- 1Department of Animal Science, North Dakota State University, Fargo, ND, United States
- 2Department of Statistics, North Dakota State University, Fargo, ND, United States
Solar radiation may be an important weather variable that has not been included in previous dry matter intake (DMI) prediction models. Solar radiation affects the overall effective ambient temperature, which in turn contributes to the net gain of heat in an animal’s body. This experiment examined ambient temperature and solar radiation with DMI in beef steers. Data from 790 beef steers collected between 2011 and 2018 using an Insentec feeding system was used. Daily data was condensed into weekly averages (n = 13,895 steer-weeks). The variables considered for this study were DMI (2.50 to 23.60 kg/d), body weight (197 to 796 kg), calculated dietary energy density (NEm; 0.79 to 2.97 Mcal/kg), ambient temperature (-23.73 to 21.40°C), two-week lag of ambient temperature (-20.73 to 23.56°C), monthly lag of ambient temperature (-17.95 to 22.74°C), solar radiation (30.8 to 297.1 W/m2), two-week lag of solar radiation (34.6 to 272 W/m2) and monthly lag of solar radiation (43.7 to 256.6 W/m2). Residuals of DMI fitting week of the year (fixed) and experiment (random) were used to generate scatter plots with other explanatory variables to identify if non-linear relationships existed. Body weight and NEm had both linear and quadratic relationships with DMI, while the relationship with DMI for other variables was linear. The MIXED procedure of SAS with Toeplitz variance-covariance structure was used to determine the final model of DMI. After accounting for body weight and NEm in the model, two-week lag of ambient temperature and monthly lag of solar radiation interacted together (P = 0.0001), and this accounted for 0.7790 (R2) variation in DMI and improved the model fit. Therefore, these two variables and their interactions should be considered in DMI prediction equations of beef steers.
Introduction
It has been well established that the thermal environment has significant influence on livestock species (NRC, 1981). Animals compensate for changes in their thermal environment by either adjusting the amount of energy they consume, improving their method of heat dissipation or altering their metabolism. Thornton et al. (2009) reported that when animals do not acclimatize to a sudden change in weather, the result is reduction in production, or even death. The change in the environment due to climate change poses a risk to livestock production, and this necessitates accounting for more of the environmental (weather) variables that influence dry matter intake. This will enable producers to provide for their livestock more accurately with the amount of nutrients and energy to reduce their vulnerability to normal and extreme weather conditions.
Thermoregulation in cattle is dependent on the breed, physiological class, age, and diet. Thermoregulation is achieved by the interaction of extrinsic environment with the intrinsic environment, which results in a response for the maintenance of homeostasis. The response could be in the form of lowering metabolism, vasoconstriction, or increasing the quantity of hairs (Nakamura and Morrison, 2008). Collier et al. (2019) summarized the effect of varying thermal conditions on feed intake in Holstein cows in a controlled environment. They observed a decrease in feed intake as the thermal environment increased from a temperature humidity index (THI) of 57 to 72 (cool to hot). They also reported that an array of environmental factors such as ambient temperature, solar radiation, relative humidity and windspeed are known to have either direct or indirect effects on livestock. However, to the best of our knowledge, DMI estimation models for beef cattle do not account for the effect that solar radiation may have on DMI. In addition, the current DMI models available may not fit the northern Great Plains of North America well, where temperatures can be as low as -30°C in the winter (Block et al., 2001). Therefore, improving these models may be beneficial. Our objective was to examine how much variation in DMI is accounted for by ambient temperature and solar radiation.
Materials and methods
Data collection
Data used for this experiment were collected from the Beef Cattle Research Complex (BCRC) of North Dakota State University, Fargo, North Dakota located at latitude 46.9027853 degrees North and longitude -96.8418183 degrees West. An Insentec feeding system (RIC feeding system; Hokofarm Group, Marknesse, The Netherlands), which records the amount of feed intake, number of visits, time of visit and meals for each animal, was used for the data collection. The data used were from 10 experiments that were conducted between 2011 to 2018 (Table 1). The Insectec system does a good job of reducing feed waste, for this study, feed disappearance from the bunk is assumed to be the intake by the animals.
Weather data
Data for weather variables were obtained from the North Dakota Agricultural Weather Network (NDAWN) station, which is 2.33 km from the BCRC (NDAWN, 2021). Each NDAWN station is assumed by NDAWN to adequately represent all weather conditions, except rainfall, in a 32 km radius. For this study, daily summaries of each weather variable were averaged for each week and these weekly averages were used in the analysis as described below.
Weather variables modeled for this study included: ambient temperature, the average air temperature of the surrounding environment for a 24-hour period from midnight to midnight (°C); solar radiation, sum of all hourly totals of incident solar radiation energy for a 24-hour period from midnight to midnight. Total incident solar radiation flux density is measured in Watts/m2 at approximately 2 m above the soil surface with a pyranometer, and two-week lag and monthly lag of each weather variable was also considered. Two-week lag is the average of the previous two week’s weather variable while monthly lag is the average of the previous month’s weather variable.
Non-weather variables
The non-weather variables considered for this experiment include weekly average of daily dry matter intake (DMI) in kg, weekly average body weight (BW) in kg, dietary concentration of net energy for maintenance (NEm) Mcal/kg of DM, experiment, and the week of the year. Week of the year ranged from week 1 to 22 and week 38 to 52.
Data management
The daily feed intake data were averaged into weekly averages to reduce the day-to-day fluctuation. Dry matter analysis (AOAC, 2010) of the diet was conducted on samples collected weekly and used, along with as-fed feed intake, to calculate DMI consumed by each animal. Weekly BW for each animal was calculated from BW data collected monthly by using simple linear regression. Daily ambient temperature and solar radiation were averaged per week to align with weekly DMI Dietary NEm was calculated by using the equation of Lofgreen and Garrett (1968) and Zinn and Chen (1998) using initial BW, final BW, average daily gain (ADG) and average DMI. Table 2 shows the descriptive statistics of the variables used for this study.
Statistical analysis
Data were analyzed using the MIXED procedure of SAS 9.4 software (SAS Institute Inc., 2015) and within-individual relationship due to repeated measures per steer was accounted for using the Toeplitz covariance structure. Raw DMI was initially fit to output residuals adjusted for week of the year (fixed) and experiment (random). DMI residuals were then investigated for linear correlations and scatter plots with weight, NEm, and weather variables using CORR procedure of SAS. Raw DMI was then fit with a base model, which included linear and quadratic effects of BW and NEm, week of the year (fixed), and experiment (random) effects. The base model was expanded in a stepwise addition of weather variables as fixed covariates using maximum likelihood (ML) to compare fit. Each version of each weather variable (no-lag, two-week lag, monthly lag) were fit independently of other versions so that each weather variable was present in the model only once. Akaike information criterion (AIC) and Bayesian information criterion (BIC) values were used to assess model fit during the stepwise process. Once the final model was determined, parameter estimates were generated using the restricted maximum likelihood estimation (REML) procedure and solution statement. Coefficient of determination (R2) for each model was calculated using the glmmQPL function in R (v 4.2.1, R Core Team, 2022).
