- 1Research Group PREMEDOC INV-GIUV-270642, Departament de Prehistòria, Arqueologia i Història Antiga, Universitat de València, Valencia, Spain
- 2Institut Català de Paleoecologia Humana i Evolució Social, Campus Sescelades, Tarragona, Spain
- 3Universitat Rovira y Virgili (URV), Área de Prehistoria, Tarragona, Spain
- 4Departament de Prehistòria, Arqueologia i Història Antiga, Universitat de València, Valencia, Spain
Recent approaches have described the evolutionary dynamics of the first Neolithic societies as a cycle of rise and fall. Several authors, using mainly c14 dates as a demographic proxy, identified a general pattern of a boom in population coincident with the arrival of food production economies followed by a rapid decline some centuries afterward in multiple European regions. Concerning Iberia, we also noted that this phenomenon correlates with an initial development of archeological entities (i.e., “cultures”) over large areas (e.g., the Impresso-Cardial in West Mediterranean), followed by a phase of “cultural fragmentation” by the end of Early Neolithic. This results in a picture of higher cultural diversity as an effect of more limited spread of cultural artifacts. In this work, we propose to apply a network approach to the analysis of material culture. In particular, we consider the spatiotemporal patterns of material culture as an emergent effect of local interaction processes. As recent research has pointed out, the spatiotemporal variability of material culture is an emergent phenomenon resulting from individual and group interactions whose structure resembles those of spatially structured complex networks. Our results suggest that the observed global patterns could be explained by the network dynamics, especially by structural (measured as the betweenness centrality) and geographical position of some nodes. The appearance and disappearance of nodes in specific positions correlate with the observed changes in the pattern of material culture distribution throughout the Early Neolithic (c. 7700–6700 cal BP) in East Iberia. In our view, this could be explained by the special role played by those nodes facilitating or limiting the information flow over the entire network. Network growth and posterior fragmentation seem to be the key drivers behind these dynamics.
Introduction
While the origins of the first agricultural societies have long received considerable attention, this is not the case of the subsequent course of events. Since the work by Bocquet-Appel (2008) noted the existence of a Neolithic Demographic Transition, different approaches have described the evolutionary dynamics of the first Neolithic societies as a cycle of rise and fall. Several authors, using mainly radiocarbon dates as a demographic proxy, identified a general pattern of a boom in population coincident with the arrival of food production economies followed by a rapid decline some centuries afterward in multiple European regions (Shennan et al., 2013), including Iberian peninsula (Bernabeu et al., 2014; Balsera et al., 2015).
In Iberia, the arrival of the earliest Neolithic groups cannot be rolled back beyond c. 7700/5700 cal BP/BC (Bernabeu and Martí Oliver, 2014; Martins et al., 2015). During the second half of the eighth millennium, there were considerable transformations. In recent research focused on South-eastern Iberia (Bernabeu et al., 2013), we identified co-occurring changes in social organization, demography, and economy that followed the initial establishment of agriculture. The development of social inequalities, and shifts in settlement and population took place during this period, in a context of a sharp increase in the scale and complexity of socio-spatial networks. Soon after, by the beginning of seventh millennium BP, this reorganization of human society stopped.
These dynamics of the first agricultural societies in Iberia seem to fit with the “boom and bust” pattern described by c14 curves (see above). Interestingly, we noted (Bernabeu et al., 2014, 2016) that this phenomenon correlates with an initial development of archeological entities (i.e., “cultures”) over large areas (e.g., LBK in central Europe and Impresso-Cardial in West Mediterranean countries), followed by a phase of “cultural fragmentation” by the end of Early Neolithic (c. 7000–6800 cal BP). Archeologically, this process results in a picture of more regional “cultures” covering the same regions as an effect of more limited spread of “cultural items/artifacts.” This is especially evident in the geographical distribution of ceramic styles (Bernabeu et al., 2011 for a recent “state of the art” about pottery productions of Early Neolithic in Iberia).
Internal (Shennan et al., 2013; Bernabeu et al., 2014) and externals drivers (Gronenborn, 2009, 2010) have been proposed to explain this phenomenon. Whatever the reason, both drivers may well have had consequences for human societies, but they are regionally and locally variable. This variability is due to differences reflecting the importance of biotic factors and human economic behavior. Since both factors—ecology and human behavior—are historically contingent, it would be surprising to find the same effects everywhere (Bernabeu et al., 2016). But interpretations of these phenomena usually involve qualitative extrapolation to geographically extensive areas of information derived from one or very few localized data sets and focusing on a limited set of artifact variability (usually some key ceramic decorations). Such an approach makes their evaluation difficult, thus leaving their widespread applicability in question.
Assuming this, it seems clear that we do not only need new, high-quality proxy records both of human and/or environmental conditions, but we also need a way to understand how different local dynamics could result in the emergence of those global patterns of change that we are trying to explain [see the “micro-macro link” concept in Coleman (1994)]. One way of overcoming this situation is to incorporate information from wider regions and analyze them from a systemic perspective. Network theory and method could help us in this task. Identifying geographical patterns of interactions among ancient groups is a key feature for understanding patterns of network structure. In combination with time, we can use this information as a proxy of network dynamics through c. 7700–6700 cal BP/5700–4700 cal BC in Eastern Iberia.
From an evolutionary perspective, the extent to which human culture varies over space and time is determined by a complex interplay between patterns of inheritance, interaction, and adaptation (Mace and Jordan, 2011; Crema et al., 2014; Shennan et al., 2015); distinguishing how these three types of processes generate observable patterns of cultural variation over time and space is one of the primary research questions in archeology and anthropology (Shennan et al., 2015: p. 103–104). In this work, we will focus on “interaction,” the process through which a social network is built.
Our goal is to address this particularly challenging scenario through the application of network analysis techniques. Specifically, we aim to (a) characterize the formation of archeological entities (i.e., cultures) that extended over large areas, and their subsequent fragmentation during the Neolithic transition; and (b) evaluate whether these macroscopic phenomena could be explained from local/regional network size and dynamics of site networks, as the macro effect (in terms of network connectivity) of micro changes such as the disappearance of a node or group of nodes (focusing specially on central ones, which are responsible of the transmission of information to the entire network).
To our knowledge, our study is the first approach to apply network analysis to such early stages of European prehistory [see Coward (2010) and Mills et al. (2013) for applications in other contexts]. In so doing, we hope to gain new insights into the recursive interactions between information exchange, socioeconomic transformation, and demographic change. Moreover, the chosen scenario stresses aspects such as the appropriateness of the sort of data used to characterize social networks and information flow (i.e., pottery decoration), and the representativeness of archeological record.
Materials and Methods
Interactions among individuals and groups in human societies can be represented as networks, in which the nodes are social agents and the connections between the nodes (edges or links in network terminology) represent their interactions (Wasserman and Faust, 1994). This allows for the application of a wide range of mathematical tools, to understand their evolutionary dynamics. During the last decade, the analysis of complex networks has raised significant interest, especially since its ubiquity across scientific fields was uncovered (Newman, 2010).
The impact of these theoretical and methodological tools on issues concerning exchange and social interaction is evident, and their utility has been perceived by archeologists (Knappett, 2013; Mills et al., 2013; Collar et al., 2015). However, the application of network analysis’ tools to archeological data requires addressing a number of issues concerning, for instance, objective clarification and the appropriateness of the available data (Brughmans, 2013). In this section, we first introduce the kind of relational data we use, how we collected, and organized these data. Then, we focus on issues about network construction and analysis.
Relational Data
It is clear that “culture” is a complex concept that includes many different factors. Limiting our focus to those found to be more evident in Neolithic contexts, we can use either lithic or pottery as archeological proxies of the “Neolithic culture.” For our preliminary purposes, we are using pottery; and because pottery decoration is one of the classic markers of Early Neolithic, we decided to use “decoration” as an archeological proxy to trace social relationships. Two different decorative aspects may be equally valid for the purposes addressed here: we can use a motif-based approach or a technique-based approach. We decided to use the latter to avoid the problem of fragmentation of ceramics that seriously handicaps the correct reading of the motif and affect the final size of the available sample.
Using methodological tools developed in previous works (Manen et al., 2010; Bernabeu et al., 2011, 2017), we first defined the techniques and then use the combinations of those to determine the “technological styles” represented in the Early Neolithic. As a result, we obtained a total of 42 substyles, based on the use of only one technique or on a combination of two or more techniques (Figure 1, examples).
