- 1Department of Psychology, Bishop's University, Sherbrooke, QC, Canada
- 2Department of Psychology, University of Waterloo, Waterloo, ON, Canada
- 3Foothills Medical Centre, Calgary, AB, Canada
Introduction: Going through life mindlessly appears to produce feelings of boredom and depression, suggesting that cognitive deficits can lead both directly and indirectly to emotional problems. Under this hypothesis, there are numerous possible routes from attention to affective issues, including through the sense of self-efficacy – a judgement about our ability to successfully achieve goals – and the experience of psychological stress. The present study assessed potential pathways from everyday inattentiveness, through the presumed intermediate experiences of diminished self-efficacy and psychological stress, to the experience of depressive affect.
Method: In two studies we collected questionnaire responses from large groups of participants (N = 188, Study 1; N = 399, Study 2), assessing individual differences in trait inattention, attention-related errors, self-efficacy, stress, and depression.
Results: Via path analyses we tested several predictions: 1) the frequency of attention lapses predicts depressive affect; 2) attention-related errors mediate effects of inattention in predicting both self-efficacy and stress; 3) self-efficacy and stress directly influence depressive affect. The results indicate the proposed Attention-to-Affect models provide good fit overall. They also indicate a reversal of the causal flow, while consistent with traditional views, does not adequately fit the data.
Discussion: That the Attention-to-Affect models provide good fit for the data is consistent with the view that everyday inattention contributes to the emotional distress that creates depression. While this view is contrary to the typical conception of attention problems as consequences of depression, it is consistent with our own previous findings. Accordingly, our results continue to suggest it is important for future research to further validate this pathway and to consider directly remedying attentional issues as a potentially important part of depression treatment strategies.
Influences of inattention on perceived self-efficacy, stress, and depression
Absentminded lapses of attention and a general tendency not to be fully mindful of our present experiences are common threads running through most of our daily lives. Although generally taken to be rather trivial events, they can sometimes have dramatic and even life-threatening consequences (Reason, 1984). Indeed, not paying attention to the critical task of driving the train may have been the primary cause of a major commuter train crash near Chatsworth, California, when an engineer became too focused on sending text messages from his cell phone to either notice or respond to operating signals telling him he should stop the train (National Transportation Safety Board, 2008). Such examples highlight the dramatic consequences that disruptions in attention can have when they happen to occur at particularly inopportune moments, but, given the pervasiveness of attention lapses in everyday life, particularly inopportune moments may not be a necessary component in this process. Indeed, the cumulative effects of inattention may have the potential for less obvious and dramatic, but ultimately more persistent emotional consequences. In our earlier work we presented data consistent with the view that seemingly minor episodes of everyday inattention, and their resulting minor cognitive and behavioral errors, are potentially important factors in the development of depression (Carriere et al., 2008). The present paper extends this earlier argument by testing two follow-up hypotheses that the influences of everyday inattention on depression are mediated, in part, by (1) one's general perceived self-efficacy and (2) one's overall experience of psychological stress.
To examine the potential influence of inattention on depression we (Carriere et al., 2008) previously conducted a large-scale questionnaire study and used structural equation modeling to test and compare two hypotheses: (1) that chronic cognitive failures in attention are an initial cause of depression (the Attention-to-Affect hypothesis), and (2) the reverse hypothesis that depression causes non-specific attention and memory failures (the Affect-to-Attention hypothesis). These hypotheses are both based on the commonly held belief that there is a causal connection between attention and depression, but their corresponding models differ with respect to how this causal link plays out in everyday life. To evaluate these hypotheses each model was compared against the null hypothesis, addressing the question of whether either an Attention-to-Affect or Affect-to-Attention model was capable of explaining the observed relations among the relevant variables. These analyses showed only the Attention-to-Affect model provided good fit for the data, by modeling attention lapses and associated everyday mistakes as a common cause of both memory failures and depression (Carriere et al., 2008). This causal flow is consistent with findings from MacLeod and colleagues (MacLeod et al., 2002), but contrasts with the traditional view of how emotion and attention are linked. Indeed, although not often articulated as an explicit hypothesis (owing, presumably, to the ubiquity of this view), the more common perspective is that emotions influence our attention. Indeed, for example, this appears to be the received view in most research on attentional biases in anxiety and depression (e.g., MacLeod et al., 1986; Gotlib et al., 1988; Dalgleish and Watts, 1990; Mogg et al., 1995; see also a review in Ingram et al., 2008) and on the attentional effects of encountering emotionally salient information (e.g., biases toward negative emotions; Fenske and Eastwood, 2003). With respect to the latter view, however, it is worth noting that attention has also been shown to have reciprocal effects on later emotional evaluation of stimuli (Fenske and Raymond, 2006).
Self-efficacy as a potential mediator of attention-to-affect
Given the above, we now seek to elaborate on the Attention-to-Affect hypothesis by evaluating another path by which attention lapses could influence our affective state—one's sense of self-efficacy. Self-efficacy is a judgement about our ability to successfully achieve our goals—usually in reference to a specific situation or activity (Bandura, 1977). Our sense of self-efficacy reflects both the expectation that we are capable of performing a task and that most others are not substantially more capable of performing the same task (Davis and Yates, 1982). Such beliefs apply not only to special skills or talents, but also to our ability to perform everyday tasks or cope with novel life challenges. These latter beliefs reflect a generalized sense of self-efficacy (Tipton and Worthington, 1984), which could be undermined in the face of chronic attention lapses interfering with our ability to perform even simple everyday tasks.
Reduced self-efficacy involves the belief that one's failures are the result of an inherent lack of ability rather than a consequence of situational factors or simply insufficient effort (Bandura, 1997). The obvious parallels between such beliefs and the counterproductive, negative affect-laden biases that are typical of depression support Bandura's claim that depression is a potential outcome of decreased self-efficacy. In particular, as he noted, if through our sense of inefficacy we come to believe our successes are the result of luck and failures represent our actual ability, depression will result in part because we devalue our accomplishments and overvalue our failures. Ultimately, because failure at a task is seen as more meaningful than success, even when initial failures are followed with later successes, we may still be less willing than others to engage in the same task again. On this view, reduced self-efficacy is thought to create cognitive biases that produce depression, and potentially influence attention by continually directing it away from information critical to successful task completion (Bandura, 1997). Furthermore, given that our sense of self-efficacy is relative to the ability of others, depression, and an unwillingness to even attempt tasks, is an especially likely result when we expect others would not have difficulty completing the same tasks with which we have had trouble (Davis and Yates, 1982).
According to the Attention-to-Affect hypothesis (Carriere et al., 2008) frequent attention lapses lead to attention-related errors that interfere in task success and should precede reductions in self-efficacy for that task. Given that attention lapses occur even in simple, everyday activities—particularly those we know are not problematic for most people—it follows that these continual mistakes could produce a generally diminished sense of self-efficacy and may then lead to disengagement from everyday behavior in general and eventually to depression. The present research thus applied the Attention-to-Affect hypothesis to illuminate more precisely the structure of these relations. In particular, we sought to evaluate the potential causal flow from attentional errors—specifically attention lapses and subsequent attention-related errors—through to decreased self-efficacy and on to a proneness to experience depression.
