- 1Cancer Epigenomics Laboratory, Department of Clinical Analysis, Toxicology and Food Sciences, School of Pharmaceutical Sciences of Ribeirao Preto, University of Sao Paulo, Ribeirao Preto, Brazil
- 2Structural Genomics Consortium and Institute of Pharmaceutical Chemistry, Buchmann Institute for Molecular Life Sciences, Johann Wolfgang Goethe University, Frankfurt am Main, Germany
- 3Laboratory of Comparative and Translational Oncology (LOCT), Department of Veterinary Medicine, Faculty of Animal Science and Food Engineering, University of Sao Paulo, Pirassununga, Brazil
Stemness is a phenotype associated with cancer initiation and progression, malignancy, and therapeutic resistance, exhibiting particular molecular signatures. Targeting stemness has been proposed as a promising strategy against breast cancer stem cells that can play a key role in breast cancer progression, metastasis, and multiple drug resistance. Here, using a previously published one-class logistic regression machine learning algorithm (OCLR) built on pluripotent stem cells to predict stemness in human cancer samples, we provide the stemness index (mRNAsi) of different canine non-tumor and mammary cancer cells. Then, we confirmed that inhibition of BET proteins by (+)-JQ1 reduces stemness in a high mRNAsi canine cancer cell. Furthermore, using public data, we observed that (+)-JQ1 can also decrease stemness in human triple-negative breast cancer cells. Our work suggests that mRNAsi can be used to estimate stemness in different species and confirm epigenetic modulation by BET inhibition as a promising strategy for modulating the stemness phenotype in canine and human mammary cancer cells.
Introduction
Breast cancer represents one in four cancers diagnosed among women worldwide (24.5%) (Sung et al., 2021) while mammary cancer accounts for more than 50% of the diagnosed tumors in female dogs, of which half are malignant (Gupta et al., 2012; Goldschmidt et al., 2016; Baioni et al., 2017). Surgery is the elective treatment for canine mammary cancer (CMC) with the use of adjuvant therapy such as chemotherapy and radiotherapy in advanced cases. However, these therapeutic strategies have limitations, and it is still an open question if they offer a significant benefit to dogs as they do not prevent high rates of recurrence and poor prognosis of malignant CMCs (Simon et al., 2006; Marconato et al., 2008; Stratmann et al., 2008; Tran et al., 2016). Triple-negative breast cancer (TNBC) is an aggressive human breast cancer associated with poorer prognosis, characterized by the lack of estrogen/progesterone receptors (ER/PR) and HER2 expression. Thus, it exhibits limited therapeutic options since hormonal therapy, which is widely used in hormone receptor-positive tumors, is not effective in TNBC, and only chemotherapy is routinely used for the treatment of the disease (Collignon et al., 2016). Therefore, innovative and effective therapies warrant being tested in malignant and aggressive types of mammary cancer both in women and female dogs, resulting in a promising field in comparative oncology.
Stemness is a phenotype originally observed in stem cells (SCs), allowing the SCs to divide and keep, at least one new undifferentiated SC, as well as to differentiate into mature cells of different adult organs (Shenghui et al., 2009). In cancer, this phenotype is associated with the cancer stem cell (CSC) concept and consequently interconnected with intratumoral heterogeneity, initiation, progression, multidrug resistance, and metastasis (Batlle and Clevers, 2017). Therefore, considerable efforts to design innovative approaches to target these cells and their phenotypes have been made (Borah et al., 2015). Cancer stemness scores have been analyzed through CSC and non-CSC on previously published stemness signatures (Pinto et al., 2015; Ng et al., 2016). However, those approaches are limited to a small set of genes and few tumor samples. Thus, the stemness index (mRNAsi) based on stem cell expression of 12,945 genes reveals an opportunity to estimate stemness as a cellular state (Malta et al., 2018).
Epigenetic therapy has been highlighted as a potential and innovative therapeutic strategy in human clinical cancer therapy (Muller et al., 2011; Dawson and Kouzarides, 2012), especially in hematological malignancies (Fenaux et al., 2010; Diesch et al., 2016). In addition, several studies demonstrate the potential of epigenetic inhibitors in solid tumors (Jin et al., 2021) and new classes of epigenetic drugs targeting epigenetic writers (DNMTs, HKMTs, PRMTs, and HATs), erasers (HDMs and HDACs), and readers (MBDs, Bromodomains, and chromodomains) have been developed and tested. Unlike in human oncology, epigenetic inhibitors remain little in use in veterinary oncology. Recently, we observed that the inhibition of bromodomain and extraterminal (BET) family of proteins, an epigenetic reader of histone acetylation, using (+)-JQ1, is a promising strategy to inhibit self-renewal and tumorigenicity in CMC cells using 3D in vitro models. Low concentrations of (+)-JQ1 inhibited tumorsphere and colony formation and were able to downregulate important genes associated with self-renewal pathways including WNT, NOTCH, Hedgehog, and PI3K/AKT/mTOR (Xavier et al., 2019). These results strongly indicated that BET inhibition modulates malignant phenotypes such as self-renewal and could directly impact tumorigenic cells such as cancer stem cells (CSCs) in CMC cells. (+)-JQ1 has also been promising in target stemness in TNBC through inhibition of self-renewal and cell cycle arrest in G0/G1 (Serrano-Oviedo et al., 2020). In addition, other bromodomain inhibitors such as BETi OTX-015 and I-BET151 have demonstrated similar results (Qiao et al., 2020; Serrano-Oviedo et al., 2020). In an in vivo breast cancer model, a more specific BET inhibitor MS417, which binds to BD1 and BD2 of Bromodomains 4, showed a more pronounced antitumor effect in comparison with (+)-JQ1 (Shi et al., 2014).
Here, we applied a one-class logistic regression (OCLR) machine-learning algorithm (Malta et al., 2018) to extract transcriptomic feature sets from our previous studies and determine the stemness index in different CMC cells. The OCLR algorithm was benchmarked against random forest and SVM-based methods (Sokolov et al., 2016; Malta et al., 2018). The advantage of this algorithm is that no negative samples are required for the training step, thereby eliminating the need for a background set. Also, we are able to extract the most interesting features (e.g., genes, probes) based on the estimation of the model parameters. Thus, we confirm that BET inhibition by (+)-JQ1 inhibits stemness in a high mRNAsi CMC cell, CF41.Mg. Finally, using public data, we observed similar results in a human TNBC cell line, MDA-MB-231, suggesting BET inhibition as a promising strategy to target stemness both in canine and human mammary cancer cells. In addition, these findings highlight the canine cancer model as a good model to study the therapeutic effects of epigenetic therapy in mammary cancer.