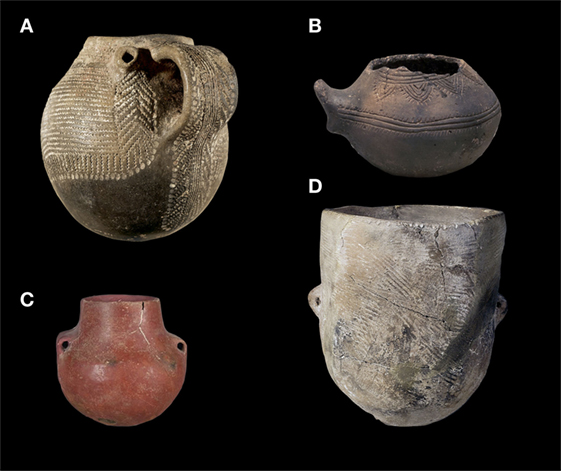
Figure 1. Examples of technological styles used in this work. (A) Cardial (from Cova de l’Or, Alicante); (B) Epicardial (from La Lámpara, Soria); (C) Almagra (from Sima del Lentisco; Cádiz); (D) Peinada (from Cova de les Cendres, Alicante).
Then, we recorded the presence/absence of these styles in all the Iberian sites and layers whose radiocarbon dates fell in the temporal period of interest here. The list was compiled through the direct review of materials and collections, as well as literature review when direct access was not possible. At first step, we only used those layers or features properly dated.
Bayesian Approach
In order to expand the available sample also included some other sites not dated, but that from which is chronologically possible to obtain reliable information based on Bayesian statistics following recent works (Ortman et al., 2007; Fernández-López de Pablo and Barton, 2015). This statistical analysis was limited to sites located South to the Ebro river, because only in this area, we have reliable archeological sequences about which to build a chronological strong model.
A chronological model was produced using Oxcal, version 4.2.3 (Bronk Ramsey, 2009), based on the main archeological sites with a good chronological stratigraphy: Cova de les Cendres and Cova de l’Or among others. After a series of previous analyses, our chronological model incorporated 33 radiocarbon dates (Figure 2) organized in 11 phases, and its statistical likelihood, the so-called Amodel, is 134. Of these, only the first 8 corresponded to the Early Neolithic and were of interest to this work.
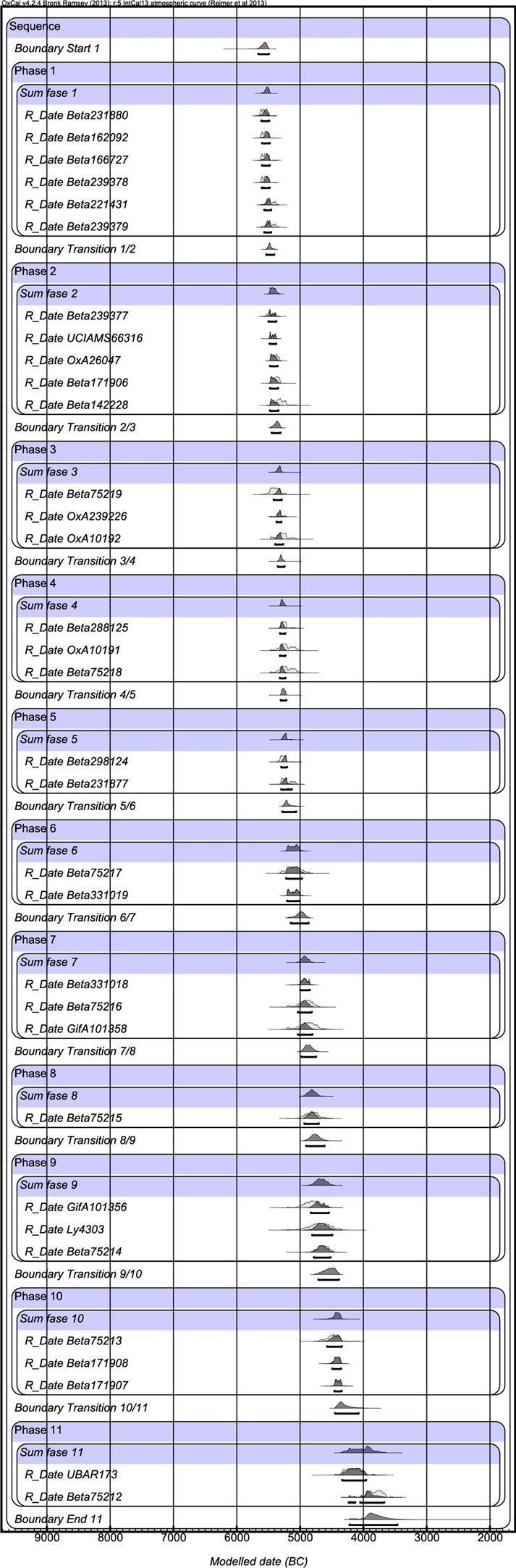
Figure 2. Bayesian model. Phase modeling results showing the probability distribution of radiocarbon dates for area based on continuous hypothesis.
Following Fernández-López de Pablo and Barton (2015), the radiocarbon chronology was converted to a prior probability following several steps. First, we need to calculate the frequency of each pottery style relative to the total styles recorded. Then, we calculate the mean of the relative frequency of each pottery style for each of temporal phase where it is present. After that, we have a prior probability of the pottery styles. Next, posterior probabilities for each chronological phase were computed on the basis of style counts for each site/level without radiocarbon dates following the equations applied by Fernández-López de Pablo and Barton (2015): Eqs 2–4. All these operations were computed using R (Core Team, 2013).
After applying this Bayesian approach, the database accounted for a total of 86 sites and 128 layers/structures/levels located in the Centre-East of Iberia to use in our analysis (Figure 3; Supplementary Material S1).
Managing Time: Defining our Temporal Windows
Once we compiled the database, our next challenge was to split the archeological information in a way allowing us to observe the evolutionary dynamics of networks throughout the Early Neolithic. Taking into account the characteristics of the Iberian empirical record, as well as the uncertainly of radiocarbon dating, we defined time windows of 100 years. Establishing more temporal divisions would imply fragmenting the already small sample into very limited pottery assemblages that would be of no use for our analysis.
Next, in order to assign each archeological site or layer to one or more of the above-defined 100 years’ temporal windows, we applied the following procedure: First, we took as a reference the 2 sigma probability interval for each calibrated date (95%). This was done independently of the technique used to define such a date (i.e., either radiocarbon dating or Bayesian inference). On the other hand, we assigned any site/layer to a specific temporal window if, at least, 25% of the chronological interval fell in that temporal window. Figure 4 exemplifies the method to assign the temporal range for each radiometric date. After calibration, the date Revilla 5 has a span from 7429 to 7292 cal BP. That is, the date covers 29 years (29%) of the period 7500–7400, but only 8% of the period 7300–7200. Consequently, we placed this specific feature of the Revilla in the first temporal window, but not in the second one.
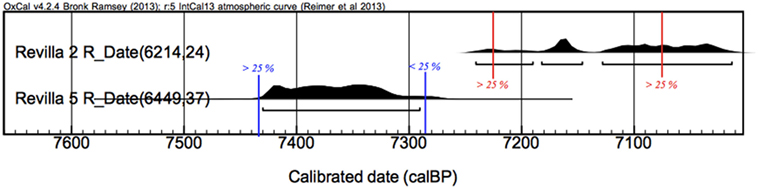
Figure 4. Method to assign each calibrated date to one or more specific times windows. In this case, Revilla 2, after calibration, covers the time windows 7300–7200, 7200–7100, and 7100–7000. However, Revilla 5 after its calibrations covers only the temporal windows 7500–7400 and 7400–7300 (see text for more details).
In short, the application of this protocol has allowed us to populate 10 time windows between 7700 and 6700 cal BP with sites. Table 1 shows the number of sites (nodes) and ceramic styles considered for each of the windows. Finally, it should be noted that the first time window defined (7700–7600) is not taken into account in this work since the number of documented sites is very small and the size of the sample can significantly affect the results. In other words, we are using in this work nine time windows (7600–6700).
Network Construction
The previous step provided us with the set of nodes to be included at each temporal window. Then, we defined the links among such nodes (i.e., we created the actual networks). As already explained above, we used similarity in ceramic decoration techniques as the main connectivity criterion. More specifically, in our networks, two nodes were connected if their cultural profiles (in terms of absence/presence of decoration styles) were similar enough. This approach raises two obvious issues: (a) How to quantify similarity among cultural profiles. (b) How to define a threshold of enough similarity.
The literature on network analysis applied to archeology offers several possible ways to address these two issues, ranging from the simple coincidence to the usage of different similarity indices. The need for standard methods or criteria to select the option fitting best to each particular case study has been discussed only recently (Liu et al., 2016).
