- 1Department of Design, Production and Management, University of Twente, Enschede, Netherlands
- 2eLaw Center for Law and Digital Technologies, Leiden University, Leiden, Netherlands
Policymakers need to consider the impacts that robots and artificial intelligence (AI) technologies have on humans beyond physical safety. Traditionally, the definition of safety has been interpreted to exclusively apply to risks that have a physical impact on persons’ safety, such as, among others, mechanical or chemical risks. However, the current understanding is that the integration of AI in cyber-physical systems such as robots, thus increasing interconnectivity with several devices and cloud services, and influencing the growing human-robot interaction challenges how safety is currently conceptualised rather narrowly. Thus, to address safety comprehensively, AI demands a broader understanding of safety, extending beyond physical interaction, but covering aspects such as cybersecurity, and mental health. Moreover, the expanding use of machine learning techniques will more frequently demand evolving safety mechanisms to safeguard the substantial modifications taking place over time as robots embed more AI features. In this sense, our contribution brings forward the different dimensions of the concept of safety, including interaction (physical and social), psychosocial, cybersecurity, temporal, and societal. These dimensions aim to help policy and standard makers redefine the concept of safety in light of robots and AI’s increasing capabilities, including human-robot interactions, cybersecurity, and machine learning.
Introduction
The robotic industry is developing rapidly, affecting different aspects of modern working life. Collaborative robots, so-called cobots, can among other things, support human workers in a shared workspace, nurses working with lifting robots, doctors with intelligent diagnostic systems, and information system designers in public administration. The rate at which these developments occur is faster than ever before (European Agency for Safety and Health at Work, 2020). As pointed out by the Organisation for Economic Cooperation and Development (OECD, 2019), while about 14% of jobs are highly automatable in OECD countries, another 32% of jobs are likely to change radically as individual tasks keep getting automated within these jobs.
While robots help staff extend the professional service they provide, create new opportunities, entail resource efficiency, and increase productivity, it is unclear how such professions adhere and adapt to this new reality. Collaborative robots support different types of interaction, including physical and social, and may evoke social responses from workers or involve psychosocial elements like trust (Di Dio et al., 2020). For the physical elements, robot and AI deployments may increase the risk of collision for the cobots’ equipment, negatively impacting workers’ safety and short-term health. For the other elements, particularly mental health, which is often neglected and largely underestimated, the human-robot interactions may be sporadic or geared toward supporting long-term engagement over time, often involving emotion and memory adaptations that designers manipulate to combat user interest decline (Ahmad, Mubin and Orlando, 2017). The literature alerts that, given our human tendency to form bonds with the entities with whom we interact and the human-like capabilities of these devices, users may have strong connections with robots that may include dependency, deception, and overtrust (Robinette et al., 2016; Wagner, Borenstein et al., 2018).
In the chemical industry, robots are widely applied for inspection in confined spaces. Some robotic solutions are advancing industrial inspection capabilities with autonomous legged robots, bringing complete visibility and higher-quality data collection to chemical processing plants (Anybotics, 2021). Operators may use inspection robots within confined dangerous spaces to inspect defects in pipelines for inspection robots. To do this, operators may use multiple interaction interfaces, including a “screen” interface, and involving ergonomic constraints (while manoeuvring the robotic agent). Furthermore, the operation may involve high cognitive loads when manipulating the robotic agent to prevent hazards. Figure 1 illustrates an example of operator-control related hazards, which includes overheating of hardware parts of the robot, due to suboptimal control of speed and orientation of the robot by the operator. This is usually via interacting with the monitoring pendant (an example shown in Figure 1) while controlling the agent to navigate the confined pipeline, which may contain hazardous substances (Li et al., 2020).
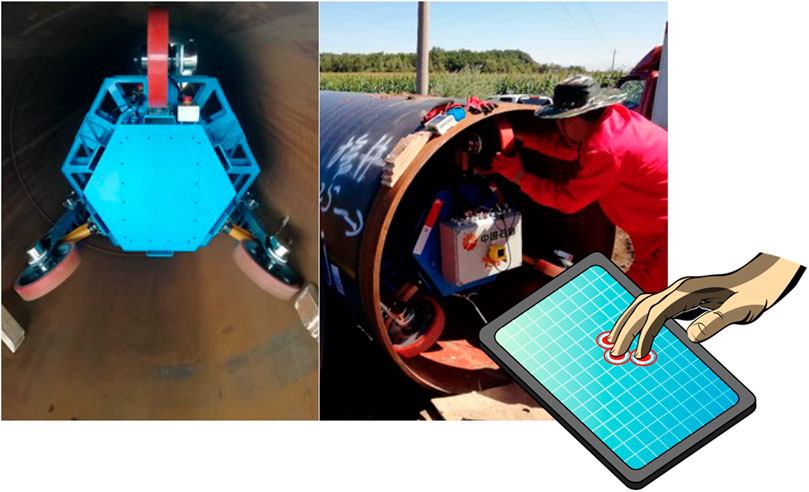
FIGURE 1. Example of a robot used for petroleum pipelines inspection (modified from Li et al., 2020).
As one can imagine, the nature of interactions, and their inherent safety, change with the introduction of these developments. In this respect, regulatory frameworks typically focused on ensuring physical safety by separating the robot from the human operator. However, industrial environments increasingly incorporate robots that interact directly with humans and it is unsure how safety should be addressed in such cases. In this sense, the definition of safety has been traditionally interpreted to exclusively apply to risks that have a physical impact on persons’ safety, such as, among others, collision risks. However, the increasing use of service and collaborative robots in shared workspaces that interact with users socially (also known as social robots) challenge the way safety has been addressed (Fosch-Villaronga and Virk, 2016). Moreover, the integration of AI in cyber-physical systems such as robots, the increasing interconnectivity with other devices and cloud services, and the growing human-machine interaction challenges the safety concept’s narrowness.
The recent advances in AI demand a broader understanding of safety, covering cybersecurity, and mental health to address safety comprehensively. Moreover, the expanding use of machine learning techniques will more frequently demand evolving safety mechanisms to safeguard the substantial modifications taking place over time. In this sense, this paper puts forward some recommendations to shed light on multiple dimensions of safety in light of AI’s increasing capabilities, including human-machine interactions, cybersecurity, and machine learning, to truly insure safety in human-robot interactions.
Mapping Different Perspectives on Safety in the Industrial Context
Definitions: Machinery, Robots and AI
According to the Council Directive 2006/42/EC a machine is defined as an assembly:
- fitted with or intended to be fitted with a drive system other than directly applied human or animal effort, consisting of linked parts or components, at least one of which moves, and which are joined together for a specific application;
- ready to be installed and able to function as it stands only if mounted on a means of transport, or installed in a building or a structure,
- partly completed machinery which, to achieve the same end, are arranged and controlled so that they function as an integral whole;
- of linked parts or components, at least one of which moves and which are joined together, intended for lifting loads and whose only power source is directly applied human effort;
There is no clear and explicit reference to robot/cobot equipment or AI agents within this definition. To have a specific understanding of what a robot is, it is necessary to adopt the definition offered by the ISO 8373:2012 that describes a robot as an automatically controlled, reprogrammable, multipurpose manipulator programmable in three or more axes, which can be either fixed in place or mobile for use in industrial automation applications. To bridge the gap between public policymaking and private setting, other scholars have defined robots as a movable machine that performs tasks either automatically or with a degree of autonomy (Fosch-Villaronga and Millard, 2019).
Having a consensus about the definition of AI is even more challenging. The European Commission has defined it as “systems that display intelligent behaviour by analyzing their environment and taking actions—with some degree of autonomy—to achieve specific goals. AI-based systems can be purely software-based, acting in the virtual world (e.g., voice assistants, image analysis software, search engines, speech and face recognition systems), or AI can be embedded in hardware devices (e.g., advanced robots, autonomous cars, drones, or Internet of Things applications) (HLEG on AI, 2019). Within this complex definition, what is clear is that AI capabilities such as machine learning (Salay et al., 2017) or human-machine interaction have far-reaching implications that challenge many concepts and areas, including standards such as the ISO 26262:2018 on functional safety for road vehicles, and the European Directive for product safety 2001/95/EC.
