- 1Program in Physical Therapy, Washington University School of Medicine, St. Louis, MO, United States
- 2Department of Neurology, Washington University School of Medicine, St. Louis, MO, United States
- 3Program in Occupational Therapy, Washington University School of Medicine, St. Louis, MO, United States
- 4Department of Psychological & Brain Sciences, Washington University in St. Louis, St. Louis, MO, United States
- 5Department of Psychiatry, Washington University School of Medicine, St. Louis, MO, United States
- 6Shirley Ryan Ability Lab, Chicago, IL, United States
- 7Division of Cardiology, Washington University School of Medicine, St. Louis, MO, United States
Introduction: Rehabilitation is facing a critical practice gap: Patients seek out rehabilitation services to improve their activity in daily life, yet recent work demonstrates that rehabilitation may be having a limited impact on improving this outcome due to lack of objective data on patients’ activity in daily life. Remote monitoring using wearable sensor technology is a promising solution to this address this gap. The purpose of this study was to understand patient and clinician awareness of the practice gap and preferences for integrating wearable sensor technology into rehabilitation care.
Methods: This study used a mixed-methods approach consisting of surveys and 1:1 interviews with clinicians (physical and occupational therapists or assistants) employed at an outpatient rehabilitation clinic within an academic medical center and patients seeking care at this clinic. Data were analyzed using descriptive statistics and thematic analysis.
Results: Data saturation was reached from nineteen clinicians and ten patients. Both clinicians and patients recognized the importance of measuring activity outside the clinic and viewed wearable sensor technology as an objective measurement tool. Most clinicians (63%) preferred continuous (vs. intermittent) monitoring within a care episode and most patients (60%) were willing to sync their sensor data as often as instructed by their provider. To maximize integration into clinical workflows, clinicians voiced a preference for availability of sensor data in the electronic health record.
Conclusions: Clinicians and patients value the use of wearable sensor technology to improve measurement of activity outside the clinic environment and expressed preferences for how this technology could best be integrated into routine rehabilitation care.
Introduction
Rehabilitation clinicians (e.g., physical and occupational therapists) are key providers for improving movement and overall function in individuals with disabilities. One of the most common reasons patients seek out rehabilitation services is to improve their upper and/or lower limb (e.g., walking) activity in daily life (1, 2). In-clinic measures of a person's capacity for activity (i.e., what a person can do measured by standardized assessments) have historically been used as a surrogate measure of what a person actually does (activity performance) in daily life (3). This assumption posits that if a person's capacity for activity improves that they will have resultant improvements in their activity performance in daily life (4). Results from several seminal studies, however, have disproven this assumption and reinforced that activity capacity and activity performance are different constructs (5–8).
A clinical trial of people with chronic stroke randomized participants into one of three interventions groups to determine which intervention would yield the greatest change in activity performance (measured by steps/day using an activity tracker): a high-intensity walking intervention, a step activity monitoring behavioral intervention, or a group that received both interventions (8). Results showed that the interventions had differential effects on activity capacity vs. activity performance in daily life and that improvements in activity capacity were not necessarily accompanied by improvements in activity performance in daily life (see Table 3 in Thompson et al., 2024 for details) (8). Similarly, a longitudinal cohort study enrolling people receiving outpatient rehabilitation care demonstrated that this discrepancy is not unique to the research realm but exists in actual clinic practice in which the majority (59%) of participants improved in their activity capacity but not in their activity performance in daily life (5). A possible explanation for these findings was that only 21% of clinician- documented goals were aimed at improving activity performance in daily life (9). These data highlight a critical practice gap between the goals of patients (improving activity performance in daily life) and what is typically measured and intervened on in rehabilitation practice (activity capacity) and suggest that rehabilitation may not yet be optimized for improving outcomes most salient to patients (1, 2).
One potential explanation for this practice gap is whether or not clinicians are aware of the gap between activity capacity and activity performance. If clinicians are not aware of the practice gap, then efforts to address this gap in clinical practice will fall short (10). A second potential explanation is that the tools needed to directly measure activity performance in daily life, such as wearable sensors, are either unavailable or rarely used in most rehabilitation clinics (4, 11). Rehabilitation clinicians have historically relied on self-report measures of activity performance in daily life, but previous work has shown that a person's self-report of their activity performance in daily life is neither consistent nor accurate compared to direct (sensor-based) measures (12–14). A third potential reason for this practice gap is the lack of technology integration into clinical workflows, which is a common barrier to the adoption of many digital health interventions in healthcare settings (15–17). To explore these potential reasons, stakeholder engagement is needed to: (1) understand awareness of the problem, (2) identify key barriers and facilitators that should be considered in implementation protocols, and (3) design workflows that seamlessly integrate the technology into rehabilitation practice and pose minimal burden on stakeholders (10, 18).
While previous studies have investigated clinician and patient perceptions on wearable sensors (19, 20) and digital health technology more generally (21–23), no studies have investigated clinician and patient perspectives on using wearables sensors to monitor activity performance in daily life within a rehabilitation care episode. To facilitate integration of wearable sensor technology into rehabilitation practice, we employed a mixed methods approach consisting of surveys and one-on-one interviews with patients and clinicians to answer four research questions (RQ):
RQ1. How do clinicians perceive the constructs of activity capacity and activity performance and their relationship in outpatient rehabilitation?
RQ2. How do clinicians and patients perceive the value of activity performance monitoring in outpatient rehabilitation care?
RQ3. What approaches, if any, are currently being used to measure activity performance in daily life in outpatient rehabilitation practice, and what are important considerations regarding these approaches?
RQ4. What are the data collection and workflow preferences of clinicians and patients for integrating wearable sensor technology into rehabilitation care?
Methods
Participants
Licensed physical and occupational therapist clinicians employed within Washington University's outpatient rehabilitation system were recruited using targeted email blasts and flyers. Targeted emails helped ensure the clinician sample was representative of both physical and occupational therapists who were treating a variety of movement problems resultant from both neurological and musculoskeletal diagnoses, and that the sample included clinicians with a range of experience using activity performance monitoring in their practice. Patients were recruited via clinician referral and included if they met the following criteria: (1) currently being seen in outpatient rehabilitation at Washington University for upper and/or lower limb problems, (2) access to a mobile phone, and (3) no significant cognitive or communication deficits that would limit their ability to participate in an interview with a research team member. Access to a mobile phone was required, as some of the interview questions asked about preferences for syncing data via a mobile app. Washington University Human Research Protection Office approved the study, and all participants signed informed consent prior to engaging in study activities.
Procedures
All participants completed two surveys, a demographic survey and a survey evaluating their experiences with wearable sensor technology, and a one-on-one interview. Participants were provided the option to complete the surveys electronically using REDCap (Research Electronic Data Capture) (24, 25) or in-person prior to the start of the interview. Interviews were semi-structured, approximately 45 min in length, and completed in person (in an outpatient rehabilitation clinic) or via Zoom. Patient-specific and clinician-specific semi-structured interview guides were created, reviewed, and revised by study team members, which included rehabilitation clinicians, researchers, and educators, until consensus was achieved. Interview guides included questions exploring global reflections on concepts related to wearable sensor technology and questions to support individual clinic efforts to integrate wearable sensors into rehabilitation practice (e.g., data collection and workflow integration preferences). Table 1 summarizes the quantitative and qualitative question response data that was used to answer each research question. Prior to the first interview, an in-service was provided to clinicians to discuss the project and answer any questions. All interviews were conducted by two of the authors (AEM or CLH) and audio recorded and transcribed using Zoom. To ensure accuracy in the transcribed data, a research team member conducted a thorough review of all interview transcripts against the original audio recordings and made necessary corrections prior to analysis.
