- 1Faculty of Health Sciences, Instituto de Investigación Biomédica de Málaga (IBIMA), Málaga, Spain
- 2Grupo de Clinimetria (FE-14), Instituto de Investigación Biomédica de Málaga (IBIMA), Málaga, Spain
- 3Faculty of Medicine, Department of Human Anatomy, Legal Medicine and History of Science, Legal Medicine Area, University of Malaga, Malaga, Spain
- 4Physical Medicine and Rehabilitation Unit, Regional University Hospital of Malaga, Malaga, Spain
- 5Instituto de Investigación Médica de Málaga, IBIMA, Malaga, Spain
- 6Mental Health Unit, Regional University Hospital of Malaga, Malaga, Spain
- 7ITWare, Budapest, Hungary
- 8Faculty of Science and Technology, Bournemouth University, Poole, United Kingdom
- 9Arthaus, Production Trade and Service Company Arthaus Doo Import-Export Skopje, Skopje, Macedonia
- 10Sensor ID Snc, Boiano, Italy
- 11School of Clinical Science, Faculty of Health Science, Queensland University Technology, Brisbane, QLD, Australia
Background: Sedentary behavior (SB) negatively impact health and is highly prevalent in the population. Digital behavior change interventions (DBCIs) have been developed to modify behaviors such as SB by technologies. However, it is unknown which behavior change techniques (BCTs) are most frequently employed in SB as well as the effect associated with DBCIs in this field. The aim of this systematic review was: (a) to evaluate the BCT most frequently employed in digital health including all technologies available and interventions aimed at increasing physical activity (PA), reducing sedentary time, and improving adherence to exercise in the clinical population, and (b) to review the effect associated with DBCIs in this field.
Methods: The database used was Medline, as well as Scopus, Scielo, and Google Scholar. For the search strategy, we considered versions of behavior/behavioral, mHealth/eHealth/telemedicine/serious game/gamification. The terms related to PA and SB were included, the criteria for inclusion were randomized clinical trials (RCTs), adults, intervention based on digital media, and outcome variable lifestyle modification; a last 5 years filter was included. Michie's Taxonomy was used to identify BCTs. The study was registered under the number PROSPERO CRD42019138681.
Results: Eighteen RCTs were included in the present systematic review, 5 of them healthy adults, and 13 of them with some illness. Studies included 2298 sedentary individuals who were followed up for 5 weeks−3 years. The most used BCTs were goal setting, problem solving, review outcomes/goals, feedback on behavior and outcomes of behavior, self-monitoring of behavior, social support, information about health consequences, and behavior practice/rehearsal. The effect associated with DBCIs showed improvements, among several related to PA and physiologic self-reported and anthropometric outcomes.
Conclusion: The BCTs most used in digital health to change outcomes related to SB were goals and planning, feedback and monitoring, social support, natural consequences, repetition, and substitution. Besides these findings, DBCIs are influenced by several factors like the type of intervention, patients' preferences and values, or the number of BCTs employed. More research is needed to determine with precision which DBCIs or BCTs are the most effective to reduce SB in the clinical population.
Introduction
Our daily behavior heavily influences our health. According to the County Health Rankings, a 30% variation in health can be accounted for by healthy behaviors, such as smoking, alcohol intake, diet, and physical activity (PA) levels (1). Regarding PA, the World Health Organization (WHO) recommends a minimum of moderate and vigorous-intensity PA per week (2). The WHO defined moderate-intensity PA as any activity with a metabolic equivalent of task (MET) value between 3 and 5, and vigorous intensity PA as ≥6 MET. More recently, the term “sedentary behavior” (SB) was introduced to define any behavior characterized by energy expenditure of 1.5 MET or less, undertaken while in a sitting or reclining posture (3).
The latest research has shown that prolonged SB is positively associated with a range of health concerns including all-cause mortality, cardiovascular disease, type 2 diabetes, metabolic syndrome, and several types of cancers (4, 5). Despite this harmful effect to health, modern society provides many opportunities for prolonged sitting (6) and SB is highly prevalent in the majority of people's time (55–69% of the day) (7). Therefore, behavior change in SB is of great interest.
Behavior change techniques (BCTs) have been defined as irreducible, observable, and replicable components of an intervention designed to redirect behavior (8). In this way BCTs refer to a component of an intervention designed to alter or redirect causal processes that regulate behavior. In 2013, Michie et al. classified 93 hierarchically clustered techniques, distributed in 16 groups, called BCT Taxonomy (v1) (9). The BCT Taxonomy helps to identify the effectiveness of BCTs, including those developed by apps for physical activity (10, 11). According to Yardley et al. (12), digital behavior change interventions (DBCIs) employ digital technologies to support behavior change and can be used to promote health.
