- 1Wageningen Food and Biobased Research, Wageningen, Netherlands
- 2Department of Business, Strategy and Political Sciences, USN School of Business, University of South-Eastern Norway, Kongsberg, Norway
One way to mitigate the negative impacts of climate change, is for society to move towards a biobased economy, where fossil resources are replaced by biobased ones. This replacement requires the development of biobased supply chains that differ significantly from the conventional supply chain. For example, seasonality and variability of the feedstocks create specific challenges for biobased systems and call for customized solutions for the design and operation of biobased chains. As a result, the modelling efforts to support decision-making processes for biobased logistics and supply chains have some different requirements. This paper presents a systematic literature review on logistics and supply chain modelling studies for the biobased economy published in a period of 2011–2020. The literature analysis shows that most modelling studies for the biobased economy are strategic optimization models aiming to minimize economic impact. As biomass source, forest and agricultural residues are mostly used, and fuel and energy are the most common biobased applications. Modelling strategies, biomass sources and applications are however diversifying, which is what we encourage for future research. Also, not only focusing on economic optimization but also optimizing social and environmental performance is an important future research direction, to deal with the sustainability challenges the world is facing.
1 Introduction
To avoid climate change by greenhouse gas emissions and its harmful consequences, our society needs to move away from the current fossil-based economy towards a biobased economy with biomass as a renewable resource (Sanders et al., 2010). This requires the design of biomass supply chains at reasonable costs and with low environmental impacts, which are broadly accepted by society (Nunes et al., 2020). In some regions, biobased supply chains are already established, such as the sugar cane to ethanol supply chain in Brazil and the palm oil supply chain in South East Asia (Lewandowski, 2018). However, in other many regions and with other sources or applications, biobased supply chains are still under development. Taking a logistics and supply chain modelling perspective for the bioeconomy allows for a systems approach, and look beyond individual products or factories. Logistics and supply chain models that are suitable to help decision makers to design and set up new supply chains for specific biobased products will stimulate the biobased economy (Atashbar et al., 2016).
Logistics and supply chain models for the biobased products are complex because of seasonal availability and variability of the biomass sources, scattered geographical distributions, quality variations, biomass deterioration, diverse conversion technologies and by-products, and inter-dependencies among logistics operations (Caputo et al., 2005; You et al., 2012; Malladi and Sowlati, 2018). In order to support the decision-making, supply chain models for the biobased economy that have to deal with all these different aspects tend to become very complex.
Contrary to biofuel and bioenergy logistics and supply chain models, supply chain models that focus on other types of biobased products such as biochemicals and biomaterials have not been subject to review broadly yet. However, there have been some reviews on biorefinery supply chain models (Pérez-Fortes et al., 2011; Wang et al., 2015; De Buck et al., 2020). Biorefinery supply chains are still studied in a fragmented and partial manner, and the complexity of biorefinery supply chains makes it hard to optimize them (Pérez et al., 2017). The review of De Buck et al. (2020) focuses mainly on the processes within the biorefinery itself. Pérez et al. (2017) focus on sustainability, but not from a modelling perspective and Wang et al. (2015) focus on bioenergy production. Also, Bussemaker et al. (2017) note that biorefinery supply chain analysis has traditionally focused on biofuel or bioheat production and tools for the evaluation of non-biofuel products like biochemicals and biomaterials are increasingly needed.
This paper aims to review logistics and supply chain models for the biobased economy, for all biomass sources and all biobased products. For this purpose, we systematically selected studies on logistics and supply chain modelling for the biobased economy. They were categorized and analyzed based on their scope (supply chain perspective, decision level, biomass source and application), their modelling choices (modelling and solution approach) as well as their goal. This way, this study provides a detailed overview of developments in logistics and supply chain models for the biobased economy in the last decade. Moreover, this study aims to provide an analysis of the gaps in literature so that a future research agenda can be formulated.
The remainder of the article is structured as follows. Section 2 provides the reader with further theoretical background and concept definitions of biomass logistics and supply chains for the biobased economy. In Section 3 we discuss our methodology, in Section 4 we show and discuss the general results and the results for each section of the analytical framework. In Section 5 we draw conclusions and in Section 6 we suggest directions for future research.
2 Theoretical Background
2.1 Concepts Related to Biomass Logistics and Supply Chains for the Biobased Economy
As biobased is often referred to as alternative to fossil based, we could state that it applies to all products can be made from fossil origin but can be replaced by organic resources. This means bio-based would include biochemicals, biomaterials, biofuels and bioenergy. The term “bioeconomy” is not necessarily focused on replacing fossil resources but it is more focused on the origin itself. Therefore it does not only entail bio-based but also food and animal feed (hereafter referred to as “feed”), which have always been of organic origin. This would thus make the bio-based economy a subset of the bioeconomy. Birner (2018) describes the replacement of fossil based resources by bio-based ones as an opportunity inherent in the concept of the bioeconomy. It should be noted however that the distinction between the bioeconomy and the biobased economy is not always clear, and can differ between sources and countries. Sometimes the terms are used interchangeably, for example in Lewandowski (2018).
The biobased economy value chain is integrated with the food and feed production chain through biorefineries that use biomass to deliver biomass components that can be used to make biobased products. Biomass that is used as a feedstock is an organic substance of one of the following types: agricultural crops and residues including lignocellulosic crops and residues (wood, grasses, or non-edible parts of plants) and food crops, fresh biomass (such as grass) and aquatic biomass, such as algae and seaweed (Sharma et al., 2018).
The International Energy Agency (IEA) Bioenergy Task 42 “Biorefining in the Circular Economy” defines biorefinery as the sustainable processing of biomass into a spectrum of marketable biobased products and bioenergy (Lindorfer et al., 2019). In a biorefinery, biomass sources are pre-treated, and then isolation and extraction are performed of valuable biomass components such as carbohydrates, proteins, natural fibers, lignin, specialties and oil and fats that form the basis for further conversion into biobased products (Yue et al., 2014). Various types of conversion can be distinguished: biotechnological and chemical conversion that can be combined with synthesis and modification and polymer processing. Groups of biobased products that can be distinguished are biochemicals, biomaterials, biofuels and bioenergy. Those biobased products can range from high-value, low volume fine chemicals such as pharmaceuticals, cosmetics, food additives to high-volume materials such as biofuels and fibers (Langeveld et al., 2010). A schematic representation of a biomass supply chain for the biobased economy is shown in Figure 1.
2.2 Biobased Logistics Models
Like all mathematical models, biobased logistics models have a goal, or objective function. Often, this is an economic objective, but performance can also be expressed in another sustainability dimension with a social or an environmental objective.
We categorize the scope of the model in four aspects, namely supply chain perspective, decision level, biomass source and biobased application. The supply chain perspective refers to which part of the supply chain is modeled: does the model describe the whole chain, or a part of the chain? Does it have a circular perspective? Decision levels can be divided into strategic, tactical and operational levels. In supply chain management, strategic decisions include network design, facility planning, location planning, capacity decisions; tactical decisions include distribution planning, amount of flow, mode of transportation; and operational decisions include production scheduling, customer demands and pricing (Kumar et al., 2020). The biobased source describes which source is used, and the application describes in which type of application the biobased source is being used after the biorefinery process.
In terms of modelling perspective, we consider three dimensions: the modelling choices (related to probability, time, and objectives), the solution approach (optimization, simulation, a combination thereof, game theory), and whether the modelers have chosen to incorporate modelling approaches from other disciplines. We will further elaborate on the solution approaches and the incorporation of modelling methods from other disciplines in Sections 2.3, 2.4.
2.3 Solution Approaches for Biobased Logistics Models
Mathematical models can be solved through optimization, simulation or a combination thereof (Bierlaire, 2015). Mathematical optimization models are designed to find the optimal solution given a set of constraints and a goal function. For example, to find the optimal location of a biorefinery to minimize transportation costs given some geographical restrictions. Different methods exist to solve an optimization model, for example, rigorous optimization, fuzzy programming and machine learning.
