- 1Department of Information Engineering (DII), University of Pisa, Pisa, Italy
- 2E. Piaggio Research Center, University of Pisa, Pisa, Italy
- 3Istituto di Linguistica Computazionale, CNR, Pisa, Pisa
Verbal communication is an expanding field in robotics showing a significant increase in both the industrial and research field. The application of verbal communication in robotics aims to reach a natural human-like interaction with robots. In this study, we investigated how salient terms related to verbal communication in robotics have evolved over the years, what are the topics that recur in the related literature, and what are their trends. The study is based on a computational linguistic analysis conducted on a database of 7,435 scientific publications over the last 2 decades. This comprehensive dataset was extracted from the Scopus database using specific key-words. Our results show how relevant terms of verbal communication evolved, which are the main coherent topics and how they have changed over the years. We highlighted positive and negative trends for the most coherent topics and the distribution over the years for the most significant ones. In particular, verbal communication resulted in being highly relevant for social robotics. Potentially, achieving natural verbal communication with a robot can have a great impact on the scientific, societal, and economic role of robotics in the future.
Introduction
Robots are becoming increasingly pervasive in our everyday life, entering our homes (Abdi et al., 2018; Van Patten et al., 2020), places of work (Robla-Gómez et al., 2017), hospitals (Azeta et al., 2017), and schools (Belpaeme et al., 2018). Since humans need to communicate and cooperate with these machines, and because we are accustomed to communicating with other people, the same social norms and kind of communication that apply to humans might also apply to robots. As Nass and colleagues demonstrated in their book “The Media Equation” (Reeves and Nass, 1996), people often respond socially to computers in ways similar to how they would interact socially with other people. Therefore, the need to develop a robot that can behave socially has pushed researchers to incorporate a form of communication similar to what humans use in the design, such as the non-verbal communication (Breazeal et al., 2005; Brooks and Arkin, 2007; Mutlu et al., 2009; Cominelli et al., 2018), as well as the verbal one (Nakamura and Sawada, 2006; Crowelly et al., 2009; Niculescu et al., 2013).
Although the role of non-verbal behaviors (Burgoon et al., 2016) is of undeniable importance, verbal communication has a primary role in human-human interaction. Indeed, the voice is one of the most powerful tools that mankind uses to convey emotions and intentions (Cowen et al., 2019) and language allows people to convey meaningful messages encoded in written or spoken words (Krauss, 2002). Therefore, developing conversational agents that can interact using natural language, be it for entertainment, controlled, or actioned, is of great interest. Moreover, spoken natural language interaction has some advantages compared to non-verbal language. It makes human-robot communication natural, accurate and efficient (Liu and Zhang, 2017) allowing for the possibility of the robot to cooperate, to be trained from non-expert humans, and to efficiently behave in a social environment (Mavridis, 2015).
Nevertheless, it is not yet possible to naturally communicate with a robot just as we do with other humans. There are several challenges which need to be addressed both from a technological and scientific point of view. For instance, robots still have difficulties in correctly capturing sound from distant speakers (Kumatani et al., 2012), dealing with environmental noise (Jensen et al., 2005), managing speech interruption from the user (i.e., barge-in problem (Huang et al., 2001)) and identifying the talking person when multiple users are present (Gomez et al., 2014). Moreover, the age of a user can also be considered to be an issue as there is a lack of speech recognition systems for children due to their pitch characteristics and speech disfluencies (Kennedy et al., 2017) and, elderly people might have dysarthria which might impair a regular communication flow (Kumar and Kumar, 2016).
Beyond the current technical limits of developing and improving this type of communication, there is an undeniable and significant increase of interest in verbal communication, testified by the last decade’s increase of publications related to the use of the voice in robots (Figure 1). A significant increasing trend is also observed in the industrial area. According to the International Federation of Robotics (IFR) figures, fields that are experiencing considerable growth are public relation robots and entertainment and leisure robots (IFR, 2018). The incorporation of speech recognition and speech generation abilities in robots have obtained encouraging results in several research fields such as educational robotics (Budiharto et al., 2017), collaborative robotics (Huang and Mutlu, 2016; Gustavsson et al., 2017), surgical robotics (Zinchenko et al., 2017), assistive robotics (Wu et al., 2014; Zhou et al., 2018), robot therapy (Barakova et al., 2015; Ramamurthy and Li, 2018), humanoid robotics (Ding and Shi, 2017), and navigation robotics (Draper et al., 2013; Schulz et al., 2015).
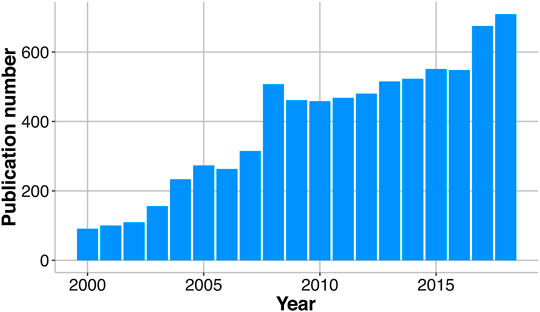
FIGURE 1. The annual amount of verbal communication robot-related publications since the year 2000. In the past 18 years, the number of publications gradually increased, reaching a maximum in current time.