Study 1
To examine the hypothesis that perceived self-efficacy mediates the association between failures of basic cognitive mechanisms and depression, we conducted a path analysis using structural equation modeling in AMOS (Arbuckle, 2005) to examine the relations among five self-report questionnaires. Frequency of attention lapses and associated cognitive errors were assessed via the Mindful Attention Awareness Scale–Lapses Only and the Attention-Related Cognitive Errors Scale (MAAS-LO and ARCES: Carriere et al., 2008). We assessed depression via the Beck Depression Inventory—Second Edition (BDI-II: Beck et al., 1996). Self-efficacy was measured via the Generalized Self Efficacy scale (GSE: Schwarzer and Jerusalem, 1995).
Based on previous findings (Carriere et al., 2008) and the present arguments, we predicted the MAAS-LO would explain a significant amount of the variance in the BDI-II, while the ARCES would function as a mediator between the MAAS-LO and GSE, such that the ARCES would not explain a significant amount of the variance in the BDI-II once relations with the MAAS-LO and GSE were accounted for. The ARCES is the key variable in this case, since it allows us to place specific a priori constraints on the model, which are necessary for evaluating causal claims with correlational data, and it is most clearly recognized as an effect of inattention (i.e., it is non-sensical to instead argue that attention-related errors cause the inattention that was necessary for them to have occurred). With these same data we also evaluated the more conventional Affect-to-Attention hypothesis that negative affect creates cognitive biases which influence attention (e.g., through reduction of attentional capacity by rumination) and our perceived self-efficacy.
Method
Participants
Participants were from an international sample of 188 respondents who completed all five of the questionnaires below via our public attention lapses research website. Participants included in the analyses completed all four questionnaires and had no more than two missing responses for each questionnaire; 158 participants had zero missing responses. Participants received no compensation for completing the questionnaires, aside from the information already available to them on our website. Not all participants opted to provide demographic information and given that this study was conducted online we have no information for these participants beyond their questionnaire responses. Of those participants who opted to provide their demographic information, which represents the majority of the sample, there were 85 males and 97 females with a mean age of 36.1, (SD = 13.3; n = 178).
Measures
After first receiving the initial demographics questionnaire, the four questionnaires below were completed in random order across participants. In addition, the individual items within each questionnaire were randomly ordered, such that no two participants were likely to receive the exact same ordering of questionnaires and items. To accommodate occasional missing responses, item mean scores were calculated by averaging across the responses provided.
The 12-item Mindful Attention Awareness Scale–Lapses Only (MAAS-LO; Carriere et al., 2008) was selected as the measure of attention lapses. MAAS-LO items, such as “I find it difficult to stay focused on what's happening in the present,” ask about inattentive behavior in everyday situations using a six-point Likert scale ranging from almost never (1) to almost always (6). Responses indicating a greater frequency suggest a greater propensity toward everyday attention lapses. It is important to explicitly acknowledge here that, aside from removal of three items and the scale being direct-scored rather than reverse-scored, the MAAS-LO is identical to the MAAS as originally developed by Brown and Ryan (2003).
A revised version of the Attention-Related Cognitive Errors Scale (ARCES; Cheyne et al., 2006) was incorporated as an assessment of notable cognitive and behavioral outcomes of attention lapses. The revised ARCES (Carriere et al., 2008) is a 12-item questionnaire measuring the frequency with which one experiences a variety of cognitive failures, for example: “I have absent-mindedly misplaced frequently used objects, such as keys, pens, glasses, etc.” The ARCES employs a five-point Likert scale from never (1) to very often (5).
The 10-item General Self-Efficacy scale (GSE; Schwarzer and Jerusalem, 1995) was selected as a measure of one's perceived self-efficacy. The GSE includes items such as “I can always manage to solve difficult problems if I try hard enough” and “I am confident that I could deal efficiently with unexpected events,” and uses a four-point Likert scale ranging from not at all true (1) to exactly true (4).
We used the Beck Depression Inventory (BDI-II: Beck et al., 1996) to measure depression. The BDI-II is a 21-item scale that was designed to address the diagnostic criteria for depression outlined in the DSM-IV (American Psychiatric Association, 1994). The BDI-II asks participants to select from a list of statements the one that best describes how they have been feeling throughout the last 2 weeks. Accordingly, the BDI-II includes statements such as “I am so sad or unhappy that I can't stand it” to indicate depression, and related normal mood statements such as “I don't criticize or blame myself more than usual.”
Data analysis
It is worth highlighting that our research is based on correlational data, and it is a well-known truism that correlations do not guarantee the presence of causation (Fiedler et al., 2011). Likewise, the absence of temporal precedence as is typically found in correlational study designs further complicates matters and in the case of 3-variable mediation models it is impossible to statistically distinguish multiple competing causal hypotheses (Kline, 2015). While these statements offer good guidance about the need to exercise caution when working with causal models and correlational data, they are also often misconstrued as making the stronger claim that we can never reasonably infer causation from correlational data or use cross-sectional correlational data to examine any form of mediation model. As argued by Simon (1954), it is indeed quite reasonable, though certainly not conclusive, to infer the causal ordering of a set of correlational data as long as we (1) include at least one additional relevant variable, and (2) can apply specific a priori assumptions that constrain which of these variables do not involve a direct causal relation. This method, though argued only with respect to equations involving three variables by Simon (1954), is foundational to the process of path analyses in structural equation modeling—the statistical technique employed in our previous paper and again here. Shipley (1999) also makes a very similar argument with respect to structural equation modeling, noting that “although correlation does not imply causation, causation does necessarily imply particular types of zero-order correlations or partial correlations as well as other constraints on the patterns of covariation between the variables” (p. 377; emphasis added). This is to say that, given a sufficiently complex set of variables and clear causal hypotheses about how they ought to relate to one another, it is possible to test these hypotheses by comparing a theoretically constrained covariation matrix against the observed patterns of covariation. If the causal hypotheses are true, the theoretically derived covariation matrix should correspond to the observed covariations, and yield good model fit. When competing causal hypotheses require different patterns of covariation, it likewise becomes possible to test which hypotheses, if any, provide sufficient correspondence to the observed covariation matrix. Of course, in this case, the otherwise valuable inclusion of temporal precedence to the order of data collection would make testing differing causal hypotheses on the same data more problematic. Therefore, assuming the amount of time and research funds is held constant, a researcher's study design options are limited to either collecting a single larger sample of data, which is generally preferable for minimizing chance model over specification, or multiple smaller samples of data including different patterns of temporal precedence. The former approach was precisely the case for our previous research on the Attention-to-Affect model (Carriere et al., 2008), which sufficiently explained the observed covariations among the tendency to be inattentive, to make attention-related mistakes in everyday life, and to experience depression. The similarly theoretically constrained covariation matrix of the Affect-to-Attention model, which involved a reversal in the causal role of depression, could not adequately explain the observed covariation matrix, however, suggesting its underlying causal hypothesis was flawed. In the present study we will employ the same analysis method, while continuing to expand the complexity of the potential mediation process, and exploit similar expectancies of covariation and non-covariation among the cross-sectionally measured constructs in order to evaluate and contrast the Attention-to Affect and Affect-to-Attention models. All analyses were run using jamovi version 2.3.21. Path analyses were performed via the pathj module with normal Maximum Likelihood estimation and structural equation models discussed in the Latent Variable Path Analyses in the Supplementary Figures S1, S2 were run via the SEMLj module, also using normal Maximum Likelihood estimation.
Results and discussion
Consistent with our earlier findings (Cheyne et al., 2006; Carriere et al., 2008), the ARCES and MAAS-LO were found to have good distributional and psychometric properties. All measures showed a good range of scores, no significant deviations from normality in skewness and kurtosis, and demonstrated very satisfactory internal consistency (see Supplementary Table S1, for detailed information).