Results
Correlation between BW, NEm and residuals of DMI
The correlation between BW and NEm, and residuals of DMI, adjusted for week of the year (fixed) and experiment (random), confirmed that linear and quadratic relationships exist after examining the trend of the scatter plots and testing the linear and quadratic effects of BW and NEm in the model. The correlation coefficients for the relationships for BW and NEm with DMI were 0.2312 (P < 0.0001; Figure 1.) and -0.073 (P< 0.0001; Figure 2.), respectively. Therefore, both the linear and quadratic effect of BW and NEm were retained in the model (Table 3).
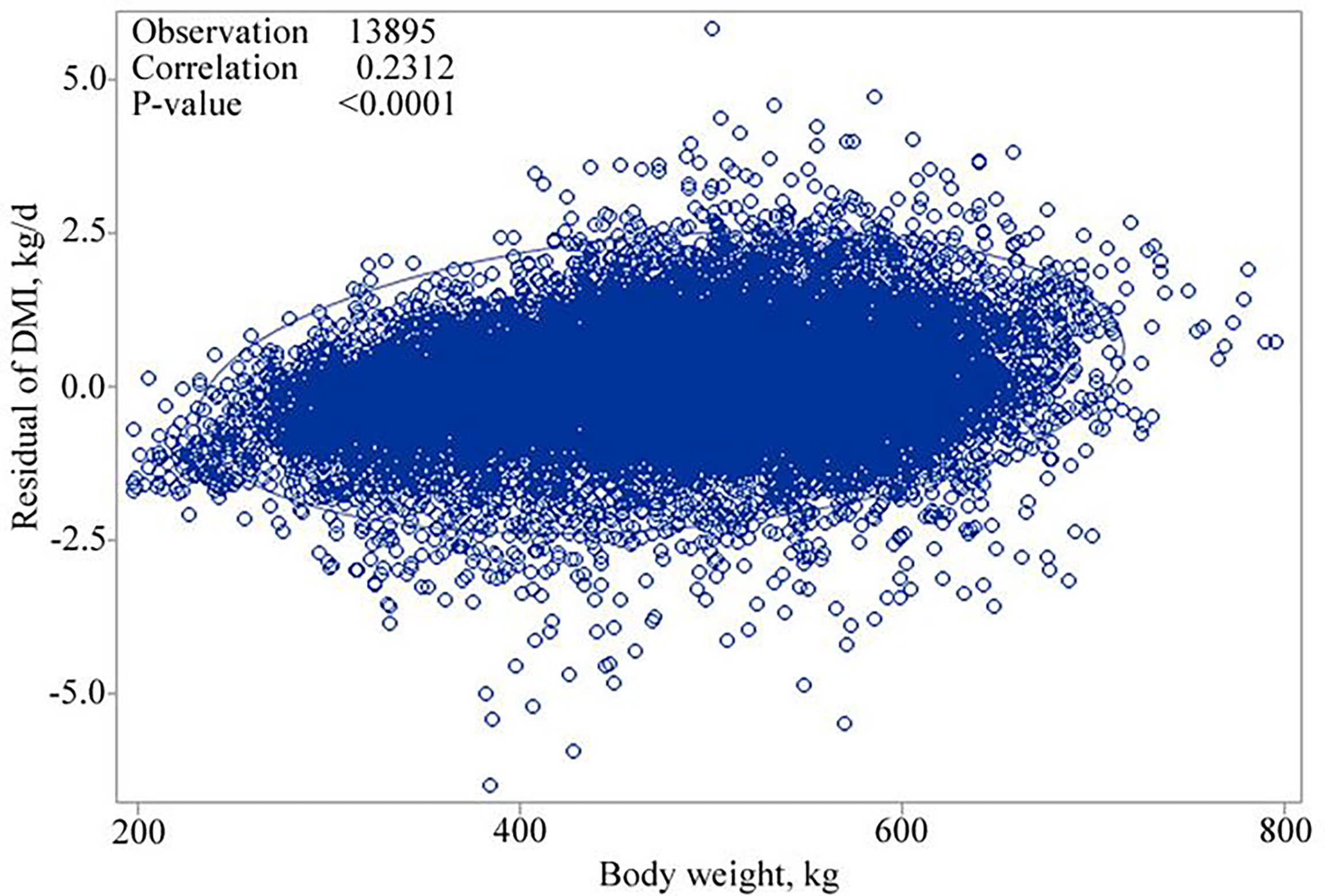
Figure 1 Scatter plot showing the relationship of residuals of dry matter intake (DMI) against body weight, kg.
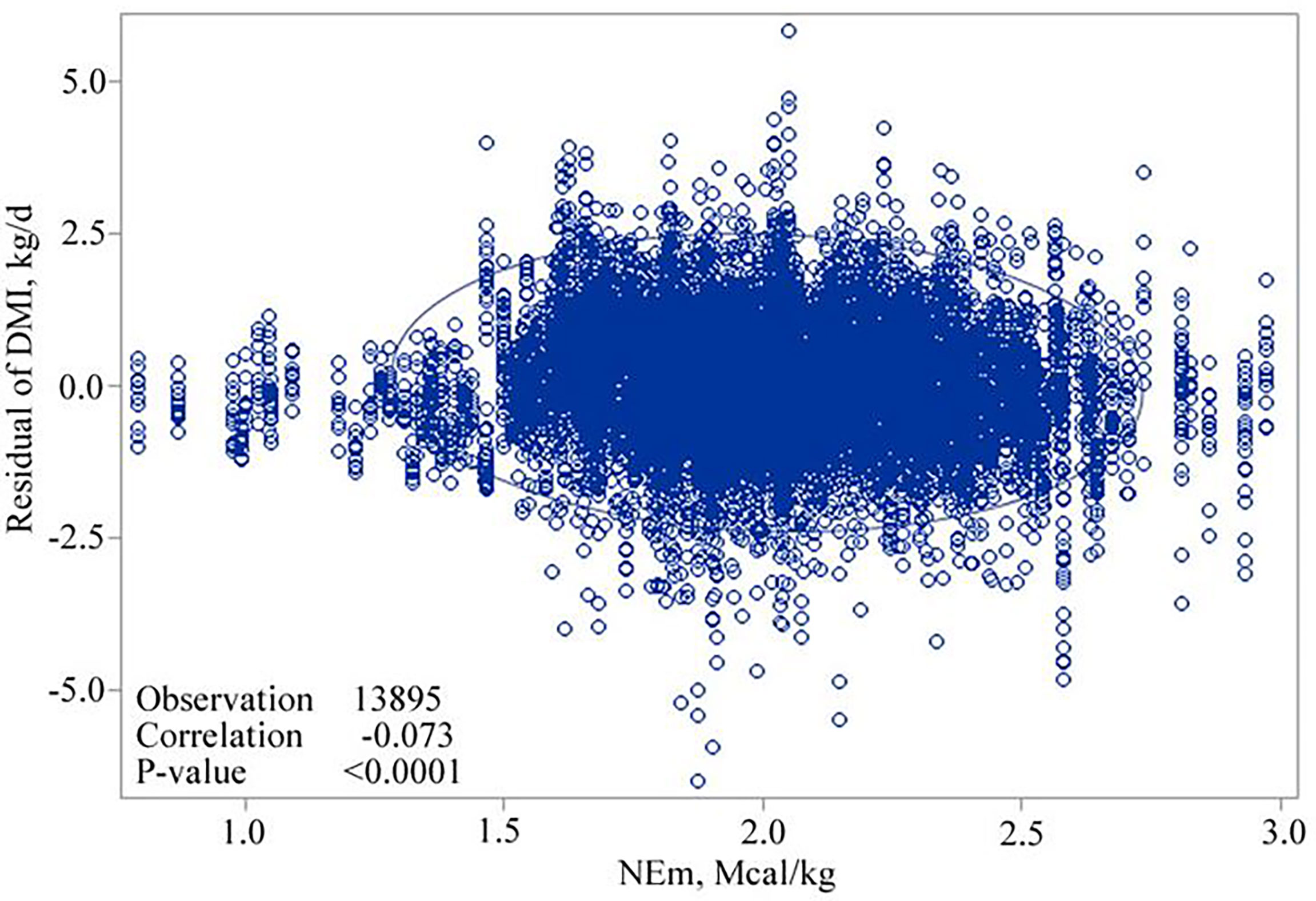
Figure 2 Scatter plot showing the relationship of residuals of dry matter intake (DMI) against net energy for maintenance (NEm, Mcal/kg of DM).