The increasing number of publications has made it more difficult to understand and track advances in the field (Landhuis, 2016; Altbach and de Wit, 2019). Thus, the aim of this work is to discover promising trends in the verbal communication field by performing a deep and systematic analysis of the research literature. To avoid any bias and author subjectivity, different text mining techniques were used in a bottom-up approach to retrieve research fields and keywords from scientific publications. As observed from previous work, bibliometric techniques leverage statistics to successfully extract useful information such as the identification of the fundamental “pillars” that support a research theme (Buter and Van Raan, 2013), the discovery of promising trends in the robotics field (Goeldner et al., 2015; Mejia and Kajikawa, 2017), of topics in conversational content (Yeh et al., 2016) and of relationships between social and technology issues (Ittipanuvat et al., 2014).
Investigating the emergent topics and keywords from thousands of publications belonging to verbal communication in robotics throughout the last decades can reveal important hidden topics or technology domains. This information can be extremely valuable to drive future research to applications where technology and needs intersect. In particular, we address the following questions:
1. How did the salient terms related to verbal communication in robotics evolve?
2. Are there any specific applications that involved the use of verbal communication?
3. Do they have any noteworthy trends in the last decade?
4. If they do, what can these trends reveal?
The paper is structured as follows. In Section 1, the criteria used to select publications are introduced. In Section 2, the contrastive analysis is described. In Section 3, the pre-processing step is explained. In Section 4, it is illustrated how topics are modeled. Section 5 reports the model evaluation. Section 6 shows the topics evaluation. In the last sections (7, 8), we summarize the results and discuss possible future scenarios.
1 Selection Criteria
Publications related to the verbal communication in robotics were retrieved from Scopus using the following query: “TITLE-ABS-KEY ((voice OR speech OR verbal OR talk OR dialogue OR spoken OR conversation) AND robot) AND PUBYEAR >1999”. Since the main focus is on current trends and topics, the research was restricted only to works published from the year 2000. A total of 7,435 articles (titles and abstracts) were extracted on February 20, 2019. The framework of the natural language processing (NLP) tools used in this research is shown in Figure 2. The dataset can be accessed from the following GitHub repository https://github.com/vargas95/nlp_social_robotics.
2 Contrast Analysis
All publications were divided into four groups based on the publication year. Intervals range from 2000 to 2004, from 2005 to 2009, from 2010 to 2014, and from 2015 to 2019. In this way, specific keywords that are representative for a certain interval of time can be identified. The extraction of domain-specific terms denoting domain entities was performed using the NLP tool T2K (Sagri et al., 2019). By default, the automatically POS–tagged and lemmatized text is searched for candidate domain-specific terms, expressed by either single nominal terms or complex nominal structures with modifiers (adjectival and prepositional modifiers). To select the terms representative for a certain interval of time, a contrastive analysis was performed: the list of extracted terms was ranked with respect to the variation of the term frequency inverse document frequency (tf-idf) scores (Salton and Buckley, 1988) calculated for two different intervals of time. Two different contrastive analyses were performed: the analyzed interval of time 1) vs all other intervals and 2) only vs the past intervals. The contrastive analysis was performed for each group keeping only the first 19 main words. Results are displayed in Tables 1–4. It is worth noting that the contrast against past or all intervals is the same for the range
3 Pre-Processing
A series of pre-processing steps were applied to convert the text in a specific structure to subsequently perform text mining analysis. Specifically, texts are transformed into a document-term matrix, where each row represents one document, each column represents a term, and the associated value defines the term’s frequency. Then, the text was subjected to lemmatization, i.e., an algorithm to convert the word to its lemma based on its intended meaning. In particular, lemmatization represents a better choice compared to stemming for topic modeling as it tries to correctly identify the intended part of speech and meaning of a word in a sentence or in a document. After that, the raw text was converted in a corpus on which other common transformations were applied, such as tokenization, conversion to lower case, and the removal of numbers, punctuation and stop words. Lastly, once the corpus was converted into a document-term matrix, it was filtered using the tf-idf measure. In particular, this operation allows us to weight each word based on the following formulas:
The weight of a term is directly proportional to the number of times a term occurs within a document, but it is inversely proportional with the frequency of the term among the collection. In this way, tf-idf measures the importance of a term among a collection of documents. To further constrain the number of words, a threshold on the weights has been applied equal to the median of the tf-idf scores (Silge and Robinson, 2017).
The difference between non-filtered text and the one filtered with tf-idf is highlighted in Figure 3 and Figure 4, are the wordclouds displaying the most frequent words. It is clearly visible how words that are frequent in the non-filtered text such as “robot”, “human” and “system” become of secondary importance when text is filtered with tf-idf and more informative words such as “emotion”, “agent” and “dialogue” become relevant.