Given the rise of new technologies, DBCIs have been developed using technologies such as mobile applications and websites (13). Technology-enhanced solutions such as mobile applications, activity monitors, prompting software, texts, emails, and websites are being harnessed to reduce SB (14). Previous systematic reviews and meta-analyses have studied the beneficial effect of DBCIs on SB by both increasing PA or reducing sedentary time (14–16). However, one of these meta-analyses studied technologies limited to computer, mobile, and wearable technology devices in a healthy population (14); and another meta-analysis including all digital devices/technologies, focused on older adults aged ≥50 years (15).
Currently, there have been different systematic reviews that have studied the beneficial effect of DBCIs on SB by increasing PA, reducing sedentary time, and improving adherence to exercise for digital health in people with different clinical conditions. A systematic review with a meta-analysis (2017) aimed at evaluating the effectiveness of digital technology-enhanced interventions to reduce SB as well as to examine the BCTs used. However, this study was limited to computer, mobile, and wearable technology devices in a healthy population (14). Secondly, there was more recent study (2019) with the design of a systematic review that addressed a wider range of technology devices under the name of Human Computer Interaction. However, it targeted several behaviors. In addition to physical activity and sedentary behavior, it included diet, sleep, smoking, and others. Furthermore, this study also focused on the healthy population (16). In addition to that, another recent systematic review with meta-analysis (2019) was aimed at assessing the efficacy of DBCIs on PA and SB. Although this study included all digital technologies and included people with different clinical conditions, it only focused on older adults aged ≥50 years (15). However, the BCTs used in DBCIs and their usability to reduce SB are not known.
Would it be possible to reduce SB and modify PA habits through exercise proposals with DBCIs in clinical and healthy populations according to published research? The growing research of DBCIs aimed at decreasing SB and the general interest in people with different clinical conditions (also named clinical population) make it necessary to revise this topic. Therefore, this systematic review's objective was to identify more frequently used BCTs and explore the effectiveness of DBCIs used in health interventions to reduce SB, increase PA, and improve adherence to exercise in clinical and healthy populations.
Methods
A systematic review was conducted following PRISMA 2009 guidelines (17). This study aimed to do a systematic review of the evidence concerning the most frequently used BCTs in digital health and the effect associated with DBCIs for reducing SB by increasing PA levels, reducing sedentary time, and improving adherence to exercise in adults. PROSPERO study registration number: CRD42019138681.
To be included in this review, studies had to meet the criteria outlined below (based on the PICO model).
Population: Adults (healthy or clinical population).
Intervention: Exercise proposals through DBCIs with the aim of reducing SB, increasing PA, or exercise adherence.
Control/comparison: Non-DBCIs or general clinical practice.
Outcomes: Exercise questionnaires, clinical variables, exercise measurements (e.g., time registry, inertial sensors).
The database used was Medline through the search engines Pubmed, CINAHL, PEDro, and EMBASE. Results were completed with the databases Scopus, Scielo, and Google Scholar. A filter of the last five years was applied to obtain the best possible evidence. The search was performed between May and June, 2019.
For the search strategy, we considered different variations of the terms (“behavior” OR “behavior” OR “behavioral”) AND (“mHealth” OR “eHealth” OR telemedicine OR “gamification” OR “serious game”). In the same way, the terms related to sedentary behavior (“sedentar* behavior*),” levels of PA (“exercise*,” “physical activity”), and adherence (“adherence”) to exercise were included.
The inclusion criteria for the studies were randomized clinical trials, PEDro scale score more than 5 (18), adult population, intervention based on digital media (mobile phone, tablet, computer, web systems, and recommender systems), BCTs used, and outcome variable lifestyle modification (related to PA, SB, or exercise adherence). The languages of the manuscripts should be English, French, Italian, Portuguese, or Spanish. The defined exclusion criteria were systematic reviews, meta-analyses, no use of BCTs, and absence of goals related to SB or PA.
Three independent reviewers participated in each of the study selection phases. The search results were filtered by title and abstract according to eligibility criteria before full reading. Conflicts of inclusion were resolved by agreement between reviewers or by a fourth reviewer. A 4-phase flow chart of PRISMA (17) was performed to correctly stratify the results of the search (Figure 1). The internal and external validity of the manuscript included in the systematic review was evaluated by means of the PEDro scale (18) (Table 1). For each of the manuscripts included in the review, the following elements were identified: author, year of publication, population, age of the groups involved, distribution of the groups (Table 2), outcome related to SB, PA, or exercise adherence, and change relative to BCT (Table 3). We used Michie's Taxonomy to identify the different BCTs used in the studies (9).