Base model, ambient temperature, and solar radiation
Table 4 provides model summary statistics when fitting each weather variable independently of each other to the base model. Inclusion of all three forms (no-lag, two-week lag, and monthly lag) in a single model was not possible due to multicollinearity. All three ambient temperature variables were significant sources of variation, with the two-week lag providing the best model fit statistics (Table 4). Model parameters when including the two-week lag regressor are provided in Table 5.
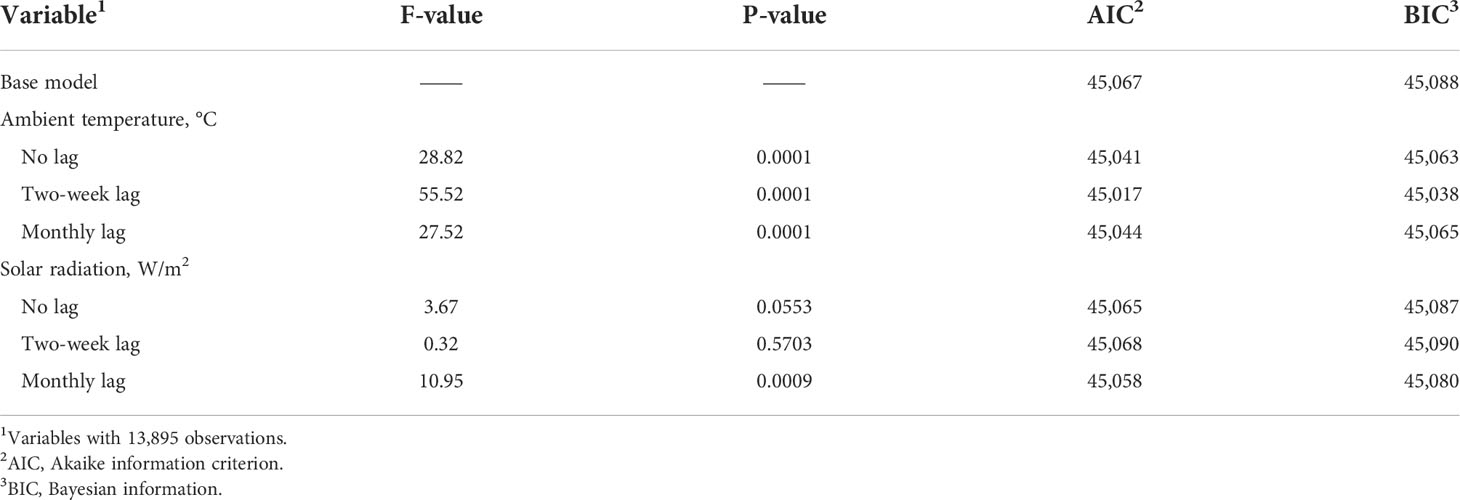
Table 4 AIC, BIC, F and P values of each weather variable considered when added to the base model individually using maximum likelihood estimation method (ML).
For solar radiation, only monthly lag of solar radiation was significant and improved model fit statistics compared to the base model (Table 4). Model parameters, when including the monthly lag regressor of solar radiation are provided in Table 6. When the main effect of two-week lag of ambient temperature and monthly lag of solar radiation were included in the model, only two-week lag of ambient temperature was significant (P < 0.005) in the model (Table 7). Although, the main effect of monthly lag of solar radiation was not significant when considered with two-week lag of ambient temperature, monthly lag of solar radiation was significant when included in the model alone. This prompted examination of an interaction between these two variables.
When the interaction between two-week lag of solar radiation and monthly lag of solar radiation was included in the model while retaining the main effects of each, the fit improved and indicated a significant interaction (Table 8). The BIC values were reduced by 5 points indicating an improvement in model fit relative to the previous model that had no interaction between ambient temperature and solar radiation included. The reason the main effects were insignificant could be because of a cancellation effect that the main effects and the interactions had on each other. When only the interaction between two-week lag of ambient temperature and monthly lag of solar radiation were added to the base model, the interaction was highly significant (P = 0.0001), and the AIC and BIC values were lower indicating the model was improved and has a better model fit (Table 9; Figure 3). Since the interaction between two-week lag of ambient temperature and monthly lag of solar radiation gave a better model fit, the interaction was left in the model while their main effects were removed. AIC, BIC, and R2 values for the models are shown (Table 10). It is important to note that there is no agreed way of calculating R2 in mixed models of SAS. Therefore, R statistical software was implemented using the method described by Nakagawa et al. (2017). Caution should be taken when interpreting the R2 values because it is an index that is likely to interpret only a few aspects of model fit to the data and should not be used to determine the quality of the model but rather should be used along with the AIC and BIC values (Nakagawa and Schielzeth, 2013; Nakagawa et al., 2017).