There are movements to address and define this field on both sides. An example is the establishment of the Sub-Group on AI, connected products, and other new challenges in product safety to the Consumer Safety Network (CSN) at the European Commission; and the creation of an ad hoc working group (SC 42) at the ISO level. In this sense, and once again (Fosch-Villaronga and Golia, 2018), regulation and standardization approaches are working separately while it is increasingly clear that a joint and more comprehensive approach to safety is deemed necessary (see Figure 2).
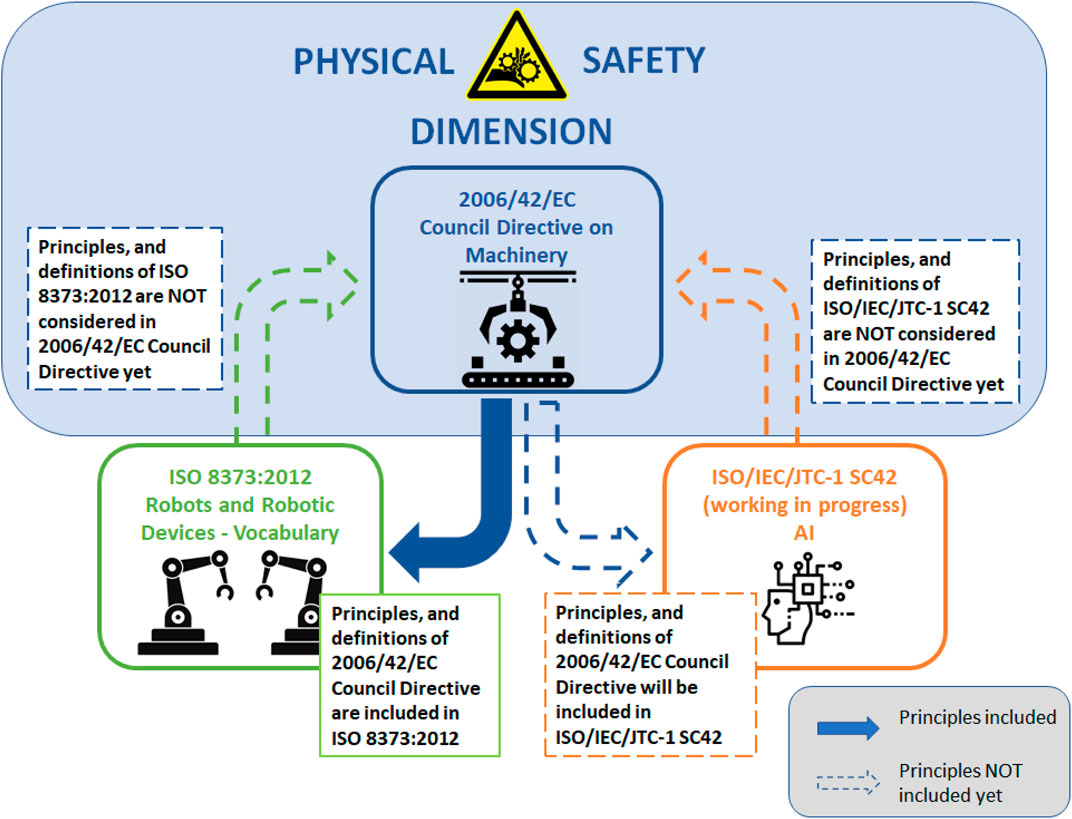
FIGURE 2. Relation between machinery, robot/collaborative robot and AI from a regulation perspective.
For now, in May 2021, the European Institutions released a “Proposal for a Regulation of the European Parliament and of the Council on machinery products” establishing a regulatory framework for placing machinery on the Single Market (Machinery Proposed Regulation, 2021). The regulation is designedly technology-neutral, laying down essential health and safety requirements to be complied with, without designating any specific technical solution to comply with those provisions. Although very new and not yet binding, it indicates that the concept of safety is not yet clear, as now it switched to a safety component.
The Physicality of the Concept of Safety
Even though safety has an overarching common meaning, laws, regulations and standards have adopted different definitions for different industrial sectors, consequently generating confusion, lack of clarity, and a lack of homogeneity in the understanding of what safety means. Take for instance the definition of safety enshrined in the current Directive 2001/95/EC on General Product Safety defines safety on its Art. 2. b): “safe product” shall mean any product which, under normal or reasonably foreseeable conditions of use including duration and, where applicable, putting into service, installation and maintenance requirements, does not present any risk or only the minimum risks compatible with the product’s use, considered to be acceptable and consistent with a high level of protection for the safety and health of persons. The article also establishes different features to consider part of this safety, including the characteristics of the products, the effect on other products, the presentation of the product, and also the different categories of consumers, in particular children and elderly.
This legal definition of safe products is quite broad, as said, and it can be understood as covering all kinds of risks that can, directly or indirectly, cause harm to consumers. However, the concept of safety is also present in other things that are not products directly used by the public (i.e., consumers) but that, still, is used by other humans (i.e., operators). In this sense, to have a whole picture of the evolution in terms of safety regulation in the European market, it is necessary to look at the end of the 1980s where different safety regulations framing safety at the workplace emerged.
The first game-changing pieces of legislation to promote safety in an industrial context were the Council Directive 89/391/EEC of June 12, 1989 on the introduction of measures to encourage improvements in the safety and health of workers at work (OSH Directive) and the Council Directive 89/392/EEC of June 14, 1989 on the approximation of the laws of the Member States relating to machinery. These pieces of legislation were issued simultaneously not accidentally, as both underlined how safety in an industrial setup could be achieved by providing safe workplaces (in terms of work organization and training) and safe equipment (referring to machinery and tools). Moreover, this framework aimed to create the first European common ground and harmonized regulation to level off the differences between member countries and facilitate the machinery’ circulation.
The general principles stated in the Council Directive 89/391/EEC are generally still applicable to foster workplace safety. Still, the definition of safety is not directly defined in the corpus (see Art. 3 Directive 89/391/EEC). The Council Directive 89/392/EEC went through several revisions to include the technology’s progress in terms of machinery and safety systems. The in-force Council Directive on machinery is the 2006/42/EC to the date of writing. Despite all the revisions, the concept of safety is neither spelt out in this corpus, only being addressed what is a “safety component” which refers to any component 1) which serves to fulfil a safety function, 2) which is independently placed on the market, 3) the failure and/or malfunction of which endangers the safety of persons, and 4) which is not necessary for the machinery to function, or for which normal components may be substituted for the machinery to function.
However, recent innovations in Artificial Intelligence (AI) and cyber systems, including robots/cobots raise several concerns including whether these robots pose additional risks for workers, lead to unequal treatment, or even commercial exploitation (Vrousalis, 2013; Kapeller et al., 2020). These advancements challenge the Council Directive 2006 42/EC’s validity and applicability to these new fields, which led the institutions to go through a thorough revision of this instrument. One of the first aspects that changed over the years is the concept of machinery, as it is currently unclear whether this definition encompasses all the new cyber-physical equipment such as robot/collaborative robot and AI agents. The fact that current standards do not refer to machinery but to robots and robotic devices and the other way round, that enacted/proposed legislation focuses on machinery, confuses this panorama further.
Another aspect was the concept of safety, which had been traditionally interpreted as physical. Although great advancements in this area, still, in February 2021, the regulatory scrutiny board opinion on the “Proposal for a Regulation of the European Parliament and the Council on machinery products”1 had some reservations concerning the evidence on the scope and magnitude of the problems regarding safety requirements or how the instrument is going to be future-proof given the evolving safety risk that AI entails.