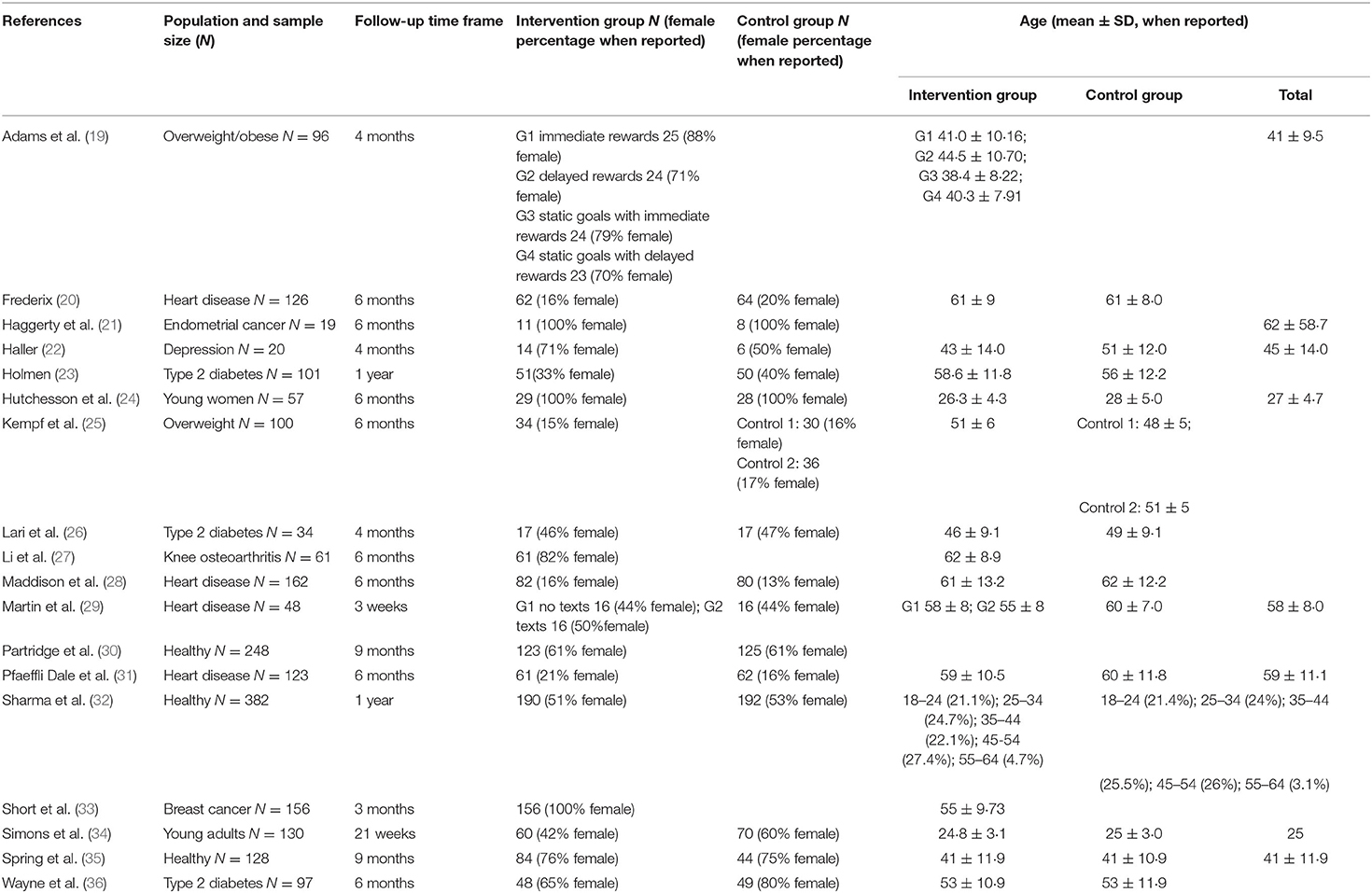
Table 2. Population data of the 18 randomized control trials that report behavior change techniques used in digital health interventions for reducing sedentary behavior.
Results
We identified 1297 articles in five searches. We retrieved 96 papers for full-text review, of which 18 RCT studies were identified as eligible for inclusion (Figure 1). Therefore, we analyzed individual data from the 18 RCTs with the PEDro internal validity scale (Table 1).