Simulation models can be used to analyze “what happens if” type of questions, and to compare multiple scenarios with each other. For example, to compare the effect of different fuel demand scenarios on the operations of a biorefinery. Optimization and simulation can also be combined, for example to test the robustness of the solution provided by the optimization (Kleijnen and Gaury, 2003).
2.4 Combination With Other Models and Computational Methods
In most studies, the inputs are given and the outputs are the main result of the model. Sometimes however, the inputs and output can also be subject to model calculations. In the context of biobased supply chains, we see the following models and computational methods being combined with optimization and simulation models: life cycle assessment (LCA), geo-information systems (GIS) and process simulation. LCA can be used to calculate environmental effects of a production chain. This can be done after the optimization, but it can also be part of the optimization, when the goal is, for example, to minimize environmental impact. GIS can be used to feed the mathematical model with geographical data, and it can be used to depict the suggested outcome on a map. Process simulation is used to estimate the parameters and effects of chemical processes (Chaves et al., 2016).
Figure 2 summarizes the structural dimensions and related analytical categories used in this study.
Table 3 shows the options considered for each of the dimensions of the framework.
3 Methodology
This study reviews the literature of logistics and supply chain modelling for the biobased economy to define the current status of the research in this field and to identify research gaps and future research directions. To this aim, we follow a structured process to ensure reproducibility and objectivity, inspired by the studies of Agi et al. (2020) and Wee and Banister (2016):
- Literature delimitation: defining the search boundaries.
- Material collection: defining the search strategy for strategically selecting the papers. The outcome of this step is a database containing the list of papers to review (hereafter: “the database”).
- Descriptive analysis: describing formal aspects of the papers, such as the publication date and journal.
- Category selection: specifying the categories in which the papers will be classified.
- Material evaluation: discussing the papers and their specific characteristics. By analyzing the source materials, we will provide insights in on logistics and supply chain modelling literature and reveal promising future research directions.
3.1 Literature Delimitation
In this section, we describe the criteria used to define the search boundaries. This study reviews the literature on logistics and supply chain models for the biobased economy. Therefore, each article that is included in the analysis should be about biobased logistics or the biobased supply chain, and there should be a (quantitative/mathematical) model described in the study. Studies were selected from Scopus and Web of Science, published in English in peer-reviewed journals. Review papers were only used for the introduction of this study but not for the content analysis. Conference papers, book chapters and technical reports were also excluded. As we aimed to focus on supply chain models, studies were not considered for further analysis if their main aim was to generate data, for example, macroeconomic studies, life cycle assessment (LCA) studies and geo-information systems (GIS) models. If those models however were combined with, or used as an input for a supply chain model, they were considered for further analysis.
3.2 Material Collection and Refinement
In our survey of publications, we queried the Thomson Reuters bibliographic database Web of Science and Elsevier’s Scopus database since both are commonly used for bibliometric analysis, but they also differ substantially in coverage (Mongeon and Paul-Hus, 2016). Moreover, we used backward and forward snowballing to extend our literature base with additional relevant papers.
We searched for the combination of specific keywords in the title, abstract, and author’s keywords. The set of keywords used includes:
- “logistic*” OR “supply chain”: to focus the search on supply chain/logistics studies;
- “biobased” OR “bio-based”: to focus the search on biobased applications;
- “model*”: to limit the results to studies containing a modelling approach;
- “biorefinery”: we noted that studies on biorefineries are often about the biobased supply chain, however they do not always use the word “biobased”. In order to not miss out on those publications, we included this search term.
The asterisk (*) is used to include different possible endings/spellings of a word, such as modelling, modeling, models.
Two keyword combinations were used:
[(biobased OR bio-based ) AND (logistic* OR (supply AND chain )) AND model*)], and [ (biorefinery AND model* AND (logistic* OR (“supply chain*”))].
The search was done for the past decade; so studies published between 2011 and 2020 were included.
All four co-authors of this study separately scored all studies article based on the title, abstract and keywords in the following manner:
- A high score (1) if and only if:
• The article is about biobased or a biorefinery.
• The article is about supply chain or logistics.
• The article is based on a quantitative approach, a modelling component is considered.
- A medium score (0.5) was given when there was doubt if the above requirements were met.
- A low score of (0) was given when at least one of the requirements was not met.
The paper was only considered for further analysis when the summed score of the (4) authors was equal to or higher than 3. The initial search resulted in a significant paper database (436 studies), which reduced in size after duplication removal and scoring them on relevance (84 studies). Figure 3 shows the initial results as well as how the number of papers was reduced by duplication exclusion and scoring.
4 Literature Analysis
In this Section 4, we first provide an overall description of our literature review database. Then, we provide the reader with a literature analysis per the analytical framework category. The final literature review database contained 84 papers. Table 1 gives the overview of the journals in which the studies were published and Table 2 shows the years of publication. The 84 papers were published in 23 different journals. Journal of Cleaner Production was found as the most common outlet for this research topic, followed by Applied Energy. Of the studies in the literature database, 38% were published between 2011 and 2015, and 62% was published between 2016 and 2020.
Table 3 summarizes the categories used to score and analyze the studies in the database.
For each category, we give a short explanation and the main findings related to that category. A summary of scoring results is given in Table 4 and the complete scoring results are given in Supplementary Table S1.
4.1 Biomass Source
The following biomass source types were found in the studies: forest/forest residues, agricultural residues, food waste or side-streams, energy crops, the biological fraction of municipal solid waste (MSW) and biomass of aquatic origin. Figure 4 gives an overview of all biomass sources in a pie chart. Some studies did not specify which biomass source was used, often they just used “biomass” to describe it. In Figure 4, those studies are referred to with “not specified.”’
The biomass sources agricultural residues (35%), forest residues (27%) and energy crops (24%) were most prevalent. The results change over the years: up to 2013, these three were the only sources described in the studies, and from 2014, also municipal solid waste (MSW), food waste, biomass of aquatic origin are mentioned as biomass sources.
4.2 Biobased Products
As discussed in Section 2, we define the biobased products: chemicals, fertilizer, materials, energy and fuel. However, since there is no universally agreed upon definition, and some studies also consider food and feed as part of the biobased products, we also consider those applications in our studies.
Figure 5 shows that fuel and energy (76%) is the most common application area. The other application areas (chemicals, fertilizers, materials, feed and food) sum up to 24%, and have mainly started to show up in studies published in or after 2018.
In Section 2 we discussed that bioeconomy and biobased are sometimes used interchangeably, and we argued that biobased could be considered an alternative to fossil based while bioeconomy entails everything with of organic origin (applications: biobased and food and feed). Our results showed only three studies that include food and feed applications came up in the search results showing that either most studies also use this definition or that food and feed studies are a longstanding subject of its own and are therefore not marked as biobased.
4.3 Supply Chain and Logistics Perspective
For each study, we checked if it focused on the whole supply chain, part of the supply chain, and/or if it had a circular perspective. Finally, we checked if collaboration or supply chain integration was discussed.
About two-third studies focused on the whole supply chain (69%) and about one-third (31%) focused on a specific part of the supply chain. Only three studies considered a circular perspective. Of all studies, 13% consider supply chain integration and/or collaboration. This is a low percentage since biorefineries can process streams from multiple actors and for multiple markets (Guo et al., 2020), and logistics collaboration can make it easier to achieve sustainability improvements (Stellingwerf et al., 2018).
4.4 Decision Level
We found most papers use a strategic decision level (59%), some approach the problem from a tactical decision level (30%) and a small group chooses an operational decision level (11%). The majority of the studies takes a strategic approach, which links to the majority of the studies taking a whole chain perspective.
Some studies (11) combine decision levels. Alizadeh et al. (2019) are the only authors combining all three decision levels. Most others (8) combine strategic and tactical decision levels, and two studies (Geraili et al., 2016; Gargalo et al., 2017) combine strategic and operational decisions levels.
4.5 Modelling Method and Solution Approach
For the modelling methods classification, we checked which choices were made in terms of probability, time and goal of the model. In terms of probability, we found that 67% of the models uses a deterministic approach, 10% use a stochastic approach, and 23% combines both approaches. In terms of time, 25% uses a single-period model and 75% uses a multi-period model. In terms of objective function, 70% uses a single objective, and 30% uses a multi-objective approach. Section 4.6 describes specifically which objective functions were used.