Materials and methods
RNAseq data generation
RNA of different CMC cells including CF41.Mg (CRL-6232™, ATCC), E20, M5, and M25 (Cordeiro et al., 2018; Xavier et al., 2018) was extracted using TRIzol. CF41.Mg cells were allowed to grow as tumorspheres in ultra-low attachment 24-well plates for 96 h with 100 nM of (+)-JQ1 or 100 nM of (−)-JQ1, without inhibitor replenishing, as previously described (Xavier et al., 2019). Then, the tumorspheres were collected and RNA was extracted using the RNeasy Mini Kit (QIAGEN, United Kingdom). The RNA quality and quantities were assessed using automated capillary gel electrophoresis on a Bioanalyzer 2100 with RNA 6000 Nano Labchips according to the manufacturer’s instructions (Agilent Technologies, Ireland). Only samples that presented an RNA integrity number (RIN) higher than 8 were considered for the sequencing. RNA libraries were constructed using the TruSeq™ Stranded mRNA LT Sample Prep Protocol and sequenced on Illumina HiSeq 2500 equipment in a HiSeq Flow Cell v4 using the HiSeq SBS Kit v4 (2 × 100 pb). All RNA-Seq data from (+)-JQ1-treated and (−)-JQ1-treated tumorspheres have been previously published (Xavier et al., 2019). RNA-seq data from TNBC human cell lines were downloaded from GEO (GSE102407, GSE115550, and GSE55922). RNA-seq data from induced pluripotent canine stem cells (iPSs) and canine mammary stroma samples were downloaded from GEO (GSE152489, GSE165639, and GSE135183).
Alignment and differential expression
Sequencing quality was evaluated using the software FastQC (http://www.bioinformatics.babraham.ac.uk/projects/fastqc), and no additional filter was performed. Sequence alignment against the canine reference genome (CanFam3.1) and human reference genome (GRCh38) was performed using STAR (Dobin et al., 2013), according to the standard parameters and including the annotation file (Ensembl release 89). Secondary alignments, duplicated reads, and read failing vendor quality checks were removed using Samtools (Li et al., 2009). Alignment quality was confirmed using Qualimap (García-Alcalde et al., 2012). Gene expression was estimated by reading counts using HTseq (Anders et al., 2015) and normalized as counts per million reads (CPMs). Only genes presenting at least one CPM in at least three samples were kept for differential expression (DE) analysis. DE was performed using the EdgeR package (Robinson et al., 2009) on the R environment, based on the negative binomial distribution. The Benjamini–Hochberg procedure was used to control the false discovery rate (FDR). Functional enrichment analysis of DE genes was performed using STRING (Jensen et al., 2009; Szklarczyk et al., 2019) and EnrichR (Chen et al., 2013) using Reactome 2016, GO Biological Process 2021, and GO Molecular Function 2021 databases.
Stemness index model
To predict the stemness phenotype in canine samples, we applied a machine learning algorithm described by Malta et al. (2018) and generated a one-class logistic regression (OCLR) algorithm using human stem cell (iPS and ES) data from Progenitor Cell Biology Consortium (PCBC) to estimate stemness (mRNAsi) index in human tumors. The stem cell transcriptomic matrix used to train OCLR contains 12,945 gene expression values. Furthermore, this model was validated using leave-one-out cross-validation that leaves one stem cell out of training and then applies the model to it as well in the non-SC cells (their differentiated ecto-, meso- and endoderm progenitors). Moreover, Malta’s model presented a high correlation among other cancer stem cell signatures, promoting significant insights into pan-cancer biological and clinical features. The workflow to generate the mRNAsi is available at https://github.com/ArtemSokolov/PanCanStem.
Stemness index prediction
First, we converted the gene IDs from Canis lupus familiaris to Homo sapiens using tables of Ensembl data downloaded via the BioMart mining tool (https://www.ensembl.org/biomart/martview/). We then mapped ENSEMBL gene ID to the gene symbol using the org. Hs.eg.db package, from the Bioconductor repository. Genes that had no such intersection were dropped. The previously described prediction stemness index model derived from pluripotent cells (Malta et al., 2018) was applied to gene expression profiles of canine and human mammary cancer cell lines, leading to their mRNAsi. The indices were scaled to 0–1 (0 being a low stemness score and 1 being high) by subtracting the minimum and dividing by the maximum. The top genes of stemness signature (439 genes) were selected based on the Euclidean distance of the stemness signature model’s weight absolute values. From the 12,953 genes in the original model, we apply 11,251 genes due to the conversion gene ID output. In relation to stemness prediction in human cell lines and canine stem cells, we applied 12,257 and 7,929 genes, respectively, as a result of the number of genes common among RNAseq from GEO datasets.
Statistical analysis
Stemness index was compared between CMCs using one-way ANOVA with post hoc Tukey’s test. Unpaired T-test was used to compare the stemness index between (+)-JQ1-treated and non-treated tumorspheres. Correlation analysis was determined using the Pearson correlation coefficient. Significant differences were considered when p-value < 0.05. For functional enrichment analyses, the p-value was adjusted for multiple tests, and the Benjamini and Hochberg method was used to test multiple categories in a group of functional gene sets. Genes presenting FDR ≤0.01 and log-fold change (LogFC) > 1; <−1 were considered differentially expressed (DE).
Results
Stemness index algorithm can be applied to canine species
To test if the previously described machine learning algorithm for stemness prediction in human cancer can be applied to non-human species, we applied the algorithm to estimate the stemness index of canine mammary cancer (CMC) cell lines. The efficiency conversion of canine to human gene ID was 51% (16,405/32,740 genes), and we rebuilt the prediction model using the overlapping genes between the original prediction model and the canine dataset. We validated the canine prediction model of stemness by applying it to public data comprising canine non-tumor somatic and pluripotent stem cells. The canine pluripotent stem cells presented a higher stemness index (mRNAsi) than somatic cells represented by mammary gland stroma, matched cancer-associated stroma and fibroblasts (Figures 1A,B), confirming that the algorithm runs efficiently in canine molecular profiles.