They all provided data on BCTs used in DBCIs to change SB. Studies population were all sedentary subjects, 5 of them healthy adults (24, 30, 32, 34, 35), and 13 of them with some pathological diagnosis [overweight/obese (19, 25), type 2 diabetes mellitus (23, 26, 36), heart disease (20, 28, 29, 31), cancer (21, 33), depression (22), and osteoarthritis (27) (Table 2)]. These studies included 2,298 individuals who were followed up for 5 weeks−3 years.
BCTs from Michie's Taxonomy were identified in the 18 RCTs. The most used were goal setting, problem solving, review outcomes/goals, feedback on behavior and outcomes of behavior, self-monitoring of behavior, social support, information about health consequences, and behavior practice/rehearsal (Table 3). All the interventions used text messages and most of them added other communication tools (calls, emails, among others), mobile apps, and some type of activity tracker. Types of interventions, control comparison, and outcomes measured in the 18 RCTs can be seen in Table 4, where the reader may also find the effects of intervention associated with DBCIs.
Discussion
This systematic review analyzed the BCTs most frequently used and the effectiveness of DBCIs used in health interventions aimed at reducing SB, increasing PA, and improving adherence to exercise in clinical and healthy populations.
Regarding the BCTs most frequently used in DBCIs to change outcomes related to SB, results showed that they were goals and planning, feedback and monitoring, social support, natural consequences, repetition, and substitution (Table 3). Therefore, we recognize them as the most important psychological strategies to improve SB change from the 19 groups of BCTs proposed by Michie et al. (9). These findings concur with previous literature showing that the most widely used BCTs are self-monitoring, goal-setting, credible source, feedback on behavior, and social support (13, 15). Among these groups of BCTs, the most frequently used was goal setting (n = 10) as part of the goals and planning cluster (Table 3). More specifically, it was used in five studies involving healthy adults aimed at promoting PA (19, 29, 30, 34, 35) and other five studies involving patients suffering from cardiac-related disease (28, 31), cancer (33), diabetes (36), or musculoskeletal impairments. (27). The second most used BCT was self-monitoring of behavior (n = 9) as part of the feedback and monitoring cluster. However, only two out of nine studies included healthy adults (19, 35). In light of these findings, goal setting is more frequently used in healthy populations than self-monitoring of behavior.
A previous meta-analysis limited to studies in a healthy population found that the most frequently used BCTs were “prompts and cues,”, “self-monitoring of behavior,” “social support,” and “goal settings,” which were coded between 10 and 5 times (14). However, in this study, only 20/93 available BCTs were used (14). Another meta-analysis that focused on adults aged ≥50 years found that the most common BCTs were “social support,” “goal setting,” “feedback on behavior,” and “self-monitoring” (15). These last three BCTs along with “credible source” comprise the most common BCTs in a meta-analysis targeting PA and diet in cancer survivors, although no studies were found to assess SB in this cancer population (16). These BCTs are among the most used in the present systematic review (Table 3).
Regarding the effect associated with DBCIs, the interventions included in the 18 RCTs showed improvements among several outcomes such as an increase in daily steps, MET, and International Physical Activity Questionnaire (IPAQ). When the clinical population (non-healthy) was evaluated, other complementary outcomes were addressed as well as improvements in self-reported outcomes such as quality of life, self-efficacy, or barriers to exercise, and improvements in anthropometric outcomes such as weight loss or body circumferences (Table 4). Smart texts were grouped as positive reinforcement messages, sent when a participant was on track to attain or had already attained his or her daily goal, and booster messages, to motivate individuals when they were not tracking to surpass their step goal (29, 32). Short message service (SMS) based on a health promotion model could be used to change beliefs (self-efficacy, family support, coping with barriers) and physical activity (25).
There is evidence that DBCIs to promote PA and/or reduce SB result in increases in total PA and reductions in SB in older adults aged ≥50 years, at least in the short term (15). Stephenson et al. (14), in their meta-analysis on interventions in the workplace, found that SB showed a mean reduction of 40 min/workday in the intervention group at the endpoint follow-up. Greater reductions in SB were found in studies where self-report/proxy measures (53 min/day) of SB were used compared to objective measures (35 min/day) (14).
Besides findings regarding the effects associated with DBCIs, the interventions employed among studies are not common, so it is not possible to know the implication of BCTs among interventions, which concur with previous literature. A systematic review showed that DBCIs are an effective tool to increase PA and reduce body mass index in cancer survivors. However, authors agreed that outcome measures were not common (13). Likewise, the theoretical models applied are not clearly described by the authors (13–15). In this way, risk of bias and heterogeneity of this type of study was high. This is due to the fact that there is a wide variability of DBCIs, different types of intervention, and different assessment instruments (13, 14). Despite this, studies show an increase in vigorous PA, a reduction in body mass index, and a reduction in fatigue in cancer patients (13). Also, the use of computer, mobile, and wearable technology have been shown to be effective tools to reduce SB. Likewise, the permanence of life habits acquired in different studies must be analyzed in the long term through follow-up and without complementary behavior change systems (15).