Thus, in terms of probability, deterministic models are most common, in terms of time, a multi-period approach is most common, and in terms of goal, the single objective is most often used. The multi-period aspects of the models can be linked to the seasonal nature of biobased supply chains. Despite the fact that deterministic models are most common within this selection of studies, there is an increase of the number of stochastic models over the decade.
The solution approaches found can be grouped into optimization, simulation, a combination of optimization and simulation, and game theory. Next to that, part of the studies use combined methods in which either heuristics or simulation or both are combined with GIS, LCA, or process simulation. Note that, in terms of solution methods, with simulation, we mean event-driven simulation. While in the combined methods, we check for process simulation which is a method used in chemical engineering to help with analysis, design and optimization of chemical processes (Chaves et al., 2016). Figure 6 depicts the division of those methods among the studies.
Figure 6 shows that optimization is most frequently used (75%, 64 studies), followed by a combination of optimization and simulation (18%); simulation is used in 6% of the cases, and 1% of the studies (1 study) used game theory.
Of the studies that used optimization, most used rigorous optimization; 8 used heuristics techniques to get to a solution within an acceptable computation time, 3 used fuzzy programming, and 1 used machine learning. Note that half of the studies using heuristics appeared in 2019 and 2020, so that seems a recent development.
Of the 84 studies that used optimization, simulation, or a combination of both, 33 studies combined this with LCA, GIS or process simulation. Table 5 shows which combinations of solution methods and computational models were used.
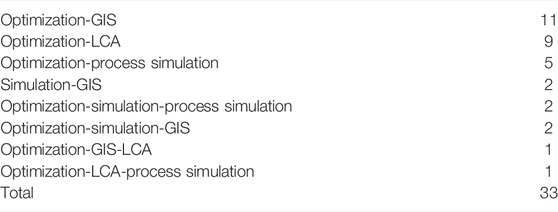
TABLE 5. Overview of the number of studies combining solution approach with another computational model.
GIS was used in 17 studies, LCA in 11 studies, and process simulation in seven studies.
4.6 Modelling Goal/Sustainability Approach
The objective functions found can be grouped into economic, environmental and social objectives, and into a combination of those. Figure 8 shows the division of the different objective functions over the studied papers.
Figure 6 shows that most studies focus on a single economic objective (72%). This is an interesting observation since almost all of the studies give environment-related reasons as a justification for executing their study. 16% of the studies combined economic and environmental objectives and 11% had objectives in the areas of all three sustainability pillars: people, planet and profit, for example, Chávez et al. (2017). Over time, there is no clear trend in terms of objective functions.
4.7 Geographical Region
For all studies we checked if they had a case study, and if the authors described where the case study was executed or on which geographical region the data were based. Most studies (63 out of the 84) described the case study location, the others did not describe their location, did not have a case study, or their case study was based on a hypothetical example. Figure 7 shows the division of case studies according to continents.
Figure 8 shows that most studies (62%) were performed in North America, followed by Europe (15%), South America (11%), Asia (10%) and Africa (2%). The case studies are not evenly distributed geographically. This is striking since the biobased economy is something that is relevant to the whole world.
Since most studies were performed in North America, we checked if there were differences between the studies that were performed there, and the studies that were performed in the rest of the world. We took the rest of the world together since we only have a few of those in our database, so if we grouped them per continent, we would not be able to make statements about them. The results are shown in Table 6.
Most categories do not show much differences between North America and the rest of the world. However, the following areas show interesting differences. Studies in North America focus less on using agricultural residues compared to the rest of the world, and less often specify the type of biomass used. Also, all studies using biomass of aquatic origin were performed outside of North America.
Outside of North America, collaboration or integration was studied relatively more often. In terms of solution approach, it is striking that optimization was used in all of the studies outside of North America (sometimes combined with simulation), and in North America, simulation was applied more often. In terms of combination with other computational methods, GIS is applied a lot more often in North America, while LCA is more common in the rest of the world. Both North America and the rest of the world focus on economic objectives, but studies performed in the rest of the world more often also used environmental and social objectives.
5 Discussion and Conclusion
5.1 Summary of Main Findings
In this study, we have collected, selected, and analyzed studies in the last decade on logistics and supply chain models for the biobased economy. We have found that most models are optimization models aimed at minimizing economic impact. Some more recent studies also combine the optimization methods with other methods such as LCA, GIS and process simulation–primarily used to obtain input data for the optimization model. In the studied literature, the main biomass sources are forest (residues) and agricultural residues. The main biobased product applications described are fuel and energy. However, both feedstock and application areas are diversifying. Since 2015 we have seen an increase in type of sources and applications in the publications that we studied.
Over three quarter of the case studies where the location was described, was executed in or based on data from North America; we do not think this is a good representation of the amount of biobased initiatives and research over the world. We also found that studies focus mainly on the strategic decision level and mostly cover the whole supply chain, or at least the supply chain up to the biorefinery. Finally, most of the times models are used to make supply chain design decisions. The fact that biobased logistics models often take a strategic perspective is possibly because biobased supply chains are relatively new, and therefore, a majority of studies will focus on design-related problems.
The abovementioned findings are in line with the results reported in other studies. Acuna et al. (2019) describe that existing biomass logistics and supply chain model studies mainly focus on economic optimization, and Ghaderi et al. (2016) state that most of those models focus on single-feedstock supply chains. Malladi and Sowlati (2018) discuss that most biobased logistics models are about supply chain design of biofuel and bioenergy production, and that recently, supply chain models that focus on other types of biobased products have started to develop.
One of the limitations of the methodology used is that studies that are relevant but do not use the exact wording that we searched for, will not have appeared in the results. Also our scoring method is not completely objective. However, we have aimed to make our literature search as clear and reproducible as possible.
5.2 Biobased Logistics and Sustainability
Many studies mention sustainability as a reason to focus on biobased rather than fossil resources. However, the majority of the models in the studies discussed only have an economic objective. Some studies combine economic and environmental objectives, and even fewer studies also consider a social objective. A recent literature review of biorefinery supply chain design (Pérez et al., 2017) confirm this observation: they show that very few investigations have considered all sustainability dimensions when designing the strategic planning of biorefineries in a territory. The focus on the economic aspects can be explained by the low-profit margin in the biobased sector. Additionally, some of the presented works aim at performing a feasibility study for a specific source or region, and accordingly, economic viability has been a primary concern. Despite this fact, in our opinion, future research should include all three sustainability pillars. Therefore, there is a need for more modelling efforts that look at economic, environmental and social objectives in a holistic way.
In operations research, especially sustainability-focused studies, often a trade-off between multiple objectives is calculated. However, in the studies analyzed for this review, only few authors incorporated such a trade-off curve. Therefore, this is a possible future research direction as well, which was also pointed out by Malladi and Sowlati (2018). In terms of methodology, this will imply using, for example, Multi-Attribute Decision Making models, Multi-Criteria Decision Making models and the epsilon-constraint method (Mavrotas, 2009; Huang et al., 2011).
5.3 Biobased Logistics and Complexity
Almost all studies are about real case studies. Generally, they combine model and a specific biomass source and a biobased product application. However, over the studied decade, there has been an increase in using the more general term “biomass.” So both source and application remain unspecified. The complexity of the supply chain models has also increased. At the beginning of the decade, most studies applied operations research methods to a case study and more recently, the complexity of biobased logistics (with uncertain and seasonal supply, and multiple inputs leading to multiple outputs) has given rise to supply chain models that are not focused on solving a real life problem but on solving an abstract version of a problem. The added complexity is also a reason for the recent increase in the use of heuristics and different types of mathematical approaches. Other studies confirm these observations: Ko et al. (2018) describe that biomass supply chains are more complex than standard fossil-based supply chains due to their specific challenges. Atashbar et al. (2016) mention that there is a need for further integration and optimization of the whole supply chain, taking into account more complexity. This current study shows that biobased logistics models are starting to develop into that area.
