- 1Department of Mathematical Modeling, Statistics and Bioinformatics, Ghent University, Ghent, Belgium
- 2Department of Pathology, VU University Medical Center, Amsterdam, Netherlands
- 3Department of Pathology, Cancer Research Center Groningen, University of Groningen, University Medical Center Groningen, Groningen, Netherlands
- 4Department of Gynecologic Oncology, Cancer Research Center Groningen, University of Groningen, University Medical Center Groningen, Groningen, Netherlands
Most next generation sequencing experiments generate more data than is usable for the experimental set up. For example, methyl-CpG binding domain (MBD) affinity purification based sequencing is often used for DNA-methylation profiling, but up to 30% of the sequenced fragments cannot be mapped uniquely to the reference genome. Here we present and evaluate a methodology for the identification of viruses in these otherwise unused paired-end MBD-seq data. Viral detection is accomplished by mapping non-reference alignable reads to a comprehensive set of viral genomes. As viruses play an important role in epigenetics and cancer development, 92 (pre)malignant and benign samples, originating from two different collections of cervical samples and related cell lines, were used in this study. These samples include primary carcinomas (n = 22), low- and high-grade cervical intraepithelial neoplasia (CIN1 and CIN2/3 - n = 2/n = 30) and normal tissue (n = 20), as well as control samples (n = 17). Viruses that were detected include phages, adenoviruses, herpesviridae and HPV. HPV, which causes virtually all cervical cancers, was identified in 95% of the carcinomas, 100% of the CIN2/3 samples, both CIN1 samples and in 55% of the normal samples. Comparing the amount of mapped fragments on HPV for each HPV-infected sample yielded a significant difference between normal samples and carcinomas or CIN2/3 samples (adjusted p-values resp. <10−5, <10−5), reflecting different viral loads and/or methylation degrees in non-normal samples. Fragments originating from different HPV types could be distinguished and were independently validated by PCR-based assays in 71% of the detections. In conclusion, although limited by the a priori knowledge of viral reference genome sequences, the proposed methodology can provide a first confined but substantial insight into the presence, concentration and types of methylated viral sequences in MBD-seq data at low additional cost.
1. Introduction
The advent of next generation sequencing (NGS) has initiated a revolution in molecular biology. Due to massively parallel sequencing, new insights could be revealed in genetics, transcriptomics and more recently epigenomics. However, the processing of the sheer amount of data produced by these methods proved to be a challenge. Identification of nucleotide sequences is often the first step in many NGS analyses, yet a substantial fraction cannot be properly identified. These unidentified fragments might arise from low-complexity regions (e.g., repeats), bacteria, viruses, other organisms or artificial noise (e.g., adaptor dimers, Head et al., 2014).
Previous studies have identified viruses by screening reads of RNA-seq from human samples. With this approach, the occurrence of EBV and CMV could be demonstrated in colorectal cancer and a landscape of viruses could be identified in a range of cancers (Khoury et al., 2013; Salyakina and Tsinoremas, 2013). In this project, we interrogated fragments from methyl-CpG binding domain enrichment based sequencing (MBD-seq) for a putative viral origin, thereby evaluating whether a similar approach could also be successful for DNA methylation studies.
MBD-seq is a methodology for the detection of CpG-methylation, an epigenetic modification that is essential for cellular differentiation and in processes such as genomic imprinting, X-chromosome inactivation and silencing of transposable elements (Jones, 2012). This method is based on the enrichment of CpG methylated fragments using methyl binding domains followed by massive parallel sequencing. By mapping these fragments to a reference genome, the putatively methylated locus can be determined. Though affected by several biases, the amount of mapped fragments to a locus can be considered as a proxy for the methylation degree of that locus. MBD-seq has been shown to be sufficiently sensitive, specific and cost effective for genome-wide studies (Serre et al., 2010; Aberg et al., 2012).
Viruses play an important role in public health. Aside from causing infectious disease, some are known to be clear risk factors for the development of cancer. Currently known oncoviruses include human papilloma virus (HPV), Epstein-Barr virus (EBV), Kaposi's sarcoma associated herpesvirus (KSHV), Human cytomegalovirus (CMV) and Merkel cell polyomavirus (MCP). It is estimated that viruses have a causal role in about 16% of all human cancers (Schiller and Lowy, 2010; de Martel et al., 2012). Therefore, prevention and vaccination for these viral infections could prevent the occurrence of the cancers they cause. Viral DNA detection has been previously achieved by a range of other methods (Bexfield and Kellam, 2011). State-of-the art methods are particularly sequencing based, for example combined with enrichment techniques or ultra deep sequencing (Allander et al., 2001; John et al., 2011; Lysholm et al., 2012). Enrichment based methods are however dependent on viral particles, which restrains them from detecting integrated viruses. Deep sequencing on the other hand gives an unbiased representation, but severely reduces the efficiency (Willner and Hugenholtz, 2013). With the advent of sequencing based viral research, also the need for specific bioinformatics tools became urgent (Fancello et al., 2012).