Data analysis
Demographic and quantitative survey response data were analyzed using descriptive statistics in R (R Core Team 2021, version 4.2.1) (26). These statistics included medians, interquartile ranges, counts and percentages, depending on the type of data. Interview data were analyzed through a deductive and inductive thematic approach depending on the research question (27, 28). Each interview was independently coded by two researchers, AEM and CLH, using the qualitative research software, HyperRESEARCH. Each clinician and patient's narrative transcript served as a “case” for analysis. For RQ1, AEM and CLH separately coded clinicians' responses to determine if their description of the constructs of activity capacity and activity performance matched published definitions (3) in order to quantify whether the clinician did or did not have an understanding of these constructs as they relate to their clinical practice. A third researcher, MDB, was used when there was a discrepancy between the two primary coders. For RQ 2–4, the initial coding process involved inductively identifying codes based on the clinician's and patient's own words as they related to each research question (27, 28). After independently coding 3–5 interviews, AEM and CLH held meetings to discuss and group similar codes, refining and creating a revised code book to guide further coding for each research question. For example, in regard to RQ3 exploring approaches to monitoring activity performance in daily life, “cost/access” and “insurance/reimbursement concerns” were highlighted as two separate considerations brought up by two different participants. These two codes were combined to generate the theme “Cost/access considerations for patients to purchase a wearable sensor” since both codes included cost issues for purchasing a wearable sensor. This iterative process continued as AEM and CLH independently coded additional sets of 3–5 transcripts, followed by meetings to discuss, revise, and consolidate codes into themes organized by each research question. This iterative coding, revision, and theme generation process continued until all cases were coded and new codes related to each research question ceased to emerge. Clinicians and patients were recruited until data saturation for each cohort was reached and no new codes were gleaned from additional interviews. After all interviews had been analyzed, AEM and CLH met to ensure a consolidated list of themes by research question had been developed. The final list of themes as organized by research question and overall conceptualization of the data was presented to the research team for feedback. A conceptual model was then generated to display the process, pitfalls, and opportunities of differing methods of measuring activity performance in daily life within outpatient rehabilitation practice.
Results
Recruitment occurred from September 2023 through March 2024. Twenty-two occupational and physical therapist clinicians were contacted, 21 were screened for eligibility (1 did not respond to the request to complete eligibility questions), and 19 were consented and completed all study activities (1 did not complete the informed consent document and 1 declined to participate). Fifteen patients were referred from clinicians and 10 were screened, consented, and completed all study activities (5 did not respond after being contacted by the research team).
Clinician experience using any type of wearable motion sensor (e.g., Fitbit, Apple Watch) in their practice ranged from 0 to 6 + years. Six patients were currently using a wrist-worn sensor or mobile app to monitor their activity, two had previous experience using technology to monitor their activity but were not currently doing so, and two did not have any experience. Tables 2, 3 display descriptive statistics for the clinician and patient cohorts, respectively. Supplementary Table S1 includes the final list of themes associated with each research question.
Research question 1. How do clinicians perceive the constructs of activity capacity and activity performance and their relationship in outpatient rehabilitation?
Adjudication by a third reviewer (MDB) was required on three cases to determine whether clinicians understood the distinction between activity capacity and activity performance. This resulted in sixteen out of nineteen clinicians (84.2%) who accurately described the difference between activity capacity and activity performance during the interview. The remaining three clinicians (15.8%) did not accurately describe the distinction between these two constructs. In the first excerpt below, a clinician demonstrates understanding of activity capacity, by example of the 6-minute walk test, and activity performance, by example of a patient increasing their day-to-day activity. In the second excerpt below, the clinician's response indicates a lack of understanding of the difference between activity capacity and activity performance.
“I’ve also had people that, you know, they make a lot of progress on test measures like [the 6-minute walk test] as they’re working with me and they are also getting more active in their day-to-day, too.” – Clinician 4
“I think from an assessment standpoint that most of them assess function, vs. capacity. So really we assess them and give them some feedback on that assessment and say, okay, I want you to do this at home and we’re gonna reassess using those same assessments.” - Clinician
When sharing their perceptions of the relationship between activity capacity and activity performance in daily life, several clinicians voiced that in-clinic assessments of activity capacity provide only a “snapshot” of the patient and are not necessarily informative of the patient's activity performance in daily life.
“I think based on my clinical observations, we are only really getting a snippet of things when we’re taking our [in-clinic] measures…Yes, we may see some improvements on [in-clinic measures], but I don’t know that is always getting the full picture of [the patient] outside the clinic. That is where there's kind of a gap of like how do we monitor something like that. Sometimes subjectively we can get that as far as like they feel like they’ve done more, or they walk around longer in a store… but to actually have something quantifiable, there is I think a little bit of a gap there.” – Clinician 3
“Some patients, you know, their increase in activity level is definitely reflected in their 6-minute walk test and vice-versa, and other times it's not… Sometimes they might do really well in the clinic, because they’re motivated, they’re kind of tested on this measure and they know, this is gonna get sent to my doctor or my family member is gonna to read this note, and so…they do really well, but then I know at home they’re not very active from their report or their caregiver's report.” – Clinician 8
Although some clinicians could not accurately distinguish the constructs of activity capacity and activity performance, all clinicians acknowledged the limitations of brief, in-clinic assessments for understanding a patient's activity performance in daily life.
Research question 2: How do clinicians and patients perceive the value of activity performance monitoring in outpatient rehabilitation care?
When surveyed, twelve clinicians responded that it is “very important” to be able to monitor their patients' activity outside of clinic sessions, with six clinicians reporting this is “somewhat important”, and one clinician who was neutral on this issue (Figure 1, left panel). Patient perceptions were generally similar, with the majority (n = 6) of patients responding it is “very important” to measure how much they are moving in daily life and for their physical and/or occupational therapist to be able to measure this (n = 8; Figure 1, middle and right panels). Patients expanded on these concepts during interviews, in which several patients who were currently using a wearable sensor reported that having the device helps them stay accountable with their activity.
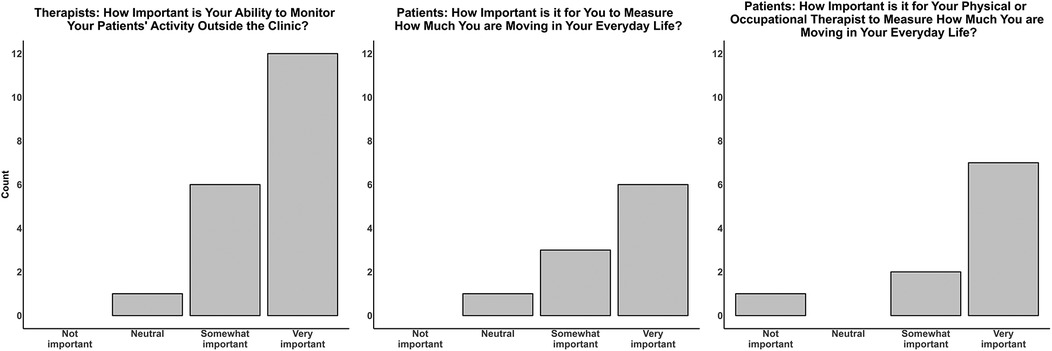
Figure 1. Perceived importance of activity performance monitoring by clinicians and patients. The left bar graph displays clinician ratings on the importance of measuring their patients’ activity outside the clinic. The middle bar graph displays patient ratings on the importance of measuring their own activity in daily life. The right bar graph displays patients’ perceived importance of their therapist clinician to be able to measure their activity in daily life.
“And if I’m getting lazy or tired and I put [activity] off, then I’ll look at [the sensor] and say ‘no, I got to get going’, and I will get going and do it.” – Patient 28
In addition to personal accountability, some patients reported that wearing a wearable sensor during rehabilitation care could also help them stay accountable to their physical and/or occupational therapist.