This study showed an increase in biomass sources and products. In the definition we used, feed and food are not part of the biobased products. However, several studies considered feed as a biobased outlet, and there was even one study that also considered food as biobased outlet. With the introduction of a further collaboration between food and biobased sectors, supply chains will become more integrated and the distinction between the biobased economy and the bioeconomy will become less clear. Guo et al. (2020) do consider food, feed, and biobased together and show that these chains can be complementary.
6 Future Research Agenda
6.1 A Global Perspective
Most of the studies we analyzed base their findings on case studies in North America. Other parts of the world are underrepresented in this topic, while geographical differences influence whole biobased supply chain. Therefore, we encourage scholars to pick a case study outside of North America.
6.2 Biobased Supply Chain Integration
Of the studied papers, 13% consider supply chain collaboration or integration. An integrated approach can help to deal with uncertainty in supply because of, for example, fluctuating yields. In the case of a biorefinery, integrating supply chains can help to benefit from economies of scale. The integration would also imply involving multiple actors (or their interests) in the decisions process. A modelling approach to tackle this is (cooperative) game theory. Only a couple of articles used such a multi-actors methodology for analyzing decisions in a biobased supply chain [i.e., (Jonkman et al., 2019) and Golecha and Gan (2016)]. However, most other studies assume there is only one actor (or problem owner) or one objective function for all supply chain actors. Considering multi-actor characteristics of biobased supply chains is an important direction for future research. In terms of methods, game theory and agent-based modelling are potentially useful to tackle the challenges in integration and collaboration in biobased chains. Torres et al. (2015) divide use a multi-actor approach by dividing a biorefinery optimization problem in two subproblems: the supply and the demand of intermediates. The actors on both sides of the problem can optimize their part separately while their solutions are coordinated to ensure a feasible biorefinery complex. In their follow-up study, they propose and develop a game theoretical framework and specific methodologies, which allow the optimal design of distributed processing systems, through the decentralized strategies of independent actors (Torres and Stephanopoulos, 2016). Their approach, combining chemical engineering, mathematical optimization and game theory, is very promising. However, only one of the citing studies uses game theory (Chang et al., 2018) but not in a biorefinery context. Thus, there is still enough room for researchers to cooperate and explore this research direction.
6.3 Supply Chain Resilience
Since supply chains are becoming more interconnected and interdependent, supply chain disruptions can become a risk. To deal with this, supply chain resilience has been studied in different contexts, for example, the chemical supply chains (Behdani et al., 2019) and food supply chains (Bottani et al., 2019). One study in our review database incorporates resilience (Maheshwari et al., 2017). They study resiliency optimization of the biomass to biofuel supply chain. To deal with future challenges, more studies on the biobased economy should consider incorporating resilience in their supply chain models.
6.4 Circularity in Biobased Supply Chain Models
In many studies, waste streams are considered as a source of biomass. However, only two studies in our literature database considered circularity or had a circular perspective. Our study has shown that the biobased economy does not only include relatively lower value biofuel and bioenergy products, but also higher value biobased products such as biochemicals and biomaterials that can potentially be re-used. Therefore, the scope of biobased logistics supply chain models should extend to also consider use (so also include the demand side) and re-use and take a circular perspective. This will further increase the complexity of the models because of fluctuation in supply, in expected yield and in composition. Moreover, these re-use streams are often difficult to preserve (De buck et al., 2020). The study of Yeo et al. (2020) is an example of how we expect more future research to consider circularity and complexity in biobased logistics is. They model circular supply chain design for palm oil by, for example, reusing the waste streams for produce electricity. In their supply chain model, they integrate the process design of biorefineries. Also, graph theory is used to test the supply chains.
In this study, we grouped a study into “studies the whole supply chain” it started with the biobased source (e.g., tree trunks) and considered the supply chain up to the market. However, biomass is generally grown on land and the market involves consumers that use a product and dispose of it (depending on the nature of the product). Considering circularity in supply chains is more than considering re-use of water in a biorefinery, it involves a cradle-to-cradle approach where the use and re-use is considered in the design phase (Muscat et al., 2021). It requires a holistic perspective and collaboration with people from other disciplines.
Because of externalities like carbon emissions, we expect policies to stimulate the use of biobased products and to reduce, and in time phase out, the use of fossil products. Both fossil and biobased products fulfil a demand for carbon. When that demand cannot be fulfilled from fossil resources anymore, it needs to be fulfilled by recycling, by using biomass and by converting CO2 into useful products using Carbon Capture and Utilization (CCU) technologies (Muscat et al., 2021). CCU technologies can be combined with bioconversions, as they often emit or consume carbon dioxide. These technologies are under development and to support these developments, smart supply chain decisions should be made. This is a promising topic for future research.
6.5 The Demand Side
Next to enabling circularity, another reason to consider the demand side is that will help choosing to produce in-demand, higher value products. This will become more relevant when moving from fuel and energy production to different products. To predict demand, studies could use macro-economic models to predict which products will be in demand and adjust the processes in the biorefinery accordingly.
The food supply chain is an example of a chain that is more demand-oriented, and research in food supply chain management has focused on valorization and diversification of the different (side) streams in the production process. Also, there is a vast amount of literature focusing on food quality management in the supply chain, for example (Tromp et al., 2016; Stellingwerf et al., 2021). As biomass supplied in biobased logistics can be subject to decay, models considering food quality in logistics could be used as in inspiration to develop models that help optimize the supply and product quality in the biobased supply chain.
Biobased supply chains (and models describing them) are increasingly becoming multi-feedstock, and multi-application, which means that there are an increasing number of variables to consider when making an optimal decision. Next to this increased complexity, multiple objectives should be considered to be able to meet sustainability requirements, and those objectives can be conflicting. This will make decisions and models to support those decisions increasingly complex. To deal with that complexity, future research should continue to incorporate methods that help reduce calculation time.
6.6 Sustainability and Collaboration in Biobased Logistics
To make logistics and supply chain models contribute to a sustainable biobased economy, researchers should aim to incorporate people, planet and profit objectives and circular perspectives into their studies. This will cause increased complexity, which requires new methodologies and collaboration from researchers from different fields and backgrounds. Our study shows the complexity of biobased logistics: knowledge from different fields such as chemical processes in bioreactors, sustainability analysis, operations research and mathematical modelling, needs to be combined to address challenges in these fields. This way, these biobased logistics supply chain models can contribute to tackling the challenges that the biobased economy has to offer.
Author Contributions
HS: concept development, data gathering, data analysis, writing article, revising article. EA: concept development, writing article, reviewing. BB: concept development, data analysis, writing article, reviewing. XG: concept development, reviewing.
Funding
This work is part of the research programme TransSonic, which is (partly) financed by the Netherlands Organisation for Scientific Research (NWO); also it was funded by Wageningen Food and Biobased Research.
Conflict of Interest
The authors declare that the research was conducted in the absence of any commercial or financial relationships that could be construed as a potential conflict of interest.
Publisher’s Note
All claims expressed in this article are solely those of the authors and do not necessarily represent those of their affiliated organizations, or those of the publisher, the editors and the reviewers. Any product that may be evaluated in this article, or claim that may be made by its manufacturer, is not guaranteed or endorsed by the publisher.
Acknowledgments
Special thanks to the experts who joined the seminar on this study for their valuable feedback and opinions on the topic and their future research suggestions.
Supplementary Material
The Supplementary Material for this article can be found online at: https://www.frontiersin.org/articles/10.3389/fceng.2022.778315/full#supplementary-material
References
Aboytes-ojeda, M., Castillo-Villar, K. K., and Roni, M. S. (2020). A Decomposition Approach Based on Meta-Heuristics and Exact Methods for Solving a Two-Stage Stochastic Biofuel Hub-And-Spoke Network Problem. J. Clean. Prod. 247, 119176. doi:10.1016/j.jclepro.2019.119176
Acuna, M., Sessions, J., Zamora, R., Boston, K., Brown, M., and Ghaffariyan, M. R. (2019). Methods to Manage and Optimize Forest Biomass Supply Chains: A Review. Curr. For. Rep. 5, 124–141. doi:10.1007/s40725-019-00093-4
Agi, M. A., Faramarzi-Oghani, S., and HAZıR, Ö. (2020). Game Theory-Based Models in Green Supply Chain Management: a Review of the Literature. Int. J. Prod. Res., 1–20. doi:10.1080/00207543.2020.1770893
Alizadeh, M., Ma, J., Marufuzzaman, M., and Yu, F. (2019). Sustainable Olefin Supply Chain Network Design Under Seasonal Feedstock Supplies and Uncertain Carbon Tax Rate. J. Clean. Product. 222, 280–299.
