respective results were achieved with the pyEMMA package version 2.5.6 (Scherer et al., 2015).
Results
μs-Dynamics in the Folded State
We profile differences in the dynamics within the fully folded state of the three Bet v 1 variants with classic MD (cMD) simulations of 1 μs length. In Figure 1A we visualize similarities in the captured conformational ensembles with a 2D-RMSD plot; Blue indicates high structural similarity to the crystal structure and red denotes structural rearrangements. This analysis depicts only small fluctuations in the structure of the wild-type protein Bet v 1a. The stabilized mutant Bet v 1a-D69I, shows even subtler fold dynamics, which largely concur with the wild-type ensemble. In contrast, the 2D-RMSD of Bet v 1d, the destabilized variant, points toward a larger conformational diversity within the captured ensemble. To quantify the structural fluctuations observed in the simulations, we calculate the RMSF for each system. We find comparable dynamics for the wild-type protein and the stabilized mutant, only between residue 90 and 100 the wild-type protein is slightly more mobile. Bet v 1d on the other hand shows an overall higher flexibility than the other variants. This trend is also reflected by the number of native contacts formed during the simulations (see Figure 1C). Clearly, for the less stable variant, Bet v 1d, the distribution of native contacts is broader and shifted toward less contacts. Furthermore, we calculate the Shannon entropy of the distributions in this contact space as an alternate measure of flexibility. With an entropy of 17.4 J/ (mol·K) Bet v 1d again arises as the most flexible system, compared to Bet v 1a with 15.1 J/ (mol·K) and Bet v 1a-D69I with 13.8 J/ (mol·K).
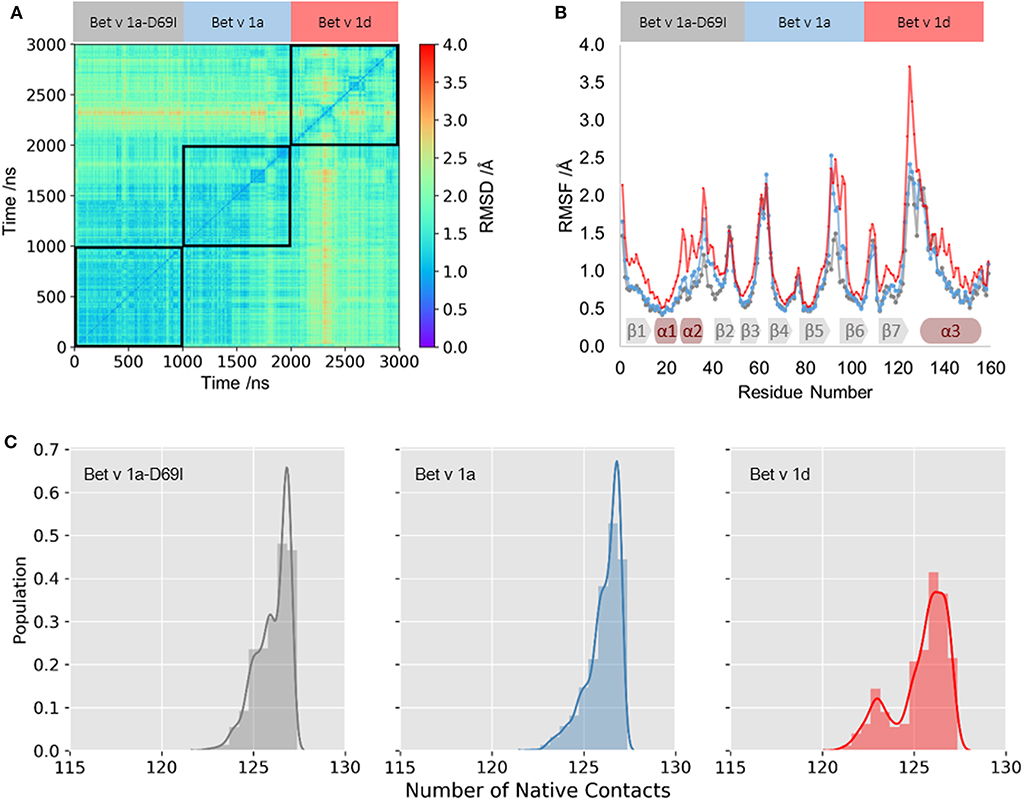
Figure 1. Fold dynamics of Bet v 1 variants. (A) The conformational space captured in 1 μs cMD simulations of the stabilized variant Bet v 1a-D69I, wild-type protein Bet v 1a and the destabilized variant Bet v 1d, respectively, was profiled via a 2D-RMSD analysis based on all Cα atoms. (B) To quantify the local differences in flexibilities we calculate the RMSF for each residue based all Cα atoms. The secondary structure elements found in the crystal structure are depicted on the bottom. (C) From the distribution of native contacts between backbone N and O atoms of helix1 and helix2 we calculate entropies as a measure of backbone rearrangements.
To further analyze the captured conformational space of each system, focusing on the early cleavage site, we construct a free energy surface in a combined PCA space as described in the methods section (Figure 2A). We find one narrow minimum, which represents the conformational ensemble of Bet v 1a-D69I. In comparison to this, the conformational space of Bet v 1a is slightly broader. However, the observed dynamics are still represented as one broad minimum in the combined PCA space. The ensemble of Bet v 1d on the other hand, is by far the most diverse and shows transitions between three separated minima.