Taking into account the nature of our data (i.e., absence/presence of styles), we decided to use the Jaccard similarity index. Notice that more common similarity indices in archeology (the most usual being the Brainerd–Robinson coefficient) are especially suitable for data based on counts. Alternative (and, maybe, more intuitive) similarity measures would include simple or double coincidence (i.e., so a link between two nodes would be established whenever they shared, at least one or two styles). However, such options do not take into account the total number of styles present at each node. Since nodes in our case showed very different values of style diversity, we discarded using absolute coincidences as similarity criterion.
Moreover, we noted that the total number of decoration styles recorded changed strongly across temporal windows (i.e., ranging from 18 to 37). This heterogeneity could introduce biases when comparing structural characteristics of networks across temporal windows. Specifically, lower style variability in certain temporal windows could facilitate high similarity values. On the contrary, a broader variability of styles would (a priori) difficult high similarity scores.
In order to prevent such biases, we introduced a compensation mechanism by normalizing the value of the Jacquard index according to the following formula (Eq. 1):
where Nsubstyles corresponds to the total number of substyles present in that particular temporal window and Max(Nsubstyles) is the maximum across temporal windows.
Finally, once defined our similarity criterion, we addressed issue (b) above (i.e., determining how much similarity was enough to establish a link between two nodes). We performed several preliminary experiments by constructing networks with different threshold values (within the [0,1] range) and comparing their structural characteristics. We found 0.25 to be the maximum value guaranteeing that all nodes where connected in all cases. In other words, for threshold values above 0.25, the link density of networks tended to drop drastically, and some presented several isolated nodes (see an example in Figure 5).
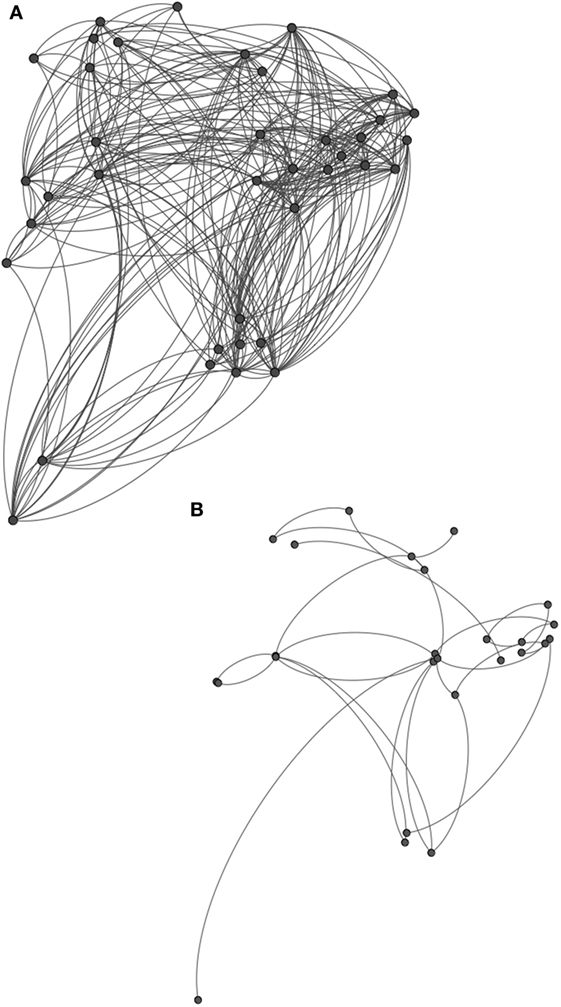
Figure 5. Graph of the resulting networks in the same time window (7300–7200), but applying different Jaccard values to establish a link: (A) Jaccard = 0.25 (N = 38; M = 298); (B) Jaccard = 0.5 (N = 26; M = 30). After performing several experiments, we decide to use the Jaccard = 0.25 because higher values clearly reduce the archeological sample; and to avoid the effect spurious relationship (chance, very common traditions) using lower Jaccard values.
Node Turnover Quantification
As part of our analysis of the obtained networks, we compared the evolution of several structural measures with changes in system size (i.e., number of nodes). In order to better understand the processes underlying such changes, instead of taking just the net variation of the number of nodes, we looked for a methodology to quantify node replacement (i.e., measuring the amount of appearing and disappearing nodes from one network to the next one in chronological order). For example, a reduction of N/2 nodes from one temporal window to the following can be the result of the disappearance of half of the nodes in the network (while keeping the other half), or the complete replacement of a network of N nodes by a new one of size N/2. Identifying whether the system experimented a size reduction (i.e., former case) or a complete replacement (i.e., later scenario) is highly useful to characterize the evolution of the system under study.
After unsuccessfully searching across the literature on network analysis, we decided to propose our own node turnover ratio (NTR) indicator (Eq. 2):
where Nap is the amount of nodes appearing in the network under study (i.e., that show up but were not present in the previous network), Ndis corresponds to the count of “disappearing nodes” (i.e., that were present in the previous network, but not in the current one), and Ntot stands for the total number of nodes in the current network.
The indicator is constructed in such a way that positive (negative) values correspond to net increasing (decreasing) of the number of nodes. Moreover, since it is normalized by Ntot, its range of values is bounded between +1 (i.e., the network is composed exclusively by “new” nodes) and −1 (i.e., there is no network at all, since all nodes have disappeared).
Reproducibility and Open-Source Materials
To enable re-use of our materials and improve reproducibility and transparency, we include the entire oxcal and R code used for all the analysis and visualizations contained in this paper in our SOM at https://doi.org/10.5281/zenodo.321697. All of the figures, tables, and statistical tests can be reproduced with the code and data of this repository. Information is licensed under MIT.
Results
In this section, we use different scales to describe the structure and dynamics of the networks across the nine temporal windows (7600–6700). First, we built normalized and non-normalized networks for each temporal window, as described in Section “Managing Time: Defining our Temporal Windows.” Then, we calculated several structural metrics for each one of such networks (see Table 2). In this study, we use the metrics obtained from normalized networks.
Among the analyzed structural measures, we decided to focus our study on link density and clustering coefficient. The main reason for such a choice is that these measures (especially clustering) are commonly related in Network Science with social cohesion and stability (Wasserman and Faust, 1994; Newman, 2010), which are social features of high interest for our work. Finally, we changed the scale of our analysis, focusing our interest in the node behavior. Using the betweenness centrality, we looked for a better understanding of network-level dynamics from the appearance/disappearance on the important nodes and its geographical position.
Evolution of Density and Clustering
When looking at normalized measurements, we observed a similar behavior for the two signals, but showing a different trend: as density is decreasing, clustering is growing monotonically. As in the previous case, the turning point between these two patterns are located around 7400–7300 so that after this time window, the pattern of growing clustering and decreasing density is more clear (see Figure 6).
From the beginning to the 7300–7400 windows, density and clustering decreased but, while density falls dramatically, clustering decreases very slowly (changes between window are ≤0.05). After this window, we found two stability phases: one with a medium-high density values (0.34/0.35; windows 7300–7200 and 7200–7100) and other with the lowest density values (0.24) in the last two windows. Between them, we found a phase with clear instability (from 7100 to 6900), when density is going down and up. During all this time, the behavior of clustering is unstable, showing the trend to grow that reaches its maximum in the last window.
Network Size and Structural Characteristics
The qualitative interpretation presented above led us to perform a deeper, quantitative analysis on the role of demographic evolution (here understood as the number of sites represented at each temporal window) in our case study. Figure 6 presents the temporal evolution of the density, clustering, and the number of sites (i.e., nodes in the network). In order to facilitate visual inspection, the later was normalized (i.e., by dividing each value by the maximum one). First of all, we note that the behavior of the number of nodes points out a three-stage pattern: first, from the beginning to 7400–7300, the network grows constantly. Then, there is a stabilization stage from 7300 to 6900, when networks reach its highest values; and finally, there is a clear decline in the last two windows.
One can observe that the evolution of density and clustering are somehow related to the above-described demographic changes (as both density and clustering depend, to some extent, on the number of nodes). However, the number of nodes alone cannot explain the behavior of these two metrics. Such an observation was confirmed by the correlation between the three signals, as we obtained values of −0.304 and 0.209 for network size–density and network size–clustering correlations, respectively.
As a natural step further, we turned our analysis to node turnover along the period under study. In other words, we focused on how many sites appeared and disappeared at each temporal window and which were their main characteristics (i.e., geographical location and network position). In order to get a deeper understanding of node turnover occurring in the system, we computed our indicator NTR to all networks. Table 3 lists the resulting values, as well as the corresponding partial counts.
