A systematic review of genotype-by-climate interaction studies in cattle, pigs, and chicken
- Animal Breeding and Genomics, Wageningen University and Research, Wageningen, Netherlands
The genetic progress achieved by animal breeding programs may be affected by genotype-by-climate interactions (GxC). This systematic literature review assesses the scientific evidence for GxC on multiple traits of cattle, pigs, and poultry. Two search engines (Scopus, Web of Science) were queried for original peer-reviewed scientific (English full-text) studies. We included (1) observational studies and designed experiments considering dairy or beef cattle, swine, chicken or turkeys, where (2) at least one production, fertility, or health trait was tested for GxC, (3) the existence of GxC was tested directly based on temperature, relative humidity, or climatic indices for heat or cold stress, and (4) genetic effects within a breed or line were investigated. The search resulted in 46 eligible studies, with a (low) risk that the requirement of full-text English studies may have resulted in some work not included in this review. Our review shows an increase in GxC studies from 2015 onwards. Cattle was the most studied species (n = 36; 78.3%), whereas eligible studies on turkeys were lacking. Climatic parameters used in the studies ranged from well-known parameters (e.g., temperature, temperature-humidity index) to more complex indices combining temperature, relative humidity, wind speed and solar radiation. All observational studies (n = 40; 87.0%) used weather station data. In total, 75 traits were studied, which were predominantly production traits regardless of species. Studies on fertility and health traits have been emerging from 2010 onwards, but their numbers still lag far behind those for production traits. Genotype-by-climate interaction was confirmed in 54.0% of the study outcomes. This systematic review shows that little is known about the role of GxC in health and fertility traits in cattle, and for all traits in pigs and poultry in general. As current evidence shows that genotype-by-climate interaction is common across species and traits, we suggest to collect detailed climatic data and use them to assess the presence of GxC in indoor and outdoor production systems, as well.
1 Introduction
The phenotype of an animal is not only determined by its genetic potential, but also by the environmental influences, and their interactions, it is subjected to. Although breeding programs and breeding value estimations account for variation that is due to environmental influences (e.g., contemporary group effects), current practices do not explicitly include all of the environmental settings that play a crucial role in the performance of livestock. Some of the main physical environmental factors affecting livestock on farms include air temperature, relative humidity, solar radiation, atmospheric pressure, and wind speed. Data on environmental parameters such as temperature and humidity are widely available (either measured in the barn, at the farm, or collected from a nearby weather station), but currently only used to monitor and control the climate in the barns (Fournel et al., 2017; Chen and Chen, 2019).
Advances in automated high-throughput data recording from sensors, cameras and other technologies have created opportunities in utilizing big data for a range of applications that go beyond monitoring the status of the animals and their environment (Koltes et al., 2019). These types of applications, phrased collectively as Precision Livestock Farming (PLF), can not only help with increasing efficiency and productivity, but also with improving animal health and welfare (Berckmans, 2017). In the last decade, an large amount of phenotypic data, as well as a considerable amount of environmental parameters have been collected using PLF technologies. At the same time, a significant share of the environmental data has been mainly used to inform management practice, but it has hardly been used in breeding programs or breeding value estimations, despite its potential added value (Koltes et al., 2019).
Heat stress impairs animal health and is currently an important challenge for livestock productivity (Shaji et al., 2015). If an animal is exposed to temperatures outside of its comfort zone, extra energy expenditure is required to maintain homeothermy, which leads to heat- or cold stress. The difference between normal and lethal body temperatures is especially small for hyperthermy (only about 3-6°C above normal body temperature), compared to hypothermia (15-25°C below normal body temperature) (Nardone et al., 2006). The detrimental effects of heat stress are well-studied in livestock, ranging from decreased feed intake, reduced fertility, as well as decreased production and altered carcass composition (Johnson, 2018). Considering that global temperatures are projected to rise by 1.4 to 3.0°C by the end of this century, the impact of heat stress on livestock is expected to become even more pronounced (IPCC, 2013). Additionally, climate change may increase the number of heat waves in temperate regions, thereby affecting livestock systems and specific breeds or lines that are often not used to dealing with such conditions (Johnson, 2018). Cattle are typically housed in open barns or kept on pasture, therefore, they are more directly exposed to the weather, and thus, to the effects of climate change. However, indoor housed livestock, such as pigs and chicken can also be affected by climatic stress (Mikovits et al., 2019; Kamphuis et al., 2020; Tiezzi et al., 2020), at least partly due to imperfect climate control.
Vulnerability to heat stress is influenced by many factors (e.g., genetics, nutrition, life stage), where animals that have high energy demands (i.e., high yielding breeds) are most susceptible (Bernabucci et al., 2010; Rashamol et al., 2019; Saeed et al., 2019; Godde et al., 2021). Furthermore, increased selection pressure for high productivity in livestock is known to negatively affect thermoregulation, the ability of an organism to maintain a steady internal body temperature (Thornton et al., 2021). Changes in the environment often lead to systematic phenotypic differences (e.g., thermoregulatory ability) known as phenotypic plasticity (De Jong and Bijma, 2002). Genotypes exhibiting highly variable phenotypes across environments are termed ‘plastic’, while those genotypes that display low variability across environments are referred to as ‘robust’. Genetic differences in phenotypic plasticity often surface as genotype-by-environment interactions (GxE), revealing that different genotypes respond in different ways to environmental variation. Genotype-by-environment interactions can lead to scaling of genetic effects, where the difference in phenotypic performance between genotypes changes in magnitude according to the environment, or re-ranking of animals in different environments (Lynch and Walsh, 1998; Calus, 2006). Re-ranking is often measured by the genetic correlation between phenotypes of the same trait expressed in different environments, and it has been suggested that genetic correlations below 0.8 are an indication of meaningful GxE (Robertson, 1959; Banos and Smith, 1991; Mulder and Bijma, 2006). As the demand of animals suitable for altered conditions is expected to rise with climate change, GxE is also expected to gain more emphasis in animal breeding in the near future (Strandén et al., 2022).
Several statistical models, including reaction norms, have been proposed to estimate GxE, which can be used to select animals for plastic traits such as robustness, or improved thermoregulation (De Jong and Bijma, 2002). When genotypes are exposed to different environments, and the environments can be described on a continuous scale, reaction norm models are suitable tools to estimate GxE. In the reaction norm models, breeding values and genetic parameters are modelled as a function of a continuous environmental parameter using random regression, which allows for better estimation of GxE effects compared to an arbitrary grouping of environments (Calus, 2006). Information on the thermoregulatory capacity of animals can serve as a valuable addition to current high-throughput phenotyping technologies, which can be used to generate difficult-to-measure, real-time, non-invasive and accurate animal-level phenotypes (Bresolin and Dórea, 2020).
Genotype-by-climate interactions (GxC) are a specific case of GxE, where the environment is described by climatic parameters. In this systematic review, we provide an overview of the effects of temperature, relative humidity, heat stress and cold stress on production-, fertility-, and health-related phenotypes of cattle, swine and poultry through GxC, based on the available scientific literature. The outcomes of this study will shed light on the potential benefits of incorporating longitudinal environmental data, such as temperature and relative humidity, in phenotype prediction models. Specifically, we aim to answer the following questions:
I. Which spatial (e.g. nearby weather station, on farm- or at animal level) and temporal (e.g. hourly, daily, weekly) resolution of climatic data (temperature, relative humidity, indices for heat and cold stress) have been used so far to assess the existence of GxC in the phenotypes of dairy and beef cattle, swine, chicken, and turkeys?