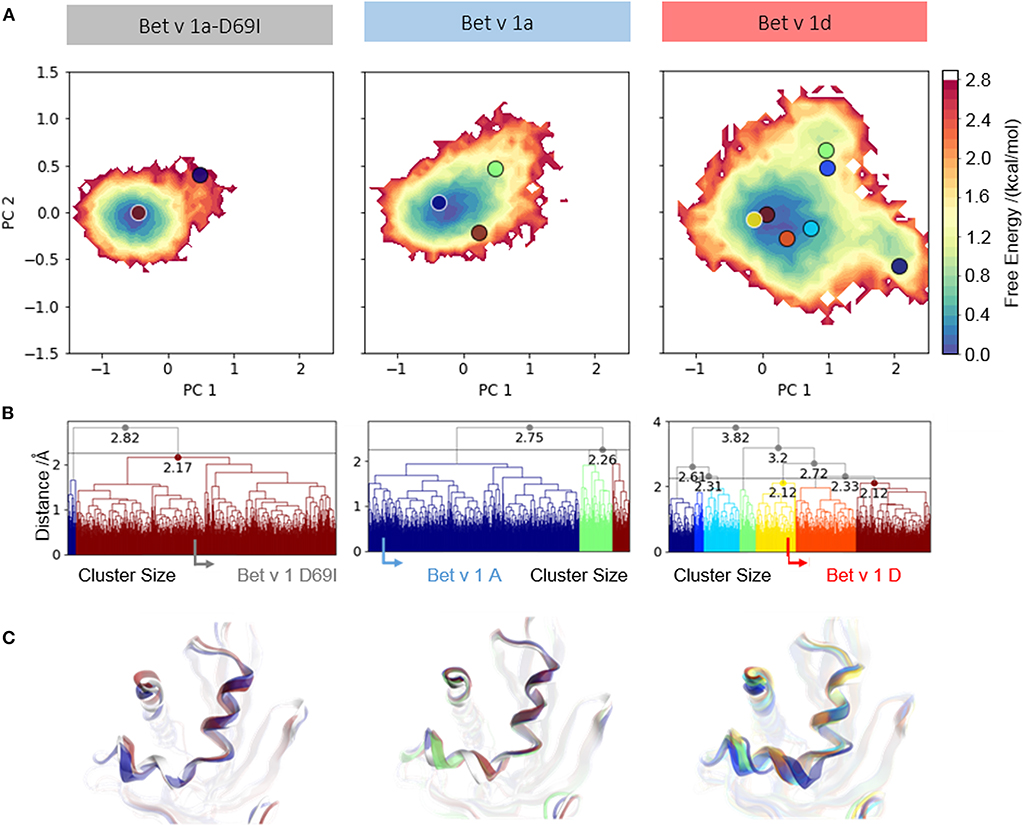
Figure 2. Conformational space of residues around the early cleavage site of Bet v 1 variants. (A) The captured ensembles were color-coded according to Gibbs free energies and projected onto the first two eigenvectors of the combined PCA space. (B) Clustering of the conformational space of helix 1 and helix 2 resulted in two clusters for the stabilized variant Bet v 1a-D69I, three clusters for the wild-type protein Bet v 1a and seven clusters for the destabilized variant Bet v 1d, at a distance cut-off of 2.5 Å. The native structure of each variant is denoted with an arrow in the respective dendrogram. (C) Representative structures were extracted from each cluster to visualize conformational differences and were projected onto the PCA space.
We cluster each trajectory applying the same distance cutoff of 2.5 Å to gain further insight into the captured structural rearrangements (Figures 2B,C). The increase in the number of resulting clusters from Bet v 1a-D69I over Bet v 1a to Bet v 1d indicates more diversity in the respective underlying data. As depicted in Figure 2C, the resulting representative structures show only marginal deviation for Bet v 1a-D69I and Bet v 1a. For Bet v 1d we find a slight shift of both helices. However, the conformational changes observed in 1 μs of cMD simulation are small, also for Bet v 1d, the proteolytically least stable system. Additionally, we project the representative structures from each cluster onto the combined PCA space in Figure 2A to link structural and energetic information.
Local Unfolding Dynamics
To profile whether we can capture a relation between proteolytic susceptibility and the thermodynamics and kinetics of partial unfolding events, we apply a combination of enhanced sampling techniques and classic MD simulations (see section Methods). This approach allows us to profile distinct features along the unfolding pathway for each Bet v 1 variant. To compare the structural diversities of each ensemble we again perform PCA on the combined trajectories. In Figure 3A structural data from each variant is projected onto the two largest eigenvectors and color-coded according to the MSM reweighted free energy. Also on this extended timescale the stabilized mutant Bet v 1a-D69I clearly shows a narrower free energy surface than the wild-type protein Bet v 1a. The conformational landscape of Bet v 1a-D69I shown in the PCA space displays one deep and distinct free energy minimum at (1, 0) and two significantly less favorable minima around (0.5, 0.2) and (−1, 0.2). The free energy landscape of the residues around the early cleavage site of Bet v 1a is much broader than for Bet v 1a-D69I, indicating a higher structural diversity. However, also for Bet v 1a we only observe one deep and distinct minimum in free energy around (1, 0). The remaining surface is characterized by rather flat and unfavorable areas. The conformational space sampled by Bet v 1d is only slightly broader than the wild-type, with the most distinct minimum again around (1, 0). However, in terms of free energy we evidently find an overall shallower and much more favorable surface for Bet v 1d. On the one hand, this analysis suggests a higher probability of partially unfolded states within the Bet v 1d ensemble. On the other hand, the projection onto the PCA space also implies lower barriers between the individual minima, i.e., conformational states. These observations are in line with the transition timescales estimated from the MSM (Figure 3B). While for Bet v 1a and Bet v 1a-D69I the fastest unfolding process takes place at timescales around 21 μs and 57 μs, Bet v 1d unfolds already within 9 μs. To obtain a more detailed view on the partial unfolding mechanism we additionally analyze the most probable transition pathway using the net reactive flux (see Supplementary Figure 2). Furthermore, we retrieve the probabilities of each conformational state via the MSM stationary distribution of each model. For the folded state a probability of 95 ± 2% is estimated for the stabilized mutant Bet v 1a-D69I, 87 ± 3% for Bet v 1a, and 65 ±6 % for Bet v 1d.
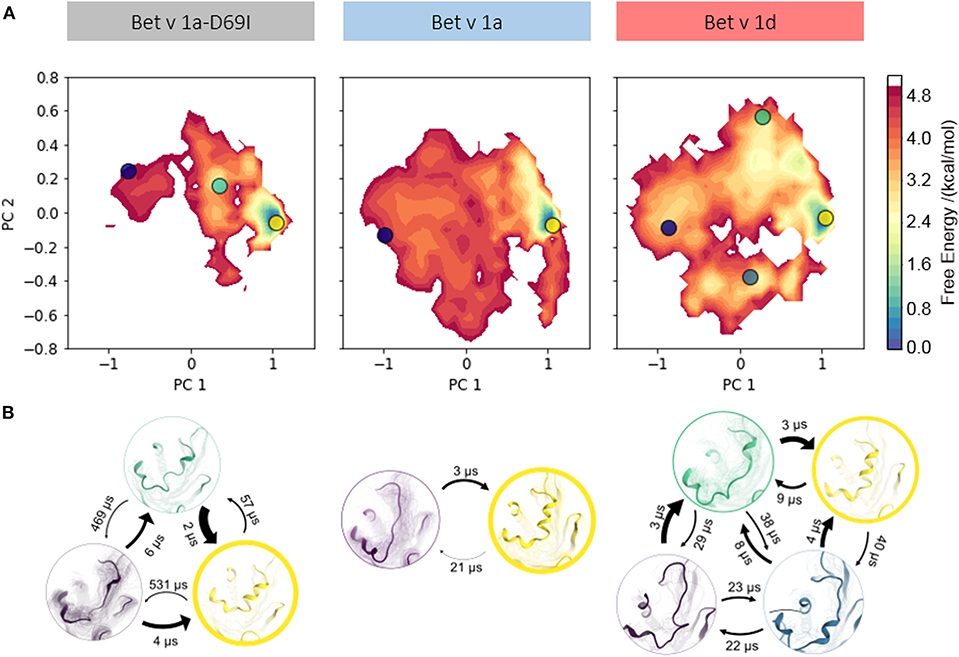
Figure 3. (A) Free energy landscape during local unfolding. The accumulated structural information from the seeded cMD simulations were color-coded according to the reweighted free energies and projected onto the first two eigenvectors of the combined PCA space. (B) We constructed an MSM of the partial unfolding process of each variant. Estimating the state populations of the stabilized variant Bet v 1a-D69I, the wild-type protein Bet v 1a and the destabilized variant Bet v 1d, we found 95 ± 2, 87 ± 3, and 65 ± 6% of the ensembles in the folded state, respectively.