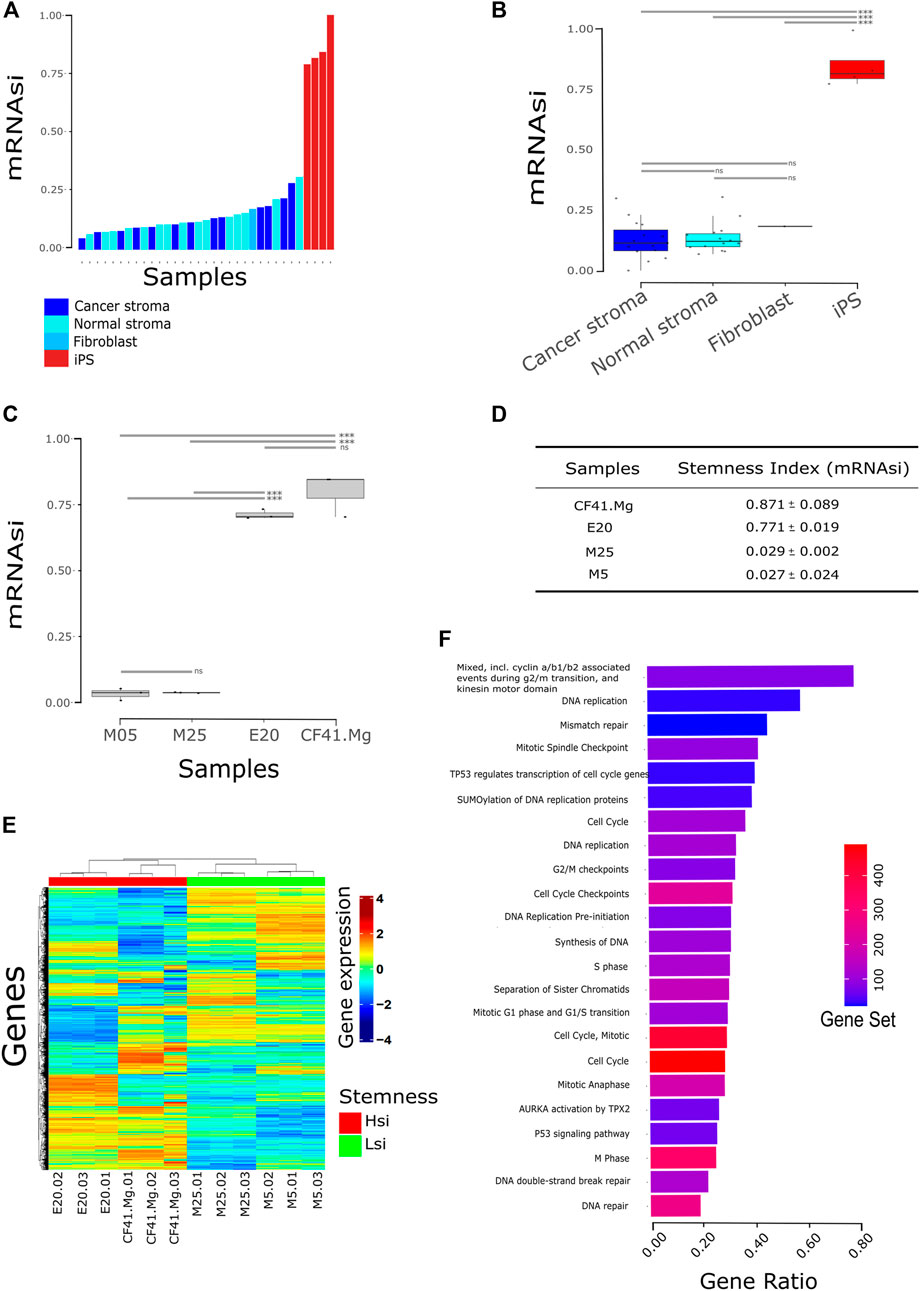
FIGURE 1. Stemness index (mRNAsi) stratifies canine cell lines. (A) Bars correspond to the mRNAsi predicted to each sample comparing stem cells to normal cells (n = 35); (B) Same from (A) visualized by the group in boxplot and stratified by a median. Samples were accessed upon GSE152489, GSE165639, and GSE135183. iPS: induced pluripotent stem cell fibroblast-derived; normal stroma: normal mammary gland stroma; cancer stroma: cancer-associated stroma (match normal stroma dog patient). *** = p < 0.001; ns = not statistical significance; (C) mRNAsi of canine cell lines from the previously published dataset. CF41.Mg and E20 cells present the highest mRNAsi in comparison with M5 and M25. *** = p < 0.001; ns = not statistical significance (one-way ANOVA); (D) discovery dataset statistic; (E) heatmap of gene expression clustering Hsi cells (CF41.Mg and E20) and Lsi (M5 and M25) in distinct groups. Hsi: high stemness index; Lsi: low stemness index; (F) pathway analysis from upregulated genes in Hsi cells (FDR <0.05). Gene ratio is the proportion of genes from differential expression output related to the number of genes annotated to the biological pathway—gene set. Database accessed: KEGG, Reactome, STRING.
We then applied the algorithm to estimate the stemness index of four CMC cell lines: CF41.Mg, E20, M5, and M25. We observed the highest mRNAsi in CF41.Mg and E20 in comparison with M5 and M25 cell lines (Figures 1C,D; p < 0.0001). We then classified CMC cells into two different groups based on the mRNAsi: CF41.Mg and E20 cell lines as high mRNAsi (Hsi) and M5 and M25 as low mRNAsi (Lsi) (Figure 1E and Supplementary Table S1).
A differential gene expression analysis between Hsi and Lsi showed 1,933 upregulated genes and 1,926 downregulated genes in the Hsi cell lines in comparison with Lsi cell lines (FDR <0.01 and LogFC >1; < −1). Interestingly, functional enrichment analysis showed that upregulated genes in Hsi cell lines were related, mainly, to cell cycle and DNA replication pathways such as cyclin a/b1/b2, mitotic spindle checkpoint, G2/M checkpoints, cell cycle checkpoints, S phase, mitotic G1 phase and G1/S transition, M phase, p53 signaling pathway, AURKA activation by TPX2, TP53 regulates transcription of cell cycle genes, SUMOylation of DNA replication proteins, synthesis of DNA, DNA replication pre-initiation, separation of sister chromatids, DNA double-strand break repair, and DNA repair (Figure 1F, Supplementary Table S2). In addition, several genes of stemness signature were upregulated in Hsi cell lines including ITGB3, EZH2, ITGA3, PXN, PTK2, TGFBI, SOX2, KLF4, MYC, AURKA, AURKB, CDH1, EPCAM, TPX2, IGF2BP2, PODXL, CDK1, DDX21, NOTCH1, PBX1, TP53, WNT5A, WNT7A, WNT7B, BIRC5, TTK, KIF14, and KIF18A (Supplementary Table S3).
Spherical cancer models, such as tumorsphere formation assay, are relevant for studying stemness in cancer. Thus, we further explored the correlation between the mRNAsi and tumorsphere formation ability of the CMC cells observed in our previous study (Xavier et al., 2018). Surprisingly, we did not observe any correlation between the number of tumorspheres and mRNAsi in the CMC cells (r = −0.2653; p = 0.73).
mRNAsi confirms stemness inhibition by (+)-JQ1 in canine mammary cancer cells
In our previous study, we determined the BET protein inhibitor, (+)-JQ1, as a promising compound to inhibit stemness in CMC cells through the decrease of tumorsphere and colony formation and downregulation of important genes associated with self-renewal pathways (Xavier et al., 2019). Here, we confirmed that (+)-JQ1-treated tumorspheres to the CF41.Mg cell line exhibit a lower mRNAsi than tumorspheres treated with the matched inactive control compound (−)-JQ1 (Figure 2A; p = 0.00014).
