Exploring the Potential of Precision Livestock Farming Technologies to Help Address Farm Animal Welfare
- School of Agriculture, Policy and Development, University of Reading, Reading, United Kingdom
The rise in the demand for animal products due to demographic and dietary changes has exacerbated difficulties in addressing societal concerns related to the environment, human health, and animal welfare. As a response to this challenge, Precision Livestock Farming (PLF) technologies are being developed to monitor animal health and welfare parameters in a continuous and automated way, offering the opportunity to improve productivity and detect health issues at an early stage. However, ethical concerns have been raised regarding their potential to facilitate the management of production systems that are potentially harmful to animal welfare, or to impact the human-animal relationship and farmers' duty of care. Using the Five Domains Model (FDM) as a framework, the aim is to explore the potential of PLF to help address animal welfare and to discuss potential welfare benefits and risks of using such technology. A variety of technologies are identified and classified according to their type [sensors, bolus, image or sound based, Radio Frequency Identification (RFID)], their development stage, the species they apply to, and their potential impact on welfare. While PLF technologies have promising potential to reduce the occurrence of diseases and injuries in livestock farming systems, their current ability to help promote positive welfare states remains limited, as technologies with such potential generally remain at earlier development stages. This is likely due to the lack of evidence related to the validity of positive welfare indicators as well as challenges in technology adoption and development. Finally, the extent to which welfare can be improved will also strongly depend on whether management practices will be adapted to minimize negative consequences and maximize benefits to welfare.
Introduction
One of the biggest challenges our society is facing is the ability to feed a growing population, which is expected to reach around 9.7 billion people by 2050, while minimizing environmental impacts, ensuring human health (FAO, 2018), and addressing the public's rising concern over animal welfare (European Commission, 2016). In the UK, animal welfare standards have been a key subject of public concern, particularly with proposed changes to trade and agricultural policies in light of Brexit (Main and Mullan, 2017). In addition, there have been government commitments to achieving net zero and other environmental improvements in the Agriculture Act, Environment Bill and 25-years Environment Plan. The National Farmers' Union, e.g., has set a 2040 target for net zero emissions in the agriculture sector, and the Agriculture Act and associated plan to improve farm productivity indicates that English farmers can receive financial support to produce “public goods” such as environmental or animal welfare improvements (DEFRA, 2021).
However, meeting these commitments is challenging, not least because global meat production is expected to double by 20501. This increase in production may be achieved by a combination of expansion in animal numbers and increased productivity, which will be particularly important in the poultry and pig sector (Gilbert et al., 2015). While it is not possible to predict precisely what agriculture will look like in 2050 (factors such as income distribution, dietary choices and technological innovations will have an important influence), the FAO suggests that in a “business-as-usual” scenario, animal herds are likely to increase by 46% globally compared to 2012, with poultry numbers increasing over five-fold, three-fold for pigs, and two-fold for small and large ruminants (FAO, 2018). This increase in animal numbers could make their management more challenging, especially if, as was observed in the EU, the number of farmers continues to decrease (Eurostat, 2020). In the UK, while livestock numbers remained stable between 2018 and 2019, the labor force on commercial holdings decreased by 0.3% (DEFRA, 2019). Having fewer farmers to look after larger numbers of animals may make it more difficult to address animal health and welfare challenges.
As a response to these challenges, the development of new technologies has gained momentum. Among these developments are Precision Livestock Farming (PLF) technologies, which are designed to support farmers in livestock management by monitoring and controlling animal productivity, environmental impacts, as well as health and welfare parameters in a continuous, real-time and automated manner (Berckmans, 2014). A variety of systems using technologies such as sensors, cameras or microphones can directly alert farmers via connected devices (e.g., phones, computers, or tablets) about detected anomalies, thus allowing farmers to intervene at an early stage. Research is pointing toward the great potential for these “smart technologies” to help livestock farmers in monitoring the welfare of their animals and several countries are already investing in their development, reflecting their potential to be part of strategies to move toward sustainable agriculture (Rose and Chilvers, 2018; Norton et al., 2019).
While their potential is promising, the use of these new technologies also raises ethical concerns, such as their potential impact on the human-animal relationship, the objectification of animals, the notion of care and farmers' identity as animal keepers (Bos et al., 2018; Werkheiser, 2018, 2020). The human-animal relationship is an important aspect which can influence both animal welfare and productivity. The behavior of stock people, which is influenced by their attitudes toward farm animals, has an influence on animals' fearfulness toward humans, with positive behaviors leading to decreased levels of avoidance and negative handling increasing fearfulness toward humans (Hemsworth and Barnett, 1991; Waiblinger et al., 2002; Probst et al., 2012). In addition, it also influences productivity. For example, reduced milk yields were found on dairy farms where farmers had more negative attitudes toward interactions with cows during milking (Waiblinger et al., 2002). Aversive handling was also shown to impact the growth performance of pigs and negative relationships were found between level of fearfulness toward humans and egg production (Hemsworth and Barnett, 1991; Cransberg et al., 2000). On the other hand, habituation, early positive contact and genetic dispositions can be important factors to influence the quality of the HAR (Mota-Rojas et al., 2020). For example, studies found that young broiler chickens exposed to positive human contact had greater growth rates, and that positive attitudes were associated with more use of positive behaviors (Gross and Siegel, 1979; Lensink et al., 2000). If PLF technologies are used to facilitate and/or replace certain tasks involving human-animal interactions and to reduce time spent on observing individual animals by ‘replacing farmers eyes and ears’ (Berckmans, 2014), it could be questioned whether PLF could impact the HAR by reducing the frequency of human-animal interactions and impacting farmers' attitudes toward their animals and hence their behavior. Animals may have less opportunity to become habituated to people and farmers if the frequency of neutral or positive interactions is reduced (this may be particularly true on larger farms where opportunities for human-animal contacts are usually reduced) (Rushen et al., 1999; Cornou, 2009; Mota-Rojas et al., 2020). Similarly, concerns were also raised in regards to the extent to which PLF could redefine the notion of care, and whether farmers attitudes may shift further toward reducing animals to “tracking devices” and focus primarily on productivity (e.g., disease prevalence or costs of medical treatments) while overlooking the animal's qualitative experiences (Bos et al., 2018).
Taking these benefits and ethical challenges into consideration, it seems important to evaluate the extent to which these technologies can actually address the issue of animal welfare. The notion of animal welfare is complex to define and, while the focus has long revolved around minimizing negative experiences such as pain and suffering, studies in animal behavior and neuroscience have led scientists to highlight the importance of positive affects in animal welfare (Boissy et al., 2007; Yeates and Main, 2008). Affective states relate to feelings or emotions which can vary in intensity, duration, level of arousal and how pleasant or unpleasant they are. While survival-related affects reflect the animal's internal physiological state (e.g., thirst or hunger), situation-related affects reflect the animal's perception of its external circumstances (e.g., comfort, playfulness, depression, loneliness) (Mellor, 2015a). Positive animal welfare cannot be achieved with a sole emphasis on minimizing negative experiences; opportunities to experience positive affects (e.g., by allowing animals to engage in rewarding goal-directed behaviors such as through affiliative interactions, exploring, or play) must also be provided (Mellor and Beausoleil, 2015). Taking these aspects into account, the “Five Domains Model” (FDM) has been developed to facilitate the assessment of animal welfare and considers both negative and positive affective states (Mellor and Beausoleil, 2015). The first three domains (labeled “nutrition,” “physical environment,” and “health”) include survival-related factors, while the fourth (labeled “behavioral interactions”) includes situation-related factors. Based on these four domains it is then possible to evaluate the associated affective consequences within a fifth domain, “mental state” (Mellor et al., 2020). The method can be updated using the latest scientific evidence in animal welfare and can be used in different animal-related sectors (Mellor, 2017).
Using the FDM as a framework, this study thus aims to understand better the potential of PLF technologies to help address the notion of animal welfare by looking at a non-exhaustive, yet wide range of technologies. To this end, PLF developments in a variety of farmed species were identified along with their development stages to distinguish better between commercially available technologies and technologies that are further away from being fully developed. Secondly, the potential welfare benefits and risks of PLF are explored along with their potential ability to promote/address affective states.
Methods
Identification of PLF Technologies
A combination of methods was used to identify PLF technologies. These include searches on scientific papers databases, visiting technology exhibitions, input from colleagues as well as during a workshop organized by the author. These methods are further described below.
Research Papers
Search Criteria
The databases Scopus (Elsevier) and Web of Science were used to search for papers relevant to this study. The search was conducted between February and April 2020. Only research articles were selected, with no limits on date of publication. Each search included: a keyword related to Precision Livestock Farming, a species, and either the words “welfare,” “health,” or “behavior” (see Table 1). Considering the variety of methods that could relate to PLF technologies, the selection of PLF-related keywords was based on categories that were commonly being referred to in related literature reviews (Benjamin and Yik, 2019; Halachmi et al., 2019; Li et al., 2019; Norton et al., 2019; Astill et al., 2020). These include the use of image-based technology (e.g., using 2D or 3D cameras, computer vision, optical flow, thermal cameras), sound (e.g., using microphones or sonars), sensors [e.g., using accelerometers, pressure or infrared sensors (IR)], Radio-Frequency Identification (RFID) and wireless technologies. It is acknowledged that by using these specific keywords and databases, other types of technologies may have been omitted. It was not the goal of this paper to review all possible PLF technologies for all species, but rather to obtain a general view of current developments and discuss how these apply to animal health and welfare monitoring. The species were selected on the basis of being the main species farmed in the UK. To complete our search, relevant papers referenced in review articles and not present in the databases were also considered.
Selection of Papers
Only accessible papers written in English were considered. From the author's understanding based on the literature and the workshop organized by the author (of which more details can be found in Section Workshop), the definition of PLF technologies can be understood differently by different people. In this study, PLF refers to technologies that are, or have the potential to be, automated, and allowing to monitor animal health, welfare, and environmental parameters continuously and in real-time. Technologies such as virtual fencing or milking robots were, e.g., not considered in our study. Papers were selected when the aim of the study was to present a method to automatically monitor farm animal health, welfare, or behavior parameters. These included, e.g., monitoring lameness, respiratory diseases, heat, body temperature, or environmental conditions. Methods at various stages of development were considered, from proof-of-concepts to validated, fully automated systems. Papers were not selected when the purpose was mainly to refine existing models or algorithms such as to improve image resolution or the detection of certain parts of the body (as they were not about PLF systems in themselves). Papers were also not selected when they addressed transport or post-slaughter issues, when they applied to other contexts than farming (e.g., monitoring of wild animals or applications for laboratory studies), or when authors concluded that the proposed methods did not present satisfying enough results for the purpose of their study.
Commercialized Technologies
Commercially available PLF technologies were found in several ways, including visiting technology exhibitions, finding mentions in research or news articles, getting recommendations from colleagues, as well as during a workshop organized by the author of this paper (see next section). When mentions of particular technologies were found, the websites of the relevant companies were visited, and technologies were selected when they allowed to automatically monitor health and welfare parameters of farm animals.
Workshop
The workshop, called “Current developments in Precision Livestock Farming (PLF) technologies: What can we measure and what are the welfare benefits and challenges' was funded by the Animal Welfare Research Network (AWRN) and organized by the first author. Over 150 international participants registered to the online workshop, however, places were limited to 100 participants due to the video conferencing software used (Zoom 5.4). Participants were selected on a “first come, first served” basis with the condition of participants having to be members of the AWRN (or currently applying to become a member). Approximately 90 participants logged in at the start of the workshop, which included researchers and students (59 and 15%, respectively), industry workers (8%), NGOs (4%), vets (4%), civil servants (4%), assurance schemes workers (4%), and farmers (2%). Most attended the workshop activities, and ~70 participants remained until the end. Four 30 min keynote presentations (including questions and answers) and two, 1-h activities (including presentations of the outcomes by the participants) allowed the participants to discuss current developments in PLF for several species (from proof-of-concepts to commercially available technologies), their benefits, potential challenges (to animal welfare and beyond) and solutions. An outline of the workshop is presented in Annex 1. Participants were split into eight different groups during the activities, each focusing on one or two livestock sectors. During the first activity, participants were asked to discuss up to five commercially available and up to five “promising” PLF technologies that have the most potential to improve animal welfare for their selected species, and to qualitatively discuss the chosen technologies' potential benefits to welfare. Volunteers in each group presented the outcomes of their discussion to the rest of the participants in 3 min each, sometimes using visual support showing notes taken during the discussions (e.g., whiteboard from the Zoom software or via a Microsoft PowerPoint slide). In a second activity, participants (divided into the same groups) were asked to qualitatively discuss the risks and challenges of using PLF technologies (to their species and beyond), and how these could be minimized. Results were presented in the same way as for the first activity. The first author took notes during these presentations and collected copies of the whiteboards or PowerPoint slides where available. The outputs of these discussions, as well as technologies and welfare benefits and risks mentioned during the keynote presentations, were used to complement the findings of this study (e.g., if the author had omitted specific technologies or benefits and risks that were not initially identified). As the outcomes of the workshop related to animal welfare, but also to aspects beyond the scope of this study (since they are closely related with other aspects such as impacts on farmers, consumers and other stakeholders), only the outcomes directly related to animal welfare (PLF technologies, benefits and risks to welfare) were used to complement the findings of this review.