Besides differences in DBCIs employed, there are several factors that may influence their effectiveness. One of them is the time slot. For example, immediate rewards have been shown to be more effective at stimulating change behavior than delayed rewards in overweight/obese adults. In addition, adaptive goal-setting encourages better goal keeping in the long-term than static goals (19). Another factor is the number of BCTs employed. Joining different BCTs and self-monitoring of the behavior has a summative effect, as was seen in Kempf's study (25). Overweight adults equipped with telemonitoring devices (scales and pedometers) and supported by mental coaches, demonstrated large reductions of body weight after 12 months of lifestyle intervention and the within-group analysis revealed a larger reduction in body weight for the intervention with the combination of telemedical coaching and telemonitoring of PA than only telemonitoring (23). Wayne (36) shows similar data about the summative effect between coaching and monitoring, they found accelerated improvement in the group with mobile phone monitoring added to a health coaching strategy than in the group with coaching alone within a diabetes population. They describe a faster improved adoption and adherence to health behaviors with the combined strategy (36). In this way, delivering tailored modules on a monthly schedule and combining them with weekly action planning is a good example to improve PA in breast cancer survivors (33). The use of self-management techniques in exercise programs could enhance PA adherence among healthy subjects and the clinical population [e.g., knee osteoarthritis (27) or heart disease (29)], encouraging users to keep their motivation through the combination of message strategies and interventions similar to pedometer data through a Smartphone interface.
Lately, other factors influencing the effects of DBCIs have been difficult to analyze, like patients' preferences and values, namely the patient-centered approach. It is associated with a higher rate of patient satisfaction, better adherence to suggested lifestyle changes, and more cost-effective care (37). However, in most systematic reviews, a person-based approach is limited to a few examples, and there is a lack of defining user characteristics in digital health (12). Another factor is the number of BCTs employed, as evidence suggests that incorporating a multitude of BCTs is more effective than those using few or a single BCT in terms of long-term changes (38). For example, in older patients, a minimum of three groups of BCTs are necessary to obtain significant effects on PA (15). Finally, the social, cultural context and environment are key factors influencing SB and must be taken into consideration when developing DBCIs targeting SB (39).
However, the effectiveness and costs of real-time remote exercise monitoring and coaching to traditional face-to-face rehabilitation must be considered (28). This kind of intervention is at least as favorable as a face-to-face program. Immediate post intervention feedback and at longer-term follow-up (24 weeks) could be considered in this type of intervention (28). Moreover, remote intervention realized a 70% reduction in programmer delivery costs, and very few adverse events were attributed to participation in the programmer. Specialists provided real-time individualized audio coaching, feedback, and social support throughout real-time exercise monitoring (28).
The limitation of the present study refers to data analysis. This systematic review focused on the last five years due to the existence of similar studies; however, this ensured that the most recent studies would be captured. The studies included in the systematic review did not have an outcome variable measured with a common instrument and sometimes did not offer numerical data on the changes produced. This fact makes statistical treatment difficult through meta-analysis. For future RCTs evaluating DBCIs in SB that could be assessed in a meta-analysis, standardized outcomes would be recommended, at least the most studied, such as daily steps, MET, and levels of PA measured by IPAQ (see Table 4). In addition, current evidence showed a high heterogeneity in the clinical population. A greater number of RCTs would allow further meta-analysis in a given clinical population. Besides the mentioned limitations, this is the first study to analyze in depth and compare the results of applying BCTs in increasing PA or interrupting SB in different populations. The demonstrated effectiveness of BCTs in intervention protocols related to PA could be implemented in other types of treatment to increase adherence.
Conclusion
The present systematic review showed that the most common BCTs aimed at reducing SB in DBCIs were goal setting, problem solving, review outcomes/goals, feedback on behavior and outcomes of behavior, self-monitoring of behavior, social support, information about health consequences, and behavior practice/rehearsal. The DBCIs among studies included revealed beneficials effects in outcomes such as an increase in MET, PA levels, or daily steps; improvements in self-reported outcomes such as quality of life, self-efficacy, or barriers to exercise; and improvements in anthropometric outcomes such as weight loss or body circumferences. Besides these findings, DBCIs are influenced by several factors like the type of intervention, patients' preferences, and values or the number of BCTs employed. Therefore, more research is required to determine with precision which DBCIs are the most effective to reduce SB in the clinical population.
