- 1Student Research Committee, Faculty of Medicine, Hormozgan University of Medical Sciences, Bandar Abbas, Iran
- 2Student Research Committee, Department of Clinical Biochemistry, School of Medicine, Shahid Beheshti University of Medical Sciences, Tehran, Iran
- 3Department of Internal Medicine, Faculty of Medicine, Hormozgan University of Medical Sciences, Bandar Abbas, Iran
- 4Student Research Committee, Faculty of Para-Medicine, Hormozgan University of Medical Sciences, Bandar Abbas, Iran
- 5Department of Medical Genetics, Faculty of Medicine, Hormozgan University of Medical Sciences, Bandar Abbas, Iran
- 6Molecular Medicine Research Center, Hormozgan Health Institute, Hormozgan University of Medical Sciences, Bandar Abbas, Iran
Background: Alzheimer’s disease (AD) is a degenerative condition characterized by progressive cognitive impairment and dementia. Findings have revolutionized current knowledge of miRNA in the neurological conditions. Two regulatory mechanisms determine the level of mature miRNA expression; one is miRNA precursor processing, and the other is gene expression regulation by transcription factors. This study is allocated to the in-silico investigation of miRNA’s SNPs and their effect on other cell mechanisms.
Methods: We used databases which annotate the functional effect of SNPs on mRNA-miRNA and miRNA-RBP interaction. Also, we investigated SNPs which are located on the promoter or UTR region.
Results: miRNA SNP3.0 database indicated several SNPs in miR-339 and miR-34a in the upstream and downstream of pre-miRNA and mature miRNAs. While, for some miRNAs miR-124, and miR-125, no polymorphism was observed, and also miR-101 with ΔG -3.1 and mir-328 with ΔG 5.8 had the highest and lowest potencies to produce mature microRNA. SNP2TFBS web-server presented several SNPs which altered the Transcription Factor Binding Sites (TFBS) or generated novel TFBS in the promoter regions of related miRNA. At last, RBP-Var database provided a list of SNPs which alter miRNA-RBP interaction pattern and can also influence other miRNAs’ expression.
Discussion: The results indicated that SNPs microRNA affects both miRNA function and miRNA expression. Our study expands molecular insight into how SNPs in different parts of miRNA, including the regulatory (promoter), the precursor (pre-miRNA), functional regions (seed region of mature miRNA), and RBP-binding motifs, which theoretically may be correlated to the Alzheimer’s disease.
Introduction
Alzheimer’s disease (AD) is a chronic neurodegenerative disease which slowly develops and worsens during the time. This disease manifests itself in the gradual and progressive loss of consciousness and memory. Currently, the prevalence of Alzheimer’s disease among middle-aged people in developed countries is about 5.1% (Mirzaii-Fini et al., 2018). Increasing life expectancy has led to an increase in people over the age of 60 in the world, as well as an increase in the prevalence of neurological diseases such as dementia. Based on a 2015 Alzheimer’s report, it is projected to reach more than 130 million people in the world by 2050 (Podhorna et al., 2020).
miRNAs, short double-stranded RNAs (dsRNA) about 18-24 nucleotides in length, negatively regulate the gene expression by direct binding 3′-untranslated region (UTR) of target messenger RNA (mRNA) and reduce its stability and translatability. This process is governed by the seed region (positions among 2nd-8th in miRNA) of miRNA (John et al., 2004). Several miRNAs have function in various processes including cell proliferation, cell death, lipid metabolism, neural pattern, hematopoietic differentiation, and immunity (Wahid et al., 2010). In recent years, studies have focused on the role of microRNAs in the complex diseases such as neurodegenerative diseases (Femminella et al., 2015). Several miRNAs regulate the genes which involved in the development of Alzheimer’s disease (Reddy et al., 2017).
The seed sequence binding to the target occurs in various ways which can be complete or incomplete (Witkos et al., 2011). Since miRNAs are small functional units, a single base change in both precursor blocks, as well as the mature miRNA sequence, may affect microRNAs evolution resulting in producing novel miRNA by different biological functions (Dong et al., 2013). Mutation in pri or pre-miRNA may affect the stability or processing of miRNA or mRNA. Mutation in the pri-mRNA or Cisor trans promoter may affect mature miRNAs’ transcription rate (Georges et al., 2007). The presence of SNPs in the miRNA’s seed regions is considerably influenced the miRNA’s target loss and gain (generates a novel repertoire of target genes); thus, altering the miRNA biological function significantly (Xu et al., 2013; Zhang Y. et al., 2019). Transcription factors (TFs) are the fundamental regulators of biological mechanisms which bind to transcriptional regulatory motifs (e.g., promoters, enhancers) to regulate their target genes’ expression in a sequence-specific manner (Lambert et al., 2018). Since the interaction of TFs and TF binding sites is integrated into gene regulatory systems, the variations at the TF or binding site alter this interaction and may lead to increasing or reducing the number of TFs by specific binding preferences; ultimately, impaired gene expression (Buroker et al., 2015). The biogenesis and maturation pathway of miRNA is a highly regulated mechanism. RNA-binding proteins (RBPs) are potent effectors which play a significant role in optimal miRNA biogenesis and function pathways in several sequential steps, including their efficient precursor’s processing, transfer, subcellular location, degradation, and biological activity and specificity (Van Kouwenhove et al., 2011; Treiber et al., 2017). SNPs may affect RBP-mediated post-transcriptional regulatory processes of gene expression via several mechanisms, including altering miRNA-target interaction, secondary RNA structure stability, and RBP-miRNA interplay (Figure 1; Mao et al., 2016; Treiber et al., 2017). SNPs located on the gene or its promoter, and these SNPs can also be associated to some diseases (Boutz et al., 2007; Delay et al., 2011; Roy and Mallick, 2017).
This study aims to investigate in-silico analysis of SNPs in miRNAs which control the genes involved in Alzheimer’s disease and possibly damage neuronal cells. For this purpose, we computationally evaluated the functional effect of polymorphisms in these miRNAs controlling the neurodegenerative function. The results may be useful to determine candidate SNPs for further functional analyzing and investigating causal SNPs underlying Alzheimer’s and developing hypotheses and testing to develop Alzheimer’s treatments.
Materials and Methods
Selection of miRNAs That Involve in Alzheimer
Hormozgan University of Medical Science’s ethics committee approved this research (ethical code: IR/HUMS.REC.270). Upstream miRNAs of genes directly involved in Alzheimer’s disease has been gained from recent review article and other major joints. In this study, PubMed, Embase, ScienceDirect, Cochrane Library, and Google Scholar databases were reviewed. Relevant keywords including microRNA, miRNA, AND Alzheimer’s disease, were used applying Medical Subject Heading (MeSH); finally we selected the articles to investigate the relationship among these microRNAs in Alzheimer’s disease. These miRNAs are recognized to be associated to Alzheimer’s disease and neurodegeneration.
miRNA Involvement in the Pathogenesis of AD
To check which miRNAs are connected in AD’s pathogenesis, we used Human Disease MicroRNA Database 3.0 (HMDD v3.0)1, as a curated database which considers experiment-supported data for microRNA linkages and human disease, and we labeled them for connecting to Alzheimer’s diseases.
In silico Prediction of SNPs Occurring in miRNA Genes
The website of An-Yuan Guo’s bioinformatics Lab2 has provided numerous databases for in silico studies. The tone of most important parts of this site is miRNASNPV33, which makes it possible to check the potential effect of SNPs in miRNA maturation and function. miRNASNP includes SNPs in pre-miRNAs of human and other species, target gain and loss by SNPs in miRNA seed regions or 3′UTR of target mRNAs (Xie et al., 2020).
In silico Investigation of SNPs Occurring in miRNA Promoter Genes
In this study, all microRNA promoters which involved in Alzheimer’s disease, were extracted. Ensemble (with genome assembly GRCh38.p13)4 was used to identify the promoter areas of microRNAs. Obtained areas were checked at the UCSC5 site, and all SNPs in promoter area were retrieved from database. SNP2TFBS web-server6, 7, was performed to analyze the functional effect of SNPs in transcription factor binding (TFB) affinity patterns (Treiber et al., 2017). It is in the Human genome assembly GrCH37/hg1 from the curated JASPAR CORE 2014 vertebrate motif database through Position Weight Matrix (PWM) calculation. We used the SNPViewer tool, a web-service that employs its rsID identifier to search for SNPs to identify changes altering the transcription factor binding areas (Treiber et al., 2017).
In silico Investigation Impact of miRNAs SNPs on Their Interaction With RNA Binding Proteins and Expression of Other miRNAs
In this section, the RBP-Var database8 was employed to annotate the functional effect of SNPs on RNA binding protein affinity pattern and post-transcriptional interaction and regulation of miRNA, including its maturation, transportation from the nucleus to cytoplasm, and function. The data source for RBP-Var database was provided from starBase, CLIPdb, GEO, CISBP-RNA, RBPDB, dbSNP v142, RADAR, DARNED, TargetScan, miRanda, miRNASNP, MuTher, SCAN, seeQTL, GTEx, Harvard, and dsQTL Browser (Mao et al., 2016). All SNPs occurring in the miRNA gene (related to pri-miRNA, pre-miRNA, mature miRNA) were considered and uploaded to search box related to dbSNP. Finally, for determining and characterizing the conserved cis-motifs of RBP-RNA interaction (motif matches) in the transcriptome, RBP-Var uses all positional weight matrices of two databases, CISBP-RNA and RBPDB in AURA database. In this way, all potential k-mers are aligned with the transcriptome employing MAST in the MEME suite, a motif discovery algorithm, to present the final motif mapping with its default parameters, a match score > 0, and p-Values < 0.0001 (Mao et al., 2016).