CpG methylation is known to play various roles in the life cycle of viruses and their oncogenicity (Hoelzer et al., 2008; Poreba et al., 2011). For example, papillomaviruses are generally hypomethylated when being actively replicated, but are heavily methylated while inserted into the host genome (Hoelzer et al., 2008). HPV might be mediating the methylation of its own genome, as HPV16's viral protein E7 is found to bind and stimulate the activity of DNA methyltransferase 1 (Dnmt1) (Burgers et al., 2007). Also, viral DNA hypermethylation of HPV is more prominent in carcinomas than in asymptomatic infections or dysplasia (Fernandez et al., 2009; Marongiu et al., 2014). In EBV, hypermethylation helps to hide its presence by inhibiting expression of viral latency proteins that could be recognized by cytotoxic T-cells (Paulson and Speck, 1999). Even the latency stage and the tumor type are associated with different methylation patterns of the EBV genome (zur Hausen, 2006). Adenoviruses have also been proven to be de novo methylated by insertion, but never in a free DNA stage (Doerfler, 2009). As several tumor-promoting and potentially methylable viruses remain to be identified, we aim at identifying viruses in the typically ignored non-reference aligned sequence reads of MBD-seq experiments.
Here, we demonstrate the usability and relevance of this approach on a collection of cervical samples, including cervical cancer and cervical intraepithelial neoplasia (CIN), which are putative cervical cancer precursors (Steenbergen et al., 2014). Cervical cancer is the third most occurring cancer among women worldwide and estimated prevalences of HPV in cervical cancer range above 99%, strongly supporting the causal role of HPV in cancer development (Walboomers et al., 1999; Ferlay et al., 2010). Cervical tissue is known to be frequently infected by HPV (Clifford et al., 2005) and HPV is often methylated (Hoelzer et al., 2008). Therefore, cervical samples make an ideal test set for the detection of methylated viruses, HPV in particular.
2. Materials and Methods
2.1. Samples and MBD-SEQ
Of the 92 samples, 39 samples originated from the VU University Medical Center (VUmc) in Amsterdam, further referred to as Set 1. Of this set, 10 samples were obtained from carcinoma, 12 are high-grade cervical intraepithelial neoplasia (CIN2/3), 3 are low-grade cervical intraepithelial neoplasia (CIN1) and 15 originate from cell cultures (See Table 1). These included 2 isolates of primary human foreskin keratinocytes (labeled EK), 10 DNA isolates of keratinocytes transfected with full length HPV16 and HPV18 DNA and the plasmid pcDNAIneo (Invitrogen) (different passages of cell lines FK16A, FK16B, FK18A, FK18B; Steenbergen et al., 1996), 2 DNA isolates of keratinocytes transduced with HPV16E6E7 cloned in the retroviral vector LZRS-MS-IERS-NEO/pBr (Kim et al., 2006; Steenbergen et al., 2013) and the cervical cancer cell line SiHa. In addition, 52 samples were collected from patients visiting the Department Gynecologic Oncology of the University Medical Center Groningen (UMCG), further referred to as Set 2. Of these samples, 12 samples are from carcinomas, 18 from High-grade cervical intraepithelial neoplasia (CIN2/3), 2 from leukocytes and 20 from normal cervical tissue. The two leukocyte samples were pooled samples from each 2 persons. This study has been approved by the ethical committees of UMCG and VUmc, adhering to the declaration of Helsinki.
To obtain the DNA methylation profiles, the MethylCap kit from Diagenode was combined with Illumina Genome Analyzer IIx paired-end sequencing as described in (De Meyer et al., 2013) except for using 500 ng of input DNA instead of 200 ng. Due to data corruption in a compressed format, data for one CIN1 (complete) and one normal (partially) sample were unavailable for further processing. Therefore, only 2 CIN datasets were available, resulting in a total of 91 samples for analysis. Bowtie 1.0.0 was used to subsequently map the obtained paired-end reads (51 bp) from fastq-files to the human reference genome of NCBI v37 (Langmead et al., 2009). A maximum insert size was set at 400 bp and up to 3 mismatches were allowed in the seed sequence to avoid too stringent mapping. For other parameters, the default settings were used. DNA molecules for which the paired-end reads could not be mapped to the reference genome will be further referred to as “non-canonical” fragments, whereas “canonical” fragments will be used to refer to fragments that could be aligned to the reference genome. The non-canonical fragments can be obtained from our website (http://www.biobix.be/viralmbd/).
2.2. Virus Detection
We aimed to identify fragments of viral origin. This was achieved by searching for sequence similarity between the non-canonical reads and a set of viral reference genomes. For this purpose, we used FR-HIT (Niu et al., 2011). All viral genomes from NCBI and EMBL-EBI were used for the construction of a set of viral reference genomes (http://www.ebi.ac.uk/genomes/virus.html & http://www.ncbi.nlm.nih.gov/genomes/GenomesGroup.cgi) (Wheeler et al., 2006; Leinonen et al., 2011). For reference genomes with a sequence similarity of over 95% (cut-off), the shortest genomes were removed with CD-HIT-EST (Fu et al., 2012). This prevents a bias for those fragments for which there are more similar reference genomes. Mapping of paired reads on different, but very similar reference genomes are not being withheld and would therefore otherwise create false negatives. In order to diminish false positive identifications, several precautions were taken. First, reads featured by low complexity (dust-score >4) were filtered out with prinseq-lite (Schmieder and Edwards, 2011). Second, FR-hit was forced to utilize the complete reads by using the “global mapping” strategy and only the best hits with an e-value smaller than 10−5 were used. Finally, only if both best hits from each paired-end originated from the same virus, viral identification was affirmed. Duplicated fragments, which have the same start for their first read and the same end position for their second read, were removed. By default, FR-hit masks reference sequences for low complexity regions, however since such a filtering is performed on the reads, this function was disabled. The end result of this approach is a dataset of virus (v) specific counts (Nvs) for each sample (s). Whenever we observed an Nvs > 0, we reported the virus to be present for that sample. Scripts for the execution of the pipeline can be found here: https://github.com/klamens/viral-pipeline.