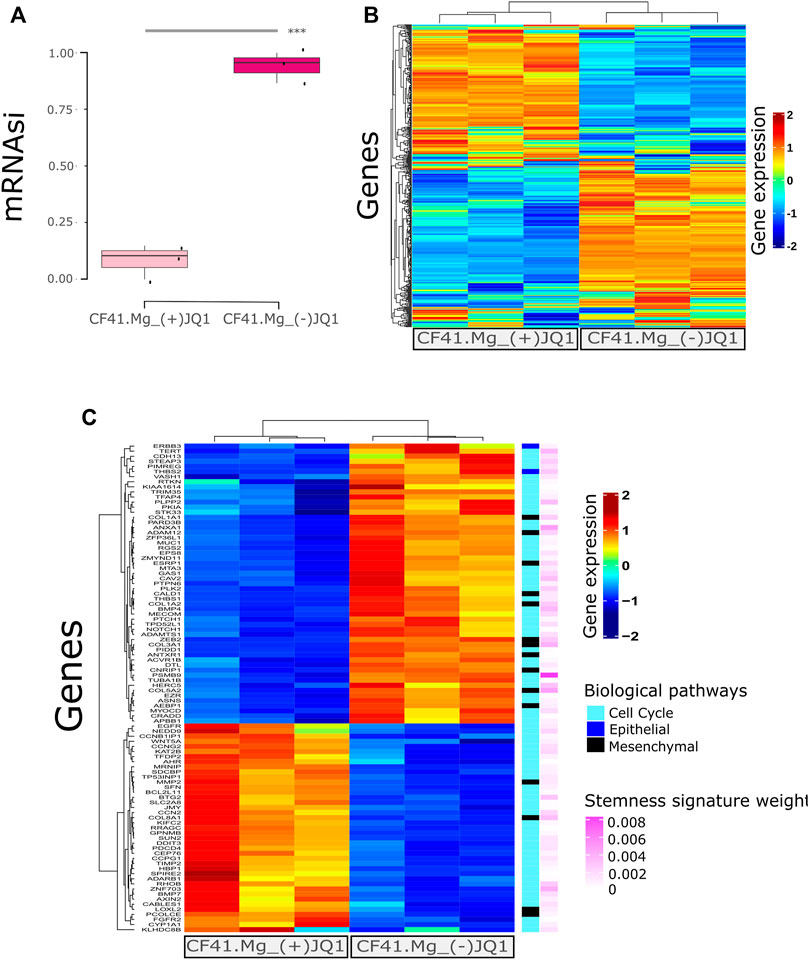
FIGURE 2. Stemness inhibition by (+)-JQ1 correlates to mRNAsi prediction in canine mammary cancer cells. (A) CF41.Mg tumorspheres upon (+)-JQ1 treatment. *** = p < 0.001 (t-test) compared to (−)-JQ1 (B) Heatmap of gene expression levels of CF41.Mg-(+)-JQ1 treatment compared to (−)-JQ1 regarding the most relevant genes in stemness signature (439 genes). (C) Heatmap showing (+)-JQ1-treatment effect compared to (−)-JQ1 in differential gene expression levels (FDR ≤0.01 and log-fold change (LogFC) > 1); < −1. Epithelial and mesenchymal genes were retrieved from Mak 2016 Epithelial-to-Mesenchymal Transition signature. Cell cycle gene sets were retrieved from GSEA signatures. All of the genes are present in Malta’s stemness signature with the respective importance (absolute value of gene weight).
We further explored the top genes comprising the mRNAsi signature and observed their expression to differ between (+)-JQ1-treated tumorsphere and (−)-JQ1-treated tumorsphere genes (Figure 2B). We also identified differentially expressed genes between (+)-JQ1-treated tumorspheres and (−)-JQ1-treated tumorspheres and explored EMT and cell cycle genes that are supposed to be associated with stemness. Genes clustered differently between the two groups (Figure 2C) and some of these EMT/cell cycle genes were downregulated in (+)-JQ1-treated tumorspheres including ZEB2, THBS1, THBS2, BMP4, CDH13, and NOTCH1. In addition, (+)-JQ1 upregulated the expression of G2/M checkpoint- and cell death-associated genes such as BTG2, CCNG2, BMP7, and BCL2L11.
mRNAsi confirms stemness inhibition by (+)-JQ1 in triple-negative breast cancer cells
We also investigated if (+)-JQ1 could decrease mRNAsi in human TNBC cells using public data. We found two studies that treated MDA-MB-231, a well-established model of TNBC, with (+)-JQ1. The former study included transcript levels from 1 µM (+)-JQ1-treated MDA-MB-231 cells versus DMSO-treated MDA-MB-231 cells for 24 h (Zanconato et al., 2018), while the latter included transcript levels from 500 nM (+)-JQ1-treated MDA-MB-231 cells versus DMSO-treated MDA-MB-231 cells for 18 h (Ren et al., 2018). We applied the OCLR stemness prediction algorithm to these RNAseq data. In the first study, we observed that mRNAsi of (+)-JQ1-treated cells was significantly lower than the mRNAsi of DMSO-treated cells (Figure 3A; p = 0.0004906). Then, we observed the differentially expressed genes between (+)-JQ1-treated MDA-MB-231 and DMSO-treated cells. We could find 1,617 downregulated and 1,601 upregulated genes in (+)-JQ1-treated MDA-MB-231 cells in comparison with DMSO-treated cells. Downregulated genes in (+)-JQ1-treated MDA-MB-231 cells were strongly enriched for several pathways associated with cell cycles such as DNA replication, cell cycle, G2/M DNA replication checkpoint, G0 and early G1, G2/M DNA damage checkpoint, mitotic spindle checkpoint, cyclin A/B1/B2 associated events during G2/M transition, cell cycle checkpoints, mitotic G1 phase and G1/S transition, S phase, and M phase (Figure 3B, Supplementary Table S4).
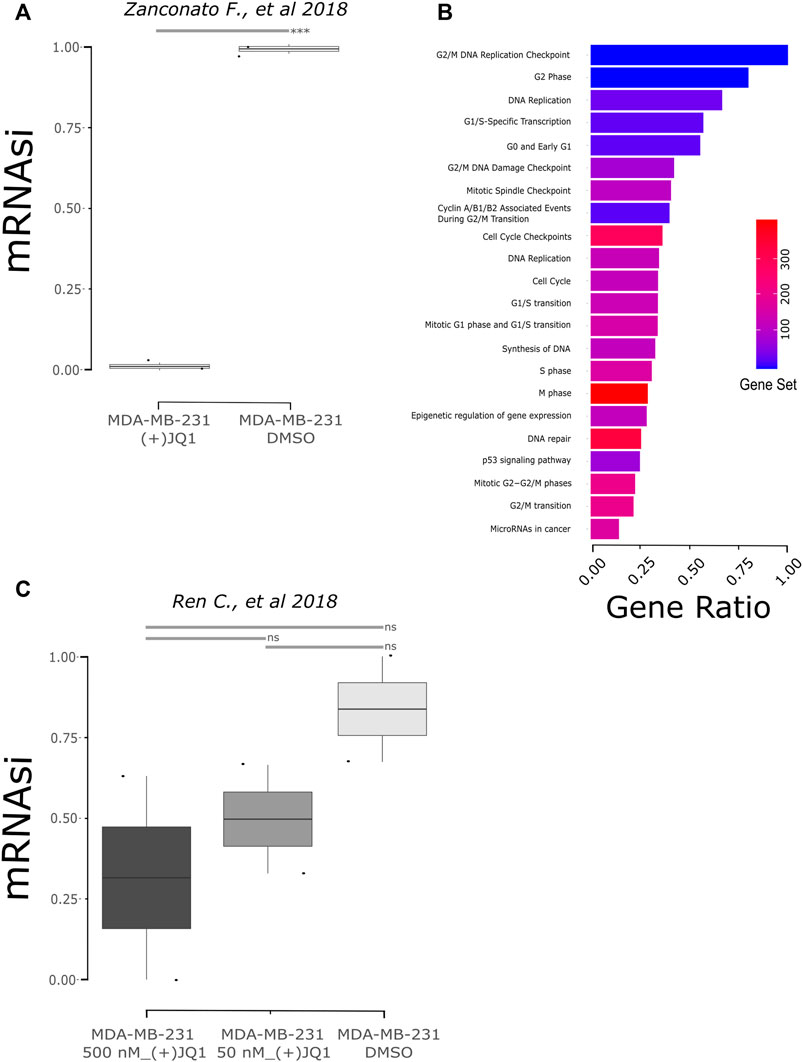
FIGURE 3. Stemness inhibition by (+)-JQ1 correlates to mRNAsi prediction in human breast cancer cells (MDA-MB-231). (A) mRNAsi of MDA-MB-231 cells upon JQ1 treatment from the GSE102407 study (Zanconato et al., 2018). *** = p < 0.001 (t-test). (B) Pathway analysis from downregulated genes in (+)-JQ1-treated MDA-MB-231 cells (FDR ≤0.05). Gene ratio is the proportion of genes from differential expression output related to the number of genes annotated to the biological pathway – gene set. Database accessed: KEGG and Reactome. (C) mRNAsi of MDA-MB-231 cells upon JQ1 treatment from the GSE115550 study (Ren et al., 2018). ns = not statistical significance (one-way ANOVA).