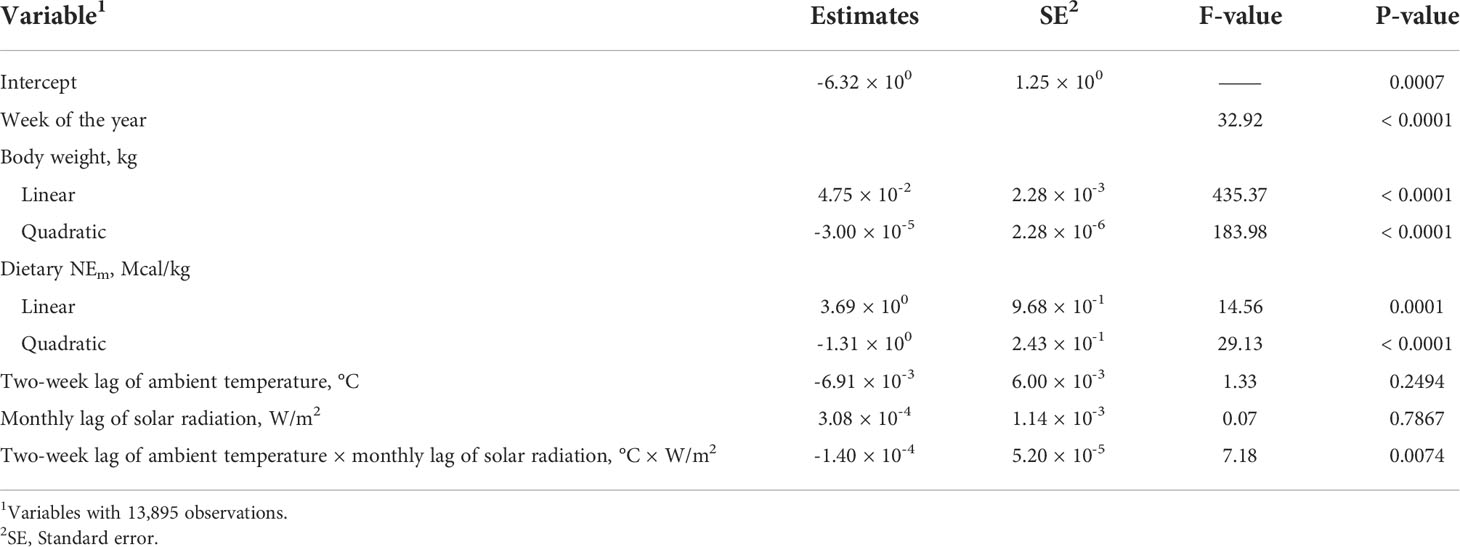
Table 8 Base model, two-week lag of ambient temperature and monthly lag of solar radiation and their interaction.
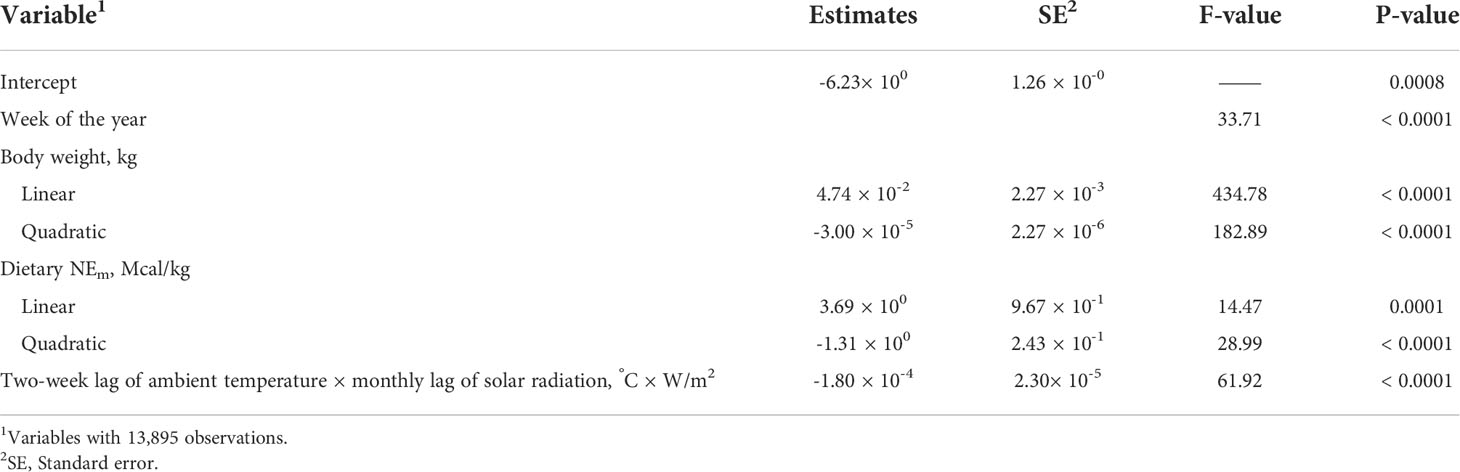
Table 9 Base model and interaction between two-week lag of ambient temperature and monthly lag of solar radiation using restricted maximum likelihood estimation method (final model).
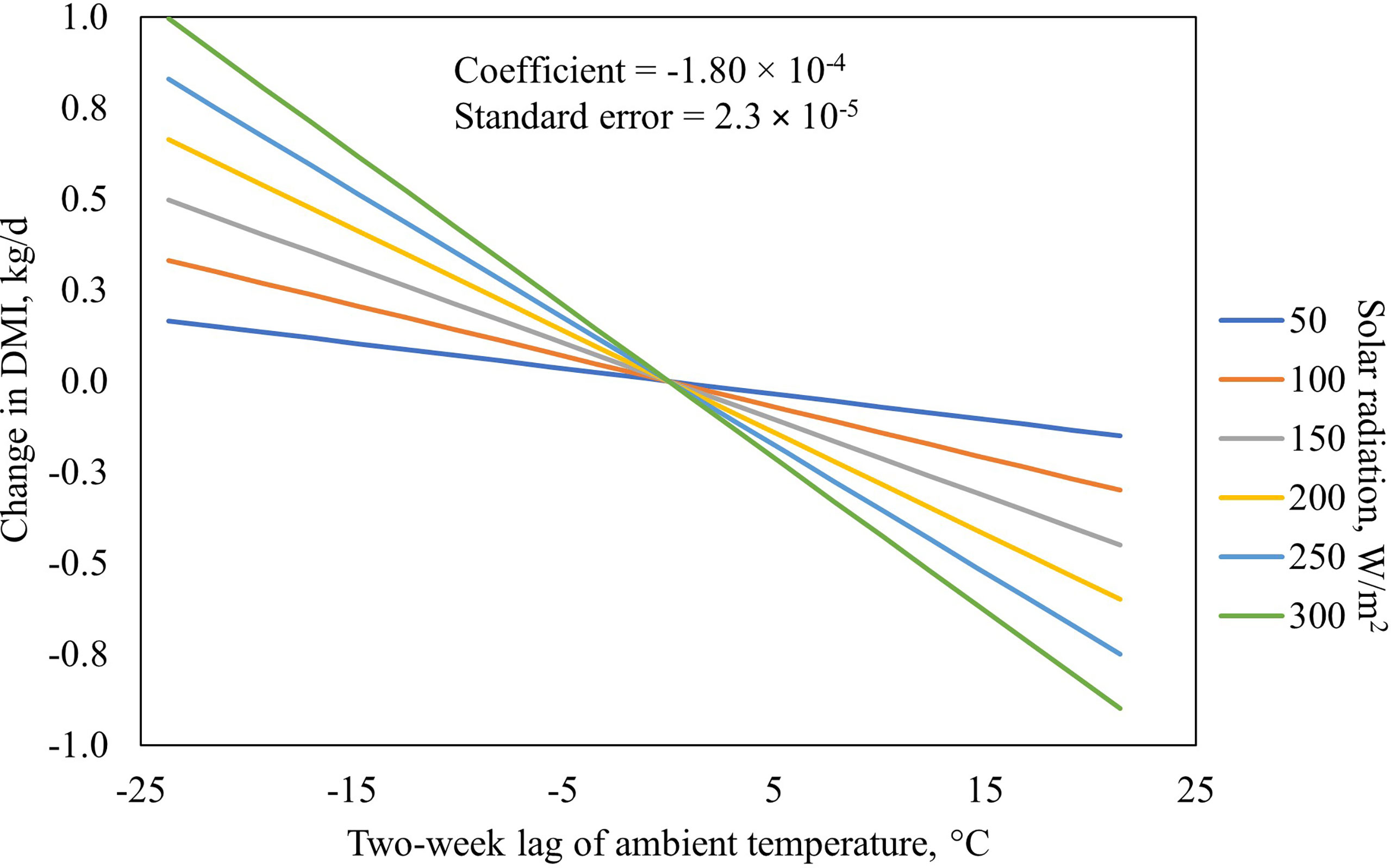
Figure 3 The final model interaction between two-week lag of ambient temperature and monthly lag of solar radiation and their influence on dry matter intake (DMI). F-value = 61.92. P-value < 0.0001.