NTR values present a progressive decrease throughout the period under study, with significant drops at temporal windows previously identified as turning point (i.e., 7400–7300 and 7100–7000). Moreover, the second drop matches with a signal change, from net growth to net reduction of the evolving system. Underlying such dynamics, we observe (a) a progressive increase of node disappearance across time windows, with a central period of stable values (from 7400–7300 to 6900–7000); and (b) a sharp transition around the second turning point from high to low node creation, followed by a slight increase. Taking all these observations in mind, we can organize the evolution of the networks into three stages:
Stage 1: the network experiences a strong growth clearly in the first three windows. Node creation is high so that NTR shows its highest positive values. Density and clustering decrease.
Stage 3: network declines (in the last two windows). At the same time that nodes are clearly declining, the behavior of density and clustery differs, so that while the latter is growing, the former fall off.
Between the two (Stage 2), there is a group of windows that we could define as oscillating stability stage. Here, the network grows and decreases, but reaches its highest values; one can envisage a more or less clear difference between the first two windows and the last ones (7100–6900). The behavior of density and NTR seems to point in this sense.
All to all, these observations enrich our three-stage interpretation of the phenomenon under study. NTR provides us with a valuable aggregated view over node turnover. Such a view can be complemented by analyzing separately the individual nodes disappearing from our networks, in order to figure out whether such changes at the local scale could have impacted the evolution of the system as a whole. To address this, we looked at the structural centrality of each disappearing node at last temporal window where it was present.
Shifting the Scale of our Analysis: Betweenness Centrality
We used betweenness as a centrality measure focusing the intermediation role of nodes under study. Notice that this choice fits perfectly to the criteria we had used to construct our networks, which highlighted information transfer among nodes. For each temporal window, we first computed the betweenness centrality of all nodes. Then, as a simple way of assessing the relative importance of each node among the rest and being able to compare values across temporal networks, we calculated their z-scores. Finally, we took the z-score values of the nodes to disappear at the following temporal window.
Table 4 shows these values for all temporal windows. By looking at their signs (i.e., negative or positive), we differentiate two situations. First, until 7200–7100, most of the disappearing nodes presented a negative z-score (indicating that their centralities were below the mean, so they tended to occupy peripheral positions in their networks). On the contrary, from 7100 to 7000 onward, negative and positive values balanced. A higher ratio of positive z-scores would suggest that sites occupying bridging or intermediating positions between styles were being abandoned, leading to a situation with progressively less contact between the corresponding cultural groups.
A similar picture can be obtained by looking at the sign of the sum of z-scores, despite a relevant difference in the window 6900–6800. It should be noted that the negative z-score values represented in a particular window are only noticeable in the next one, when the nodes indicated in gray disappear or change its betweenness centrality value. This means that from 7100 to 6800, there is a period of clear instability.
Betweenness centrality is a measure of global importance of a node within a network. For this reason, we expected to find correlations between the disappearance of high betweenness’ nodes (i.e., periods showing positive z-score) and sharp changes of density and clustering signals. Our assumption is satisfied in general but there are some exceptions, especially for the clustering. This suggests that the global approach is not enough to understand the mechanisms underlying the process under study and should be complemented with other sort of information. In particular, we decided to analyze separately subsets of the whole network defined by their geographical positioning. In other words, we incorporated certain geographical constraints (which were not included in our network construction procedure).
Network Position vs Geographical Location
In particular, we grouped the nodes into 11 regions and computed the average betweenness value for each region at each time window (Table 5). The regions were defined based on geographical criteria and limiting imbalances in the number of nodes per region. These regional betweenness’ values indicate the relative importance of each region within the whole system through time.
In the previous section, we observed that the network size of the overall network presented a growing trend that reaches its maximum at window 7200–7100 and a decreasing one, from this point to the end. However, this phenomenon did not reflect homogeneously at regional level. For example, some regions remained unpopulated (or, at least, there are not archeological evidences available) until maximum expansion was reached. Others, on the contrary, became unpopulated by the end. The Lower Ebro region display one of the most interesting behaviors (Figure 7). Starting in the 7500–7400, the mean betweenness grows reaching its maximum at 7300–7200. After this, two important falls (at 7100–7000 and 6900–6800) define its decline so that at the end, Lower Ebro does not seem to play any significance.
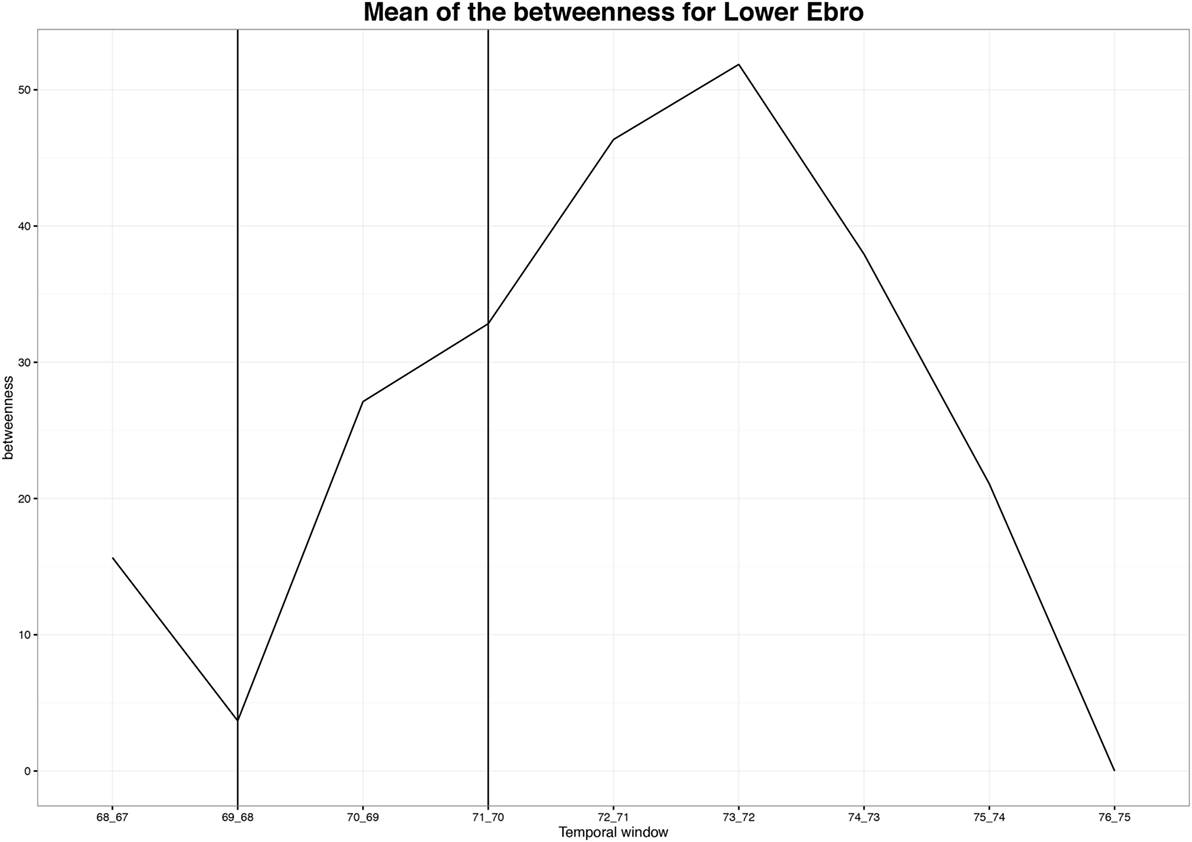
Figure 7. Mean betweenness for Lower Ebro region through time. Not the co-occurrence of between the drop of average betweenness in this region and the sharp decrease of the overall link density in the network (vertical lines).
This role is key to explain both the information flow between nodes and regions during this stage and its breakdown during the last windows. In this sense, looking at the distribution maps of nodes at the windows just before and after the drop of density (from 7200–7100 to 6900–6800) could be illustrative. Despite change present in all time windows, those occurring in windows 7100–7000 and 6900–6800 are especially relevant, because nodes located at the Lower Ebro reduce notably their betweenness centrality (see Figures 7 and 8).