Pearson Product-Moment correlation coefficients are presented in Table 1. All observed coefficients were moderate to large. As predicted, the ARCES and MAAS-LO were positively correlated, and both were negatively correlated with the GSE. Furthermore, as predicted, the GSE was also negatively associated with the BDI-II. Overall, the correlations between attentional and mood measures replicated our previous findings (Carriere et al., 2008).
Our primary interest was in extending our knowledge about the role that everyday attention lapses play in the onset of depression. Hence, a model was constructed which allowed self-efficacy to mediate the relations from everyday inattention (the MAAS-LO) and attention-related errors (the ARCES) to depression (the BDI-II). To evaluate the conventional Affect-to-Attention hypothesis we simply reversed the causal paths involving depression, producing a model in which depression predicts inattention and self-efficacy.
The Attention-to-Affect model assessed the simultaneous effects of the MAAS-LO and GSE on the BDI-II, using the MAAS-LO as an exogenous variable predicting the ARCES, GSE and BDI-II. The GSE was also entered as a mediator of the effect of the MAAS-LO on the BDI-II, and the ARCES was entered as a mediator of the effect of the MAAS-LO on the GSE. This model provided reasonably good fit indices for the data, χ2 (1, N = 188) = 2.05, p = 0.152, CFI = 0.995, TLI = 0.972, RMSEA = 0.075, consistent with the Attention-to-Affect hypothesis, via changes in self-efficacy. As anticipated based on previous findings (Carriere et al., 2008) the direct path between the ARCES and BDI-II was found to be non-significant (b* = 0.105, p = 0.156) and omitted. The only notable shortcoming of this model was that the path from the MAAS-LO to the GSE was not significant (b* = −0.027, p = 0.772). This is because almost the entirety of the MAAS-LO's relation with the GSE was mediated by the ARCES in this sample—consistent with the hypothesis that one's everyday inattention leads to a decreased sense of self-efficacy primarily through the attention-related cognitive errors that also result from attention lapses. Accordingly, a more parsimonious model, removing the non-significant path from the MAAS-LO to the GSE, is shown in Figure 1A and provides very good fit indices for the data, χ2 (2, N = 188) = 2.15, p = 0.341, CFI = 0.999, TLI = 0.998, RMSEA = 0.020. For all direct and indirect effects see Supplementary Table S2.
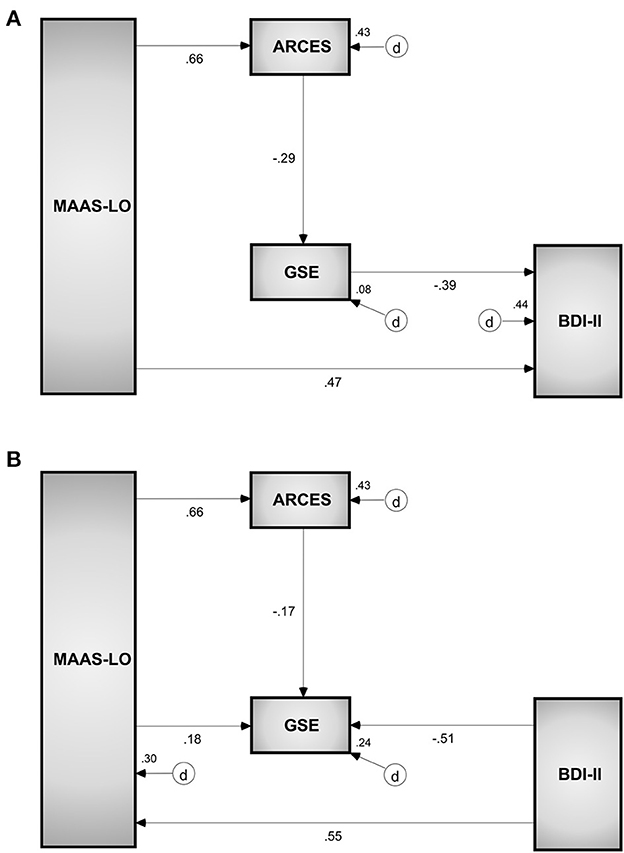
Figure 1. (A) Attention-to-affect path model with path coefficients for self-reported attention (MAAS-LO), error (ARCES) and self-efficacy (GSE) measures predicting depression (BDI-II). (B) Affect-to-attention path model with self-reported depression (BDI-II) predicting attention (MAAS-LO) and self-efficacy (GSE).
The traditional Affect-to-Attention model was tested next. This model treats the BDI-II as an exogenous variable predicting both the MAAS-LO and GSE, and with the MAAS-LO mediating the relation of the BDI-II and ARCES. The Affect-to-Attention model, which implicitly directs most research, does not specifically predict a direct influence of self-efficacy on attention-related errors—that is, without influencing attention lapses first—so in the Affect-to-Attention model that we tested the direction of the path between the ARCES and GSE remained consistent with the model shown in Figure 1A. Accordingly, the Affect-to-Attention model shown in Figure 1B matches Figure 1A, except that all paths directly connecting with the BDI-II are reversed. This model provided much poorer fit indices for the data, χ2 (1, N = 188) = 6.61, p = 0.010, CFI = 0.976, TLI = 0.854, RMSEA = 0.173. For all direct and indirect effects see Supplementary Table S3. Importantly, this model, which most closely matches the conventional Affect-to-Attention hypothesis, clearly does a poor job of representing the obtained pattern of relations and thus the implication is that conventional theories may be inadequate. Furthermore, this model requires a direct connection from the MAAS-LO to the GSE in order to better explain the observed covariance matrix, so is also potentially less parsimonious than the Attention-to-Affect model. A large source of variance left unaccounted for in the traditional Affect-to-Attention model involved the relation of the BDI-II with the ARCES, suggesting that, ultimately, the Affect-to-Attention model is not as effective in predicting the occurrence of attention-related errors as the Attention-to-Affect model is in predicting depression.
Given the present findings, the Attention-to-Affect model, shown in Figure 1A, best and most parsimoniously fits the data. Within this model, the MAAS-LO predicts all cognitive and affective variables, with the GSE mediating relations between the MAAS-LO and BDI-II. Moreover, in conjunction with attention-related cognitive errors (e.g., going to the fridge to get some milk, and instead taking out the juice), such attention lapses may be viewed as influencing our affective wellbeing via their impact on our sense of self-efficacy. Thus, the present findings once again highlight that maintaining an awareness of our actions is an important contributor not only to the outcomes of everyday activities, but potentially to our long-term emotional wellbeing.
The Attention-to-Affect model naturally focuses more on cognitive factors (i.e., attention lapses, attention-related errors, and self-efficacy) rather than affective factors (depression). Although we have thus far stressed these cognitive routes, we also acknowledge the existence of other affective mechanisms on the route from attention lapses to depression. One such potential linking mechanism is stress, particularly given that attention lapses can interfere with our ability to perform our normal, everyday activities and through such interference we may become stressed.