Data Availability Statement
The original contributions presented in the study are included in the article/Supplementary Material, further inquiries can be directed to the corresponding author/s.
Author Contributions
JM-M, CR-J, and ID-T: data search. AC-V and WT: conceptualization. AE-E, AM-C, MR-M, and MG-S: formal analysis. AB, WT, AS, AC-V, BN, and FM-C: funding acquisition, project administration, and supervision. JM-M, CR-J, ID-T, and AC-V: methodology. JM-M, CR-J, ID-T, and MR-M: writing—original draft. JM-M, CR-J, I-DT, AE-E, AM-C, MR-M, MG-S, AB, WT, AS, AC-V, BN, and FM-C: writing—review and editing. All authors contributed to the article and approved the submitted version.
Funding
This project received funding from the European Union's Horizon 2020 research and innovation program under the Marie Sklodowska-Curie grant agreement, no. 823871 (iGame).
Conflict of Interest
The authors declare that the research was conducted in the absence of any commercial or financial relationships that could be construed as a potential conflict of interest.
Supplementary Material
The Supplementary Material for this article can be found online at: https://www.frontiersin.org/articles/10.3389/fdgth.2021.620383/full#supplementary-material
References
1. Hood CM, Gennuso KP, Swain GR, Catlin BB. county health rankings: relationships between determinant factors and health outcomes. Am J Prev Med. (2016) 50:129–35. doi: 10.1016/j.amepre.2015.08.024
2. WHO. Physical activity and adults. WHO. Available online at: https://www.who.int/dietphysicalactivity/factsheet_adults/en/ (accessed May 13, 2019).
3. Tremblay MS, Aubert S, Barnes JD, Saunders TJ, Carson V, Latimer-Cheung AE, et al. Sedentary behavior research network (SBRN)—terminology consensus project process and outcome. Int J Behav Nutr Phys Act. (2017) 14:75. doi: 10.1186/s12966-017-0525-8
4. de Rezende LFM, Rodrigues Lopes M, Rey-López JP, Matsudo VKR, Luiz O do C. Sedentary behavior and health outcomes: an overview of systematic reviews. PLoS ONE. (2014) 9:e105620. doi: 10.1371/journal.pone.0105620
5. Wirth K, Klenk J, Brefka S, Dallmeier D, Faehling K, Roqué I, et al. Biomarkers associated with sedentary behaviour in older adults: a systematic review. Ageing Res Rev. (2017) 35:87–111. doi: 10.1016/j.arr.2016.12.002
6. Same RV, Feldman DI, Shah N, Martin SS, Al Rifai M, Blaha MJ, et al. Relationship between sedentary behavior and cardiovascular risk. Curr Cardiol Rep. (2016) 18:6. doi: 10.1007/s11886-015-0678-5
7. Hansen BH, Kolle E, Dyrstad SM, Holme I, Anderssen SA. Accelerometer-determined physical activity in adults and older people. Med Sci Sports Exerc. (2012) 44:266–72. doi: 10.1249/MSS.0b013e31822cb354
8. Michie S, Abraham C, Eccles MP, Francis JJ, Hardeman W, Johnston M. Strengthening evaluation and implementation by specifying components of behaviour change interventions: a study protocol. Implement Sci IS. (2011) 6:10. doi: 10.1186/1748-5908-6-10
9. Michie S, Richardson M, Johnston M, Abraham C, Francis J, Hardeman W, et al. The behavior change technique taxonomy (v1) of 93 hierarchically clustered techniques: building an international consensus for the reporting of behavior change interventions. Ann Behav Med Publ Soc Behav Med. (2013) 46:81–95. doi: 10.1007/s12160-013-9486-6
10. Conroy DE, Yang C-H, Maher JP. Behavior change techniques in top-ranked mobile apps for physical activity. Am J Prev Med. (2014) 46:649–52. doi: 10.1016/j.amepre.2014.01.010
11. Direito A, Dale LP, Shields E, Dobson R, Whittaker R, Maddison R. Do physical activity and dietary smartphone applications incorporate evidence-based behaviour change techniques? BMC Public Health. (2014) 14:646. doi: 10.1186/1471-2458-14-646
12. Yardley L, Choudhury T, Patrick K, Michie S. Current issues and future directions for research into digital behavior change interventions. Am J Prev Med. (2016) 51:814–5. doi: 10.1016/j.amepre.2016.07.019
13. Roberts AL, Fisher A, Smith L, Heinrich M, Potts HWW. Digital health behaviour change interventions targeting physical activity and diet in cancer survivors: a systematic review and meta-analysis. J Cancer Surviv Res Pract. (2017) 11:704–19. doi: 10.1007/s11764-017-0632-1
14. Stephenson A, McDonough SM, Murphy MH, Nugent CD, Mair JL. Using computer, mobile and wearable technology enhanced interventions to reduce sedentary behaviour: a systematic review and meta-analysis. Int J Behav Nutr Phys Act. (2017) 14:105. doi: 10.1186/s12966-017-0561-4
15. Stockwell S, Schofield P, Fisher A, Firth J, Jackson SE, Stubbs B, et al. Digital behavior change interventions to promote physical activity and/or reduce sedentary behavior in older adults: a systematic review and meta-analysis. Exp Gerontol. (2019) 120:68–87. doi: 10.1016/j.exger.2019.02.020
16. Wang Y, Fadhil A, Reiterer H. Health behavior change in HCI: trends, patterns, and opportunities. ArXiv190110449 Cs. (2019) 2.