Given the gaps in current legislation, private safety standards developed from international standardization organizations such as ISO/IEC covered different aspects connected to safety, including risk, harm, and hazard:
- Safety: freedom from risk which is not tolerable (ISO/IEC Guide 51:2014, 3.14)
- Risk: combination of the probability of occurrence of harm and the severity of that harm. Note 1 to entry: The probability of occurrence includes the exposure to a hazardous situation, the occurrence of a hazardous event and the possibility to avoid or limit the harm (ISO/IEC Guide 51:2014, 3.9)
- Harm: injury or damage to the health of people, or damage to property or the environment (ISO/IEC Guide 51:2014, 3.1)
- Hazard: Potential source of harm (ISO 13482:2014).
We notice that the definition of harm has been updated over the years. Namely the adjective physical before the injury has been deleted in recent updates. The reasons are that the word injury already means physical damage and that the responsible ISO/IEC Joint Working Group wanted this definition to have a broader interpretation including unreasonable psychological stress.
Psychosocial and Ethical Considerations for the Design of Robot Systems
It can be even claimed that traditionally the definition has been interpreted to exclusively apply to risks that have a physical impact on the safety of persons, such as among others mechanical or chemical risks (Consumer Safety Network, 2020). As previously mentioned, the broad definition of safety has traditionally focused on physical interaction as the focal point of hazard analysis and risk assessment. Only more recently are psychosocial influences on the safety of, especially, collaborative robots gaining more prominence (Chemweno et al., 2020). These include psychosocial influences linked to factors within a collaborative work environment such as high-job demand or nervousness working closely with a robotic agent that, in turn, triggers stressors such as fatigue, fear, or cognitive inattention (Riley, 2015). Often, these stressors likely create pathways that would lead to unsafe interaction with a robotic agent. Owing to the more recent recognition of psychosocial influences on safe work collaboration with robots, researchers have attempted to integrate implicit design features that mitigate stressors such as fear/nervousness when sharing a workspace with a robot (Chemweno et al., 2020). These features are more prominent for designs of social robots, by co-opting humanoid-like features to create confidence while interacting with the robot (Robinson, et al., 2019).
Additional psychosocial factors that influence safety when interacting with robots include the ergonomic design of the workspace and task design to consider the operator’s cognitive load (Chemweno et al., 2020). For instance, more recent research suggests that performing repetitive tasks and those requiring high cognitive capabilities such as complex assembly of a mechanical device often impedes the operator’s attention, creating a hazard pathway in shared collaborative workspaces (Bao et al., 2016). Other than interaction safety, psychosocial influences extend to long-term health effects, including depression, burn-outs, and reduced productivity for operators. Therefore, the relatively narrow view on physical human-robot interaction safety is a constraint for developing more robust design safeguards or safety assessment protocols. Moreover, this limitation is seen as discussed in this paper, where largely safety standards including the ISO 10218 (industrial robots), and the more recent ISO 15066 (collaborative robots) focus on techno-centric design safeguards. Primary, these latter safeguards embed hardware and software measures to mitigating collision risks. For instance, “soft” features such as pneumatic muscles limit injury in the event of a collision with a robotic arm (Van Damme et al., 2009).
Substantial techno-centric safeguards for collaborative robots are predominantly addressed by the ISO 15066, which proposes guidelines for designing safeguards, focusing on among other aspects, designing the collaborative workspace considering aspects such as access and a safe separation distance between the operator and robot. The separation here is achieved through passive or active sensing capabilities to prevent hazardous interaction, e.g., by including a laser “eye” to sense a breach of a safe separation distance (Michalos et al., 2015). Additional guidelines for design safeguards mentioned in the ISO 15066 norm include embedding a safety-rated monitored speed feature to limit the robot manipulator’s speed as it approaches the operator. Power and force limiting functions are also suggested to prevent hazardous contact and injury of a worker or operator. However, as discussed, the guidelines orient to techno-centric safeguards, thereby largely focusing on mitigating hazardous collisions. Seldomly, the design safeguards suggested in the current standards for robot safety, integrate psychosocial (and behavioural) factors of operators working with robots.
Nonetheless, AI presents quite interesting opportunities to integrate such psychosocial, behavioural factors in the design of robots. Though still largely at a research phase, designing “intelligent” robot systems is gaining traction. For instance, robots embedded with an “anticipatory safety reasoning system” to sense their environment and react to unsafe situations. This reasoning may involve the robot anticipating sudden, dangerous operator movement, by sensing behavioural changes and adjusting parameters such as speed of the robotic arm, or completely stopping (Frederick et al., 2019). Although quite a significant body of literature on anticipatory reasoning focuses on autonomous robots, considering such psychosocial human factors is a positive step, especially for collaborative robots anticipated shortly, to increasingly share the workspace with an operator. This is driven by industry needs to increase productivity by automating highly cognitive tasks. We should draw attention though, that integrating human behavioural factors requires a multidisciplinary effort involving engineering, industrial design, AI engineers, behavioural science, psychology, legal scholars, and safety experts, highlighting the scope of effort required to realize safe robotic systems fully.
However, the lack of established standards and norms to fully optimize multidisciplinary effort remains a critical bottleneck to overcome. More specifically, this relates to the absence of a common set of norms or guidelines robot designers can use to develop robust psychosocial safeguards and respective protocols for verifying the sufficiency of such safeguards as is commonly the case for other safety-critical systems and machinery (Fosch-Villaronga, 2019), e.g., for space exploration, aviation, and nuclear industry. However, there is a considerable body of literature recognising the importance of orienting psychosocial factors for new technologies, including implementing robotisation in workplaces. Although, achieving this orientation is recognised as a considerable challenge owing to demands in the workplace, including increased efficiency, productivity, quality and safety, suggestions that could lead to successful integration are proposed. As an example, Jones (2017) suggests an approach utilising a cooperating team-oriented approach, to implementing new technologies which integrate psychosocial factors while designing new workspaces. Their approach relies heavily on obtaining employee input throughout the implementation process for new technologies, including robots.
Mokarami et al. (2019) propose a structured integration approach, utilising questionnaires to measure psychosocial factors in the workplace, e.g., work-related stress. Based on measurements obtained using the Copenhagen Psychosocial Questionnaire (COPSOQ), the individual’s work-stressors are classified on a severity scale. The COPSOQ importantly considers technology factors, including interaction with robots. Predominantly though, psychosocial assessment and integration to technologies still largely concentrate on social robots, for example, where “empathetic features” are embedded on social robots to increase their acceptability, e.g., by patients with dementia (Rouaix et al., 2017). It is worth mentioning that psychosocial measurement is an essential starting basis for developing strategies for integrating human behavioural factors while designing robotic systems to enhance their safety. For instance, we see effort reported in studies towards utilising a measurement of pupillary response as the basis of quantifying cognitive loads for the operator (Strathearn and Ma, 2018; Borghini et al., 2020).
Although psychosocial assessment and measurement of associated human factors are an essential step towards embedding psychosocial design features to enhance safe robot interaction, measurement of such factors raises ethical questions. One may argue that utilising measurement data for designing robotic technologies may be questionable, especially in the absence of ethical standards for guiding psychosocial measurement, assessment, and use of behavioural measurement data. Bryson and Winfred (2017) argue the importance of standardising ethical design, when designing autonomous systems, including intelligent robots expected to interact more intensely with humans. They highlight ethical issues such as privacy and transparency to build trust when integrating AI into social and collaborative robots.
The development of the BS 8611:2016 focusing on ethical design and application of robot systems may partly address the aforementioned challenge, and an important step towards standardising ethics in AI systems design. Moreso, considering that psychosocial factors will continue to play an essential role in enhancing robotic systems’ safety, as designers strive to design more intelligent and interactive robot systems. Important clauses to take note of in the BS 8611:2016 is the requirement for ethical risk assessment, which presents guidance on how designers can integrate ethical considerations within the design of robot systems. The objective of risk consideration is to systematically identify and mitigate ethical risks, grouped into four broad categories: societal, application, commercial, financial, and environmental ethical risks. Particularly interesting for robots and AI is the societal, ethical risks that encompass risks such as loss of trust, infringement of privacy, confidentiality, and employment (Winfield, 2019). From a psychosocial perspective, loss of trust and deception are important considerations when we interact with robots, especially considering research studies focusing on designing “friendly” humanoid features, which may be argued to create trust when interacting with robots. Of course, one may argue that integrating humanoid features to be deceptive as it may mask a robot’s intention.