4 Topic Modeling
We retrieved topics using the Latent Dirichlet Allocation (LDA) method (Blei et al., 2003), which is a generative probabilistic model of a corpus. This algorithm takes advantage of the fact that every document is a mixture of latent topics and that each topic is characterized by a distribution over words. Although LDA is a generative process, it can be inverted using the Bayes rule in order to estimate a model’s parameters. From the document level, LDA can backtrack the topics that are likely to have generated the corpus thereby estimating the parameters’ uncertainty. The method is based on three main steps:
1. Randomly assign to each word in each document one of the K topics;
2. For each document d:
• Assume that all topics assignment, except this one, are correct;
• Compute the probability of the topic given that document:
• Compute the probability that the word belongs to a topic:
• Multiply these two probabilities and assign to the word a new topic based on that probability:
3. Continue until a steady state is reached.
The algorithm used in this work is the LDA method implemented in the R software, part of the “topicmodels” package (Hornik and Grün, 2011). As a sampling method, we selected the Gibbs method to infer unknowns from the data. Results of the LDA model consists of the a posteriori distributions:
• The probability β that a term belongs to each topic;
• The topic distribution
5 Model Evaluation
One limitation of the model is that it needs a priori the K number of topics. In order to find a “correct” number, four different metrics, which represent the goodness of the fit, can be visualized using the “ldatuning” package (Nikita, 2016). In particular, two categories of metrics can be distinguished. Then, the number of topics is selected as the one that:
• minimize the following measures:
• Arun 2010 (Arun et al., 2010)
• CaoJuan 2009 (Cao et al., 2009)
• maximize the following measures:
• Deveaud 2014 (Deveaud et al., 2014)
• Griffiths 2004 (Griffiths and Steyvers, 2004)
These measures select the best number of topics using a symmetric KL-divergence of salient distributions which are derived from the factorization of the document-term matrix. A graphic visualization of the metrics’ variation with respect to the number of topics is shown in Figure 5. It can be observed that the Deveaud2014 metric is not informative. Therefore, by analyzing the remaining three metrics, the correct number of topics might be located in the range between 60 and 150 topics. We selected, as a reasonable number, 60 topics which is the point where two of three metrics converge to their minimum or maximum point. Moreover, selecting the lower number of topics might avoid possible over-fitting.
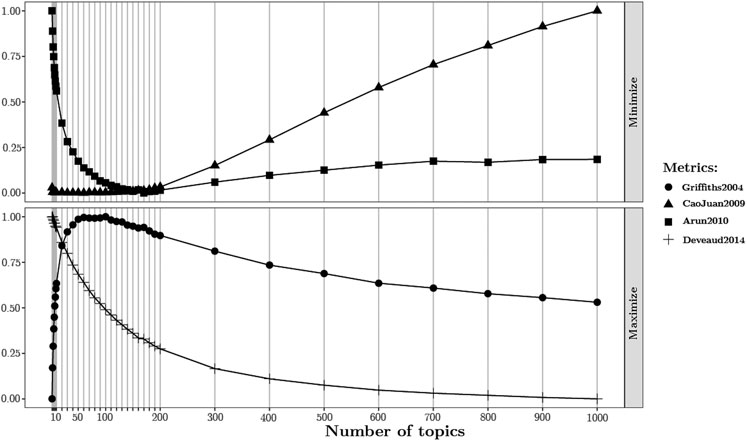
FIGURE 5. Variation of four metrics with respect to the number of topics. On the top, Arun2010 and CaoJuan2009 metrics have to be minimized. On the bottom, Deveaud2014 and Griffiths2004 have to be maximized. They define a range where the correct number of topics should be located.
6 Topics Evaluation
The LDA model is a powerful way to extract topics from a corpus of documents in an unsupervized way. However, topics might not be clearly explicable, therefore topic coherence can be used as a measure to evaluate the topic quality (coherence implementation in R (Denny, 2018)). The topic coherence metric considers the co-occurrences of words within the documents and it is defined as:
where
The topic coherence has been evaluated on the 60 topics extracted, by considering the top 10 terms for each topic and setting a user-defined threshold on the coherence value to -145. Consequently, 26 topics have been identified as top topics, which are displayed in Figure 6. The three top terms for each topic are highlighted, i.e., words which have the largest probabilities to belong to that topic. A qualitative analysis of the keywords allows us to partition topics in five supra-categories: control, application, language, interaction, signal, and hardware.
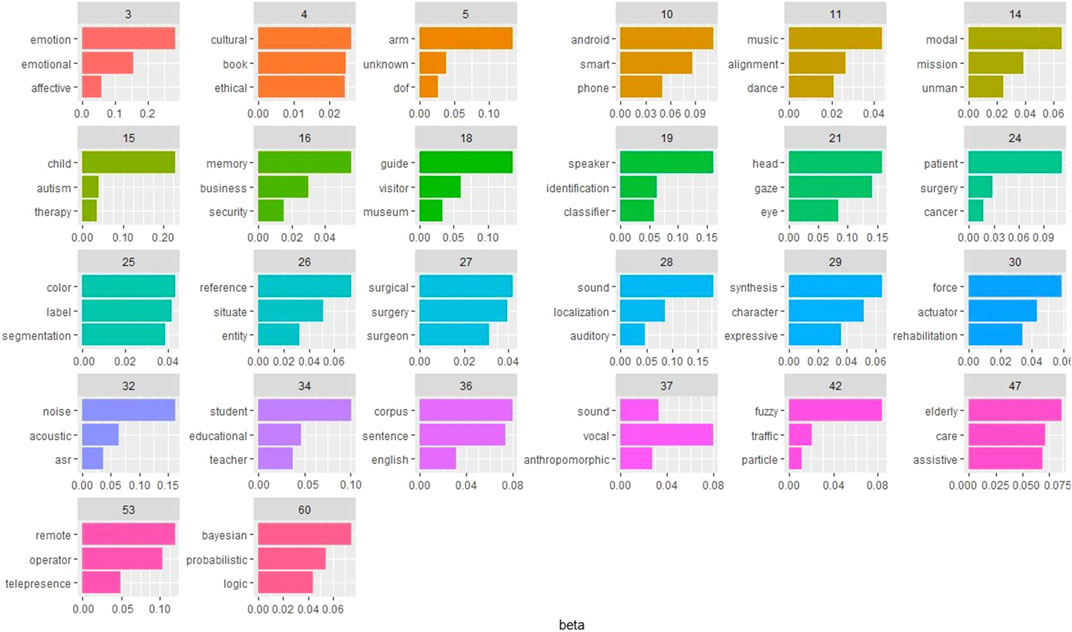
FIGURE 6. Top topics. For each topic, the three top terms are displayed. beta represent the probability that the term belongs to that specific topic.