II. Which production-, fertility-, and health-related phenotypes of these farmed animals have been covered?
III. What was the conclusion of the studies on the existence of a relevant GxC for the analyzed production-, fertility-, and health-related phenotypes?
2 Materials and methods
The review process was performed in accordance with the Preferred Reporting Items for Systematic reviews and Meta-Analyses 2020 (PRISMA 2020) statement (Page et al., 2021). The completed PRISMA 2020 checklist for this article is available in Supplementary Table 1.
2.1 Inclusion and exclusion criteria
A study was considered for inclusion if (1) dairy or beef cattle, swine, chicken or turkeys were analyzed in an observational study (where the predictors are not controlled by the researcher) or designed experiment, (2) at least one production-, fertility-, or health-related phenotype was tested for GxC, (3) the existence of GxC was tested directly based on temperature, relative humidity, or climatic indices for heat or cold stress (e.g., temperature-humidity index), (4) genetic effects within a breed or line were investigated, rather than differences between breeds or lines, and (5) it was an original peer-reviewed scientific article with English full-text. Review articles and meta-analyses were excluded from our review. No restrictions were placed on publication date or geographical location. A quality assessment of the included articles is presented in the Supplementary Material 1.
2.2 Search strategy
A systematic literature search was conducted in October 2021, using the same search terms in Scopus and Web of Science. The following terms were used for the search within “Article title, Abstract, Keywords” in Scopus and “Topic” in Web of Science: genotype AND environment* AND interact* AND (temperat* OR heat OR humid* OR cold OR “heat stress” OR “thermal stress” OR “cold stress”) AND (broiler OR hen OR chicken OR chick OR turkey OR poult* OR swine OR pig OR piglet OR boar OR gilt OR barrow OR “sow” OR “sows” OR cattle OR cow OR heifer OR bull OR steer OR calf).
2.3 Screening and data extraction
Assessment of articles for eligibility and data extraction from the full-texts were performed by two reviewers (IF, MS) in parallel. Any discrepancies were discussed among reviewers until a consensus was reached. The steps of the article selection process are shown in detail in Figure 1. Additional papers were identified for inclusion based on the reference lists of the remaining full-texts. A standardized form was used to extract data from the retained articles, including authorship, year of publication, country of data origin, type of study (experimental/observational), number of farms and animals, animal species, breed, environmental data collection method (weather station/on-farm sensor) and frequency (e.g. daily), phenotypes investigated, metrics used (e.g. genetic correlation of a trait between different climatic environments <0.8) and conclusion on GxC (yes/no/inconclusive).
2.4 Risk of bias assessment
Using the relevant domains of the ROBIS framework (Whiting et al., 2016), a risk of bias assessment of the systematic review protocol was performed and provided in the Supplementary Material 2.
3 Results and discussion
3.1 Publications
We identified 400 scientific articles in the two databases (181 in Scopus and 219 in Web of Science), of which 141 records were duplicates and removed consequently. After screening the remaining 259 papers, 177 were excluded based on title, article information or abstract content. In total, the full-text version of 82 papers was reviewed, of which 42 were included in the systematic review. Another four records were identified based on the evaluation of the reference lists of these 42 papers, and the subsequent screening and assessment, finally adding up to a total of 46 peer-reviewed scientific papers analyzed in our review (Supplementary Table 2).
The main reason for excluding studies based on their full-texts was related to the lack of direct measures of the effect of the environmental variable (temperature, relative humidity, heat or cold stress) through GxC on the phenotypes (Yalcin et al., 1997; De Greef et al., 2001; Moreira et al., 2019; Chen et al., 2021). Studies that used grouping or clustering to assess GxC also missed the direct relation between phenotype and environmental variable, and were excluded for that reason. Examples include Chen et al. (2021), who used the average performance of contemporary group as an environmental gradient in the reaction norm analyses, or Neser et al. (2008), who used temperature as one of the parameters for clustering animals. In addition, studies often compared the adaptation of different breeds to challenging environments (Melesse et al., 2013; Kim et al., 2017; Abioja et al., 2020) or compared their performance in different regions (e.g. Fennewald et al., 2018) or seasons (e.g. Bradford et al., 2016a), rather than evaluating the impact of GxC on phenotypes within breeds.
Based on the 46 papers retained for detailed analysis, a steep increase in GxC studies was observed from the 2000s, and especially after 2015 (Supplementary Figure 1). Since 2015, the number of such studies has more than doubled. The increasing number of GxC studies since the 2000s is in line with the rapidly increasing number of peer-reviewed papers on the impact of climate change on the livestock sector, as well as the adaptation towards climate change (Escarcha et al., 2018), emphasizing the increasing interest in the effect of climate and environmental parameters on animal performance.
3.2 Geographical coverage
Most of the 46 included studies originate from the American continent, with Brazil (n = 14) and the USA (n = 10) having published the largest number of papers. Combined, these two countries published 52.2% of the overall number of studies presented in our review. The third largest number of papers was published by Germany (n = 7), followed by Australia (n = 5) at the fourth place (Figure 2). The highly uneven distribution of published GxC papers shows that fewer studies are available from Asia, Africa, Europe, and also from South America (outside of Brazil). Using the Köppen-Geiger climate classification (Beck et al., 2018), more insight can be gained on the climatic ranges included in our review. Based on the listed countries, we generally cover the four main climatic groups relevant for livestock production: tropical, arid, temperate, and continental. However, more specific information on the study locations is often lacking, so it is not always possible to assess if perhaps only certain areas of a country (and thus climatic ranges) may have been included. For future studies it is advised to report detailed information on the locations covered.
3.3 Species and breed
The main characteristics of the reviewed studies are summarized in Table 1. Despite our intention to also capture studies on turkeys, no study on this species qualified for inclusion. More than three-quarters (n = 36; 78.3%) of all GxC studies was published on cattle. The steep increase in the number of GxC studies since 2015 is also largely attributable to the rapid increase of studies on cattle, but after 2015 studies on pigs have also started to appear (Figure 3). Monogastric animals are very sensitive to the thermal environment (Costantino et al., 2021), therefore, they are often raised in climate controlled production systems, at high stocking densities (Schauberger et al., 2019). Previously, it has been assumed that confined livestock systems were less vulnerable to global warming compared to outdoor housing systems (Rötter and van de Geijn, 1999), which might explain the small number of GxC papers for monogastrics. However, using data from growing-finishing pigs, Mikovits et al. (2019) concluded that the indoor climate can be more vulnerable to the effects of global warming than the outdoor situation, putting animals inside confined livestock buildings at risk of more severe heat stress. Indoor climate does not only depend on the meteorological conditions, but also on the heat production of the animals, increased indoor humidity, the thermal properties of the building, and ventilation. Tiezzi et al. (2020) found significant GxC in indoor-housed pigs by using climatic data from weather stations. They noted that unless farms implemented highly efficient climate control methods, the welfare and performance of pigs could be adversely affected by heat stress.