Perhaps also a notable clause of the BS 8611:2016 that relates to AI is the assertions of “humanity first” and “transparency needs.” The first assertion views robotics as an enabler of improving human conditions rather than an economic driver. Therefore, this implies the need to involve “all” stakeholders in the intelligent robotic systems’ design process creating safe robot systems. As discussed in this section, this involves operators who interact with robots, with psychosocial measurements expected to play an essential role in designing safe robots. Although this may also seem as straightforward, measuring human behaviour to ensure that it is primarily used ethically to improve working conditions at the expense of productivity goals remains unclear. Especially given the fact that pressure for productivity working alongside robots may be an important trigger for work-related stress, leading to long term psychological stress.
The absence of clarity also extends to transparency needs, specifically clarity on how human behavioural measurements are integrated ethically for intelligent robot designs, where AI plays an important role. For instance, Winfield (2019) argues that it should be possible to trace and determine why a robot behaves in a certain way or reaches decisions autonomously. This extends to autonomous behaviour reached by a robot, including collision avoidance when unsafe stressors are sensed from an operator, e.g., fatigue or work-related stress that is likely to cause physical or emotional harm.
Toward a Reconceptualisation of Safety, Considering Different Dimensions of Robots and AI
People have interacted and worked alongside machines since the 1st industrial revolution. However, the development of AI and its integration in the industrial setting that is now driving the 4th industrial revolution extends technology beyond just being an inanimate tool under full human control (Gunkel, 2020). In the early times of the introduction of robots in the workplace, the main focus was on physical safety, which was addressed by separating robots from human workers (Bekey, 2009). As robots became more integrated into workplaces, this separation reduced and collaborative robots (cobots) started working in the same space as humans (Bekey, 2009). This led to physical safety being the main dimension of safety addressed by safety regulations for robots (Harper and Virk, 2010; Jansen et al., 2018), and results on a rather narrowed lens through which safety is traditionally evaluated.
Smart robots assume social roles leading to an expansion of the possible dimensions of human-robot interaction (HRI) (Bankins and Formosa, 2020) and consequently safety. Such robots are increasingly employed in the workplace and interact with human workers physically and socially. As discussed previously, this new HRI may create mental, trust-related, affective, long-term health, societal, cybersecurity, and social consequences (Bankins and Formosa, 2020). Moreover, the interaction between robots and humans may hamper their privacy, autonomy, and even dignity (Fosch-Villaronga, 2019). Given the far-reaching dimensions of this phenomenon, it is essential to start reflecting on what this means for the current definitions of safety and discuss the need for a more comprehensive definition of the concept of safety that includes its multiple dimensions. As depicted in Figure 3, we can see that these different dimensions interact with each other, at different moments in times (even simultaneously), and affect one another. Depending on the context of use, the robot embodiment, and the type of HRI involved, the dimension interplay will vary. In the following subsections, we describe the different dimensions in detail for the community.
Interaction Dimension
Physical Interaction
Physical interaction between a robot and a human operator creates a physical safety dimension related to the protection of workers from injuries related to such interaction. One example is the increasing advances in social and industrial robotics and AI, which tremendously increased cobots’ autonomy and versatility, yet made them less predictable for human operators who need to collaborate with them (Jansen et al., 2018). For instance, the robot features anticipating human behaviour discussed in The physicality of the Concept of Safety . Less predictive robot behaviour makes physical safety challenging, although new sensors and increasingly improved AI and physical HRI (pHRI) algorithms enable humans and robots to work together, ensuring physical safety (Bankins and Formosa, 2020; Bicchi et al., 2009; Keemink et al., 2018; Pulikottil et al., 2021). Still, robots and automated systems at work used by workers may lead to indirect physical deterioration by resulting in awkward body postures, physical inactivity and sedentary work (Stacey et al., 2017). These are associated with heart disease, overweight, certain types of cancers, and psychological disorders (Stacey et al., 2017) and can compromise long-term physical safety.
Social Interaction
Cognitive Component
The introduction of robots in the workplace, among others, also introduces a cognitive and psychosocial behavioural interaction component. For example, more repetitive tasks with low variation may result in a perceived cognitive underload, passivity, and depression. On the other hand, this new smart interaction may lead to cognitive overload due to performance pressure to keep pace with the “perfect” robot, which is a machine that can work 24/7, without pause, and without social benefits. Even if the tasks vary between robots and human operators, their collaboration may lead to synchronization problems not in favor of the human operators. This is especially the case where the organisation is driven by business metrics, such as optimising productivity. The task allocation for the robot and operator should therefore be optimised, to better synchronise their capabilities. This intensification of work may involve additional risk factors too, such as isolation and lack of social interaction, similar to what many people experience due to the COVID-19 (Kniffin et al., 2020). This isolation may impact negatively on teamwork and the workers’ self-esteem, leading to over-reliance on technology and deskilling, which may have detrimental effects on job satisfaction and performance (Stacey et al., 2017). This further extends to ethical considerations for robot designs, considering among other factors, the need for ethical risk assessment referred to, in Psychosocial and Ethical Considerations for the Design of Robot Systems.
The interplay between physical-cognitive interaction with robots in the workplace, highlights the differences between certified safety and perceived safety (Fosch-Villaronga and Özcan, 2020) Perceived safety is “the user”s perception of the level of danger when interacting with a robot, and the user’s comfort level during the interaction’ (Bartneck et al., 2009). Indeed, “a certified robot might be considered safe objectively, but a (non-expert) user may still perceive it as unsafe or scary” (Salem et al., 2015). Being afraid of the device, for instance, affects the device’s adequate performance and the user: heartbeat may accelerate, hands may sweat. Depending on the user’s condition or position at work, these consequences may impact their perception of the device’s overall safety. In a major resolution, the European Parliament (2017) stressed: “you (referring to users) are permitted to make use of a robot without risk or fear of physical or psychological harm.” Because collaborative robots work with users and indissociably physical and cognitive aspects are at play simultaneously, special attention will have to be drawn progressively to both sides to ensure these devices’ safety. Therefore, this reinforces the need to re-evaluate how safety is conceptualised for robots, considering advances in AI-embedded design safeguards.
Psychological Component
Interaction can extend further than the physical dimension. Social robots interact with users socially and it is often zero-contact between the robot and the user, which challenges the applicability of current safeguards focussing solely on pHRI (Fosch-Villaronga, 2019). Recent advances in social and industrial robots allow robots to read social cues, anticipate behaviour, and even predict emotions (Cid et al., 2014; Hong et al., 2020), therefore giving them sophisticated social abilities (Henschel et al., 2020). How individuals interact with robots in a given context and for some time can have adverse short and long-term effects on their well-being and overall behaviour. This may create stressful HRI, especially in the cases of robots employing ruthless AI violating social conventions and expectations [for example in the case of Twitter being racist (Bouvier, 2020)]. This can have severe negative consequences for humans and in the long term compromise their psychological safety (Lasota, Fong and Shah, 2017). Also, the increasing use of emotions in HRI pushes the boundaries of what is considered acceptable or appropriate and in what precise context should be applied and encouraged, and where it should be applied with precaution (Fosch-Villaronga, 2019). For instance, researchers found that jobs with poor psychosocial quality may lead people to lower mental health than unemployment (Stacey et al., 2017). This aligns with ethical considerations, that prioritises the well-being of the worker as opposed to economic concerns of industry, outlined in the BS 8611:2016.