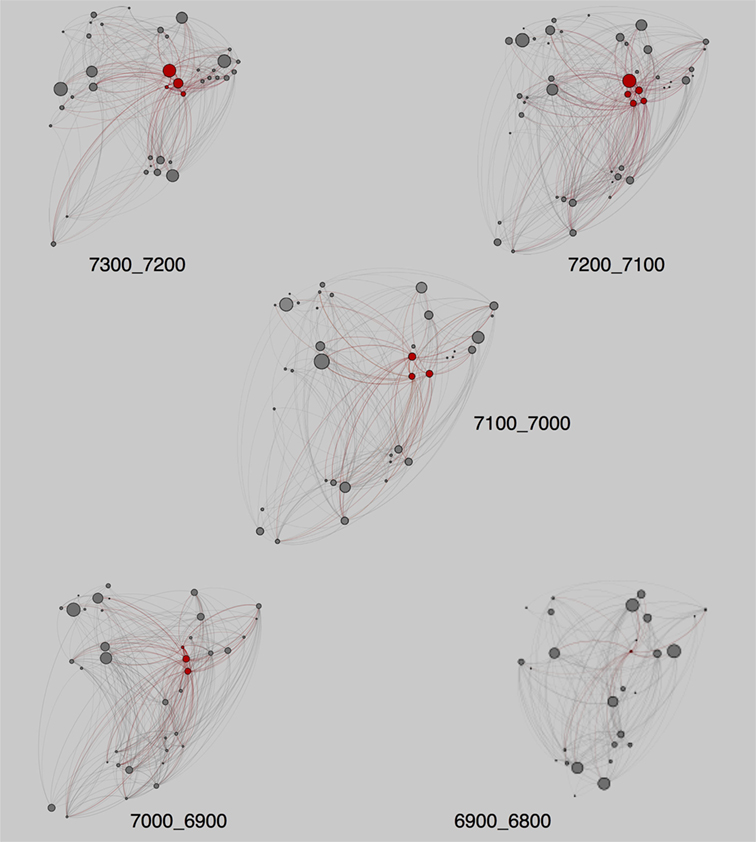
Figure 8. Graphs of the networks from 7300–7200 to 6900–6800. The size of the nodes represents its betweenness centrality. In red, nodes belonging to Lower Ebro region. Note that the drop of the betweenness centrality affecting the nodes in Lower Ebro region in windows 7100–7000 and 6900–6800 match with the fall of the density in this same temporal windows.
Discussion
As stressed above (see Introduction), most recent approaches about the Neolithic transition tend to describe the dynamics of Early Neolithic as a cycle of boom and bust. In the east of Iberia, using the c14 dates as a proxy of demography (Bernabeu et al., 2014), we identified a boom coinciding with the arrival of the Neolithic followed by a rapid decline by the end of the VIII or the beginning of VII millennium BP. This same pattern has been identified using a broader data base of radiocarbon dates covering all Iberia (Balsera et al., 2015). This demographic pattern is roughly coincident with the evolution of network size we found in this work. Moreover, as stressed in other works (Bernabeu et al., 2013, 2014, 2016), there are important changes coinciding with this cycle. In particular, we are interested in those related to the spatiotemporal patterns of material culture. During the Early Neolithic, this pattern could be described as a period in which the same items of material culture expanded over large areas, forming equally extensive archeological entities (i.e., cultures), followed by their fragmentation so that over the same areas archeologists can recognize more different entities (“cultures”) than earlier.
If we aggregate all the results obtained along Section “Results” (i.e., the evolution of structural measures including network size, as well as geographical node distribution and balance between node creation and node disappearance through temporal windows), we can differentiate three phases defining the evolution of our case study. In the following, we integrate these three phases with the archeological information of Eastern Iberia. In Figures 9–11, we show three maps that represent the style distribution in three time windows. Each of them has two maps: one represents the two major styles (Cardial and Epicardial, a style characterized by the use of impressions and incisions over the same pot); the second map shows the distribution of three minor styles: Boquique (slab-and-drag), Color (red pigments used in different ways), and Peinada (technique consisting in brushing the pot surfaces). The behavior of the minority styles is crucial to understand the fragmentation of the network occurred at the end of our study period.
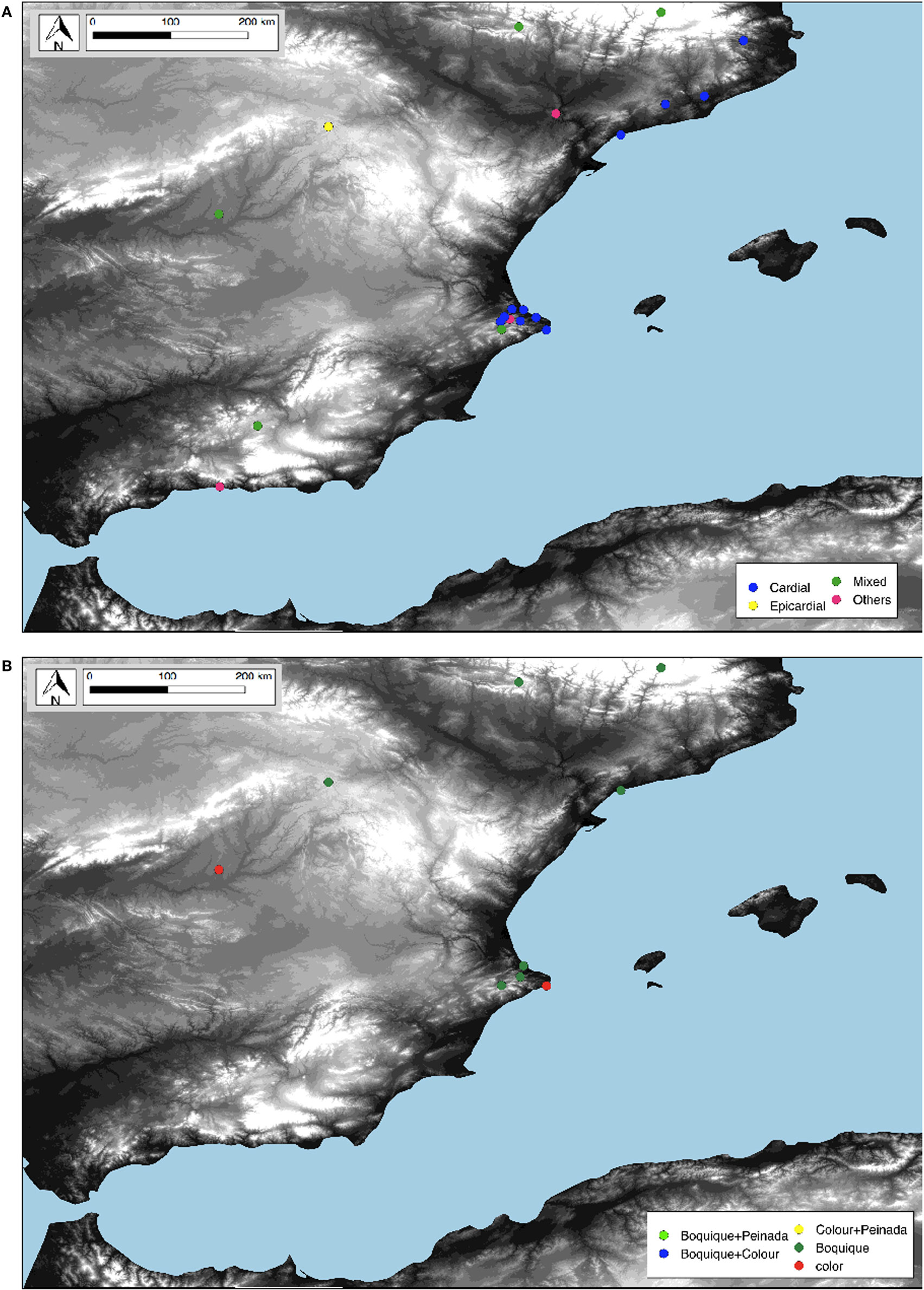
Figure 9. Pottery styles distribution at 7500–7400 time windows. (A) Cardial and Epicardial. (B) Other minor styles.