In silico Investigation of miRNAs’ SNPs on GWAS Catalog
genome-wide association study (GWA study, or GWAS), also known as whole-genome association study (WGA study, or WGAS), is a kind of study observant genome-wide set of genetic variants in different individuals whether the variant is associated to the trait. It is a study that looks at different genetic variants throughout the genome and examines in different individuals whether the variant is related to the trait. GWAS analysis typically focuses on associations between SNPs and traits, for example, major human diseases. The GWAS catalog is a freely available database that has collected genome-wide association studies (GWAS), summarizing unorganized data from different literature sources into accessible data. It has been a joint project between NHGRI and the European Bioinformatics Institute (EBI) since 2015 (MacArthur et al., 2017). We used miRSNPV3 (see text footnote 3), the “Disease” section. In the “Disease” module, the site integrated pathological information SNPs from the NHGRI GWAS catalog. For variations in miRNAs, the database provided the minimum free energy change of the pre-miRNAs secondary.
Results
In this study, dysregulated microRNAs and their targets were collected. PubMed, Embase, ScienceDirect, Cochrane Library, and Google Scholar databases were reviewed. 38 dysregulated microRNAs and their targets were collected. Basic information for these microRNAs, including precursor ID, accession number, Genome position, host gene, mature miRNA showed in Supplementary Table 1 (It is provided in the supplementary). List of microRNAs, tissue type, their target genes, and microRNAs expression level were presented in Table 1. The miRNAs involvement in the pathogenesis of AD was tagged with ∗.
In silico Prediction and Functional Annotation of SNPs Occurring in miRNA Genes
In the next step, SNPs in miRNA genes were computationally analyzed. The miRNA SNPV3.0, the database of SNPs in miRNA was used to search SNPs of miRNAs. The server performs the prediction of miRNA target loss and gains through two target prediction tools, TargetScan, and miRmap. If one target gene of miRNA for wild type allele shows in both servers, but not in the mutant allele were considered the miRNA lost this target gene. On the contrary, if one target gene for mutant allele is shown in both servers, but not in wild type of allele, SNP-bearing mutant miRNAs achieve a target gene. The analysis of variant’s functional effect on pre-miRNA processing (for mature miRNA production) was performed through ΔG calculation which was the difference between minimal free energy (MFE), predicted by RNAfold online server, of wild type and SNP- miRNA. Moreover, we showed the exact location of SNPs and alternative alleles. The position of SNPs is indicated by Pre-miRNA, mature miRNA, or seed sequence. Results revealed several SNPs in pre-miRNA, mature miRNA, and seed site as indicated in Table 2. miR-339 and miR-34a have the majority of polymorphisms in the upstream and downstream of pre-miRNA and mature miRNAs, respectively, whereas some miRNAs have no SNPs, e.g., miR-124, and miR-125. A variant in miR-101-2 (rs138231885) has the most negative ΔG (−3.1) with a high expression rate of mature miRNA, while another SNPs (rs188892061) in miR-328 has the most ΔG (5.8) with a low expression rate of mature miRNA. The results of its investigation are given in Table 2.
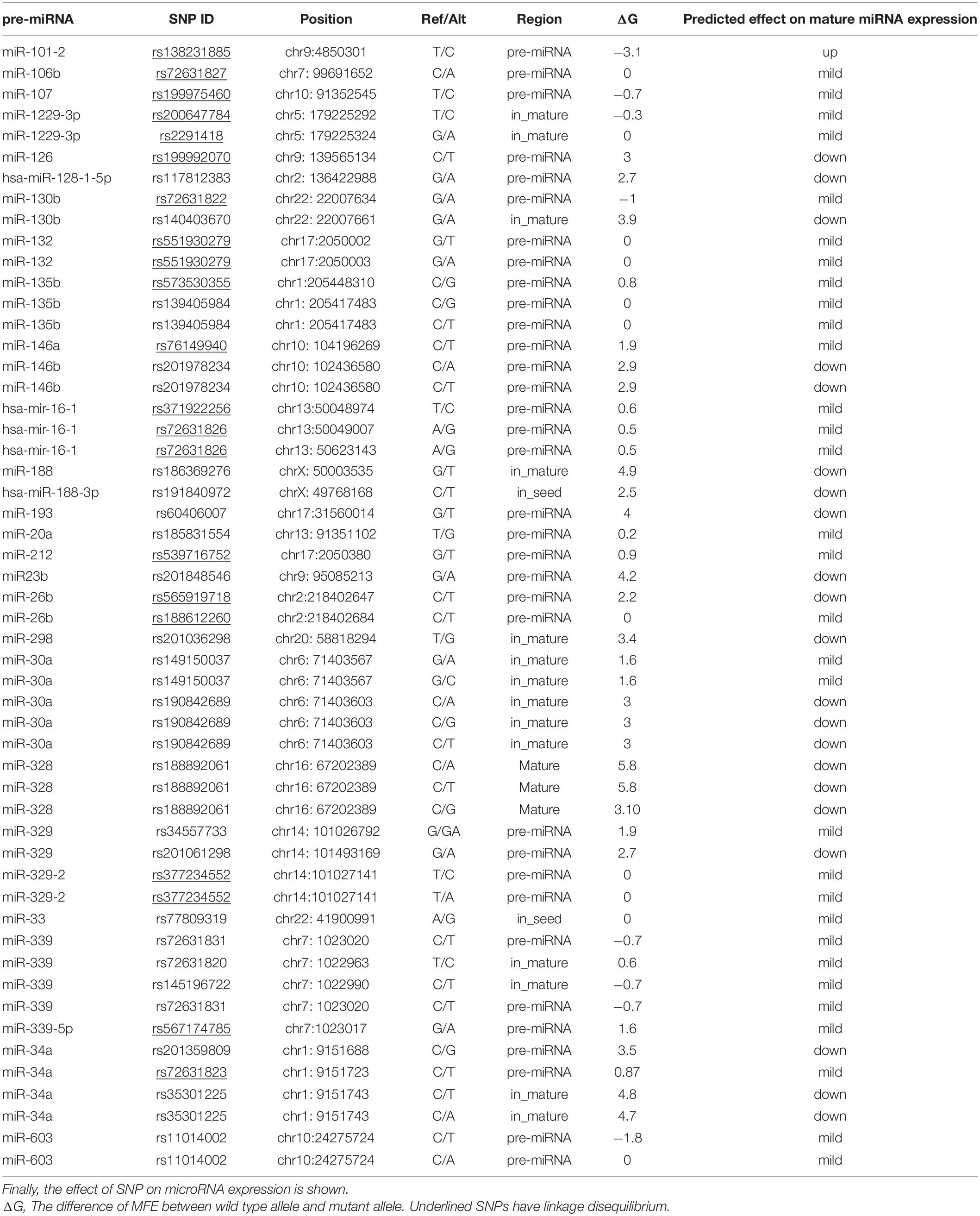
Table 2. Data collected from miRNASNPv3, it shows microRNAs SNP, frequent, its position, allele, region and enthalpy.
In silico Investigation of SNPs Occurring in miRNA Promoter Genes
SNPs’ impact was investigated in the promoter regions of miRNAs which target genes directly involved in Alzheimer’s disease. Putative TF binding sites from human genome assembly GrCH37/hg1 (for wild type allele) and 1000 Genomes project (for a mutant allele with MAF ≥ 0.001) which merged, were calculated through Position Weight Matrix (PWM) calculation (PWM score) from the curated JASPAR CORE 2014 vertebrate motif database. These SNPs affect the transcription level of miRNAs which can be increased, decreased, or neutralized. The location of SNPs, their specific numbers, and their effect are given in Table 3. As shown in Table 3, some miRNAs have several promoter regions, each of which has multiple SNPs. Nevertheless, not all of them affect expression.