2.3. Statistical Tests
Testing for association between histological origin (carcinoma, CIN2/3, CIN1, normal) and the presence of HPV in a sample was performed by Pearson's Chi-squared test with 2000 simulated permutations. Association of high-risk HPV type occurrence and histological groups was tested as well. The most abundant HPV type per sample was used for the assessment of high/low risk HPV type occurrence. When abundances of the most and second most abundant type were equal and their risk was different, the sample was rejected for testing. For a comparison of the fraction of viral fragments, Nvs-values were normalized relative to the total fraction of sequenced fragments. These normalized fractions are denoted as Rvs. The fractions of counts mapped to specific viruses were compared and tested for with the Kruskal-Wallis Test between the different histological groups. These groups included samples from carcinoma, CIN2/3, CIN1, normal and only for HERV-K113 also cell cultures and leukocytes. Post-hoc analyses were performed with the Mann-Whitney-Wilcoxon Test and p-values were adjusted for multiple testing by Bonferroni correction (Hochberg, 1988). For both the Kruskal-Wallis Test and the Mann-Whitney-Wilcoxon Test, a location shift assumption was made, resulting in testing for a difference between the medians of Rvs. Statistical analyses and graphical plot creations were performed within the statistical environment R (Wickham, 2009; R Core Team, 2012).
2.4. HPV Type Verification
Samples of Set 1 were assessed for HPV (type) presence using the GP5+/6+ PCR followed by enzyme immunoassay (EIA) read-out system using a probe cocktail of 14 high-risk HPV types (HPV16, 18, 31, 33, 35, 39, 45, 51, 52, 56, 58, 59, 66, and 68) (Jacobs et al., 1997). Reverse line blot was used to genotype all EIA-positive samples (van den Brule et al., 2002) using probes for HPV-types 6, 11, 16, 18, 26, 30, 31, 33, 34, 35, 39, 40, 43, 45, 51, 52, 53, 54, 55, 56, 57, 58, 59, 61, 66, 67, 68, 69, 70, 71, 73, 81, and 82. Samples of Set 2 were tested for presence of high-risk HPV-DNA with both the HPV GP5+/6+ general primers, and HPV16- and HPV18-specific primers (Wisman et al., 2006) as performed routinely in the ISO-15189 accredited laboratory. In all tests a serial dilution of DNA extracted from CaSki (ATCC; CRL1550; 500 integrated HPV16 copies), HeLa (ATCC; CCL2; 20–50 integrated HPV 18 copies), SiHa (ATCC; HTB35; 1–2 integrated HPV16 copies), CC10B (HPV45-positive cell line) and CC11 (HPV67 positive cell line), and HPV-negative cell lines were included as control for the analytical specificity and sensitivity of each hrHPV-PCR (Tjon Pian Gi et al., 2014). To assess the MBD-seq based HPV type identification, concordances for samples with and without the specific HPV types were calculated.
3. Results
3.1. Non-Canonical Fragments
On average, 29% (SD = 9%) of all fragments in each sample could not be aligned to the human reference genome. Of these reads, only 0.31% (SD = 0.17%) could be mapped to the viral reference genomes. In total, we tried to map reads of 4.3×108 non-canonical fragments to 6433 different viral genomes, obtained after removal of very similar genomes (see Materials and Methods). More details about the mapping statistics can be found in the Supplementary Material. As MBD-seq enriches for methylated CpGs, a high-quality dataset should include only a limited amount of fragments without any CpG, and most fragments should have multiple CpGs (De Meyer et al., 2013). This holds for both sample sets (1 and 2) as depicted in Figure 1A. Differences in the number of CpGs per fragment per sample between Sets 1 and 2 can be explained by differences in fragment length (Figure 1B). Overall, this analysis suggests that most identified viruses (see below) are indeed methylated.
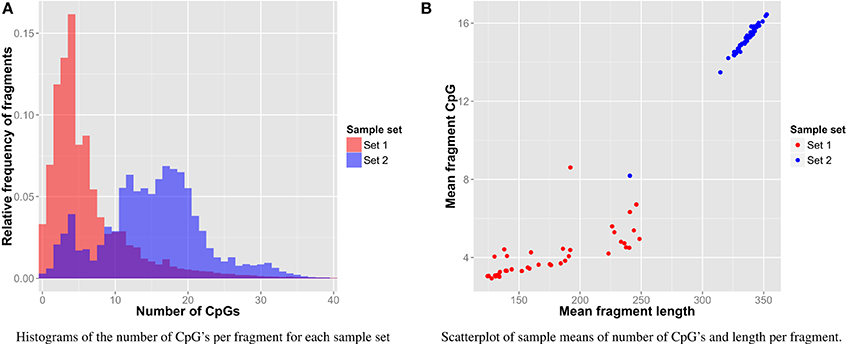
Figure 1. Fragment CpG content. (A) Histogram of CpG content per sample set. (B) Relation of CpG content and length per sample.