The trend of each topic was evaluated considering the distribution of topics among each document θ over the years. Each distribution was fitted with a linear regression and the slope was evaluated to extract the main trends. Table 5 displays the slope values computed, highlighting the statistically significant ones. A non-significant trend means that the distribution fluctuates over the years, therefore no conclusions can be drawn about their future development.
To carry out a more detailed and high-resolution analysis, we evaluated the probability of topics over the years and the normalized frequency of the significant trends, highlighting those topics which can be defined as the most frequent and significant. Results are illustrated in Figure 7. Although “child, autism and therapy” and “elderly, care and assistance” have both significant positive trends, the normalized frequency of the former is larger compared to the latter thereby suggesting that they are more important. Consequently, topics can be narrowed again focusing only on the ones that have a higher normalized frequency such as “child, autism and therapy” and “emotion, emotional and affective” for the positive significant trends and on “sound, vocal and anthropometric” and “surgical, surgery and surgeon” for the negative significant ones.
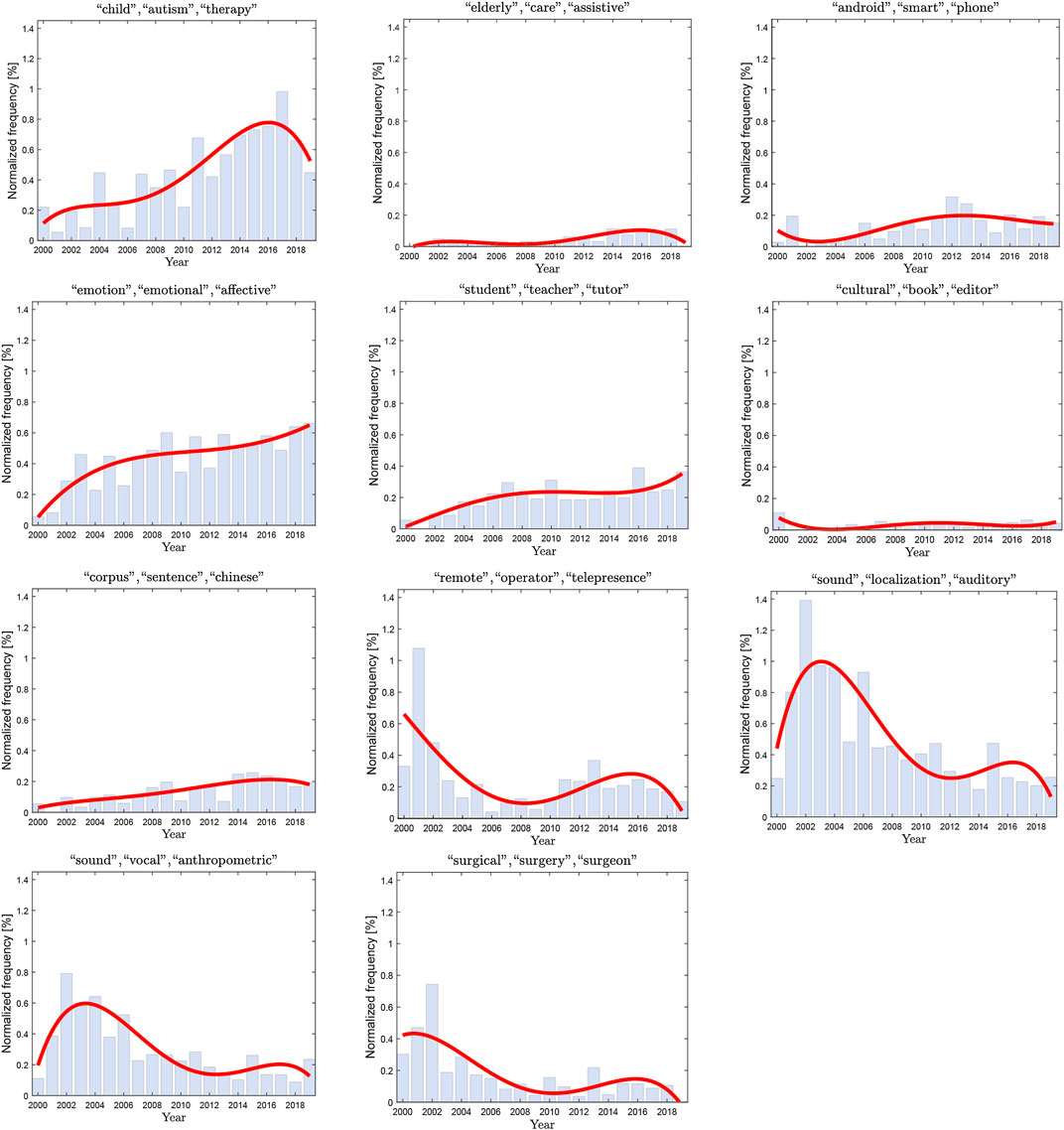
FIGURE 7. Key–words trends of significant top topics normalized and compared to the overall number of words in the collection of document for each year.