Discussion
One feature which has been shown to play a decisive role in the immunogenicity of birch pollen allergens is proteolytic stability (Scheiblhofer et al., 2017). However, it is still challenging to rationalize a protein's resilience or susceptibility to proteolytic degradation based on its sequence or a single structure alone. Here, we present a simulation-based approach to illustrate and quantify features along the transition path to a partially unfolded state, which is imperative for proteolytic cleavage.
As illustrated in Figure 1, we apply various metrics to quantify the structural fluctuations of Bet v 1 variants within the folded state. The results from 2D-RMSD and RMSF clearly identify the ensemble of the proteolytically least stable variant Bet v 1d as structurally most diverse. On the other hand, a difference in the dynamics of Bet v 1a and the stabilized mutant Bet v 1a-D69I is only marginally visible from these analyses. While the 2D-RMSD illustrates varying structural rearrangements of the overall fold, the RMSF allows to localize differences of flexibilities within each structure. One of the first cleavage sites which was experimentally observed is located in the vicinity of helix 1 and helix 2, between the residues A16 and I23 (Egger et al., 2011; Freier et al., 2015; Machado et al., 2016). Strikingly, this region shows significantly increased local flexibilities for Bet v 1d in the RMSF analysis (Figure 1B). We thus, concentrate our analysis on the dynamics observed in close vicinity to this early cleavage site.
To profile structural deviations from the crystal structure of Bet v 1 we determine the number of native contacts formed and lost during the simulations for helix 1 and helix 2 (Best et al., 2013). This alignment-independent metric intuitively characterizes the fold stability of a given protein and can also be used for an in-depth study of individual secondary structure elements. As shown in Figure 1C, we observe a significantly broader distribution of formed native contacts in helix 1 and 2 for Bet v 1d. For the wild-type protein Bet v 1a and the stabilized variant Bet v 1a-D69I the number of native contacts show similar trends and are clearly shifted to higher values. These observations again suggest Bet v 1d as the most flexible variant. To quantify the distinctions between these distributions, we calculate the Shannon entropy. The increase in entropy from Bet v 1a-D69I, over Bet v 1a to Bet v 1d implies that the proteolytically least stable variant, Bet v 1d, is also the least stable in terms of native contacts.
Additionally, we compare structural variances and the associated energetics for each ensemble by constructing free energy surfaces in the combined PCA space (Figure 2A). The captured conformational space of Bet v 1d is separated into three distinct minima and significantly more extensive compared to the Bet v 1 variants with lower cleavage rates. Bet v 1a and Bet v 1a-D69I show very similar free energy landscapes, with only one deep minimum in free energy and Bet v 1a covering a slightly broader space in this projection. The structural clustering with the same cutoff distance for all systems and the subsequent projection of the representative structures onto the combined PCA space allows for a structural interpretation of the trends discussed above. The increasing number of clusters from Bet v 1a-D69I, over Bet v 1a, to Bet v 1d, and their respective populations again indicate an increase of structural fluctuations within the early cleavage site. However, upon visual inspection of the representative structures it becomes apparent that the involved structural rearrangements are almost negligible. Only for Bet v 1d we find a notable shift in the orientation of helix 2. Nevertheless, all of the applied metrics to characterize the dynamics of the three Bet v 1 variants, clearly identify the structural ensemble of Bet v 1d as most diverse. This result indicates that already dynamics on the ns- to μs-timescale could be decisive for exceptionally high proteolytic cleavage rates.
Nevertheless, the main scope of this study is to characterize the partial unfolding process, which is prerequisite for proteolytic cleavage of Bet v 1. However, as described in the introduction, it is highly unlikely to observe such extensive movements within the timescales reachable with cMD simulations. We thus apply a simulation protocol where enhanced and classic sampling techniques are combined to model the conformational rearrangements along the transition pathway to the locally unfolded conformation.
We visualize the captured free energy surfaces in a combined PCA space (see Methods). The extend of this surface thus reflects all captured structural variances and allows a direct comparison. We find that within this projection, Bet v 1a-D69I clearly displays the most restricted conformational space. Bet v 1a and Bet v 1d cover areas of similar extent, but the free energy surface of Bet v 1d presents itself as generally more favorable than Bet v 1a. This indicates that while similar conformational states are accessible for Bet v 1a and Bet v 1d, they are much more likely to occur in the ensemble of Bet v 1d. These qualitative observations can be quantified via transition timescales and stationary distributions from the MSM. The decline of transition timescales from Bet v 1a-D69I (57 μs), over Bet v 1a (21 μs) to Bet v 1d (9 μs) shown in Figure 3B directly corresponds to a decrease in barrier height between the folded and partially unfolded conformational states. However, the essential information of the constructed model lies in the thermodynamics, i.e., the probabilities, of the dynamic equilibrium between the native fold and the partially unfolded conformational states. Consistent with the previously postulated hypothesis, we find the highest population for the folded state in Bet v 1a-D69I, the stabilized variant, with 95 ± 2%. The wild-type protein Bet v 1a shows a slightly lower probability of 87 ± 3% for the folded state. The proteolytically least stable variant, Bet v 1d, indeed exhibits by far the lowest probability of being in the fully folded state with 65 ± 6%. These findings support the long-standing postulation of a link between proteolytic susceptibility and protein dynamics, i.e., local unfolding events (Freier et al., 2015; Nakamura et al., 2016; Scheiblhofer et al., 2017).
Based on sequence or a single structure alone, it is challenging to comprehend how a few mutations far from the cleavage site result in a substantial shift in proteolytic cleavage rates. However, in describing the Bet v 1 variants as conformational ensembles we find a coherent link between local unfolding probability and proteolytic susceptibility. In many experimental and computational studies this relation has already been debated (Parsell and Sauer, 1989; Thai et al., 2004; Ohkuri et al., 2010; Asam et al., 2014; Grutsch et al., 2014, 2017; Scheiblhofer et al., 2017). Yet, to the best of our knowledge, to this point no structural model of a partially unfolded state has been suggested. Here, we demonstrate that with the current advances simulation techniques and computational resources it is now possible to sample extensive portions of a proteins conformational space, including partial unfolding.