3.2. Detected Viruses
In a first phase, the presence of specific viruses in the different sample sets was assessed (see Table 2). For all samples, fragments similar to the human endoretrovirus K113 (HERV-K113) could be identified. However, as sequence identities of mapped reads with HERV-K113 were sometimes as low as 75%, it is most likely that these fragments originate from other HERV-Ks as well. A significant difference in Rvs for these HERV-K113 similar fragments could be demonstrated between the histological groups (p < 0.0001), but post-hoc tests revealed only significantly higher HERV-K113-like fractions in the cell cultures compared to normal tissue, CIN2/3 and carcinomas (all p ≤ 0.001, data not shown).
Also phages were frequently observed in various samples, though in very low abundances in all cell culture samples and CIN1 samples. Enterobacteria phage PhiX174 is the most occurring phage. This isn't surprising, as PhiX174 is being used as a spike-in for quality and calibration control in the Illumina sequencing protocol. Other phages that were observed at lower levels were, among others, phage lambda and phage P1 (data not shown).
Human adenoviruses were discovered in multiple samples. More fragments were observed in samples originating from Set 1 compared to those of Set 2. The most occurring types were human adenovirus C and human adenovirus B. Two CIN2/3 samples contained a single fragment of the Merkel cell polyomavirus. Multiple, particularly carcinoma and CIN2/3, samples were found to contain one to 25 fragments of the Epstein-Barr virus. Human cytomegalovirus was only detected in cell culture samples. However, these fragments most likely originate from the CMV promoter which is included in the pcDNAI neo plasmid. Human herpes virus 1, 6, and 7 were also identified, each in just a single sample.
HPV was detected in all but one sample in the carcinoma group and the cell culture group. It was discovered in all samples originating from the CIN2/3 group and in 11 of the 20 normal samples. Also in both samples of CIN1 and in one of the two leukocyte samples HPV was detected. Association between the presence of HPV and cervical groups (excluding cell culture samples and leukocytes) was assessed for by Pearson's Chi-squared test with simulated (p < 0.001).
Next to assessing the (differential) presence of specific viruses, also a quantitative analysis can be performed. To illustrate the feasibility, HPV Rvs in HPV-positive samples were compared between the carcinoma, CIN2/3, CIN1 and normal groups (see also Figure 2). A significant difference between these groups was demonstrated (p = 0.0001). Post-hoc analyses reveal significant differences between the normal group and cell carcinoma and CIN2/3 samples (see Table 3). It should be noted that the absence of significance for the comparisons with CIN1 may be explained by a lack of power (n = 2).
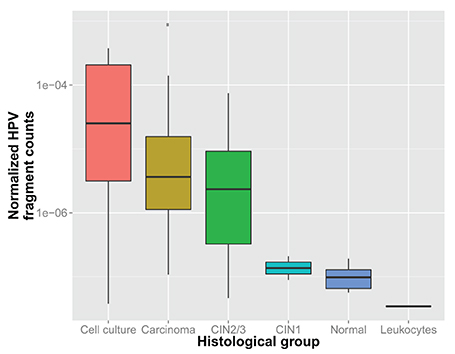
Figure 2. Normalized HPV fragment counts (Rvs) within each sample for which HPV was found, per histological group.
Often multiple HPV types were detected per sample as can be observed in Figure 3A and Table 4. In Figures 3B–D one can see which HPV types were detected in each histological group. The most detected HPV type in primary cervical samples was HPV16 (N = 30), followed by HPV31 (N = 12), HPV39 (N = 9), HPV18 (N = 6), and HPV36 (N = 6). Other HPV types could not be observed in more than 4 different samples (see Figure 3). We observed a higher relative occurrence of high-risk HPV types in carcinoma and CIN2/3 samples with HPV compared to normal samples, but the association was not significant.