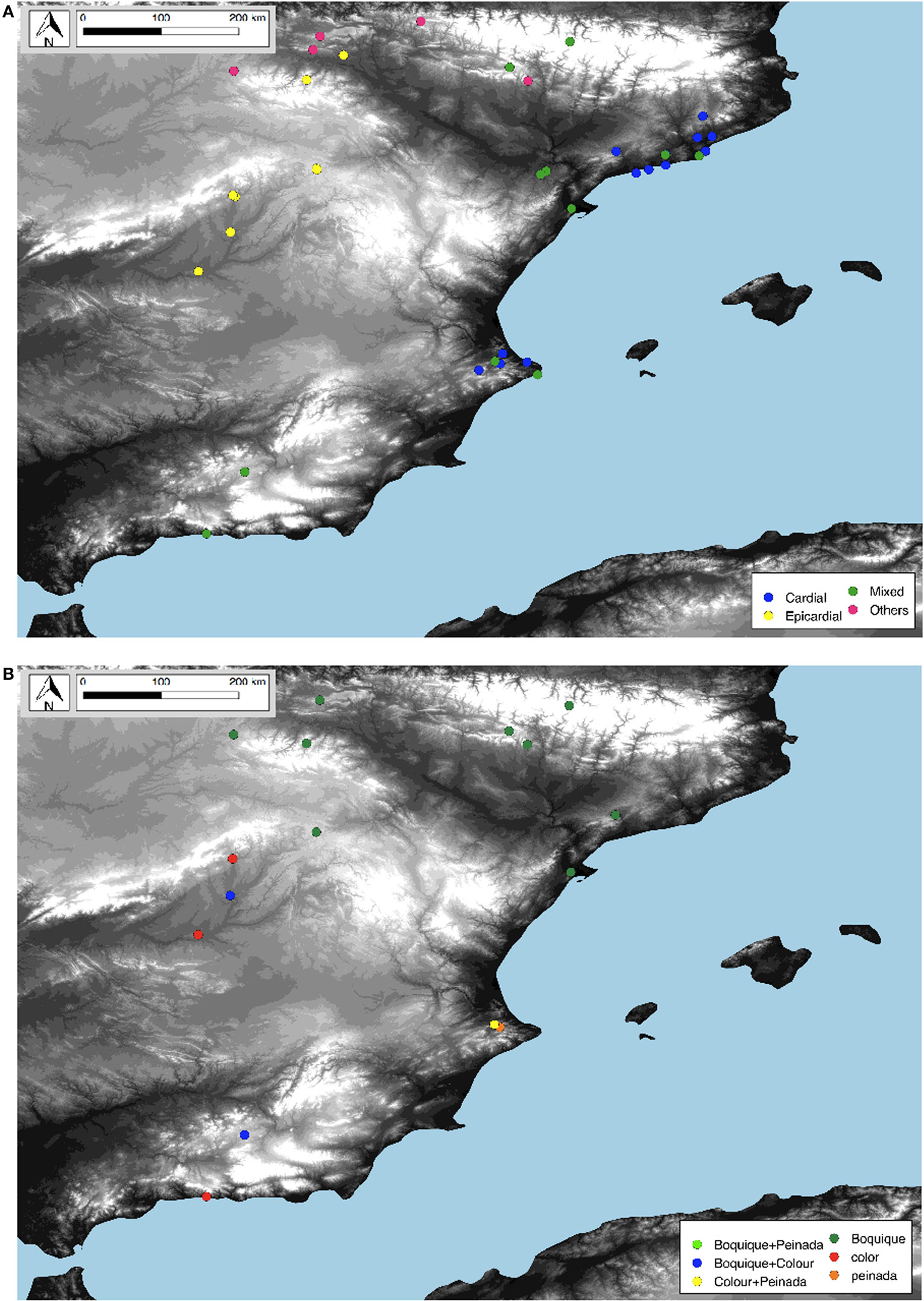
Figure 10. Pottery styles distribution at 7300–7200 time windows. (A) Cardial and Epicardial. (B) Other minor styles.
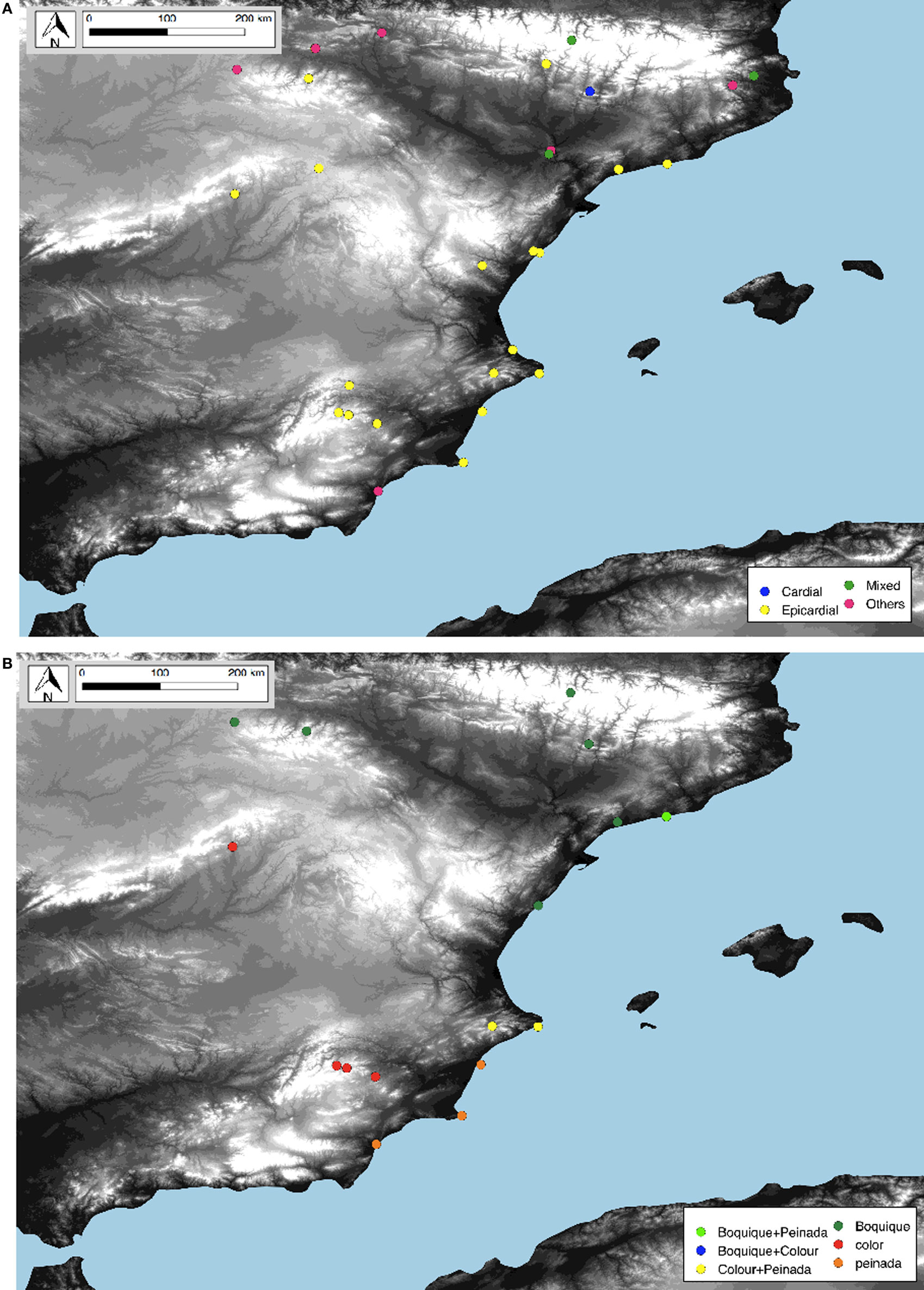
Figure 11. Pottery styles distribution at 6900–6800 time windows. (A) Cardial and Epicardial. (B) Other minor styles.
Expansion of the Cardial Network (7600–7300)
This is a growing phase, as it is shown by the increasing values of NTR across all time windows. During this phase, node distribution is very irregular and mainly concentrated along the coast. East and South-East remain empty, the occupation of N Catalonia and Lower Ebro begins during the window 7500–7400, and first evidences in Duero-Ebro correspond to the latest window (7400–7300). This suggests the existence of important geographical gaps at least until window 7400–7300. The decreasing values of density along this first phase could be related to this context of growing network size and irregular geographical distribution. Leaving aside the first window (with only 10 nodes), in window 7500–7400 N Catalonia and Nao Cape are the central regions of the network (i.e., the ones with the highest average betweenness). Both of them are coastal regions, thus highlighting the role of the maritime contact in the spread of Cardial styles along Mediterranean Iberia. By the end of this phase (window 7400–7300), there is a reconfiguration and some central regions, especially Lower Ebro, gain importance. This new scenario defines what will be typical of the next phase.