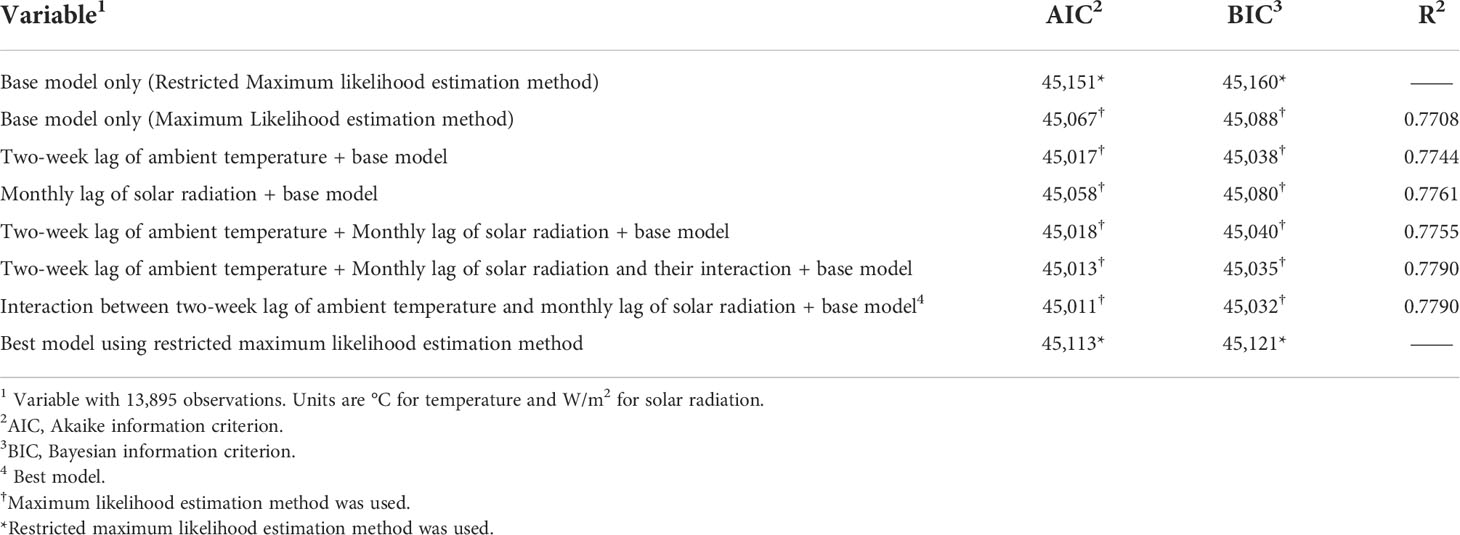
Table 10 AIC, BIC, and R2 (coefficient of determination) values of various models examining solar radiation as a predictor variable for DMI in beef steers to summarize the model fit in each stepwise process.
Discussion
Body weight as a predictor for DMI has long been reported (Lehmann, 1941; Kruger and Schulze, 1956; Conrad et al, 1964; Baile and Forbes, 1974). It is necessary to account for BW in our model so that the contribution of BW the variation in DMI by other variables can be examined more accurately. Generally, our data starts with younger, lighter calves in the fall and winter, and ends with older, heavier calves in late winter, spring, and early summer, depending on the study. When analyzed, BW of steers are distributed across season, but the effect of age, BW, or a combination may still not be completely accounted for in our base model. Dietary energy density (Mcal of NEm/kg of feed) has also been reported by many authors as a major determinant of DMI in ruminants (Crampton et al, 1957; Blaxter, 1961; Baumgardt, 1970).
Previous temperature is thought to influence basal metabolism, thereby indirectly affecting DMI (NRC, 1981). This is supported by the work of Fox and Tylutki (1998), who recommended that the average over a month should be used in prediction models to remove the day-to-day variation because temperature changes slowly from season to season. In this study, data was collected during the colder months with average weekly temperature ranging from -2 to 24°C and average weekly solar radiation from 30 to 300 W/m2; therefore, this model may not accurately represent regions with warmer temperatures and/or different solar radiation patterns. Environmental factors affecting DMI has been previously reported (NRC, 1981). Hill and Wall (2017) reported that at high temperatures, DMI typically decreases. Other factors such as growth rate and BW also affect DMI. Hill and Wall (2017) reported that thermal stress (either high or low temperature) might be better handled by efficient cattle compared to less efficient cattle. Efficient cattle are better at directing feed to growth and have been reported to have lower rectal temperatures and produce less metabolic heat (Basarab et al., 2003). NRC (1981) recommended a thermoneutral temperature range of 15 to 25°C for beef cattle. For cold weather conditions, DMI is often thought to increase. However, an apparent relationship between ambient temperature and DMI does not exist as reported by NRC (1981) because ambient temperature is most likely influenced by other variables. For example, Mader et al. (2010) reported that the strength of relationship between ambient temperature and DMI might be questioned because DMI is influenced by cattle type, body condition, management, and other environmental factors. In our study, we accounted for the variation that could be explained by BW, dietary energy density, individual differences in animals and time of the year. All possible variations that may exist from the animal and the environment which are known to affect DMI were accounted for in the base model. However, there could be other unknown variables that affect DMI that were not accounted for, such as solar radiation and the interaction between ambient temperature and solar radiation.
Solar radiation has been reported to have an influence on ambient temperature and heat loss from animals (Brosh et al., 1998). The sun angle changes daily and seasonally, which influences the thermal balance of the animal because exposed surface area and insulation are affected differentially (Keren and Olson, 2006). A perpendicularly standing animal to the sun’s ray will absorb more short-wave radiation than one standing parallel to the sun (Clapperton et al., 1965). Factors such as sky conditions, ground cover and the shape and orientation of the animal’s body also determine the amount of solar radiation absorbed (Keren, 2005). Prediction models used in the past did not examine the lag of solar radiation nor did they consider solar radiation separately, rather it was considered with other weather variables using an index named current effective temperature index (CETI) which accounts for temperature, humidity, wind speed and sunlight hours (Tedeschi and Fox, 2016). Mader et al. (2010) developed a comprehensive climate index (CCI) using ambient temperature while adjusting for relative humidity, wind speed and solar radiation. This type of indices do not explain the interaction of solar radiation or its lag with temperature on DMI.