Study 2: evaluating a theoretical model linking attention lapses and stress
Here we adopt Selye's (1984) belief that the term stress should be reserved to describe physiological or emotional outcomes while the term stressors should be used to describe those events that cause stress. This view of stress as an emotional outcome is compatible with the work of Lazarus (1993), who argued that stress can be seen as a cognitive-emotional trait which persists across situations and remains present even in the absence of external stressors. On this view, there may sometimes be no identifiable situational cause for an individual's stress; that is, stress is simply an abundance of psychological tension, arousal, and other non-specific emotional distress resulting from no particularly salient cause. A similar view was put forward by Selye (1984), who argued that stress is a potential consequence of almost all physical, cognitive, or emotional activity—that essentially everything we do or feel has the potential to produce a stress response in the body, even if only to a very minimal extent. Accordingly, to say someone is feeling stressed reflects the individual's recognition of an abundance of the stress response rather than a truly categorical change in his or her present state. Expanding on the views of Lazarus (1993), we view stress more generally as a host of unpleasant affective traits, such as general irritability, that attention lapses may help to create, and which then potentially produce further emotional distress in the form of depressive affect. The challenge, accordingly, is to determine how stress fits into the Attention-to-Affect model.
Stress and attention
Viewing stress as emotional reactivity to life in general parallels the mechanism described in “daily hassles” research (e.g., Monroe, 1983; McIntyre et al., 2008), which provides an indirect link between attention lapses and stress. We would take this view one step further, however, to suggest that chronic inattention directly serves as a stressor, perhaps in part because inattention makes it more difficult to accomplish a variety of everyday and otherwise easy-to-accomplish tasks (e.g., reading; Smallwood and Schooler, 2006). In this way, if everyday inattention happens to have extraordinary consequences, such as narrowly avoiding a plane crash, it will likely produce sufficient stress to become noticeable, and those consequences would be easily identifiable as a stressor. If we experience an extraordinary abundance of minor attention-related errors in everyday life, however, then over time our stress level could also become sufficiently elevated to be perceived as bothersome, but we would not be able to identify the cause of our stress. Of course, it is also possible that inattention requires neither extraordinary nor minor consequences in order to produce stress, since the act of being inattentive could itself serve as a stressor—as was suggested by Selye (1984).
Stress and depression
Unlike the link between attention and stress, the link between stress and depression is well documented. Indeed, stress has long been identified as an important contributor to disease in general and is thought to be an especially important factor in the initial onset and later relapse into depression (Depue, 1979). Most notably, the contributions of stressors to depression are fundamental to the diathesis-stress model of depression. Such models are based on the theory that dysfunctional beliefs or behaviors tend to produce depression only when accompanied by stressful life events (for an interesting evaluation of diathesis-stress models of depression, see Robins and Block, 1989). The development of depression after stressful events may be further influenced by the tendency to experience chronic stress (Hammen et al., 2009), and even mild levels of daily stress appear to have the potential to produce mood disturbance over short timescales (DeLongis et al., 1988). These latter examples are more akin to the experience of general psychological stress we argue could be related to inattention and help bridge the gap with the diathesis-stress model of depression. A similar general experience of personal and interpersonal psychological stress was included in a structural model used by Shen and Takeuchi (2001) to understand the development of depression among Chinese American immigrants, and they found strong prediction of depression via stress. It is worth noting that decisions made while one is depressed may also create an environment in which the probability of experiencing future stress is increased (Hammen and Shih, 2008), thus creating a potentially vicious emotional downward spiral. Such complex interactions suggest a feedback model should be necessary to explain the relation between stress and depression but, interestingly, a reanalysis of Shen and Takeuchi's (2001) data set has shown that the reciprocal effect of depression is limited at best (Kline, 2005) and so a unidirectional relation will likely be sufficient in most cases. While none of the above studies have considered the potential role of everyday inattention in producing stress, it is nonetheless clear that, if the Attention-to-Affect hypothesis is correct, stress should play a pivotal role as a mediator between inattention and depression.
Self-efficacy and coping with stress
Attempts to minimize or avoid the effects of stress on our lives have been collectively described as coping, and generally fall into two categories: emotion focused coping, whereby the individual attempts to reappraise the situation in a more benign way, and problem focused coping, where the individual attempts to change the situation (Coyne and Lazarus, 1980; Coyne et al., 1981). Both coping strategies are based on an initial appraisal of the situation and one's abilities, followed by subsequent reappraisals after initial outcomes have been assessed. As such, a stress–coping feedback loop is created, in which the apparent causal flow is dependent on how early one breaks into the process (Coyne et al., 1981). Since general self-efficacy is essentially a belief in our ability to handle unforeseen situations, it makes sense to think it would play an important role at the earliest stages of this cycle. Indeed, a number of recent studies have shown the importance of self-efficacy in reducing stress and maintaining general mental health (e.g., Jerusalem and Hessling, 2009; Rees and Freeman, 2009; Nauta et al., 2010) or preventing job stress and burnout (Schwarzer and Hallum, 2008) when individuals encounter stressful situations. Thus, consistent with previous findings (Study 1), we again hypothesized that attention-related errors would predict self-efficacy, which, based on existing coping theory, we initially hypothesize would then also predict stress. It is worth noting, however, that the Attention-to-Affect hypothesis itself affords no specific causal predictions on which of stress and self-efficacy should be impacted prior to the other so the reverse causal relation is certainly possible.
Predictions and models
Guided by the above theoretical and empirical considerations, and to further investigate routes through which attention lapses and their associated errors influence depressive affect, Study 2 used stress as a partial mediator of these relations. In this study inattention was again measured via the Mindful Attention Awareness Scale–Lapses Only (MAAS-LO; Carriere et al., 2008) and errors resulting from inattention were measured by the Attention-Related Cognitive Errors Scale (ARCES; Carriere et al., 2008). As well, self-efficacy was again measured via the General Self-Efficacy scale (GSE; Schwarzer and Jerusalem, 1995). For this study stress and depression were both measured via their respective subscales on the Depression Anxiety Stress Scales (DASS; Lovibond and Lovibond, 1995). Based on our previous findings using the BDI-II as our measure of depression, we predicted the MAAS-LO would explain a significant amount of the variance in DASS-Depression, and that stress would play an important role as a partial mediator of this relation. Furthermore, as a measure of behavioral consequences of inattention, the ARCES should act primarily as a partial mediator between the MAAS-LO and DASS-Stress. As a result, the ARCES would not explain a significant amount of variance in DASS-Depression once relations with the MAAS-LO and DASS-Stress were accounted for. In this case, both the ARCES and DASS-Stress play a critical role in allowing key a priori causal constraints to be placed on the model.
Method
Participants
Participants were 399 undergraduate students (137 males) from the University of Waterloo, who completed a series of online questionnaires examining cognitive functioning and general emotional experience, including the measures of interest for this study. The data were compiled over three consecutive terms, in order to reach a sufficient sample size. Of those participants who provided their age, the mean was 20.8 (SD = 4.85; n = 392). The selected participants completed all four questionnaires and had no more than two missing responses from any questionnaire; 355 participants had zero missing responses. As compensation for their time, participants received partial course credit.
Measures
The majority of the questionnaires used in Study 1 were retained for the present study. These included the MAAS-LO, ARCES, and GSE. New to the present study was the short form of the Depression Anxiety Stress Scales (DASS; Lovibond and Lovibond, 1995). All questionnaires were completed in random order across participants, and item mean scores were calculated for each questionnaire in order to accommodate the occasional occurrence of response omissions.