Arabi, M., Yaghoubi, S., and Tajik, J. (2019). A Mathematical Model for Microalgae-Based Biobutanol Supply Chain Network Design under Harvesting and Drying Uncertainties. Energy 179, 1004–1016. doi:10.1016/j.energy.2019.04.219
Atashbar, N. Z., Labadie, N., and Prins, C. (2016). Modeling and Optimization of Biomass Supply Chains: A Review and a Critical Look. IFAC-PapersOnLine 49, 604–615. doi:10.1016/j.ifacol.2016.07.742
Azadeh, A., Vafa Arani, H., and Dashti, H. (2014). A Stochastic Programming Approach towards Optimization of Biofuel Supply Chain. Energy 76, 513–525. doi:10.1016/j.energy.2014.08.048
Ba, B. H., Prins, C., and Prodhon, C. (2018). A Generic Tactical Planning Model to Supply a Biorefinery with Biomass. Pesqui. Oper. 38, 1–30. doi:10.1590/0101-7438.2018.038.01.0001
Bairamzadeh, S., Pishvaee, M. S., and Saidi-Mehrabad, M. (2016). Multiobjective Robust Possibilistic Programming Approach to Sustainable Bioethanol Supply Chain Design under Multiple Uncertainties. Ind. Eng. Chem. Res. 55, 237–256. doi:10.1021/acs.iecr.5b02875
Bairamzadeh, S., Saidi-Mehrabad, M., and Pishvaee, M. S. (2018). Modelling Different Types of Uncertainty in Biofuel Supply Network Design and Planning: A Robust Optimization Approach. Renew. energy 116, 500–517. doi:10.1016/j.renene.2017.09.020
Balaman, Ş. Y. (2016). Investment Planning and Strategic Management of Sustainable Systems for Clean Power Generation: An ε-constraint Based Multi Objective Modelling Approach. J. Clean. Prod. 137, 1179–1190. doi:10.1016/j.jclepro.2016.07.202
Balaman, Ş. Y., Matopoulos, A., Wright, D. G., and Scott, J. (2018). Integrated Optimization of Sustainable Supply Chains and Transportation Networks for Multi Technology Bio-Based Production: A Decision Support System Based on Fuzzy ε-constraint Method. J. Clean. Prod. 172, 2594–2617. doi:10.1016/j.jclepro.2017.11.150
Behdani, B., Lukszo, Z., and Srinivasan, R. (2019). Agent-oriented Simulation Framework for Handling Disruptions in Chemical Supply Chains. Comput. Chem. Eng. 122, 306–325. doi:10.1016/j.compchemeng.2018.09.027
Bierlaire, M. (2015). Simulation and Optimization: A Short Review. Transp. Res. Part C Emerg. Technol. 55, 4–13. doi:10.1016/j.trc.2015.01.004
Bottani, E., Murino, T., Schiavo, M., and Akkerman, R. (2019). Resilient Food Supply Chain Design: Modelling Framework and Metaheuristic Solution Approach. Comput. Industrial Eng. 135, 177–198. doi:10.1016/j.cie.2019.05.011
Bowling, I. M., Ponce-Ortega, J. M., and EL-Halwagi, M. M. (2011). Facility Location and Supply Chain Optimization for a Biorefinery. Ind. Eng. Chem. Res. 50, 6276–6286. doi:10.1021/ie101921y
Bussemaker, M. J., Day, K., Drage, G., and Cecelja, F. (2017). Supply Chain Optimisation for an Ultrasound-Organosolv Lignocellulosic Biorefinery: Impact of Technology Choices. Waste Biomass Valor 8, 2247–2261. doi:10.1007/s12649-017-0043-6
Cambero, C., and Sowlati, T. (2016). Incorporating Social Benefits in Multi-Objective Optimization of Forest-Based Bioenergy and Biofuel Supply Chains. Appl. Energy 178, 721–735. doi:10.1016/j.apenergy.2016.06.079
Cambero, C., Sowlati, T., and Pavel, M. (2016). Economic and Life Cycle Environmental Optimization of Forest-Based Biorefinery Supply Chains for Bioenergy and Biofuel Production. Chem. Eng. Res. Des. 107, 218–235. doi:10.1016/j.cherd.2015.10.040
Caputo, A. C., Palumbo, M., Pelagagge, P. M., and Scacchia, F. (2005). Economics of Biomass Energy Utilization in Combustion and Gasification Plants: Effects of Logistic Variables. Biomass Bioenergy 28, 35–51. doi:10.1016/j.biombioe.2004.04.009
Chang, H.-H., Chang, C.-T., and Li, B.-H. (2018). Game-theory Based Optimization Strategies for Stepwise Development of Indirect Interplant Heat Integration Plans. Energy 148, 90–111. doi:10.1016/j.energy.2018.01.106
Chaves, I. D. G., López, J. R. G., Zapata, J. L. G., Robayo, A. L., and Niño, G. R. (2016). Process Analysis and Simulation in Chemical Engineering. Springer.
Chavez, H., Castillo-Villar, K., and Webb, E. (2017). Development of the IBSAL-SimMOpt Method for the Optimization of Quality in a Corn Stover Supply Chain. Energies 10, 1137. doi:10.3390/en10081137
De buck, V., Polanska, M., and VAN Impe, J. (2020). Modeling Biowaste Biorefineries: A Review. Front. Sustain. Food Syst. 4, 11. doi:10.3389/fsufs.2020.00011
Delkhosh, F., and Sadjadi, S. J. (2020). A Robust Optimization Model for a Biofuel Supply Chain under Demand Uncertainty. Int. J. Energy Environ. Eng. 11, 229–245. doi:10.1007/s40095-019-00329-w
Ebadian, M., Sowlati, T., Sokhansanj, S., Stumborg, M., and Townley-Smith, L. (2011). A New Simulation Model for Multi-Agricultural Biomass Logistics System in Bioenergy Production. Biosyst. Eng. 110, 280–290. doi:10.1016/j.biosystemseng.2011.08.008
Galanopoulos, C., Barletta, D., and Zondervan, E. (2018). A Decision Support Platform for a Bio-Based Supply Chain: Application to the Region of Lower Saxony and Bremen (Germany). Comput. Chem. Eng. 115, 233–242. doi:10.1016/j.compchemeng.2018.03.024
Galanopoulos, C., Giuliano, A., Barletta, D., and Zondervan, E. (2020). An Integrated Methodology for the Economic and Environmental Assessment of a Biorefinery Supply Chain. Chem. Eng. Res. Des. 160, 199–215. doi:10.1016/j.cherd.2020.05.016
Gargalo, C. L., Carvalho, A., Gernaey, K. V., and Sin, G. (2017). Optimal Design and Planning of Glycerol-Based Biorefinery Supply Chains under Uncertainty. Ind. Eng. Chem. Res. 56, 11870–11893. doi:10.1021/acs.iecr.7b02882
Gebreslassie, B. H., Yao, Y., and You, F. (2012). Design under Uncertainty of Hydrocarbon Biorefinery Supply Chains: Multiobjective Stochastic Programming Models, Decomposition Algorithm, and a Comparison between CVaR and Downside Risk. AIChE J. 58, 2155–2179. doi:10.1002/aic.13844
Geraili, A., and Romagnoli, J. A. (2015). A Multiobjective Optimization Framework for Design of Integrated Biorefineries under Uncertainty. AIChE J. 61, 3208–3222. doi:10.1002/aic.14849
Geraili, A., Salas, S., and Romagnoli, J. A. (2016). A Decision Support Tool for Optimal Design of Integrated Biorefineries under Strategic and Operational Level Uncertainties. Ind. Eng. Chem. Res. 55, 1667–1676. doi:10.1021/acs.iecr.5b04003
Geraili, A., Sharma, P., and Romagnoli, J. A. (2014). A Modeling Framework for Design of Nonlinear Renewable Energy Systems through Integrated Simulation Modeling and Metaheuristic Optimization: Applications to Biorefineries. Comput. Chem. Eng. 61, 102–117. doi:10.1016/j.compchemeng.2013.10.005