Trust Component
An important social aspect of human-human interaction that also plays a role in workplace acceptance of AI is trust (Brown et al., 2015; Lockey et al., 2021). Workplace performance is favoured in environments where employees enjoy the trust of their superiors (Brown et al., 2015). This trust may be affected by cobots monitoring their performance or antagonizing human workers, leading to a vicious circle of deteriorating performances (Brown et al., 2015). On the other hand, over-trust of robots’ capabilities and awareness of the workspace can result in high-risk behaviour, compromising physical and mental safety, whereas limited trust can result in acceptance and collaboration problems (Schaefer et al., 2016). Monitoring the performance of workers may lead to performance anxiety, work surveillance, and overlap between work and personal life, enforcing behaviours such as being available to work at any time and result in a lack of personal time and create burnouts, as workers cannot reach the employer’s ambitious targets without working overtime (Wisskirchen et al., 2017). Already at this point, a large percentage of individuals feel compelled to read work emails at home and outside office hours and experience anxiety when away from their electronic devices, a behaviour referred to as “technostress” (Stacey et al., 2017) likely to be replicated with the use of robot technology (Vrousalis, 2013).
Anthropomorphisation Component
Anthropomorphising animate or inanimate objects is something that humans often do to reinforce trust and acceptance (Hooker and Kim, 2019). This human tendency extends from anthropomorphising pets to smart machines (Darling, 2016). However, anthropomorphising robots may lead to complex psychological HRI (Terzioglu et al., 2020). By replacing human-human interaction with something less subtle and humanising machines, we may dehumanize the workplace and therefore compromise the mental and psychological safety of human workers (Hooker and Kim, 2019). A way to avoid such outcomes is proper training of involved workers in AI similar to how our ancestors were trained to collaborate with domesticated animals, and clear ethical and social distinctions between human and robot workers (Hooker and Kim, 2019).
Cyber Dimension
The integration of AI in cyber-physical systems such as robots, the increasing interconnectivity with other devices and cloud services, and the growing human-machine interaction challenges this narrow concept of physical system safety. Cloud services allow robots to offload heavy computational tasks such as navigation, speech, or object recognition on the cloud, and mitigate this way some of the limitations posed by their physical embodiment (Fosch-Villaronga and Millard, 2019). However, “the more functions are performed across interconnected systems and devices, the more opportunities for weaknesses in those systems to arise, and the higher the risk of system failures or malicious attacks” (Michels and Walden, 2018). To date, nonetheless, there is little understanding to what extent an attacker can exploit the computational parts of a robot to affect the physical environment in industrial (Quarta et al., 2017), social (Lera et al., 2017), or medical environments (Bonaci et al., 2015), and what that would entail for the users involved in the interaction (Fosch-Villaronga and Mahler, 2021). Ethical issues of transparency and cybersecurity present also an important concern, and are yet not comprehensively addressed for robots and other cyber-physical systems, despite existing cases concerning the hacking of autonomous vehicles and healthcare devices such as pacemakers (Miller, 2019).
Data characterizing the performance of human workers can be acquired in real-time in the workplace. This may lead to a rise in productivity, and simultaneously make work more transparent and allow companies to assess employees’ performance (Wisskirchen et al., 2017). The collection of personal data either for person-specific performance, analyzing the behaviour of humans collaborating with robots, or research raises multiple ethical questions about how such data is used, their impact on human workers’ wellbeing and safety, and who has access to the information. This may create trust issues between employees and employers and create performance anxiety (Brown et al., 2015). Additionally, it is interesting to reflect on what happens to the employee who does not fit in the criteria or working patterns defined by their employer (Bowen et al., 2017).
Temporal Dimension
It is important to mention here that psychological attrition, in contrast with physical, is not limited to proximal interaction and specific time frames, as it can affect workers seamlessly even via remote interactions and outside of working hours (Lasota et al., 2017). This extension provides an instance of the temporal dimension of safety: it is no longer reserved for an immediate physical impact/harm, but such harm, such consequence, can appear at a later stage in time. This relates to the fact that, sometimes, harm appears after the continuous use of a device [in the case of wearable walking exoskeletons, abnormal muscle activation could cause a problem at a later stage causing what is called prospective liability, (Fosch-Villaronga and Özcan, 2020) or in the case of unilateral transfemoral amputation the intact limb joints have a higher prevalence of osteoarthritis, due to amputees not interacting optimally with their artificial limb (Welke et al., 2019)].
Another temporal instance is the increasing use of machine learning algorithms. Machine learning provides machines with the possibility to learn from experience and adapt over time—something that is keeping busy certification agencies and policymakers around the world (FDA, 2021; Consumer Safety Network, 2020). The safety rules should explicitly include protection against risks related to subsequently uploaded software and extended functions acquired by machine learning (Fosch-Villaronga and Mahler, 2021).
The ability to make decisions based on predictive analytics is also a new element added to the temporal dimension that may challenge the user’s safety of the user—in case of wrongly predicted or inferred actions (i.e., a wrong future) (Fosch-Villaronga and Mahler, 2021). These capabilities can lead to very unfortunate scenarios that could even widen more the knowledge asymmetry gap, but that have also ulterior consequences at the cybersecurity level.
Societal Dimension
The societal dimension of safety refers to the societal challenges and consequences of introducing robots in the workplace (Frey and Osborne, 2017). Many workers also believe that cobots aim to replace them rather than reduce their workload. Feeling that one may not be needed in the future and that may be discarded at any time, replaced by a machine, creates societal pressure, and imbalance as it mainly affects manual labour workers and may lead to stress, lower job satisfaction and burnouts (Meissner et al., 2020).
Advancements in AI and virtual/augmented reality, in combination with robotics, may extend the workplace from physical to virtual/remote facilitating the transition towards an Operator 4.0 scenario (Romero et al, 2016). This extended workplace can allow workers to reduce their hours as they approach retirement, better balance work with leisure or education, and work flexibly. Less work supervision and more flexible working schedules may lead to stress and burnout, if not accompanied by workers’ training to obtain better skills for managing and organizing their workloads to create a good work-life balance that supports wellbeing (Stacey et al., 2017). Additionally, it can provide more people with access to work and markets that might not have been accessible in the past. This may result in a surplus of job offers in the job market and consequently insecurity, low pay and working quality (Stacey et al., 2017). Employment and social security laws coupled with narrow safety definitions may not have the versatility to deal with these changes in working patterns, and thus create insecurity for workers in the future that may be unprotected and low paid. This may have ulterior consequences in the social security scheme and potentially endanger the whole welfare system.
One societal challenge is how education is changing due to the introduction of these robots, either in factories or in hospitals where the surgery success does not depend just on the surgeon any longer but on the complex interaction and interplay between the doctor, the supporting staff, and the robot (i.e., the manufacturer) (Yang et al., 2017). Latent deskilling has to do with the gradual loss of essential skills due to overreliance on AI and workplace robotics (Rinta-Kahila et al., 2018). That becomes more apparent when a workplace process or a specific product is discontinued, and it may cause severe disruptions in workers’ daily work and internal organizational processes. Together with this, another societal dimension of safety is that over-reliance on AI in the workplace may lead to knowledge asymmetries such as the inability of workers to understand the inner workings of these machines and how these impact their lives (including what rights they have and what are the obligations they come with such transformation); or the impossibility of experts to anticipate and understand the impact of their work and communicate it to various societal stakeholders and, more importantly, assume responsibility (Lockey et al., 2021).
Conclusion
Working with robots and AI may be equivalent to working with a new species to some extent. The consequences of that interaction demand an open discussion for a more comprehensive safety regulatory framework encompassing and accommodating more dimensions of safety than just physical interaction. However, it may be that the solution to many of the multidimensional safety challenges introduced by advanced AI in the workplace may be addressed with a more comprehensive view of safety.
Although being a central concept in machinery, robot, AI, and human-robot interaction, the concept of safety is not clearly defined in current norms and legislations. Traditionally, the definition of safety has been interpreted to exclusively apply to risks that have a physical impact on persons’ safety, such as, among others, mechanical or chemical risks. However, the current understanding is that the integration of AI in cyber-physical systems such as robots, the increasing interconnectivity with several devices and cloud services, and the growing human-robot interaction challenges the safety concept’s narrowness.