Stress and depression were measured via the relevant subscales of the DASS, as it provides good discrimination between depression, anxiety, and stress, and has been shown to provide good long-term stability for each subscale (Lovibond, 1998). The 21-item DASS includes 7 questions for each subscale, asking about one's experiences over the past week, and is scored using a Likert scale ranging from did not apply to me at all (0) to applied to me very much, or most of the time (3). Response values are typically doubled in the short form of the DASS, in order to retain total score compatibility with the long form which has 42 items. No doubling was necessary for the present study, however, given that mean item scores were used and that the scaling is irrelevant for correlations in any case. To measure negative affect (depression) the DASS includes statements such as “I felt downhearted and blue” and “I couldn't seem to experience any positive feelings at all,” while to measure tension (stress) it includes statements such as “I found myself getting agitated” and “I tended to over-react to situations.” While the DASS does not attempt to address the DSM-IV (or DSM-V) criteria for depression (American Psychiatric Association, 1994, 2013), its depression subscale has nonetheless been shown to correlate strongly with the previous gold standard measures, the BDI (Lovibond and Lovibond, 1995; Antony et al., 1998) and BDI-II (Gloster et al., 2008), the latter having been used in our previous models. Furthermore, for the purposes of the present study, we find the clearer focus of the DASS on the simpler and more common experience of negative affect particularly appealing as we expect inattention should predict emotional distress in general, regardless of its severity.
Results and discussion
Once again, all measures were found to have good distributional and psychometric properties, with only the GSE reflecting a larger degree of kurtosis (though still acceptable, for full details see Supplementary Table S4). Pearson Product-Moment correlation coefficients are presented in Table 2. All coefficients were significant, and the majority was moderate to large. As predicted by the theory discussed earlier, both the MAAS-LO and ARCES show strong relations with DASS-Stress. Furthermore, consistent with the findings of Study 1 and the present theory, the GSE showed strong relations with the DASS-Stress, and DASS-Depression.
Attention-to-affect model
The hypothesis that attention lapses, and their resulting cognitive errors, would predict depression in part through their influence on our general sense of self-efficacy and stress level was addressed using structural equation modeling. Based on stress coping theory and recent findings that self-efficacy helps prevent a variety of negative mental health outcomes, this also involved adding a direct causal path from the GSE to DASS-Stress. Consistent with the hypothesis that attention lapses produce depression via changes in self-efficacy and stress, this model, shown in Figure 2A, provided good fit for the data, χ2 (2, N = 399) = 4.29, p = 0.116, CFI = 0.996, TLI = 0.980, RMSEA = 0.054. In this model the ARCES was found to partially mediate the relation of the MAAS-LO with DASS-Stress, with both direct and indirect effects remaining significant (see Supplementary Table S5 for all direct and indirect effects).
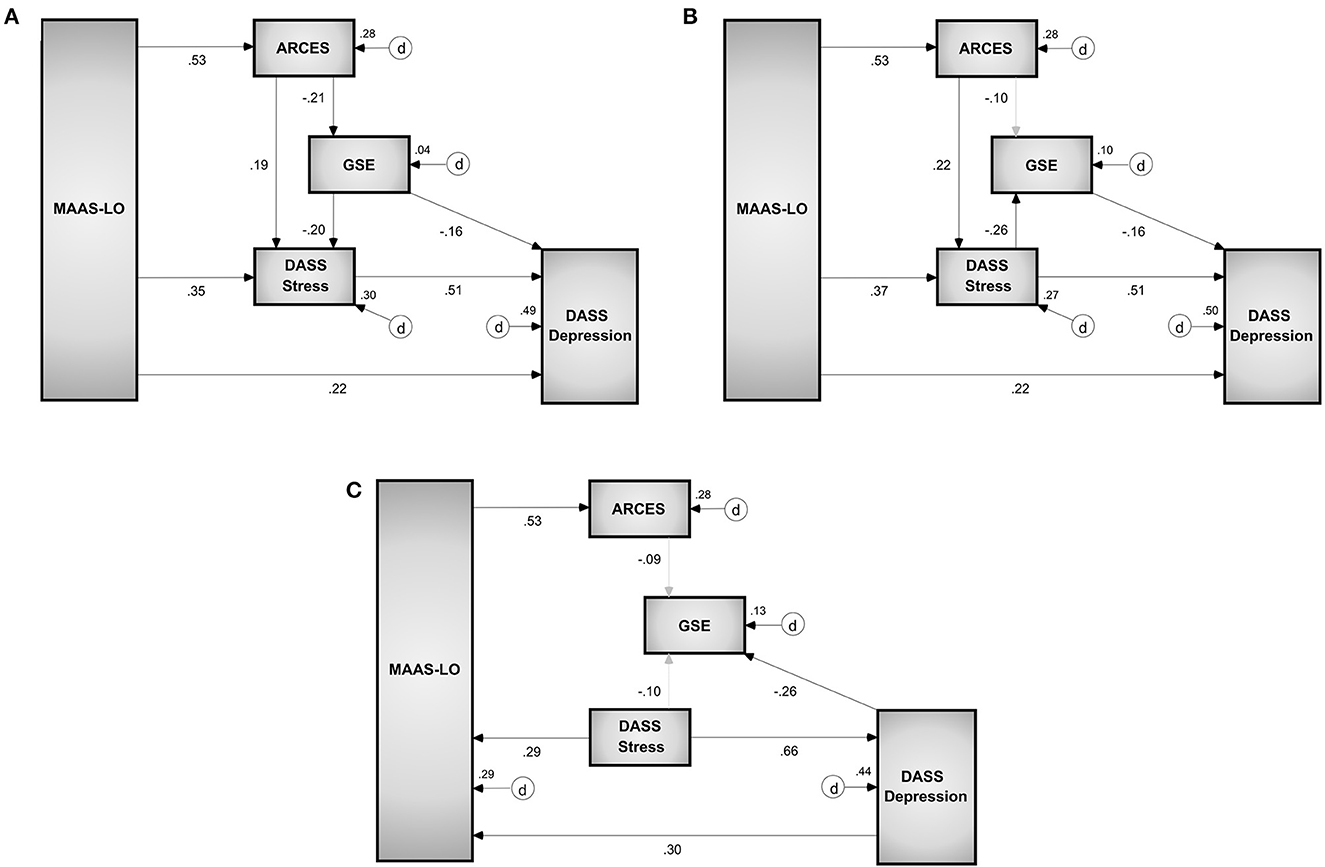
Figure 2. (A) Attention-to-affect path model with path coefficients for self-reported attention (MAAS-LO), error (ARCES), stress (DASS-stress), and self-efficacy (GSE) measures predicting depression (DASS-depression). (B) Attention-to-affect model with stress predicting self-efficacy. (C) Affect-to-attention path model with self-reported depression predicting attention and self-efficacy. Non-significant paths are shown in gray.
In Study 1 we found the relation of the MAAS-LO with the GSE was fully explained by the ARCES. The present model therefore assumed this path should be omitted. The results showed the largest residual covariance was between the MAAS-LO and the GSE, although it was within acceptable levels and, if added, the direct path coefficient was small and not significant (b* = −0.10, p = 0.119), resulting in only a small improvement in overall model fit. Accordingly, through this replication we can now be confident that the effect of inattention on self-efficacy is due to the fact that attention lapses lead us to make attention-related errors when completing everyday tasks. That is, it is only when we notice the negative effect inattention has on completing tasks that our sense of self-efficacy is diminished. Interestingly, there was a more parsimonious way to account for the residual covariance of the MAAS-LO and GSE in this model, which also adds an alternative view of the relation between inattention and self-efficacy. Based on the reviewed stress coping theory, for the Figure 2A we placed a path from the GSE to DASS-Stress. Reversing this direction, however, allows both the ARCES and DASS-Stress to function as mediators of the relation between the MAAS-LO and GSE, and is consistent with the alternative view that stress is likely to first contribute to a diminished sense of self-efficacy. Shown in Figure 2B, this revised Attention-to-Affect model has relatively little impact on most path coefficients, though the direct effect of the ARCES on the GSE is substantially diminished and only marginally significant (see Supplementary Table S6 for all direct and indirect effects). This model is, notably, equally consistent with the Attention-to-Affect hypothesis that attention lapses produce depression via changes in self-efficacy and stress, and provided good fit for the data, χ2 (2, N = 399) = 1.27, p = 0.529, CFI = 1.00, TLI = 1.01, RMSEA = 0.000. Thus, the present data are most consistent with the view that both inattention-induced stress and attention-related mistakes in everyday life generally lead one to experience a reduced sense of self-efficacy, prior to the onset of depression.