“I think it would be helpful, because if I didn’t do it, I know [my therapist] is gonna be watching.” – Patient 25.
Of the three clinicians who did not clearly distinguish between activity capacity and activity performance in RQ1, two rated the importance of being able to monitor their patients' activity outside the clinic as “somewhat important” and one rated this as “very important”. The other four clinicians who rated “somewhat important” and single clinician who rated “neutral” on the importance of being able to monitor their patients' activity outside of clinic visits were able to accurately distinguish between activity capacity and activity performance. Thus, there was not correspondence between the perceived importance of being able to monitor patients’ activity outside the clinic and ability to distinguish between the constructs of activity capacity and activity performance. Our sample size was small, however, and this may be useful to investigate in future work. Overall, the majority of clinicians and patients valued activity performance monitoring in outpatient rehabilitation care.
Research Question 3. What approaches, if any, are currently being used to measure activity performance in daily life in outpatient rehabilitation practice and what are important considerations regarding these approaches?
Clinicians described two main approaches to monitoring activity performance in outpatient rehabilitation care: (1) the use of self-report measures or activity logs, and (2) consumer-grade wearable sensors owned or acquired by the patient. Almost all clinicians mentioned using self-report measures or activity logs to measure their patients’ activity performance in daily life. Clinicians expressed several limitations with using self-report measures or activity logs including high patient burden and questionable reliability of information. Consequently, clinicians voiced that this approach often results in data loss and inaccurate or unusable data.
“If [a patient] is doing a walking program and it's more just self-report, okay, well, is that accurate? Are [they] just telling me what I want to hear?” – Clinician 1
Seventy-nine percent (15/19) of clinicians reported having some experience with using consumer-grade wearable sensors with patients who already owned or were willing to purchase a device, and 21% (4/19) reported no experience (Table 2). Clinicians expressed that this approach was often more desirable than measuring activity performance using self-report measures as it increased reliability of the information by having quantifiable data from a wearable sensor.
“I think [using a wearable sensor] helps with the confidence [of my clinical decisions] at least because it gives me more objective information of what they’re actually truly doing outside of the clinic…So that changes the education piece at least and having that data really helps because, like I said, sometimes it's not even that they’re not trying to be honest or forthcoming with information, they just don’t realize how little they’re [the patient] is actually moving.” – Clinician 4
Despite finding that most clinicians perceived activity performance monitoring as “somewhat” or “very important” and reported experience using consumer-grade sensors with their patients, only 40% of patients reported using a consumer-grade sensor in collaboration with their therapist clinician during outpatient rehabilitation care. This finding may be related to important themes for consideration raised by clinicians and patients for integrating wearable sensors into rehabilitation care (Tables 4, 5, respectively). These considerations could be viewed as a barrier or facilitator, depending on the context. For example, the cost for patients to purchase a wearable sensor was reported as a barrier if the patient did not have the financial means. It was reported as a facilitator, however, when the patient was able to purchase a device and because of the increasing number of consumer devices available at lower price points (29). Considerations voiced by both clinicians and patients included cost, comfort with technology, presence of patient impairments that may impact adherence to remote performance monitoring, and trust (or lack-there-of) in the accuracy of the sensor.
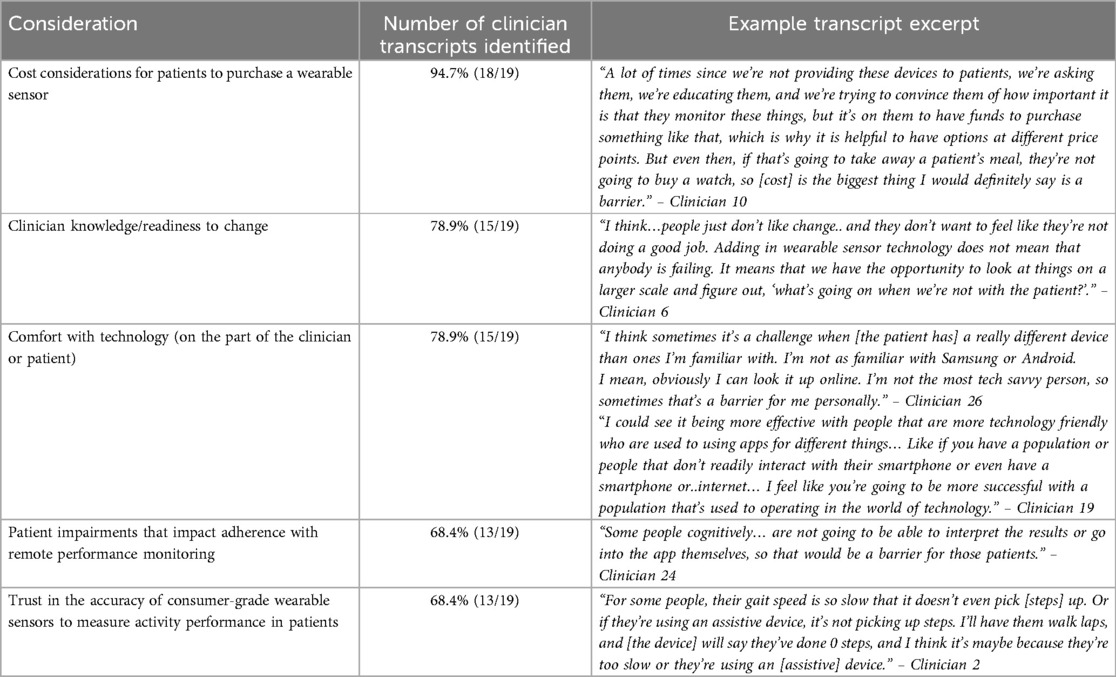
Table 4. Top five clinician-reported considerations to integrating wearable sensor technology into rehabilitation care.
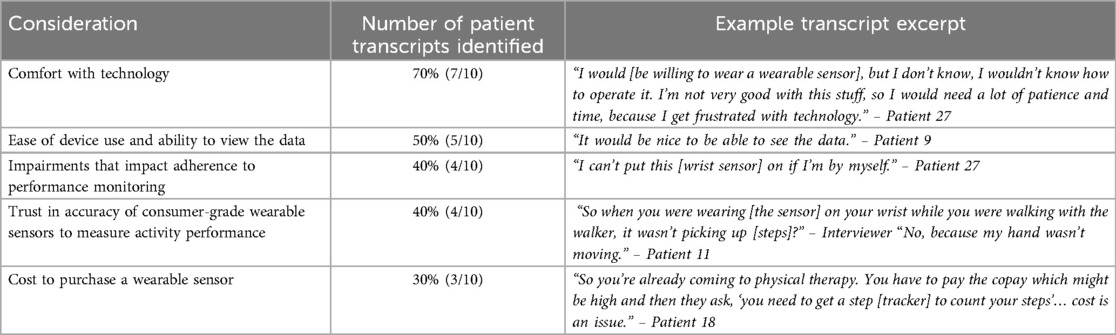
Table 5. Top five patient-reported considerations to integrating wearable sensor technology into rehabilitation care.
Despite these considerations, all clinicians interviewed recognized the potential for wearable sensor technology to bridge the gap between activity capacity and activity performance and improve outcomes for individuals seeking rehabilitation care.
“Measuring capacity and performance is one of the most important things in outpatient therapy, especially because a lot of times we see that the way that a patient is moving or walking or transferring in our clinic can be a lot different than how they’re moving outside of the clinic, especially in the amount that they’re moving outside the clinic… I think that's why we like to use external devices like step trackers and heart rate monitors…and all those things, because then it's not necessarily on the patient's report of how much they’re doing. We actually have concrete evidence and that makes a big difference, and it helps in terms of education, too… I think having ways to assess actual performance makes a big difference and success in outpatient therapy especially.” – Clinician 10
Research question 4. What are the data collection and workflow preferences of clinicians and patients for integrating wearable sensor technology into rehabilitation care?