When we analyzed the mRNAsi of samples of the second study, we also observed a decrease in stemness after treatment, although not significantly (Figure 3C; p = 0.6886). To further investigate these data, we also observed the differentially expressed genes between (+)-JQ1-treated MDA-MB-231 and DMSO-treated cells. (+)-JQ1-treated cells had 447 upregulated and 1,007 downregulated genes in comparison with DMSO-treated cells. Interestingly, downregulated genes were not enriched for cell cycle–associated pathways in this study but for pathways such as microRNAs in cancer, proteoglycans in cancer, MAPK signaling pathway, PI3K-AKT pathway, pathways in cancer, and TGF-beta receptor signaling.
Discussion
In the present study, we used a one-class logistic regression (OCLR) machine-learning algorithm to quantify cancer stemness and generate a stemness index (mRNAsi) in canine mammary cancer cells and we confirmed that the BET proteins inhibitor (+)-JQ1 is able to decrease stemness in the high mRNAsi CMC cell, CF41.Mg. In addition, using public data, we showed that BET inhibition by (+)-JQ1 decreased stemness in the human TNBC cell line, MDA-MB-231.
Stemness is an important phenotype in cancer progression and malignancy and has been studied using in vivo and in vitro models. In addition, stemness phenotype can exhibit a particular molecular signature. Malta et al. (2018) took advantage of this profile and proposed the OCLR algorithm that can be applied to determine a stemness index in different cells and tissues in addition to being able to reveal the stemness levels in different human cancers such as lung cancer (Liao et al., 2020; Zhang et al., 2020), bladder cancer (Pan et al., 2019), medulloblastoma (Lian et al., 2019), breast cancer (Pei et al., 2020), and glioma (Tan et al., 2021). In this study, we showed that mRNAsi can also be determined in canine species correlating to stemness features.
We applied the OCLR algorithm to four different CMC cells from our cell bank. These cell lines were previously classified into mesenchymal-like cells (M5, M25, and CF41.Mg) and epithelial-like cells (E20) with mesenchymal-like cells being able to generate tumorspheres in low-adherent plates, while E20 was unable to do so (Xavier et al., 2018). In addition, mesenchymal-like cells' upregulated genes were enriched for important pathways associated with epithelial–mesenchymal transition (EMT), invasiveness, and tumorigenicity (Santos et al., 2021). Based on these previously established phenotypes, our hypothesis was that mesenchymal-like cells would exhibit higher mRNAsi than E20 since several studies report an association between EMT and stemness (Fabregat et al., 2016). However, the E20 cell line showed the highest mRNAsi along with CF41.Mg. Despite these findings being controversial, Malta et al. (2018) also observed a negative association between stemness and EMT gene signatures and a positive association between epithelial phenotypes and increased stemness index, which can explain the high mRNAsi in the E20 cell line. Furthermore, the E20 cell line expresses high levels of EpCAM, considered a mediator of stemness and marker of cancer stem cells in breast cancer (Al-Hajj et al., 2003; Visvader and Lindeman, 2008; Munz et al., 2009; Wang et al., 2018). However, the lack of correlation between the mRNAsi and tumorsphere formation in these cells still needs to be better studied and understood. So far, tumorsphere and colony formation, which would feature self-renewal and tumorigenicity, seems to be associated with overexpression of EMT pathways and genes including ZEB1 and ZEB2, in mesenchymal-like CMC cells.
In a previous study, we found that inhibiting BET proteins, an epigenetic reader associated with progression and malignancy in different tumors, by low concentrations of (+)-JQ1 decreased the number of tumorspheres and colony formation of CMC cells. This was accompanied by G2/M cell cycle arrest and downregulation of genes associated with self-renewal pathways including WNT, NOTCH, Hedgehog, and PI3K/AKT/mTOR (Xavier et al., 2019). Here, we confirmed that (+)-JQ1 can also decrease stemness in CF41.Mg tumorspheres. Likewise, we demonstrated that (+)-JQ1, in specific conditions, can decrease stemness in human TNBC cells. Other relevant studies have observed the effects of BET protein inhibition in TNBC. Da Motta et al. (2017) demonstrated that (+)-JQ1 decreased the number of TNBC spheroids and tumor growth targeting FOXM1 and LM O 4 directly impacting angiogenesis, cell proliferation, hypoxia, and metastasis. Furthermore, it was demonstrated that (+)-JQ1 can influence TNBC stemness using different approaches such as overexpression of CSC markers, self-renewal ability, and high invasiveness (Serrano-Oviedo et al., 2020). Different approaches have also been evaluated to further understand possible mechanisms of TNBC resistance to BET inhibitors, and synergistic strategies have been implanted (Shu et al., 2016; Ge et al., 2020). Also, other BET inhibitors such as OTX015 (MK-8628) demonstrated antitumor activity in TNBC using in vitro and in vivo models and are in clinical trials (Vázquez et al., 2017). The potential use of other epigenetic inhibitors against cancer stemness has been reported before when a connectivity map analysis identified entinostat, trichostatin-a, and vorinostat as potential compounds targeting stemness (Malta et al., 2018). All these findings reinforce BET inhibition as a promising strategy to target stemness and, to the best of our knowledge, ours is the first study that investigates the effect of (+)-JQ1 in cancer stemness using a machine learning algorithm.
Here, we show that stemness levels are closely associated with cell cycle and DNA replication. First, we observed that upregulated genes in Hsi E20 and CF41.Mg cell lines were enriched to several pathways associated with cell cycle. Individually, we observed overexpression of Aurora Kinase A (AURKA) and Aurora Kinase B (AURKB) in Hsi cells, considered essential for self-renewal (Eterno et al., 2016; Yang et al., 2017) and stemness (Pei et al., 2020) in breast cancer stem cells. In addition, the Yamanaka factors SOX2, MYC, and KLF4 were found overexpressed in Hsi cells. On the other hand, (+)-JQ1-treated MDA-MB-231 cells showed downregulation of several genes enriched for cell cycle and DNA replication pathways including AURKA and AURKB, and at the same time, important stemness-associated genes such as ZEB2, KIF22, MCM4/6/7 were also downregulated (Morimoto et al., 2020; Dong et al., 2021). We could not find a significant difference in mRNAsi between (+)-JQ1 and DMSO-treated cells in the second study that we investigated, although the stemness seems to decrease after the treatment. Interestingly, cells treated with a smaller concentration of the compound and reduced incubation time exhibited a minor impact of (+)-JQ1 on modulation of gene expression in MDA-MB-231 cells. In the first study, we found 3,218 differentially expressed genes, while only 1,454 genes were disturbed in the second study. Moreover, these genes were not enriched for stemness or cell cycle pathways despite having been enriched for cancer-associated pathways, suggesting that (+)-JQ1 can interfere in different features depending on concentration and incubation time. Thus, the fact that (+)-JQ1 was used at different concentrations and incubation times hinders the comparison between the two studies and is a limitation of our analysis.