As pointed out above, one of the most striking issues discussed in the literature about this case study is the following: If, as commonly assumed, the spread of Neolithic to inner Iberia started from coastal sites, why didn’t it include Cardial styles? According to our results, this could be (at least partially) explained by the role played by the Lower Ebro region as intermediator. Notice that this is the region where the Ebro Valley (a natural corridor to inner parts of Iberia) meets the Mediterranean Coast. During the 7500–7400 time window, Cardial styles are represented in almost all regions (both coastal and inland). Epicardial is present in a few sites, but always together with Cardial styles (Figure 9A, mixed styles). In Lower Ebro, we have only recorded one site, and its record shows neither Cardial nor Epicardial styles. In the next window (7400–7300), old sites with Cardial styles located at Upper Ebro and Inland regions disappeared, and the new ones developed only the Epicardial style. Lower Ebro showed a pattern combining Cardial (only in coastal sites) and Epicardial (only in inland sites). The isolation of inland Cardial sites during the first time window (due to its absence in Lower Ebro) could have facilitated the disappearance of this style from inner Iberia in subsequent time windows. If this is not a result of our poor knowledge about the archeological record, the absence of Cardial styles in the inner regions could be explained as their isolation, something similar to the Tasmanian effect (Henrich, 2003).
The Cardial–Epicardial Network (7300–7200 and 7200–7100)
This is a stability phase, marked by the highest values of network size and a moderately positive NTR. During this phase, the network is characterized by the intermediary role played by the Lower Ebro region (see Table 5). From an archeological viewpoint, this phase is when Cardial and Epicardial styles develop separately in coastal and inland regions, respectively (Figure 10A). Along the period, we observe two phenomena: first, Epicardial tends to expand back over “Cardial regions,” and old Cardial styles remain only in areas occupied from the very beginning. This suggests scenario with more dynamic areas in inner Iberia and stagnation along the coast. Second, other styles emerge, but with a more limited geographical scope.
The Breakdown (7100–7000 to the End)
This third phase is characterized by a decline of network size and the lowest negative values of NTR (signaling that node disappearance exceeded node creation). At the same time, density is going down, reaching its lowest values in the last two windows in a context where clustering tends to increase.
The phase begins with an instability period (7100–7000 to 6900–6800). Density shows a noisy behavior since it stabilizes at their lowest values from 6900 to 6800 on. This period coincides with a sharp drop of Lower Ebro’s mean betweenness (see Table 5; Figure 11). After the 7000–6900 time window, the Lower Ebro region ceases to play the role played previously in the transmission of information. Interestingly, the regions acquiring more relevance (Nao Cape and SE to the South, Upper Ebro and Duero-Ebro to the North) are placed at the periphery of the network. This fact, together with an increase of the average clustering coefficient, would suggest a progressive division of the network into clusters, which would restrict information flows across the network and limit them to regions. From an archeological viewpoint, this translates into a severe decrease of the Cardial style (restricted to N Catalonia and Central Pyrenees) to play a marginal role during the last two windows, and the survival of Epicardial in limited areas. Finally, Figure 11B shows the scenario regarding the other styles. Boquique remains restricted to Northern regions, Color mainly in Southern inland regions, and Peinada in Southern coastal regions. In our view, this could be explained as the effect of network fragmentation affecting the way in which information flows (O’Brien and Bentley, 2011: p. 315) and favoring a more regional pattern in the distribution of cultural items.
This scenario marks the end of a time, the time of Early Neolithic. Several recent studies observe correlation and propose a causal relationship between climate and cultural change during the neolithization of Europe, including Gronenborn (2009, 2010) for central Europe and Cortés Sánchez et al. (2012) for the Iberian Peninsula. Of course, global climatic events as well as changes in human economic or social behavior may well have had effects for human societies. However, as some of us have already pointed out (Bernabeu et al., 2014, 2016), these effects were regionally variable and could not explain the above-introduced general dynamics of the first Neolithic. Consequently, we need to identify mechanisms linking local scenarios and general changes (Bernabeu et al., 2014, 2016).
As an alternative to purely climate-driven (or other global) drivers, we propose to examine (Bernabeu et al., 2014) the dynamics of land-use/landscape interactions of agricultural communities over generations. Results obtained by the MedLand project designed to carry out computational experiments on the long-term, recursive interactions between society, land use, and environmental change (Barton et al., 2010, 2012). Such results suggest that some kinds of agricultural practices could have an expansive effect over small communities located at valley bottoms, but as communities grow above a locally determined threshold size, the consequences of identical land-use practices change. Specifically, an imbalance between soil erosion and accumulation would be created, with the potential for leaving a catchment unsuitable for farming over the long term.
How might this kind of process have been responsible for the changes described at the entire system? The answer to this question may lie on the strategies that can mitigate such environmental degradation as reducing community size through emigration or fission (and colonization of new areas), or increasing the area devoted to grazing relative to cultivation. Some indicators suggest that this second option took place between the end of the VIII and the beginning of the VII millennium BP in several areas (Bernabeu et al., 2016). In any case, both strategies may have removed key local groups (i.e., nodes) from social networks, potentially resulting in the fragmentation of networks established earlier, limiting the information flow over the entire network and then modifying the system at a global scale. That is, changing the spatiotemporal patterns of “cultural diffusion.”
This can be described as a network through which information flows widely over extensive regions at first, favoring the formation of equally extensive archeological entities, while by the end, information flows more narrowly, facilitating the formation of differentiated archeological entities on these same regions. And then, it allows us to apply the methodological and theoretical tools developed by network science. The results obtained in this work suggest that the application of these methodologies may be a promising way of analyzing the cyclic behavior described for the first Neolithic in Iberia.
Concluding Remarks and Future Work
Summarizing, this study applied network analysis to address Early Neolithic in Iberia through the evolution of Cardial pottery decorative techniques. Specifically, we performed a three-step methodology. First, we divided the period under study into nine temporal windows. Then, we constructed networks based on cultural similarity across settlements. Finally, we compared diachronically these networks focusing on the evolution of their structural features and the cultural diversity observable at each node of the network. The work delivered two relevant outputs. First, it improved our understanding of the cyclic behavior of the first Neolithic. Second, focusing on the Iberian Peninsula, it provided a first explanation to the lack of diffusion of Cardial styles from coastal to inner areas.
In order to perform this diachronic study, we proposed two new analytical tools that could be highly useful in subsequent diachronic network analyses using archeological data. In particular, this second contribution of the article consisted on (a) a normalization procedure to compensate the effect of strongly disparate amount of evidences on similarity measures across site archeological records and (b) a bounded indicator of node turnover (i.e., replacement of old nodes by new nodes in subsequent network snapshots).
Still, this study has two main limitations that we aim to address in further works, in order to get a better understanding of Iberian early Neolithic societies. First, a significant part of our original database had to be discarded because the chronological assignation of some pieces was not precise enough. We addressed this by applying, to a region in the database, a Bayesian method that provides probability densities of dates. By generalizing this method to the whole database, we expect the size of the useful data to increase significantly. The second limitation is related to geographical constraints. Our study has shown the potential of combining network and geographical positioning to understand processes related to cultural change. However, we did not introduce geographical constraints when we built our networks, so making possible for regions far away from each other to be connected through long-range links. While this is correct, such long-range links can cover (at least partially) some of the phenomena we were analyzing in this work (e.g., regionalization of decoration techniques by the end of the period under study). Consequently, future works will reproduce this study using networks taking into account the geographical scenario.
Author Contributions
All the authors designed the research, performed the analysis, and wrote the manuscript. JA and SP-G compiled the data and defined the temporal windows to be considered. SL constructed the networks.
Conflict of Interest Statement
The research presented in this manuscript was conducted in the absence of any commercial or financial relationships that could be construed as a potential conflict of interest.
Acknowledgments
The authors thank Michael Barton (Arizona State University) for providing them with the R code used to calculate prior and posterior probabilities (see Relational Data), as well as the two anonymous reviewers and the editor, who helped to improve earlier versions of this work.
Funding
This work was partially funded by the research project of Government of Spain EVOLPAST (HAR2015-68962) and the research grant from the Agència de Gestió d’Ajuts Universitaris i de Recerca (AGAUR 2014-SGR-900). SL is supported by the Ramón y Cajal programme through the grant RYC-2012-01043.
Supplementary Material
The Supplementary Material for this article can be found online at http://journal.frontiersin.org/article/10.3389/fdigh.2017.00007/full#supplementary-material.