Classification
The different technologies found using the above methods were classified by the first author of this paper with the help of the co-authors and a colleague (expert in the fields of agricultural technologies and animal welfare) in tables according to their type (e.g., image, sensors, sound, RFID, bolus), their application (e.g., detection of lameness or estrus) and their development stage categories. Each table was associated to a Physical/Functional Domain of the Five Domains Model (“nutrition,” “physical environment,” “health,” or “behavioral interactions”). The technologies' potential welfare benefits and risks and their potential to address affective experiences based on the fifth domain (“mental state”) were also discussed.
Technology Types and Applications
To simplify the tables, the “technology type” category was kept broad. For example, although technically different, accelerometers and infrared sensors were both classified into a broader “sensor” category. Similarly, some applications were grouped within categories. For example, the applications related to “feed intake,” “grazing,” “jaw movements,” “rumination,” or “bites” were all grouped into the “feeding behavior” category. Similarly, “ammonia concentrations” or “particle matter concentrations” were classified into the “air/water quality” category. The category “disease/parasites monitoring” includes technologies aiming to detect ill animals with diseases/parasites such as Bovine Respiratory Disease (BRD) or sea lice in salmon. “Activity” included behavior monitoring such as walking, standing, lying or swimming. The technologies were classified according to the specific aims of the papers. For example, when the aim was to determine whether a technology could accurately detect walking and lying patterns, the technology was placed into the “activity” category within the “behavioral interactions” domain. Similarly, when the specific aim was to accurately detect estrus in cattle, the technology was placed into the “estrus” category within the “health” domain, even if the technology was based on activity data.
Development Stages
The development stage categories were inspired by the Technology Readiness Levels (TRLs) developed by the National Aeronautics and Space Administration (NASA). The technologies were assigned within three categories which are broadly comparable with TRLs: “proof-of-concept phase” (P1), “validation phase” (P2) and “commercialization phase” (P3). Technologies were assigned into the P3 category when the systems were commercially available. Papers which included steps to validate specific technologies or where further papers were published to validate the method were assigned into the P2 category, while those which did not were classified into the P1 category. When several papers addressed a similar application with a similar type of technology, only the highest category was shown. It is acknowledged that the grouping into wider categories may not allow to precisely reflect the state of development of each different type of technologies, especially as developments and further validation may have occurred between the initial search and the writing of the paper or may have been omitted due to the restricted number of keywords. Instead, it allows to obtain an overview of current developments and to discuss their potential to address animal welfare.
Welfare Implications
The classification into the different domains was based on the updated Five Domains Model (FDM) table developed by Mellor et al. (2020). Classification under the first four physical domains was based on the parameters monitored by the technologies (e.g., technologies monitoring feeding behaviors were classified into the “nutrition” domain, while technologies monitoring lameness were classified under the “health” domain). Discussions on affective states were based on the FDM table which provides examples of positive and negative factors with their associated inferred negative or positive affective experiences from the fifth domain. For example, under the “physical environmental conditions” section of the table, “air pollutants: NH3, CO2, dust, smoke” is associated with the negative affects “respiratory discomfort” (e.g., breathlessness, air passage irritation/pain). For this reason, if a technology was designed to help farmers monitor air pollutants such as NH3, the author suggested that the use of such technology could have an impact on respiratory discomfort. Similarly, a technology monitoring water intake would have been suggested to have a possible impact on the associated negative affect “thirst.” Where there were many affects associated with specific factors, only a few examples were suggested to avoid lengthy paragraphs. For example, the FDM indicates that the presence of injuries or diseases may be associated to the following negative affects: pain (many types), breathlessness, debility, weakness, sickness, malaise, nausea, and dizziness. To avoid listing all possible affects, the authors selected either those related to a specific condition (such as breathlessness related to respiratory diseases) or those that were most likely to be understood by a wider audience (such as feelings of sickness resulting from diseases). Finally, welfare benefits and risks were discussed both in relation to the specific domains and across domains in a separate section (Section Welfare Benefits and Risks Across Domains). These were identified in the research papers found in this study, technology company websites, within the wider PLF literature and during the workshop.
Results
Research Paper Selection
The search revealed 793 research articles in total. After manual selection of papers which we considered relevant to our study, we retained 247 papers. Excluded papers included those that did not focus on specific PLF technologies, papers related to technologies other than PLF, papers that were not accessible or that were in a language other than English. Excluded papers also included duplicates, papers that did not relate to farming or to the species of interest (such as wild or laboratory animals) or that addressed stages of production which we did not consider in this review (e.g., slaughter). A number of excluded papers also included those that were not related to animals (e.g., human medicine). Selected papers included 101 papers related to cattle, 68 to pigs, 37 to poultry, 15 to fish, and 26 to other species (including turkeys, goats, and sheep).
In the following sections, technologies relating to the physical/functional domains of the Five Domains Model are described along with a discussion on their domain-specific welfare implications based on the fifth domain. These are followed by a section (Welfare Benefits and Risks Across Domains) on welfare benefits and risks across domains.
Nutrition
The monitoring of drinking and feeding behaviors (which includes grazing, ruminating, jaw movements, chewing, or feed intake), and gastrointestinal health were the main applications related to the “nutrition” domain (Table 2). The cattle sector appears to benefit from a wider variety of PLF technologies at later development stages in comparison to other species, although commercially available technologies can also be found for pigs, poultry and fish. For small ruminants, technologies mainly range from the proof-of-concept phase “P1” to the validation phase “P2.”
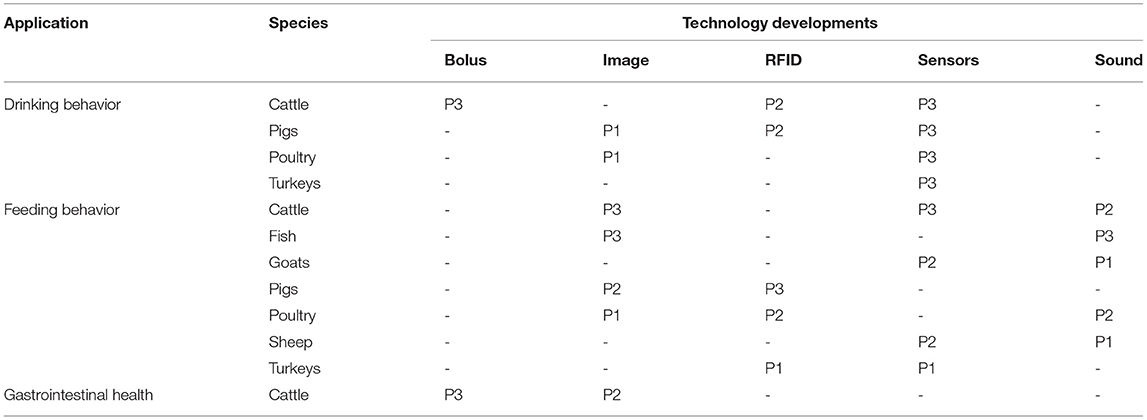
Table 2. Development stages of PLF technologies related to the “nutrition” domain of the Five Domains Model for different species (expressed in phases—P1, proof-of concept stages; P2, validation stages; P3, commercialization phases).
Commercially Available Technologies
In cattle, smart camera systems using computer vision combined with deep learning can monitor eating time and feed availability at group level, while neck collars equipped with 3D accelerometers continuously monitor rumination and eating time in individual animals. Gastrointestinal health can also be monitored using boluses sitting in cattle reticulum which measure pH and temperature. In pigs, RFID ear tags are used as part of electronic feeding systems, while in the aquaculture sector, hydroacoustic-based technologies and cameras combined with machine learning allow to monitor fish pellet consumption and appetite. Finally, water consumption can be monitored with commercially available boluses in cattle and with sensors in cattle, pigs, and poultry.
Technologies in Development
Other systems which are currently in the development stages (categories P1 to P2) can monitor ingestive behaviors in free-ranging cattle, goats and sheep using acoustic monitoring (Navon et al., 2013; Chelotti et al., 2016). In poultry, Aydin (2016) developed a sound-based monitoring system to detect short-term feeding behaviors of broiler chickens by recording pecking sounds. RFID systems have been used to monitor feeding patterns in pigs (Maselyne et al., 2016b; Adrion et al., 2018), turkeys (Tu et al., 2011) and laying hens (Li et al., 2017). Image analysis and binocular vision techniques have been developed to monitor feeding in pigs (Yang et al., 2020) and poultry (Xiao et al., 2019), while sensor-based systems can monitor feed intake in goats (Campos et al., 2019) and turkeys (Chagneau et al., 2006). Technologies at phase P2 also introduced the possibility to use 3D-vision to automatically assess reticulo-ruminal motility in cattle (Song et al., 2019). Finally, drinking behavior can be monitored using RFID in pigs (Maselyne et al., 2016a) and a combination of sensors and RFID have been used in cattle (Williams et al., 2020). Accelerometers have been used to monitor drinking in calves (Roland et al., 2018), while camera-based systems have been developed to monitor drinking behavior in pigs (Kashiha et al., 2013a) and chickens (Xiao et al., 2019).
Welfare Implications
Using PLF to monitor drinking and feeding behaviors and gastrointestinal health could help provide additional support to minimize the experience of survival-related negative affects such as thirst, hunger or gastrointestinal pain. As changes in drinking or feeding patterns can be indicative of health compromises such as diseases (Nicol, 2011), we suggest that feelings of sickness could be minimized provided that farmers are taking adequate management decisions based on the data (e.g., providing animals with appropriate resources or treatment). In parallel, positive affects such as comfort of good health, gastrointestinal comfort and pleasures associated with drinking and eating could be promoted. However, studies suggest that positive affective states relating to most survival-related factors are usually short-lived (Mellor and Beausoleil, 2015), hence these technologies may mainly have an impact on the negative-to-neutral valence range.
Physical Environment
Table 3 shows that air or water quality, animal crowding and distribution and heating/ventilation are the main applications related to the “physical environment” domain. The monitoring of environmental factors is generally based on image and sensor technologies in the fish, poultry and pig sectors, most of them being commercially available.
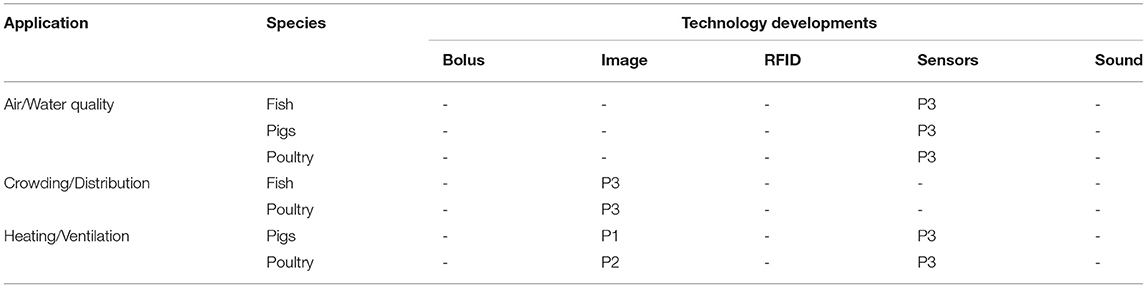
Table 3. Development stages of PLF technologies related to the “physical environment” domain of the Five Domains Model for different species (expressed in phases—P1, proof-of concept stages; P2, validation stages; P3, commercialization phases).
Technology Developments
The monitoring of air/water quality includes the detection of a variety of parameters such as toxic molecules concentrations, pH, CO2, temperature, or oxygen levels which can have important impacts on animal health and welfare. Sensors are commercially available to measure these environmental variables in the aquaculture, poultry and pig sectors. They are also available to monitor heating and ventilation in pig and poultry barns, while image-based systems using animal postures or distribution are still in early development stages (P1 to P2) (Shao et al., 1997; Xin, 1999; Kashiha et al., 2013b). Finally, animal distribution can be detected with commercially available cameras in the aquaculture and poultry sector.