The presented workflow builds upon specific experimental data. On the one hand, a reliable starting structure for the protein in focus is imperative to perform MD simulations in general. On the other hand, this particular approach relies on knowledge of early cleavage sites, which are determinant for the initial processing of the allergen and in consequence are the focus of this study. However, in principle the presented approach is generalizable and broadly applicable. Furthermore, the required data on structure and proteolytic stability is already available for a broad range of allergen proteins (Gadermaier et al., 2011; Toda et al., 2011; Kitzmuller et al., 2015; Pablos et al., 2018). We consider the presented study as a proof of concept, that once this data is available, it is possible estimate how a particular mutation, no matter how far away from the cleavage site, impacts the cleavage rate. We thus envisage an iterative workflow where initial experimental data on the structure and proteolytic stability of one protein builds the foundation for computational models on the proteolytic stability of a multitude of mutants. These predictions can then be used to guide the experimental efforts toward the most promising candidates. The resulting cleavage data in turn can be used to refine the models and enhance prediction accuracy. Similar strategies have already demonstrated the reliability of predictions from MD simulations combined with MSM analysis on the impact of point mutations on protein stability (Zimmerman et al., 2018). Given the tremendous clinical relevance of allergen proteins we thus suggest physics-based computational models as promising tools for the design recombinant proteins for allergen-specific immunotherapy (Curin et al., 2018).
Conclusions
In this study, we employ classic and enhanced sampling techniques to test whether we are able to characterize the relation between protein dynamics and proteolytic susceptibility. Mimicking the environmental conditions of endolysosomal degradation, we observe a link between the probability of partial unfolding and proteolytic susceptibility for three Bet v 1 variants.
Based on experimental data we estimate how a particular mutation, no matter how far away from the cleavage site, impacts the conformational ensemble and thus cleavage rate. Further testing will be necessary to optimize and strengthen the presented protocol; however, the required experimental data are already available for a broad range of allergen proteins and the approach is fully generalizable. The relation of proteolytic susceptibility and immunogenicity of exogenous proteins presents a promising opportunity for the design of recombinant proteins as vaccines for allergen-specific immunotherapy (Marth et al., 2014; Valenta et al., 2016; Scheiblhofer et al., 2017). With this study, we demonstrate the feasibility of employing MD simulations to characterize structural ensembles of allergen proteins in the context of proteolytic stability.
Data Availability Statement
All datasets generated for this study are included in the article/Supplementary Material.
Author Contributions
All authors listed have made a substantial, direct and intellectual contribution to the work, and approved it for publication.
Funding
This work was supported by the Austrian Science Fund (FWF) via the grant P30565 and grant P30737.
Conflict of Interest
The authors declare that the research was conducted in the absence of any commercial or financial relationships that could be construed as a potential conflict of interest.
Acknowledgments
We express utmost gratitude to Richard Weiss from the University of Salzburg for a multitude of fruitful discussions and detailed lectures on molecular immunology. The authors acknowledge that parts of the calculations were performed on the Vienna Scientific Cluster (VSC).
Supplementary Material
The Supplementary Material for this article can be found online at: https://www.frontiersin.org/articles/10.3389/fmolb.2020.00018/full#supplementary-material
Abbreviations
Bet v 1, major birch pollen allergen (betula verrucosa); MD, molecular dynamics; MHC2, major histocompatibility complex molecules type 2; NMR, nuclear magnetic resonance; cMD, classic molecular dynamics; MDMD, metadynamics molecular dynamics; PDB, protein data bank; MSM, Markov state model; PCA, principle component analysis; tICA, time-lagged independent component analysis; PCCA+, Perron cluster cluster analysis; RMSD, root mean squared distance; RMSF, root mean squared fluctuation
References
Aalberse, R. C. (2000). Structural biology of allergens. J. Allergy Clin. Immunol. 106, 228–238. doi: 10.1067/mai.2000.108434
Abraham, M. J., Murtola, T., Schulz, R., Páll, S., Smith, J. C., Hess, B., et al. (2015). GROMACS: High performance molecular simulations through multi-level parallelism from laptops to supercomputers. SoftwareX 1, 19–25. doi: 10.1016/j.softx.2015.06.001
Ackaert, C., Kofler, S., Horejs-Hoeck, J., Zulehner, N., Asam, C., von Grafenstein, S., et al. (2014). The impact of nitration on the structure and immunogenicity of the major birch pollen allergen Bet v 1.0101. PLoS ONE 9:e104520. doi: 10.1371/journal.pone.0104520
Adelman, S. A., and Doll, J. D. (1976). Generalized langevin equation approach for atom-solid-surface scattering—general formulation for classical scattering off harmonic solids. J. Chem. Phys. 64, 2375–2388. doi: 10.1063/1.432526
Ahammer, L., Grutsch, S., Kamenik, A. S., Liedl, K. R., and Tollinger, M. (2017). Structure of the major apple allergen Mal d 1. J. Agric. Food Chem. 65, 1606–1612. doi: 10.1021/acs.jafc.6b05752
Apostolovic, D., Stanic-Vucinic, D., de Jongh, H. H., de Jong, G. A., Mihailovic, J., Radosavljevic, J., et al. (2016). Conformational stability of digestion-resistant peptides of peanut conglutins reveals the molecular basis of their allergenicity. Sci. Rep. 6:29249. doi: 10.1038/srep29249
Asam, C., Batista, A. L., Moraes, A. H., de Paula, V. S., Almeida, F. C., Aglas, L., et al. (2014). Bet v 1—a trojan horse for small ligands boosting allergic sensitization? Clin. Exp. Allergy 44, 1083–1093. doi: 10.1111/cea.12361
Barducci, A., Bussi, G., and Parrinello, M. (2008). Well-tempered metadynamics: a smoothly converging and tunable free-energy method. Phys. Rev. Lett. 100:020603. doi: 10.1103/PhysRevLett.100.020603
Berendsen, H. J. C., Postma, J. P. M., Vangunsteren, W. F., Dinola, A., and Haak, J. R. (1984). Molecular-dynamics with coupling to an external bath. J. Chem. Phys. 81, 3684–3690. doi: 10.1063/1.448118
Berkner, H., Seutter von Loetzen, C., Hartl, M., Randow, S., Gubesch, M., Vogel, L., et al. (2014). Enlarging the toolbox for allergen epitope definition with an allergen-type model protein. PLoS ONE 9:e111691. doi: 10.1371/journal.pone.0111691
Best, R. B., Hummer, G., and Eaton, W. A. (2013). Native contacts determine protein folding mechanisms in atomistic simulations. Proc. Natl. Acad. Sci. U.S.A. 110, 17874–17879. doi: 10.1073/pnas.1311599110
Biedermann, T., Winther, L., Till, S. J., Panzner, P., Knulst, A., and Valovirta, E. (2019). Birch pollen allergy in Europe. Allergy 74, 1237–1248. doi: 10.1111/all.13758
Biswas, M., Lickert, B., and Stock, G. (2018). Metadynamics enhanced markov modeling of protein dynamics. J. Phys. Chem. B 122, 5508–5514. doi: 10.1021/acs.jpcb.7b11800
Boehr, D. D., Nussinov, R., and Wright, P. E. (2009). The role of dynamic conformational ensembles in biomolecular recognition. Nat. Chem. Biol. 5, 789–796. doi: 10.1038/nchembio.232
Bollen, M. A., Wichers, H. J., Helsper, J. P. F. G., Savelkoul, H. F. J., and van Boekel, M. A. J. S. (2010). Thermodynamic characterization of the PR-10 allergens Bet v 1, Api g 1 and Dau c 1 and pH-dependence of nApi g 1 and nDau c 1. Food Chem. 119, 241–248. doi: 10.1016/j.foodchem.2009.06.013
Bowman, G. R. (2016). Accurately modeling nanosecond protein dynamics requires at least microseconds of simulation. J. Comput. Chem. 37, 558–566. doi: 10.1002/jcc.23973
Bowman, G. R., Pande, V. S., and Noé, F. (2013). An Introduction to Markov State Models and Their Application to Long Timescale Molecular Simulation. Dordrecht: Springer Science & Business Media. doi: 10.1007/978-94-007-7606-7
Case, D. A., Ben-Shalom, I. Y., Brozell, S. R., Cerutti, D. S., Cheatham, T. E. III, Cruzeiro, V. W. D., et al. (2018). AMBER 2018. San Francisco, CA: University of California.