Ghaderi, H., Pishvaee, M. S., and Moini, A. (2016). Biomass Supply Chain Network Design: an Optimization-Oriented Review and Analysis. Industrial crops Prod. 94, 972–1000. doi:10.1016/j.indcrop.2016.09.027
Ghani, N. M. A. M. A., Szmerekovsky, J. G., and Vogiatzis, C. (2019). Plant Capacity Level and Location as a Mechanism for Sustainability in Biomass Supply Chain. Energy Syst., 1–35. doi:10.1007/s12667-019-00361-z
Golecha, R., and Gan, J. (2016). Optimal Contracting Structure between Cellulosic Biorefineries and Farmers to Reduce the Impact of Biomass Supply Variation: Game Theoretic Analysis. Biofuels, Bioprod. Bioref. 10, 129–138. doi:10.1002/bbb.1626
Guo, X., Voogt, J., Annevelink, B., Snels, J., and Kanellopoulos, A. (2020). Optimizing Resource Utilization in Biomass Supply Chains by Creating Integrated Biomass Logistics Centers. Energies 13, 6153. doi:10.3390/en13226153
HajibabaI, L., and Ouyang, Y. (2013). Integrated Planning of Supply Chain Networks and Multimodal Transportation Infrastructure Expansion: Model Development and Application to the Biofuel Industry. Computer‐Aided Civ. Infrastructure Eng. 28, 247–259. doi:10.1111/j.1467-8667.2012.00791.x
He-lambert, L., English, B. C., Lambert, D. M., Shylo, O., Larson, J. A., Yu, T. E., et al. (2018). Determining a Geographic High Resolution Supply Chain Network for a Large Scale Biofuel Industry. Appl. Energy 218, 266–281. doi:10.1016/j.apenergy.2018.02.162
He-lambert, L., Shylo, O., English, B. C., Eash, N. S., Zahn, J. A., and Lambert, D. M. (2019). Supply Chain and Logistic Optimization of Industrial Spent Microbial Biomass Distribution as a Soil Amendment for Field Crop Production. Resour. Conservation Recycl. 146, 218–231. doi:10.1016/j.resconrec.2019.03.028
Hong, J.-D., Feng, K., and Xie, Y. (2014). A Simulation-Based Robust Biofuel Facility Location Model for an Integrated Bio-Energy Logistics Network. J. Industrial Eng. Manag. 7, 1415–1432. doi:10.3926/jiem.1196
Huang, I. B., Keisler, J., and Linkov, I. (2011). Multi-criteria Decision Analysis in Environmental Sciences: Ten Years of Applications and Trends. Sci. total Environ. 409, 3578–3594. doi:10.1016/j.scitotenv.2011.06.022
Jonkman, J., Kanellopoulos, A., and Bloemhof, J. M. (2019). Designing an Eco-Efficient Biomass-Based Supply Chain Using a Multi-Actor Optimisation Model. J. Clean. Prod. 210, 1065–1075. doi:10.1016/j.jclepro.2018.10.351
Judd, J. D., Sarin, S. C., and Cundiff, J. S. (2012). Design, Modeling, and Analysis of a Feedstock Logistics System. Bioresour. Technol. 103, 209–218. doi:10.1016/j.biortech.2011.09.111
Kang, S., Heo, S., Realff, M. J., and Lee, J. H. (2020). Three-stage Design of High-Resolution Microalgae-Based Biofuel Supply Chain Using Geographic Information System. Appl. Energy 265, 114773. doi:10.1016/j.apenergy.2020.114773
Kim, J., Realff, M. J., and Lee, J. H. (2011a). Optimal Design and Global Sensitivity Analysis of Biomass Supply Chain Networks for Biofuels under Uncertainty. Comput. Chem. Eng. 35, 1738–1751. doi:10.1016/j.compchemeng.2011.02.008
Kim, J., Realff, M. J., Lee, J. H., Whittaker, C., and Furtner, L. (2011b). Design of Biomass Processing Network for Biofuel Production Using an MILP Model. Biomass bioenergy 35, 853–871. doi:10.1016/j.biombioe.2010.11.008
Kim, S., Kim, S., and Kiniry, J. R. (2018). Two-phase Simulation-Based Location-Allocation Optimization of Biomass Storage Distribution. Simul. Model. Pract. Theory 86, 155–168. doi:10.1016/j.simpat.2018.05.006
Kleijnen, J. P. C., and Gaury, E. (2003). Short-term Robustness of Production Management Systems: A Case Study. Eur. J. operational Res. 148, 452–465. doi:10.1016/s0377-2217(02)00437-x
Ko, S., Lautala, P., and Handler, R. M. (2018). Securing the Feedstock Procurement for Bioenergy Products: A Literature Review on the Biomass Transportation and Logistics. J. Clean. Product. 200, 205–218.
Kumar, R., Ganapathy, L., Gokhale, R., and Tiwari, M. K. (2020). Quantitative Approaches for the Integration of Production and Distribution Planning in the Supply Chain: a Systematic Literature Review. Int. J. Prod. Res., 1–27. doi:10.1080/00207543.2020.1762019
Lainez-aguirre, J. M., Pérez-Fortes, M., and Puigjaner, L. (2017). Economic Evaluation of Bio-Based Supply Chains with CO 2 Capture and Utilisation. Comput. Chem. Eng. 102, 213–225. doi:10.1016/j.compchemeng.2016.09.007
Langeveld, J., Dixon, J., and Jaworski, J. (2010). Development Perspectives of the Biobased Economy: a Review. Crop Sci. 50, S142–S151. doi:10.2135/cropsci2009.09.0529
Leão, R. R. D. C. C., Hamacher, S., and Oliveira, F. (2011). Optimization of Biodiesel Supply Chains Based on Small Farmers: A Case Study in Brazil. Bioresour. Technol. 102, 8958–8963.
Lee, M., Cho, S., and Kim, J. (2017). A Comprehensive Model for Design and Analysis of Bioethanol Production and Supply Strategies from Lignocellulosic Biomass. Renew. Energy 112, 247–259. doi:10.1016/j.renene.2017.05.040
Lewandowski, I. (2018). Bioeconomy: Shaping the Transition to a Sustainable, Biobased Economy. Springer Nature.
Li, Y., Brown, T., and Hu, G. (2014). Optimization Model for a Thermochemical Biofuels Supply Network Design. J. Energy Eng. 140, 04014004. doi:10.1061/(asce)ey.1943-7897.0000158
Lin, T., Rodríguez, L. F., Davis, S., Khanna, M., Shastri, Y., Grift, T., et al. (2016a). Biomass Feedstock Preprocessing and Long-Distance Transportation Logistics. Gcb Bioenergy 8, 160–170. doi:10.1111/gcbb.12241
Lin, T., Rodríguez, L. F., Shastri, Y. N., Hansen, A. C., and Ting, K. C. (2014). Integrated Strategic and Tactical Biomass-Biofuel Supply Chain Optimization. Bioresour. Technol. 156, 256–266. doi:10.1016/j.biortech.2013.12.121
Lin, T., Rodríguez, L. F., Shastri, Y. N., Hansen, A. C., and Ting, K. (2013). GIS-enabled Biomass-Ethanol Supply Chain Optimization: Model Development and Miscanthus Application. Biofuels, Bioprod. Bioref. 7, 314–333. doi:10.1002/bbb.1394
Lin, Y., Pan, F., and Srivastava, A. (2016b). A Linear Programming Optimization Model of Woody Biomass Logistics Integrating Infield Drying as a Cost-Saving Preprocess in Michigan. For. Prod. J. 66, 391–400. doi:10.13073/fpj-d-15-00077
Lindorfer, J., Lettner, M., Hesser, F., Fazeni, K., Rosenfield, D., Annevelink, B., et al. (2019). Technical, Economic and Environmental Assessment of Biorefinery Concepts: Developing a Practical Approach for Characterisation. London, United Kingdom: IEA Bioenergy Task.