The large number of cattle studies enabled us to further analyze the published articles by cattle breed purpose. These were dominated by dairy cattle studies (26/36; 72.2%), which started in the early 2000s, followed by the appearance of beef cattle studies more than 10 years later, and more recently, dual-purpose cattle have also been studied (Supplementary Figure 2).
The genetic variability for heat tolerance is known to differ considerably between species, breeds, and individuals (Renaudeau et al., 2012; Tesema et al., 2019). This means that any measure of the genetic variability in thermotolerance (measured as GxC), may be influenced by the type of breed included in the analysis. For cattle, 11 different breeds as well as crossbreds were studied, although the majority of these papers focused on Holsteins (22/36; 61.1%). Chicken studies mostly focused either on commercial lines or multiple different lines (e.g. dwarf and normal layers of light and medium heavy lines), whereas two studies measured specifically on Hubbard White Mountain chickens. Pigs were predominately crossbreds, or belonged to multiple different lines, but specifically the Large White breed was studied in one paper.
Regardless of species, those breeds that have a long history in strong selection for production traits (e.g. Holstein cattle) are at substantial risk of decreased thermotolerance, due to the negative genetic association between heat tolerance and production traits (Nguyen et al., 2016; Osei-Amponsah et al., 2019). The higher susceptibility to heat stress in such breeds can be counteracted by explicitly selecting for thermotolerance, and by properly considering GxC in the breeding goal (Carabaño et al., 2017).
3.4 Study type and design
Most GxC studies are observational, including all papers on cattle and the majority of papers on pigs (Table 1). Based on the studies included in our review, only experimental studies have been conducted on chickens. Study sizes vary widely both in terms of farm and animal numbers. Studies on cattle included by far the largest numbers of farms, whereas each chicken study (experiments) used data from one farm only. Information on the number of farms was lacking in 22 studies (47.8%). In terms of animal numbers, cattle and pig studies were comparable in magnitude. The largest study included in our review was Zwald et al. (2003), who analyzed the test day milk records of more than 16.4 million Holstein cows from nearly 234 thousand herds in 17 countries.
Most observational GxC studies (n = 35; 87.5%) applied random regression or reaction norm models in their analyses. All observational studies on pigs and 83.3% of the studies on cattle used reaction norms. The remaining observational studies typically categorized records by the climatic parameter (e.g., into quintiles), and performed a two-trait (e.g., Espasandin et al., 2013; Halli et al., 2021) or multiple-trait (Zwald et al., 2003) analysis. In experimental studies, the statistical model used is largely determined by the experimental design. Environmental temperature was mostly fitted as a fixed factor variable, e.g. in mixed models (Loyau et al., 2016) or ANOVA (Adams and Rogler, 1968; Deeb and Cahaner, 2002), but separate mixed models were fitted for each temperature treatment group in Deeb et al. (2002), and subsequently variances and least square means of sire families were compared between temperature treatment groups.
3.5 Climatic parameters
There are many parameters that can be used to represent aspects of the climate, and subsequently to quantify their effect on an animal’s performance. Studies eligible for inclusion typically used a statistic (e.g., daily mean) of either temperature or of its combination with relative humidity as temperature-humidity index (THI), to characterize thermal conditions. For instance, Negri et al. (2021) defined diurnal temperature variation as the difference between the daily maximum and minimum temperatures, and used this climatic parameter in their models. We observed two main approaches to characterize thermal conditions using THI: either a statistic of THI was assigned to a period of pre-defined duration (e.g., mean THI over the last 60 days of gestation in Menéndez-Buxadera et al., 2020; sum of THI units from birth to 205 days in Santana et al., 2016), or a heat stress limit was pre-defined and a duration statistic was assigned to it (e.g. number of days with daily mean THI ≥60 in Halli et al., 2021). Nevertheless, there are more physical environmental factors that may affect cold or thermal load. In their study on cold stress effects on beef cattle, Toghiani et al. (2020) calculated a Comprehensive Climate Index (proposed by Mader et al., 2010) combining temperature, relative humidity, wind speed, and solar radiation into a single index. The summer heat load index used by Bryant et al. (2007) also encompassed temperature, relative humidity, wind speed, and solar radiation. Studies measuring the heat stress effect in outdoor systems also found closer correlations of heat stress with climate parameters if the effect of solar radiation was added (Galán et al., 2018).
Overall, 87.0% of the studies (n = 40) obtained climatic information from weather stations, and in only 13.0% (n = 6) of the studies, this information originated directly from the farm. We found a complete distinction in the source of climatic data by study type: all observational studies used weather station data, whereas climatic data were collected directly on the farm in all experimental studies. In the latter case, the temperature was often programmed by the researchers, as experiments were often conducted in climate-controlled barns or chambers (n = 4). Records collected from weather stations can be used as a surrogate for on-farm climatic conditions, used to assess the impact of heat stress on livestock performance (Campos et al., 2022). However, distances between farms and weather stations may differ considerably between regions and countries. In general terms, the further away a weather station is, the less likely the it will accurately represent the weather conditions on a farm (Campos et al., 2022). For example, in a study performed in Germany (Kipp et al., 2021), the distance between dairy farms and weather stations ranged between 8 and 36 kilometers. However, for many of the included studies that used climatic data from weather stations, the distance between the farm and the weather station was not reported (Bohmanova et al., 2008; Bohlouli et al., 2013; Braz et al., 2021) or large (e.g., up to 144 km in Bradford et al., 2016b). Based on these findings, it might be possible to improve the quality of climatic data in GxC studies via on-farm sensors. For example, the thermal environment inside the barn depends not only on the outdoor climate, but also on the animals as sources of heat and humidity, the thermal properties of the building, and the climate control (Mikovits et al., 2019). Tiezzi et al. (2020) also suggested that pig breeders should record indoor environmental conditions for a more accurate assessment of heat stress. On the one hand, climatic parameters would be measured closer to the animals by on-farm sensors (either inside or outside the barn), better reflecting their immediate environment. On the other hand, a certain degree of standardization in using on-farm climatic sensors might be required, because sensor placement and accuracy could influence the resulting data, among other things.
The frequency of climatic data collection was further analyzed within the observational studies (Supplementary Figure 3). In most of these studies, climatic data were collected at least once per day (n = 33; 82.5%), whereas some authors used monthly data (n = 2; 5.0%) or even 10-year averages (n = 2; 5.0%). More frequent measurements provide more information, and some climatic stress parameters require multiple measurements per day. For example, in the study of Toghiani et al. (2020) on the GxC resulting from cold stress in beef cattle, first the Comprehensive Climate Index was computed for every half hour from the weather data, which was then used to calculate the daily cold load score, reflecting the daily sum of cold stress exposure of an animal.
In addition to the diversity in approaches to quantifying thermal load, the effect of heat- or cold stress may be masked by climate control measures (e.g. shade, fans, heating or cooling systems), and details of the application of these measures are often not recorded (Ravagnolo et al., 2000). If such measures for heat abatement are in place on a farm, the effect of heat stress via GxC might be underestimated. It is also important to realize that the climatic effect on the animals’ performance not only reflects the effect of the weather on the test days but also the weather preceding the test days (Ravagnolo et al., 2000; Kamphuis et al., 2020). Hence, it is important to consider management practices, and to consider the effect of the weather across different time periods, to allow proper interpretation of the relation of the climatic indices to the animals’ performance.