The paper, therefore, brought together different dimensions relevant for a re-definition of safety required by the introduction of new technologies. These dimensions relate to interaction humans and these devices have (physical and social), their cyber and intangible components, the temporal nature of such interactions and consequences, and the potential societal effects these may have in the long run. A dimension from an AI perspective is quantifying these interactions. This will potentially yield new anticipatory algorithms that would allow collaborative agents to adapt their behaviour to mitigate hazardous contacts in shared workspaces. Some work in this direction includes, Strathearn and Ma (2018) where the authors explore applying psycho-social indicators to model operator behaviour and anticipate operator actions likely to lead to safety hazards.
We acknowledge though that, to be effective, such a concept needs to be modular and adaptive to the particular needs of each sector. Unfortunately, the proposal for a regulation on safety products does not guide in this respect (Machinery Proposed Regulation, 2021). Therefore, we call on different sectors where robots and AI are well integrated, including chemical engineering, to consider the new multidimensional concept of safety that we propose.
While the paper acknowledges that these dimensions may bring uncertainties concerning what are the assessment methods to ensure safety from a multi-dimensional viewpoint, these dimensions aim to stimulate the discussions among the community and help policy and standard makers redefine the concept of safety in light of robots and AI’s increasing capabilities, including human-robot interactions, cybersecurity, and machine learning. Over time, if different sectors share their lessons learned on how they understood and applied the different dimensions of safety we could generate knowledge that could support further revisions of this legislative instrument (Fosch-Villaronga and Heldeweg, 2018).
As a future direction, the concept of safety should be revised in light of the different dimensions here exposed, mainly physical, psychological, cybersecurity, temporal, and societal. To do so, multidisciplinary conversations and more research need to happen among researchers, legal scholars, and other relevant stakeholders at multiple levels in the public and private fields. One avenue could be to discuss this topic within relevant standardization organizations such as CEN/CENELEC, the European Standardization body, and incorporate such reflections in CENELEC Workshop Agreements (CWA). The authors took the first step in this direction and did so in the context of the H2020 COVR project, in which they presented the ideas developed in this article to the CEN/WS 08 “Safety in close human-robot interaction: procedures for validation tests.” The reflections will be successfully incorporated into the CWA. Afterward, the researchers will work on different case studies to see how these theoretical insights revolving around the concept of safety translate into specific case scenarios.
Data Availability Statement
The original contributions presented in the study are included in the article/supplementary material, further inquiries can be directed to the corresponding author.
Author Contributions
AM, PC, KN, and EF-V conceived and wrote the paper. AM, EF-V, and KN carried out the mapping on different safety perspectives. PC and KN carried out the reconceptualisation of safety. EF-V attracted part of the funding.
Funding
This project is part of LIAISON, a subproject of the H2020 COVR Project that has received funding 673 from the European Union’s Horizon 2020 research and innovation programme under grant agreement 674 No 779966.
Conflict of Interest
The authors declare that the research was conducted in the absence of any commercial or financial relationships that could be construed as a potential conflict of interest.
Publisher’s Note
All claims expressed in this article are solely those of the authors and do not necessarily represent those of their affiliated organizations, or those of the publisher, the editors and the reviewers. Any product that may be evaluated in this article, or claim that may be made by its manufacturer, is not guaranteed or endorsed by the publisher.
Footnotes
1See https://ec.europa.eu/transparency/regdoc/rep/2/2021/EN/SEC-2021-165-1-EN-MAIN-PART-1.PDF.
References
Ahmad, M., Mubin, O., and Orlando, J. (2017). A systematic review of adaptivity in human-robot interaction. Mti 1 (3), 14. doi:10.3390/mti1030014
Anybotics (2021). Animal Robotics. Retrieved from Available at: https://www.anybotics.com/anymal-makes-the-case-at-basf-chemical-plant/.
Bankins, S., and Formosa, P. (2020). When AI meets PC: exploring the implications of workplace social robots and a human-robot psychological contract. European Journal of Work and Organizational Psychology 29 (2), 215–229. doi:10.1080/1359432X.2019.1620328
Bao, S. S., Kapellusch, J. M., Merryweather, A. S., Thiese, M. S., Garg, A., Hegmann, K. T., and Silverstein, B. A. (2016). Relationships between job organisational factors, biomechanical and psychosocial exposures. Ergonomics 59 (2), 179–194. doi:10.1080/00140139.2015.1065347
Bartneck, C., Kulić, D., Croft, E., and Zoghbi, S. (2009). Measurement instruments for the anthropomorphism, animacy, likeability, perceived intelligence, and perceived safety of robots. Int J of Soc Robotics 1 (1), 71–81. doi:10.1007/s12369-008-0001-3
Bekey, G. (2009). “Current Trends in Robotics: Technology and Ethics,” in Robot Ethics: The Ethical and Social Implications of Robotics (MIT Press), 17–34.
Bicchi, A., Peshkin, M. A., and Colgate, J. E. (2009). “Safety for Physical Human-Robot Interaction,” in Springer Handbook of Robotics 57, 1335–1348. doi:10.1007/978-3-540-30301-5_58
Bonaci, T., Herron, J., Yusuf, T., Yan, J., Kohno, T., and Chizeck, H. J. (2015). To make a robot secure: An experimental analysis of cyber security threats against teleoperated surgical robots. arXiv preprint arXiv:1504.04339. doi:10.1145/2735960.2735980
Borenstein, J., Wagner, A. R., and Howard, A. (2018). Overtrust of pediatric health-care robots: A preliminary survey of parent perspectives. IEEE Robot. Automat. Mag. 25 (1), 46–54. doi:10.1109/mra.2017.2778743
Borghini, G., Ronca, V., Vozzi, A., Aricò, P., Di Flumeri, G., and Babiloni, F. (2020). Monitoring performance of professional and occupational operators. Handbook of clinical neurology 168, 199–205. doi:10.1016/b978-0-444-63934-9.00015-9
Bouvier, G. (2020). Racist call-outs and cancel culture on Twitter: The limitations of the platform’s ability to define issues of social justice. Discourse, Context & Media 38, 100431. doi:10.1016/j.dcm.2020.100431
Bowen, J., Hinze, A., Griffiths, C. J., Kumar, V., and Bainbridge, D. I. (2017). “Personal data collection in the workplace: Ethical and technical challenges,” in HCI 2017: Digital Make Believe - Proceedings of the 31st International BCS Human Computer Interaction Conference, 03 July 2017 (ACM), 1–11. doi:10.14236/ewic/HCI2017.57
Brown, S., Gray, D., McHardy, J., and Taylor, K. (2015). Employee trust and workplace performance. Journal of Economic Behavior & Organization 116, 361–378. doi:10.1016/j.jebo.2015.05.001
Chemweno, P., Pintelon, L., and Decre, W. (2020). Orienting safety assurance with outcomes of hazard analysis and risk assessment: A review of the ISO 15066 standard for collaborative robot systems. Safety Science 129, 104832. doi:10.1016/j.ssci.2020.104832
Cid, F., Moreno, J., Bustos, P., and Núñez, P. (2014). Muecas: A multi-sensor robotic head for affective human robot interaction and imitation. Sensors 14 (5), 7711–7737. doi:10.3390/s140507711
European Parliament (2017). Civil Law Rules on Robotics European Parliament resolution of 16 February 2017 with recommendations to the Commission on Civil Law Rules on Robotics (2015/2103(INL)). Retrieved from Available at: https://www.europarl.europa.eu/doceo/document/TA-8-2017-0051_EN.pdf.
Consumer Safety Network (2020). Opinion of the Sub-group On Artificial Intelligence (AI), Connected Products And Other New Challenges in Product Safety to the Consumer Safety Network. Retrieved from Available at: https://ec.europa.eu/consumers/consumers_safety/safety_products/rapex/alerts/repository/content/pages/rapex/docs/Subgroup_opinion_final_format.pdf (last accessed January 27, 2020).