Affect-to-attention model
The present study substantially increased the complexity of our proposed Attention-to-Affect model and, accordingly, also any proposed alternative Attention-to-Affect models. It is perhaps unlikely that even among experts in clinical psychology a single alternative model could be easily agreed upon based on the existing literature alone. Some alternative models may rival the fit of our Attention-to-Affect model, but we suggest they would likely do so by subtly misrepresenting the currently held theories about how attention and affect are related with respect to self-efficacy and stress. Of course, other alternative Affect-to-Attention models may represent theory well, but provide poorer model fit and thus continue to call the underlying theory into question. It is not our claim to have addressed all potential alternatives, nor our desire to overfit the data in order to produce the best possible alternative model as a comparison. Rather, we have attempted to test our best estimate of a theoretically defensible alternative Affect-to-Attention model for all the measures included in this study.
In Figure 2C we present an Affect-to-Attention model that treats DASS-Stress as the sole exogenous variable, and provided poor overall fit to the observed data, χ2 (3, N = 399) = 19.2, p < 0.001, CFI = 0.972, TLI = 0.905, RMSEA = 0.116. In this model the direct path from DASS-Stress to the GSE is not significant (p = 0.081), suggesting most of its influence is via DASS-Depression (see Supplementary Table S7 for all direct and indirect effects). Removing this path does not, of course, substantially improve model fit. Indeed, the largest sources of residual covariance in this model are in the relations between the ARCES, DASS-Stress, and DASS-Depression. Critically, we are not aware of any theoretical reason to believe either stress or depression could produce attention-related cognitive errors except indirectly through their effect on attention or perhaps some other more action-oriented third variable, which, notably, the more parsimonious Attention-to-Affect model does not require. Accordingly, there is no theoretically-defensible way to add direct causal connections from either stress or depression to attention-related cognitive errors. Overall, the present findings suggest that, once again, the Affect-to-Attention model is not as effective in predicting attention-related errors as the Attention-to-Affect model is in predicting depression and, therefore, the importance of everyday inattention to the experience of depression is worthy of greater consideration.
General discussion
Starting from our earlier model (Carriere et al., 2008), which postulated attention lapses as a common cause of a sequence of cognitive, behavioral, and affective outcomes, the present studies were designed to investigate additional potential intermediates between attention lapses and affective dysfunction. The addition of self-efficacy as a collection of beliefs about our ability to perform everyday tasks (Study 1) and stress as an individual tendency to experience an unpleasant abundance of tension, arousal and general emotional distress (Study 2) allowed the expansion of our causal model from initial attention failures to affective outcomes. Once again, the present findings provided good support for the view of attention lapses as a cause of depression. The present findings are also consistent with a view of attention lapses as potential stressors in their own right—capable of producing stress without the consequent major life events more typically identified as causes of stress. There remains the distinct possibility, however, that everyday inattention also increases the likelihood that we encounter difficult life situations, and so stressful life events are still an important component of the attention–stress relation. As we did not specifically inquire about these participants' experiences of stressful life events, the present findings cannot directly address this question.
It is worthwhile highlighting once again that relation of the MAAS-LO with the GSE was fully explained by the ARCES in both Study 1 and Study 2. Accordingly, we conclude that reduced self-efficacy results from attention lapses that lead us to make attention-related errors when completing everyday tasks, although stress may play an important role in this process as well. That is, it is only when we either experience stress or notice the negative effect inattention has on completing tasks, that our sense of self-efficacy is diminished. Thus, future studies looking to model the effect of inattention on self-efficacy should incorporate at least attention-related errors as a mediator of this relation.
A limitation of the present models is that they rely solely on relations observed among self-report questionnaire assessments of attention and emotion. This limitation is a consequence of the present research focusing on trait-level tendencies of the individual. That is, we investigated general tendencies to be inattentive, stressed, or depressed in everyday life regardless of specific situations one might encounter. Taking these general tendencies to the level of specific situations may not be easily accomplished because any given situation will inevitably introduce its own complexities. Such complexities are often unexpected and difficult to account for, and thus correct interpretation of the results is made more difficult. For example, it is probable that, although attention lapses may serve an important role in the etiology of depression, depressed affect will be associated with self-focused depressive rumination that provides additional attentional load and is associated with mind-wandering away from important characteristics of the task at hand (Smallwood et al., 2003, 2007)—and this process is likely to play out over both long and short timescales. Indeed, real-life scenarios are likely to involve reciprocal relations between trait inattention, trait and situational stress, and depression where a general tendency toward inattention increases the likelihood of stressful events occurring (such as in the example of the distracted Chatsworth train engineer from the introduction) and stressful events then lead to depression, both of which create new cognitive loads that make maintaining attention even more difficult, and the cycle repeats. Likewise, stressful life events could be responsible for the creation of short-term attentional difficulties and depression, and once these events are resolved the other effects will not necessarily persist. Notably, the studies discussed here, being focused solely on trait-level cognitive and emotional processes, do not preclude any such possibilities. What they do suggest is that everyday attentiveness should serve as a protective factor, minimizing the potential impact of traumatic life events, and that with careful control and interpretation, future studies might benefit from including measures of attention lapses and an attempt to better understand the long-term cognitive, emotional and behavioral interactions that play an important role in the onset and maintenance of depression. In the end, that several different studies have produced similar findings, even while involving different mediators of the link between attention lapses and affective distress, should make their findings more compelling. Accordingly, the results obtained in the present studies should be interpreted as a stimulus for additional research on the potential involvement of seemingly harmless lapses of attention in everyday life in the development of highly problematic emotional states.
It is worth noting that, although progressing down a somewhat different causal path, the present line of research is perfectly compatible with the findings of Killingsworth and Gilbert (2010). Through an experience sampling study these researchers have shown a significant relation between mind wandering in everyday situations and future happiness, with happiness reportedly decreasing following reports of mind wandering. The effect of mind wandering on happiness was independent of, and larger than, the emotional effect of the activity performed at the time. Furthermore, mind wandering was associated with decreased happiness when the content of that thought was either neutral or negative, relative to the same activity performed without mind wandering. Interestingly, mind wandering also did not lead to an increase in happiness when the topic was pleasant. Together these findings suggest mind wandering has a general negative effect on our emotional state, which may be only partially counteracted by thinking about pleasant topics. Of course, other slightly more recent work similarly examining the connection between mind wandering and negative mood produced contradictory results, where sadness predicted mind wandering but mind wandering did not predict sadness on short timescales (Poerio et al., 2013). Again, though similar in some respects to the present studies, these findings are based on reports of mind wandering rather than attention lapses or attention-related errors, and momentary happiness rather than depression over longer timescales. Nevertheless, there are obvious links between inattention and mind wandering, and between depression and momentary happiness, and so it is good to see some potential convergence on the same general conclusion in these separate lines of research.