7 Results and Discussion
The various steps of the computational linguistic analysis conducted in the presented study led to meaningful answers to the questions we posed in the introduction. In this section, we outline the results that emerged from the data analysis. Not only does the analysis provide a complete overview about the past and the present of verbal communication in robotics, but it also provides an idea of what future scenarios and most promising applications might be.
How did the salient terms related to verbal communication in robotics evolve?
Results from our study show that this field seems to be highly technology-driven. In particular, the contrastive analysis highlights devices or technology that define a specific interval of time. Starting with the
(2) Are there any specific applications that involved the use of verbal communication?
A deeper analysis was performed taking advantage of the LDA model. In this way, main coherent topics and keywords were retrieved, specifically, each topic is defined with three keywords (Figure 6). A qualitative analysis allows us to interpret the topics and to further partition them in five supra-categories, i.e., control, application, language, interaction, signal and hardware.
(3) Do they have any trend in the last decade?
The overall trends of the topics were evaluated distinguishing the significant upward and downward ones (Table 5). The main topics with upward trends are related to social robotics and its applications, whereas downward topics are more related with the technological aspects and the use of voice as a controller. Significant trends were also analyzed with single-year resolution and their frequency was normalized with respect to the overall number of words per year. In this way, among the topics with a significant trend, the more frequent ones can be distinguished. Specifically, the upward significant and more frequent topics are “child”, “autism”, “therapy” and “emotion”, “emotional” and “affective”. On the other hand, there are “sound”, “vocal”, “anthropometric” and “surgical”, “surgery” and “surgeon”. This data suggests that verbal communication appears to be more successful as a communication channel to socially interact with a robot, rather than a tool, or an interface for controlling the latter.
(4) If they do, what can these trends reveal?
Two categories have been identified as promising ones: autism therapy and affective interaction. Looking into the literature, the use of robots in the teaching procedure for children with autism spectrum disorder seems to be effective in enhancing specific social and communication behaviors which are not achieved by humans (Fachantidis et al., 2018). Moreover, children displayed more expression when interacting with a robot capable of performing affective interaction, i.e., to convey emotions and to adapt its behavior (Niculescu et al., 2013). While autism therapy reached a peak in 2017, the affective interaction had a steady increase over the years. On the other hand, applications which were shown to have a significant downward trend concerns the use of anthropometric sounds and vocalization and the use of verbal communication related to surgical activities. One limitation that might explain the downward trend of the first topic is that human realism of a character’s face and voice can evoke feelings of eeriness (Mitchell et al., 2011), especially if not accompanied by an equal level of realism in the cognitive part of the robot, and thus, its behavior. Regarding the trend of the second topic, an issue might be related to the uncertainty that might arise when using the voice command for controlling tasks that require very high precision and accuracy such as surgical operations.
8 Conclusions
The presented study revealed that verbal communication is a research field that is continuously expanding in different areas of robotics. This increasing interest is driven by the desire of a natural human-like interaction with a robot. More than 7,000 scientific publications about the verbal communication field in robotics were analyzed by means of a contrastive analysis and topic mining technique with a related trend analysis. One of the most notable results was the identification of different topics describing the verbal communication field. Specifically, they resulted in being partitioned in five supra-categories: control, application, language, interaction, signal, and hardware. Another main result was that verbal communication for robotics proved to be highly technology-driven, and that several technologies, associated to specific time intervals, emerged as significant for its development. Moreover, two promising research fields related to social robotics were identified: autism therapy and affective interaction. While autism therapy reached a peak in 2017, the affective interaction had a steady increase over the years. On the other hand, the two most significant downward trends identified were vocal interaction and vocal control in surgical robotics. Reasons can be identified in the mismatch between human-like esthetic vs. behavioral realism, and in the uncertainty related to a voice command for precise and accurate tasks such as surgical operations. These findings show that verbal communication is expanding in the robotics field, finding different applications that can have a future translation in the market. Potentially, achieving a natural verbal communication with a robot can have a great impact in the scientific, societal and economic role of robotics in future. Nonetheless, due to the current technical limitations, it is confirmed that the use of voice is accepted and gladly applied in robotics if used for a social affective interaction with a robot, but it is not well liked, or is even mistrusted, when it must be used for applications in which human health or security are in danger. This scenario will probably change only if new technologies are proven to be highly secure, and those still have to be found or introduced in this field.
Although we tried to avoid any biases by implementing a computational pipeline that can extract topics and trends in a rigorous way, those might eventually emerge in some parts of the work. For instance, the query to retrieve the dataset, which is inevitably based on our knowledge. Moreover, this study presented the application of one computational linguistic method. A more extensive analysis could be carried out by comparing different methodologies together with different metrics.
Data Availability Statement
The names of the repository/repositories and accession number(s) can be found in the article/Supplementary Material.