On average, clinicians reported being willing to spend 16.25 ± 7.19 min to issue a wearable sensor to a patient, including initializing the sensor to start collecting data, assisting the patient with downloading an associated mobile app, and instructions for wearing and syncing (Figure 2 left panel). The majority (63%) of clinicians reported a preference for continuous monitoring in which they would issue a sensor to their patient within the first few therapy sessions and advise the patient to wear it until discharge from outpatient services (Figure 2, middle panel). All patients reported a willingness to wear a wearable sensor prescribed by their therapist clinician during outpatient rehabilitation care. As some research-based protocols used to measure upper limb activity performance require participants to wear two sensors, one on each wrist (30, 31), patients were also asked about their willingness to wear sensors on each wrist during outpatient rehabilitation care. All patients reported being willing to do so, with four reporting aesthetic concerns: “I’m not comfortable wearing devices on both wrists at a social event… Is it okay if I take them off and put them back on when I get home?” – Patient 12. Data syncing preferences generally fell into two categories: as often as their therapist clinician instructed (60%) or once per day at maximum (40%) (Figure 2, right panel).
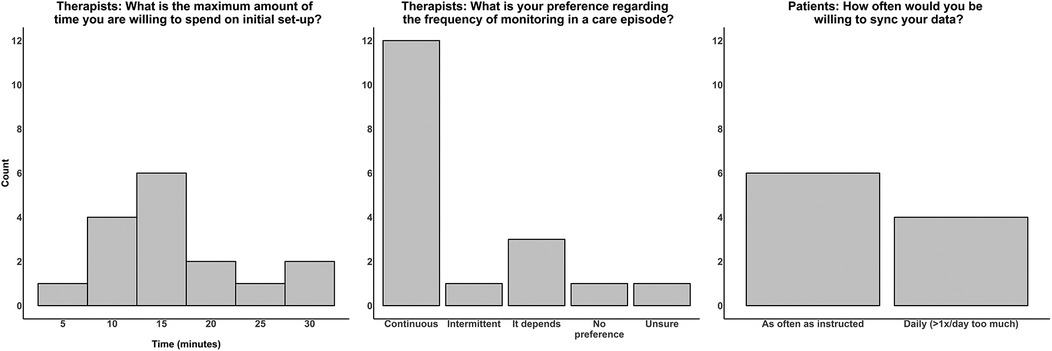
Figure 2. Data collection preferences of clinicians and patients. The left plot displays a histogram of the maximum number of minutes clinicians were willing to spend on initial set-up of the sensor (mean ± standard deviation: 16.25 ± 7.19 min). The middle bar graph displays clinician preferences for the frequency of performance monitoring within a therapy care episode, with continuous monitoring being the most common preference. The right bar graph displays patients’ willingness to sync their data via a mobile app, in which the majority responded they would be willing to do so as often as their therapist clinician instructed.
Interviews with clinicians concluded by discussing possible strategies to best integrate wearable sensor technology into their local clinical workflows. Clinicians identified alternative approaches to integrating wearable sensors into clinical practice which included a clinic inventory of sensors that clinicians could use for activity performance monitoring while the patient was being seen for outpatient rehabilitation services. Three clinicians mentioned that if the clinic were to purchase the same model of sensor, this may minimize the amount of time needed to manage the technology during clinic visits since the sensor would be familiar to clinicians.
“I’m pretty sure the only way to overcome a lot of these barriers would be to having clinics providing wearable sensors like this, because otherwise, it's kind of on the patient and they have all these barriers that I’ve talked about that I feel like limit them.” – Clinician 10
Another solution for integrating wearable sensor technology into clinical workflows included the integration of activity performance data into the electronic health record (EHR) for clinicians to easily access.
“I think that if this [sensor] information could be integrated into the medical record, it would change the game, because all of a sudden it makes it easier for a therapist who has really high expectations of productivity.” – Clinician 6
Challenges with these approaches from the clinician perspective included patients forgetting to sync their data which could result in the data not being available in the EHR, device loss resulting in increased financial burden on the clinic, and concerns about continued monitoring after the patient discharges from therapy services if the device is reclaimed by the clinic. Several suggestions were voiced during patient interviews to help alleviate these issues, including developing mechanisms to generate reminders to sync data, developing detailed user manuals with pictures, creating a help line to contact for technical support if needed, and creating a handout displaying options for consumer grade sensors at various price points for patients to consider purchasing for continued monitoring after discharge.
Conceptual model
The thematic analysis conducted in this study led to the development of a conceptual model that describes the workflow and process considerations for activity performance monitoring in outpatient rehabilitation care that takes into consideration themes generated from this analysis (Figure 3). This swim lane diagram highlights the process, pitfalls, and opportunities of the various approaches to activity performance monitoring as described by both patients and clinicians. Each row represents an approach discussed, and each column represents activities occurring in the clinic (dark grey) or in the patient's free-living environment (light grey). This model provides a visual representation of the data across research questions and provides broader implications for clinical practice and future research.
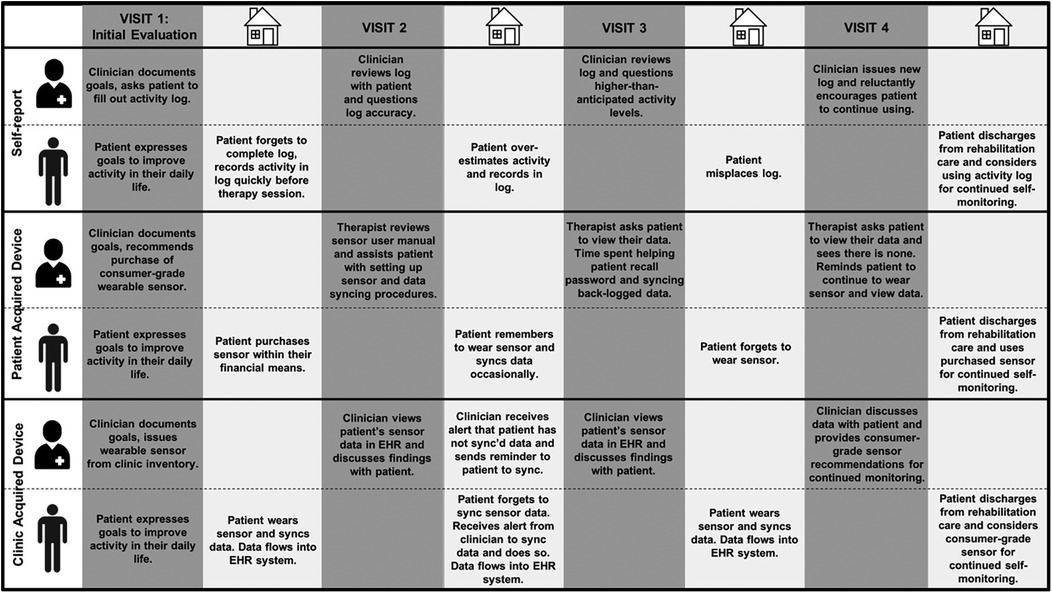
Figure 3. Swim lanes of clinicians and patients during Various approaches to activity performance monitoring in rehabilitation. Dark grey columns reflect actions occurring during clinic visits. Lighter grey columns reflect actions occurring outside the clinic while the patient is in their free-living environment. The figure depicts three approaches to activity performance monitoring in rehabilitation care discussed during interviews with patients and clinicians. The top third panel (“Self-report”) displays swim lanes and examples of reported challenges when self-report measures, such as activity logs, are used. The middle third panel (“Patient Acquired Device”) displays swim lanes and examples of reported challenges when consumer-grade devices acquired by the patient are used. The bottom third panel (“Clinic Acquired Device”) displays swim lanes and possible challenges that could occur with a clinic-acquired device model, in which the clinic invests in sensors that clinicians can use for activity performance monitoring in their patients. EHR, electronic health record.