The better model fit we observed in this study between the interaction between solar radiation and ambient temperature indicates that solar radiation is important and could better explain the variation in DMI than just temperature alone. Others (Bakken, 1981; Mader et al., 2010; Tedeschi and Fox, 2016) have considered ambient temperature and some weather variables together, combining them into an index. This shows that multiple weather variables interact together to affect DMI suggesting that combining weather variables into an index should be discouraged. It is important to note that, although the main effect of week of the year was accounted in this study, there might be an interaction between week of the year and temperature or solar radiation. We did not try to account for this interaction in this study to avoid complexity in the models.
National Academies of Science, Engineering, and Medicine (NASEM, 2016) reported that solar radiation accentuates the effect of temperature. In our model, solar radiation accentuated the effect that low and high temperature had on DMI. Interestingly, in Figure 3, it can be observed that, with increasing temperature and reduction in solar radiation, DMI increased. This could be attributed to the interaction between temperature and solar radiation and how they influence each other. This could suggest that on sunny days with high temperature, DMI decreases but on sunny days with extremely low temperature, DMI increases. In extreme cold temperatures, the NEm requirement of cattle increases linearly and, therefore, the animal needs to increase energy intake from feed to meet the requirement for increased heat production and maintenance of homeostasis. There is a dearth of information on the effect of solar radiation on animals in extremely cold weather conditions. Most reported studies examined the effect of solar radiation on animals in warm to hot weather conditions (Mader et al., 2006; Mader at al., 2010; Melton et al, 2018; Lees et al., 2019). Studies that examined the effect of cold weather conditions on animals (Siple and Passel, 1945) did not examine the effect of solar radiation on DMI. Siple and Passel (1945) developed a windchill index (WCI), relating ambient temperature (Ta) and wind speed (WS) to the time for freezing water for cold conditions. Mader et al. (2006) developed adjustments to the THI based on panting scores and measures of wind speed and solar radiation but only two studies were conducted in cold weather conditions in the data they examined. Olson (1938) examined the effect of sunlight on dairy cattle that were exposed to sunlight or without sunlight and fed the same amount of feed. The no-sunlight group had better growth than the sunlight group because the sunlight group were housed outside and maintained under cold winter conditions. This corroborates the effect of extreme cold weather on energy requirements and growth. If the intake of the animal does not increase to meet the energy demand for heat production, growth performance is compromised. On the other hand, under high ambient temperatures, livestock are expected to have decreased DMI to reduce their metabolic heat production. Mader et al. (2010) reported that solar radiation and ambient temperature have a linear relationship, which is similar with what we observed in this study. Heat input from metabolic heat production and solar radiation, and heat output from evaporative and non-evaporative avenues are the factors that determine body temperature in cattle (Brosh et al., 1998). As temperature decreased to below the lower critical temperature, the animal becomes cold stressed, and the maintenance energy requirement increases. Although it is often assumed that DMI increases with decreasing temperature in cold weather, Donald (1988) reported that animals under severe cold stress tend to have reduced intake. However, in this study, DMI increased with increasing solar radiation and reduction in temperature. This may be because increases in solar radiation lessen the negative effect of the cold stress on the animal resulting in an increase DMI. Olson and Wallander (2002) reported that during extreme cold weather, cattle spent more time standing to maximize heat gain from solar radiation instead of lying down. However, our final model indicates that DMI increased with increasing solar radiation and decreasing temperature, whereas DMI decreased with increasing solar radiation and increasing temperature. DMI changed less when solar radiation was minimal. This may be because increases in solar radiation lessens the negative effect of cold stress or enhances the negative effect of hotter ambient temperatures on the animal resulting in changes in DMI.
Solar radiation is known to influence thermal balance of ruminants. Study by Sevi et al. (2001) examined the effect of solar radiation on Comisana ewes. They reported that solar radiation and the interaction between solar radiation and feeding time had significant effect on rectal temperatures. This indicates that solar radiation influences thermal balance, energy metabolism and could be attributed to the change in DMI at different intensities of solar radiation. Solar radiation has been reported to directly affect the surface that an animal has contact with as well as the temperature of the animal, especially in dark-hided cattle (Mader et al., 2006). Kennedy et al. (1986) reported that in cold weather, ruminal motility and digesta passage increases, which could be contributing factors to the observed increases in DMI. Sunshine hours and day length both contribute to solar radiation reaching an animal directly or indirectly (absorbed by surrounding surfaces and the ground). Dahl et al. (2000) observed a positive relationship between milk production and day length which could be because of reduced melatonin production with increasing photoperiod. The influence of day length and temperature on performance of Swedish red and white bulls fed ad libitum concentrates or ad libitum forage and concentrates was reported to observe an increase in DMI as day length increased (Mossberg and Jönsson, 1996). This is similar to the result in this study where increased solar radiation in cold weather was observed to increase DMI but caused a reduced DMI in warmer temperatures.
It was reported by NASEM (2016) that other adverse weather conditions can increase the effects of ambient temperature. However, the response to temperature varies between animals (Young, 1981). The observed increase in DMI as two-week lag of temperature decreases, and monthly lag of solar radiation increases, could also be attributed to the long-term effect of solar radiation on melatonin. Light inhibits melatonin secretion by inhibiting the production of N-acetyltransferase, the primary enzyme for melatonin synthesis (Hickman et al., 1999). Melatonin slows metabolism, increases fat deposition and decreases feed intake and, ultimately, productivity of animals. With more light and solar radiation, we speculate that this caused a reduction in melatonin secretion over time, therefore, contributing to the observed increase in feed intake. However, more research is needed on the relationships between solar radiation, melatonin secretion, DMI, and growth.
Conclusion
To summarize, our results showed that variation in DMI was better explained by having the interaction between two-week lag of ambient temperature and monthly lag of solar radiation in the prediction model as opposed to ambient temperature alone. This indicates that solar radiation could be a good predictor and explain some variation in DMI occurring because of thermal effects. Furthermore, using a model similar to the model developed in this study may be a better alternative than using THI or CCI as an index combining effects of individual weather variables.
Implications
Changes in and the interaction between solar radiation and temperature were associated with changes in DMI. We suggest that these variables may be important and should be considered in DMI prediction equations. Dry matter intake changes in response to adverse weather conditions. Dry matter intake is influenced by several factors and how cattle respond to changes in DMI is highly variable among individuals. Understanding the variables that influence DMI will help in increasing the accuracy of DMI prediction models, which will in turn assist producers and feedlot managers better manage daily feed delivery and feed inventories. Further research to examine how other weather variables such as windspeed and dewpoint interact with temperature and solar radiation to influence DMI and ADG is needed.
Data availability statement
The original contributions presented in the study are included in the article/supplementary material. Further inquiries can be directed to the corresponding author.