Welfare Implications
Monitoring environmental parameters could help address negative affective experiences by minimizing thermal, physical, respiratory and olfactory discomfort due to inappropriate temperatures or, e.g., inappropriate levels of ammonia. Ensuring optimal environmental conditions could benefit welfare by minimizing risks of infectious and respiratory diseases and heat stress, as well as promoting feelings of comfort. In addition, monitoring animal distribution can also indicate welfare compromises or equipment malfunctions (e.g., heating or ventilation systems) (Kashiha et al., 2013b). The potential impacts on survival-related affective experiences remain within the negative-to-neutral valence range.
Health
A variety of technologies at different development stages monitor parameters related to the “health” domain, from specific diseases to foot health and stress, as well as physiological parameters such as heart rate or temperature. Most commercially available technologies appear to apply to cattle, but they can also be found for pigs, poultry, as well as for sheep and fish (Table 4).
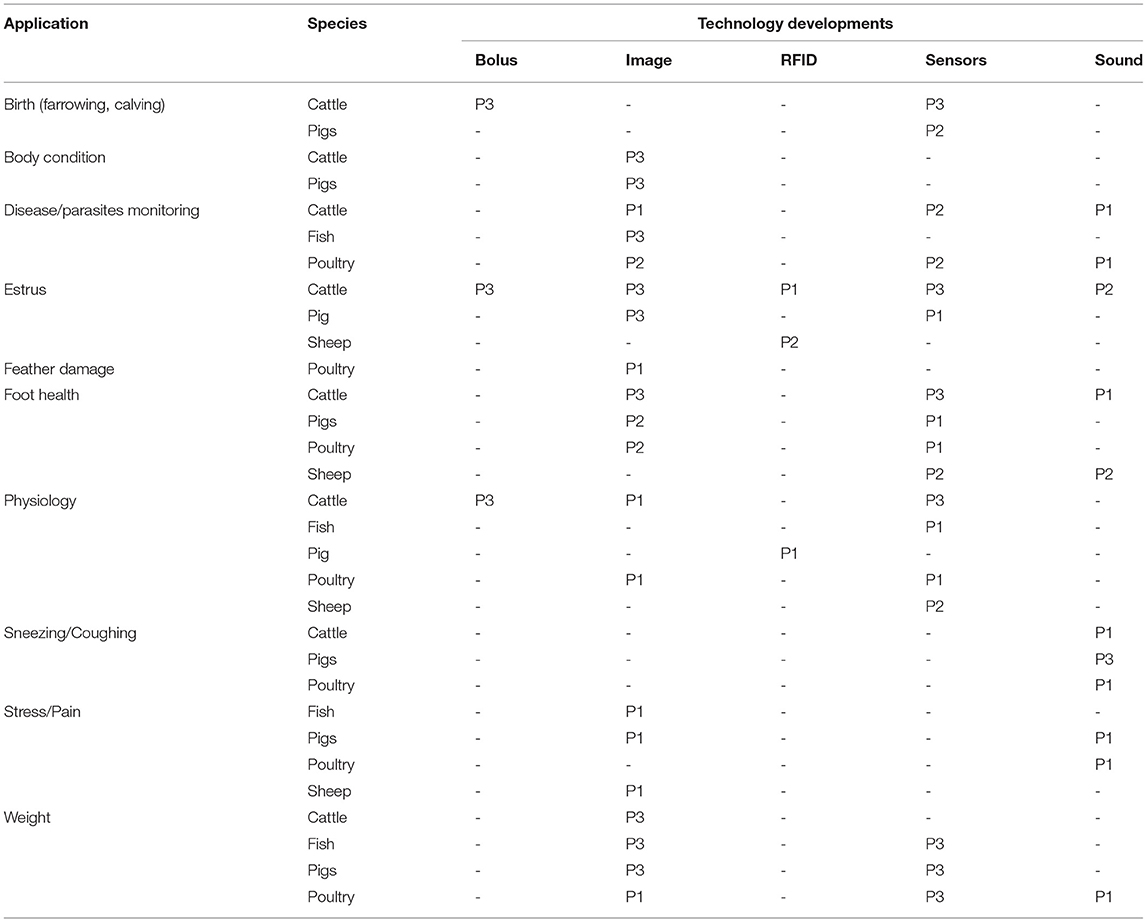
Table 4. Development stages of PLF technologies related to the “health” domain of the Five Domains Model for different species (expressed in phases—P1, proof-of concept stages; P2, validation stages; P3, commercialization phases).
Commercially Available Technologies
In cattle, body-mounted accelerometers can be used to detect calving, estrus and lameness based on activity data, while cameras combined with machine learning can help determine standing heat, body condition scores (BCS), assess lameness, and estimate weight. Boluses placed in the reticulum can also be used to monitor estrus, calving and physiological factors such as body temperature or pH, and ear sensors can monitor temperature. In the pig sector, camera-based systems can determine BCS, estrus and weight, while microphones placed in barns can detect coughing sounds and monitor respiratory health. In aquaculture, image-based systems can allow the detection of sea lice, and sensors and cameras can estimate fish growth. Finally, automatic weighing systems are available to detect the average weight of poultry flocks.
Technologies in Development
Growth rate can be measured in broiler chickens using technologies at development stages ranging from P1 to P2, using sound analysis (Fontana et al., 2015, 2017) or 3D cameras (Mortensen et al., 2016).
Estrus in cattle can be monitored based on individual vocalizations and caller identification (Röttgen et al., 2020) or with proximity loggers (Corbet et al., 2018). This can also be monitored using RFID technology in sheep (Alhamada et al., 2016), while sensor-based systems can detect pig farrowing (Manteuffel et al., 2015; Pastell et al., 2016; Liu et al., 2018).
Diseases such as mastitis in cattle or campylobacter infection in chickens can be monitored using sensor, sound and image-based technologies at phases P1 and P2 both in poultry (Okada et al., 2014; Banakar et al., 2016; Colles et al., 2016; Grilli et al., 2018) and cattle (Steensels et al., 2016; Vandermeulen et al., 2016; Yazdanbakhsh et al., 2017; Zaninelli et al., 2018; Watz et al., 2019).
Physiological parameters such as respiration rate, temperature or heart rate can be monitored in cattle using image or sensor-based technologies at development stages P1 to P2 (Nogami et al., 2013; Stewart et al., 2017; Strutzke et al., 2019) as well as in poultry (Hyun et al., 2007; Xiong et al., 2019), fish (Martos-Sitcha et al., 2019), and sheep (Dos et al., 2018; Fuchs et al., 2019).
Lameness can be detected in pigs using images and sensors (Pluym et al., 2013; Stavrakakis et al., 2015), while gait scores can be evaluated in poultry using optical flow and sensors (De Alencar Nääs et al., 2010; Dawkins et al., 2017; Van Hertem et al., 2018). Sensors can be used to detect lameness in sheep (Shrestha et al., 2018; Kaler et al., 2020) and sound-based systems to monitor lameness and foot lesions in cattle (Volkmann et al., 2019).
Technologies in the P1 and P2 phases can monitor coughs in cattle (Carpentier et al., 2018) and sneezing in poultry using sound-based technologies (Carpentier et al., 2019). Similarly, stress or signs of pain can be monitored in pigs (Schön et al., 2004) and poultry (Lee et al., 2015), as well as by using camera-based technologies in fish (Israeli, 1996), pigs (da Fonseca et al., 2020) or sheep using facial recognition (McLennan and Mahmoud, 2019). Finally, image processing can be used to detect asphyxia in sows during parturition (Okinda et al., 2018) or to predict feather damage in poultry (Lee et al., 2011).
Welfare Implications
The identified technologies could help address animal affective experiences such as pain, weakness or sickness emanating from diseases or physical injuries. For example, the early detection of coughing can indicate the onset of respiratory diseases which, if treated adequately, have the potential to prevent the experience of breathlessness which can cause significant threats to welfare (Beausoleil and Mellor, 2015). Similarly, monitoring foot health or predicting feather pecking outbreaks in poultry could help minimize painful experiences provided that appropriate management decisions are taken. This in turn could promote feelings of comfort linked to good health and functional capacity. In some cases, the automatic detection of estrus, whilst mostly beneficial for productivity, could reduce the need for stressful handling (e.g., in pigs), hence potentially addressing negative affective states such as anxiety or fearfulness. As for the “nutrition” and “physical environment” domains, the impacts on affective experiences remain within the negative-to-neutral valence range. As highlighted during the workshop, the early detection of diseases could help reduce their spread and support management decisions such as early interventions, better colostrum management, reducing the use of antibiotics, reducing stressful handling or preventing injurious events such as feather pecking.
Behavioral Interactions
Many PLF technologies are based on animal activity patterns, such as lying, walking/swimming or standing. As shown in Table 5, commercially available systems to monitor activity have been developed for most farmed species, particularly using image- and sensor-based technologies. Other technologies have been developed to detect agonistic behaviors, as well as social interactions and maternal behaviors in pigs, cattle and poultry. However, those generally remain at earlier development stages (P1 to P2).
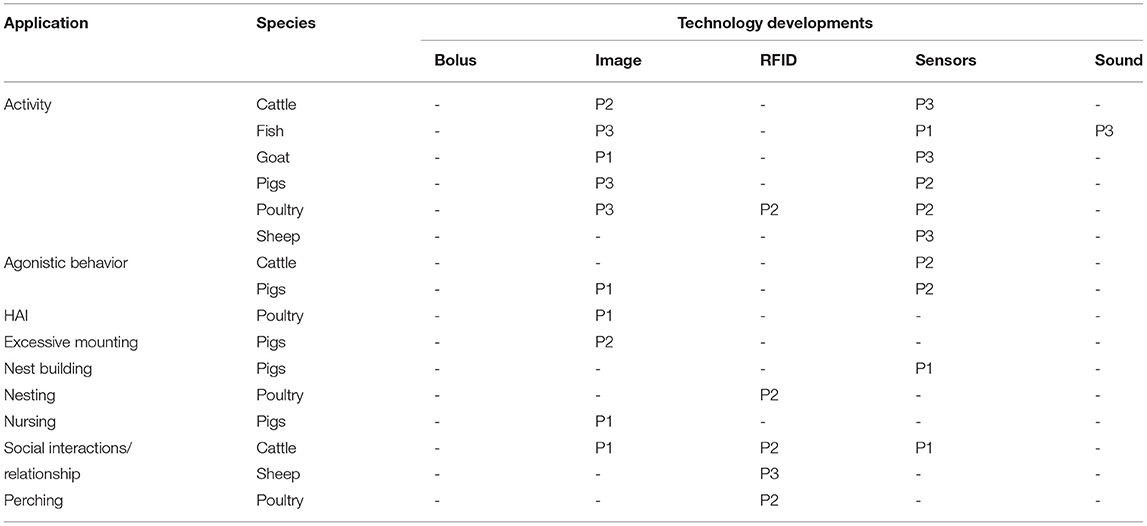
Table 5. Development stages of PLF technologies related to the “behavioral interactions” domain of the Five Domains Model for different species (expressed in phases—P1, proof-of concept stages; P2, validation stages; P3, commercialization phases).
Commercially Available Technologies
Accelerometers are mostly available for ruminants and are usually attached to the animals' bodies and allow to monitor behavior, location, or postures of individual animals such as lying, standing or walking. Image-based systems can be found in the aquaculture, pig and poultry sectors, whilst hydroacoustic-based systems allow to monitor fish movements. In sheep, pedigree match makers using RFID tags can be used to identify the maternal pedigree of lambs and to monitor behavior traits of lambs and ewes in extensive systems, which could provide information on potential changes in relationships (Brown et al., 2011; Morris et al., 2012).
Technologies in Development
Other technologies at earlier development stages can help monitor activity, such as drones in goats (Vayssade et al., 2019), RFID in poultry (Zhang et al., 2016) and sensors in fish (Martos-Sitcha et al., 2019), pigs (Mainau et al., 2009; Thompson et al., 2016), and poultry (Quwaider et al., 2010; Van Der Sluis et al., 2019). In pigs, tail biting or fighting can be monitored using depth sensors (Lee et al., 2016; Chen et al., 2019), 3D cameras and computer vision (Viazzi et al., 2014; D'eath et al., 2018). Excessive mounting can be detected using image analysis (Nasirahmadi et al., 2016), while nest building can be detected using accelerometer data (Oczak et al., 2015). Nursing behavior can also be monitored using video analysis (Yang et al., 2019). In cattle, systems have been developed to monitor agonistic behaviors based on sensors (Foris et al., 2019), while image-based technologies can monitor mounting behaviors (Chung et al., 2015; Guo et al., 2019) and social interactions (Guzhva et al., 2016), and accelerometers can estimate locomotor play in calves (Luu et al., 2013). Proximity interactions of individual dairy cows within large herds can also be monitored using local positioning sensor network (Chopra et al., 2020). Image- and RFID-based technologies in the poultry sector allow to monitor human-animal interactions (HAI) (Lian et al., 2019), nesting (Li et al., 2017), and perching behaviors (Nakarmi et al., 2014; Wang et al., 2019). Finally, RFID can used to explore social behavior in cattle such as cow-calf affiliations (Swain and Bishop-Hurley, 2007; Boyland et al., 2013).