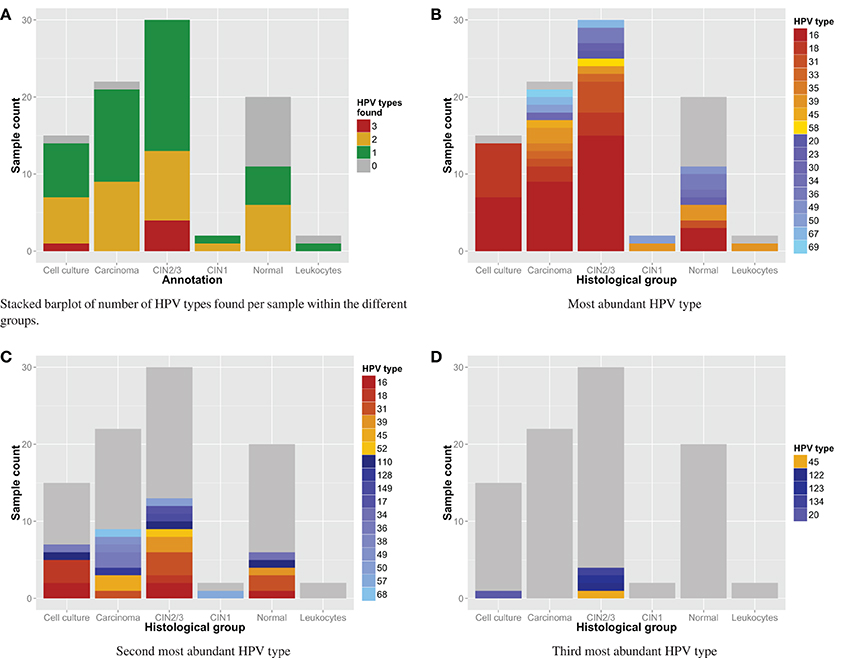
Figure 3. (A) detection of HPV types: number of HPV types found per sample. (B–D) Stacked barplot of HPV types found per sample with n-th most fragments within the different groups. Red to gold and blue colored types correspond with respectively high and low risk HPV types.
Though the HPV type analysis yielded relevant results, the overall accuracy of this approach should be evaluated as well. Therefore, verification of the HPV types was performed using independent methods (see Materials and Methods), which we consider here as gold standard. The independent validation of the HPV types yielded a positive verification in 71% of the detections. For HPV types indicated to be present by these methods, results were 66% concordant with the MBD-seq approach. Vice versa, verified absence of viruses was 98% concordant with the proposed methodology. The more fragments that were detected for an identified HPV type, the more likely it was to be validated as can be seen in Table 5. As the verification methodology differed between Sets 1 and 2, results per sample collection can be observed in Figure 4.
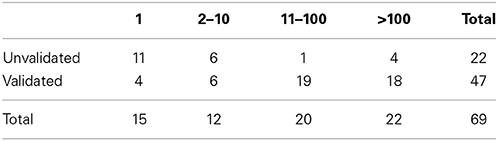
Table 5. Overview of the number of validated HPV types according to the amount of detected fragments.
4. Discussion
In this study, we demonstrated that the non-canonical fraction of MBD-seq fragments can be used to identify viruses. Considering the increasing importance of sequencing methods, this strategy can provide key evidence regarding the involvement of specific viruses in pathologies at minimal additional cost. Given the roles of DNA methylation in virus biology, the outlined pipeline is capable to generate valuable hypotheses from otherwise unused data. As the outlined application has also several disadvantages (see below), the generated hypotheses should of course be additionally validated by state-of-the art methods. The observed CpG content in many cervical samples, in comparison with De Meyer et al. (2013), suggests that most viral mapped fragments are methylated. It should be noted that unmethylated viral fragments picked up as “noise” may also be relevant, but that the sensitivity for these fragments will most likely be too low to link it to the specific pathology under study.
Recently, some studies already achieved viral identification in RNA-seq experiments by comparable methods (Chen et al., 2013; Salyakina and Tsinoremas, 2013). These studies could find substantial presence of oncoviruses by their transcripts. However, integrated viruses may be temporarily transcriptionally silent, often by DNA methylation, making the proposed methodology a good complement to RNA-seq for viral identification as transcriptionally silenced viruses will also be detected. Moreover, it is capable of revealing epigenetic information about the clinical virus biology. Our method is generic and could be used in combination with other NGS techniques. However, FR-HIT does not account for splicing events which might restrict its applicability to RNA-seq data.
The outlined approach was used on cervical samples of different origin, both histologically and study-wise, and multiple viruses were detected. Not unexpectedly, fragments originating from HERV-K were observed in every sample, which can be considered as a positive control as HERV-K is an endogenous retrovirus (Hohn et al., 2013). Significantly more HERV-K fragments could be observed in the cell culture samples vs. normal tissue, CIN2/3 and carcinomas, which might reflect methylation differences between cell culture and primary samples (Smiraglia, 2001; Varley et al., 2013). This result therefore provides a first indication that also a quantitative comparison of virus count data may yield relevant information. Other expected detections include Phage PhiX174 DNA from Illumina spike-ins and CMV that originated from the pcDNAI neo plasmid in cell culture samples. Indeed, plasmids have been shown to be methylated, which can interfere with specific experiments (Hong et al., 2001).
Interestingly, we detected several oncoviruses in the cervical samples besides HPV. Merkel cell polyomavius, known to cause the Merkel cell sarcoma, was found to be present in two CIN2/3 samples (Feng et al., 2008). Another identified oncovirus is the Epstein-Barr virus. Although not significant, an apparent association between the presence of the Epstein-Barr virus and histological group hints toward its oncogenic role in cervical cancer as has been stated in (Szostek et al., 2009). However, since the counts for Epstein-Barr were low, viral fragments originating from infiltrating lymphocytes is at least an equivalent alternative (Grywalska et al., 2013). Results from this study therefore indicate that additional research should be performed regarding the impact of Epstein-Barr virus and Merkel cell polyomavirus superinfection in CIN2/3 and carcinomas, preferably in far larger groups.