Chodera, J. D., and Noe, F. (2014). Markov state models of biomolecular conformational dynamics. Curr. Opin. Struct. Biol. 25, 135–144. doi: 10.1016/j.sbi.2014.04.002
Conus, S., and Simon, H. U. (2010). Cathepsins and their involvement in immune responses. Swiss Med. Wkly. 140:w13042. doi: 10.4414/smw.2010.13042
Curin, M., Khaitov, M., Karaulov, A., Namazova-Baranova, L., Campana, R., Garib, V., et al. (2018). Next-generation of allergen-specific immunotherapies: molecular approaches. Curr. Allergy Asthma Rep. 18:39. doi: 10.1007/s11882-018-0790-x
Darden, T., York, D., and Pedersen, L. (1993). Particle mesh ewald - an N.Log(N) method for ewald sums in large systems. J. Chem. Phys. 98, 10089–10092. doi: 10.1063/1.464397
Dror, R. O., Dirks, R. M., Grossman, J. P., Xu, H., and Shaw, D. E. (2012). Biomolecular simulation: a computational microscope for molecular biology. Annu. Rev. Biophys. 41, 429–452. doi: 10.1146/annurev-biophys-042910-155245
Egger, M., Jurets, A., Wallner, M., Briza, P., Ruzek, S., Hainzl, S., et al. (2011). Assessing protein immunogenicity with a dendritic cell line-derived endolysosomal degradome. PLoS ONE 6:e17278. doi: 10.1371/journal.pone.0017278
Eichhorn, S., Horschlager, A., Steiner, M., Laimer, J., Jensen, B. M., Versteeg, S. A., et al. (2019). Rational design, structure-activity relationship, and immunogenicity of hypoallergenic Pru p 3 variants. Mol. Nutr. Food Res. 63:e1900336. doi: 10.1002/mnfr.201900336
Fernandez-Quintero, M. L., Loeffler, J. R., Kraml, J., Kahler, U., Kamenik, A. S., and Liedl, K. R. (2018). Characterizing the diversity of the CDR-H3 loop conformational ensembles in relationship to antibody binding properties. Front. Immunol. 9:3065. doi: 10.3389/fimmu.2018.03065
Ferrari, E., Casali, E., Burastero, S. E., Spisni, A., and Pertinhez, T. A. (2016). The allergen mus m 1.0102: dissecting the relationship between molecular conformation and allergenic potency. Biochim. Biophys. Acta 1864, 1548–1557. doi: 10.1016/j.bbapap.2016.08.003
Foo, A. C. Y., Thompson, P. M., Perera, L., Arora, S., DeRose, E. F., Williams, J., et al. (2019). Hydrophobic ligands influence the structure, stability, and processing of the major cockroach allergen Bla g 1. Sci. Rep. 9:18294. doi: 10.1038/s41598-019-54689-8
Freier, R., Dall, E., and Brandstetter, H. (2015). Protease recognition sites in Bet v 1a are cryptic, explaining its slow processing relevant to its allergenicity. Sci. Rep. 5:12707. doi: 10.1038/srep12707
Gadermaier, G., Hauser, M., Egger, M., Ferrara, R., Briza, P., Santos, K. S., et al. (2011). Sensitization prevalence, antibody cross-reactivity and immunogenic peptide profile of Api g 2, the non-specific lipid transfer protein 1 of celery. PLoS ONE 6:e24150. doi: 10.1371/journal.pone.0024150
Gajhede, M., Osmark, P., Poulsen, F. M., Ipsen, H., Larsen, J. N., Joost van Neerven, R. J., et al. (1996). X-ray and NMR structure of Bet v 1, the origin of birch pollen allergy. Nat. Struct. Biol. 3, 1040–1045. doi: 10.1038/nsb1296-1040
Garrido-Arandia, M., Gomez-Casado, C., Diaz-Perales, A., and Pacios, L. F. (2014). Molecular dynamics of major allergens from alternaria, birch pollen and peach. Mol. Inform. 33, 682–694. doi: 10.1002/minf.201400057
Grutsch, S., Fuchs, J. E., Ahammer, L., Kamenik, A. S., Liedl, K. R., and Tollinger, M. (2017). Conformational flexibility differentiates naturally occurring Bet v 1 isoforms. Int. J. Mol. Sci. 18:1192. doi: 10.3390/ijms18061192
Grutsch, S., Fuchs, J. E., Freier, R., Kofler, S., Bibi, M., Asam, C., et al. (2014). Ligand binding modulates the structural dynamics and compactness of the major birch pollen allergen. Biophys. J. 107, 2972–2981. doi: 10.1016/j.bpj.2014.10.062
Hart, K. M., Ho, C. M., Dutta, S., Gross, M. L., and Bowman, G. R. (2016). Modelling proteins' hidden conformations to predict antibiotic resistance. Nat. Commun. 7:12965. doi: 10.1038/ncomms12965
Hartl, A., Hochreiter, R., Stepanoska, T., Ferreira, F., and Thalhamer, J. (2004). Characterization of the protective and therapeutic efficiency of a DNA vaccine encoding the major birch pollen allergen Bet v 1a. Allergy 59, 65–73. doi: 10.1046/j.1398-9995.2003.00335.x
Henzler-Wildman, K., and Kern, D. (2007). Dynamic personalities of proteins. Nature 450, 964–972. doi: 10.1038/nature06522
Hofer, F., Dietrich, V., Kamenik, A. S., Tollinger, M., and Liedl, K. R. (2019). pH-Dependent protonation of the Phl p 6 pollen allergen studied by NMR and cpH-aMD. J. Chem. Theory Comput. 15, 5716–5726. doi: 10.1021/acs.jctc.9b00540
Hsing, L. C., and Rudensky, A. Y. (2005). The lysosomal cysteine proteases in MHC class II antigen presentation. Immunol. Rev. 207, 229–241. doi: 10.1111/j.0105-2896.2005.00310.x
Husslik, F., Hanschmann, K. M., Kramer, A., Seutter von Loetzen, C., Schweimer, K., Bellinghausen, I., et al. (2015). Folded or not? Tracking Bet v 1 conformation in recombinant allergen preparations. PLoS ONE 10:e0132956. doi: 10.1371/journal.pone.0132956
Jacob, T., von Loetzen, C. S., Reuter, A., Lacher, U., Schiller, D., Schobert, R., et al. (2019). Identification of a natural ligand of the hazel allergen Cor a 1. Sci. Rep. 9:8714. doi: 10.1038/s41598-019-44999-2