Council Directive 89/391/EEC 1989 on the introduction of measures to encourage improvements in the safety and health of workers at work.
Council Directive 89/392/EEC 1989 on the approximation of the laws of the Member States relating to machinery.
Darling, K. (2016). “Extending legal protection to social robots: The effects of anthropomorphism, empathy, and violent behavior towards robotic objects,” in Robot law. Editors R. Calo, A. M. Froomkin, and I. Kerr (Edward Elgar Publishing), 213–232.
Di Dio, C., Manzi, F., Peretti, G., Cangelosi, A., Harris, P. L., Massaro, D., et al. (2020). Shall I Trust You? From Child Human-Robot Interaction to Trusting Relationships. Front. Psychol. 11, 469. doi:10.3389/fpsyg.2020.00469
Directive 2001/95/EC European Parliament and of the Council of 3 December 2001 on general product safety.
Directive 2006/42/EC European Parliament and of the Council of 17 May 2006 on machinery, and amending Directive 95/16/EC (recast).
European Agency for Safety and Health at Work (2020). Digitalisation and occupational safety and health. Bilbao. doi:10.2802/559587
HLEG on AI (2019) European Commission Concept of Safety. High-Level Expert Group. Retrieved from Available at: https://ec.europa.eu/newsroom/dae/document.cfm?doc_id=56341 (last accessed 27 January 2020).
Food and Drug Administration (2021). Artificial Intelligence and Machine Learning in Software as a Medical Device. Retrieved from Available at: https://www.fda.gov/medical-devices/software-medical-device-samd/artificial-intelligence-and-machine-learning-software-medical-device (last accessed January 27, 2020).
Fosch Villaronga, E. (2019). “"I Love You," Said the Robot: Boundaries of the Use of Emotions in Human-Robot Interactions,” in Emotional Design in Human-Robot Interaction. Editors H. Ayanoğlu, and E. Duarte (Cham: Springer), 93–110. doi:10.1007/978-3-319-96722-6_62019
Fosch Villaronga, E., and Golia, A. J. (2019). Robots, standards and the law: Rivalries between private standards and public policymaking for robot governance. Computer Law & Security Review 35 (2), 129–144. doi:10.1016/j.clsr.2018.12.009
Fosch-Villaronga, E., and Mahler, T. (2021). Cybersecurity, safety and robots: Strengthening the link between cybersecurity and safety in the context of care robots. forthcoming: Computer Law & Security Review.
Fosch-Villaronga, E., and Millard, C. (2019). Cloud robotics law and regulation. Robotics and Autonomous Systems 119, 77–91. doi:10.1016/j.robot.2019.06.003
Fosch-Villaronga, E., and Özcan, B. (2020). The Progressive Intermittent Between Design, Human Needs and the Regulation of Care Technology: The Case of Lower-Limb Exoskeletons. Int. J. Soc. Robot. 12 (4), 959–972.
Villaronga, E. F., and Virk, G. S. (2016). “Legal Issues for Mobile Servant Robots,”. Advances in Robot Design and Intelligent Control. RAAD 2016. Advances in Intelligent Systems and Computing. Editors A. Rodić, and T. Borangiu (Cham: Springer), 540, 605–612. doi:10.1007/978-3-319-49058-8_66
Frederick, P., Del Rose, M., and Cheok, K. (2019). Autonomous Vehicle Safety Reasoning Utilizing Anticipatory Theory.
Frey, C. B., and Osborne, M. A. (2017). The future of employment: How susceptible are jobs to computerisation?. Technological forecasting and social change 114, 254–280. doi:10.1016/j.techfore.2016.08.019
Gunkel, D. J. (2020). Mind the gap: responsible robotics and the problem of responsibility. Ethics Inf Technol 22 (4), 307–320. doi:10.1007/s10676-017-9428-2
Harper, C., and Virk, G. (2010). Towards the Development of International Safety Standards for Human Robot Interaction. Int J of Soc Robotics 2 (3), 229–234. doi:10.1007/s12369-010-0051-1
Henschel, A., Hortensius, R., and Cross, E. S. (2020). Social Cognition in the Age of Human-Robot Interaction. Trends in Neurosciences 43 (6), 373–384. doi:10.1016/j.tins.2020.03.013
Hong, A., Lunscher, N., Hu, T., Tsuboi, Y., Zhang, X., Alvesdos, S. F. d. R.R., Nejat, G., and Benhabib, B. (2020). “A Multimodal Emotional Human-Robot Interaction Architecture for Social Robots Engaged in Bidirectional Communication\vspace*7pt,” in IEEE Trans. Cybern, 06 March 2020 (IEEE), 1–15. doi:10.1109/tcyb.2020.2974688
Hooker, J., and Kim, T. W. (2019). Humanizing Business in the Age of Artificial Intelligence. Humanizing Business in the Age of AI 1, 1–24. Retrieved from Available at: http://public.tepper.cmu.edu/jnh/humanizingBusiness4.pdf (last accessed February 1, 2021).
Jansen, A., van der Beek, D., Cremers, A., Neerincx, M., and van Middelaar, J. (2018). Emergent Risks To Workplace Safety; Working in the Same Space As a Cobot.
Jones, D. R. (2017). “Psychosocial Aspects of New Technology Implementation,” in International Conference on Applied Human Factors and Ergonomics (Cham: Springer), 3–8. doi:10.1007/978-3-319-60828-0_1
Kapeller, A, Felzmann, H, Fosch-Villaronga, E, and Hughes, AM (2020). A Taxonomy of Ethical, Legal and Social Implications of Wearable Robots: An Expert Perspective. Sci Eng Ethics, 1–19. doi:10.1007/s11948-020-00268-4
Keemink, A. Q., van der Kooij, H., and Stienen, A. H. (2018). Admittance control for physical human-robot interaction. The International Journal of Robotics Research 37, 1421–1444. doi:10.1177/0278364918768950
Kniffin, K. M., Narayanan, J., Anseel, F., Antonakis, J., Ashford, S. P., Bakker, A. B., Bamberger, P., Bapuji, H., Bhave, D. P., Choi, V. K., Creary, S. J., Demerouti, E., Flynn, F. J., Gelfand, M. J., Greer, L. L., Johns, G., Kesebir, S., Klein, P. G., Lee, S. Y., Ozcelik, H., Petriglieri, J. L., Rothbard, N. P., Rudolph, C. W., Shaw, J. D., Sirola, N., Wanberg, C. R., Whillans, A., Wilmot, M. P., and Vugt, M. v. (2020). COVID-19 and the Workplace: Implications, Issues, and Insights for Future Research and Action. American Psychologist 76, 63–77. doi:10.1037/amp0000716
Lasota, P. A., Fong, T., and Shah, J. A. (2017). A Survey of Methods for Safe Human-Robot Interaction. FNT in Robotics 5 (3), 261–349. doi:10.1561/2300000052
Lera, F. J. R., Llamas, C. F., Guerrero, Á. M., and Olivera, V. M. (2017). “Cybersecurity of robotics and autonomous systems: privacy and safety,” in Robotics: Legal, Ethical and Socioeconomic Impacts. IntechOpen. Editor G. Dekoulis. doi:10.5772/intechopen.69796 https://cdn.intechopen.com/pdfs/56025.pdf
Li, H., Li, R., Zhang, J., and Zhang, P. (2020). Development of a pipeline inspection robot for the standard oil pipeline of China national petroleum corporation. Applied Sciences 10 (8), 2853. doi:10.3390/app10082853
Lockey, S., Gillespie, N., and Holm, D. (2021). A Review of Trust in Artificial Intelligence: Challenges,. Vulnerabilities and Future Directions, 5463–5472.