Maheshwari, P., Singla, S., and Shastri, Y. (2017). Resiliency Optimization of Biomass to Biofuel Supply Chain Incorporating Regional Biomass Pre-processing Depots. Biomass bioenergy 97, 116–131. doi:10.1016/j.biombioe.2016.12.015
Malladi, K. T., and Sowlati, T. (2018). Biomass Logistics: A Review of Important Features, Optimization Modeling and the New Trends. Renew. Sustain. Energy Rev. 94, 587–599. doi:10.1016/j.rser.2018.06.052
Mansoornejad, B., Pistikopoulos, E. N., and Stuart, P. R. (2013). Scenario-based Strategic Supply Chain Design and Analysis for the Forest Biorefinery Using an Operational Supply Chain Model. Int. J. Prod. Econ. 144, 618–634. doi:10.1016/j.ijpe.2013.04.029
Martinkus, N., Latta, G., Brandt, K., and Wolcott, M. (2018). A Multi-Criteria Decision Analysis Approach to Facility Siting in a Wood-Based Depot-And-Biorefinery Supply Chain Model. Front. Energy Res. 6, 124. doi:10.3389/fenrg.2018.00124
Marvin, W. A., Schmidt, L. D., and Daoutidis, P. (2013). Biorefinery Location and Technology Selection through Supply Chain Optimization. Ind. Eng. Chem. Res. 52, 3192–3208. doi:10.1021/ie3010463
Mavrotas, G. (2009). Effective Implementation of the ε-constraint Method in Multi-Objective Mathematical Programming Problems. Appl. Math. Comput. 213, 455–465. doi:10.1016/j.amc.2009.03.037
Mazzetto, F., Ortiz-Gutiérrez, R. A., Manca, D., and Bezzo, F. (2013). Strategic Design of Bioethanol Supply Chains Including Commodity Market Dynamics. Ind. Eng. Chem. Res. 52, 10305–10316. doi:10.1021/ie401226w
Medina-gonzález, S., Shokry, A., Silvente, J., Lupera, G., and Espuña, A. (2020). Optimal Management of Bio-Based Energy Supply Chains under Parametric Uncertainty through a Data-Driven Decision-Support Framework. Comput. Industrial Eng. 139, 105561.
Mobini, M., Sowlati, T., and Sokhansanj, S. (2011). Forest Biomass Supply Logistics for a Power Plant Using the Discrete-Event Simulation Approach. Appl. energy 88, 1241–1250. doi:10.1016/j.apenergy.2010.10.016
Mongeon, P., and Paul-hus, A. (2016). The Journal Coverage of Web of Science and Scopus: a Comparative Analysis. Scientometrics 106, 213–228. doi:10.1007/s11192-015-1765-5
Murillo-alvarado, P. E., Guillén-Gosálbez, G., Ponce-Ortega, J. M., Castro-Montoya, A. J., Serna-González, M., and Jiménez, L. (2015). Multi-objective Optimization of the Supply Chain of Biofuels from Residues of the Tequila Industry in Mexico. J. Clean. Prod. 108, 422–441. doi:10.1016/j.jclepro.2015.08.052
Muscat, A., DE Olde, E. M., Ripoll-Bosch, R., VAN Zanten, H. H. E., Metze, T. A. P., Termeer, C. J. A. M., et al. (2021). Principles, Drivers and Opportunities of a Circular Bioeconomy. Nat. Food 2, 561–566. doi:10.1038/s43016-021-00340-7
Ng, R. T. L., Kurniawan, D., Wang, H., Mariska, B., Wu, W., and Maravelias, C. T. (2018). Integrated Framework for Designing Spatially Explicit Biofuel Supply Chains. Appl. Energy 216, 116–131. doi:10.1016/j.apenergy.2018.02.077
Ng, R. T. L., and Maravelias, C. T. (2017). Design of Biofuel Supply Chains with Variable Regional Depot and Biorefinery Locations. Renew. Energy 100, 90–102. doi:10.1016/j.renene.2016.05.009
Nunes, L. J. R., Causer, T. P., and Ciolkosz, D. (2020). Biomass for Energy: A Review on Supply Chain Management Models. Renew. Sustain. Energy Rev. 120, 109658. doi:10.1016/j.rser.2019.109658
Osmani, A., and Zhang, J. (2014). Economic and Environmental Optimization of a Large Scale Sustainable Dual Feedstock Lignocellulosic-Based Bioethanol Supply Chain in a Stochastic Environment. Appl. energy 114, 572–587. doi:10.1016/j.apenergy.2013.10.024
Osmani, A., and Zhang, J. (2017). Multi-period Stochastic Optimization of a Sustainable Multi-Feedstock Second Generation Bioethanol Supply Chain − A Logistic Case Study in Midwestern United States. Land use policy 61, 420–450. doi:10.1016/j.landusepol.2016.10.028
Panteli, A., Giarola, S., and Shah, N. (2018). Supply Chain Mixed Integer Linear Program Model Integrating a Biorefining Technology Superstructure. Ind. Eng. Chem. Res. 57, 9849–9865. doi:10.1021/acs.iecr.7b05228
Pérez, A. T. E., Camargo, M., Rincón, P. C. N., and Marchant, M. A. (2017). Key Challenges and Requirements for Sustainable and Industrialized Biorefinery Supply Chain Design and Management: a Bibliographic Analysis. Renew. Sustain. Energy Rev. 69, 350–359.
Pérez, A. T. E., Rincón, P. C. N., Camargo, M., and Marchant, M. D. A. (2019). Multiobjective Optimization for the Design of Phase III Biorefinery Sustainable Supply Chain. J. Clean. Prod.
Pérez-fortes, M., Arranz-Piera, P., Laínez, J. M., Velo, E., and Puigjaner, L. (2011). Optimal Location of Gasification Plants for Electricity Production in Rural Areas. Comput. Aided Chem. Eng. 29, 1809–1813. doi:10.1016/B978-0-444-54298-4.50140-9
Pérez-fortes, M., Laínez-Aguirre, J. M., Arranz-Piera, P., Velo, E., and Puigjaner, L. (2012). Design of Regional and Sustainable Bio-Based Networks for Electricity Generation Using a Multi-Objective MILP Approach. Energy 44, 79–95.
Punnathanam, V., and Shastri, Y. (2020). Efficient Optimization of a Large-Scale Biorefinery System Using a Novel Decomposition Based Approach. Chem. Eng. Res. Des. 160, 175–189. doi:10.1016/j.cherd.2020.05.023
Roni, M. S., Thompson, D. N., and Hartley, D. S. (2019). Distributed Biomass Supply Chain Cost Optimization to Evaluate Multiple Feedstocks for a Biorefinery. Appl. Energy 254, 113660. doi:10.1016/j.apenergy.2019.113660
Sanders, J., Langevald, H., Kuikman, P., Meeusen, M., and Meijer, G. (2010). The Biobased Economy: Biofuels, Materials and Chemicals in the Post-oil Era. London, United Kingdom: Routledge.
Santibañez-Aguilar, J. E., González-Campos, J. B., Ponce-Ortega, J. M., Serna-González, M., and EL-Halwagi, M. M. (2014). Optimal Planning and Site Selection for Distributed Multiproduct Biorefineries Involving Economic, Environmental and Social Objectives. J. Clean. Prod. 65, 270–294.
Santibañez-Aguilar, J. E., Guillen-Gosálbez, G., Morales-Rodriguez, R., Jiménez-Esteller, L., Castro-Montoya, A. J., and Ponce-Ortega, J. M. (2016a). Financial Risk Assessment and Optimal Planning of Biofuels Supply Chains under Uncertainty. Bioenergy Res. 9, 1053–1069.
Santibañez-Aguilar, J. E., Morales-Rodriguez, R., González-Campos, J. B., and Ponce-Ortega, J. M. (2016b). Stochastic Design of Biorefinery Supply Chains Considering Economic and Environmental Objectives. J. Clean. Prod. 136, 224–245.