In conclusion, our findings support that the mRNAsi algorithm can be used to determine cancer stemness in species other than humans and consist of an unbiased and systematic metric to evaluate stemness at the molecular level. In addition, we confirmed that BET inhibition by (+)-JQ1 decreases stemness in CMCs and, in specific conditions, inhibits this phenotype in human triple-negative breast cancer cells. Finally, we increase the knowledge about the similar mechanisms that orchestrate the phenotype both in canine and human species.
Data availability statement
The datasets presented in this study can be found in online repositories. The names of the repository/repositories and accession number(s) can be found in the article/Supplementary Material.
Author contributions
MM, PX, and TM conducted the study, contributing with concept/design, acquisition of data, and data analysis/interpretation. MM wrote the first version of the manuscript. PX and TM corrected the final version of the manuscript. SM contributed to the interpretation of data and corrected the final version of the manuscript.
Funding
This study has been supported by grants from the Sao Paulo Research Foundation (FAPESP) (2014/02493-7, 2017/11966-4, 2019/05778-6, 2018/00583-0, 2019/14928-1, 2021/00283-9). SM is grateful for support from the Structural Genomics Consortium (SGC), a registered charity (No: 1097737) that receives funds from Bayer AG, Boehringer Ingelheim, Bristol Myers Squibb, Genentech, Genome Canada through Ontario Genomics Institute, Janssen, Merck KGaA, Pzer and Takeda and by the German Cancer Research Center DKTK and the Frankfurt Cancer Institute (FCI). This project has received funding from the Innovative Medicines Initiative 2 Joint Undertaking (JU) under grant agreement No 875510. The JU receives support from the European Union’s Horizon 2020 research and innovation program and EFPIA and Ontario Institute for Cancer Research, Royal Institution for the Advancement of Learning McGill University, Kungliga Tekniska Hoegskolan, Diamond Light Source Limited.
Acknowledgments
The authors are grateful to Prof. Heidge Fukumasu for allowing the use of transcriptomic data from Canine Mammary Cancer cells.
Conflict of interest
The authors declare that the research was conducted in the absence of any commercial or financial relationships that could be construed as a potential conflict of interest.
Publisher’s note
All claims expressed in this article are solely those of the authors and do not necessarily represent those of their affiliated organizations, or those of the publisher, the editors, and the reviewers. Any product that may be evaluated in this article, or claim that may be made by its manufacturer, is not guaranteed or endorsed by the publisher.
Author disclaimer
This communication reflects the views of the authors, and the JU is not liable for any use that may be made of the information contained herein.
Supplementary material
The Supplementary Material for this article can be found online at: https://www.frontiersin.org/articles/10.3389/fddsv.2022.953988/full#supplementary-material
References
Al-Hajj, M., Wicha, M. S., Benito-Hernandez, A., Morrison, S. J., and Clarke, M. F. (2003). Prospective identification of tumorigenic breast cancer cells. Proc. Natl. Acad. Sci. U. S. A. 100 (7), 3983–3988. doi:10.1073/pnas.0530291100
Anders, S., Pyl, P. T., and Huber, W. (2015). HTSeq-A Python framework to work with high-throughput sequencing data. Bioinformatics 31 (2), 166–169. doi:10.1093/bioinformatics/btu638
Baioni, E., Scanziani, E., Vincenti, M. C., Leschiera, M., Bozzetta, E., Pezzolato, M., et al. (2017). Estimating canine cancer incidence: findings from a population-based tumour registry in northwestern Italy. BMC Vet. Res. 13 (1), 203. doi:10.1186/s12917-017-1126-0
Batlle, E., and Clevers, H. (2017). Cancer stem cells revisited. Nat. Med. 23 (10), 1124–1134. doi:10.1038/nm.4409
Borah, A., RochAni, A., Maekawa, T., and Kumar, D. S. (2015). Targeting self-renewal pathways in cancer stem cells: clinical implications for cancer therapy. Oncogenesis 4 (11), e177. doi:10.1038/oncsis.2015.35
Chen, E. Y., Tan, C. M., Kou, Y., Duan, Q., Wang, Z., Meirelles, G. V., et al. (2013). Enrichr: interactive and collaborative HTML5 gene list enrichment analysis tool. BMC Bioinforma. 14 (1), 128. doi:10.1186/1471-2105-14-128
Collignon, J., Lousberg, L., Schroeder, H., and Jerusalem, G. (2016). Triple-negative breast cancer: treatment challenges and solutions. Breast Cancer 8, 93–107. doi:10.2147/BCTT.S69488
Cordeiro, Y. G., Xavier, P. L. P., Rochetti, A. L., Alexandre, P. A., Mori, C. M. C., Strefezzi, R. F., et al. (2018). Transcriptomic profile reveals molecular events associated to focal adhesion and invasion in canine mammary gland tumour cell lines. Vet. Comp. Oncol. 16 (1), E89–E98. doi:10.1111/vco.12339
Da Motta, L. L., LedakI, I., Purshouse, K., Haider, S., De Bastiani, M. A., Baban, D., et al. (2017). The BET inhibitor JQ1 selectively impairs tumour response to hypoxia and downregulates CA9 and angiogenesis in triple negative breast cancer. Oncogene 36 (1), 122–132. doi:10.1038/onc.2016.184
Dawson, M. A., and Kouzarides, T. (2012). Cancer epigenetics: from mechanism to therapy. Cell 150 (1), 12–27. doi:10.1016/j.cell.2012.06.013
Diesch, J., Zwick, A., Garz, A. K., Palau, A., Buschbeck, M., and Gotze, K. S. (2016). A clinical-molecular update on azanucleoside-based therapy for the treatment of hematologic cancers. Clin. Epigenetics 8 (1), 71. doi:10.1186/s13148-016-0237-y
Dobin, A., Davis, C. A., Schlesinger, F., Drenkow, J., Zaleski, C., Jha, S., et al. (2013). STAR: ultrafast universal RNA-seq aligner. Bioinformatics 29 (1), 15–21. doi:10.1093/bioinformatics/bts635
Dong, J., Li, J., Li, Y., Ma, Z., Yu, Y., and Wang, C. Y. (2021). Transcriptional super-enhancers control cancer stemness and metastasis genes in squamous cell carcinoma. Nat. Commun. 12 (1), 3974. doi:10.1038/s41467-021-24137-1