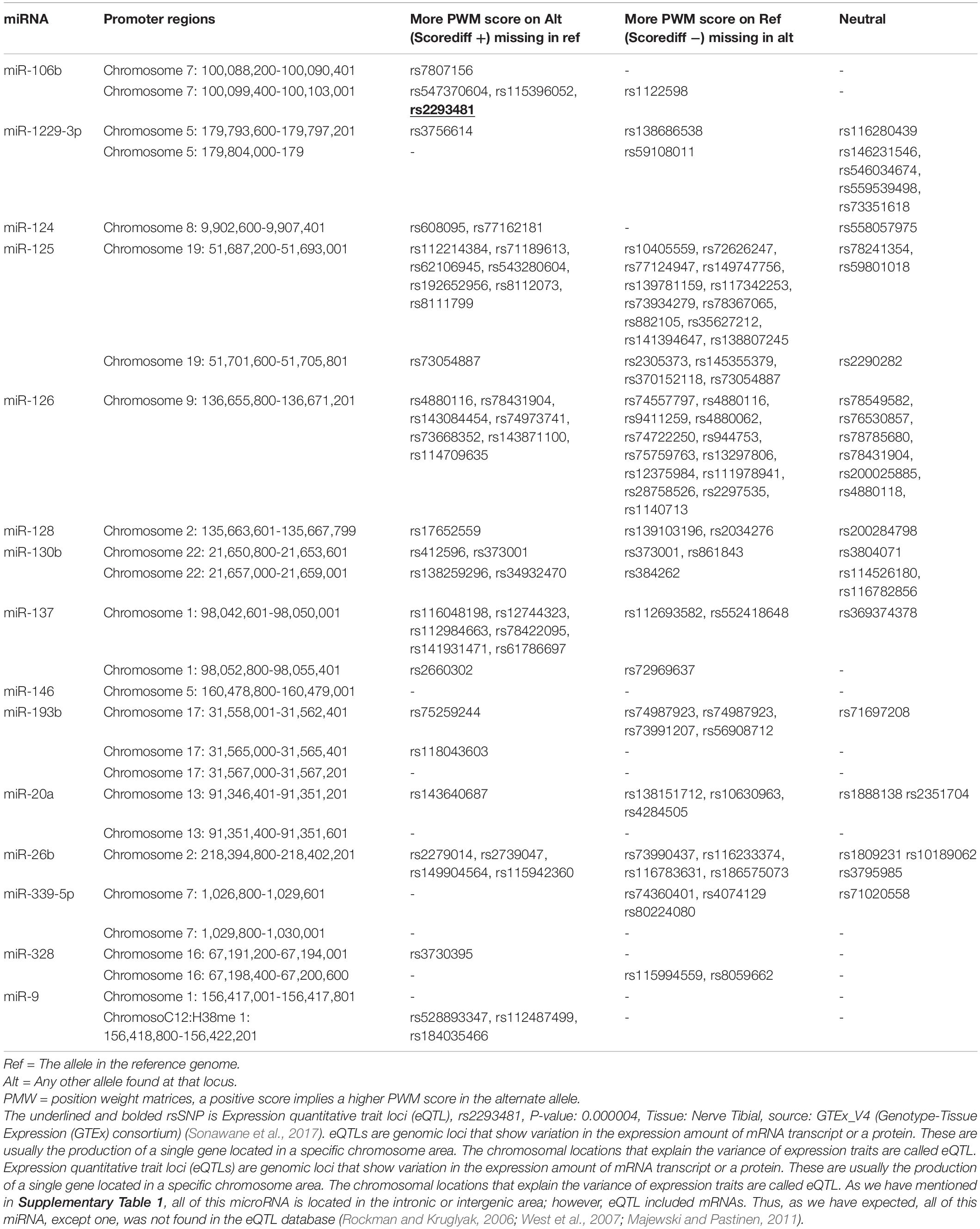
Table 3. List of SNPs are located in the promoter region and their effect on transcription factor binding performed by SNP2TFBS web-server.
Scorediff column describes the difference in PWM scores between alternating (mutant) and reference (wild type) alleles. Hence, a positive score means a larger PWM score in the alternating allele.
SNPs are only listed in the table which may affect miRNAs expression through affecting transcription factor binding sites for the transcription factor to bind. The meaning of reference genome (Ref) is a wild type allele in the table, and the alternate genome (Alt) is a mutant allele.
In silico Investigation Impact of miRNAs SNPs on Their Interaction With RNA Binding Proteins and Expression of Other miRNAs
The interplay between RNA-binding proteins (RBPs) and miRNA together is considered as critical players to regulate many cellular processes of neuronal development and function (Hafner et al., 2010). The interaction between miRNAs and RNA-binding proteins is other issue which is affected by SNPs. As Table 4 shows, the most affected RNA binding proteins are the AGO family, PTBP1, WDR33, and DGCR8. Ago family are ubiquitously expressed which bind to miRNAs or siRNAs to guide post-transcriptional gene silencing either by destabilizing the mRNA or by translation repression (Höck and Meister, 2008). PTBP has a role in pre-mRNA splicing (Zhang et al., 2015), and WDR33 acts in 3′UTR polyadenylation (Chan et al., 2014). We investigated SNPs’ effect on other cell processes such as the maturation of microRNAs and their transfer to cell. The miRNAs sequences were scanned to identify conserved motifs of RBP-RNA interaction. Motifs discovered in RBPs-RNA and promoters by MEME Suite are shown in Table 5. Other salient point is considering the effect of microRNAs’ SNPs on the expression of another microRNA derived from the studying microRNAs which the results were shown in the Supplementary Table 2. This table contains the microRNA containing the SNPs and its effect (loss or gain) on the target microRNA and its P-value. All steps are summarized in Figure 2.
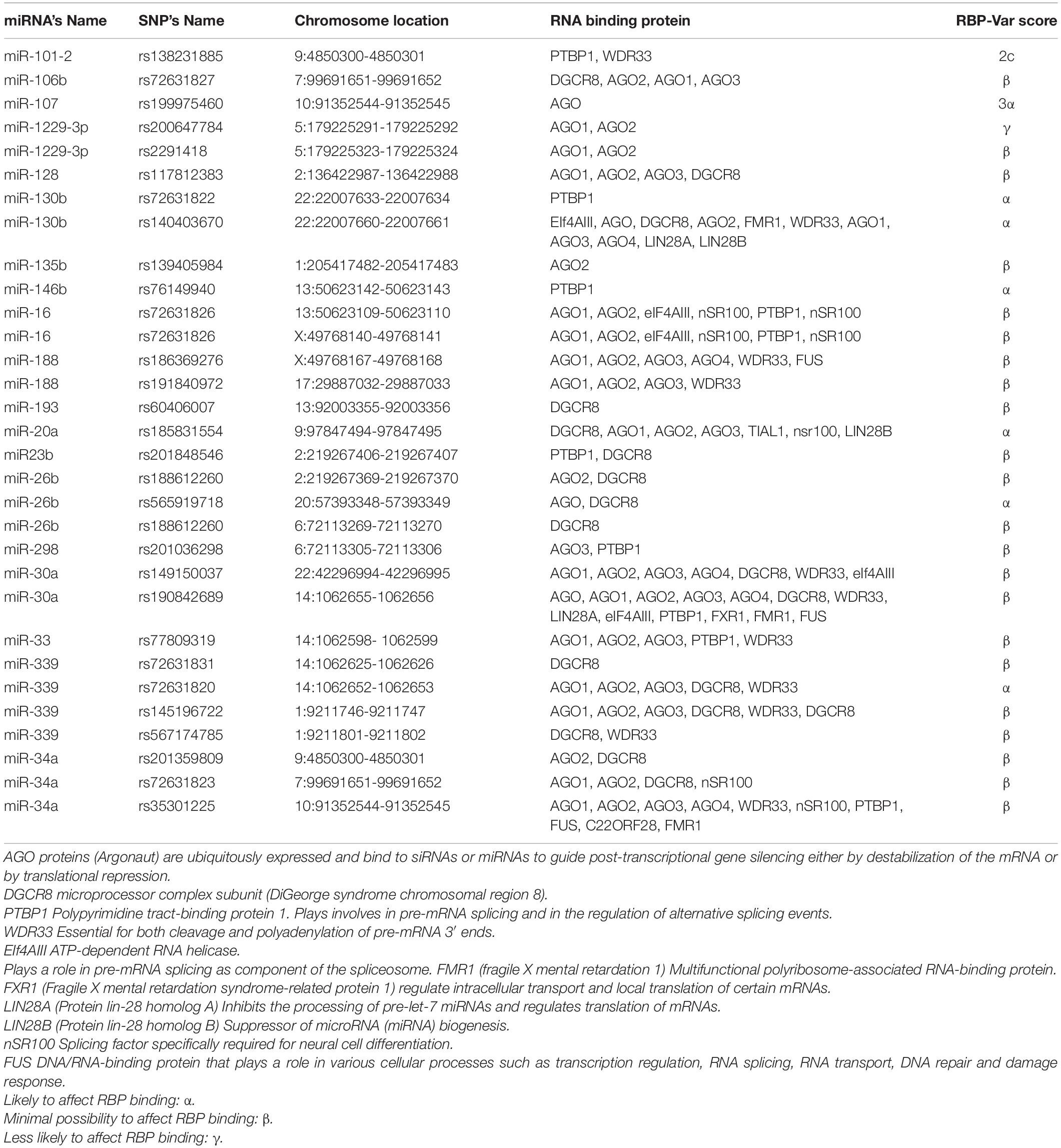
Table 4. Catalog of SNPs in miRNAs and their impact on miRNA- RNA Binding Protein interaction pattern provided by RBP-Var2 database.