Discussion
The purpose of this study was to investigate clinician and patient perspectives on integrating wearable sensor technology into rehabilitation care. Using a mixed-methods approach, we found that both clinicians and patients value activity performance monitoring and had preferences for how this monitoring could best be integrated into rehabilitation care. Discussions with clinicians and patients yielded a conceptual model of three different approaches for integrating sensors into rehabilitation care, each with its own barriers and facilitators.
In RQ1, we found that most, but not all, clinicians were able to articulate the distinction between the constructs of activity capacity and activity performance. Improvement in this area could be achieved through reinforcement of these constructs in physical and occupational therapy education programs, or in clinical settings through journal clubs or case presentations that emphasize the constructs of activity capacity and activity performance. Despite this, all clinicians expressed limitations with in-clinic assessments of activity capacity, including sentiments that they do not provide a full picture of the patient and are not necessarily reflective of an individual's activity performance in daily life. Collectively, these findings suggest that most clinicians are aware of the discrepancy between activity capacity and activity performance in outpatient rehabilitation practice. This was a critical question to investigate since the success of digital health interventions hinges upon a clearly defined problem, which requires stakeholders to both understand and acknowledge the problem (18). It is important to note, however, that clinicians did not state that in-clinic capacity-based assessments are not important. In-clinic measures of activity capacity are an essential component of rehabilitation practice (32, 33), predictive of therapy outcomes (34), and useful for quantifying responses to clinical interventions (35, 36). Thus, the take-away message from RQ1 is not that clinicians felt assessments of activity capacity are not valuable; rather, they perceived activity capacity assessments as a distinct construct from activity performance assessments, and that assessments of one construct do not necessarily inform or align with the other in outpatient clinical practice.
In RQ2, we found that most clinicians and patients value activity performance monitoring. As previous studies demonstrate the importance of stakeholder buy-in when implementing new practices (including digital tools) in health care settings, this is a desirable finding (18, 21). Since not all clinicians and patients felt this was important, however (Figure 1), additional education on the importance of activity performance monitoring will likely be warranted to encourage buy-in. Thus, clinics seeking to implement activity performance monitoring could measure how much clinicians and patients value performance monitoring and, if needed, provide additional education to facilitate their implementation efforts and uptake of this practice (10, 37).
RQ3 generated important considerations for measuring activity performance in outpatient rehabilitation care using two current approaches: self-report and consumer-grade sensors acquired by the patient. Clinicians described barriers with self-report measures that align with those reported in previous work, including recall bias and over- and under-reporting of activity performance compared to sensor-based assessment (12, 13). Offsetting these barriers is the low cost involved with this approach. Clinics seeking to implement a low-cost approach to activity performance monitoring could consider developing an activity log template and/or having activity calendars readily available for clinicians to issue to their patients to record their activity. This approach can also be helpful if a patient does not have the financial means to purchase a consumer-grade wearable sensor, the alternative approach to activity performance monitoring described by clinicians and patients. Using a consumer-grade sensor acquired by the patient, clinicians and patients appreciated receiving “concrete” data from the sensor but mutually agreed that cost, comfort with using technology, the capabilities of the patient, and accuracy of the sensor need to be considered before pursuing this approach. Clinics seeking to implement this approach could consider developing a handout of options of consumer grade sensors at various price points for patients to consider, as described by several clinicians. Gathering user manuals of common devices to have on hand for clinicians to refer to may alleviate concerns related to clinician familiarity with the wide variety of consumer sensors available. This wide variety in options has also resulted in varying levels of accuracy across sensors, an important consideration voiced by both clinicians and patients (38–44). There are various levels of validation to consider when determining whether a sensor is appropriate to use in a specific patient or clinical population, some of which are the responsibility of the manufacturer and some fall on the entity interested in using the sensor (e.g., clinical trial sponsor, researcher, clinician) (45). Clinicians seeking guidance here could consider comparing manual counts to the device output in the clinic to help estimate the accuracy (and overall appropriateness) of a particular device (46).
In RQ4, clinicians expressed preferences for continuous monitoring and to keep initial set-up time of the sensor to less than ∼16 min of therapy session time. Several clinicians justified their preference for continuous monitoring as “more is better” in terms of data generated from the sensor and obtaining a comprehensive picture of the patient's activity performance in daily life. This approach, however, will need to be weighed against higher participant burden to wear the sensor continuously, the potential of having to purchase a greater number of sensors in the clinic investment model (Figure 3, bottom panel), and larger quantities of data generated that need to be processed and presented in a digestible format to both clinicians and patients. How to best handle the enormous amounts of data generated is an important topic in remote monitoring research, as is understanding the delicate balance between providing clinicians with the appropriate amount of information needed and overwhelming them with streams of unnecessary data (47). Patient preferences included a willingness to wear one or two sensors, with some aesthetic concerns expressed with the two-sensor approach, and data syncing on a daily basis or as often as instructed by their therapist clinician. Adherence to wearing one or two sensors is generally high in the research setting (48–50); however, compliance with sensor wearing in routine clinical care outside the rigidity of research protocols remains understudied (51). Thus, future work should consider measuring adherence to wearing (and, if applicable, syncing) sensors in routine rehabilitation care and understanding patient characteristics associated with adherence, as this has been shown to be an important factor in the efficacy of remote monitoring protocols (17, 52). Elucidating patient characteristics associated with adherence will also inform which patients may need additional supports to participate in remote activity performance monitoring, an important consideration to using wearable sensors voiced by both clinicians and patients.
Discussions with clinicians and patients yielded a conceptual model highlighting three approaches to activity performance monitoring in rehabilitation care, each with barriers and facilitators (Figure 3). The key difference between these approaches can be distilled down to the entity that incurs the cost of a wearable sensor, which may change in the future as wearable sensor technology evolves and the ever-changing landscape of health care reimbursement shifts. Regardless of the approach taken, evidence suggests that new technologies or practices are most likely to be adopted when integrated within clinical workflows (18, 21, 53). In the context of remote monitoring, integrating data from the technology into the EHR maximizes the probability that the data will be seen and used (4, 18, 54). Indeed, several clinicians suggested sensor data integration with the EHR to help minimize the time required to access and view their patient's data. This might be easier to achieve with a consistent device model(s) to minimize challenges related to sensor interoperability and variability in how issues related to patient privacy are managed across sensor vendors. Regardless of how activity performance monitoring is implemented in rehabilitation care, efforts should be made to do so as most patients' goals are related to improving activity performance in daily life (1, 2). These findings add to this impetus by demonstrating that both patients and clinicians value activity performance monitoring and view wearable sensor technology as a solution to bridge the wide gap between the goals of patients (improving activity performance in daily life) and what is typically measured and intervened on in rehabilitation practice (activity capacity) (5).