Author Contributions
AMV is the first author of this paper, he studied the state-of-the-art of verbal communication in robotics and did the data mining from Scopus, performing then the analysis reported in the manuscript; LC supervised the work selecting methods and discussing results; FDO contributed as computational linguistic expert; EPS is a full professor of bioengineering who supervised the entire work giving a strong contribution to the organization, writing, and proofing of the presented paper.
Conflict of Interest
The authors declare that the research was conducted in the absence of any commercial or financial relationships that could be construed as a potential conflict of interest.
References
Abdi, J., Al-Hindawi, A., Ng, T., and Vizcaychipi, M. P. (2018). Scoping review on the use of socially assistive robot technology in elderly care. BMJ open 8, e018815. doi:10.1136/bmjopen-2017-018815
Altbach, P. G., and de Wit, H. (2019). Too much academic research is being published. Int. Higher Edu. 59, 2–3. doi:10.6017/ihe.2019.96.10767
Arun, R., Suresh, V., Veni Madhavan, C. E., and Narasimha Murthy, M. N. (2010). “On finding the natural number of topics with latent dirichlet allocation: some observations,” in Pacific-Asia conference on knowledge discovery and data mining. Berlin, Heidelberg: Springer, 391–402.
Azeta, J., Bolu, C., Abioye, A. A., and Oyawale, F. A. (2017). A review on humanoid robotics in healthcare. MATEC Web Conf. 153 (5), 02004. doi:10.1051/matecconf/201815302004
Barakova, E. I., Bajracharya, P., Willemsen, M., Lourens, T., and Huskens, B. (2015). Long-term lego therapy with humanoid robot for children with asd. Expet Syst. 32, 698–709. doi:10.1111/exsy.12098
Belpaeme, T., Kennedy, J., Ramachandran, A., Scassellati, B., and Tanaka, F. (2018). Social robots for education: a review. Sci. Robot. 3, aat5954. doi:10.1126/scirobotics.aat5954
Blei, D. M., Ng, A. Y., and Jordan, M. I. (2003). Latent dirichlet allocation. J. Mach. Learn. Res. 3, 993–1022. doi:10.1162/jmlr.2003.3.4-5.993
Breazeal, C., Kidd, C. D., Thomaz, A. L., Hoffman, G., and Berlin, M. (2005). “Effects of nonverbal communication on efficiency and robustness in human-robot teamwork,” in IEEE/RSJ international conference on intelligent robots and systems, Sendai, Japan, September–October 2–28, 2005 (Edmonton, AB, Canada: IEEE), 708–713.
Brooks, A. G., and Arkin, R. C. (2007). Behavioral overlays for non-verbal communication expression on a humanoid robot. Aut. Robots 22, 55–74. doi:10.1007/s10514-006-9005-8
Budiharto, W., Cahyani, A. D., Rumondor, P. C. B., and Suhartono, D. (2017). Edurobot: intelligent humanoid robot with natural interaction for education and entertainment. Procedia. Comp. Sci. 116, 564–570. doi:10.1016/j.procs.2017.10.064
Burgoon, J. K., Guerrero, L. K., and Manusov, V. (2016). Nonverbal communication (routledge). London, United Kingdom: Taylor and Francis, 509.
Buter, R., and Van Raan, A. (2013). Identification and analysis of the highly cited knowledge base of sustainability science. Sustain. Sci. 8, 253–267. doi:10.1007/s11625-012-0185-1
Cao, J., Xia, T., Li, J., Zhang, Y., and Tang, S. (2009). A density-based method for adaptive lda model selection. Neurocomputing 72, 1775–1781. doi:10.1016/j.neucom.2008.06.011
Cominelli, L., Mazzei, D., and De Rossi, D. E. (2018). Seai: social emotional artificial intelligence based on damasio’s theory of mind. F. Robot. AI 5, 6. doi:10.3389/frobt.2018.00006
Cowen, A. S., Elfenbein, H. A., Laukka, P., and Keltner, D. (2019). Mapping 24 emotions conveyed by brief human vocalization. Am. Psychol. 74, 698. doi:10.1037/amp0000399
Crowelly, C. R., Villanoy, M., Scheutzz, M., and Schermerhornz, P. (2009). “Gendered voice and robot entities: perceptions and reactions of male and female subjects,” in 2009 IEEE/RSJ International conference on intelligent robots and systems, St. Louis, MO, USA, October 10–15, 2009 (IEEE), 3735–3741.