The most prevalent oncovirus however, as expected, was HPV. As the prevalence of HPV in cervix and its causal role in cervical cancer is well documented, the virus detection efficiency of the proposed methodology verifies the capabilities of our method (Clifford et al., 2005; Armstrong, 2010). The role of HPV in cervical cancer was shown by two comparisons. First, there is a significant association of HPV occurrence and histological group. Second, in HPV positive samples we observed a significant increase in total HPV fragments per sample in cell culture, carcinoma or CIN2/3 samples vs. normal samples. The latter observation could be due to more HPV and/or more HPV methylation. More DNA methylation of the HPV genome in carcinomas is in accordance with observations for HPV16 and HPV18 as reported by Fernandez et al. (2009).
However, note that the quantitative evaluation of methylated viruses may also be affected by the global genomic methylation state. Genomic hypermethylation, as often observed in cell lines (Smiraglia, 2001), might suppress viral estimates as their relative abundance in the total methylated fraction may drop. On the other hand, overall hypermethylation may also lead directly to increased viral methylation, and therefore higher sensitivity for MBD-based capturing. A similar reasoning may be relevant for tumor samples, which might feature global hypomethylation (Li et al., 2014). In other words, the overall methylation state will have an important impact, but the exact effect depends on how much viral methylation itself or the detection of methylation is affected by it.
Phages were detected in primary samples from both sample sets and were absent in all cell culture samples. This is not unexpected as the female genital tract is featured by complex microbiological flora and phage genomes have since long been reported to be methylated (Krüger and Bickle, 1983; Martin et al., 2012). The presence of human adenoviruses might be explained by contamination. Both human adenovirus B and C are known to play a role in respiratory diseases, which might explain a possible way of contamination (Jones et al., 2007). The remarkable difference of human adenovirus fragment occurrence between the sample sets reinforces this hypothesis. Observation of HPV in one leukocyte sample might be explained by contamination as well.
Hence, for virus detection with a low fragment count, one should be cautious in concluding viral presence. The high sensitivity of NGS will cause the results regarding presence or not to be easily affected by contamination Yozwiak et al. (2012). For example, HPV39 was detected several times at low fragment count in samples that were run in the same illumina Genome Analyzer lane as one sample with a remarkable high HPV39 fragment count. Also, the high amount of HPV39 positive samples seems to deviate from its relative low prevalence in Europe, this in contrast with the other HPV types (16,18,31) (Clifford et al., 2005). These fragments were most likely categorized in the wrong sample due to carryover associated with common inaccuracies in Illumina multiplex sequencing (Kircher et al., 2012). Improper identifications due to wrong mapping is less likely as viral genomes with high similarity were represented by only one reference genome per group. Furthermore, we checked some of the single HPV hits by blasting them to NCBI nucleotide archive which gave us best hits for the found HPV's. Contamination might therefore partly explain the seemingly high superinfection rate of HPV types. One might therefore opt to only call virus presence upon identification with a minimum fragment count, for example 10 (as also suggested by Yozwiak et al., 2012 and Salyakina and Tsinoremas, 2013). Additionally, the use of double indexing during Illumina multiplex sequencing will remove a major experimental source of carryover contamination (Kircher et al., 2012). For example, HPV detections in samples of Set 1 with more than 10 fragments could all but two be verified. Alternatively, next to contamination, MBD-seq might also be featured by a higher sensitivity due to enrichment for methylation, compared to the methylation naive verification methods. However, it will likely not detect viruses of which no methylated DNA is present.
Another limitation of this best mapping hit based approach is that it enterily depends on existing known viral genomes. In this study, only full genomes of NCBI and ENA were used. However, as the portion of sequenced genomes (6433 in our dataset) is very limited compared to the amount of mammalian viruses estimated at 320.000 (Anthony et al., 2013), it is very likely that many viruses will be missed by this method. Related viruses can be detected by lowering the stringency of sequence similarity. However, this implies an increasing difficulty to distinguish viral types. Distinct viral types will also be harder to distinguish when the set of reference genomes increases as more similar genomes enter. This problem can be solved by clustering and removing similar genomes or by technological advances that increase the length of the sequenced reads. Finally, also horizontal gene transfer or ancestral viral integrations may create false positives. De novo assembly of viruses using unmapped fragments largely avoids the dependency on current knowledge and mapping problems, but will require large coverages to obtain sufficient amounts of viral fragments and will be hampered by unmethylated regions of the viral genome.
Generally, we can conclude that this method is effective in detecting fragments of methylated viral DNA. This could be verified by HPV detection in the cervix case study, demonstrating (i) association of HPV presence and histological group (ii) differential quantities of HPV fragments in HPV positive samples between normal samples and carinoma or CIN2/3 samples (iii) type detection with good concordance as verified by independent methods. In other words, if the impact of HPV in cervical cancer would have been unknown, it might have been picked up by the outlined approach, though additional validation would of course have been absolutely necessary. It is therefore clear that the methodology can generate novel knowledge regarding the presence of viruses in disease, and that the inherent disadvantages are by far outweighed by the major benefit of obtaining information regarding the presence of any sequenced virus in otherwise typically discarded data.