Welfare Implications
The monitoring of specific behaviors and situation-related factors could help to obtain a better understanding of levels of welfare and help evaluate animals' responses to their environment as well as supporting management decisions that may promote the experience of positive affects and minimize negative ones, hence having an impact on the negative-to-positive valence range. Ensuring that animals can engage in natural and rewarding behaviors which are important for their welfare such as nest building or nursing in pigs, social interactions in cows and sheep or nesting and perching in poultry, could indeed help minimize feelings of frustration and promote affects such as feeling maternally rewarded, protected or socially engaged. In pigs e.g., monitoring nest building behaviors can help decrease the time sows are kept in farrowing crates without increasing piglet mortality, while monitoring nesting or perching behaviors in poultry can help in housing system design and management. In addition, being able to monitor agonistic behaviors can provide a better understanding of how social relationships (e.g., dominance) are influenced by the animals' environment and to encourage measures that will help minimize fearfulness or anxiety by reducing risks of aggression and injuries, while promoting feelings of security. Finally, monitoring the HAR could have important impacts on animal welfare if adequate measures are put in place to reduce the occurrence of negative interactions and promote positive ones (e.g., gentle as opposed to rough handling, or talking softly as opposed to shouting).
Welfare Benefits and Risks Across Domains
In addition to the domain-specific welfare impacts suggested above, more general welfare benefits of the identified technologies include the potential to help support management decisions such as early intervention to ensure good health, reduce the use of antibiotics and prevent disease outbreaks, sometimes in systems where monitoring can be difficult (e.g., in extensive systems or where large numbers of animals are kept together). In addition, monitoring animals at individual level (e.g., using body-mounted devices or boluses) could help better understand the animals' specific needs.
During the workshop, questions were raised as to whether the use of wearable sensors (or those placed inside the animals) could cause discomfort or potential injuries to the animals. Ear tags e.g., which are often required for identification and traceability purposes can be a potential source of damage to the animals' ears, with severity depending on the type of tag (Edwards and Johnston, 1999). Although sensors in the form of neck collars do not require the same type of interventions, their potential impacts on animal behavior and welfare should be further studied. In addition, some of the technologies do not yet allow the monitoring of individuals, but do so at group level (in particular on farms with high number of animals e.g., poultry or fish). While these technologies could be beneficial for the detection of welfare compromises, the interpretation of the data must be done carefully, as management decisions made at group level could be detrimental for the welfare of those individuals whose needs differ from others (e.g., different nutrition or treatment requirements). For example, group monitoring of feed or water intake may not reflect social competition, which may hence be overlooked. Although studies have looked at possibilities to identify competitive interactions at the feedbunk, those were considered not practical due to high costs and labor (Huzzey et al., 2014).
Other concerns relate to the potential to reduce the frequency of visual or physical examination which could impact stockpeople's attitudes and behavior toward their animals, hence having a potential effect on the human-animal relationship (HAR) and animal welfare. This could be particularly problematic on systems with larger numbers of animals (e.g., poultry or aquaculture), where opportunities to become habituated to people are already limited. Finally, over-reliance on PLF technologies, which was also a concern raised during the workshop, could increase risks of harm if system failures were to occur, in particular where systems are fully automated.
Discussion
The results from this study indicate that while PLF technologies can have a variety of benefits and may have a good potential to help minimize negative experiences, their current ability to contribute to promoting positive welfare remains limited. In addition, there are welfare risks associated with their use which must be considered, such as their potential impact on the human-animal relationship or to animal management. As Buller et al. (2020, p. 5) argue, “If it is to make a substantive contribution to addressing genuine animal welfare concerns, PLF technology must therefore address […] the effective monitoring and identification of systemic welfare failures and the active enhancement of opportunities for positive welfare experiences.”
A wide range of commercially available technologies aim to reduce the occurrence and impact of health issues, such as sensors detecting lameness in cattle, microphones monitoring respiratory health in pigs, or cameras monitoring the presence of parasites in fish. They are also widely available to monitor and improve productivity such as growth in poultry or estrus in dairy cattle to increase pregnancy rate and optimize insemination. Most technologies monitoring parameters related to the “nutrition,” “physical environment,” and “behavioral interactions” domains such as feeding or drinking behavior, air/water quality or activity are also designed with the aim to optimize productivity and to minimize the impacts of diseases. Indeed, changes in feeding or drinking behaviors can indicate signs of illnesses (Nicol, 2011), while inappropriate environmental conditions can be detrimental to animal health and lead to increased mortality (Zhang et al., 2011; Segner et al., 2012). Finally, a variety of technologies that are still in early development stages have focused on preventing the occurrence of undesired behaviors which can cause significant injuries such as tail biting in pigs or feather pecking in poultry (Bilcik and Keeling, 1999; Di Giminiani et al., 2017).
The use of these technologies could have important benefits for welfare if the data are used to support farmers in making effective management decisions. Indeed, PLF could allow the early detection of health issues and reduce the occurrence of negative affective experiences, such as pain resulting from lameness or breathlessness caused by respiratory diseases. In their study e.g., Taneja et al. (2020) developed a system which allowed to detect lameness 3 days before it was visually captured by farmers, with an accuracy of 87%. Berckmans et al. (2015) showed that respiratory problems in pigs were detected up to 2 weeks earlier compared to farmers' and veterinarians' routine observations, thanks to a sound based PLF system. In addition, Kashiha et al. (2013b) developed a system which allowed to detect issues in broiler houses based on animal distribution index, which enables early intervention to minimize impacts on bird welfare. Timely detection of diseases could help reduce the need for antibiotics hence responding to the major global issue which is antimicrobial resistance resulting from the excessive use of antibiotics affecting both animals and humans (Trevisi et al., 2014; McEwen and Collignon, 2018). In addition, PLF could also allow monitoring larger numbers of animals more easily (e.g., using wearable sensors to monitor health status or smart cameras to monitor larger groups), including on extensive systems where the detection of sick or injured animals is often difficult (Rutter, 2014), as well as reducing potential stress resulting from repeated handling and moving of animals (e.g., manual weight detection in pigs) (Kashiha et al., 2014). Furthermore, the use of PLF technologies could also help other actors (e.g., veterinarians or farm advisors) support more efficient and farm-specific management decisions based on the data collected, although this may require improvements in relation to the sharing of data (Rojo-Gimeno et al., 2019).
While health is undeniably an integral part of animal welfare, it does not in itself guarantee “good” welfare. Studies in neuroscience indicate that negative affective states relating to most survival-related factors, such as thirst or hunger, can at best be neutralized and do not necessarily lead to anything more than short-lived positive welfare states (Mellor and Beausoleil, 2015). Minimizing these negative experiences can therefore shift a negative welfare state toward a more neutral one. However, moving toward a positive welfare state requires opportunities to live positive experiences. These include, e.g., affiliative interactions, play, or autogrooming, which are believed to have rewarding properties, and have the potential to indicate positive affective states (Boissy et al., 2007). Mellor (2015b, p.21) hence suggested that “welfare reference standards should now be chosen to more strongly reflect a need for such [welfare-enhancing exploratory, foraging and affiliative behaviors] opportunities to be provided.” Some of the technologies identified in this study monitor these types of behaviors (e.g., play and social interactions in cattle, nest building behaviors in pigs or perching in poultry), however, at present, they appear to be mostly at early development stages.
The use of such technologies could help getting a better understanding of aspects of welfare that have often received less attention and help support management decisions that could improve animal welfare by promoting positive affects such as feeling engaged, confident or being maternally rewarded, and by minimizing negative ones such as fearfulness or frustration from not being able to express natural behaviors. In pig production e.g., sows are often kept in farrowing crates during parturition to restrain their movements and avoid piglets from being crushed. In those conditions, pre-partum sows are not able to perform nest-building behaviors, which they are highly motivated to perform to provide shelter and comfort to their young (Wischner et al., 2009). Predicting the onset of farrowing using automated monitoring systems could therefore help in management decisions such as restricting the time sows are kept in farrowing crates only to the critical period where piglets are most vulnerable, hence providing the sows with opportunities to perform those highly motivated behaviors (Oczak et al., 2015), and potentially having an effect on the negative-to-positive valence range.
It could be argued that, while positive animal welfare has gained increased attention in animal welfare science, further research is still required regarding the feasibility, validity and reliability of positive welfare indicators, making their current applicability within welfare assessment protocols difficult. For example, while play behavior appears to be a valid indicator of positive welfare as it only occurs when all other needs are met (Held and Špinka, 2011), the low incidence of this behavior in farming conditions makes it difficult to use as part of current welfare assessments (Jensen and Kyhn, 2000; Napolitano et al., 2016). Similarly, while social licking can have positive effects on individual cows, the behavior might also reflect social tension within a herd (Napolitano et al., 2016). As raised by participants of the workshop, one particular challenge to technology development and implementation in the aquaculture sector may also be related to the existing debate around whether fish can feel pain or experience particular emotions despite growing evidence suggesting that they do (Sneddon, 2019). This limitation in terms of validity and feasibility could explain why technologies with a potential to monitor and promote positive welfare are still in early development stages. Progress is however made in this area: a recent study reviewed promising valid and reliable positive welfare indicators that could be used in welfare assessments of ruminants (Mattiello et al., 2019). These indicators were mostly related to the physical environment, behavioral interactions and mental state domains of the FDM and included, e.g., ear or tail posture, half-closed eyes, low-frequency calls or ruminating. From a technical point of view, it would appear that developing technologies monitoring these types of indicators is possible, as a variety of systems identified in the present study have been developed to monitor specific postures, vocalizations or behaviors such as rumination.
Another important aspect to consider in addition to technical feasibility is whether these particular types of technologies would likely be adopted by farmers since the widespread uptake of precision technologies thus far has been rather slow, including in dairy farming as a result of “innovation uncertainty” (Eastwood and Renwick, 2020). In their study, Vigors and Lawrence (2019) interviewed farmers on their perception of positive animal welfare and found that as a whole, farmers prioritized the reduction of negative experiences, and mostly considered that by doing so, positive welfare would arise as a result. Most of the interviewed farmers considered that different positive welfare indicators such as social interaction or play did not require farmers' direct input or management (except from preventing negative interactions to occur, for example) but that those would happen as a result of other management-based inputs. For this reason, the adoption potential of technologies aimed at monitoring such indicators could be challenging, as they may not be perceived as being a priority. Highlighting the benefits of promoting positive welfare such as the effect on productivity and also on farmers' well-being [see Vigors and Lawrence (2019)], could help enhance the acceptability of those indicators and therefore the technology adoption potential. Indeed, Lima et al. (2018) found that farmers' beliefs (including usefulness and practicality) played an important role in the adoption of Electronic Identification (EID) technology. They suggested that communicating the positive effects of such tools, including on performance, was likely to help enhance technology adoption.
More generally and as raised during the workshop, another potential limitation to PLF technologies adoption may relate to a lack of validation of some technologies which could result in a lack of trust by farmers but also the possibility for welfare-compromising issues to be missed by the technologies. The validation of technologies is usually required to predict how a system would perform under realistic operating conditions, and in the case of PLF, developments must take into account the complexity of living organisms, which are “individually different, time-varying and dynamic” (Norton and Berckmans, 2017). This complexity may explain why a wide range of PLF technologies still require further validation. In their study, Larsen et al. (2021) found that only 23% of publications related to PLF in pigs were properly validated, and a recent review indicated that only 14% of commercially available sensors in dairy cattle were externally validated (Stygar et al., 2021).