3.6 Analyzed traits and presence of GxC
The traits covered by the GxC studies were extracted and summarized. Altogether, 75 different traits were distinguished in the studies included in our review. Reflecting on one of our research questions, these traits were classified into three trait groups (production, fertility, or health). The dominance of production traits in the GxC studies was clear, as in each species at least two-thirds of the papers studied production traits (Figure 4). As some papers covered more than one trait group (e.g. Loyau et al., 2016), the number of studies presented in Figure 4 sum up to more than the actual number of papers included in our review. Few studies are available on the GxC effects on fertility and health traits. Even though more research effort has been made in this direction since 2010, the number of studies on fertility and health traits lags far behind compared to those focusing on production (Supplementary Figure 4). There are several plausible reasons that may explain the strong emphasis on production traits. Production traits are economically important, and have been dominating breeding goals until about two decades ago (Neeteson-van Nieuwenhoven et al., 2013; Miglior et al., 2017). Due to this, and the relative ease of recording, production traits have been recorded for a longer time and more extensively than e.g. health traits. Moreover, the impact of climatic events is more apparent on production compared to fertility, where it may take time to see any effect (Cammack et al., 2009; Miglior et al., 2017). Finally, both higher frequency of recording, as well as generally having higher heritabilities compared to fertility and health traits (Cammack et al., 2009; Egger-Danner et al., 2015; Miglior et al., 2017), makes that GxC studies for production traits are typically more powerful, and therefore also more conclusive.
Trait groups were further divided into trait subgroups, in order to get a more detailed picture of the research directions (Figure 5). Production traits included milk characteristics, growth, carcass, and feed efficiency, among others. Genotype-by-climate interaction studies on dairy cattle have mostly focused on milk characteristics, udder health, and female fertility. Growth traits were often studied in beef cattle, broilers, and pigs, whereas feed efficiency and carcass traits have sparked particular interest in broiler and pig GxC studies, respectively. There has been more focus on selection for feed efficiency in pigs and chickens compared to cattle, where phenotyping is more difficult to implement on a larger scale (Ouweltjes et al., 2022).
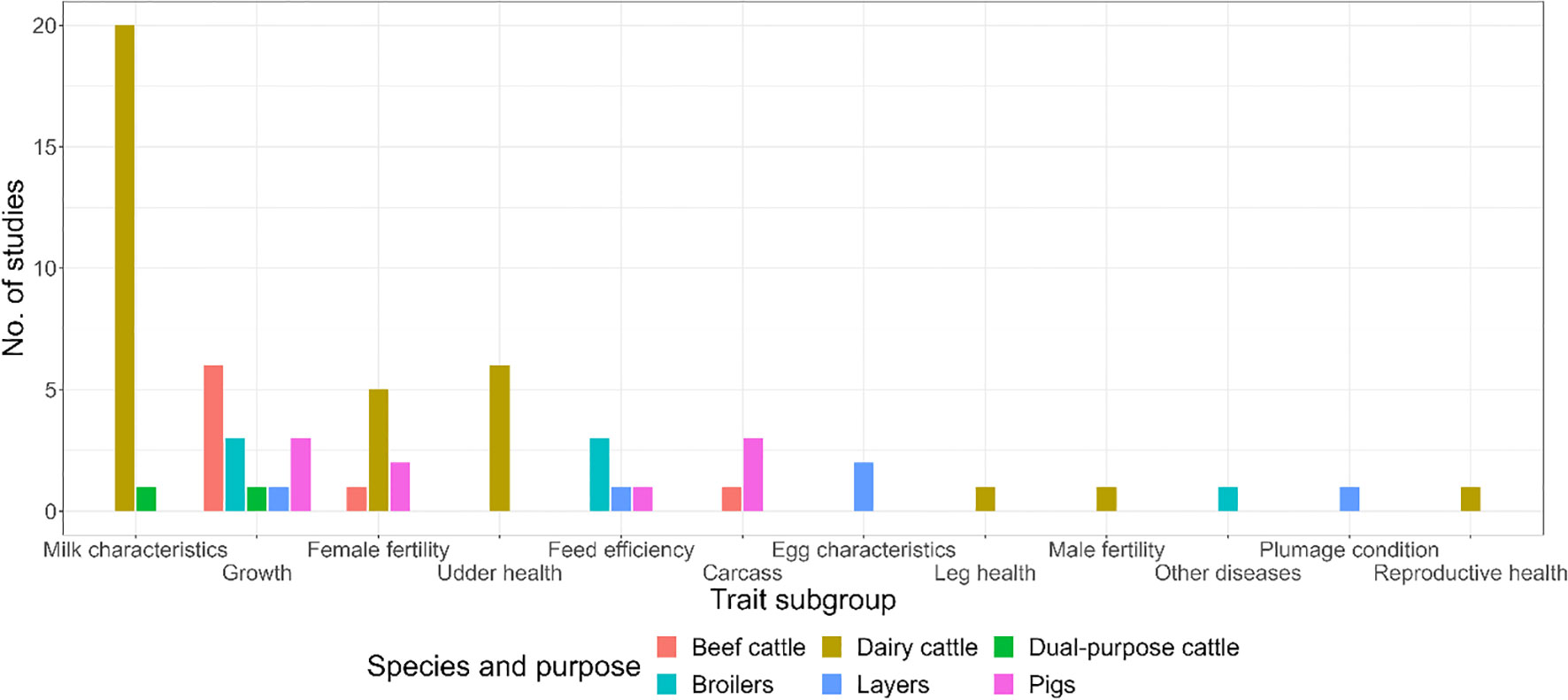
Figure 5 The number of genotype-by-climate interaction studies by trait subgroups, species and breed purpose.
In total, we summarized 139 study outcomes from the 75 traits considered in our review (Supplementary Figures 5-7). For 75 of the study outcomes (54.0%), authors concluded that the trait was affected by GxC in their study (Table 1). Authors reported no evidence for GxC in 62 outcomes (44.6%), whereas in 2 outcomes (1.4%) study results were inconclusive. For those trait subgroups that had been studied in at least 4 different papers (no such subgroup was found in pigs), we summarized the number of outcomes and the authors’ conclusions on the presence of GxC (Figure 6). Genotype-by-climate interaction was confirmed in less than half of the outcomes (41.0%) for milk characteristics. On the other hand, the presence of GxC was reported in more than half of the outcomes for cattle growth (64.3%), female fertility (66.7%), and udder health (71.4%). In chickens, GxC was found in 80.0% and 62.5% of the outcomes for growth and feed efficiency, respectively.