Jorgensen, W. L., Chandrasekhar, J., Madura, J. D., Impey, R. W., and Klein, M. L. (1983). Comparison of simple potential functions for simulating liquid water. J. Chem. Phys. 79, 926–935. doi: 10.1063/1.445869
Kamenik, A. S., Kahler, U., Fuchs, J. E., and Liedl, K. R. (2016). Localization of millisecond dynamics: dihedral entropy from accelerated MD. J. Chem. Theory Comput. 12, 3449–3455. doi: 10.1021/acs.jctc.6b00231
Karplus, M., and Weaver, D. L. (1976). Protein-folding dynamics. Nature 260, 404–406. doi: 10.1038/260404a0
Kitzmuller, C., Zulehner, N., Roulias, A., Briza, P., Ferreira, F., Fae, I., et al. (2015). Correlation of sensitizing capacity and T-cell recognition within the Bet v 1 family. J. Allergy Clin. Immunol. 136, 151–158. doi: 10.1016/j.jaci.2014.12.1928
Kofler, S., Asam, C., Eckhard, U., Wallner, M., Ferreira, F., and Brandstetter, H. (2012). Crystallographically mapped ligand binding differs in high and low IgE binding isoforms of birch pollen allergen bet v 1. J. Mol. Biol. 422, 109–123. doi: 10.1016/j.jmb.2012.05.016
Kohlhoff, K. J., Shukla, D., Lawrenz, M., Bowman, G. R., Konerding, D. E., Belov, D., et al. (2014). Cloud-based simulations on google exacycle reveal ligand modulation of GPCR activation pathways. Nat. Chem. 6, 15–21. doi: 10.1038/nchem.1821
Labute, P. (2009). Protonate3D: assignment of ionization states and hydrogen coordinates to macromolecular structures. Proteins 75, 187–205. doi: 10.1002/prot.22234
Laio, A., and Gervasio, F. L. (2008). Metadynamics: a method to simulate rare events and reconstruct the free energy in biophysics, chemistry and material science. Rep. Prog. Phys. 71:126601. doi: 10.1088/0034-4885/71/12/126601
Laio, A., and Parrinello, M. (2006). “Computing free energies and accelerating rare events with metadynamics,” in Computer Simulations in Condensed Matter Systems: From Materials to Chemical Biology, Vol. 1, eds M. Ferrario, G. Ciccotti, and K. Binder (Berlin; Heidelberg: Springer), 315–347.
Latorraca, N. R., Venkatakrishnan, A. J., and Dror, R. O. (2017). GPCR dynamics: structures in motion. Chem. Rev. 117, 139–155. doi: 10.1021/acs.chemrev.6b00177
Leone, V., Marinelli, F., Carloni, P., and Parrinello, M. (2010). Targeting biomolecular flexibility with metadynamics. Curr. Opin. Struct. Biol. 20, 148–154. doi: 10.1016/j.sbi.2010.01.011
Lu, W., Negi, S. S., Schein, C. H., Maleki, S. J., Hurlburt, B. K., and Braun, W. (2018). Distinguishing allergens from non-allergenic homologues using Physical-Chemical Property (PCP) motifs. Mol. Immunol. 99, 1–8. doi: 10.1016/j.molimm.2018.03.022
Machado, Y., Freier, R., Scheiblhofer, S., Thalhamer, T., Mayr, M., Briza, P., et al. (2016). Fold stability during endolysosomal acidification is a key factor for allergenicity and immunogenicity of the major birch pollen allergen. J. Allergy Clin. Immunol. 137, 1525–1534. doi: 10.1016/j.jaci.2015.09.026
Madala, P. K., Tyndall, J. D., Nall, T., and Fairlie, D. P. (2010). Update 1 of: proteases universally recognize beta strands in their active sites. Chem. Rev. 110, PR1–PR31. doi: 10.1021/cr900368a
Maier, J. A., Martinez, C., Kasavajhala, K., Wickstrom, L., Hauser, K. E., and Simmerling, C. (2015). ff14SB: Improving the accuracy of protein side chain and backbone parameters from ff99SB. J. Chem. Theory Comput. 11, 3696–3713. doi: 10.1021/acs.jctc.5b00255
Marković-Housley, Z., Degano, M., Lamba, D., von Roepenack-Lahaye, E., Clemens, S., Susani, M., et al. (2003). Crystal structure of a hypoallergenic isoform of the major birch pollen allergen Bet v 1 and its likely biological function as a plant steroid carrier. J. Mol. Biol. 325, 123–133. doi: 10.1016/S0022-2836(02)01197-X
Marth, K., Focke-Tejkl, M., Lupinek, C., Valenta, R., and Niederberger, V. (2014). Allergen peptides, recombinant allergens and hypoallergens for allergen-specific immunotherapy. Curr. Treat Options Allergy 1, 91–106. doi: 10.1007/s40521-013-0006-5
Mitropoulou, A. N., Bowen, H., Dodev, T. S., Davies, A. M., Bax, H. J., Beavil, R. L., et al. (2018). Structure of a patient-derived antibody in complex with allergen reveals simultaneous conventional and superantigen-like recognition. Proc. Natl. Acad. Sci. U.S.A. 115, E8707–E8716. doi: 10.1073/pnas.1806840115
MOE (2019). MOE (Molecular Operating Environment), 1st Edn. Montréal, QC: Chemical Computing Group (CCG). doi: 10.4324/9780429311604-1
Mogensen, J. E., Wimmer, R., Larsen, J. N., Spangfort, M. D., and Otzen, D. E. (2002). The major birch allergen, Bet v 1, shows affinity for a broad spectrum of physiological ligands. J. Biol. Chem. 277, 23684–23692. doi: 10.1074/jbc.M202065200
Moingeon, P., Floch, V. B., Airouche, S., Baron-Bodo, V., Nony, E., and Mascarell, L. (2016). Allergen immunotherapy for birch pollen-allergic patients: recent advances. Immunotherapy 8, 555–567. doi: 10.2217/imt-2015-0027
Molgedey, L., and Schuster, H. G. (1994). Separation of a mixture of independent signals using time delayed correlations. Phys. Rev. Lett. 72, 3634–3637. doi: 10.1103/PhysRevLett.72.3634
Moraes, A. H., Asam, C., Almeida, F. C. L., Wallner, M., Ferreira, F., and Valente, A. P. (2018). Structural basis for cross-reactivity and conformation fluctuation of the major beech pollen allergen Fag s 1. Sci. Rep. 8:10512. doi: 10.1038/s41598-018-28358-1