Efforts to integrate digital health tools into clinical care settings should address a clearly defined problem (18). This study, therefore, focused on understanding stakeholder perspectives on a specific problem: the gap between activity capacity and activity performance in rehabilitation care. Despite this intentionally narrow focus, the findings from this study align with recommendations and stakeholder preferences identified in other areas of medicine. For example, the importance of integrating digital tools into clinical workflows has been recognized in the cardiovascular (55) and endocrinology fields (56). Across studies (15, 53, 54, 57, 58), including this one, EHR integration is often suggested as one approach to workflow integration and minimizing clinician burden. The expansion of digital health tools and EHR interoperability has created new possibilities for remotely collecting data from patients outside of traditional clinic visits (59). These new and exciting possibilities, however, also come with new challenges for health care organizations who must possess the infrastructure and capacity to handle large and more complex streams of data (59). This study generated key insights on multiple approaches to remote patient data collection, from less complex to more complex approaches (Figure 3), to inform remote data collection efforts of organizations with varying levels of resources and capabilities. Regardless of the approach, however, remote monitoring programs will not be useful if processes for data collection are too burdensome or costly for patients. These concerns were expressed by both clinicians and patients in our study and have also been emphasized in the cardiovascular (17), oncology (60), and endocrinology (61) realms. Thus, while use cases for digital technology differ across populations and clinical problems, commonalities exist across studies that must be taken into consideration as health care systems seek to embrace more complex digital ecosystems.
Limitations
There are several important limitations to consider when interpreting the results of this work. The clinicians interviewed were employed within an academic medical center and, in many cases, connected to researchers and well-versed in many aspects of rehabilitation research. Conducting this study outside of an academic medical center or at a clinic less familiar with research would have likely generated different findings, such as lower ratings on the perceived importance of activity performance monitoring and less time willing to spend on sensor set-up. Our findings therefore likely represent a more optimistic perspective on integrating wearable sensor technology into rehabilitation care. A related limitation is the small sample size, particularly with the patient cohort. While we reached saturation of information gleaned, the overall number of people queried is small and limits the ability to draw definitive conclusions. Thus, the perspectives of clinicians and patients in this study may or may not reflect how clinicians and patients feel about these issues in general. It is unknown how these results generalize to other settings. Efforts to integrate wearable sensor technology (and activity performance monitoring in any form) will likely be setting-specific and ideally built around clinician and patient preferences. Thus, findings from this study may not be informative for efforts to integrate wearable sensor technology at some clinics. Finally, we acknowledge that wearable sensors, and digital health technology as a whole, is an ever-evolving area. The pathway from device verification to clinical validation is a rigorous but necessary process to ensure data generated from these technologies are of high quality and will be useful in healthcare contexts (45). This study is not exhaustive of all the challenges that occur with integrating digital technologies, such as wearable sensors, into healthcare settings. Rather, this work proposes various approaches and their respective strengths and weaknesses to integrating wearable sensors into rehabilitation care.
Conclusions
Rehabilitation clinicians and patients value activity performance monitoring in rehabilitation care and view wearable sensor technology as a solution to bridge the wide gap between the goals of patients (improving activity performance in daily life) and what is typically measured and intervened on in rehabilitation practice (activity capacity). These findings serve as a launching point for future studies to investigate the implementation of wearable sensors into rehabilitation care, measure the usability of systems that integrate sensor data into EHR systems, and downstream effects on practice and outcomes. Critically, these initiatives will pave the way for future opportunities to harness the potential of digital technologies to improve the delivery and outcomes of rehabilitation care.
Data availability statement
The raw data supporting the conclusions of this article will be made available by the authors, without undue reservation.
Ethics statement
The studies involving humans were approved by Washington University Institutional Review Board. The studies were conducted in accordance with the local legislation and institutional requirements. The participants provided their written informed consent to participate in this study.
Author contributions
AM: Conceptualization, Data curation, Formal analysis, Funding acquisition, Investigation, Methodology, Project administration, Visualization, Writing – original draft, Writing – review & editing. CH: Conceptualization, Data curation, Formal analysis, Investigation, Methodology, Supervision, Writing – original draft, Writing – review & editing. MB: Formal analysis, Writing – review & editing. EF: Conceptualization, Methodology, Writing – review & editing. CN: Methodology, Writing – review & editing. TM: Conceptualization, Methodology, Writing – review & editing. CL: Conceptualization, Funding acquisition, Methodology, Supervision, Writing – review & editing.
Funding
The author(s) declare that financial support was received for the research and/or publication of this article. Foundation for Physical Therapy Research Digital Physical Therapy Grant (PI: Miller); Washington University Institute of Clinical and Translational Sciences (ICTS) Just-In-Time Core Usage Funding Program (mHRC- JIT996; PI: Lang); NIH R01/R37 HD068290 (PI: Lang); NIH T32 HD007434 (PI: Lang); NIH K08 MH120341 (PI: Fitzsimmons-Craft).
Acknowledgments
We are grateful to the clinicians and patients who participated in this study.
Conflict of interest
The authors declare that the research was conducted in the absence of any commercial or financial relationships that could be construed as a potential conflict of interest.
Generative AI statement
The author(s) declare that no Generative AI was used in the creation of this manuscript.
Publisher's note
All claims expressed in this article are solely those of the authors and do not necessarily represent those of their affiliated organizations, or those of the publisher, the editors and the reviewers. Any product that may be evaluated in this article, or claim that may be made by its manufacturer, is not guaranteed or endorsed by the publisher.
Supplementary material
The Supplementary Material for this article can be found online at: https://www.frontiersin.org/articles/10.3389/fdgth.2025.1534419/full#supplementary-material
References
1. Waddell KJ, Birkenmeier RL, Bland MD, Lang CE. An exploratory analysis of the self-reported goals of individuals with chronic upper-extremity paresis following stroke. Disabil Rehabil. (2016) 38(9):853–7. doi: 10.3109/09638288.2015.1062926
2. Bohannon RW, Andrews AW, Smith MB. Rehabilitation goals of patients with hemiplegia. Int J Rehabil Res. (1988) 11(2):181–4.
3. International Classification of Functioning, Disability, and Health: ICF. Version 1.0. Geneva: World Health Organization (2001). ©2001; 2001.