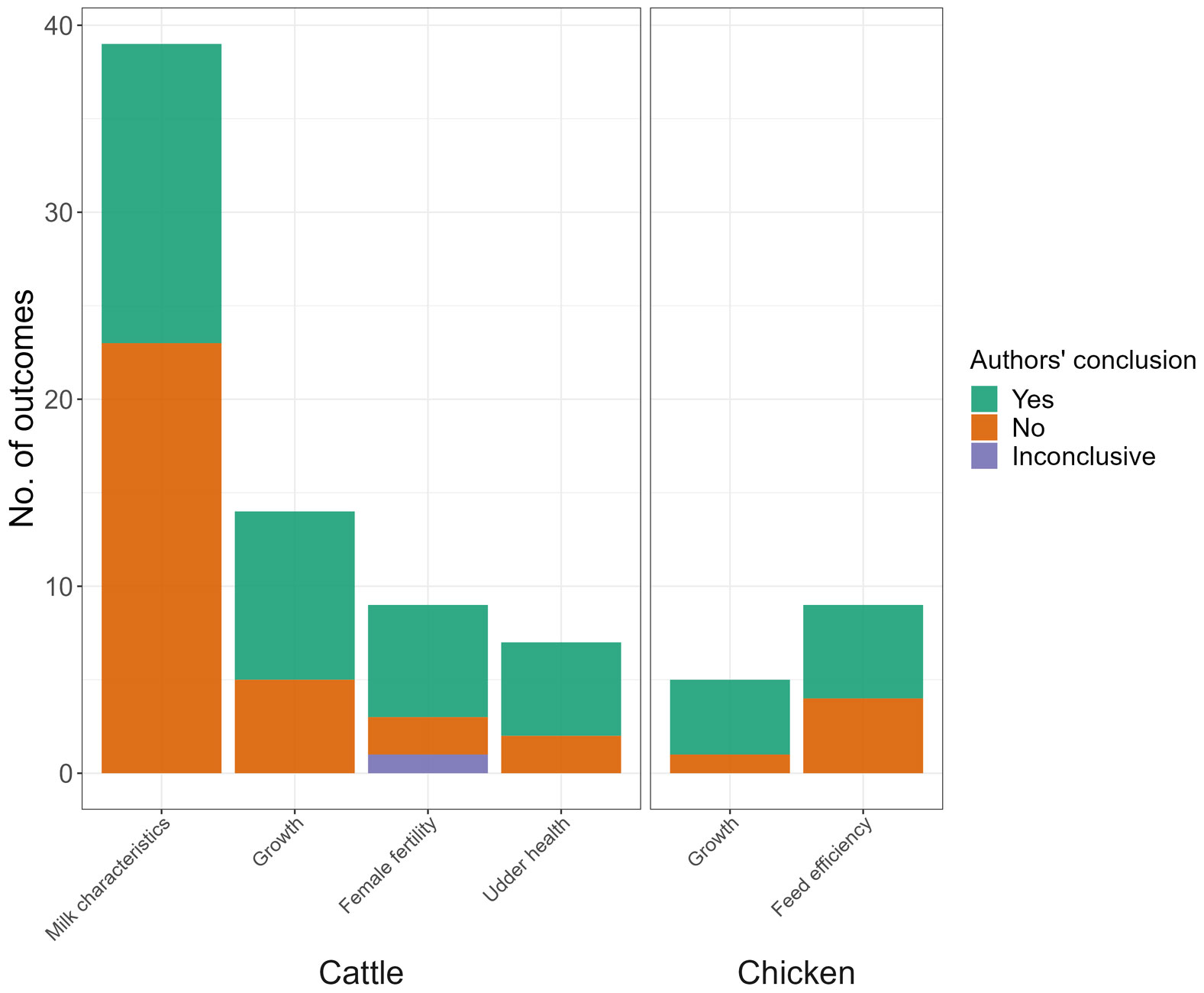
Figure 6 Presence of genotype-by-climate interaction in the most studied trait subgroups, based on the authors’ conclusions.
Robertson (1959) suggested that the genetic correlation should fall below 0.8 to have “biological or agricultural importance”, and this threshold has later been substantiated with results from simulation studies and is widely adopted in the literature to conclude on the existence of GxE. Using a simulated dairy cattle breeding scheme for two countries, Banos and Smith (1991) showed that with genetic correlations between countries being less than 0.8, animals selected as parent in one country were unlikely selected as parent in the other country. Similarly, Mulder and Bijma (2006) showed that with genetic correlations greater than 0.8 to 0.9, exchange between dairy cattle breeding schemes was beneficial, while for genetic correlations below 0.8 this was only the case for relatively small breeding programs that benefitted from larger ones. Numerical values of genetic correlations reported in the reviewed papers are summarized in Supplementary Figure 8. The point estimates of the genetic correlation vary widely within each trait group in all the species. Overall, the genetic correlation was reported for 87 outcomes across all species, out of which 42 (48.3%) were smaller than 0.8. The number and percentage of genetic correlations below 0.8 was 28 (53.8%) in cattle, 7 (26.9%) in chickens, and 7 (77.8%) in pigs, respectively. Numerous papers failed to report any measure of spread (e.g. the standard error of the correlation coefficient), making it difficult to summarize these figures in a meta-analysis.
In summary, a wide range of traits has been studied for GxC, and the number of traits is expected to further increase, both due to continuous expansion of animal breeding goals, the more widespread adoption of advanced phenotyping tools, and increased frequency of recording phenotypes and climatic variables. The majority of papers found a significant effect of climatic variables on the phenotypes of cattle, swine and chickens through GxC. Even though it is possible that studies having found GxC might be more likely to be published, potentially leading to publication bias, such bias is less likely in the context of GxC, as the lack of interaction is just as relevant for the practice as its existence.
3.7 Recommendations for researchers
Based on our findings, we make the following recommendations for researchers on conducting and reporting GxC studies:
I. We encourage researchers to perform further studies on GxC in livestock other than dairy cattle, especially swine and poultry.
II. The number of farms, as a basic information about the collected data, should be reported.
III. When reporting the materials, information on farm management should be provided, if available, especially on heat abatement or climate control.
IV. If climatic data from weather stations are used, descriptive statistics (mean, median, minimum, maximum) of the distance between farms and the weather stations should be provided.
V. Furthermore, we encourage the use of climatic data collected from properly calibrated on-farm sensors, to better reflect the immediate environment of the animals.
VI. The decision criteria used to conclude on the existence of GxC should be explicitly mentioned in the materials and methods.
VII. When using reaction norm models, it is advised to report genetic correlations rather between the e.g. 5th and 95th quantile rather than between the extreme values of the climatic parameters, as the estimated accuracy between extremes may be inaccurate due to the limited amount of data. The value of climatic parameters at which the lowest genetic correlation is found should be reported, along with the value of the lowest genetic correlation and the corresponding measure of spread (e.g. SE or CI).
VIII. Limitations of the data, experimental set-up, or methodology, and the potential sources of biases should be discussed.
3.8 Recommendations for practitioners
Based on our findings, and in consultation with industry experts, we make the following recommendations for practitioners:
I. The trait to investigate should be measured frequently (e.g., daily or weekly), if the nature of the trait allows. The resulting time-series data can be analyzed for the effect of short-duration heatwaves.
II. If GxC exists, the need for differentiation in breeding goals between environments should be investigated. This should be considered by the parties responsible for implementing the breeding goal, which, depending on the species could be either breeding companies, or a studbook, or both.
III. Tools should be made available to support producers when they face GxC. This includes, among other things, the education of producers in the interpretation of environment-specific breeding goals and possibly new breeding values (which are more difficult to understand), and guidance on how to select for improved heat tolerance.
Efforts to study the effects of GxC have strongly increased in the previous decade, but these efforts have mostly been focused on production traits regardless of species. Limited knowledge exists about GxC effects on fertility and health traits of cattle, and all traits of poultry and pigs. Knowledge on GxC on production, fertility, and health traits of turkeys is currently lacking. The majority of papers still estimated a significant effect of temperature, relative humidity and THI on the traits of cattle, swine and chickens through GxC. More standardization is needed to estimate the impact of climate on the performance of animals more accurately, especially on reporting the methodology and results of GxC studies. Detailed climatic data should be collected and used to assess the presence of GxC, to potentially improve the prediction of phenotypes and breeding value estimates, in indoor and outdoor production systems, as well.