Author Contributions
Ed Schuuring, Renske D. M. Steenbergen and G. Bea A. Wisman provided the data regarding the cervical samples and performed the HPV type verifications for these samples. Tim De Meyer and Klaas Mensaert conceived the general idea and approach. Klaas Mensaert and Tim De Meyer have designed the pipeline, analyzed the data and wrote the manuscript. Wim Van Criekinge and Olivier Thas have contributed to the conceptual development and provided critical advice. All authors have reviewed the article and approved the final manuscript.
Conflict of Interest Statement
The authors declare that the research was conducted in the absence of any commercial or financial relationships that could be construed as a potential conflict of interest.
Acknowledgment
We acknowledge the financial support of the N2N Multidisciplinary Research Partnership of the University of Ghent for both Klaas Mensaert and Tim De Meyer.
Supplementary Material
The Supplementary Material for this article can be found online at: http://www.frontiersin.org/journal/10.3389/fgene.2015.00016/abstract
References
Aberg, K. A., McClay, J. L., Nerella, S., Xie, L. Y., Clark, S. L., Hudson, A. D., et al. (2012). MBD-seq as a cost-effective approach for methylome-wide association studies: demonstration in 1500 case–control samples. Epigenomics 4, 605–621. doi: 10.2217/epi.12.59
Pubmed Abstract | Pubmed Full Text | CrossRef Full Text | Google Scholar
Allander, T., Emerson, S. U., Engle, R. E., Purcell, R. H., and Bukh, J. (2001). A virus discovery method incorporating DNase treatment and its application to the identification of two bovine parvovirus species. Proc. Natl. Acad. Sci. U.S.A. 98, 11609–11614. doi: 10.1073/pnas.211424698
Pubmed Abstract | Pubmed Full Text | CrossRef Full Text | Google Scholar
Anthony, S. J., Epstein, J. H., Murray, K. A., Navarrete-Macias, I., Zambrana-Torrelio, C. M., Solovyov, A., et al. (2013). A strategy to estimate unknown viral diversity in mammals. mBio 4:e00598-13. doi: 10.1128/mBio.00598-13
Pubmed Abstract | Pubmed Full Text | CrossRef Full Text | Google Scholar
Armstrong, E. P. (2010). Prophylaxis of cervical cancer and related cervical disease: a review of the cost-effectiveness of vaccination against oncogenic HPV types. J. Manag. Care Pharm. 16, 217–230.
Bexfield, N., and Kellam, P. (2011). Metagenomics and the molecular identification of novel viruses. Vet. J. 190, 191–198. doi: 10.1016/j.tvjl.2010.10.014
Pubmed Abstract | Pubmed Full Text | CrossRef Full Text | Google Scholar
Burgers, W. A., Blanchon, L., Pradhan, S., de Launoit, Y., Kouzarides, T., and Fuks, F. (2007). Viral oncoproteins target the DNA methyltransferases. Oncogene 26, 1650–1655. doi: 10.1038/sj.onc.1209950
Pubmed Abstract | Pubmed Full Text | CrossRef Full Text | Google Scholar
Chen, Y., Yao, H., Thompson, E. J., Tannir, N. M., Weinstein, J. N., and Su, X. (2013). VirusSeq: software to identify viruses and their integration sites using next-generation sequencing of human cancer tissue. Bioinformatics 29, 266–267. doi: 10.1093/bioinformatics/bts665
Pubmed Abstract | Pubmed Full Text | CrossRef Full Text | Google Scholar
Clifford, G. M., Gallus, S., Herrero, R., Muńoz, N., Snijders, P. J. F., Vaccarella, S., et al. (2005). Worldwide distribution of human papillomavirus types in cytologically normal women in the international agency for research on cancer HPV prevalence surveys: a pooled analysis. Lancet 366, 991–998. doi: 10.1016/S0140-6736(05)67069-9
Pubmed Abstract | Pubmed Full Text | CrossRef Full Text | Google Scholar
de Martel, C., Ferlay, J., Franceschi, S., Vignat, J., Bray, F., Forman, D., et al. (2012). Global burden of cancers attributable to infections in 2008: a review and synthetic analysis. Lancet Oncol. 13, 607–615. doi: 10.1016/S1470-2045(12)70137-7
Pubmed Abstract | Pubmed Full Text | CrossRef Full Text | Google Scholar
De Meyer, T., Mampaey, E., Vlemmix, M., Denil, S., Trooskens, G., Renard, J.-P., et al. (2013). Quality evaluation of methyl binding domain based kits for enrichment DNA-methylation sequencing. PLoS ONE 8:e59068. doi: 10.1371/journal.pone.0059068
Pubmed Abstract | Pubmed Full Text | CrossRef Full Text | Google Scholar
Doerfler, W. (2009). Epigenetic mechanisms in human adenovirus type 12 oncogenesis. Semin. Cancer Biol. 19, 136–143. doi: 10.1016/j.semcancer.2009.02.009
Pubmed Abstract | Pubmed Full Text | CrossRef Full Text | Google Scholar