4. Lang CE, Barth J, Holleran CL, Konrad JD, Bland MD. Implementation of wearable sensing technology for movement: pushing forward into the routine physical rehabilitation care field. Sensors (Basel). (2020) 20(20):1–21. doi: 10.3390/s20205744
5. Lang CE, Holleran CL, Strube MJ, Ellis TD, Newman CA, Fahey M, et al. Improvement in the capacity for activity versus improvement in performance of activity in daily life during outpatient rehabilitation. J Neurol Phys Ther. (2022) 47:16–25. doi: 10.1097/NPT.0000000000000413
6. Zajac JA, Cavanaugh JT, Baker T, Duncan RP, Fulford D, Girnis J, et al. Does clinically measured walking capacity contribute to real-world walking performance in Parkinson’s disease? Parkinsonism Relat Disord. (2022) 105:123–7. doi: 10.1016/j.parkreldis.2022.11.016
7. Somerville E, Blenden G, Kretzer D, Holden B, Bollinger RM, Krauss MJ, et al. Differences in daily activity performance between inpatient rehabilitation facility and home among stroke survivors. Neurorehabil Neural Repair. (2024) 38(6):403–12. doi: 10.1177/15459683241246266
8. Thompson ED, Pohlig RT, McCrtney KM, Hornby TG, Kasner SE, Raser-Schramm J, et al. Increasing activity after stroke: a randomized controlled trial of high-intensity walking and step activity intervention. Stroke. (2024) 55(1):5–13. doi: 10.1161/STROKEAHA.123.044596
9. Bland MD, Holleran CL, Newman CA, Fahey M, Nordahl TJ, DeAngelis TR, et al. ICF Classification of therapeutic goals for outpatient episodes of neurorehabilitation in post-stroke and Parkinson disease. Disabil Rehabil. (2023) 46:1–7. doi: 10.1080/09638288.2023.2290201
10. Graham ID, Logan J, Harrison MB, Straus SE, Tetroe J, Caswell W, et al. Lost in knowledge translation: time for a map? J Contin Educ Health Prof. (2006) 26(1):13–24. doi: 10.1002/chp.47
11. Langan J, Subryan H, Nwogu I, Cavuoto L. Reported use of technology in stroke rehabilitation by physical and occupational therapists. Disabil Rehabil Assist Technol. (2018) 13(7):641–7. doi: 10.1080/17483107.2017.1362043
12. Waddell KJ, Lang CE. Comparison of self-report versus sensor-based methods for measuring the amount of upper limb activity outside the clinic. Arch Phys Med Rehabil. (2018) 99(9):1913–6. doi: 10.1016/j.apmr.2017.12.025
13. Resnick B, Michael K, Shaughnessy M, Nahm ES, Kobunek S, Sorkin J, et al. Inflated perceptions of physical activity after stroke: pairing self-report with physiologic measures. J Phys Act Health. (2008) 5(2):308–18. doi: 10.1123/jpah.5.2.308
14. Rand D, Eng JJ. Predicting daily use of the affected upper extremity 1 year after stroke. J Stroke Cerebrovasc Dis. (2015) 24(2):274–83. doi: 10.1016/j.jstrokecerebrovasdis.2014.07.039
15. Whitelaw S, Pellegrini DM, Mamas MA, Cowie M, Van Spall HGC. Barriers and facilitators of the uptake of digital health technology in cardiovascular care: a systematic scoping review. Eur Heart J Digit Health. (2021) 2(1):62–74. doi: 10.1093/ehjdh/ztab005
16. Iqbal FM, Aggarwal R, Joshi M, King D, Martin G, Khan S, et al. Barriers to and facilitators of key stakeholders influencing successful digital implementation of remote monitoring solutions: mixed methods analysis. JMIR Hum Factors. (2024) 11:e49769. doi: 10.2196/49769
17. Bhatia A, Maddox TM. Remote patient monitoring in heart failure: factors for clinical efficacy. Int J Heart Fail. (2021) 3(1):31–50. doi: 10.36628/ijhf.2020.0023
18. Smuck M, Odonkor CA, Wilt JK, Schmidt N, Swiernik MA. The emerging clinical role of wearables: factors for successful implementation in healthcare. NPJ Digit Med. (2021) 4(1):45. doi: 10.1038/s41746-021-00418-3
19. Demers M, Cain A, Bishop L, Gunby T, Rowe JB, Zondervan DK, et al. Understanding stroke survivors’ preferences regarding wearable sensor feedback on functional movement: a mixed-methods study. J Neuroeng Rehabil. (2023) 20(1):146. doi: 10.1186/s12984-023-01271-z
20. Stock R, Gaarden AP, Langørgen E. The potential of wearable technology to support stroke survivors’ motivation for home exercise - focus group discussions with stroke survivors and physiotherapists. Physiother Theory Pract. (2023) 40:1–12. doi: 10.1080/09593985.2023.2217987
21. Jarvis K, Thetford C, Turck E, Ogley K, Stockley RC. Understanding the barriers and facilitators of digital health technology (DHT) implementation in neurological rehabilitation: an integrative systematic review. Health Serv Insights. (2024) 17:11786329241229917. doi: 10.1177/11786329241229917
22. Demers M, Winstein CJ. A perspective on the use of ecological momentary assessment and intervention to promote stroke recovery and rehabilitation. Top Stroke Rehabil. (2021) 28(8):594–605. doi: 10.1080/10749357.2020.1856557
23. Liu L, Miguel Cruz A, Rios Rincon A, Buttar V, Ranson Q, Goertzen D. What factors determine therapists’ acceptance of new technologies for rehabilitation – a study using the unified theory of acceptance and use of technology (UTAUT). Disabil Rehabil. (2015) 37(5):447–55. doi: 10.3109/09638288.2014.923529
24. Harris PA, Taylor R, Minor BL, Elliott V, Fernandez M, O'Neal L, et al. The REDCap consortium: building an international community of software platform partners. J Biomed Inform. (2019) 95:103208. doi: 10.1016/j.jbi.2019.103208
25. Harris PA, Taylor R, Thielke R, Payne J, Gonzalez N, Conde JG. Research electronic data capture (REDCap)–a metadata-driven methodology and workflow process for providing translational research informatics support. J Biomed Inform. (2009) 42(2):377–81. doi: 10.1016/j.jbi.2008.08.010
26. Team RC. R: A Language and Environment for Statistical Computing. Vienna, Austria: R Foundation for Statistical Computing (2013).
27. Braun V, Clarke V. Thematic Analysis. APA Handbook of Research Methods in Psychology, Vol 2: Research Designs: Quantitative, Qualitative, Neuropsychological, and Biological. APA Handbooks in Psychology®. Washington, DC, US: American Psychological Association (2012). p. 57–71.
28. Braun V, Clarke V. Using thematic analysis in psychology. Qual Res Psychol. (2006) 3(2):77–101. doi: 10.1191/1478088706qp063oa
29. Muzny M, Henriksen A, Giordanengo A, Muzik J, Grøttland A, Blixgård H, et al. Dataset of wearable sensors with possibilities for data exchange. Data Brief. (2020) 28:104978. doi: 10.1016/j.dib.2019.104978
30. Lang CE, Waddell KJ, Klaesner JW, Bland MD. A method for quantifying upper limb performance in daily life using accelerometers. J Vis Exp. (2017) 122:1–8. doi: 10.3791/55673
31. Bailey RR, Klaesner JW, Lang CE. Quantifying real-world upper-limb activity in nondisabled adults and adults with chronic stroke. Neurorehabil Neural Repair. (2015) 29(10):969–78. doi: 10.1177/1545968315583720
32. Bland MD, Moore JL, Anderl E, Eikenberry M, McCarthy A, Olivier GN, et al. Knowledge translation task force for core measures clinical practice guideline: a short report on the process and utilization. Implement Sci Commun. (2024) 5(1):43. doi: 10.1186/s43058-024-00580-1
33. Moore JL, Potter K, Blankshain K, Kaplan SL, O'Dwyer LC, Sullivan JE. A core set of outcome measures for adults with neurologic conditions undergoing rehabilitation: A CLINICAL PRACTICE GUIDELINE. J Neurol Phys Ther. (2018) 42(3):174–220. doi: 10.1097/npt.0000000000000229
34. Lohse K, Bland MD, Lang CE. Quantifying change during outpatient stroke rehabilitation: a retrospective regression analysis. Arch Phys Med Rehabil. (2016) 97(9):1423–30 e1. doi: 10.1016/j.apmr.2016.03.021
35. Boyne P, Miller A, Kubalak O, Mink C, Reisman DS, Fulk G. Moderate to vigorous intensity locomotor training after stroke: a systematic review and meta-analysis of mean effects and response variability. J Neurol Phys Ther. (2023) 48:15–26. doi: 10.1097/npt.0000000000000456
36. Lang CE, Strube MJ, Bland MD, Waddell KJ, Cherry-Allen KM, Nudo RJ, et al. Dose response of task-specific upper limb training in people at least 6 months poststroke: a phase II, single-blind, randomized, controlled trial. Ann Neurol. (2016) 80(3):342–54. doi: 10.1002/ana.24734
37. Goodrich DE, Miake-Lye I, Braganza MZ, Wawrin N, Kilbourne AM. The QUERI Roadmap for Implementation and Quality Improvement. Washington (DC): Department of Veterans Affairs (US) (2020).