On the whole, the present results provide good support for the hypothesis that chronic attention lapses may set in motion processes that ultimately lead to depression, including those working via their influence on our stress level. This process is likely to be, in part, also mediated by the effects of failures of attention on our general sense of our ability to accomplish both mundane and novel tasks. These models redefine the role of attention lapses in our everyday emotional experiences and are all the more compelling as a result of the consistency with which attempts to address the various causal relations underlying these experiences produce similarly well-fitting models. As a result, the present findings suggest that direct attentional training exercises could be an important, and presently underutilized, method of preventing onset or relapse of depressive episodes. Of course, the present research is only an initial foray in this domain, and it is worth noting again that the present models contrast the most simplified views of the interrelations among attention, self-efficacy, stress, and depression. We do not believe there is any debate over the validity of these interrelations, nor the belief that causality is involved in these relations. The Affect-to-Attention models we tested therefore represent what we believe are the most typical theoretical perspectives taken when discussing causation among these particular variables; those models failed to explain the data. The Attention-to-Affect models likewise represent our preferred alternative theoretical perspectives on the causal roles, after recognizing that seemingly minor inattentiveness is certainly capable of producing a wide range of major life consequences and that at least one study has already suggested it is possible to treat major depressive disorder with only attention training (Papageorgiou and Wells, 2000). But it is undoubtedly the case that in the real world the interrelations are more complex than any of our models allow. Indeed, the primary causal flow may be from attention-to-affect in general, but from affect-to-attention (particularly from stressors to inattention) in many real-world scenarios. It is clear there is a great deal of additional research to do with regard to testing even more potential pathways from either attention-to-affect or affect-to-attention (e.g., anxiety is likely to be another important variable) and we will not fully understand the connection between attention and affect without considering more complex alternatives from all plausible causal perspectives.
Data availability statement
The raw data supporting the conclusions of this article will be made available by the authors, without undue reservation.
Ethics statement
The studies involving human participants were reviewed and approved by University of Waterloo Research Ethics Board. The patients/participants provided their written informed consent to participate in this study.
Author contributions
JSAC is the main author, responsible for most data analysis, and writing. AN was responsible for data collection in Study 2 and assisting in developing the theoretical models. JAC and DS was a supervisor and assisted with proofreading and conceptual development of the models. All authors contributed to the article and approved the submitted version.
Funding
This work was funded by NSERC Discovery grant (RGPIN-2019-04071).
Conflict of interest
The authors declare that the research was conducted in the absence of any commercial or financial relationships that could be construed as a potential conflict of interest.
Publisher's note
All claims expressed in this article are solely those of the authors and do not necessarily represent those of their affiliated organizations, or those of the publisher, the editors and the reviewers. Any product that may be evaluated in this article, or claim that may be made by its manufacturer, is not guaranteed or endorsed by the publisher.
Supplementary material
The Supplementary Material for this article can be found online at: https://www.frontiersin.org/articles/10.3389/fcogn.2023.1125197/full#supplementary-material
References
American Psychiatric Association (1994). Diagnostic and Statistical Manual of Mental Disorders, 4th edn. Washington, DC: Author.
American Psychiatric Association (2013). Diagnostic and Statistical Manual of Mental Disorders, 5th edn. Washington, DC: Author. doi: 10.1176/appi.books.9780890425596
Antony, M., Bieling, P. J., Cox, B. J., Enns, M. W., and Swinson, R. P. (1998). Psychometric properties of the 42-item and 21-item versions of the Depression Anxiety Stress Scales in clinical groups and a community sample. Psychol. Assess. 10, 176–181. doi: 10.1037/1040-3590.10.2.176
Bandura, A. (1977). Self-efficacy: toward a unifying theory of behavioral change. Psychol. Rev. 84, 191–215. doi: 10.1037/0033-295X.84.2.191
Bandura, A. (1997). Self-Efficacy: The Exercise of Control. New York, NY: W. H. Freeman and Company.
Beck, A. T., Steer, R. A., and Brown, G. K. (1996). Manual for the Beck Depression Inventory-II. San Antonio, TX: The Psychological Corporation. doi: 10.1037/t00742-000
Brown, K. W., and Ryan, R. M. (2003). The benefits of being present: mindfulness and its role in psychological wellbeing. J. Person. Soc. Psychol. 84, 822–848. doi: 10.1037/0022-3514.84.4.822
Carriere, J. S. A., Cheyne, J. A., and Smilek, D. (2008). Everyday attention lapses and memory failures: the affective consequences of mindlessness. Consc. Cognit. 17, 835–847. doi: 10.1016/j.concog.2007.04.008
Cheyne, J. A., Carriere, J. S. A., and Smilek, D. (2006). Absent-mindedness: lapses of conscious awareness and everyday cognitive failures. Consc. Cognit. 15, 578–592. doi: 10.1016/j.concog.2005.11.009
Coyne, J. C., Aldwin, C., and Lazarus, R. S. (1981). Depression and coping in stressful episodes. J. Abnorm. Psychol. 90, 439–447. doi: 10.1037/0021-843X.90.5.439
Coyne, J. C., and Lazarus, R. S. (1980). “Cognitive style, stress perception, and coping,” in Handbook on Stress and Anxiety: Contemporary Knowledge, Theory, and Treatment, eds I. L. Kutash, and L. B. Schlesinger (San Francisco, CA: Jossey-Bass).
Dalgleish, T., and Watts, F. N. (1990). Biases of attention and memory in disorders of anxiety and depression. Clin. Psychol. Rev. 10, 589–604. doi: 10.1016/0272-7358(90)90098-U
Davis, F. W., and Yates, B. T. (1982). Self-efficacy expectancies vs. outcome expectancies as determinants of performance deficits and depressive affect. Cognit. Therapy Res. 6, 23–35. doi: 10.1007/BF01185724
DeLongis, A., Folkman, S., and Lazarus, R. S. (1988). The impact of daily stress on health and mood: psychological and social resources as mediators. J. Person. Soc. Psychol. 54, 486–495. doi: 10.1037/0022-3514.54.3.486
Fenske, M. J., and Eastwood, J. D. (2003). Modulation of focused attention by faces expressing emotion: evidence from flanker tasks. Emotion 3, 327–343. doi: 10.1037/1528-3542.3.4.327
Fenske, M. J., and Raymond, J. E. (2006). Affective influences of selected attention. Curr. Direct. Psychol. Sci. 15, 312–316. doi: 10.1111/j.1467-8721.2006.00459.x
Fiedler, K., Schott, M., and Thorsten, M. (2011). What mediation analysis can (not) do. J. Exp. Soc. Psychol. 47, 1231–1236. doi: 10.1016/j.jesp.2011.05.007
Gloster, A. T., Rhoades, H. M., Novy, D., Klotsche, J., Senior, A., Kunik, M., et al. (2008). Psychometric properties of the depression anxiety and stress scale-21 in older primary care patients. J. Affect. Disord. 110, 248–259. doi: 10.1016/j.jad.2008.01.023
Gotlib, I. H., McLachlan, A. L., and Katz, A. N. (1988). Biases in visual attention in depressed and non-depressed individuals. Cognit. Emot. 2, 185–200. doi: 10.1080/02699938808410923
Hammen, C., Kim, E. Y., Eberhart, N. K., and Brennan, P. A. (2009). Chronic and acute stress and the prediction of major depression in women. Depress. Anxiety 26, 718–723. doi: 10.1002/da.20571
Hammen, C., and Shih, J. H. (2008). “Stress generation and depression,” in Risk Factors in Depression, eds K. S. Dobson, and D. J. A. Dozois (New York, NY: Academic Press), 409–428. doi: 10.1016/B978-0-08-045078-0.00018-6
Ingram, R. E., Steidtmann, D. K., and Bistricky, S. L. (2008). “Information processing: attention and memory,” in Risk Factors in Depression, eds K. S. Dobson, and D. J. A. Dozois (New York, NY: Academic Press), 145–169. doi: 10.1016/B978-0-08-045078-0.00007-1
Jerusalem, M., and Hessling, J. K. (2009). Mental health promotion in schools by strengthening self-efficacy. Health Educ. 109, 329–341. doi: 10.1108/09654280910970901
Killingsworth, M. A., and Gilbert, D. T. (2010). A wandering mind is an unhappy mind. Science 330, 932. doi: 10.1126/science.1192439
Kline, R. B. (2005). Principles and Practice of Structural Equation Modeling. New York, NY: The Guildford Press.