Denny, M. J. (2018). SpeedReader: high performance text analysis. R package. Version 0.9.1. https://github.com/matthewjdenny/SpeedReader
Deveaud, R., SanJuan, E., and Bellot, P. (2014). Accurate and effective latent concept modeling for ad hoc information retrieval. Doc. Numér. 17, 61–84. doi:10.3166/dn.17.1.61-84
Ding, I.-J., and Shi, J.-Y. (2017). Kinect microphone array-based speech and speaker recognition for the exhibition control of humanoid robots. Comput. Electr. Eng. 62, 719–729. doi:10.1016/j.compeleceng.2015.12.010
Draper, M., Miller, C. A., Benton, J., Calhoun, G. L., Ruff, H., Hamell, J., et al. (2013). “Multi-unmanned aerial vehicle systems control via flexible levels of interaction: an adaptable operator-automation interface concept demonstration,” Boston, MA, USA: AIAA infotech@ aerospace (I@ A) conference, 4803. doi:10.2514/6.2013-4803
Fachantidis, N., Syriopoulou-Delli, C. K., and Zygopoulou, M. (2018). The effectiveness of socially assistive robotics in children with asd. Int. J. Dev. Disabil. 66, 1–9. doi:10.1080/20473869.2018.1495391
Goeldner, M., Herstatt, C., and Tietze, F. (2015). The emergence of care robotics - a patent and publication analysis. Technol. Forecast. Soc. Change 92, 115–131. doi:10.1016/j.techfore.2014.09.005
Gomez, R., Inoue, K., Nakamura, K., Mizumoto, T., and Nakadai, K. (2014). “Speech-based human-robot interaction robust to acoustic reflections in real environment,” in IEEE/RSJ International conference on intelligent robots and systems, Chicago, IL, USA, September 14–18 2014 (IEEE), 1367–1373.
Griffiths, T. L., and Steyvers, M. (2004). Finding scientific topics. Proc. Natl. Acad. Sci. U.S.A. 101 (Suppl. 1), 5228–5235. doi:10.1073/pnas.0307752101
Gustavsson, P., Syberfeldt, A., Brewster, R., and Wang, L. (2017). Human-robot collaboration demonstrator combining speech recognition and haptic control. Procedia CIRP 63, 396–401. doi:10.1016/j.procir.2017.03.126
Hornik, K., and Grün, B. (2011). Topicmodels: an r package for fitting topic models. J. Stat. Software 40, 1–30. doi:10.18637/jss.v040.i13
Huang, C.-M., and Mutlu, B. (2016). “Anticipatory robot control for efficient human-robot collaboration,” in The eleventh ACM/IEEE international conference on human robot interaction, Christchurch, New Zealand, March 7–10, 2016 (IEEE Press), 83–90.
Huang, X., Acero, A., and Hon, H.-W. (2001). Spoken language processing: a guide to theory, algorithm, and system development. 1st Edn. Upper Saddle River, NJ: Prentice Hall PTR, 1008.
IFR (2018). Executive summary world robotics 2018 service robots. Available at: https://ifr.org/downloads/press/Executive_Summary_WR_Service_Robots_2017_1.pdf (Accessed February 21, 2019).
Ittipanuvat, V., Fujita, K., Sakata, I., and Kajikawa, Y. (2014). Finding linkage between technology and social issue: a literature based discovery approach. J. Eng. Technol. Manag. 32, 160–184. doi:10.1016/j.jengtecman.2013.05.006
Jensen, B., Tomatis, N., Mayor, L., Drygajlo, A., and Siegwart, R. (2005). Robots meet humans-interaction in public spaces. IEEE Trans. Ind. Electron. 52, 1530–1546. doi:10.1109/tie.2005.858730
Kennedy, J., Lemaignan, S., Montassier, C., Lavalade, P., Irfan, B., Papadopoulos, F., et al. (2017). “Child speech recognition in human-robot interaction: evaluations and recommendations,” in 2017 ACM. IEEE International conference on human-robot interaction (ACM), Vienna, Austria, March 6–9, 2017 (New York, NY, United States: IEEE), 82–90.
Krauss, R. M. (2002). “The psychology of verbal communication,” in International encyclopaedia of the social and behavioral Sciences. London, United Kingdom: Elsevier, 16161–16165.
Kumar, S. A., and Kumar, C. S. (2016). “Improving the intelligibility of dysarthric speech towards enhancing the effectiveness of speech therapy,” in International conference on advances in computing, communications and informatics (ICACCI), Jaipur, India, September 21–24, 2016 (IEEE), 1000–1005.
Kumatani, K., Arakawa, T., Yamamoto, K., McDonough, J., Raj, B., Singh, R., et al. (2012). “Microphone array processing for distant speech recognition: towards real-world deployment,” in Proceedings of the 2012 Asia pacific signal and information rocessing association annual summit and conference, Hollywood, CA, USA, December 3–6, 2012 (IEEE), 1–10.
Landhuis, E. (2016). Scientific literature: information overload. Nature 535, 457–458. doi:10.1038/nj7612-457a
Liu, K., Wu, C.-Y., and Song, K.-T. (2017). A study on speech recognition control for a surgical robot. IEEE Trans. Ind. Inf. 13, 607–615. doi:10.1109/tii.2016.2625818
Liu, R., and Zhang, X. (2017). Systems of natural-language-facilitated human-robot cooperation: a review. Preprint repository name [Preprint]. Available at: arXiv:1701.08269 (Accessed January 28, 2017).
Mavridis, N. (2015). A review of verbal and non-verbal human-robot interactive communication. Robot. Autonom. Syst. 63, 22–35. doi:10.1016/j.robot.2014.09.031
Mejia, C., and Kajikawa, Y. (2017). Bibliometric analysis of social robotics research: identifying research trends and knowledgebase. Appl. Sci. 7, 1316. doi:10.3390/app7121316
Mitchell, W. J., Szerszen, K. A., Lu, A. S., Schermerhorn, P. W., Scheutz, M., and MacDorman, K. F. (2011). A mismatch in the human realism of face and voice produces an uncanny valley. Iperception 2, 10–12. doi:10.1068/i0415.PMID:23145223
Mutlu, B., Yamaoka, F., Kanda, T., Ishiguro, H., and Hagita, N. (2009). “Nonverbal leakage in robots: communication of intentions through seemingly unintentional behavior,” in Proceedings of the 4th ACM/IEEE international conference on Human robot interaction (ACM), La Jolla, CA, March 1–13, 2009 (IEEE), 69–76.