Santibañez-Aguilar, J. E., Rivera-Toledo, M., Flores-Tlacuahuac, A., and Ponce-Ortega, J. M. (2015). A Mixed-Integer Dynamic Optimization Approach for the Optimal Planning of Distributed Biorefineries. Comput. Chem. Eng. 80, 37–62.
Schröder, T., Lauven, L.-P., Sowlati, T., and Geldermann, J. (2019). Strategic Planning of a Multi-Product Wood-Biorefinery Production System. J. Clean. Prod. 211, 1502–1516.
Sharara, M. A., Sahoo, K., Reddy, A. D., Kim, S., Zhang, X., Dale, B., et al. (2020). Sustainable Feedstock for Bioethanol Production: Impact of Spatial Resolution on the Design of a Sustainable Biomass Supply-Chain. Bioresour. Technol. 302, 122896. doi:10.1016/j.biortech.2020.122896
Sharma, B., Clark, R., Hilliard, M. R., and Webb, E. G. (2018). Simulation Modeling for Reliable Biomass Supply Chain Design under Operational Disruptions. Front. Energy Res. 6, 100. doi:10.3389/fenrg.2018.00100
Sharma, B., Ingalls, R. G., Jones, C. L., Huhnke, R. L., and Khanchi, A. (2013). Scenario Optimization Modeling Approach for Design and Management of Biomass-To-Biorefinery Supply Chain System. Bioresour. Technol. 150, 163–171. doi:10.1016/j.biortech.2013.09.120
Singh, A., Chu, Y., and You, F. (2014). Biorefinery Supply Chain Network Design under Competitive Feedstock Markets: an Agent-Based Simulation and Optimization Approach. Ind. Eng. Chem. Res. 53, 15111–15126. doi:10.1021/ie5020519
Soren, A., and Shastri, Y. (2019). Resilient Design of Biomass to Energy System Considering Uncertainty in Biomass Supply. Comput. Chem. Eng. 131, 106593. doi:10.1016/j.compchemeng.2019.106593
Stellingwerf, H. M., Groeneveld, L. H. C., Laporte, G., Kanellopoulos, A., Bloemhof, J. M., and Behdani, B. (2021). The Quality-Driven Vehicle Routing Problem: Model and Application to a Case of Cooperative Logistics. Int. J. Prod. Econ. 231, 107849. doi:10.1016/j.ijpe.2020.107849
Stellingwerf, H. M., Laporte, G., Cruijssen, F. C. A. M., Kanellopoulos, A., and Bloemhof, J. M. (2018). Quantifying the Environmental and Economic Benefits of Cooperation: A Case Study in Temperature-Controlled Food Logistics. Transp. Res. Part D Transp. Environ. 65, 178–193. doi:10.1016/j.trd.2018.08.010
Sukumara, S., Faulkner, W., Amundson, J., Badurdeen, F., and Seay, J. (2014). A Multidisciplinary Decision Support Tool for Evaluating Multiple Biorefinery Conversion Technologies and Supply Chain Performance. Clean. Techn Environ. Policy 16, 1027–1044. doi:10.1007/s10098-013-0703-6
Sun, F., Sarin, S. C., Cundiff, J. S., and Sert, I. O. (2020). Design of Cost-Effective Sorghum Biomass Feedstock Logistics-A Comparison of Different Systems. Biomass Bioenergy 143, 105823. doi:10.1016/j.biombioe.2020.105823
Torres, A., Cybulska, I., Fang, C., Thomsen, M., Schmidt, J., and Stephanopoulos, G. (2015). A Novel Approach for the Identification of Economic Opportunities within the Framework of a Biorefinery. Comput. Aided Chem. Eng. doi:10.1016/b978-0-444-63577-8.50041-3
Torres, A. I., and Stephanopoulos, G. (2016). Design of Multi-Actor Distributed Processing Systems: A Game-Theoretical Approach. AIChE J. 62, 3369–3391. doi:10.1002/aic.15395
Tromp, S.-O., Haijema, R., Rijgersberg, H., and VAN DER Vorst, J. G. A. J. (2016). A Systematic Approach to Preventing Chilled-Food Waste at the Retail Outlet. Int. J. Prod. Econ. 182, 508–518. doi:10.1016/j.ijpe.2016.10.003
Wang, L., Agyemang, S. A., Amini, H., and Shahbazi, A. (2015). Mathematical Modeling of Production and Biorefinery of Energy Crops. Renew. Sustain. Energy Rev. 43, 530–544. doi:10.1016/j.rser.2014.11.008
Wang, Y., Ebadian, M., Sokhansanj, S., Webb, E., and Lau, A. (2017). Impact of the Biorefinery Size on the Logistics of Corn Stover Supply - A Scenario Analysis. Appl. energy 198, 360–376. doi:10.1016/j.apenergy.2017.03.056
Wee, B. V., and Banister, D. (2016). How to Write a Literature Review Paper? Transp. Rev. 36, 278–288. doi:10.1080/01441647.2015.1065456
Yeo, J. Y. J., How, B. S., Teng, S. Y., Leong, W. D., Ng, W. P. Q., Lim, C. H., et al. (2020). Synthesis of Sustainable Circular Economy in Palm Oil Industry Using Graph-Theoretic Method. Sustainability 12, 8081. doi:10.3390/su12198081
You, F., Tao, L., Graziano, D. J., and Snyder, S. W. (2012). Optimal Design of Sustainable Cellulosic Biofuel Supply Chains: Multiobjective Optimization Coupled with Life Cycle Assessment and Input-Output Analysis. AIChE J. 58, 1157–1180. doi:10.1002/aic.12637
Yue, D., You, F., and Snyder, S. W. (2014). Biomass-to-bioenergy and Biofuel Supply Chain Optimization: Overview, Key Issues and Challenges. Comput. Chem. Eng. 66, 36–56. doi:10.1016/j.compchemeng.2013.11.016
Zhang, F., Johnson, D. M., and Wang, J. (2016). Integrating Multimodal Transport into Forest-Delivered Biofuel Supply Chain Design. Renew. Energy 93, 58–67. doi:10.1016/j.renene.2016.02.047
Zhang, L., and Hu, G. (2013). Supply Chain Design and Operational Planning Models for Biomass to Drop-In Fuel Production. Biomass bioenergy 58, 238–250. doi:10.1016/j.biombioe.2013.08.016
Zhang, Y., Hu, G., and Brown, R. C. (2014). Integrated Supply Chain Design for Commodity Chemicals Production via Woody Biomass Fast Pyrolysis and Upgrading. Bioresour. Technol. 157, 28–36. doi:10.1016/j.biortech.2014.01.049
Zhu, X., Li, X., Yao, Q., and Chen, Y. (2011). Challenges and Models in Supporting Logistics System Design for Dedicated-Biomass-Based Bioenergy Industry. Bioresour. Technol. 102, 1344–1351. doi:10.1016/j.biortech.2010.08.122
Keywords: bioeconomy, biorefinery, optimization, simulation, sustainability
Citation: Stellingwerf HM, Guo X, Annevelink E and Behdani B (2022) Logistics and Supply Chain Modelling for the Biobased Economy: A Systematic Literature Review and Research Agenda. Front. Chem. Eng. 4:778315. doi: 10.3389/fceng.2022.778315
Received: 16 September 2021; Accepted: 13 April 2022;
Published: 09 May 2022.
Edited by:
Edwin Zondervan, University of Twente, NetherlandsReviewed by:
Ana Inés Torres, Universidad de la República, UruguayFernando Daniel Mele, Universidad Nacional de Tucumán, Argentina
Copyright © 2022 Stellingwerf, Guo, Annevelink and Behdani. This is an open-access article distributed under the terms of the Creative Commons Attribution License (CC BY). The use, distribution or reproduction in other forums is permitted, provided the original author(s) and the copyright owner(s) are credited and that the original publication in this journal is cited, in accordance with accepted academic practice. No use, distribution or reproduction is permitted which does not comply with these terms.
*Correspondence: Helena Margaretha Stellingwerf, aGVsZWVuLnN0ZWxsaW5nd2VyZkB3dXIubmw=