Data availability statement
The original contributions presented in the study are included in the article/Supplementary Material. Further inquiries can be directed to the corresponding author.
Author contributions
IF: Conceptualization, Data curation, Formal Analysis, Methodology, Visualization, Writing – original draft, Writing – review & editing. MS: Conceptualization, Data curation, Formal Analysis, Methodology, Writing – original draft, Writing – review & editing. MC: Conceptualization, Project administration, Supervision, Writing – original draft, Writing – review & editing. CK: Conceptualization, Project administration, Supervision, Writing – original draft, Writing – review & editing.
Funding
The author(s) declare financial support was received for the research, authorship, and/or publication of this article. This work was supported by the Dutch Ministry of Economic Affairs (TKI Agri & Food project LWV20054) and the Breed4Food partners Cobb Europe, CRV, Hendrix Genetics and Topigs Norsvin.
Acknowledgments
The authors would like to thank the Breed4Food partners Cobb Europe, CRV, Hendrix Genetics and Topigs Norsvin for providing valuable feedback during the work. Furthermore, we thank Mathijs van Pelt for his valuable recommendations from a breeding company perspective.
Conflict of interest
The authors declare that the research was conducted in the absence of any commercial or financial relationships that could be construed as a potential conflict of interest.
The authors declare that this study received funding from Cobb Europe, CRV, Hendrix Genetics and Topigs Norsvin. The funders had the following involvement in the study: interpretation of data, reviewing this article, and a decision to submit it for publication.
Publisher’s note
All claims expressed in this article are solely those of the authors and do not necessarily represent those of their affiliated organizations, or those of the publisher, the editors and the reviewers. Any product that may be evaluated in this article, or claim that may be made by its manufacturer, is not guaranteed or endorsed by the publisher.
Supplementary material
The Supplementary Material for this article can be found online at: https://www.frontiersin.org/articles/10.3389/fanim.2023.1324830/full#supplementary-material
References
Abioja M. O., Omotara O. P., Iyasere O. S., Abiona J. A., Egbeyale L. T., Akinjute O. F. (2020). Comparative study of adaptation in three chicken genotypes under humid tropical conditions of Nigeria. J. Anim. Physiol. Anim. Nutr. (Berl.). 104, 1401–1409. doi: 10.1111/jpn.13381
Adams R., Rogler J. (1968). The effects of environmental temperature on the protein requirements and response to energy in slow and fast-growing chicks. Poult. Sci. 47, 579–586. doi: 10.3382/ps.0470579
Banos G., Smith C. (1991). Selecting bulls across countries to maximize genetic improvement in dairy cattle. J. Anim. Breed. Genet. 108, 174–181. doi: 10.1111/j.1439-0388.1991.tb00172.x
Beck H. E., Zimmermann N. E., McVicar T. R., Vergopolan N., Berg A., Wood E. F. (2018). Present and future Köppen-Geiger climate classification maps at 1-km resolution. Sci. Data 5, 1–12. doi: 10.1038/sdata.2018.214
Berckmans D. (2017). General introduction to precision livestock farming. Anim. Front. 7, 6–11. doi: 10.2527/af.2017.0102
Bernabucci U., Lacetera N., Baumgard L. H., Rhoads R. P., Ronchi B., Nardone A. (2010). Metabolic and hormonal acclimation to heat stress in domesticated ruminants. Animal 4, 1167–1183. doi: 10.1017/S175173111000090X
Bohlouli M., Shodja J., Alijani S., Eghbal A. (2013). The relationship between temperature-humidity index and test-day milk yield of Iranian Holstein dairy cattle using random regression model. Livest. Sci. 157, 414–420. doi: 10.1016/j.livsci.2013.09.005
Bohmanova J., Misztal I., Tsuruta S., Norman H. D., Lawlor T. J. (2008). Short communication: Genotype by environment interaction due to heat stress. J. Dairy. Sci. 91, 840–846. doi: 10.3168/jds.2006-142
Bradford H. L., Fragomeni B. O., Bertrand J. K., Lourenco D. A. L., Misztal I. (2016a). Regional and seasonal analyses of weights in growing Angus cattle. J. Anim. Sci. 94, 4369–4375. doi: 10.2527/jas.2016-0683
Bradford H. L., Fragomeni B. O., Bertrand J. K., Lourenco D. A. L., Misztal I. (2016b). Genetic evaluations for growth heat tolerance in Angus cattle. J. Anim. Sci. 94, 4143–4150. doi: 10.2527/jas.2016-0707
Braz C. U., Rowan T. N., Schnabel R. D., Decker J. E. (2021). Genome-wide association analyses identify genotype-by-environment interactions of growth traits in Simmental cattle. Sci. Rep-UK. 11, 13335. doi: 10.1038/s41598-021-92455-x
Bresolin T., Dórea J. R. R. (2020). Infrared spectrometry as a high-throughput phenotyping technology to predict complex traits in livestock systems. Front. Genet. 11, 923. doi: 10.3389/fgene.2020.00923
Bryant J. R., López-Villalobos N., Pryce J. E., Holmes C. W., Johnson D. L., Garrick D. J. (2007). Environmental sensitivity in New Zealand dairy cattle. J. Dairy. Sci. 90, 1538–1547. doi: 10.3168/jds.S0022-0302(07)71639-9
Calus M. P. L. (2006). Estimation of genotype × environment interaction for yield, health, and fertility in dairy cattle, PhD thesis, (Wageningen, Netherlands: Wageningen University).