Meissner, A., Trübswetter, A., Conti-Kufner, A. S., and Schmidtler, J. (2021). Friend or Foe? Understanding Assembly Workers' Acceptance of Human-robot Collaboration. J. Hum.-Robot Interact. 10 (1), 1–30. doi:10.1145/3399433
Michalos, G., Makris, S., Tsarouchi, P., Guasch, T., Kontovrakis, D., and Chryssolouris, G. (2015). Design considerations for safe human-robot collaborative workplaces. Procedia CIrP 37, 248–253. doi:10.1016/j.procir.2015.08.014
Michels, J. D., and Walden, I. (2018). “How Safe is Safe Enough? Improving Cybersecurity in Europe's Critical Infrastructure Under the NIS Directive,”. Paper No. 291/2018 (Queen Mary School of Law Legal Studies Research Paper).
Miller, C. (2019). Lessons learned from hacking a car. IEEE Des. Test 36 (6), 7–9. doi:10.1109/mdat.2018.2863106
Mokarami, H., and Toderi, S. (2019). Reclassification of the work-related stress questionnaires scales based on the work system model: A scoping review and qualitative study. Wor 64 (4), 787–795. doi:10.3233/wor-193040
OECD (2019). OECD Employment Outlook 2019: The Future of Work. Paris: OECD Publishing. doi:10.1787/9ee00155-en
Machinery Proposed Regulation (2021) Proposal for a Regulation of the European Parliament and of the Council on machinery products’ establishing a regulatory framework for placing machinery on the Single Market. Retrieved from Available at: https://eur-lex.europa.eu/resource.html?uri=cellar:1f0f10ee-a364-11eb-9585-01aa75ed71a1.0001.02/DOC_1&format=PDF.
Pulikottil, T. B., Pellegrinelli, S., and Pedrocchi, N. (2021). A software tool for human-robot shared-workspace collaboration with task precedence constraints. Robotics and Computer-Integrated Manufacturing 67, 102051. doi:10.1016/j.rcim.2020.102051
Quarta, D., Pogliani, M., Polino, M., Maggi, F., Zanchettin, A. M., and Zanero, S. (2017). “An experimental security analysis of an industrial robot controller,” in 2017 IEEE Symposium on Security and Privacy, San Jose, CA, USA, 22-26 May 2017 (IEEE), 268–286.
Riley, P. (2015). Australian Principal Occupational Health, Safety and Well-Being Survey, Analysis Policy and Observation.
Rinta-Kahila, T., Penttinen, E., Salovaara, A., and Soliman, W. (2018). “Consequences of Discontinuing Knowledge Work Automation - Surfacing of Deskilling Effects and Methods of Recovery,” in Proceedings of the 51st Hawaii International Conference on System Sciences. doi:10.24251/hicss.2018.654
Robinson, N. L., Cottier, T. V., and Kavanagh, D. J. (2019). Psychosocial health interventions by social robots: systematic review of randomized controlled trials. Journal of medical Internet research 21 (5), e13203. doi:10.2196/13203
Romero, D., Stahre, J., Wuest, T., Noran, O., Bernus, P., Fast-Berglund, Å., and Gorecky, D. (2016). “Towards an operator 4.0 typology: a human-centric perspective on the fourth industrial revolution technologies,” in International conference on computers & industrial engineering (CIE46), 1–11.
Rouaix, N., Retru-Chavastel, L., Rigaud, A. S., Monnet, C., Lenoir, H., and Pino, M. (2017). Affective and engagement issues in the conception and assessment of a robot-assisted psychomotor therapy for persons with dementia. Frontiers in psychology 8, 950. doi:10.3389/fpsyg.2017.00950
Salay, R., Queiroz, R., and Czarnecki, K. (2017). An analysis of ISO 26262: Using machine learning safely in automotive software. CoRR abs/1709.02435. http://arxiv.org/abs/1709.02435.
Salem, M., Lakatos, G., Amirabdollahian, F., and Dautenhahn, K. (2015). “Towards safe and trustworthy social robots: ethical challenges and practical issues,” in International conference on social robotics, Paris, France, October 26-30, 2015 (Springer), 584–593. doi:10.1007/978-3-319-25554-5_58
Schaefer, K. E., Chen, J. Y. C., Szalma, J. L., and Hancock, P. A. (2016). A Meta-Analysis of Factors Influencing the Development of Trust in Automation. Hum Factors 58, 377–400. doi:10.1177/0018720816634228
Stacey, N., Ellwood, P., Bradbrook, S., Reynolds, J., and Williams, H. (2017). Key trends and drivers of change in information and communication technologies and work location Key trends and drivers of change in information and communication technologies and work location. European Agency for Safety and Health at Work.
Strathearn, C., and Ma, M. (2018). Biomimetic pupils for augmenting eye emulation in humanoid robots. Artif Life Robotics 23 (4), 540–546. doi:10.1007/s10015-018-0482-6
Robinette, P., Li, W., Allen, R., Howard, A. M., and Wagner, A. R. (2016). “Overtrust of robots in emergency evacuation scenarios,” in 2016 11th ACM/IEEE International Conference on Human-Robot Interaction (HRI), Christchurch, New Zealand, 7-10 March 2016 (IEEE), 101–108. doi:10.1109/HRI.2016.7451740
Terzioglu, Y., Mutlu, B., and Sahin, E. (2020). “Designing social cues for collaborative robots: The role of gaze and breathing in human-robot collaboration,” in ACM/IEEE International Conference on Human-Robot Interaction, March 2020 (ACM), 343–357. doi:10.1145/3319502.3374829
Van Damme, M., Vanderborght, B., Verrelst, B., Van Ham, R., Daerden, F., and Lefeber, D. (2009). Proxy-based sliding mode control of a planar pneumatic manipulator. The International Journal of Robotics Research 28 (2), 266–284. doi:10.1177/0278364908095842
Vrousalis, N. (2013). Exploitation, vulnerability, and social domination. Philos Public Aff 41 (2), 131–157. doi:10.1111/papa.12013
Welke, B., Jakubowitz, E., Seehaus, F., Daniilidis, K., Timpner, M., Tremer, N., Hurschler, C., and Schwarze, M. (2019). The prevalence of osteoarthritis: Higher risk after transfemoral amputation?-A database analysis with 1,569 amputees and matched controls. PLoS ONE 14, e0210868. doi:10.1371/journal.pone.0210868
Winfield, A. (2019). Ethical standards in robotics and AI. Nat Electron 2 (2), 46–48. doi:10.1038/s41928-019-0213-6
Wisskirchen, G., Thibault, B., Bormann, B. U., Muntz, A., Niehaus, G., Soler, G. J., and Von Brauchitsch, B. (2017). Artificial Intelligence and Robotics and Their Impact on the Workplace. IBA Global Employment Institute, 120.
Keywords: robots, cobots, artificial intelligence, safety, machinery directive, psychosocial aspects at work
Citation: Martinetti A, Chemweno PK, Nizamis K and Fosch-Villaronga E (2021) Redefining Safety in Light of Human-Robot Interaction: A Critical Review of Current Standards and Regulations. Front. Chem. Eng. 3:666237. doi: 10.3389/fceng.2021.666237
Received: 09 February 2021; Accepted: 14 July 2021;
Published: 27 July 2021.
Edited by:
Maria Chiara Leva, Technological University Dublin, IrelandReviewed by:
Rajagopalan Srinivasan, Indian Institute of Technology Madras, IndiaBrenno Menezes, Hamad bin Khalifa University, Qatar
Helen Durand, Wayne State University, United States
Copyright © 2021 Martinetti, Chemweno, Nizamis and Fosch-Villaronga. This is an open-access article distributed under the terms of the Creative Commons Attribution License (CC BY). The use, distribution or reproduction in other forums is permitted, provided the original author(s) and the copyright owner(s) are credited and that the original publication in this journal is cited, in accordance with accepted academic practice. No use, distribution or reproduction is permitted which does not comply with these terms.
*Correspondence: Alberto Martinetti, YS5tYXJ0aW5ldHRpQHV0d2VudGUubmw=
†These authors have contributed equally to this work and share first authorship