Technology adoption does not, however, guarantee that the technologies will be used in an optimal way in relation to welfare. Firstly, covering the many different ways welfare can be affected would require farmers to invest in multiple systems, as most technologies can only monitor a few parameters at a time and systems are often not connected to each other, adding a difficulty to data interpretation (Knight, 2020). Indeed, there is still a lack of integration of PLF technologies making it more challenging to determine effective mechanisms for intervention (Buller et al., 2020). It is also important to stress that most PLF technologies are monitoring systems, meaning that while they can alert farmers to detected issues, the decision to act on the data provided ultimately lies in the farmers' hands. The extent to which welfare can be improved therefore depends on how the technologies and resulting data are used, and especially whether management decisions are restricted to “curing” symptoms once they have appeared or whether those decisions would be adapted to prevent issues arising in the first place. Indeed, participants at the workshop believed that there could be a risk that management would be adapted to fit the use of technologies rather than focusing on welfare improvements, such as adapting light hours and levels to fit cameras or having more barren environments to minimize background noises.
In addition, there could be a risk that a greater recognition of issues among livestock keepers would result in greater acceptance of those issues rather than act as a call to action. In the case of lameness in dairy herds e.g., which is considered one of the most important welfare issue in dairy farming, a study found that a majority of farmers (90%) did not perceive lameness as being a major issue on their farm, even though the average lameness prevalence was high (36%) (Leach et al., 2010). According to Horseman et al. (2014), this may not necessarily be exclusively attributed to farmers not being able to detect lame cows, but could rather be linked to how farmers perceive lameness, as well as their understanding of the benefits of promptly treating lame cows. Indeed, it appears that farmers are more likely to treat severely lame cows more rapidly, leaving simply impaired cows untreated for longer, even though research suggests that it may be more beneficial to treat cows that are less severely lame early (Leach et al., 2010 as cited in Horseman et al., 2014). The extent to which welfare can be improved using PLF thus depends on whether the day-to-day management of animal health and welfare will be adapted with the implementation of those technologies.
It is also noted that most technologies monitoring at the individual level appear to be available for dairy cattle, while technologies monitoring smaller animals often kept in highly populated units such as poultry or fish mostly do so at group level (e.g., using cameras) hence ensuring that the “average” animal receives adequate food, water and environmental conditions (Smaldon, 2020). This is explained by higher numbers of animals with lower financial value per farm, making individual body-mounted devices costly and difficult to implement. On farms where welfare would be assessed automatically at group level, there is a risk that the individual nature of animal welfare might not be sufficiently taken into account if the interpretation of the data is not done carefully. Indeed, assessing welfare parameters at group level does not allow evaluation of whether the measure applies equally to the whole group or to some individuals only, potentially neglecting animals in much lower welfare states (Winckler, 2019). In addition, concerns raised at the workshop related to the design of the technologies which could have an impact on welfare if it is not “wearer-driven” (e.g., such as taking into account genetic variability or rearing environment). It was also questioned whether facilitating management of larger groups could lead to further intensification.
Another important welfare risk, which was also mentioned at the workshop, relate to the potential impact on the human-animal relationship (HAR). Indeed, most of the technologies identified in this study can be used to replace the need for visual but also physical examination, such as monitoring lameness, environmental conditions or feeding behaviors. Depending on how the time saved in performing these tasks is used by farmers, the potential decrease in human presence and human-animal interactions could have an effect on the HAR. Research indeed suggests that the frequency, intensity and intimacy of human-animal interactions influence the level of attachment or detachment of farmers toward their animals (Bock et al., 2007). This loss of interactions and therefore further detached relationship with animals (which may be more and more perceived as production tools) could result in a decrease in empathy and reduced concerns toward animal suffering. In addition, while some potentially stressful tasks could be avoided using PLF, others which have the potential to strengthen the HAR and that allow animals to be habituated to the presence of humans to some extent may also be decreased. This could reduce human-animal interactions to tasks which cannot be replaced by PLF such as mutilations, hence impacting the HAR negatively (Boivin et al., 1994; Hemsworth and Boivin, 2011). Indeed, Tallet et al. (2019) showed that piglets which were tail docked with a cautery iron interacted with unfamiliar humans later than piglets that were not tail docked, and Lürzel et al. (2015) observed that calves avoidance distances were higher after disbudding. In their study, Kling-Eveillard et al. (2020) found that following the implementation of PLF, some farmers perceived the HAR as having improved, while others believed it deteriorated. They also mentioned concerns that having to manage an increased amount of data may reduce the time farmers spend with animals and impact farmers' observational skills. Concerns relating to the de-skilling of farm staff were also raised during the workshop. While the social impacts of PLF on farmer's work are not detailed here, it is ultimately closely linked to animal welfare, since knowledge and husbandry skills and the ability to identify deviations in behaviors and health compromises are key characteristics of animal care (Hemsworth et al., 2009). Farm management supported by the use of PLF should therefore take these potential impacts into consideration, as a negative HAR can be detrimental to animal welfare, but also to farm productivity and job satisfaction (Waiblinger et al., 2006).
While the study aimed at exploring the potential of PLF to help improve animal welfare and the potential risks associated with their use, there are limitations in this study which must be taken into account. As mentioned in the methods section, the identification of PLF technologies was limited to a restricted number of keywords, making it possible to have omitted a variety of technologies. In addition, the different technologies and applications were classified into wider categories with only the latest development stages of all technologies within those categories shown. For this reason, the classification may not reflect the stage of development of all the different types of technologies (although as emphasized in the methods, it was not the goal of the study to determine each existing technology). Finally, it must be re-emphasized that animal welfare is complex, with many variables having a potential impact, whether positive or negative. Using the FDM as a framework helped to capture both positive and negative aspects of welfare, however it remains challenging to predict how the use of technologies will impact on welfare. In addition, the affective states and welfare benefits and risks mentioned in this paper were based on qualitative discussions and evaluation by the authors. Thus, further research aimed at evaluating those positive and negative impacts using quantitative and qualitative methods would be useful to help in technology design, both to maximize potential welfare benefits and minimize the risks. As mentioned by participants of the workshop, further validation of PLF technologies and research on positive welfare indicators as well as a better collaboration between industry, researchers and farmers should also be encouraged, as well as increasing awareness and training of all relevant stakeholders (including training to improve attitudes and behavior of stock people toward animals).
Conclusion
The potential of PLF to help reduce the duration and/or severity of diseases and injuries in livestock farming systems is promising: technologies can detect health issues at an early stage and help ensure optimal environmental conditions. However, the extent to which current PLF systems can help improve welfare appears to be limited to reducing the occurrence of negative affective states. Some technology developments related to the “behavioral interactions” domain of the FDM have the potential to help in promoting positive affective states, however, these generally remain at early development stages. This is potentially explained by a lack of evidence regarding the validity of potential positive welfare indicators and the difficulties in measuring them, as well as doubts regarding the adoption potential of such technologies. In addition, the extent to which welfare could be improved depends on whether the data obtained using PLF would be used to adapt management practices while minimizing negative consequences (such as the impact on the HAR), and whether actions would be taken to address the root cause of the issues rather than solely focusing on treating the symptoms.
Author Contributions
Conceptual work: JS led with support from DR and RB. JS: review work. Manuscript drafting: JS led with support from DR and RB. All authors contributed to the article and approved the submitted version.
Funding
This work was part of a Ph.D. project at the University of Reading. The project was funded by the Elizabeth Creak Charitable Trust. The Animal Welfare Research Network (AWRN) have funded the workshop.
Conflict of Interest
The authors declare that the research was conducted in the absence of any commercial or financial relationships that could be construed as a potential conflict of interest.
Acknowledgments
We would like to thank the Animal Welfare Research Network (AWRN) for funding the workshop, as well as all the participants for their valuable contribution to the discussions. We would also like to thank our colleague Zoe Barker from the University of Reading for helping with the classification and development stages.
Supplementary Material
The Supplementary Material for this article can be found online at: https://www.frontiersin.org/articles/10.3389/fanim.2021.639678/full#supplementary-material
Footnote
1. ^Food and Agriculture Organization. (2019). Meat & Meat Products. Available online at: http://www.fao.org/ag/againfo/themes/en/meat/home.html (accessed December 7, 2020).
References
Adrion, F., Kapun, A., Eckert, F., Holland, E. M., Staiger, M., Götz, S., et al. (2018). Monitoring trough visits of growing-finishing pigs with UHF-RFID. Comput. Electron. Agricult. 144, 144–153. doi: 10.1016/j.compag.2017.11.036
Alhamada, M., Debus, N., Lurette, A., and Bocquier, F. (2016). Validation of automated electronic oestrus detection in sheep as an alternative to visual observation. Small Rum. Res. 134, 97–104. doi: 10.1016/j.smallrumres.2015.12.032
Astill, J., Dara, R. A., Fraser, E. D. G., Roberts, B., and Sharif, S. (2020). Smart poultry management: smart sensors, big data, and the internet of things. Comp. Electr. Agricult. 170:105291. doi: 10.1016/j.compag.2020.105291
Aydin, A. (2016). Precision feeding in laying hens by sound technology. J. Tekird. Agricult. Facult. 13:3.
Banakar, A., Sadeghi, M., and Shushtari, A. (2016). An intelligent device for diagnosing avian diseases: newcastle, infectious bronchitis, avian influenza. Comp. Electr. Agricult. 127, 744–753. doi: 10.1016/j.compag.2016.08.006
Beausoleil, N., and Mellor, D. (2015). Introducing breathlessness as a significant animal welfare issue. N Zeal Vet J. 63, 44–51. doi: 10.1080/00480169.2014.940410
Benjamin, M., and Yik, S. (2019). Precision livestock farming in swinewelfare: a review for swine practitioners. Animals 9:133. doi: 10.3390/ani9040133
Berckmans, D. (2014). Precision livestock farming technologies for welfare management in intensive livestock systems. Rev. Sci. Tech. Off. Int. Epiz. 33, 189–196. doi: 10.20506/rst.33.1.2273
Berckmans, D., Hemeryck, M., and Berckmans, D. (2015). Animal sound … talks ! In: Real-time Sound Analysis for Health Monitoring in Livestock. International Symposium on Animal Environment and Welfare, 1–8.
Bilcik, B., and Keeling, L. J. (1999). Changes in feather condition in relation to feather pecking and aggressive behaviour in laying hens. Br. Poult. Sci. 40, 444–451. doi: 10.1080/00071669987188
Bock, B. B., Van Huik, M. M., Prutzer, M., Kling, F., and Dockes, E. A. (2007). Farmers' relationship with different animals: the importance of getting close to the animals. Case studies of French, Swedish and Dutch cattle, pig and poultry farmers. Int. J. Sociol. Food Agricult. 15. doi: 10.48416/ijsaf.v15i3.290
Boissy, A., Manteuffel, G., Jensen, M. B., Moe, R. O., Spruijt, B., Keeling, L. J., et al. (2007). Assessment of positive emotions in animals to improve their welfare. Physiol. Behav. 92, 375–397. doi: 10.1016/j.physbeh.2007.02.003
Boivin, X., Le Neindre, P., Garel, J. P., and Chupin, J. M. (1994). Influence of breed and rearing management on cattle reactions during human handling. Appl. Anim. Behav. Sci. 39, 115–122. doi: 10.1016/0168-1591(94)90131-7
Bos, J. M., Bovenkerk, B., Feindt, P. H., and van Dam, Y. K. (2018). The quantified animal: precision livestock farming and the ethical implications of objectification. Food Ethics. Food Ethics. 2, 77–92. doi: 10.1007/s41055-018-00029-x
Boyland, N. K., James, R., Mlynski, D. T., Madden, J. R., and Croft, D. P. (2013). Spatial proximity loggers for recording animal social networks: consequences of inter-logger variation in performance. Behav. Ecol. Sociobiol. 67, 1877–1890. doi: 10.1007/s00265-013-1622-6
Brown, D. J., Swan, A. A., and Mortimer, M. L. (2011). “Pedigree matchmaker: can it tell us more than just pedigree?” in Proceedings of the 19th Conference of the Association for the Advancement of Animal Breeding and Genetics (Perth, WA), 231–234.