Eterno, V., ZAmbelli, A., ViLLani, L., TuscAno, A., Manera, S., SpitAleri, A., et al. (2016). AurkA controls self-renewal of breast cancer-initiating cells promoting wnt3a stabilization through suppression of MIR-128. Sci. Rep. 6, 28436. doi:10.1038/srep28436
Fabregat, I., Malfettone, A., and Soukupova, J. (2016). New insights into the crossroads between EMT and stemness in the context of cancer. J. Clin. Med. 5 (3), E37. doi:10.3390/jcm5030037
Fenaux, P., Mufti, G. J., Hellstrom-Lindberg, E., Santini, V., Gattermann, N., Germing, U., et al. (2010). Azacitidine prolongs overall survival compared with conventional care regimens in elderly patients with low bone marrow blast count acute myeloid leukemia. J. Clin. Oncol. 28 (4), 562–569. doi:10.1200/JCO.2009.23.8329
García-Alcalde, F., Okonechnikov, K., Carbonell, J., Cruz, L. M., Gotz, S., Tarazona, S., et al. (2012). Qualimap: evaluating next-generation sequencing alignment data. Bioinformatics 28 (20), 2678–2679. doi:10.1093/bioinformatics/bts503
Ge, J. Y., Shu, S., Kwon, M., Jovanovic, B., Murphy, K., Gulvady, A., et al. (2020). Acquired resistance to combined BET and CDK4/6 inhibition in triple-negative breast cancer. Nat. Commun. 11 (1), 2350. doi:10.1038/s41467-020-16170-3
Goldschmidt, M. H., Peña, L., and Zappulli, V. (2016). “Tumors of the mammary gland,” in Tumors in domestic animals. Editor D. J. Meuten (Hoboken, NJ: Wiley Online Books), 723–765. doi:10.1002/9781119181200.ch17
Gupta, K., Sood, N., and Uppal, S. (2012). Epidemiological studies on canine mammary tumour and its relevance for breast cancer studies. IOSR J. Pharm. 2 (2), 322–333. doi:10.9790/3013-0220322333
Jensen, L. J., Kuhn, M., Stark, M., Chaffron, S., Creevey, C., Muller, J., et al. (2009). STRING 8 - a global view on proteins and their functional interactions in 630 organisms. Nucleic Acids Res. 37 (1), 412–416. doi:10.1093/nar/gkn760
Jin, N., George, T. L., Otterson, G. A., Verschraegen, C., Wen, H., Carbone, D., et al. (2021). Advances in epigenetic therapeutics with focus on solid tumors. Clin. Epigenetics 13 (1), 83. doi:10.1186/s13148-021-01069-7
Li, H., Handsaker, B., Wysoker, A., Fennell, T., Ruan, J., Homer, N., et al. (2009). The sequence alignment/map format and SAMtools. Bioinforma. Oxf. Engl. 25 (16), 2078–2079. doi:10.1093/bioinformatics/btp352
Lian, H., Han, Y. P., Zhang, Y. C., Zhao, Y., Yan, S., Li, Q. F., et al. (2019). Integrative analysis of gene expression and DNA methylation through one-class logistic regression machine learning identifies stemness features in medulloblastoma. Mol. Oncol. 13 (10), 2227–2245. doi:10.1002/1878-0261.12557
Liao, Y., Wang, Y., Cheng, M., Huang, C., and Fan, X. (2020). Weighted gene coexpression network analysis of features that control cancer stem cells reveals prognostic biomarkers in lung adenocarcinoma. Front. Genet. 11, 311. doi:10.3389/fgene.2020.00311
Malta, T. M., Sokolov, A., Gentles, A. J., Burzykowski, T., Poisson, L., Weinstein, J. N., et al. (2018). Machine learning identifies stemness features associated with oncogenic dedifferentiation. Cell 173 (2), 338–354. e15. doi:10.1016/j.cell.2018.03.034
Marconato, L., Lorenzo, R. M., AbramoF., , RAtto, A., and Zini, E. (2008). Adjuvant gemcitabine after surgical removal of aggressive malignant mammary tumours in dogs. Vet. Comp. Oncol. 6 (2), 90–101. doi:10.1111/j.1476-5829.2007.00143.x
Morimoto, Y., Mizushima, T., Wu, X., Okuzaki, D., Yokoyama, Y., Inoue, A., et al. (2020). miR-4711-5p regulates cancer stemness and cell cycle progression via KLF5, MDM2 and TFDP1 in colon cancer cells. Br. J. Cancer 122 (7), 1037–1049. Springer US. doi:10.1038/s41416-020-0758-1
Muller, S., Filippakopoulos, P., and Knapp, S. (2011). Bromodomains as therapeutic targets. Expert Rev. Mol. Med. 13, e29. doi:10.1017/S1462399411001992
Munz, M., Baeuerle, P. A., and Gires, O. (2009). The emerging role of EpCAM in cancer and stem cell signaling. Cancer Res. 69 (14), 5627–5629. doi:10.1158/0008-5472.CAN-09-0654
Ng, S. W. K., Mitchell, A., Kennedy, J. A., Chen, W. C., McLeod, J., Ibrahimova, N., et al. (2016). A 17-gene stemness score for rapid determination of risk in acute leukaemia. Nature 540 (7633), 433–437. doi:10.1038/nature20598
Pan, S., Zhan, Y., Chen, X., Wu, B., and Liu, B. (2019). Identification of biomarkers for controlling cancer stem cell characteristics in bladder cancer by network analysis of transcriptome data stemness indices. Front. Oncol. 9, 613. doi:10.3389/fonc.2019.00613
Pei, J., Wang, Y., and Li, Y. (2020). Identification of key genes controlling breast cancer stem cell characteristics via stemness indices analysis. J. Transl. Med. 18 (1), 74. doi:10.1186/s12967-020-02260-9
Pinto, J. P., Kalathur, R. K., Oliveira, D. V., Barata, T., Machado, R. S. R., Machado, S., et al. (2015). StemChecker: a web-based tool to discover and explore stemness signatures in gene sets. Nucleic Acids Res. 43 (W1), W72–W77. doi:10.1093/nar/gkv529
Qiao, J., Chen, Y., Mi, Y., Jin, H., Wang, L., Huang, T., et al. (2020). Macrophages confer resistance to BET inhibition in triple-negative breast cancer by upregulating IKBKE. Biochem. Pharmacol. 180, 114126. doi:10.1016/j.bcp.2020.114126
Ren, C., Zhang, G., Han, F., Fu, S., Cao, Y., Zhang, F., et al. (2018). Spatially constrained tandem bromodomain inhibition bolsters sustained repression of BRD4 transcriptional activity for TNBC cell growth. Proc. Natl. Acad. Sci. U. S. A. 115 (31), 7949–7954. doi:10.1073/pnas.1720000115
Robinson, M. D., McCarthy, D. J., and Smyth, G. K. (2009). edgeR: a Bioconductor package for differential expression analysis of digital gene expression data. Bioinformatics 26 (1), 139–140. doi:10.1093/bioinformatics/btp616
Santos, M. R., Xavier, P. L. P., Pires, P. R. L., Rochetti, A. L., Rosim, D. F., Scagion, G. P., et al. (2021). Oncolytic effect of Newcastle disease virus is attributed to interferon regulation in canine mammary cancer cell lines. Vet. Comp. Oncol. 19 (3), 593–601. doi:10.1111/vco.12699