Nakamura, M., and Sawada, H. (2006). “Talking robot and the analysis of autonomous voice acquisition,” in IEEE/RSJ International conference on intelligent robots and systems, Beijing, China, October 9–15, 2006 (IEEE), 4684–4689.
Niculescu, A., van Dijk, B., Nijholt, A., Li, H., and See, S. L. (2013). Making social robots more attractive: the effects of voice pitch, humor and empathy. Int. J. Soc. Robot. 5, 171–191. doi:10.1007/s12369-012-0171-x
Nikita, M. (2016). Ldatuning: tuning of the latent dirichlet allocation models parameters. R package. Version 0.2.0. https://github.com/nikita-moor/ldatuning.
Ramamurthy, P., and Li, T. (2018). “Buddy: a speech therapy robot companion for children with cleft lip and palate (cl/p) disorder,” in Companion of the 2018 ACM/IEEE International conference on human-robot interaction (ACM), Chicago, IL, March 5–8, 2018 (New York, NY, United States IEEE), 359–360.
Reeves, B., and Nass, C. I. (1996). The media equation: how people treat computers, television, and new media like real people and places. Cambridge, UK: Cambridge University Press, 317.
Robla-Gómez, S., Becerra, V. M., Llata, J. R., Gonzalez-Sarabia, E., Torre-Ferrero, C., and Perez-Oria, J. (2017). Working together: a review on safe human-robot collaboration in industrial environments. IEEE Access 5, 26754–26773. doi:10.1109/access.2017.2773127
Sagri, M.-T., Morini, E., Venturi, G., dell’Orletta, F., and Montemagni, S. (2019). “Defining models to observe the main phenomena characterizing the Italian education system,” in Proceedings of the 1st International Conference of the Journal Scuola Democratica, June 5-8, 2019, Cagliari, Italy: Proceedings of the 1st International Conference of the Journal Scuola Democratica,. Vol. III, 81.
Salton, G., and Buckley, C. (1988). Term-weighting approaches in automatic text retrieval. Inf. Process. Manag. 24, 513–523. doi:10.1016/0306-4573(88)90021-0
Schulz, R., Talbot, B., Lam, O., Dayoub, F., Corke, P., Upcroft, B., et al. (2015). “Robot navigation using human cues: a robot navigation system for symbolic goal-directed exploration,” in 2015 IEEE international conference on robotics and automation (ICRA), Seattle, WA, USA, May 26–30, 2015 (IEEE), 1100–1105.
Silge, J., and Robinson, D. (2017). Text mining with R: a tidy approach. 1st Edn. O’Reilly Media, Inc., 194.
Twamley, Y. H., Wrobel, J., Cornuet, M., Kerhervé, H., Damnée, S., and Rigaud, A. S. (2014). Acceptance of an assistive robot in older adults: a mixed-method study of human-robot interaction over a 1-month period in the living lab setting. Clin. Interv. Aging 9, 801. doi:10.2147/CIA.S56435
Van Patten, R., Keller, A. V., Maye, J. E., Jeste, D. V., Depp, C., Riek, L. D., et al. (2020). Home-based cognitively assistive robots: maximizing cognitive functioning and maintaining independence in older adults without dementia. Clin. Interv. Aging 15, 1129. doi:10.2147/CIA.S253236
Yeh, J.-F., Tan, Y.-S., and Lee, C.-H. (2016). Topic detection and tracking for conversational content by using conceptual dynamic latent dirichlet allocation. Neurocomputing 216, 310–318. doi:10.1016/j.neucom.2016.08.017
Keywords: social robotics, affective computing, speech synthesis, speech generation, computational linguistic analysis, data mining, topic modeling, verbal communication
Citation: Marin Vargas A, Cominelli L, Dell’Orletta F and Scilingo EP (2021) Verbal Communication in Robotics: A Study on Salient Terms, Research Fields and Trends in the Last Decades Based on a Computational Linguistic Analysis. Front. Comput. Sci. 2:591164. doi: 10.3389/fcomp.2020.591164
Received: 03 August 2020; Accepted: 29 December 2020;
Published: 15 February 2021.
Edited by:
Umberto Maniscalco, Institute for High Performance Computing and Networking (ICAR), ItalyReviewed by:
Barbara-Lewandowska-Tomaszczyk, State University of Applied Sciences in Konin, PolandRaffaele Guarasci, Institute for High Performance Computing and Networking (ICAR), Italy
Copyright © 2021 Marin Vargas, Cominelli, Dell’Orletta and Scilingo. This is an open-access article distributed under the terms of the Creative Commons Attribution License (CC BY). The use, distribution or reproduction in other forums is permitted, provided the original author(s) and the copyright owner(s) are credited and that the original publication in this journal is cited, in accordance with accepted academic practice. No use, distribution or reproduction is permitted which does not comply with these terms.
*Correspondence: Alessandro Marin Vargas, alessandro17mv@gmail.com; Lorenzo Cominelli, lorenzo.cominelli@for.unipi.it