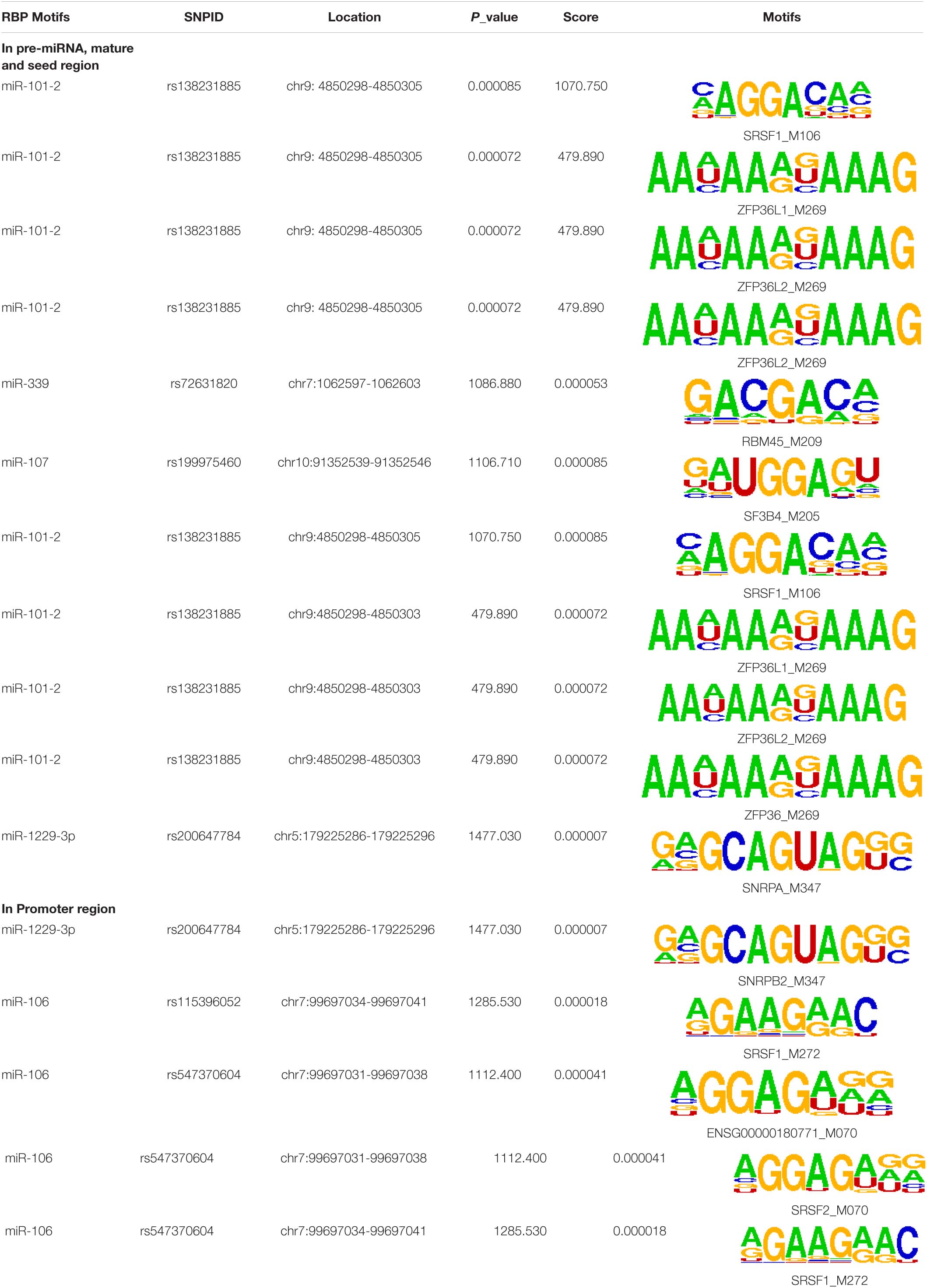
Table 5. The sequence Logos (consensus sequences) in the RNA-Binding Protein motifs of miRNA via MEME analysis by RBP-Var2.
In silico Investigation of miRNAs’ SNPs on GWAS Catalog
All microRNAs regulated in Alzheimer’s disease were located in intergenic or intronic loci, none of which were found in the GWAS database. Moreover, some new SNPs in new microRNAs have been found. Although their expression has not been measured, they include some SNPs that can affect their regulation. Table 6 demonstrates that miR-4653 has the least amount of ΔG and the most effect on miR-4653 expression. On the contrary, miR-4698 has the most ΔG and the least impact on miR-4698 expression. GWAS catalog numbers also have been mention in Table 6.
The underlined and bolded rsSNP is Expression quantitative trait loci (eQTL), rs2293481, P-value: 0.000004, Tissue: Nerve Tibial, source: GTEx_V4 (Genotype-Tissue Expression (GTEx) consortium) (Sonawane et al., 2017). eQTLs are genomic loci that show variation in the expression amount of mRNA transcript or a protein. These are usually the production of a single gene located in a specific chromosome area. The chromosomal locations that explain the variance of expression traits are called eQTL. Expression quantitative trait loci (eQTLs) are genomic loci that show variation in the expression amount of mRNA transcript or a protein. These are usually the production of a single gene located in a specific chromosome area. The chromosomal locations that explain the variance of expression traits are called eQTL. As we have mentioned in Supplementary Table 1, all of this microRNA is located in the intronic or intergenic area; however, eQTL included mRNAs. Thus, as we have expected, all of this miRNA, except one, was not found in the eQTL database (Rockman and Kruglyak, 2006; West et al., 2007; Majewski and Pastinen, 2011).
Discussion
Given the level of information and advances in the bioinformatics, computational predictions of causal factors are served as a complementary strategy to facilitate the experimental characterization of multifactorial diseases. Although up to 92% of mammalian genes could be regulated by miRNA, only a few target pairs of miRNAs have been empirically analyzed (Boissonneault et al., 2009). Several problems including complexity, expensive, and overcome technical challenges such as tissue specificity, low expression, 3′ UTR selection, and miRNA stabilization, make current techniques a challenge for the experimental validation of relationships between miRNAs and their mRNA targets (Andrés-León et al., 2017). Identifying functional SNPs in genes and analyzing their effects on phenotypes may provide an opportunity for a more in-depth understanding of the potential impact of producing such alterations. SNPs in human miRNA genes influence biogenesis, expression level, and biological function. Impaired miRNA processing may generate isomiR which can change in Drosha and/or Dicer processing sites, leading to a complete change in downstream processes including the targeted mRNA transcripts, regulatory pathway, and complex phenotypes, and diseases (Starega-Roslan et al., 2015). Researchers have designed various efficient bioinformatics tools to annotate the potential effects of SNPs. All microRNAs involved in Alzheimer’s disease and their target genes were collected. Also, we briefly introduced theoretical methods to predict these functional SNPs. The results show that miR-298, miR-328, miR-124, miR-135b miR-188-3p, mir-29c, miR-339-5p, and miR-107 target the BACE1 gene. Also, in 2009, Boissonneault et al. confirmed that dysfunctional interaction between miR-328 and BACE1 could be associated to Alzheimer’s disease.; Therefore, this gene plays a vital role in Alzheimer’s disease (Cole and Vassar, 2007; Boissonneault et al., 2009). Yan and Vassar (2014) have done a comprehensive search on BACE1 as a critical gene target for the therapy of Alzheimer’s disease. They asserted that β secretase, β-site amyloid precursor protein cleaving enzyme 1 (BACE1), launches producing toxic amyloid β (Aβ) through separating the extracellular domain of APP which plays a crucial role in Alzheimer’s disease pathogenesis (Yan and Vassar, 2014). In Alzheimer’s disease, amyloid bodies accumulate outside the neurons in some areas of brain and fibrous protein structures in the cell body of neurons, causing some changes in nerve cells’ proteome and disruption. One of the most critical proteins involved in Alzheimer’s disease is amyloid precursor protein (APP). APP protein, expressed in the nervous system cells, is involved in binding cells to each other, cell contact, and binding to the extracellular matrix and cytoskeleton. In addition, miR-101, miR-16, and miR-188 directly target APP gene (Vilardo et al., 2010; Zhang R. et al., 2014; Zhang et al., 2015). Three types of proteolytic enzymes could process APP protein, including BACE1, to form a peptide called amyloid-beta. Normally, the number of these fragments is small in the cells, and they quickly decompose; but if this balance is disturbed in the proteome of nerve cells and the amount of these components increases, spherical protein structures are formed, resulting in Alzheimer’s disease (Mullan et al., 1992; Zhang Y.W. et al., 2011; Jonsson et al., 2012). A 2019 study by Wang et al. on microRNAs involved in Alzheimer’s disease showed that the most common target was BACE1, or the direct target of BACE1, APP which underscores the importance of these genes (Mullan et al., 1992). Investigating other target genes in microRNAs has found that many of them, including the MAPK pathway, is the upstream of BACE1 and induce higher expression of BACE1 in its downstream (Figure 3; Kitagishi et al., 2014; Matsuda et al., 2018; Shal et al., 2018; Meng et al., 2020).