Buller, H., Blokhuis, H., Lokhorst, K., Silberberg, M., and Veissier, I. (2020). Animal welfare management in a digital world. Animals 10, 1–12. doi: 10.3390/ani10101779
Campos, D. P., Abatti, P. J., Bertotti, F. L., De Paula Vieira, A., Hill, J. A. G., and da Silveira, A. L. F. (2019). Short-term fibre intake estimation in goats using surface electromyography of the masseter muscle. Biosyst. Eng. 183, 209–220. doi: 10.1016/j.biosystemseng.2019.04.021
Carpentier, L., Berckmans, D., Youssef, A., Berckmans, D., van Waterschoot, T., Johnston, D., et al. (2018). Automatic cough detection for bovine respiratory disease in a calf house. Biosyst. Eng. 173, 45–56. doi: 10.1016/j.biosystemseng.2018.06.018
Carpentier, L., Vranken, E., Berckmans, D., Paeshuyse, J., and Norton, T. (2019). Development of sound-based poultry health monitoring tool for automated sneeze detection. Comp. Electr. Agricult. 162, 573–581. doi: 10.1016/j.compag.2019.05.013
Chagneau, A. M., Laviron, F., Lamy, S., Bouvarel, I., Picard, M., Lessire, M., et al. (2006). Short-term number of pecks and feed intake levels: a link with the physical characteristics of feed in four-week-old Turkeys. Poultry Sci. 85, 923–931. doi: 10.1093/PS/85.5.923
Chelotti, J. O., Vanrell, S. R., Milone, D. H., Utsumi, S. A., Galli, J. R., Rufiner, H. L., et al. (2016). A real-time algorithm for acoustic monitoring of ingestive behavior of grazing cattle. Comp. Electr. Agricult. 127, 64–75. doi: 10.1016/j.compag.2016.05.015
Chen, C., Zhu, W., Liu, D., Steibel, J., Siegford, J., Wurtz, K., et al. (2019). Detection of aggressive behaviours in pigs using a RealSence depth sensor. Comp. Electr. Agricult. 166:105003. doi: 10.1016/j.compag.2019.105003
Chopra, K., Hodges, H. R., Barker, Z. E., and Diosdado, J. A. V. (2020). Proximity Interactions in a Permanently Housed Dairy Herd : Network Structure, Consistency, and Individual Differences. Front. Vet. Sci. 7:583715. doi: 10.3389/fvets.2020.583715
Chung, Y., Choi, D., Choi, H., Park, D., Chang, H.-H., and Kim, S. (2015). Automated detection of cattle mounting using side-view camera. KSII Trans. Internet Inform. Syst. 9:24. doi: 10.3837/tiis.2015.08.024
Colles, F. M., Cain, R. J., Nickson, T., Smith, A. L., Roberts, S. J., Maiden, M. C. J., et al. (2016). Monitoring chicken flock behavior provides early warning of infection by human pathogen Campylobacter. Proc. Royal Soc. B Biol. Sci. 283, 1–6. doi: 10.1098/rspb.2015.2323
Corbet, N. J., Patison, K. P., Menzies, D. J., and Swain, D. L. (2018). Using temporal associations to determine postpartum oestrus in tropical beef cows. Anim. Product. Sci. 58, 1465–1469. doi: 10.1071/AN17781
Cornou, C. (2009). Automation systems for farm animals: potential impacts on the human-animal relationship and on animal welfare. Anthrozoos 22, 213–220. doi: 10.2752/175303709X457568
Cransberg, P. H., Hemsworth, P. H., and Goleman, G. J. (2000). Human factors affecting the behaviour and productivity of commercial broiler chickens. Br. Poultry Sci. 41, 272–279. doi: 10.1080/713654939
da Fonseca, F. N., Abe, J. M., de Alencar Nääs, I., da Silva Cordeiro, A. F., do Amaral, F. V., and Ungaro, H. C. (2020). Automatic prediction of stress in piglets (Sus Scrofa) using infrared skin temperature. Comp. Electr. Agricult. 168:105148. doi: 10.1016/j.compag.2019.105148
Dawkins, M. S., Roberts, S. J., Cain, R. J., Nickson, T., and Donnelly, C. A. (2017). Early warning of footpad dermatitis and hockburn in broiler chicken flocks using optical flow, bodyweight and water consumption. Vet. Record. 180:499. doi: 10.1136/vr.104066
De Alencar Nääs, I., Correia De Lima, I., Paz, A., Marta,;, Baracho, S., Gomes De Menezes, A., et al. (2010). Assessing locomotion deficiency in broiler chicken. Sci. Agric. 67, 129–135. doi: 10.1590/S0103-90162010000200001
D'eath, R. B., Jack, M., Futro, A., Talbot, D., Zhu, Q., Barclay, D., et al. (2018). Automatic early warning of tail biting in pigs: 3D cameras can detect lowered tail posture before an outbreak. PLoS ONE 13:194524. doi: 10.1371/journal.pone.0194524
DEFRA (2021). The Path to Sustainable Farming : An Agricultural Transition Plan 2021 to 2024. London: DEFRA.
Di Giminiani, P., Edwards, S. A., Malcolm, E. M., Leach, M. C., Herskin, M. S., and Sandercock, D. A. (2017). Characterization of short- and long-term mechanical sensitisation following surgical tail amputation in pigs. Scient. Rep. 7, 1–9. doi: 10.1038/s41598-017-05404-y
Dos, D., Costa, S., Turco, S. H. N., Ramos, R. P., Silva, F. M. F. M., and Freire, M. S. (2018). Electronic monitoring system for measuring heart rate and skin temperature in small ruminants. Engenh. Agrícola. 38, 166–172. doi: 10.1590/1809-4430-Eng.Agric.v38n2p
Eastwood, C. R., and Renwick, A. (2020). Innovation uncertainty impacts the adoption of smarter farming approaches. Front. Sust. Food Syst. 4:24. doi: 10.3389/fsufs.2020.00024
Edwards, D. S., and Johnston, A. M. (1999). Welfare implications of sheep ear tags. Vet. Rec. 144, 603–606.
European Commission (2016). Special Eurobarometer 442: Attitudes of Europeans Towards Animal Welfare. Animal Welfare. doi: 10.2875/884639
Eurostat (2020). Agriculture, Forestry, and Fishery Statistics: 2020 edition. Luxembourg: Publications Office of the European Union.
Fontana, I., Tullo, E., Butterworth, A., and Guarino, M. (2015). An innovative approach to predict the growth in intensive poultry farming. Comp. Electr. Agricult. 119, 178–183. doi: 10.1016/j.compag.2015.10.001
Fontana, I., Tullo, E., Carpentier, L., Berckmans, D., Butterworth, A., Vranken, E., et al. (2017). Sound analysis to model weight of broiler chickens. Poultry Sci. 96, 1–6. doi: 10.3382/ps/pex215
Foris, B., Thompson, A., von Keyserlingk, M., Melzer, N., and Weary, D. (2019). Automatic detection of feeding- and drinking-related agonistic behavior and dominance in dairy cows. J. Diary Sci. 102, 9176–86. doi: 10.3168/jds.2019-16697
Fuchs, B., Sørheim, K. M., Chincarini, M., Brunberg, E., Stubsjøen, S. M., Bratbergsengen, K., et al. (2019). Heart rate sensor validation and seasonal and diurnal variation of body temperature and heart rate in domestic sheep. Vet. Anim. Sci. 8:100075. doi: 10.1016/j.vas.2019.100075
Gilbert, M., Conchedda, G., Van Boeckel, T. P., Cinardi, G., Linard, C., Nicolas, G., et al. (2015). Income disparities and the global distribution of intensively farmed chicken and pigs. PLoS ONE. 10:e013338. doi: 10.1371/journal.pone.0133381
Grilli, G., Borgonovo, F., Tullo, E., Fontana, I., Guarino, M., and Ferrante, V. (2018). A pilot study to detect coccidiosis in poultry farms at early stage from air analysis. Biosyst. Eng. 173, 64–70. doi: 10.1016/j.biosystemseng.2018.02.004
Gross, W. B., and Siegel, P. B. (1979). Adaptation of chickens to their handler, and experimental results. Avian Dis. 23, 708–714. doi: 10.2307/1589747
Guo, Y., Zhang, Z., He, D., Niu, J., and Tan, Y. (2019). Detection of cow mounting behavior using region geometry and optical flow characteristics. Comp. Electr. Agricult. 163:104828. doi: 10.1016/j.compag.2019.05.037
Guzhva, O., Ard,ö, H., Herlin, A., Nilsson, M., Åström, K., and Bergsten, C. (2016). Feasibility study for the implementation of an automatic system for the detection of social interactions in the waiting area of automatic milking stations by using a video surveillance system. Comp. Electr. Agricult. 127, 506–509. doi: 10.1016/j.compag.2016.07.010
Halachmi, I., Guarino, M., Bewley, J., and Pastell, M. (2019). Smart animal agriculture: application of real-time sensors to improve animal well-being and production. Ann. Rev. Anim. Biosci. 7, 403–425. doi: 10.1146/annurev-animal-020518-114851
Held, S. D. E., and Špinka, M. (2011). Animal play and animal welfare. Anim. Behav. 81, 891–899. doi: 10.1016/j.anbehav.2011.01.007
Hemsworth, P. H., and Barnett, J. L. (1991). The effects of aversively handling pigs, either individually or in groups, on their behaviour, growth and corticosteroids. Appl. Anim. Behav. Sci. 30, 61–72. doi: 10.1016/0168-1591(91)90085-C
Hemsworth, P. H., Barnett, J. L., and Coleman, G. J. (2009). The integration of human-animal relations into animal welfare monitoring schemes. Anim. Welfare. 18, 335–345.
Horseman, S. V., Roe, E. J., Huxley, J. N., Bell, N. J., Mason, C. S., and Whay, H. R. (2014). The use of in-depth interviews to understand the process of treating lame dairy cows from the farmers' perspective. Anim. Welfare 23, 157–165. doi: 10.7120/09627286.23.2.157
Huzzey, J. M., Weary, D. M., Tiau, B. Y. F., and Von Keyserlingk, M. A. G. (2014). Short communication: automatic detection of social competition using an electronic feeding system. J. Dairy Sci. 97, 2953–2958. doi: 10.3168/jds.2013-7434
Hyun, H. Y., Yeong, H. B., and Wongi, M. (2007). “Implantable wireless sensor network to monitor the deep body temperature of broilers,” in Proceedings - SERA 2007: Fifth ACIS International Conference on Software Engineering Research, Management, and Applications (Busan), 513–517. doi: 10.1109/SERA.2007.91
Israeli, D. (1996). Monitoring the behavior of hypoxia-stressed Carassius auratus using computer vision. Aquacult. Eng. 15, 423–440. doi: 10.1016/S0144-8609(96)01009-6
Jensen, M. B., and Kyhn, R. (2000). Play behaviour in group-housed dairy calves, the effect of space allowance. Appl. Anim. Behav. Sci. 67, 35–46. doi: 10.1016/S0168-1591(99)00113-6
Kaler, J., Mitsch, J., Vázquez-Diosdado, J. A., Bollard, N., Dottorini, T., and Ellis, K. A. (2020). Automated detection of lameness in sheep using machine learning approaches: novel insights into behavioural differences among lame and non-lame sheep. Roy. Soc. Open Sci. 7:190824. doi: 10.1098/rsos.190824
Kashiha, M., Bahr, C., Haredasht, S. A., Ott, S., Moons, C. P. H., Niewold, T. A., et al. (2013a). The automatic monitoring of pigs water use by cameras. Comp. Electr. Agricult 90, 164–169. doi: 10.1016/j.compag.2012.09.015
Kashiha, M., Bahr, C., Ott, S., Moons, C. P. H., Niewold, T. A., Ödberg, F. O., et al. (2014). Automatic weight estimation of individual pigs using image analysis. Comp. Electr. Agricult. 107, 38–44. doi: 10.1016/j.compag.2014.06.003
Kashiha, M., Pluk, A., Bahr, C., Vranken, E., and Berckmans, D. (2013b). Development of an early warning system for a broiler house using computer vision. Biosyst. Eng. 116, 36–45. doi: 10.1016/j.biosystemseng.2013.06.004
Kling-Eveillard, F., Allain, C., Boivin, X., Courboulay, V., Créach, P., Philibert, A., et al. (2020). Farmers' representations of the effects of precision livestock farming on human-animal relationships. Livestock Sci. 238:104057. doi: 10.1016/j.livsci.2020.104057
Knight, C. H. (2020). Review: Sensor techniques in ruminants: more than fitness trackers. Animal 14, s187–s195. doi: 10.1017/S1751731119003276.