Serrano-Oviedo, L., Nuncia-Cantarero, M., Morcillo-Garcia, S., Nieto-Jimenez, C., Burgos, M., Corrales-Sanchez, V., et al. (2020). Identification of a stemness-related gene panel associated with BET inhibition in triple negative breast cancer. Cell. Oncol. 43 (3), 431–444. doi:10.1007/s13402-020-00497-6
Shenghui, H., Nakada, D., and Morrison, S. J. (2009). Mechanisms of stem cell self-renewal. Annu. Rev. Cell Dev. Biol. 25 (1), 377–406. doi:10.1146/annurev.cellbio.042308.113248
Shi, J., Wang, Y., Zeng, L., Wu, Y., Deng, J., Zhang, Q., et al. (2014). Disrupting the interaction of BRD4 with diacetylated twist suppresses tumorigenesis in basal-like breast cancer. Cancer Cell 25 (2), 210–225. Elsevier Inc.. doi:10.1016/j.ccr.2014.01.028
Shu, S., Lin, C. Y., He, H. H., Witwicki, R. M., Tabassum, D. P., Roberts, J. M., et al. (2016). Response and resistance to BET bromodomain inhibitors in triple-negative breast cancer. Nature 529 (7586), 413–417. doi:10.1038/nature16508
Simon, D., Schoenrock, D., Baumgärtner, W., and Nolte, I. (2006). Postoperative adjuvant treatment of invasive malignant mammary gland tumors in dogs with doxorubicin and docetaxel. J. Veterinary Intern. Med. 20 (5), 1184–1190. doi:10.1892/0891-6640(2006)20[1184:PATIMM]2.0.CO;2
Sokolov, A., Paull, E. O., and Stuart, J. M. (2016). One-class detection of cell states in tumor subtypes. Pac. Symposium Biocomput. 21, 405–416. doi:10.1142/9789814749411_0037
Stratmann, N., Failing, K., Richter, A., and Wehrend, A. (2008). Mammary tumor recurrence in bitches after regional mastectomy. Veterinary Surg. 37 (1), 82–86. John Wiley & Sons, Ltd. doi:10.1111/j.1532-950X.2007.00351.x
Sung, H., Ferlay, J., Siegel, R. L., Laversanne, M., Soerjomataram, I., Jemal, A., et al. (2021). Global cancer statistics 2020: GLOBOCAN estimates of incidence and mortality worldwide for 36 cancers in 185 countries. CA. Cancer J. Clin. 71 (3), 209–249. doi:10.3322/caac.21660
Szklarczyk, D., Gable, A. L., Lyon, D., Junge, A., Wyder, S., Huerta-Cepas, J., et al. (2019). STRING v11: protein-protein association networks with increased coverage, supporting functional discovery in genome-wide experimental datasets. Nucleic Acids Res. 47 (D1), D607–D613. Oxford University Press. doi:10.1093/nar/gky1131
Tan, J., Zhu, H., Tang, G., Liu, H., Wanggou, S., Cao, Y., et al. (2021). Molecular subtypes based on the stemness index predict prognosis in glioma patients. Front. Genet. 12, 616507. doi:10.3389/fgene.2021.616507
Tran, C. M., Moore, A. S., and Frimberger, A. E. (2016). Surgical treatment of mammary carcinomas in dogs with or without postoperative chemotherapy. Veterinary Comp. Oncol. 14 (3), 252–262. John Wiley & Sons, Ltd. doi:10.1111/vco.12092
Vázquez, R., Riveiro, M. E., Astorgues-Xerri, L., Odore, E., Rezai, K., Erba, E., et al. (2017). The bromodomain inhibitor OTX015 (MK-8628) exerts antitumor activity in triple-negative breast cancer models as single agent and in combination with everolimus. Oncotarget 8 (5), 7598–7613. doi:10.18632/oncotarget.13814
Visvader, J. E., and Lindeman, G. J. (2008). Cancer stem cells in solid tumours: accumulating evidence and unresolved questions. Nat. Rev. Cancer 8 (10), 755–768. doi:10.1038/nrc2499
Wang, M. H., Sun, R., Zhou, X. M., Zhang, M. Y., Lu, J. B., Yang, Y., et al. (2018). Epithelial cell adhesion molecule overexpression regulates epithelial-mesenchymal transition, stemness and metastasis of nasopharyngeal carcinoma cells via the PTEN/AKT/mTOR pathway. Cell Death Dis. 9 (1), 2. doi:10.1038/s41419-017-0013-8
Xavier, P. L. P., Cordeiro, Y. G., Rochetti, A. L., Sangalli, J. R., Zuccari, D. A. P. C., Silveira, J. C., et al. (2018). ZEB1 and ZEB2 transcription factors are potential therapeutic targets of canine mammary cancer cells. Vet. Comp. Oncol. 16 (4), 596–605. doi:10.1111/vco.12427
Xavier, P. L. P., Cordeiro, Y. G., Alexandre, P. A., Pires, P. R. L., Saranholi, B. H., Silva, E. R., et al. (2019). An epigenetic screening determines BET proteins as targets to suppress self-renewal and tumorigenicity in canine mammary cancer cells. Sci. Rep. 9 (1), 17363. doi:10.1038/s41598-019-53915-7
Yang, N., Wang, C., Wang, Z., Zona, S., Lin, S. X., Wang, X., et al. (2017). FOXM1 recruits nuclear Aurora kinase A to participate in a positive feedback loop essential for the self-renewal of breast cancer stem cells. Oncogene 36 (24), 3428–3440. doi:10.1038/onc.2016.490
Zanconato, F., Battilana, G., Forcato, M., Filippi, L., Azzolin, L., Manfrin, A., et al. (2018). Transcriptional addiction in cancer cells is mediated by YAP/TAZ through BRD4. Nat. Med. 24 (10), 1599–1610. doi:10.1038/s41591-018-0158-8
Keywords: (+)-JQ1, comparative oncology, mammary cancer, mRNAsi, stemness index
Citation: Marção M, Müller S, Xavier PLP and Malta TM (2022) Stemness inhibition by (+)-JQ1 in canine and human mammary cancer cells revealed by machine learning. Front. Drug. Discov. 2:953988. doi: 10.3389/fddsv.2022.953988
Received: 26 May 2022; Accepted: 07 July 2022;
Published: 10 August 2022.
Edited by:
Teresita Padilla-Benavides, Wesleyan University, United StatesReviewed by:
Hugo F. Posada-Quintero, University of Connecticut, United StatesTapan Sharma, University of Massachusetts Medical School, United States
Eduardo López-Urrutia, National Autonomous University of Mexico, Mexico
Copyright © 2022 Marção, Müller, Xavier and Malta. This is an open-access article distributed under the terms of the Creative Commons Attribution License (CC BY). The use, distribution or reproduction in other forums is permitted, provided the original author(s) and the copyright owner(s) are credited and that the original publication in this journal is cited, in accordance with accepted academic practice. No use, distribution or reproduction is permitted which does not comply with these terms.
*Correspondence: Pedro Luiz P. Xavier, cG9yZmlyaW94YXZpZXJAdXNwLmJy; Tathiane M. Malta, dGF0aGltYWx0YUB1c3AuYnI=