38. Fulk GD, Combs SA, Danks KA, Nirider CD, Raja B, Reisman DS. Accuracy of 2 activity monitors in detecting steps in people with stroke and traumatic brain injury. Phys Ther. (2014) 94(2):222–9. doi: 10.2522/ptj.20120525
39. Hui J, Heyden R, Bao T, Accettone N, McBay C, Richardson J, et al. Validity of the fitbit one for measuring activity in community-dwelling stroke survivors. Physiother Can. (2018) 70(1):81–9. doi: 10.3138/ptc.2016-40.ep
40. Klassen TD, Semrau JA, Dukelow SP, Bayley MT, Hill MD, Eng JJ. Consumer-Based physical activity monitor as a practical way to measure walking intensity during inpatient stroke rehabilitation. Stroke. (2017) 48(9):2614–7. doi: 10.1161/strokeaha.117.018175
41. Klassen TD, Simpson LA, Lim SB, Louie DR, Parappilly B, Sakakibara BM, et al. “Stepping up” activity poststroke: ankle-positioned accelerometer can accurately record steps during slow walking. Phys Ther. (2016) 96(3):355–60. doi: 10.2522/ptj.20140611
42. Henderson CE, Toth L, Kaplan A, Hornby TG. Step monitor accuracy during PostStroke physical therapy and simulated activities. Transl J Am Coll Sports Med. (2022) 7(1):1–9. doi: 10.1249/tjx.0000000000000186
43. Duclos NC, Aguiar LT, Aissaoui R, Faria C, Nadeau S, Duclos C. Activity monitor placed at the nonparetic ankle is accurate in measuring step counts during community walking in poststroke individuals: a validation study. PM R. (2019) 11(9):963–71. doi: 10.1002/pmrj.12080
44. Uswatte G, Miltner WH, Foo B, Varma M, Moran S, Taub E. Objective measurement of functional upper-extremity movement using accelerometer recordings transformed with a threshold filter. Stroke. (2000) 31(3):662–7. doi: 10.1161/01.str.31.3.662
45. Goldsack JC, Coravos A, Bakker JP, Bent B, Dowling AV, Fitzer-Attas C, et al. Verification, analytical validation, and clinical validation (V3): the foundation of determining fit-for-purpose for biometric monitoring technologies (BioMeTs). NPJ Digit Med. (2020) 3(55):1–15. doi: 10.1038/s41746-020-0260-4
46. Holleran CL, Bland MD, Lang CE. Comprehensive assessment of the activity level of the ICF using both capacity and performance measures: a case report. Arch Rehabil Res Clin Trans. (2023) 5(3):100277. doi: 10.1016/j.arrct.2023.100277
47. Claggett J, Petter S, Joshi A, Ponzio T, Kirkendall E. An infrastructure framework for remote patient monitoring interventions and research. J Med Internet Res. (2024) 26:e51234. doi: 10.2196/51234
48. Bailey RR, Klaesner JW, Lang CE. An accelerometry-based methodology for assessment of real-world bilateral upper extremity activity. PLoS One. (2014) 9(7):e103135. doi: 10.1371/journal.pone.0103135
49. Bailey RR, Lang CE. Upper-limb activity in adults: referent values using accelerometry. J Rehabil Res Dev. (2013) 50(9):1213–22. doi: 10.1682/jrrd.2012.12.0222
50. Waddell KJ, Strube MJ, Bailey RR, Klaesner JW, Birkenmeier RL, Dromerick AW, et al. Does task-specific training improve upper limb performance in daily life poststroke? Neurorehabil Neural Repair. (2017) 31(3):290–300. doi: 10.1177/1545968316680493
51. French MA, Keatley E, Li J, Balasubramanian A, Hansel NN, Wise R, et al. The feasibility of remotely monitoring physical, cognitive, and psychosocial function in individuals with stroke or chronic obstructive pulmonary disease. Digit Health. (2023) 9:20552076231176160. doi: 10.1177/20552076231176160
52. Pannunzio V, Morales Ornelas HC, Gurung P, van Kooten R, Snelders D, van Os H, et al. Patient and staff experience of remote patient monitoring—what to measure and how: systematic review. J Med Internet Res. (2024) 26:e48463. doi: 10.2196/48463
53. Lobelo F, Kelli HM, Tejedor SC, Pratt M, McConnell MV, Martin SS, et al. The wild wild west: a framework to integrate mHealth software applications and wearables to support physical activity assessment, counseling and interventions for cardiovascular disease risk reduction. Prog Cardiovasc Dis. (2016) 58(6):584–94. doi: 10.1016/j.pcad.2016.02.007
54. Tiase VL, Hull W, McFarland MM, Sward KA, Del Fiol G, Staes C, et al. Patient-generated health data and electronic health record integration: a scoping review. JAMIA Open. (2020) 3(4):619–27. doi: 10.1093/jamiaopen/ooaa052
55. Alaboud K, Shahreen M, Islam H, Paul T, Rana MKZ, Morrison A, et al. Clinicians’ perspectives in using patient-generated health data to improve ischemic heart disease management. AMIA Jt Summits Transl Sci Proc. (2022) 2022:112–9.35854732
56. Hall T, Warman MK, Oser T, Filippi MK, Manning B, Carroll JK, et al. Clinician-Reported barriers and needs for implementation of continuous glucose monitoring. J Am Board Fam Med. (2024) 37(4):671–9. doi: 10.3122/jabfm.2024.240049R1
57. Espinoza JC, Yeung AM, Huang J, Seley JJ, Longo R, Klonoff DC. The need for data standards and implementation policies to integrate insulin delivery data into the electronic health record. J Diabetes Sci Technol. (2023) 17(5):1376–86. doi: 10.1177/19322968231178549
58. Stetson PD, McCleary NJ, Osterman T, Ramchandran K, Tevaarwerk A, Wong T, et al. Adoption of patient-generated health data in oncology: a report from the NCCN EHR oncology advisory group. J Natl Compr Canc Netw. (2022) 20:1–6. doi: 10.6004/jnccn.2021.7088
59. Mann DM, Lawrence K. Reimagining connected care in the era of digital medicine. JMIR Mhealth Uhealth. (2022) 10(4):e34483. doi: 10.2196/34483
60. Graetz I, Hu X, Kocak M, Krukowski RA, Anderson JN, Waters TM, et al. Remote monitoring app for endocrine therapy adherence among patients with early-stage breast cancer: a randomized clinical trial. JAMA Netw Open. (2024) 7(6):e2417873. doi: 10.1001/jamanetworkopen.2024.17873
61. Nemlekar PM, Hannah KL, Green CR, Norman GJ. Association between adherence, A1C improvement, and type of continuous glucose monitoring system in people with type 1 diabetes or type 2 diabetes treated with intensive insulin therapy. Diabetes Ther. (2024) 15(3):639–48. doi: 10.1007/s13300-023-01529-8
Keywords: wearable sensor, technology, digital health, rehabilitation, physical therapy, occupational therapy, remote monitoring, mixed-methods
Citation: Miller AE, Holleran CL, Bland MD, Fitzsimmons-Craft EE, Newman CA, Maddox TM and Lang CE (2025) Perspectives of key stakeholders on integrating wearable sensor technology into rehabilitation care: a mixed-methods analysis. Front. Digit. Health 7:1534419. doi: 10.3389/fdgth.2025.1534419
Received: 25 November 2024; Accepted: 9 April 2025;
Published: 28 April 2025.
Edited by:
Steven L. Wolf, Emory University, United StatesReviewed by:
Kristen Fessele, Memorial Sloan Kettering Cancer Center, United StatesRichard Giordano, University of Southampton, United Kingdom
Copyright: © 2025 Miller, Holleran, Bland, Fitzsimmons-Craft, Newman, Maddox and Lang. This is an open-access article distributed under the terms of the Creative Commons Attribution License (CC BY). The use, distribution or reproduction in other forums is permitted, provided the original author(s) and the copyright owner(s) are credited and that the original publication in this journal is cited, in accordance with accepted academic practice. No use, distribution or reproduction is permitted which does not comply with these terms.
*Correspondence: Allison E. Miller, bWlsbGVyLmFsbGlzb25Ad3VzdGwuZWR1