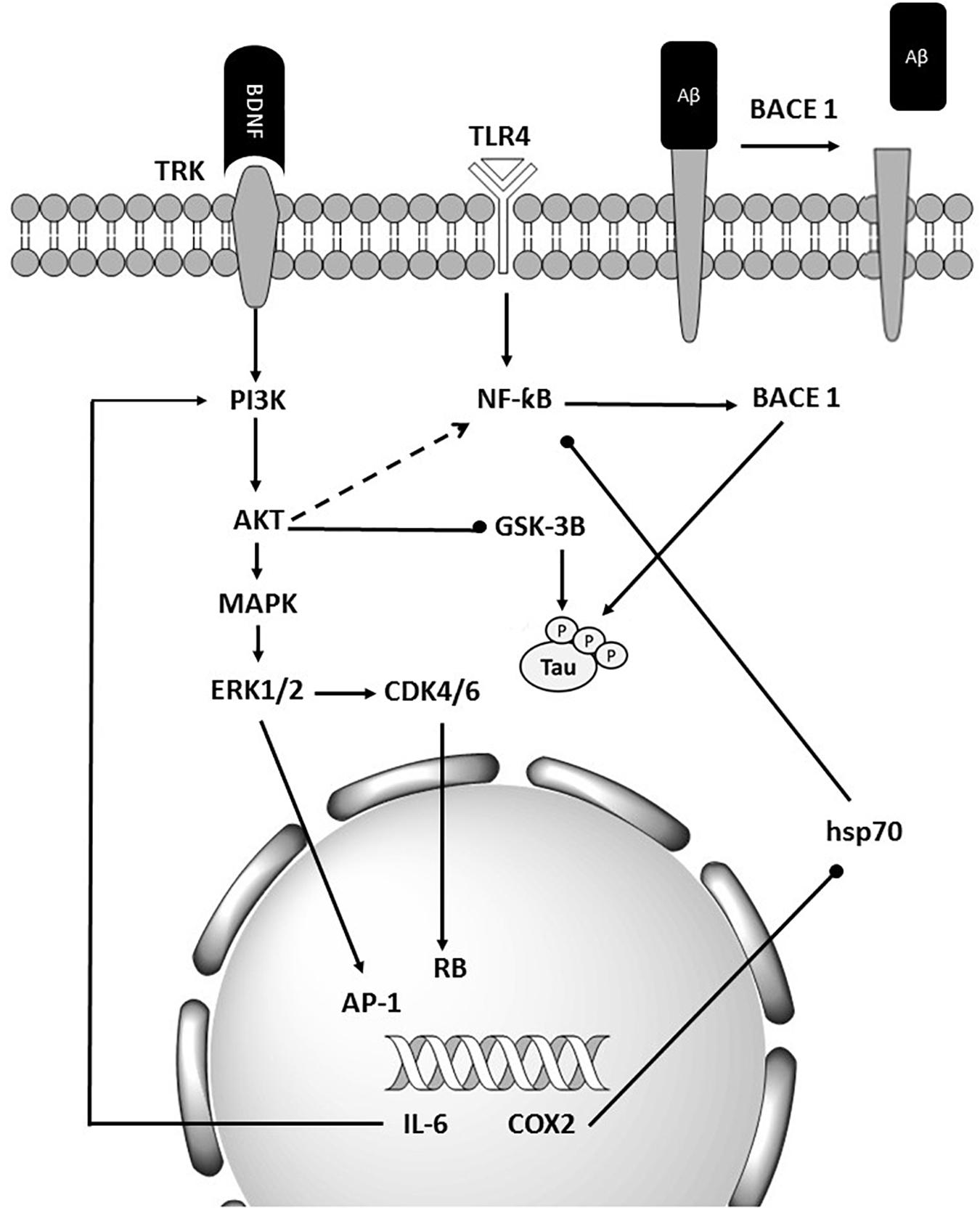
Figure 3. Schematic representation of BACE1’s importance in Alzheimer’s diseases. BACE1 is the final target of many miRNAs that are deregulated in Alzheimer’s disease. It also affected the tau and Aβ accumulation.
As the results show, the maximum number of polymorphisms was belonged to miR-339 in the upstream and downstream of mature regions in pre-miRNA and not within the seed region, while some microRNAs such as miR-124 and miR-125, there is no polymorphism in the pre-miRNA region. Imperatore et al. has declared that the level of miRNA-1229-3p which has been confirmed to regulate post-transcriptionally SORL1, is increased in the rs2291418 pre-miRNA-1229 variant. Using various biophysical techniques indicated that pre-miRNA-1229 normally forms a G-quadruplex structure in equilibrium with hairpin structure. The presence of this polymorphism, G/A, in pre-miRNA-1229 disturbs this balance (Imperatore et al., 2020).
Since interplay between miRNA and target mRNA is necessary for miRNA function, SNPs present on target binding sites of miRNAs should be evaluated before studies, especially gene expression.
Comparing ΔG (The difference of MFE between wild type allele and mutant allele) was shown in Table 2. According to the results, the highest ΔG related to miR-101 indicates the effect of T/C substitution which can increase the processing probability of pri-miRNA 101; thus, increase the production of its mature form.
According to the results of the highest ΔG in the miR-101, indicating the effect of T/C replacement can increase the processing; thus, it increases producing its mature form. According to the evidence, COX2, an inductive enzyme which catalyzes the conversion of arachidonic acid to prostanoids, plays a vital role in the plasticity of neurons and memory acquisition It seems that variant rs138231885, which is predicted to increase the expression of the mature form of miR-101-2 (performing biological function), is likely to be associated to disease risk.
The lowest number occurs in miR-328, miR-188, and miR-34. On the one hand, comparing Tables 1, 2 is shown that level expression of few microRNAs is different due to their mutations effect which could occur in them; for example, miR-101, miR-126, miR-128, miR-34a, miR-193, and miR-26; On the other hand, there are microRNAs in which effect mutations are in the same direction as their expression in Alzheimer’s disease. The miR-146a, miR-298, miR-30a, and miR-34a are from this category. Hu et al. suggested two common polymorphisms in pre-miR-125a may contribute to a genetic disorder called RPL with a disturbance in the miR-125a’s expression (Hu et al., 2011). Inoue et al. (2014) has found that miR-125 and its SNPs (rs12976445) have a negative relationship with Graves’ disease (GD) and Hashimoto’s disease (HD); moreover, not only the expression of miRNA-125 but also its efficacy has been reduced. Moreover, Landi et al. (2008) have investigated polymorphisms which have affected micro-RNA-binding sites and their attachment to targets.
The results of Table 3 provide the list of regulatory SNPs which significantly affect transcription factor binding sites for the transcription factor affinity. According to the evidence, variants placed in non-coding regions which may affect gene expression by changing the transcription factors’ binding affinity to their specific corresponding regulatory motifs may significantly be correlated to human traits and diseases.
The SNPs which affect transcription factor binding affinity could influence the microRNA expression in several states including no effect (No change occurred in the TFBS for the original TFs) (neutral), gaining function (novel transcription factor attached to modified TFBS), and loss of function (original TFs cannot bind to its specific location). Part of a regulatory region to which no TF has previously been connected may connect some TFs; hence, novel TFBSs are successfully announced. Oliveira and et al. have shown that polymorphic C allele of IL-8-845 in promoter region can influence mRNA expression levels and disease risk (de Oliveira et al., 2015).
Sun et al. have announced that the changes in miRNA-binding sequencing sites have resulted in the loss of miRNA function (Sun et al., 2009). Therefore, SNPs in miRNAs can affect the function of RNA binding proteins. The interaction between RBP and miRNA plays a vital role in regulating the gene expression and impaired mRNA processing and expression, significantly linked to neurological disease. The miRNA polymorphism effect on altering its interaction with RBP in the pathogenesis of neurological diseases is still largely unknown. Thus, more in-depth studies may be needed to evaluate altered miRNA potential: RBP interaction as a diagnostic factor to predict disease progression. The list of SNPs occurring in miRNA gene promoters and RBP binding sites are presented in Table 4 and Supplementary Table 2.
The list of SNPs occurring in miRNA gene promoters and RBP binding sites are presented in Table 4 and Supplementary Table 2.
As a result, shows and we have expected, none of the SNPs were found in the GWAS catalog. Because GWAS is a whole genome sequencing technique and it determines SNPs in complementary DNA (cDNA), not in the non-coding areas, for example, intergenic and intronic loci. Ghanbari et al. have done the only GWAS study on microRNAs and AD. They indicated that miR-1229, by targeting SORL1, which are both expressed in the human brain, can cause Alzheimer’s disease (Table 1). They also found rs2291418 in the miR-1229 precursor to being significantly associated with Alzheimer’s disease, consistent with our data (Tables 2, 4; Ghanbari et al., 2016). rs2293481 in miR-106b is expression quantitative trait loci (eQTL) with P-value: 0.000004, Tissue Nerve Tibial, source: GTEx_V4 [Genotype-Tissue Expression (GTEx) consortium] (Table 3). It is revealed that tissue specificity is driven by context-dependent regulatory pathways, providing transcriptional regulation of tissue-specific processes (Sonawane et al., 2017).
Our study presents useful information on the possible impact of SNPs and different regulatory patterns on miRNA expression and function and provides valuable insights into the pathogenesis and development of AD. Finally, it seems that genetic variants could be the proper criteria for early detection of Alzheimer’s in the future.