Larsen, M. L. V., Wang, M., and Norton, T. (2021). Information technologies for welfare monitoring in pigs and their relation to welfare quality ®. Sustainability. 13:692. doi: 10.3390/su13020692
Leach, K. A., Whay, H. R., Maggs, C. M., Barker, Z. E., Paul, E. S., Bell, A. K., et al. (2010). Working towards a reduction in cattle lameness: 1. Understanding barriers to lameness control on dairy farms. Res. Vet. Sci. 89, 311–317. doi: 10.1016/j.rvsc.2010.02.014
Lee, H.-J., Roberts, S. J., Drake, K. A., and Dawkins, M. S. (2011). Prediction of feather damage in laying hens using optical flows and Markov models. J. Roy. Soc. Interface. 8, 489–499. doi: 10.1098/rsif.2010.0268
Lee, J., Jin, L., Park, D., and Chung, Y. (2016). Automatic recognition of aggressive behavior in pigs using a kinect depth sensor. Sensors 16:631. doi: 10.3390/s16050631
Lee, J., Noh, B., Jang, S., Park, D., Chung, Y., and Chang, H. H. (2015). Stress detection and classification of laying hens by sound analysis. Asian-Austral. J. Anim. Sci. 28, 592–598. doi: 10.5713/ajas.14.0654
Lensink, J., Boissy, A., and Veissier, I. (2000). The relationship between farmers' attitude and behaviour towards calves, and productivity of veal units. Anim. Res. 49, 313–327. doi: 10.1051/animres:2000122
Li, L., Zhao, Y., Oliveira, J., Verhoijsen, W., Liu, K., and Xin, H. (2017). A UHF RFID system for studying individual feeding and nesting behaviors of group-housed laying hens. Trans. ASABE. 60, 1337–1347. doi: 10.13031/trans.12202
Li, N., Ren, Z., Li, D., and Zeng, L. (2019). Review: automated techniques for monitoring the behaviour and welfare of broilers and laying hens: towards the goal of precision livestock farming. Animal 14, 617–625. doi: 10.1017/S1751731119002155
Lian, J., Zheng, Y., Jia, W., Zhao, Y., Fan, M., Wang, D., et al. (2019). Automated recognition and discrimination of human–animal interactions using Fisher vector and hidden Markov model. Signal Image Video Process. 13, 993–1000. doi: 10.1007/s11760-019-01437-0
Lima, E., Hopkins, T., Gurney, E., Shortall, O., Lovatt, F., Davies, P., et al. (2018). Drivers for precision livestock technology adoption: a study of factors associated with adoption of electronic identification technology by commercial sheep farmers in England and Wales. PLoS ONE. 13:e0190489. doi: 10.1371/journal.pone.0190489
Liu, L. S., Ni, J. Q., Zhao, R. Q., Shen, M. X., He, C. L., and Lu, M. Z. (2018). Design and test of a low-power acceleration sensor with Bluetooth Low Energy on ear tags for sow behaviour monitoring. Biosystems Engineering. Academic Press. 176, 162–171. doi: 10.1016/j.biosystemseng.2018.10.011
Lürzel, S., Münsch, C., Windschnurer, I., Futschik, A., Palme, R., and Waiblinger, S. (2015). The influence of gentle interactions on avoidance distance towards humans, weight gain and physiological parameters in group-housed dairy calves. Appl. Anim. Behav. Sci. 172, 9–16. doi: 10.1016/j.applanim.2015.09.004
Luu, J., Johnsen, J. F., Passill,é, A. M., and de Rushen, J. (2013). Which measures of acceleration best estimate the duration of locomotor play by dairy calves? Appl. Anim. Behav. Sci. 148, 21–27. doi: 10.1016/j.applanim.2013.07.004
Main, D., and Mullan, S. (2017). A new era of UK leadership in farm animal welfare. Vet. Rec. 181, 49–50. doi: 10.1136/vr.j3273
Mainau, E., Dalmau, A., Ruiz-de-la-Torre, J. L., and Manteca, X. (2009). Validation of an automatic system to detect position changes in puerperal sows. Applied Animal Behaviour Science. 121, 96–102. doi: 10.1016/j.applanim.2009.09.005
Manteuffel, C., Hartung, E., Schmidt, M., Hoffmann, G., and Schön, P. C. (2015). Towards qualitative and quantitative prediction and detection of parturition onset in sows using light barriers. Comp. Electr. Agricult. 116, 201–210. doi: 10.1016/j.compag.2015.06.017
Martos-Sitcha, J. A., Sosa, J., Ramos-Valido, D., Bravo, F. J., Carmona-Duarte, C., Gomes, H. L., et al. (2019). Ultra-low power sensor devices for monitoring physical activity and respiratory frequency in farmed fish. Front. Physiol. 10, 1–14. doi: 10.3389/fphys.2019.00667
Maselyne, J., Adriaens, I., Huybrechts, T., De Ketelaere, B., Millet, S., Vangeyte, J., et al. (2016a). Measuring the drinking behaviour of individual pigs housed in group using radio frequency identification (RFID). Animal. 10, 1557–1566. doi: 10.1017/S1751731115000774
Maselyne, J., Saeys, W., Briene, P., Mertens, K., Vangeyte, J., De Ketelaere, B., et al. (2016b). Methods to construct feeding visits from RFID registrations of growing-finishing pigs at the feed trough. Comp. Electr. Agricult. 128, 9–19. doi: 10.1016/j.compag.2016.08.010
Mattiello, S., Battini, M., De Rosa, G., Napolitano, F., and Dwyer, C. (2019). How can we assess positive welfare in ruminants? Animals 9, 1–27. doi: 10.3390/ani9100758
McEwen, S. A., and Collignon, P. J. (2018). Antimicrobial resistance: a one health perspective. Microbiol. Spectr. 6:2017. doi: 10.1128/microbiolspec.arba-0009-2017
McLennan, K., and Mahmoud, M. (2019). Development of an automated pain facial expression detection system for sheep (Ovis aries). Animals 9, 1–7. doi: 10.3390/ani9040196
Mellor, D. J. (2015a). Enhancing animal welfare by creating opportunities for positive affective engagement. N. Zeal. Vet. J. 63, 3–8. doi: 10.1080/00480169.2014.926799
Mellor, D. J. (2015b). Positive animal welfare states and reference standards for welfare assessment. N. Zeal. Vet. J. 63, 17–23. doi: 10.1080/00480169.2014.926802
Mellor, D. J. (2017). Operational details of the five domains model and its key applications to the assessment and management of animal welfare. Animals 7:60. doi: 10.3390/ani7080060
Mellor, D. J., and Beausoleil, N. J. (2015). Extending the “Five Domains” model for animal welfare assessment to incorporate positive welfare states. Anim. Welfare. 24, 241–253. doi: 10.7120/09627286.24.3.241
Mellor, D. J., Beausoleil, N. J., Littlewood, K. E., Mclean, A. N., Mcgreevy, P. D., Jones, B., et al. (2020). The 2020 five domains model: including human-animal interactions in assessments of animal welfare. Animals 10:1870. doi: 10.3390/ani10101870
Morris, J. E., Cronin, G. M., and Bush, R. D. (2012). Improving sheep production and welfare in extensive systems through precision sheep management. Anim. Prod. Sci. 52, 665–670. doi: 10.1071/AN11097
Mortensen, A. K., Lisouski, P., and Ahrendt, P. (2016). Weight prediction of broiler chickens using 3D computer vision. Comp. Electr. Agricult. 123, 319–326. doi: 10.1016/j.compag.2016.03.011
Mota-Rojas, D., Broom, D. M., Orihuela, A., Velarde, A., Napolitano, F., and Alonso-Spilsbury, M. (2020). Effects of human-animal relationship on animal productivity and welfare. J. Anim. Behav. 8, 196–205. doi: 10.31893/JABB.20026
Nakarmi, A. D., Tang, L., and Xin, H. (2014). Automated tracking and behavior quantification of laying hens using 3D computer vision and radio frequency identification technologies. Transact. ASABE. 57, 1455–1472. doi: 10.13031/trans.57.10505
Napolitano, F., Knierim, U., Grass, F., and De Rosa, G. (2016). Positive indicators of cattle welfare and their applicability to on-farm protocols. Ital. J. Anim. Sci. 8, 355–365. doi: 10.4081/ijas.2009.s1.355
Nasirahmadi, A., Hensel, O., Edwards, S., and Sturm, B. (2016). Automatic detection of mounting behaviours among pigs using image analysis. Comp. Electr. Agricult. 124, 295–302. doi: 10.1016/j.compag.2016.04.022
Navon, S., Mizrach, A., Hetzroni, A., and Ungar, E. D. (2013). Automatic recognition of jaw movements in free-ranging cattle, goats and sheep, using acoustic monitoring. Biosyst. Eng. 114, 474–483. doi: 10.1016/j.biosystemseng.2012.08.005
Nicol, C. (2011). “Behaviour as an indicator of animal welfare” in 2011, Management and Welfare of Farm Animals: The UFAW Farm Handbook, ed J. Webster (Hoboken, NJ: John Wiley & Sons, Incorporated).
Nogami, H., Okada, H., Miyamoto, T., Maeda, R., and Itoh, T. (2013). Wearable and compact wireless sensor nodes for measuring the temperature of the base of a calf's tail. Sensors Mater. 25, 577–582. doi: 10.18494/sam.2013.907
Norton, T., and Berckmans, D. (2017). The Dairy Industry and Precision Livestock Farming Developing precision livestock farming tools for precision dairy farming. Anim. Front. 7:18. doi: 10.2527/af.2017.0104
Norton, T., Chen, C., Larsen, M. L. V., and Berckmans, D. (2019). Review: precision livestock farming: building “digital representations” to bring the animals closer to the farmer. Animal 3, 3009–3017. doi: 10.1017/S175173111900199X
Oczak, M., Maschat, K., Berckmans, D., Vranken, E., and Baumgartner, J. (2015). Classification of nest-building behaviour in non-crated farrowing sows on the basis of accelerometer data. Biosyst. Eng. 140, 48–58. doi: 10.1016/j.biosystemseng.2015.09.007
Okada, H., Suzuki, K., Kenji, T., and Itoh, T. (2014). Applicability of wireless activity sensor network to avian influenza monitoring system in poultry farms. J. Sens. Technol. 4, 18–23. doi: 10.4236/jst.2014.41003
Okinda, C., Lu, M., Nyalala, I., Li, J., and Shen, M. (2018). Asphyxia occurrence detection in sows during the farrowing phase by inter-birth interval evaluation. Comp. Electr. Agricult. 152, 221–232. doi: 10.1016/j.compag.2018.07.007
Pastell, M., Hietaoja, J., Yun, J., Tiusanen, J., and Valros, A. (2016). Predicting farrowing of sows housed in crates and pens using accelerometers and CUSUM charts. Comp. Electr. Agricult. 127, 197–203. doi: 10.1016/j.compag.2016.06.009
Pluym, L. M., Maes, D., Vangeyte, J., Mertens, K., Baert, J., Van Weyenberg, S., et al. (2013). Development of a system for automatic measurements of force and visual stance variables for objective lameness detection in sows: SowSIS. Biosystems Eng. 116, 64–74. doi: 10.1016/j.biosystemseng.2013.06.009
Probst, J. K., Spengler Neff, A., Leiber, F., Kreuzer, M., and Hillmann, E. (2012). Gentle touching in early life reduces avoidance distance and slaughter stress in beef cattle. Appl. Anim. Behav. Sci. 139, 42–49. doi: 10.1016/j.applanim.2012.03.002
Quwaider, M., Daigle, C. L., Biswas, S. K., Siegford, J. M., and Swanson, J. C. (2010). Development of a wireless body-mounted sensor to monitor location and activity of laying hens in a non-cage housing system. Trans. ASABE. 53, 1705–1713. doi: 10.13031/2013.34890
Rojo-Gimeno, C., van der Voort, M., Niemi, J. K., Lauwers, L., Kristensen, A. R., and Wauters, E. (2019). Assessment of the value of information of precision livestock farming: a conceptual framework. NJAS Wageningen J. Life Sci. 90:100311. doi: 10.1016/j.njas.2019.100311
Roland, L., Lidauer, L., Sattlecker, G., Kickinger, F., Auer, W., Sturm, V., et al. (2018). Monitoring drinking behavior in bucket-fed dairy calves using an ear-attached tri-axial accelerometer: a pilot study. Comp. Electr. Agricult. 145, 298–301. doi: 10.1016/j.compag.2018.01.008
Rose, D. C., and Chilvers, J. (2018). Agriculture 4.0: Broadening Responsible Innovation in an Era of Smart Farming. Front. Sust. Food Syst. 2:87. doi: 10.3389/fsufs.2018.00087
Röttgen, V., Schön, P. C., Becker, F., Tuchscherer, A., Wrenzycki, C., Düpjan, S., et al. (2020). Automatic recording of individual oestrus vocalisation in group-housed dairy cattle: development of a cattle call monitor. Animal 14, 198–205. doi: 10.1017/S1751731119001733
Rushen, J., Taylor, A. A., and De Passillé, A. M. (1999). Domestic animals' fear of humans and its effect on their welfare. Appl. Anim. Behav. Sci. 65, 285–303. doi: 10.1016/S0168-1591(99)00089-1
Rutter, S. M. (2014). Smart technologies for detecting animal welfare status and delivering health remedies for rangeland systems. OIE Revue Scientifique et Technique. 33, 181–187. doi: 10.20506/rst.33.1.2274
Schön, P. C., Puppe, B., and Manteuffel, G. (2004). Automated recording of stress vocalisations as a tool to document impaired welfare in pigs. Anim. Welfare 13, 105–110.