Cammack K. M., Thomas M. G., Enns R. M. (2009). Review: Reproductive traits and their heritabilities in beef cattle. Prof. Anim. Sci. 25, 517–528. doi: 10.15232/S1080-7446(15)30753-1
Campos I. L., Chud T. C. S., Oliveira H. R., Baes C. F., Cánovas A., Schenkel F. S. (2022). Using publicly available weather station data to investigate the effects of heat stress on milk production traits in Canadian Holstein cattle. Can. J. Anim. Sci. 102, 368–381. doi: 10.1139/cjas-2021-0088
Carabaño M. J., Ramón M., Díaz C., Molina A., Pérez-Guzmán M. D., Serradilla J. M. (2017). Breeding for resilience to heat stress effects in dairy ruminants. A comprehensive review. J. Anim. Sci. 95, 1813–1826. doi: 10.2527/jas.2016.1114
Chen C. S., Chen W. C. (2019). Research and development of automatic monitoring system for livestock farms. Appl. Sci. 9, 1132. doi: 10.3390/app9061132
Chen S. Y., Freitas P. H. F., Oliveira H. R., Lázaro S. F., Huang Y. J., Howard J. T., et al. (2021). Genotype-by-environment interactions for reproduction, body composition, and growth traits in maternal-line pigs based on single-step genomic reaction norms. Genet. Sel. Evol. 53, 51. doi: 10.1186/s12711-021-00645-y
Costantino A., Fabrizio E., Calvet S. (2021). The role of climate control in monogastric animal farming: The effects on animal welfare, air emissions, productivity, health, and energy use. Appl. Sci. (Basel). 11, 9549. doi: 10.3390/app11209549
Deeb N., Cahaner A. (2002). Genotype-by-environment interaction with broiler genotypes differing in growth rate. 3. Growth rate and water consumption of broiler progeny from weight-selected versus nonselected parents under normal and high ambient temperatures. Poult. Sci. 81, 293–301. doi: 10.1093/ps/81.3.293
Deeb N., Shlosberg A., Cahaner A. (2002). Genotype-by-environment interaction with broiler genotypes differing in growth rate. 4. Association between responses to heat stress and to cold-induced ascites. Poult. Sci. 81, 1454–1462. doi: 10.1093/ps/81.10.1454
De Greef K. H., Janss L. L. G., Vereijken A. L. J., Pit R., Gerritsen C. L. M. (2001). Disease-induced variability of genetic correlations: ascites in broilers as a case study. J. Anim. Sci. 79, 1723–1733. doi: 10.2527/2001.7971723x
De Jong G., Bijma P. (2002). Selection and phenotypic plasticity in evolutionary biology and animal breeding. Livest. Prod. Sci. 78, 195–214. doi: 10.1016/S0301-6226(02)00096-9
Egger-Danner C., Cole J. B., Pryce J. E., Gengler N., Heringstad B., Bradley A., et al. (2015). Invited review: Overview of new traits and phenotyping strategies in dairy cattle with a focus on functional traits. Animal 9, 191–207. doi: 10.1017/S1751731114002614
Escarcha J. F., Lassa J. A., Zander K. K. (2018). Livestock under climate change: A systematic review of impacts and adaptation. Climate 6, 54. doi: 10.3390/cli6030054
Espasandin A. C., Urioste J. I., Naya H., Alencar M. M. (2013). Genotype × production environment interaction for weaning weight in Angus populations of Brazil and Uruguay. Livest. Sci. 151, 264–270. doi: 10.1016/j.livsci.2012.11.015
Fennewald D. J., Weaber R. L., Lamberson W. R. (2018). Genotype by environment interaction for stayability of Red Angus in the United States. J. Anim. Sci. 96, 422. doi: 10.1093/jas/skx080
Fournel S., Laberge B., Rousseau A. N. (2017). Rethinking environment control strategy of confined animal housing systems through precision livestock farming. Biosyst. Eng. 155, 96–123. doi: 10.1016/j.biosystemseng.2016.12.005
Galán E., Llonch P., Villagrá A., Levit H., Pinto S., Del Prado A. (2018). A systematic review of non-productivity-related animal-based indicators of heat stress resilience in dairy cattle. PloS One 13 (11), e0206520. doi: 10.1371/journal.pone.0206520
Godde C. M., Mason-D’Croz D., Mayberry D. E., Thornton P. K., Herrero M. (2021). Impacts of climate change on the livestock food supply chain; a review of the evidence. Glob. Food Secur. 28, 100488. doi: 10.1016/j.gfs.2020.100488
Halli K., Brügemann K., Bohlouli M., Yin T., König S. (2021). Heat stress during late pregnancy and postpartum influences genetic parameter estimates for birth weight and weight gain in dual-purpose cattle offspring generations. J. Anim. Sci. 99, 1–12. doi: 10.1093/jas/skab106
IPCC (2013). Climate change 2013: the physical science basis. Contribution of Working Group I to the fifth assessment report of the Intergovernmental Panel on Climate Change (Cambridge, United Kingdom and New York, NY, USA: Cambridge University Press).
Johnson J. S. (2018). Heat stress: Impact on livestock well-being and productivity and mitigation strategies to alleviate the negative effects. Anim. Prod. Sci. 58, 1404–1413. doi: 10.1071/AN17725
Kamphuis C., Duenk P., Veerkamp R. F., Visser B., Singh G., Nigsch A., et al. (2020). Machine learning to further improve the decision which boar ejaculates to process into artificial insemination doses. Theriogenology 144, 112–121. doi: 10.1016/j.theriogenology.2019.12.017
Kim J., Hanotte O., Mwai O. A., Dessie T., Bashir S. F., Diallo B., et al. (2017). The genome landscape of indigenous African cattle. Genome Biol. 18, 34. doi: 10.1186/s13059-017-1153-y
Kipp C., Brügemann K., Yin T., Halli K., König S. (2021). Genotype by heat stress interactions for production and functional traits in dairy cows from an across-generation perspective. J. Dairy. Sci. 104, 10029–10039. doi: 10.3168/jds.2021-20241
Koltes J. E., Cole J. B., Clemmens R., Dilger R. N., Kramer L. M., Lunney J. K., et al. (2019). A vision for development and utilization of high-throughput phenotyping and big data analytics in livestock. Front. Genet. 10, 1197. doi: 10.3389/fgene.2019.01197
Loyau T., Zerjal T., Rodenburg T. B., Fablet J., Tixier-Boichard M., Pinard-Van Der Laan M. H., et al. (2016). Heritability of body surface temperature in hens estimated by infrared thermography at normal or hot temperatures and genetic correlations with egg and feather quality. Animal 10, 1594–1601. doi: 10.1017/S1751731116000616
Lynch M., Walsh B. (1998). Genetics and analysis of quantitative traits, 1st (Sunderland: Sinauer Associates).