Ethics statement
Ethical review and approval was not required for the animal study because all data had been collected previously. All previous studies were reviewed and approved by the North Dakota State University Animal Care and Use Committee.
Author contributions
MY compiled the data, analyzed the data, and wrote the manuscript. KS helped with raw data collection, formatting, and manuscript review. LHH contributed to the analysis of the data and manuscript review. RD contributed to the analysis of the data. MB helped with raw data collection, formatting, data analysis, manuscript preparation, review, and submission.
Conflict of interest
The authors declare that the research was conducted in the absence of any commercial or financial relationships that could be construed as a potential conflict of interest.
Publisher’s note
All claims expressed in this article are solely those of the authors and do not necessarily represent those of their affiliated organizations, or those of the publisher, the editors and the reviewers. Any product that may be evaluated in this article, or claim that may be made by its manufacturer, is not guaranteed or endorsed by the publisher.
References
AOAC. (2010). Official methods of analysis of AOAC International. AOAC International, Horwitz W., Latimer G. 18th ed. rev 3 (Gaithersburg, MD: AOAC Int.).
Baile C. A., Forbes J. M. (1974). Control of feed intake and regulation of energy balance in ruminants. Physiol. Rev. 54, 160–214. doi: 10.1152/physrev.1974.54.1.160
Bakken G. S. (1981). How many equivalent black-body temperatures are there? J. Therm. Biol. 6, 59–60. doi: 10.1016/0306-4565(81)90045-0
Basarab J. A., Price M. A., Aalhus J. L., Okine E. K., Snelling W. M., Lyle K. L. (2003). Residual feed intake and body composition in young growing cattle. Can. J. Anim. Sci. 83, 189–204. doi: 10.4141/A02-06
Baumgardt B. R. (1970). “Control of feed intake in the regulation of energy balance,” in Physiology of digestion and metabolism in the ruminant. Ed. Phillipson A. T. (New-castle-Upon-Tyne: Oriel Press), 235–253.
Blaxter K. L. (1961). Efficiency of feed conversion by different classes of livestock in relation to food production. Fed. Proc. 20, 268–274.
Block H. C., McKinnon J. J., Mustafa A. F., Christensen D. A. (2001). Evaluation of the 1996 NRC beef model under western Canadian environmental conditions. J. Anim. Sci. 79, 267–275. doi: 10.2527/2001.791267x
Brosh A., Aharoni A. A., Degen A. A., Wright D., Young B. A. (1998). Effects of solar radiation, dietary energy, and time of feeding on thermoregulatory responses and energy balance in cattle in a hot environment. J. Anim. Sci. 76, 2671–2677. doi: 10.2527/1998.76102671x
Clapperton J., Joyce J. P., Blaxter K. L. (1965). Estimates of the contribution of solar radiation to the thermal exchanges of sheep at a latitude of 55° north. J. Agric. Sci. (Cambridge) 64, 37–49. doi: 10.1017/S0021859600010455
Collier R. J., Baumgard L. H., Zimbelman R. B., Xiao Z. Y. (2019). Heat stress: Physiology of acclimation and adaptation anim. Front. Anim. 9, 12–19. doi: 10.1093/af/vfy031
Conrad H. R., Pratt A. D., Hibbs J. W. (1964). Regulation of feed intake in dairy cows; change in importance of physical and physiological factors with increasing digestibility. J. Dairy Sci. 47, 54–62. doi: 10.3168/jds.S0022-0302(64)88581-7
Crampton E. W. (1957). Interrelations between digestible nutrient and energy content, voluntary dry matter intake, and the overall feeding value of forages. J. Anim. Sci. 16, 546–552. doi: 10.1093/ansci/16.3.546
Dahl G. E., Buchanan B. A., Tucker H. A. (2000). Photoperiodic effects on dairy cattle: a review. J. Dairy. Sci. 83, 885–893. doi: 10.3168/jds.S0022-0302(00)74952-6
Donald G. W. (1988). Effects of cold stress on cattle performance and management factors to reduce cold stress and improve performance. Bovine Practitioner 23, 88–92. doi: 10.21423/bovine-vol0no23p88-93
Fox D. G., Tylutki T. P. (1998). Accounting for the effects of environment on the nutrient requirements of dairy cattle. J. Dairy Sci. 81, 3085–3095. doi: 10.3168/jds.S0022-0302(98)75873-4
Hickman A. B., Klein D. C., Dyda F. (1999). Melatonin biosynthesis: The structure of serotonin n-acetyltransferase at 2.5 Å resolution suggests a catalytic mechanism. Mol. Cell. 3, 23–32. doi: 10.1016/s1097-2765(00)80171-9
Hill D. L., Wall E. (2017). Weather influences feed intake and feed efficiency in a temperate climate. J. Dairy Sci. 100, 2240–2257. doi: 10.3168/jds.2016-11047
Islas A., Gilbery T. C., Goulart R. S., Dahlen C. R., Bauer M. L., Swanson K. C. (2014). Influence of supplementation with corn dried distillers grains plus solubles to growing calves fed medium-quality hay on growth performance and feeding behavior. J. Anim. Sci. 92, 705–771. doi: 10.2527/jas.2013-7067
Kennedy P. M., Christopherson R. J., Milligan L. P. (1986). Digestive responses to cold. In: Control of digestion and metabolism in ruminants. Eds. Milligan L. P., Grovum W. L., Dobson A. (Engle-wood Cliffs. NJ: Prentice Hall), p. 285–306.
Keren I. N. (2005). “Thermal balance model for cattle grazing winter range”, in Master’s thesis submitted to the department of animal and range sciences (Bozeman, MT: Montana State University).
Keren E. N., Olson B. E. (2006). Thermal balance of cattle grazing winter range: Model development. J. Therm. Biol. 31, 371–377. doi: 10.2527/2006.8451238x
Knutson E. E., Menezes A. C. B., Sun X., Fountoura A. B. P., Liu J. H., Bauer M. L., et al. (2020). Effect of feeding a low-vitamin a diet on carcass and production characteristics of steers with a high or low propensity for marbling. Animal 14, 2308–2314. doi: 10.1017/S1751731120001135
Kruger L., Schulze G. (1956). Uber futteraufnahme und verzehrleistungen bei milchkuhen II. die sattigungsbewertung der futtermittel. Zuch tungskunde 28, 438–450.