Conclusion
Briefly, following a deep screening of miRNAs that play a determining role in Alzheimer’s disease, several resources were implemented to annotate SNP’s functional effect in the miRNA gene. For a comprehensive study, we investigated various aspects of the mined SNPs effect on biogenesis and miRNA function, including pre-miRNA processing level, miRNA-target interaction, transcript level, and miRNA-RBPs interaction. This study theoretically provided a collection of candidate causal SNPs in different parts of the miRNA gene that could be considered for future practical study in Alzheimer’s disease management.
Data Availability Statement
The original contributions presented in the study are included in the Supplementary Material, further inquiries can be directed to the corresponding author/s.
Ethics Statement
This research was approved by ethics committee of the Hormozgan University of Medical Science (ethical cod: IR/HUMS.REC.270).
Author Contributions
MM wrote the manuscript. MM, RM, HA, AN, and PM collected the data. PM revised the literature and contributed to the conception and design of the study. All authors contributed to the critical revision, edition, and final approval of the manuscript.
Funding
This research was partially supported by the Hormozgan University of Medical Science (Grant Code: 990278).
Conflict of Interest
The authors declare that the research was conducted in the absence of any commercial or financial relationships that could be construed as a potential conflict of interest.
Acknowledgments
We thank our colleagues from the Student Research Committee for providing insight and expertise that greatly assisted the research although they may not agree with all the conclusions of this manuscript.
Supplementary Material
The Supplementary Material for this article can be found online at: https://www.frontiersin.org/articles/10.3389/fnins.2021.631852/full#supplementary-material
Footnotes
- ^ http://www.cuilab.cn/hmdd
- ^ http://bioinfo.life.hust.edu.cn/guo_lab#!/
- ^ http://bioinfo.life.hust.edu.cn/miRNASNP#!/
- ^ http://asia.ensembl.org/index.html
- ^ ftp://ccg.vital-it.ch/snp2tfbs
- ^ https://genome.ucsc.edu/
- ^ http://ccg.vital-it.ch/snp2tfbs/
- ^ http://www.rbp-var.biols.ac.cn/
References
Absalon, S., Kochanek, D. M., Raghavan, V., and Krichevsky, A. M. (2013). MiR-26b, upregulated in Alzheimer’s disease, activates cell cycle entry, tau-phosphorylation, and apoptosis in postmitotic neurons. J. Neurosci. 33, 14645–14659. doi: 10.1523/jneurosci.1327-13.2013
Andrés-León, E., Cases, I., Alonso, S., and Rojas, A. M. (2017). Novel miRNA-mRNA interactions conserved in essential cancer pathways. Sci. Rep. 7, 1–13. doi: 10.1080/07391102.2020.1800511
Banzhaf-Strathmann, J., Benito, E., May, S., Arzberger, T., Tahirovic, S., Kretzschmar, H., et al. (2014). MicroRNA-125b induces tau hyperphosphorylation and cognitive deficits in Alzheimer’s disease. EMBO J. 33, 1667–1680. doi: 10.15252/embj.201387576
Boissonneault, V., Plante, I., Rivest, S., and Provost, P. (2009). MicroRNA-298 and microRNA-328 regulate expression of mouse beta-amyloid precursor protein-converting enzyme 1. J. Biol. Chem. 284, 1971–1981. doi: 10.1074/jbc.m807530200
Boutz, P. L., Chawla, G., Stoilov, P., and Black, D. L. (2007). MicroRNAs regulate the expression of the alternative splicing factor nPTB during muscle development. Genes Dev. 21, 71–84. doi: 10.1101/gad.1500707
Buroker, N., Ning, X., Li, K., Zhou, Z., and Cen, W. (2015). SNPs, Linkage Disequilibrium and Transcriptional Factor Binding Sites Associated with Acute Mountain Sickness among Han Chinese at the Qinghai-Tibetan Plateau. Int. J. Genomic Med. 3:120.
Chan, S. L., Huppertz, I., Yao, C., Weng, L., Moresco, J. J., Yates, J. R., et al. (2014). CPSF30 and Wdr33 directly bind to AAUAAA in mammalian mRNA 3′ processing. Genes Dev. 28, 2370–2380. doi: 10.1101/gad.250993.114
Chen, W., Wu, L., Hu, Y., Jiang, L., Liang, N., Chen, J., et al. (2020). MicroRNA-107 Ameliorates Damage in a Cell Model of Alzheimer’s Disease by Mediating the FGF7/FGFR2/PI3K/Akt Pathway. J. Mol. Neurosci. 70, 1589–1597.
Cole, S. L., and Vassar, R. (2007). The Alzheimer’s disease β-secretase enzyme, BACE1. Mol. Neurodegenerat. 2:22. doi: 10.1186/1750-1326-2-22
Croce, N., Gelfo, F., Ciotti, M. T., Federici, G., Caltagirone, C., Bernardini, S., et al. (2013). NPY modulates miR-30a-5p and BDNF in opposite direction in an in vitro model of Alzheimer disease: a possible role in neuroprotection? Mol. Cell Biochem. 376, 189–195. doi: 10.1007/s11010-013-1567-0
de Oliveira, J. G., Rossi, A. F. T., Nizato, D. M., Cadamuro, A. C. T., Jorge, Y. C., Valsechi, M. C., et al. (2015). Influence of functional polymorphisms in TNF-α, IL-8, and IL-10 cytokine genes on mRNA expression levels and risk of gastric cancer. Tumor Biol. 36, 9159–9170. doi: 10.1007/s13277-015-3593-x
Delay, C., Calon, F., Mathews, P., and Hébert, S. S. (2011). Alzheimer-specific variants in the 3′UTR of Amyloid precursor protein affect microRNA function. Mol. Neurodegenerat. 6:70. doi: 10.1186/1750-1326-6-70
Dickson, J. R., Kruse, C., Montagna, D. R., Finsen, B., and Wolfe, M. S. (2013). Alternative polyadenylation and miR-34 family members regulate tau expression. J. Neurochem. 127, 739–749.
Dong, H., Lei, J., Ding, L., Wen, Y., Ju, H., and Zhang, X. (2013). MicroRNA: function, detection, and bioanalysis. Chem. Rev. 113, 6207–6233. doi: 10.1021/cr300362f
Fang, M., Wang, J., Zhang, X., Geng, Y., Hu, Z., Rudd, J. A., et al. (2012). The miR-124 regulates the expression of BACE1/beta-secretase correlated with cell death in Alzheimer’s disease. Toxicol. Lett. 209, 94–105. doi: 10.1016/j.toxlet.2011.11.032
Femminella, G. D., Ferrara, N., and Rengo, G. (2015). The emerging role of microRNAs in Alzheimer’s disease. Front. Physiol. 6:40. doi: 10.3389/fphys.2015.00040
Geekiyanage, H., and Chan, C. (2011). MicroRNA-137/181c regulates serine palmitoyltransferase and in turn amyloid beta, novel targets in sporadic Alzheimer’s disease. J. Neurosci. 31, 14820–14830. doi: 10.1523/jneurosci.3883-11.2011
Georges, M., Coppieters, W., and Charlier, C. (2007). Polymorphic miRNA-mediated gene regulation: contribution to phenotypic variation and disease. Curr. Opin. Genet. Dev. 17, 166–176. doi: 10.1016/j.gde.2007.04.005
Ghanbari, M., Ikram, M. A., de Looper, H. W. J., Hofman, A., Erkeland, S. J., Franco, O. H., et al. (2016). Genome-wide identification of microRNA-related variants associated with risk of Alzheimer’s disease. Sci. Rep. 6:28387.
Guo, T., Feng, Y., Liu, Q., Yang, X., Jiang, T., Chen, Y., et al. (2014). MicroRNA-320a suppresses in GBM patients and modulates glioma cell functions by targeting IGF-1R. Tumor Biol. 35, 11269–11275. doi: 10.1007/s13277-014-2283-4
Hafner, M., Landthaler, M., Burger, L., Khorshid, M., Hausser, J., Berninger, P., et al. (2010). Transcriptome-wide identification of RNA-binding protein and microRNA target sites by PAR-CLIP. Cell 141, 129–141. doi: 10.1016/j.cell.2010.03.009
Höck, J., and Meister, G. (2008). The Argonaute protein family. Genome Biol. 9:210. doi: 10.1186/gb-2008-9-2-210
Hu, Y., Liu, C.-M., Qi, L., He, T.-Z., Shi-Guo, L., Hao, C.-J., et al. (2011). Two common SNPs in pri-miR-125a alter the mature miRNA expression and associate with recurrent pregnancy loss in a Han-Chinese population. RNA Biol. 8, 861–872. doi: 10.4161/rna.8.5.16034
Imperatore, J. A., Then, M. L., McDougal, K. B., and Mihailescu, M. R. (2020). Characterization of a G-quadruplex structure in pre-miRNA-1229 and in its Alzheimer’s disease-associated variant rs2291418: implications for miRNA-1229 maturation. Int. J. Mol. Sci. 21:767. doi: 10.3390/ijms21030767
Inoue, Y., Watanabe, M., Inoue, N., Kagawa, T., Shibutani, S., Otsu, H., et al. (2014). Associations of single nucleotide polymorphisms in precursor-microRNA (miR)-125a and the expression of mature mi R-125a with the development and prognosis of autoimmune thyroid diseases. Clin. Exp. Immunol. 178, 229–235. doi: 10.1111/cei.12410
John, B., Enright, A. J., Aravin, A., Tuschl, T., Sander, C., and Marks, D. S. (2004). Human microRNA targets. PLoS Biol 2:e363. doi: 10.1371/journal.pbio.0020363
Jonsson, T., Atwal, J. K., Steinberg, S., Snaedal, J., Jonsson, P. V., Bjornsson, S., et al. (2012). A mutation in APP protects against Alzheimer’s disease and age-related cognitive decline. Nature 488, 96–99.