17. Liberati A, Altman DG, Tetzlaff J, Mulrow C, Gøtzsche PC, Ioannidis JPA, et al. The PRISMA statement for reporting systematic reviews and meta-analyses of studies that evaluate health care interventions: explanation and elaboration. PLoS Med. (2009) 6:e1000100. doi: 10.1371/journal.pmed.1000100
18. Yamato TP, Maher C, Koes B, Moseley A. The PEDro scale had acceptably high convergent validity, construct validity, and interrater reliability in evaluating methodological quality of pharmaceutical trials. J Clin Epidemiol. (2017) 86:176–81. doi: 10.1016/j.jclinepi.2017.03.002
19. Adams MA, Hurley JC, Todd M, Bhuiyan N, Jarrett CL, Tucker WJ, et al. Adaptive goal setting and financial incentives: a 2 ×2 factorial randomized controlled trial to increase adults' physical activity. BMC Public Health. (2017) 17:286. doi: 10.1186/s12889-017-4231-x
20. Frederix I, Hansen D, Coninx K, Vandervoort P, Vandijck D, Hens N, et al. Medium-term effectiveness of a comprehensive internet-based and patient-specific telerehabilitation program with text messaging support for cardiac patients: randomized controlled trial. J Med Internet Res. (2015) 17:e185. doi: 10.2196/jmir.4799
21. Haggerty AF, Hagemann A, Barnett M, Thornquist M, Neuhouser ML, Horowitz N, et al. A randomized, controlled, multicenter study of technology-based weight loss interventions among endometrial cancer survivors. Obes Silver Spring Md. (2017) 25 (Suppl 2):S102–8. doi: 10.1002/oby.22021
22. Haller N, Lorenz S, Pfirrmann D, Koch C, Lieb K, Dettweiler U, et al. Individualized web-based exercise for the treatment of depression: randomized controlled trial. JMIR Ment Health. (2018) 5:e10698. doi: 10.2196/10698
23. Holmen H, Torbjørnsen A, Wahl AK, Jenum AK, Småstuen MC, Arsand E, et al. A mobile health intervention for self-management and lifestyle change for persons with type 2 diabetes, part 2: one-year results from the norwegian randomized controlled trial renewing health. JMIR MHealth UHealth. (2014) 2:e57. doi: 10.2196/mhealth.3882
24. Hutchesson MJ, Callister R, Morgan PJ, Pranata I, Clarke ED, Skinner G, et al. A targeted and tailored ehealth weight loss program for young women: the be positive be healthe randomized controlled trial. Healthc Basel Switz. (2018) 6:39 doi: 10.3390/healthcare6020039
25. Kempf K, Röhling M, Martin S, Schneider M. Telemedical coaching for weight loss in overweight employees: a three-armed randomised controlled trial. BMJ Open. (2019) 9:e022242. doi: 10.1136/bmjopen-2018-022242
26. Lari H, Noroozi A, Tahmasebi R. Impact of short message service (SMS) education based on a health promotion model on the physical activity of patients with type ii diabetes. Malays J Med Sci MJMS. (2018) 25:67–77. doi: 10.21315/mjms2018.25.3.7
27. Li LC, Sayre EC, Xie H, Falck RS, Best JR, Liu-Ambrose T, et al. Efficacy of a community-based technology-enabled physical activity counseling program for people with knee osteoarthritis: proof-of-concept study. J Med Internet Res. (2018) 20:e159. doi: 10.2196/jmir.8514
28. Maddison R, Rawstorn JC, Stewart RAH, Benatar J, Whittaker R, Rolleston A, et al. Effects and costs of real-time cardiac telerehabilitation: randomised controlled non-inferiority trial. Heart Br Card Soc. (2019) 105:122–9. doi: 10.1136/heartjnl-2018-313189
29. Martin SS, Feldman DI, Blumenthal RS, Jones SR, Post WS, McKibben RA, et al. mActive: a randomized clinical trial of an automated mHealth intervention for physical activity promotion. J Am Heart Assoc Cardiovasc Cerebrovasc Dis. (2015) 4:e002239. doi: 10.1161/JAHA.115.002239