Nakamura, H., Ohkuri, T., So, T., and Ueda, T. (2016). Relationship between the magnitude of IgE production in mice and conformational stability of the house dust mite allergen, Der p 2. Biochim. Biophys. Acta 1860, 2279–2284. doi: 10.1016/j.bbagen.2016.04.014
Nedialkova, L. V., Amat, M. A., Kevrekidis, I. G., and Hummer, G. (2014). Diffusion maps, clustering and fuzzy markov modeling in peptide folding transitions. J. Chem. Phys. 141:114102. doi: 10.1063/1.4893963
Noe, F., Schutte, C., Vanden-Eijnden, E., Reich, L., and Weikl, T. R. (2009). Constructing the equilibrium ensemble of folding pathways from short off-equilibrium simulations. Proc. Natl. Acad. Sci. U.S.A. 106, 19011–19016. doi: 10.1073/pnas.0905466106
Ohkuri, T., Nagatomo, S., Oda, K., So, T., Imoto, T., and Ueda, T. (2010). A protein's conformational stability is an immunologically dominant factor: evidence that free-energy barriers for protein unfolding limit the immunogenicity of foreign proteins. J. Immunol. 185, 4199–4205. doi: 10.4049/jimmunol.0902249
Pablos, I., Eichhorn, S., Machado, Y., Briza, P., Neunkirchner, A., Jahn-Schmid, B., et al. (2018). Distinct epitope structures of defensin-like proteins linked to proline-rich regions give rise to differences in their allergenic activity. Allergy 73, 431–441. doi: 10.1111/all.13298
Pande, V. S., Beauchamp, K., and Bowman, G. R. (2010). Everything you wanted to know about Markov State models but were afraid to ask. Methods 52, 99–105. doi: 10.1016/j.ymeth.2010.06.002
Parsell, D. A., and Sauer, R. T. (1989). The structural stability of a protein is an important determinant of its proteolytic susceptibility in Escherichia-coli. J. Biol. Chem. 264, 7590–7595.
Pekar, J., Ret, D., and Untersmayr, E. (2018). Stability of allergens. Mol. Immunol. 100, 14–20. doi: 10.1016/j.molimm.2018.03.017
Pree, I., Reisinger, J., Focke, M., Vrtala, S., Pauli, G., van Hage, M., et al. (2007). Analysis of epitope-specific immune responses induced by vaccination with structurally folded and unfolded recombinant Bet v 1 allergen derivatives in man. J. Immunol. 179, 5309–5316. doi: 10.4049/jimmunol.179.8.5309
Prinz, J. H., Wu, H., Sarich, M., Keller, B., Senne, M., Held, M., et al. (2011). Markov models of molecular kinetics: generation and validation. J. Chem. Phys. 134:174105. doi: 10.1063/1.3565032
Röblitz, S., and Weber, M. (2013). Fuzzy spectral clustering by PCCA+: application to Markov state models and data classification. Adv. Data Anal. Classif. 7, 147–179. doi: 10.1007/s11634-013-0134-6
Ryckaert, J.-P., Ciccotti, G., and Berendsen, H. J. (1977). Numerical integration of the cartesian equations of motion of a system with constraints: molecular dynamics of n-alkanes. J. Comput. Phys. 23, 327–341. doi: 10.1016/0021-9991(77)90098-5
Salomon-Ferrer, R., Gotz, A. W., Poole, D., Le Grand, S., and Walker, R. C. (2013). Routine microsecond molecular dynamics simulations with AMBER on GPUs. 2. explicit solvent particle mesh ewald. J. Chem. Theory Comput. 9, 3878–3888. doi: 10.1021/ct400314y
Scheiblhofer, S., Laimer, J., Machado, Y., Weiss, R., and Thalhamer, J. (2017). Influence of protein fold stability on immunogenicity and its implications for vaccine design. Expert Rev. Vaccines 16, 479–489. doi: 10.1080/14760584.2017.1306441
Scherer, M. K., Trendelkamp-Schroer, B., Paul, F., Perez-Hernandez, G., Hoffmann, M., Plattner, N., et al. (2015). PyEMMA 2: A software package for estimation, validation, and analysis of markov models. J. Chem. Theory Comput. 11, 5525–5542. doi: 10.1021/acs.jctc.5b00743
Scheurer, S., Toda, M., and Vieths, S. (2015). What makes an allergen? Clin. Exp. Allergy 45, 1150–1161. doi: 10.1111/cea.12571
Schwantes, C. R., Shukla, D., and Pande, V. S. (2016). Markov state models and tICA reveal a nonnative folding nucleus in simulations of NuG2. Biophys. J. 110, 1716–1719. doi: 10.1016/j.bpj.2016.03.026
Schweimer, K., Sticht, H., Nerkamp, J., Boehm, M., Breitenbach, M., Vieths, S., et al. (1999). NMR spectroscopy reveals common structural features of the birch pollen allergen Bet v 1 and the cherry allergen Pru a 1. Appl. Magnet. Reson. 17, 449–464. doi: 10.1007/BF03162177
Seutter von Loetzen, C., Reuter, A., Spiric, J., Schulenborg, T., Bellinghausen, I., Volker, E., et al. (2019). Quality and potency profile of eight recombinant isoallergens, largely mimicking total Bet v 1-specific IgE binding of birch pollen. Clin. Exp. Allergy 49, 712–723. doi: 10.1111/cea.13356
Shukla, D., Hernandez, C. X., Weber, J. K., and Pande, V. S. (2015). Markov state models provide insights into dynamic modulation of protein function. Acc. Chem. Res. 48, 414–422. doi: 10.1021/ar5002999
Soh, W. T., Aglas, L., Mueller, G. A., Gilles, S., Weiss, R., Scheiblhofer, S., et al. (2019). Multiple roles of Bet v 1 ligands in allergen stabilization and modulation of endosomal protease activity. Allergy 74, 2382–2393. doi: 10.1111/all.13948
Spangfort, M. D., Mirza, O., Ipsen, H., Van Neerven, R. J., Gajhede, M., and Larsen, J. N. (2003). Dominating IgE-binding epitope of Bet v 1, the major allergen of birch pollen, characterized by X-ray crystallography and site-directed mutagenesis. J. Immunol. 171, 3084–3090. doi: 10.4049/jimmunol.171.6.3084