Kim, J., Yoon, H., Horie, T., Burchett, J. M., Restivo, J. L., Rotllan, N., et al. (2015). microRNA-33 Regulates ApoE Lipidation and Amyloid-beta Metabolism in the Brain. J. Neurosci. 35, 14717–14726. doi: 10.1523/jneurosci.2053-15.2015
Kim, W., Noh, H., Lee, Y., Jeon, J., Shanmugavadivu, A., McPhie, D. L., et al. (2016). MiR-126 Regulates Growth Factor Activities and Vulnerability to Toxic Insult in Neurons. Mol. Neurobiol. 53, 95–108. doi: 10.1007/s12035-014-8989-x
Kitagishi, Y., Nakanishi, A., Ogura, Y., and Matsuda, S. (2014). Dietary regulation of PI3K/AKT/GSK-3β pathway in Alzheimer’s disease. Alzheimer’s Res. Ther. 6, 1–7.
Lambert, S. A., Jolma, A., Campitelli, L. F., Das, P. K., Yin, Y., Albu, M., et al. (2018). The human transcription factors. Cell 172, 650–665.
Landi, D., Gemignani, F., Naccarati, A., Pardini, B., Vodicka, P., Vodickova, L., et al. (2008). Polymorphisms within micro-RNA-binding sites and risk of sporadic colorectal cancer. Carcinogenesis 29, 579–584. doi: 10.1093/carcin/bgm304
Li, L., Xu, Y., Zhao, M., and Gao, Z. (2020). Neuro-protective roles of long non-coding RNA MALAT1 in Alzheimer’s disease with the involvement of the microRNA-30b/CNR1 network and the following PI3K/AKT activation. Exp. Mol. Pathol. 117:104545. doi: 10.1016/j.yexmp.2020.104545
Li, X., Wang, S.-W., Xi-Ling, L., Yu, F.-Y., and Cong, H.-M. (2020). Knockdown of long non-coding RNA TUG1 depresses apoptosis of hippocampal neurons in Alzheimer’s disease by elevating microRNA-15a and repressing ROCK1 expression. Inflamm. Res. 69, 897–910. doi: 10.1007/s00011-020-01364-8
Long, J. M., Ray, B., and Lahiri, D. K. (2014). MicroRNA-339-5p down-regulates protein expression of beta-site amyloid precursor protein-cleaving enzyme 1 (BACE1) in human primary brain cultures and is reduced in brain tissue specimens of Alzheimer disease subjects. J. Biol. Chem. 289, 5184–5198. doi: 10.1074/jbc.m113.518241
Lukiw, W. J. (2020). microRNA-146a Signaling in Alzheimer’s disease (AD) and prion disease (PrD). Front. Neurol. 11:462. doi: 10.3389/fneur.2020.00462
MacArthur, J., Bowler, E., Cerezo, M., Gil, L., Hall, P., Hastings, E., et al. (2017). The new NHGRI-EBI Catalog of published genome-wide association studies (GWAS Catalog). Nucleic Acids Res. 45, D896–D901.
Majewski, J., and Pastinen, T. (2011). The study of eQTL variations by RNA-seq: from SNPs to phenotypes. Trends Genet. 27, 72–79. doi: 10.1016/j.tig.2010.10.006
Mao, F., Xiao, L., Li, X., Liang, J., Teng, H., Cai, W., et al. (2016). RBP-Var: a database of functional variants involved in regulation mediated by RNA-binding proteins. Nucleic Acids Res. 44, D154–D163.
Matsuda, S., Nakagawa, Y., Tsuji, A., Kitagishi, Y., Nakanishi, A., and Murai, T. (2018). Implications of PI3K/AKT/PTEN signaling on superoxide dismutases expression and in the pathogenesis of Alzheimer’s disease. Diseases 6:28. doi: 10.3390/diseases6020028
Meng, L., Li, X.-Y., Shen, L., and Ji, H.-F. (2020). Type 2 diabetes mellitus drugs for Alzheimer’s disease: current evidence and therapeutic opportunities. Trends Mol. Med. 26, 597–614. doi: 10.1016/j.molmed.2020.02.002
Mirzaii-Fini, F., Dowlati, M. A., Dehghani Ashkezari, M., and Kouchaki, E. (2018). Investigating the association of Val/Met polymorphism of the BDNF gene with the incidence of disease in patients with Alzheimer and comparison with healthy elderly people in Iran. FEYZ J. Kashan Univ. Med. Sci. 22, 617–623.
Moncini, S., Lunghi, M., Valmadre, A., Grasso, M., Del Vescovo, V., Riva, P., et al. (2017). The miR-15/107 Family of microRNA Genes Regulates CDK5R1/p35 with Implications for Alzheimer’s Disease Pathogenesis. Mol. Neurobiol. 54, 4329–4342. doi: 10.1007/s12035-016-0002-4
Mullan, M., Crawford, F., Axelman, K., Houlden, H., Lilius, L., Winblad, B., et al. (1992). A pathogenic mutation for probable Alzheimer’s disease in the APP gene at the N–terminus of β–amyloid. Nature Genet. 1, 345–347. doi: 10.1038/ng0892-345
Muller, M., Kuiperij, H. B., Claassen, J. A., Kusters, B., and Verbeek, M. M. (2014). MicroRNAs in Alzheimer’s disease: differential expression in hippocampus and cell-free cerebrospinal fluid. Neurobiol. Aging 35, 152–158. doi: 10.1016/j.neurobiolaging.2013.07.005
Pereira, P. A., Tomas, J. F., Queiroz, J. A., Figueiras, A. R., and Sousa, F. (2016). Recombinant pre-miR-29b for Alzheimer s disease therapeutics. Sci. Rep. 6:19946.
Podhorna, J., Winter, N., Zoebelein, H., Perkins, T., and Walda, S. (2020). Alzheimer’s diagnosis: real-world physician behavior across countries. Adv. Ther. 37, 883–893. doi: 10.1007/s12325-019-01212-0
Reddy, P. H., Tonk, S., Kumar, S., Vijayan, M., Kandimalla, R., Kuruva, C. S., et al. (2017). A critical evaluation of neuroprotective and neurodegenerative MicroRNAs in Alzheimer’s disease. Biochem. Biophys. Res. Commun. 483, 1156–1165. doi: 10.1016/j.bbrc.2016.08.067
Rockman, M. V., and Kruglyak, L. (2006). Genetics of global gene expression. Nat. Rev. Genet. 7, 862–872. doi: 10.1038/nrg1964
Roy, J., and Mallick, B. (2017). Altered gene expression in late-onset Alzheimer’s disease due to SNPs within 3′ UTR microRNA response elements. Genomics 109, 177–185. doi: 10.1016/j.ygeno.2017.02.006
Santa-Maria, I., Alaniz, M. E., Renwick, N., Cela, C., Fulga, T. A., Van Vactor, D., et al. (2015). Dysregulation of microRNA-219 promotes neurodegeneration through post-transcriptional regulation of tau. J. Clin. Invest. 125, 681–686. doi: 10.1172/jci78421
Sarkar, S., Jun, S., Rellick, S., Quintana, D. D., Cavendish, J. Z., and Simpkins, J. W. (2016). Expression of microRNA-34a in Alzheimer’s disease brain targets genes linked to synaptic plasticity, energy metabolism, and resting state network activity. Brain Res. 1646, 139–151. doi: 10.1016/j.brainres.2016.05.026
Sethi, P., and Lukiw, W. J. (2009). Micro-RNA abundance and stability in human brain: specific alterations in Alzheimer’s disease temporal lobe neocortex. Neurosci. Lett. 459, 100–104. doi: 10.1016/j.neulet.2009.04.052
Shal, B., Ding, W., Ali, H., Kim, Y. S., and Khan, S. (2018). Anti-neuroinflammatory potential of natural products in attenuation of Alzheimer’s disease. Front. Pharmacol. 9:548. doi: 10.3389/fphar.2018.00548
Sonawane, A. R., Platig, J., Fagny, M., Chen, C.-Y., Paulson, J. N., Lopes-Ramos, C. M., et al. (2017). Understanding tissue-specific gene regulation. Cell Rep. 21, 1077–1088.
Souza, V. C., Morais, G. S. Jr., Henriques, A. D., Machado-Silva, W., Perez, D. I. V., Brito, C. J., et al. (2020). Whole-blood levels of MicroRNA-9 are decreased in patients with late-onset Alzheimer disease. Am. J. Alzheimer’s Dis. Dementias 35:1533317520911573.