30. Partridge SR, McGeechan K, Bauman A, Phongsavan P, Allman-Farinelli M. Improved confidence in performing nutrition and physical activity behaviours mediates behavioural change in young adults: mediation results of a randomised controlled mHealth intervention. Appetite. (2017) 108:425–33. doi: 10.1016/j.appet.2016.11.005
31. Pfaeffli Dale L, Whittaker R, Jiang Y, Stewart R, Rolleston A, Maddison R. Text message and internet support for coronary heart disease self-management: results from the text4heart randomized controlled trial. J Med Internet Res. (2015) 17:e237. doi: 10.2196/jmir.4944
32. Sharma M, Banerjee B, Ingle GK, Garg S. Effect of mHealth on modifying behavioural risk-factors of non-communicable diseases in an adult, rural population in Delhi, India. mHealth. (2017) 3:42. doi: 10.21037/mhealth.2017.08.03
33. Short CE, Rebar A, James EL, Duncan MJ, Courneya KS, Plotnikoff RC, et al. How do different delivery schedules of tailored web-based physical activity advice for breast cancer survivors influence intervention use and efficacy? J Cancer Surviv Res Pract. (2017) 11:80–91. doi: 10.1007/s11764-016-0565-0
34. Simons D, De Bourdeaudhuij I, Clarys P, De Cocker K, Vandelanotte C, Deforche B. Effect and process evaluation of a smartphone app to promote an active lifestyle in lower educated working young adults: cluster randomized controlled trial. JMIR MHealth UHealth. (2018) 6:e10003. doi: 10.2196/10003
35. Spring B, Pellegrini C, McFadden HG, Pfammatter AF, Stump TK, Siddique J, King AC, Hedeker D. Multicomponent mHealth intervention for large, sustained change in multiple diet and activity risk behaviors: the make better choices 2 randomized controlled trial. J Med Internet Res. (2018) 20:e10528. doi: 10.2196/10528
36. Wayne N, Perez DF, Kaplan DM, Ritvo P. Health coaching reduces HbA1c in Type 2 diabetic patients from a lower-socioeconomic status community: a randomized controlled trial. J Med Internet Res. (2015) 17:e224. doi: 10.2196/jmir.4871
38. Pagoto S, Bennett GG. How behavioral science can advance digital health. Transl Behav Med. (2013) 3:271–6. doi: 10.1007/s13142-013-0234-z
Keywords: behavior, change, technique, digital intervention, sedentary behavior
Citation: Martín-Martín J, Roldán-Jiménez C, De-Torres I, Muro-Culebras A, Escriche-Escuder A, Gonzalez-Sanchez M, Ruiz-Muñoz M, Mayoral-Cleries F, Biró A, Tang W, Nikolova B, Salvatore A and Cuesta-Vargas AI (2021) Behavior Change Techniques and the Effects Associated With Digital Behavior Change Interventions in Sedentary Behavior in the Clinical Population: A Systematic Review. Front. Digit. Health 3:620383. doi: 10.3389/fdgth.2021.620383
Received: 22 October 2020; Accepted: 04 May 2021;
Published: 08 July 2021.
Edited by:
Wendy Chapman, The University of Melbourne, AustraliaReviewed by:
Jeff Sigafoos, Victoria University of Wellington, New ZealandXia Jing, Clemson University, United States
Copyright © 2021 Martín-Martín, Roldán-Jiménez, De-Torres, Muro-Culebras, Escriche-Escuder, Gonzalez-Sanchez, Ruiz-Muñoz, Mayoral-Cleries, Biró, Tang, Nikolova, Salvatore and Cuesta-Vargas. This is an open-access article distributed under the terms of the Creative Commons Attribution License (CC BY). The use, distribution or reproduction in other forums is permitted, provided the original author(s) and the copyright owner(s) are credited and that the original publication in this journal is cited, in accordance with accepted academic practice. No use, distribution or reproduction is permitted which does not comply with these terms.
*Correspondence: Antonio I. Cuesta-Vargas, YWN1ZXN0YUB1bWEuZXM=
†These authors have contributed equally to this work