Lees A. M., Sejian V., Wallage A. L., Steel C. C., Mader T. L., Lees J. C., et al. (2019). The impact of heat load on cattle. Animals. 9, 322. doi: 10.3390/ani9060322
Lofgreen G. P., Garett W. N. (1968). A system for expressing net energy requirements and feed values for growing and finishing beef cattle. J. Anim. Sci. 27, 793–806. doi: 10.2527/jas1968.273793x
Mader T. L., Davis M. S., Brown-Brandl T. (2006). Environmental factors influencing heat stress in feedlot cattle. J. Anim. Sci. 84, 712–719. doi: 10.2527/2006.843712x
Mader T. L., Johnson L. J., Gaughan J. B. (2010). A comprehensive index for assessing environmental stress in animals. J. Anim. Sci. 88, 2153–2165. doi: 10.2527/jas.2009-2586
Melton B. M., Winders T., Boyd B. M., Macken C. N., Watson A. K., MacDonald J. C., et al. (2018). Impact of shade on performance, body temperature, and heat stress of finishing cattle in Eastern Nebraska. 10th Int. Livestock Environ. Symposium (ILES X). doi: 10.13031/iles.18-098
Mossberg I., Jönsson H. (1996). The influence of day length and temperature on food intake and growth rate of bulls given concentrate or grass silage ad libitum in two housing systems. Anim. Sci. 62, 233–240. doi: 10.1017/S1357729800014533
Nakagawa S., Johnson P. C. D., Schielzeth H. (2017). The coefficient of determination R2 and intraclass correlation coefficient from generalized linear mixed-effects models revisited and expanded. J. R. Soc. Interface 14, 20170213. doi: 10.1098/rsif.2017.0213
Nakagawa S., Schielzeth H. (2013). A general and simple method for obtaining R2 from generalized linear mixed models. Me. Eco. Evol. 4, 133–142. doi: 10.1111/j.2041-210x.2012.00261
Nakamura K., Morrison S. F. (2008). A thermosensory pathway that controls body temperature. Nat. Neurosci. 11, 62–71. doi: 10.1038/nn2027
National Academies of Science, Engineering, and Medicine (2016). Nutrient requirements of beef cattle. 8th Rev. Ed (Washington DC: The National Academies).
North Dakota Agricultural weather station (NDAWN) (2021). Available at: https://ndawn.ndsu.nodak.edu/ (Accessed January 15, 2021).
NRC (1981). Effect of environment on nutrient requirements of domestic animals (Washington DC: National Academy Press).
Olson T. M. (1938) Effect of sunlight on the growth, production, and reproduction of dairy cattle. bulletins. paper 319. Available at: http://openprairie.sdstate.edu/agexperimentsta_bulletins/319.
Olson B. E., Wallander R. T. (2002). Influence of winter weather and shelter on activity patterns of beef cows. Can. J. Anim. Sci. 82, 491–501. doi: 10.4141/A01-070
Prezotto L. D., Gilbery T. C., Bauer M. L., Islas A., Swanson K. C. (2017). Influence of limit-feeding and time of day of feed availability to growing calves on growth performance and feeding behavior in cold weather. J. Anim. Sci. 95, 5137–5144. doi: 10.2527/jas2017.1925
R Core Team (2022). R: A language and environment for statistical computing (Vienna, Austria: R foundation for Statistical Computing). Available at: https://www.R-project.org/.
Rodenhuis M. A., Keomanivong F. E., Gaspers J. J., Gilbery T. C., Underdahl S. R., Bauer M. L., et al. (2017). Influence of grain source and dried corn distiller’s grains plus solubles oil concentration on finishing cattle performance and feeding behavior. Can. J. Anim. Sci. 97, 545–552. doi: 10.1139/cjas-2016-021
SAS Institute Inc. (2015). Base SAS 9.4 Procedures Guide. 5th ed. (Cary, NC: SAS Institute Inc.). Available: http://support.sas.com.
Sevi A., Annicchiarico G., Albenzio M., Taibi L., Muscio A., Dell’Aquila S. (2001). Effects of solar radiation and feeding time on behavior, immune response, and production of lactating ewes under high ambient temperature. J. Dairy Sci. 84, 629–640. doi: 10.3168/jds.S0022-0302(01)74518-3
Siple P. A., Passel C. F. (1945). Measurements of dry atmospheric cooling in subfreezing temperatures. Proc. Am. Philos. Society. 89, 177–199.
Sitorski G. L., Bauer M. L., Swanson K. C. (2019). Effect of metabolizable protein intake on growth performance, carcass characteristics, and feeding behavior in finishing steers. Transl. Anim. Sci. 3, 1173–1181. doi: 10.1093/tas/txz124
Swanson K. C., Carlson Z. E., Ruch M. C., Gilbery T. C., Underdahl S. R., Keomanivong F. E., et al. (2017). Influence of forage source and forage inclusion level on growth performance, feeding behavior, and carcass characteristics in finishing steers. J. Anim. Sci. 95, 1325–1334. doi: 10.2527/jas.2016.1157
Swanson K. C., Gaspers J. J., Keomanivong F. A., Gilbery T. C., Lardy G. P., Bauer M. L. (2018). Influence of feeding direct-fed microbial supplementation on growth performance and feeding behavior in naturally fed and conventionally fed finishing cattle with different dietary adaptation periods. J. Anim. Sci. 96, 3370–3380. doi: 10.1093/jas/sky194
Swanson K. C., Islas A., Carlson Z. E., Goulart R. S., Gilbery T. C., Bauer M. L. (2014). Influence of dry-rolled corn processing and increasing dried corn distillers grains plus solubles inclusion for finishing cattle on growth performance and feeding behavior. J. Anim. Sci. 92, 2531–2537. doi: 10.2527/jas.2013-7547
Tedeschi L. O., Fox D. G. (2016). The ruminant nutrition system: An applied model for predicting nutrient requirements and feed utilization in ruminants, XanEdu Acton, MA, USA.
Thornton P. K., Van de Steeg J., Notenbaert A., Herrero M. (2009). The impacts of climate change on livestock and livestock systems in developing countries: A review of what we know and what we need to know. Agric. Syst. 101, 113–127. doi: 10.16/j.agsy.2009.05.002
Trotta R. J., Maddock Carlin K. R., Swanson K. C. (2019). Effects of ractopamine hydrochloride supplementation on feeding behavior, growth performance, and carcass characteristics of finishing steers. Trans. Anim. Sci. 3, 1143–1152. doi: 10.1093/tas/txz114
Young B. A. (1981). Cold stress as it affects animal production. J. Anim. Sci. 52, 154–163. doi: 10.2527/jas1981.521154x
Keywords: steers, dry matter intake, solar radiation, temperature, prediction, cattle
Citation: Yusuf M, Swanson KC, Hulsman Hanna LL, Degges R and Bauer ML (2022) Solar radiation and temperature as predictor variables for dry matter intake in beef steers. Front. Anim. Sci. 3:975093. doi: 10.3389/fanim.2022.975093
Received: 21 June 2022; Accepted: 16 August 2022;
Published: 02 September 2022.
Edited by:
Todd Riley Callaway, University of Georgia, United StatesReviewed by:
Michael Gonda, South Dakota State University, United StatesTryon Wickersham, Texas A&M University, United States
Blake Wilson, Oklahoma State University, United States
Copyright © 2022 Yusuf, Swanson, Hulsman Hanna, Degges and Bauer. This is an open-access article distributed under the terms of the Creative Commons Attribution License (CC BY). The use, distribution or reproduction in other forums is permitted, provided the original author(s) and the copyright owner(s) are credited and that the original publication in this journal is cited, in accordance with accepted academic practice. No use, distribution or reproduction is permitted which does not comply with these terms.
*Correspondence: Marc L. Bauer, bWFyYy5iYXVlckBuZHN1LmVkdQ==