Starega-Roslan, J., Witkos, T. M., Galka-Marciniak, P., and Krzyzosiak, W. J. (2015). Sequence features of Drosha and Dicer cleavage sites affect the complexity of isomiRs. Int. J. Mol. Sci. 16, 8110–8127. doi: 10.3390/ijms16048110
Sun, G., Yan, J., Noltner, K., Feng, J., Li, H., Sarkis, D. A., et al. (2009). SNPs in human miRNA genes affect biogenesis and function. RNA 15, 1640–1651. doi: 10.1261/rna.1560209
Tian, N., Cao, Z., and Zhang, Y. (2014). MiR-206 decreases brain-derived neurotrophic factor levels in a transgenic mouse model of Alzheimer’s disease. Neurosci. Bull. 30, 191–197. doi: 10.1007/s12264-013-1419-7
Tiribuzi, R., Crispoltoni, L., Porcellati, S., Di Lullo, M., Florenzano, F., Pirro, M., et al. (2014). miR128 up-regulation correlates with impaired amyloid β (1-42) degradation in monocytes from patients with sporadic Alzheimer’s disease. Neurobiol. Aging 35, 345–356. doi: 10.1016/j.neurobiolaging.2013.08.003
Treiber, T., Treiber, N., Plessmann, U., Harlander, S., Daiß, J.-L., Eichner, N., et al. (2017). A compendium of RNA-binding proteins that regulate microRNA biogenesis. Mol. Cell 66:270.–284.
Van Kouwenhove, M., Kedde, M., and Agami, R. (2011). MicroRNA regulation by RNA-binding proteins and its implications for cancer. Nat. Rev. Cancer 11, 644–656. doi: 10.1038/nrc3107
Vilardo, E., Barbato, C., Ciotti, M., Cogoni, C., and Ruberti, F. (2010). MicroRNA-101 regulates amyloid precursor protein expression in hippocampal neurons. J. Biol. Chem. 285, 18344–18351. doi: 10.1074/jbc.m110.112664
Wahid, F., Shehzad, A., Khan, T., and Kim, Y. Y. (2010). MicroRNAs: synthesis, mechanism, function, and recent clinical trials. Biochim. Biophys. Acta 1803, 1231–1243. doi: 10.1016/j.bbamcr.2010.06.013
Wang, J., Chen, C., and Zhang, Y. (2020). An investigation of microRNA-103 and microRNA-107 as potential blood-based biomarkers for disease risk and progression of Alzheimer’s disease. J. Clin. Lab. Anal. 34:e23006.
Wang, L., Liu, J., Wang, Q., Jiang, H., Li, Z., and Liu, R. (2019). MicroRNA-200a-3p mediates neuroprotection in Alzheimer-related deficits and attenuates amyloid-beta overproduction and tau hyperphosphorylation via co-regulating BACE1 and PRKACB. Front. Pharmacol. 10:806. doi: 10.3389/fphar.2019.00806
Weinberg, R. B., Mufson, E. J., and Counts, S. E. (2015). Evidence for a neuroprotective microRNA pathway in amnestic mild cognitive impairment. Front. Neurosci. 9:430. doi: 10.3389/fnins.2015.00430
West, M. A., Kim, K., Kliebenstein, D. J., Van Leeuwen, H., Michelmore, R. W., Doerge, R., et al. (2007). Global eQTL mapping reveals the complex genetic architecture of transcript-level variation in Arabidopsis. Genetics 175, 1441–1450. doi: 10.1534/genetics.106.064972
Witkos, T. M., Koscianska, E., and Krzyzosiak, W. J. (2011). Practical aspects of microRNA target prediction. Curr. Mol. Med. 11, 93–109. doi: 10.2174/156652411794859250
Wong, H. K., Veremeyko, T., Patel, N., Lemere, C. A., Walsh, D. M., Esau, C., et al. (2013). De-repression of FOXO3a death axis by microRNA-132 and -212 causes neuronal apoptosis in Alzheimer’s disease. Hum. Mol. Genet. 22, 3077–3092. doi: 10.1093/hmg/ddt164
Xie, G.-Y., Xia, M., Miao, Y.-R., Luo, M., Zhang, Q., and Guo, A.-Y. (2020). FFLtool: a web server for transcription factor and miRNA feed forward loop analysis in human. Bioinformatics 36, 2605–2607. doi: 10.1093/bioinformatics/btz929
Xu, Y., Li, L., Xiang, X., Wang, H., Cai, W., Xie, J., et al. (2013). Three common functional polymorphisms in microRNA encoding genes in the susceptibility to hepatocellular carcinoma: a systematic review and meta-analysis. Gene 527, 584–593. doi: 10.1016/j.gene.2013.05.085
Yan, R., and Vassar, R. (2014). Targeting the β secretase BACE1 for Alzheimer’s disease therapy. Lancet Neurol. 13, 319–329. doi: 10.1016/s1474-4422(13)70276-x
Yang, G., Song, Y., Zhou, X., Deng, Y., Liu, T., Weng, G., et al. (2015). MicroRNA-29c targets beta-site amyloid precursor protein-cleaving enzyme 1 and has a neuroprotective role in vitro and in vivo. Mol. Med. Rep. 12, 3081–3088. doi: 10.3892/mmr.2015.3728
Yang, T. T., Liu, C. G., Gao, S. C., Zhang, Y., and Wang, P. C. (2018). The Serum Exosome Derived MicroRNA− 135a,− 193b, and− 384 Were Potential Alzheimer’s Disease Biomarkers. Biomed. Environ. Sci. 31, 87–96.
Zhang, B., Chen, C. F., Wang, A. H., and Lin, Q. F. (2015). MiR-16 regulates cell death in Alzheimer’s disease by targeting amyloid precursor protein. Eur. Rev. Med. Pharmacol. Sci. 19, 4020–4027.
Zhang, C., Lu, J., Liu, B., Cui, Q., and Wang, Y. (2016). Primate-specific miR-603 is implicated in the risk and pathogenesis of Alzheimer’s disease. Aging 8, 272–290. doi: 10.18632/aging.100887
Zhang, R., Zhang, Q., Niu, J., Lu, K., Xie, B., Cui, D., et al. (2014). Screening of microRNAs associated with Alzheimer’s disease using oxidative stress cell model and different strains of senescence accelerated mice. J. Neurol. Sci. 338, 57–64. doi: 10.1016/j.jns.2013.12.017
Zhang, Y., Bai, R., Liu, C., Ma, C., Chen, X., Yang, J., et al. (2019). MicroRNA single-nucleotide polymorphisms and diabetes mellitus: A comprehensive review. Clin. Genet. 95, 451–461.
Zhang, Y., Xing, H., Guo, S., Zheng, Z., Wang, H., and Xu, D. (2016). MicroRNA-135b has a neuroprotective role via targeting of beta-site APP-cleaving enzyme 1. Exp. Ther. Med. 12, 809–814. doi: 10.3892/etm.2016.3366
Zhang, Y.-W., Thompson, R., Zhang, H., and Xu, H. (2011). APP processing in Alzheimer’s disease. Mol. Brain 4, 1–13.
Zhou, B., Li, L., Qiu, X., Wu, J., Xu, L., and Shao, W. (2020). Long non-coding RNA ANRIL knockdown suppresses apoptosis and pro-inflammatory cytokines while enhancing neurite outgrowth via binding microRNA-125a in a cellular model of Alzheimer’s disease. Mol. Med. Rep. 22, 1489–1497. doi: 10.3892/mmr.2020.11203
Keywords: microRNA, miRNA, polymorphism, SNP, RNA-bindig proteins, RBP, Alzhaimer’s disease
Citation: Moraghebi M, Maleki R, Ahmadi M, Negahi AA, Abbasi H and Mousavi P (2021) In silico Analysis of Polymorphisms in microRNAs Deregulated in Alzheimer Disease. Front. Neurosci. 15:631852. doi: 10.3389/fnins.2021.631852
Received: 11 December 2020; Accepted: 18 February 2021;
Published: 24 March 2021.
Edited by:
Hua Lou, Case Western Reserve University, United StatesReviewed by:
Peng Yao, University of Rochester, United StatesThomas LaFramboise, Case Western Reserve University, United States
Copyright © 2021 Moraghebi, Maleki, Ahmadi, Negahi, Abbasi and Mousavi. This is an open-access article distributed under the terms of the Creative Commons Attribution License (CC BY). The use, distribution or reproduction in other forums is permitted, provided the original author(s) and the copyright owner(s) are credited and that the original publication in this journal is cited, in accordance with accepted academic practice. No use, distribution or reproduction is permitted which does not comply with these terms.
*Correspondence: Pegah Mousavi, pegahmousavi2017@gmail.com
†ORCID: Mahta Moraghebi, orcid.org/0000-0003-0054-2080; Mohsen Ahmadi, orcid.org/0000-0003-4751-4212; Reza Maleki, orcid.org/0000-0001-6840-2653; Ahmad Agha Negahi, orcid.org/0000-0003-3420-1436; Hossein Abbasi, orcid.org/0000-0002-8160-453X; Pegah Mousavi, orcid.org/0000-0002-5654-7561