References
Balsera, V., Díaz-del-Río, P., Gilman, A., Uriarte, A., and Vicent, J.M. (2015). Approaching the demography of late prehistoric Iberia through summed calibrated date probability distributions (7000–2000 cal BC). Quaternary International: the Journal of the International Union for Quaternary Research 386: 208–11. doi:10.1016/j.quaint.2015.06.022
Barton, C.M., Ullah, I.I., and Bergin, S. (2010). Land use, water and Mediterranean landscapes: modelling long-term dynamics of complex socio-ecological systems. Philosophical Transactions of the Royal Society A: Mathematical, Physical and Engineering Sciences 368: 5275–97. doi:10.1098/rsta.2010.0193
Barton, C.M., Ullah, I.I.T., Bergin, S.M., Mitasova, H., and Sarjoughian, H. (2012). Looking for the future in the past: long-term change in socioecological systems. Ecological Modelling 241: 42–53. doi:10.1016/j.ecolmodel.2012.02.010
Bernabeu, J., García Puchol, O., Barton, C.M., McClure, S.B., and Pardo-Gordó, S. (2016). Radiocarbon dates, climatic events and social dynamics during the early Neolithic in Iberia. Quaternary International: the Journal of the International Union for Quaternary Research 403: 201–10. doi:10.1016/j.quaint.2015.09.020
Bernabeu, J., García Puchol, O., Pardo-Gordó, S., Barton, C.M., and McClure, S.B. (2014). Socioecological dynamics at the time of Neolithic transition in Iberia. Environmental Archaeology 19: 214–25. doi:10.1179/1749631414Y.0000000032
Bernabeu, J., Manen, C., and Pardo-Gordó, S. (2017). Spatial and temporal diversity during the Neolithic spread in the Western Mediterranean. The first pottery productions. In Times of Neolithic Transition along the Western Mediterranean Fundamental Issues in Archaeology, Edited by O. García Puchol and D.C. Salazar García. Springer. doi:10.1007/978-3-319-52939-4_14
Bernabeu, J., and Martí Oliver, B. (2014). The first agricultural groups in the Iberian peninsula. In Transitions in Mediterranean How Hunters Became Farmers, Edited by J. Guilaine, C. Manen, and T. Perrin, 419–438. Toulouse: Errance & Archives d’écologie préhistorique.
Bernabeu, J., Moreno, A., and Barton, C.M. (2013). Complex systems, social networks, and the evolution of social complexity in the East of Spain from the neolithic to pre-roman times. In The Prehistory of Iberia: Debating Early Social Stratification and the State, Edited by M.C. Berrocal, L. García Sanjuán, and A. Gilman Guillén, 53–73. New York: Routledge.
Bernabeu, J., Rojo, M.A., and Molina, L. eds. (2011). Las primeras producciones cerámicas: el VI milenio cal ac en la península Ibérica. In Saguntum Papeles Lab. Arqueol. València: Departament de Prehistòria i Arqueologia, Universitat de València.
Bocquet-Appel, J.-P. (2008). Explaining the Neolithic demographic transition. In The Neolithic Demographic Transition and Its Consequences, Edited by J.-P. Bocquet-Appel and O. Bar-Yosef, 35–55. Netherlands: Springer. doi:10.1007/978-1-4020-8539-0
Bronk Ramsey, C. (2009). Bayesian analysis of radiocarbon dates. Radiocarbon 51: 337–60. doi:10.1017/S0033822200033865
Brughmans, T. (2013). Thinking through networks: a review of formal network methods in archaeology. Journal of Archaeological Method and Theory 20: 623–62. doi:10.1007/s10816-012-9133-8
Collar, A., Coward, F., Brughmans, T., and Mills, B.J. (2015). Networks in archaeology: phenomena, abstraction, representation. Journal of Archaeological Method and Theory 22: 1–32. doi:10.1007/s10816-014-9235-6
Cortés Sánchez, M., Jiménez Espejo, F.J., Simón Vallejo, M.D., Gibaja Bao, J.F., Carvalho, A.F., Martinez-Ruiz, F., et al. (2012). The Mesolithic–Neolithic transition in southern Iberia. Quaternary Research 77: 221–34. doi:10.1016/j.yqres.2011.12.003
Coward, F. (2010). Small worlds, material culture and ancient Near Eastern social networks. In Social brain, distributed mind, Edited by R. Dunbar, C. Gamble and J. Gowlett 449–79. Oxford: Oxford University Press. doi:10.5871/bacad/9780197264522.003.0021
Crema, E.R., Kerig, T., and Shennan, S. (2014). Culture, space, and metapopulation: a simulation-based study for evaluating signals of blending and branching. Journal of Archaeological Science 43: 289–98. doi:10.1016/j.jas.2014.01.002
Fernández-López de Pablo, J., and Barton, C.M. (2015). Bayesian estimation dating of lithic surface collections. Journal of Archaeological Method and Theory 22:559–583. doi:10.1007/s10816-013-9198-z
Gronenborn, D. (2009). Climate fluctuations and trajectories to complexity in the Neolithic towards a theory. Documenta Praehistorica XXXVI: 97–110. doi:10.4312/dp.36.5
Gronenborn, D. (2010). Climate, crises, and the neolithisation of central Europe between IRD-events 6 and 4. In The Spread of the Neolithic to central Europe. International Symposium, Maiz 24 June–26 June 2005, Edited by D. Gronenborn and J. Petrasch, 61–81. Mainz: Verlag des Römisch-Germanischen Zentralmuseums.
Henrich, J. (2003). Cultural group selection, coevolutionary processes and large-scale cooperation. Journal of Economic Behavior & Organization 53: 3–35. doi:10.1016/S0167-2681(03)00094-5
Knappett, C. (2013). Network Analysis in Archaeology: New Approaches to Regional Interaction. Oxford: Oxford University Press.
Liu, J.-G., Hou, L., Pan, X., Guo, Q., and Zhou, T. (2016). Stability of similarity measurements for bipartite networks. Scientific Reports 6: 18653. doi:10.1038/srep18653
Mace, R., and Jordan, F.M. (2011). Macro-evolutionary studies of cultural diversity: a review of empirical studies of cultural transmission and cultural adaptation. Philosophical Transactions of the Royal Society of London. Series B, Biological Sciences 366: 402–11. doi:10.1098/rstb.2010.0238
Manen, C., Convertini, F., Binder, D., and Sénépart, I. eds. (2010). Premières sociétés paysannes de Méditerranée occidentale. Structures des productions céramiques. Toulouse: Société préhistorique française.
Martins, H., Oms, F.X., Pereira, L., Pike, A.W., Rowsell, K., and Zilhão, J. (2015). Radiocarbon dating the beginning of the Neolithic in Iberia: new results, new problems. Journal of Mediterranean Archaeology 28: 105–31. doi:10.1558/jmea.v28i1.27503
Mills, B.J., Clark, J.J., Peeples, M.A., Hass, W.R., Roberts, J.M., Hill, J.B., et al. (2013). Transformation of social networks in the late pre-Hispanic US Southwest. Proceedings of the National Academy of Sciences United States of America 110: 5785–90. doi:10.1073/pnas.1219966110
O’Brien, M.J., and Bentley, R.A. (2011). Stimulated variation and cascades: two processes in the evolution of complex technological systems. Journal of Archaeological Method and Theory 18: 309–35. doi:10.1007/s10816-011-9110-7
Ortman, S.G., Varien, M.D., and Gripp, T.L. (2007). Empirical Bayesian methods for archaeological survey data: an application from the Mesa Verde Region. American Antiquity 72: 241–72. doi:10.2307/40035813
Shennan, S., Downey, S.S., Timpson, A., Edinborough, K., Colledge, S., Kerig, T., et al. (2013). Regional population collapse followed initial agriculture booms in mid-Holocene Europe. Nature Communications 4:1–8. doi:10.1038/ncomms3486
Shennan, S.J., Crema, E.R., and Kerig, T. (2015). Isolation-by-distance, homophily, and “core” vs. “package” cultural evolution models in Neolithic Europe. Evolution and Human Behavior: Official Journal of the Human Behavior and Evolution Society 36: 103–9. doi:10.1016/j.evolhumbehav.2014.09.006
Keywords: complex network, Neolithic, cardial culture, Iberian peninsula, cultural evolution
Citation: Bernabeu Aubán J, Lozano S and Pardo-Gordó S (2017) Iberian Neolithic Networks: The Rise and Fall of the Cardial World. Front. Digit. Humanit. 4:7. doi: 10.3389/fdigh.2017.00007
Received: 09 November 2016; Accepted: 10 March 2017;
Published: 27 March 2017
Edited by:
Ben Marwick, University of Washington, USAReviewed by:
Lewis Borck, Universiteit Leiden, NetherlandsHeather Richards-Rissetto, University of Nebraska – Lincoln, USA
Copyright: © 2017 Bernabeu Aubán, Lozano and Pardo-Gordó. This is an open-access article distributed under the terms of the Creative Commons Attribution License (CC BY). The use, distribution or reproduction in other forums is permitted, provided the original author(s) or licensor are credited and that the original publication in this journal is cited, in accordance with accepted academic practice. No use, distribution or reproduction is permitted which does not comply with these terms.
*Correspondence: Salvador Pardo-Gordó, cGFyZ29yQHV2LmVz