Kline, R. B. (2015). The mediation myth. Basic Appl. Soc. Psychol. 37, 202–213. doi: 10.1080/01973533.2015.1049349
Lazarus, R. S. (1993). From psychological stress to the emotions: a history of changing outlooks. Annu. Rev. Psychol. 44, 1–21. doi: 10.1146/annurev.ps.44.020193.000245
Lovibond, P. F. (1998). Long-term stability of depression, anxiety, and stress syndromes. J. Abnorm. Psychol. 107, 520–526.
Lovibond, P. F., and Lovibond, S. H. (1995). The structure of negative emotional states: comparison of the depression anxiety stress scales (DASS) with the beck depression and anxiety inventories. Behav. Res. Therapy 33, 335–343. doi: 10.1016/0005-7967(94)00075-U
MacLeod, C., Mathews, A., and Tata, P. (1986). Attentional bias in emotional disorders. J. Abnorm. Psychol. 95, 15–20. doi: 10.1037/0021-843X.95.1.15
MacLeod, C., Rutherford, E., Campbell, L., Ebsworthy, G., and Holker, L. (2002). Selective attention and emotional vulnerability: assessing the causal basis of their association through experimental manipulation of attentional bias. J. Abnorm. Psychol. 11, 107–123. doi: 10.1037/0021-843X.111.1.107
McIntyre, K. P., Korn, J. H., and Matsuo, H. (2008). Sweating the small stuff: How different types of hassles result in the experience of stress. Stress Health 24, 383–392. doi: 10.1002/smi.1190
Mogg, K., Bradley, B. P., and Williams, R. (1995). Attentional bias in anxiety and depression: the role of awareness. Br. J. Clin. Psychol. 34, 17–36. doi: 10.1111/j.2044-8260.1995.tb01434.x
Monroe, S. M. (1983). Major and minor life events as predictors of psychological distress: further issues and findings. J. Behav. Med. 6, 189–205. doi: 10.1007/BF00845380
National Transportation Safety Board (2008). Collision of Metrolink Train 111 with Union Pacific Freight Train LOF65-12: RAR-10/01. Washington, DC: National Transportation Safety Board. Available online at: http://ntsb.gov/Publictn/2010/RAR1001.htm (accessed June 10, 2010).
Nauta, M. M., Liu, C., and Li, C. (2010). A cross-national examination of self-efficacy as a moderator of autonomy/job strain relationships. Appl. Psychol. Int. Rev. 59, 159–179. doi: 10.1111/j.1464-0597.2008.00375.x
Papageorgiou, C., and Wells, A. (2000). Treatment of recurrent major depression with attention training. Cognit. Behav. Pract. 7, 407–413. doi: 10.1016/S1077-7229(00)80051-6
Poerio, G. L., Totterdell, P., and Miles, E. (2013). Mind-wandering and negative mood: does one thing really lead to another? Consc. Cognit. 22, 1412–1421. doi: 10.1016/j.concog.2013.09.012
Reason, J. T. (1984). “Lapses of attention in everyday life,” in Varieties of Attention, eds R. Parasuraman and D. R. Davies (New York, NY: Academic Press).
Rees, T., and Freeman, P. (2009). Social support moderates the relationship between stressors and task performance through self-efficacy. J. Soc. Clin. Psychol. 28, 244–263. doi: 10.1521/jscp.2009.28.2.244
Robins, C. J., and Block, P. (1989). Cognitive theories of depression viewed from a diathesis-stress perspective: evaluations of the models of beck and of abramson, seligman, and teasdale. Cognit. Therapy Res. 13, 297–313. doi: 10.1007/BF01173475
Schwarzer, R., and Hallum, S. (2008). Perceived teacher self-efficacy as a predictor of job stress and burnout: mediation analyses. Appl. Psychol. Int. Rev. 57, 152–171. doi: 10.1111/j.1464-0597.2008.00359.x
Schwarzer, R., and Jerusalem, M. (1995). “Generalized self-efficacy scale,” in Measures in Health Psychology: A User's Portfolio. Causal and Control Beliefs, eds J. Weinman, S. Wright, and M. Johnston (Windsor: NFER-Nelson), 35–37.
Shen, B., and Takeuchi, D. T. (2001). A structural model of acculturation and mental health status among Chinese Americans. Am. J. Commun. Psychol. 29, 387–418. doi: 10.1023/A:1010338413293
Shipley, B. (1999). Testing causal explanations in organismal biology: causation, correlation and structural equation modelling. Oikos 86, 374–382. doi: 10.2307/3546455
Simon, H. A. (1954). Spurious correlation: a causal interpretation. J. Am. Stat. Assoc. 49, 467–479.
Smallwood, J., Obonsawin, M., Baracaia, S. F., Reid, H., O'Connor, R., and Heim, D. (2003). The relationship between rumination, dysphoria, and self-referent thinking: some preliminary findings. Imag. Cognit. Person. 22, 317–342. doi: 10.2190/2N80-AVM3-4A23-LEAJ
Smallwood, J., O'Connor, R. C, Sudbery, M. V., and Obonsawin, M. (2007). Mind-wandering and dysphoria. Cognit. Emot. 21, 816–842. doi: 10.1080/02699930600911531
Smallwood, J., and Schooler, J. W. (2006). The restless mind. Psychol. Bull. 132, 946–958. doi: 10.1037/0033-2909.132.6.946
Keywords: inattention, attention lapses, self-efficacy, stress, depression
Citation: Carriere JSA, Nelson AL, Cheyne JA and Smilek D (2023) Influences of inattention on perceived self-efficacy, stress, and depression. Front. Cognit. 2:1125197. doi: 10.3389/fcogn.2023.1125197
Received: 16 December 2022; Accepted: 30 March 2023;
Published: 25 April 2023.
Edited by:
Juan Lupiáñez, University of Granada, SpainReviewed by:
Luis Cásedas, University of Granada, SpainStefano Lasaponara, Sapienza University of Rome, Italy
Copyright © 2023 Carriere, Nelson, Cheyne and Smilek. This is an open-access article distributed under the terms of the Creative Commons Attribution License (CC BY). The use, distribution or reproduction in other forums is permitted, provided the original author(s) and the copyright owner(s) are credited and that the original publication in this journal is cited, in accordance with accepted academic practice. No use, distribution or reproduction is permitted which does not comply with these terms.
*Correspondence: Jonathan S. A. Carriere, am9uYXRoYW4uY2FycmllcmVAdWJpc2hvcHMuY2E=