Mader T. L., Johnson L. J., Gaughan J. B. (2010). A comprehensive index for assessing environmental stress in animals. J. Anim. Sci. 88 (6), 2153–2165. doi: 10.2527/jas.2009-2586
Melesse A., Maak S., Pingel H., von Lengerken G. (2013). Assessing the thermo-tolerance potentials of five commercial layer chicken genotypes under long-term heat stress environment as measured by their performance traits. Acta Agric. Slov. 102, 29–38. doi: 10.2478/acas-2013-0030
Menéndez-Buxadera A., Pereira R. J., El Faro L., Santana M. L. (2020). Genotype by environment interaction due to heat stress during gestation and postpartum for milk production of Holstein cattle. Animal 14, 2014–2022. doi: 10.1017/S1751731120001068
Miglior F., Fleming A., Malchiodi F., Brito L. F., Martin P., Baes C. F. (2017). A 100-year review: Identification and genetic selection of economically important traits in dairy cattle. J. Dairy. Sci. 100, 10251–10271. doi: 10.3168/jds.2017-12968
Mikovits C., Zollitsch W., Hörtenhuber S. J., Baumgartner J., Niebuhr K., Piringer M., et al. (2019). Impacts of global warming on confined livestock systems for growing-fattening pigs: simulation of heat stress for 1981 to 2017 in Central Europe. Int. J. Biometeorol. 63, 221–230. doi: 10.1007/s00484-018-01655-0
Moreira R. P., Pinto L. F. B., Valloto A. A., Pedrosa V. B. (2019). Evaluation of genotype by environment interactions on milk production traits of Holstein cows in southern Brazil. Asian-Austral. J. Anim. 32, 459. doi: 10.5713/ajas.18.0174
Mulder H. A., Bijma P. (2006). Benefits of cooperation between breeding programs in the presence of genotype by environment interaction. J. Dairy. Sci. 89, 1727–1739. doi: 10.3168/jds.S0022-0302(06)72241-X
Nardone A., Ronchi B., Lacetera N., Bernabucci U. (2006). Climatic effects on productive traits in livestock. Vet. Res. Commun. 30, 75–81. doi: 10.1007/s11259-006-0016-x
Neeteson-van Nieuwenhoven A. M., Knap P., Avendaño S. (2013). The role of sustainable commercial pig and poultry breeding for food security. Anim. Front. 3, 52–57. doi: 10.2527/af.2013-0008
Negri R., Daltro D., dos S., Cobuci J. A. (2021). Heat stress effects on somatic cell score of Holstein cattle in a tropical environment. Livest. Sci. 247, 104480. doi: 10.1016/j.livsci.2021.104480
Neser F. W. C., Erasmus G. J., Scholtz M. M. (2008). The use of a cluster analysis in across-herd genetic evaluation for beef cattle. S. Afr. J. Anim. Sci. 38, 51–57. doi: 10.4314/sajas.v38i1.4109
Nguyen T. T. T., Bowman P. J., Haile-Mariam M., Pryce J. E., Hayes B. J. (2016). Genomic selection for tolerance to heat stress in Australian dairy cattle. J. Dairy. Sci. 99, 2849–2862. doi: 10.3168/jds.2015-9685
Osei-Amponsah R., Chauhan S. S., Leury B. J., Cheng L., Cullen B., Clarke I. J., et al. (2019). Genetic selection for thermotolerance in ruminants. Animals 9, 948. doi: 10.3390/ani9110948
Ouweltjes W., Veerkamp R., van Burgsteden G., van der Linde R., de Jong G., van Knegsel A., et al. (2022). Correlations of feed intake predicted with milk infrared spectra and breeding values in the Dutch Holstein population. J. Dairy. Sci. 105, 5271–5282. doi: 10.3168/jds.2021-21579
Page M. J., McKenzie J. E., Bossuyt P. M., Boutron I., Hoffmann T. C., Mulrow C. D., et al. (2021). The PRISMA 2020 statement: An updated guideline for reporting systematic reviews. BMJ 372, 71. doi: 10.1136/bmj.n71
Rashamol V. P., Sejian V., Pragna P., Lees A. M., Bagath M., Krishnan G., et al. (2019). Prediction models, assessment methodologies and biotechnological tools to quantify heat stress response in ruminant livestock. Int. J. Biometeorol. 63, 1265–1281. doi: 10.1007/s00484-019-01735-9
Ravagnolo O., Misztal I., Hoogenboom G. (2000). Genetic component of heat stress in dairy cattle, development of heat index function. J. Dairy. Sci. 83, 2120–2125. doi: 10.3168/jds.S0022-0302(00)75094-6
Renaudeau D., Collin A., Yahav S., De Basilio V., Gourdine J. L., Collier R. J. (2012). Adaptation to hot climate and strategies to alleviate heat stress in livestock production. Animal 6, 707–728. doi: 10.1017/S1751731111002448
Robertson A. (1959). The sampling variance of the genetic correlation coefficient. Biometrics 15, 469–485. doi: 10.2307/2527750
Rötter R., van de Geijn S. C. (1999). Climate change effects on plant growth, crop yield, and livestock. Climatic. Change 43, 651–681. doi: 10.1023/A:1005541132734
Saeed M., Abbas G., Alagawany M., Kamboh A. A., Abd El-Hack M. E., Khafaga A. F., et al. (2019). Heat stress management in poultry farms: A comprehensive overview. J. Therm. Biol. 84, 414–425. doi: 10.1016/j.jtherbio.2019.07.025
Santana M. L., Bignardi A. B., Eler J. P., Ferraz J. B. S. (2016). Genetic variation of the weaning weight of beef cattle as a function of accumulated heat stress. J. Anim. Breed. Genet. 133, 92–104. doi: 10.1111/jbg.12169
Schauberger G., Mikovits C., Zollitsch W., Hörtenhuber S. J., Baumgartner J., Niebuhr K., et al. (2019). Global warming impact on confined livestock in buildings: efficacy of adaptation measures to reduce heat stress for growing-fattening pigs. Climatic. Change 156, 567–587. doi: 10.1007/s10584-019-02525-3
Shaji S., Niyas A. P., Chaidanya K., Sejian V., Bhatta R. (2015). Ameliorative strategies to sustain livestock production during heat stress. J. Vet. Sci. Med. Diag. 4, 3. doi: 10.4172/2325-9590.1000161
Strandén I., Kantanen J., Lidauer M. H., Mehtiö T., Negussie E. (2022). Animal board invited review: Genomic-based improvement of cattle in response to climate change. Animal 16, 100673. doi: 10.1016/j.animal.2022.100673
Tesema Z., Taye M., Ayichew D. (2019). The role of phenotypic and genetic basis of livestock selection for climate change adaptation and mitigation: A review. J. Appl. Adv. Res. 4, 66–77. doi: 10.21839/jaar.2019.v4i2.251
Thornton P., Nelson G., Mayberry D., Herrero M. (2021). Increases in extreme heat stress in domesticated livestock species during the twenty-first century. Glob. Change Biol. 27, 5762–5772. doi: 10.1111/gcb.15825
Tiezzi F., Brito L. F., Howard J., Huang Y. J., Gray K., Schwab C., et al. (2020). Genomics of heat tolerance in reproductive performance investigated in four independent maternal lines of pigs. Front. Genet. 11, 629. doi: 10.3389/fgene.2020.00629
Toghiani S., Hay E., Fragomeni B., Rekaya R., Roberts A. J. (2020). Genotype by environment interaction in response to cold stress in a composite beef cattle breed. Animal 14, 1576–1587. doi: 10.1017/S1751731120000531
Whiting P., Savović J., Higgins J. P. T., Caldwell D. M., Reeves B. C., Shea B., et al. (2016). ROBIS: A new tool to assess risk of bias in systematic reviews was developed. J. Clin. Epidemiol. 69, 225–234. doi: 10.1016/j.jclinepi.2015.06.005
Yalcin S., Settar P., Ozkan S., Cahaner A. (1997). Comparative evaluation of three commercial broiler stocks in hot versus temperate climates. Poult. Sci. 76, 921–929. doi: 10.1093/ps/76.7.921
Keywords: genotype-by-environment interaction, climatic parameters, heat stress, production, fertility, health
Citation: Fodor I, Spoelstra M, Calus MPL and Kamphuis C (2023) A systematic review of genotype-by-climate interaction studies in cattle, pigs, and chicken. Front. Anim. Sci. 4:1324830. doi: 10.3389/fanim.2023.1324830
Received: 20 October 2023; Accepted: 07 December 2023;
Published: 20 December 2023.
Edited by:
Angela Cánovas, University of Guelph, CanadaReviewed by:
Francesco Tiezzi, University of Florence, ItalySeyed Abbas Rafat, University of Tabriz, Iran
Copyright © 2023 Fodor, Spoelstra, Calus and Kamphuis. This is an open-access article distributed under the terms of the Creative Commons Attribution License (CC BY). The use, distribution or reproduction in other forums is permitted, provided the original author(s) and the copyright owner(s) are credited and that the original publication in this journal is cited, in accordance with accepted academic practice. No use, distribution or reproduction is permitted which does not comply with these terms.
*Correspondence: István Fodor, istvan.fodor@wur.nl