Segner, H., Sundh, H., Buchmann, K., Douxfils, J., Sundell, K. S., Mathieu, C., et al. (2012). Health of farmed fish: its relation to fish welfare and its utility as welfare indicator. Fish Physiol. Biochem. 38, 85–105. doi: 10.1007/s10695-011-9517-9
Shao, J., Xin, H., and Harmon, J. D. (1997). Neural network analysis of postural behavior of young swine to determine the IR thermal comfort state. Trans. Am. Soc. Agricult. Eng. 40, 755–760. doi: 10.13031/2013.21306
Shrestha, A., Loukas, C., Le Kernec, J., Fioranelli, F., Busin, V., Jonsson, N., et al. (2018). Animal lameness detection with radar sensing. IEEE Geosci. Remote Sensing Lett. 15, 1189–1193. doi: 10.1109/LGRS.2018.2832650
Smaldon, J. (2020). Managing Poultry Welfare in a Transitioning World of Technology. Taunton, MA: Nuffield Farming Scholarships Trust. doi: 10.2307/j.ctvr7f6m7.10
Sneddon, L. U. (2019). Evolution of nociception and pain: evidence from fish models. Philosoph. Trans. Roy. Soc. B Biol. Sci. 374:1785. doi: 10.1098/rstb.2019.0290
Song, X., van der Tol, P., Groot Koerkamp, P., and Bokkers, E. (2019). Hot topic: automated assessment of reticulo-ruminal motility in dairy cows using 3-dimensional vision. J. Dairy Sci. 102, 9076–9081. doi: 10.3168/jds.2019-16550
Stavrakakis, S., Wei, L., Guy, J. H., Morgan, G., Ushaw, G., Johnson, G. R., et al. (2015). Validity of the Microsoft Kinect sensor for assessment of normal walking patterns in pigs. Comp. Electr. Agricult. 117, 1–7. doi: 10.1016/j.compag.2015.07.003
Steensels, M., Antler, A., Bahr, C., Berckmans, D., Maltz, E., and Halachmi, I. (2016). A decision-tree model to detect post-calving diseases based on rumination, activity, milk yield, BW and voluntary visits to the milking robot'. Animal 10, 1493–1500. doi: 10.1017/S1751731116000744
Stewart, M., Wilson, M., Schaefer, A., Huddart, F., and Sutherland, M. (2017). The use of infrared thermography and accelerometers for remote monitoring of dairy cow health and welfare. J. Dairy Sci. 100, 3893–3901. doi: 10.3168/jds.2016-12055
Strutzke, S., Fiske, D., Hoffmann, G., Ammon, C., Heuwieser, W., and Amon, T. (2019). Technical note: development of a noninvasive respiration rate sensor for cattle. J. Dairy Sci. 102, 690–695. doi: 10.3168/jds.2018-14999
Stygar, A. H., Gómez, Y., Berteselli, G. V., Dalla Costa, E., Canali, E., Niemi, J. K., et al. (2021). A systematic review on commercially available and validated sensor technologies for welfare assessment of dairy cattle. Front. Vet. Sci. 8:634338. doi: 10.3389/fvets.2021.634338
Swain, D. L., and Bishop-Hurley, G. J. (2007). Using contact logging devices to explore animal affiliations: quantifying cow-calf interactions. Appl. Anim. Behav. Sci. 102, 1–11. doi: 10.1016/j.applanim.2006.03.008
Tallet, C., Rakotomahandry, M., Herlemont, S., and Prunier, A. (2019). Evidence of pain, stress, and fear of humans during tail docking and the next four weeks in piglets (Sus scrofa domesticus). Front. Vet. Sci. 6:462. doi: 10.3389/fvets.2019.00462
Taneja, M., Byabazaire, J., Jalodia, N., Davy, A., Olariu, C., and Malone, P. (2020). Machine learning based fog computing assisted data-driven approach for early lameness detection in dairy cattle. Comp. Electr. Agricult. 171:105286. doi: 10.1016/j.compag.2020.105286
Thompson, R., Matheson, S. M., Plötz, T., Edwards, S. A., and Kyriazakis, I. (2016). Porcine lie detectors: automatic quantification of posture state and transitions in sows using inertial sensors. Comp. Electr. Agricult. 127, 521–530. doi: 10.1016/j.compag.2016.07.017
Trevisi, E., Zecconi, A., Cogrossi, S., Razzuoli, E., Grossi, P., and Amadori, M. (2014). Strategies for reduced antibiotic usage in dairy cattle farms. Res. Vet. Sci. 96, 229–233. doi: 10.1016/j.rvsc.2014.01.001
Tu, X., Du, S., Tang, L., Xin, H., and Wood, B. (2011). A real-time automated system for monitoring individual feed intake and body weight of group housed turkeys. Comp. Electr. Agricult. 75, 313–320. doi: 10.1016/j.compag.2010.12.007
Van Der Sluis, M., De Klerk, B., Ellen, E. D., De Haas, Y., Hijink, T., and Rodenburg, T. B. (2019). Validation of an ultra-wideband tracking system for recording individual levels of activity in broilers. Animals 9:580. doi: 10.3390/ani9080580
Van Hertem, T., Norton, T., Berckmans, D., and Vranken, E. (2018). Predicting broiler gait scores from activity monitoring and flock data. Biosystems Eng. 173, 93–102. doi: 10.1016/j.biosystemseng.2018.07.002
Vandermeulen, J., Bahr, C., Johnston, D., Earley, B., Tullo, E., Fontana, I., et al. (2016). Early recognition of bovine respiratory disease in calves using automated continuous monitoring of cough sounds. Comp. Electr. Agricult. 129, 15–26. doi: 10.1016/j.compag.2016.07.014
Vayssade, J. A., Arquet, R., and Bonneau, M. (2019). Automatic activity tracking of goats using drone camera. Comp. Electr. Agricult. 162, 767–772. doi: 10.1016/j.compag.2019.05.021
Viazzi, S., Ismayilova, G., Oczak, M., Sonoda, L. T., Fels, M., Guarino, M., et al. (2014). Image feature extraction for classification of aggressive interactions among pigs. Comp. Electr. Agricult. 104, 57–62. doi: 10.1016/j.compag.2014.03.010
Vigors, B., and Lawrence, A. (2019). What are the positives? Exploring positive welfare indicators in a qualitative interview study with livestock farmers. Animals 9:694. doi: 10.3390/ani9090694
Volkmann, N., Kulig, B., and Kemper, N. (2019). Using the footfall sound of dairy cows for detecting claw lesions. Animals 9:78. doi: 10.3390/ani9030078
Waiblinger, S., Boivin, X., Pedersen, V., Tosi, M. V., Janczak, A. M., Visser, E. K., et al. (2006). Assessing the human-animal relationship in farmed species: a critical review. Appl. Anim. Behav. Sci. 101, 185–242. doi: 10.1016/j.applanim.2006.02.001
Waiblinger, S., Menke, C., and Coleman, G. (2002). The relationship between attitudes, personal characteristics and behaviour of stockpeople and subsequent behaviour and production of dairy cows. Appl. Anim. Behav. Sci. 79, 195–219. doi: 10.1016/S0168-1591(02)00155-7
Wang, K., Liu, K., Xin, H., Chai, L., and Wang, Y. (2019). An RFID-based automated individual perching monitoring system for group-housed poultry. Agricult. Biosyst. Eng. 62, 695–704. doi: 10.13031/trans.13105
Watz, S., Petzl, W., Zerbe, H., Rieger, A., Glas, A., Schröter, W., et al. (2019). Technical note: automatic evaluation of infrared thermal images by computerized active shape modeling of bovine udders challenged with Escherichia coli. J. Dairy Sci. 102:5. doi: 10.3168/jds.2018-15761
Werkheiser, I. (2018). Precision Livestock Farming and Farmers' Duties to Livestock. J. Agricult. Environ. Ethics 31, 181–195. doi: 10.1007/s10806-018-9720-0
Werkheiser, I. (2020). Technology and responsibility: a discussion of underexamined risks and concerns in precision livestock farming. Anim. Front. 10, 51–57. doi: 10.1093/af/vfz056
Williams, L. R., Moore, S. T., Bishop-Hurley, G. J., and Swain, D. L. (2020). A sensor-based solution to monitor grazing cattle drinking behaviour and water intake. Comp. Electr. Agricult. 168:105141. doi: 10.1016/j.compag.2019.105141
Winckler, C. (2019). Assessing animal welfare at the farm level: do we care sufficiently about the individual?. Anim. Welfare. 28, 77–82. doi: 10.7120/09627286.28.1.077
Wischner, D., Kemper, N., and Krieter, J. (2009). Nest-building behaviour in sows and consequences for pig husbandry. Livestock Sci. 124, 1–8. doi: 10.1016/j.livsci.2009.01.015
Xiao, L., Ding, K., Gao, Y., and Rao, X. (2019). Behavior-induced health condition monitoring of caged chickens using binocular vision. Comp. Electr. Agricult. 156, 254–262. doi: 10.1016/j.compag.2018.11.022
Xin, H. (1999). Environment and behavior 1: recent advances in assessment and management of heat stress in domestic animals assessing swine thermal comfort by image. J. Anim. Sci. 77, 1–9. doi: 10.2527/1999.77suppl_21x
Xiong, X., Lu, M., Yang, W., Duan, G., Yuan, Q., Shen, M., et al. (2019). An automatic head surface temperature extraction method for top-view thermal image with individual broiler. Sensors 19:5286. doi: 10.3390/s19235286
Yang, A., Huang, H., Yang, X., Li, S., Chen, C., Gan, H., et al. (2019). Automated video analysis of sow nursing behavior based on fully convolutional network and oriented optical flow. Comp. Electr. Agricult. 167:105048. doi: 10.1016/j.compag.2019.105048
Yang, A., Huang, H., Zheng, B., Li, S., Gan, H., Chen, C., et al. (2020). An automatic recognition framework for sow daily behaviours based on motion and image analyses. Biosyst. Eng. 192, 56–71. doi: 10.1016/j.biosystemseng.2020.01.016
Yazdanbakhsh, O., Zhou, Y., and Dick, S. (2017). An intelligent system for livestock disease surveillance. Inform. Sci. 378, 26–47. doi: 10.1016/j.ins.2016.10.026
Yeates, J. W., and Main, D. C. J. (2008). Assessment of positive welfare: A review. Vet. J. 293–300. doi: 10.1016/j.tvjl.2007.05.009
Zaninelli, M., Redaelli, V., Luzi, F., Bronzo, V., Mitchell, M., Dell'Orto, V., et al. (2018). First evaluation of infrared thermography as a tool for the monitoring of udder health status in farms of dairy cows. Sensors 18:862. doi: 10.3390/s18030862
Zhang, F. Y., Hu, Y. M., Chen, L. C., Guo, L. H., Duan, W. J., and Wang, L. (2016). Monitoring behavior of poultry based on RFID radio frequency network. Int. J. Agricult. Biol. Eng. 9, 139–147. doi: 10.3965/j.ijabe.20160906.1568
Keywords: affective states, human-animal relationship, livestock production, sensors, smart farming, precision livestock farming, animal welfare
Citation: Schillings J, Bennett R and Rose DC (2021) Exploring the Potential of Precision Livestock Farming Technologies to Help Address Farm Animal Welfare. Front. Anim. Sci. 2:639678. doi: 10.3389/fanim.2021.639678
Received: 09 December 2020; Accepted: 15 April 2021;
Published: 13 May 2021.
Edited by:
Janice M. Siegford, Michigan State University, United StatesReviewed by:
Jasmeet Kaler, University of Nottingham, United KingdomSusanne Waiblinger, University of Veterinary Medicine Vienna, Austria
Copyright © 2021 Schillings, Bennett and Rose. This is an open-access article distributed under the terms of the Creative Commons Attribution License (CC BY). The use, distribution or reproduction in other forums is permitted, provided the original author(s) and the copyright owner(s) are credited and that the original publication in this journal is cited, in accordance with accepted academic practice. No use, distribution or reproduction is permitted which does not comply with these terms.
*Correspondence: Juliette Schillings, j.schillings@pgr.reading.ac.uk