Stelzl, L. S., Kells, A., Rosta, E., and Hummer, G. (2018). Dynamic histogram analysis to determine free energies and rates from biased simulations. Biophys. J. 114, 557a−558a. doi: 10.1016/j.bpj.2017.11.3047
Sun, X., Singh, S., Blumer, K. J., and Bowman, G. R. (2018). Simulation of spontaneous G protein activation reveals a new intermediate driving GDP unbinding. Elife 7:e38465. doi: 10.7554/eLife.38465.031
Thai, R., Moine, G., Desmadril, M., Servent, D., Tarride, J. L., Menez, A., et al. (2004). Antigen stability controls antigen presentation. J. Biol. Chem. 279, 50257–50266. doi: 10.1074/jbc.M405738200
Tiwary, P., and Parrinello, M. (2015). A time-independent free energy estimator for metadynamics. J. Phys. Chem. B 119, 736–742. doi: 10.1021/jp504920s
Toda, M., Reese, G., Gadermaier, G., Schulten, V., Lauer, I., Egger, M., et al. (2011). Protein unfolding strongly modulates the allergenicity and immunogenicity of Pru p 3, the major peach allergen. J. Allergy Clin. Immunol. 128, 1022–1030 e1021–1027. doi: 10.1016/j.jaci.2011.04.020
Tribello, G. A., Bonomi, M., Branduardi, D., Camilloni, C., and Bussi, G. (2014). PLUMED 2: New feathers for an old bird. Comp. Phys. Commun. 185, 604–613. doi: 10.1016/j.cpc.2013.09.018
Tscheppe, A., Palmberger, D., van Rijt, L., Kalic, T., Mayr, V., Palladino, C., et al. (2020). Development of a novel Ara h 2 hypoallergen with no IgE binding or anaphylactogenic activity. J. Allergy Clin. Immunol. 145, 229–238. doi: 10.1016/j.jaci.2019.08.036
Valenta, R. (2002). The future of antigen-specific immunotherapy of allergy. Nat. Rev. Immunol. 2, 446–453. doi: 10.1038/nri824
Valenta, R., Campana, R., Focke-Tejkl, M., and Niederberger, V. (2016). Vaccine development for allergen-specific immunotherapy based on recombinant allergens and synthetic allergen peptides: lessons from the past and novel mechanisms of action for the future. J. Allergy Clin. Immunol. 137, 351–357. doi: 10.1016/j.jaci.2015.12.1299
Valenta, R., Ferreira, F., Focke-Tejkl, M., Linhart, B., Niederberger, V., Swoboda, I., et al. (2010). From allergen genes to allergy vaccines. Annu. Rev. Immunol. 28, 211–241. doi: 10.1146/annurev-immunol-030409-101218
Verhoeckx, K., Bogh, K. L., Dupont, D., Egger, L., Gadermaier, G., Larre, C., et al. (2019). The relevance of a digestibility evaluation in the allergenicity risk assessment of novel proteins. opinion of a joint initiative of COST action ImpARAS and COST action INFOGEST. Food Chem. Toxicol. 129, 405–423. doi: 10.1016/j.fct.2019.04.052
von Loetzen, C. S., Hoffmann, T., Hartl, M. J., Schweimer, K., Schwab, W., Rösch, P., et al. (2014). Secret of the major birch pollen allergen Bet v 1: identification of the physiological ligand. Biochem. J. 457, 379–390. doi: 10.1042/BJ20130413
von Loetzen, C. S., Jacob, T., Hartl-Spiegelhauer, O., Vogel, L., Schiller, D., Spörlein-Güttler, C., et al. (2015). Ligand recognition of the major birch pollen allergen bet v 1 is isoform dependent. PLoS ONE 10:e0128677. doi: 10.1371/journal.pone.0128677
Wagner, S., Radauer, C., Bublin, M., Hoffmann-Sommergruber, K., Kopp, T., Greisenegger, E. K., et al. (2008). Naturally occurring hypoallergenic Bet v 1 isoforms fail to induce IgE responses in individuals with birch pollen allergy. J. Allergy Clin. Immunol. 121, 246–252. doi: 10.1016/j.jaci.2007.08.006
Wallnoefer, H. G., Handschuh, S., Liedl, K. R., and Fox, T. (2010). Stabilizing of a globular protein by a highly complex water network: a molecular dynamics simulation study on factor Xa. J. Phys. Chem. B 114, 7405–7412. doi: 10.1021/jp101654g
Watts, C. (2001). Antigen processing in the endocytic compartment. Curr. Opin. Immunol. 13, 26–31. doi: 10.1016/S0952-7915(00)00177-1
Watts, C. (2004). The exogenous pathway for antigen presentation on major histocompatibility complex class II and CD1 molecules. Nat. Immunol. 5, 685–692. doi: 10.1038/ni1088
Wolf, M., Aglas, L., Twaroch, T. E., Steiner, M., Huber, S., Hauser, M., et al. (2018). Endolysosomal protease susceptibility of Amb a 1 as a determinant of allergenicity. J. Allergy Clin. Immunol. 141, 1488–1491. e1485. doi: 10.1016/j.jaci.2017.10.027
Keywords: allergen proteins, molecular dynamics simulations, unfolding, proteolytic cleavage, enhanced sampling, Markov state models
Citation: Kamenik AS, Hofer F, Handle PH and Liedl KR (2020) Dynamics Rationalize Proteolytic Susceptibility of the Major Birch Pollen Allergen Bet v 1. Front. Mol. Biosci. 7:18. doi: 10.3389/fmolb.2020.00018
Received: 14 November 2019; Accepted: 31 January 2020;
Published: 20 February 2020.
Edited by:
Annalisa Pastore, King's College London, United KingdomReviewed by:
Paul Roesch, University of Bayreuth, GermanySimon Olsson, Freie Universität Berlin, Germany
Copyright © 2020 Kamenik, Hofer, Handle and Liedl. This is an open-access article distributed under the terms of the Creative Commons Attribution License (CC BY). The use, distribution or reproduction in other forums is permitted, provided the original author(s) and the copyright owner(s) are credited and that the original publication in this journal is cited, in accordance with accepted academic practice. No use, distribution or reproduction is permitted which does not comply with these terms.
*Correspondence: Klaus R. Liedl, klaus.liedl@uibk.ac.at