Fancello, L., Raoult, D., and Desnues, C. (2012). Computational tools for viral metagenomics and their application in clinical research. Virology 434, 162–174. doi: 10.1016/j.virol.2012.09.025
Pubmed Abstract | Pubmed Full Text | CrossRef Full Text | Google Scholar
Feng, H., Shuda, M., Chang, Y., and Moore, P. S. (2008). Clonal integration of a polyomavirus in human Merkel cell carcinoma. Science 319, 1096–1100. doi: 10.1126/science.1152586
Pubmed Abstract | Pubmed Full Text | CrossRef Full Text | Google Scholar
Ferlay, J., Shin, H.-R., Bray, F., Forman, D., Mathers, C., and Parkin, D. M. (2010). Estimates of worldwide burden of cancer in 2008: GLOBOCAN 2008. Int. J. Cancer 127, 2893–2917. doi: 10.1002/ijc.25516
Pubmed Abstract | Pubmed Full Text | CrossRef Full Text | Google Scholar
Fernandez, A. F., Rosales, C., Lopez-Nieva, P., Graña, O., Ballestar, E., Ropero, S., et al. (2009). The dynamic DNA methylomes of double-stranded DNA viruses associated with human cancer. Genome Res. 19, 438–451. doi: 10.1101/gr.083550.108
Pubmed Abstract | Pubmed Full Text | CrossRef Full Text | Google Scholar
Fu, L., Niu, B., Zhu, Z., Wu, S., and Li, W. (2012). CD-HIT: accelerated for clustering the next-generation sequencing data. Bioinformatics 28, 3150–3152. doi: 10.1093/bioinformatics/bts565
Pubmed Abstract | Pubmed Full Text | CrossRef Full Text | Google Scholar
Grywalska, E., Markowicz, J., Grabarczyk, P., Pasiarski, M., and RoliÅĎski, J. (2013). Epstein-Barr virus-associated lymphoproliferative disorders. Postepy Hig. Med. Dosw. 67, 481–490. doi: 10.5604/17322693.1050999
Pubmed Abstract | Pubmed Full Text | CrossRef Full Text | Google Scholar
Head, S. R., Komori, H. K., LaMere, S. A., Whisenant, T., Van Nieuwerburgh, F., Salomon, D. R., et al. (2014). Library construction for next-generation sequencing: overviews and challenges. Biotechniques 56, 61–64. doi: 10.2144/000114133
Pubmed Abstract | Pubmed Full Text | CrossRef Full Text | Google Scholar
Hochberg, Y. (1988). A sharper Bonferroni procedure for multiple tests of significance. Biometrika 75, 800–802. doi: 10.1093/biomet/75.4.800
Hoelzer, K., Shackelton, L. A., and Parrish, C. R. (2008). Presence and role of cytosine methylation in DNA viruses of animals. Nucleic Acids Res. 36, 2825–2837. doi: 10.1093/nar/gkn121
Pubmed Abstract | Pubmed Full Text | CrossRef Full Text | Google Scholar
Hohn, O., Hanke, K., and Bannert, N. (2013). HERV-K(HML-2), the Best preserved family of HERVs: endogenization, expression, and implications in health and disease. Front. Oncol. 3:246. doi: 10.3389/fonc.2013.00246
Pubmed Abstract | Pubmed Full Text | CrossRef Full Text | Google Scholar
Hong, K., Sherley, J., and Lauffenburger, D. A. (2001). Methylation of episomal plasmids as a barrier to transient gene expression via a synthetic delivery vector. Biomol. Eng. 18, 185–192. doi: 10.1016/S1389-0344(01)00100-9
Pubmed Abstract | Pubmed Full Text | CrossRef Full Text | Google Scholar
Jacobs, M. V., Snijders, P. J., van den Brule, A. J., Helmerhorst, T. J., Meijer, C. J., and Walboomers, J. M. (1997). A general primer GP5+/GP6(+)-mediated PCR-enzyme immunoassay method for rapid detection of 14 high-risk and 6 low-risk human papillomavirus genotypes in cervical scrapings. J. Clin. Microbiol. 35, 791–795.
John, S. G., Mendez, C. B., Deng, L., Poulos, B., Kauffman, A. K. M., Kern, S., et al. (2011). A simple and efficient method for concentration of ocean viruses by chemical flocculation. Environ. Microbiol. Rep. 3, 195–202. doi: 10.1111/j.1758-2229.2010.00208.x
Pubmed Abstract | Pubmed Full Text | CrossRef Full Text | Google Scholar
Jones, M. S., Harrach, B., Ganac, R. D., Gozum, M. M. A., Dela Cruz, W. P., Riedel, B., et al. (2007). New adenovirus species found in a patient presenting with gastroenteritis. J. Virol. 81, 5978–5984. doi: 10.1128/JVI.02650-06
Pubmed Abstract | Pubmed Full Text | CrossRef Full Text | Google Scholar
Jones, P. A. (2012). Functions of DNA methylation: islands, start sites, gene bodies and beyond. Nat. Rev. Genet. 13, 484–492. doi: 10.1038/nrg3230
Pubmed Abstract | Pubmed Full Text | CrossRef Full Text | Google Scholar
Khoury, J. D., Tannir, N. M., Williams, M. D., Chen, Y., Yao, H., Zhang, J., et al. (2013). Landscape of DNA virus associations across human malignant cancers: analysis of 3,775 cases using RNA-Seq. J. Virol. 87, 8916–8926. doi: 10.1128/JVI.00340-13
Pubmed Abstract | Pubmed Full Text | CrossRef Full Text | Google Scholar
Kim, M., Gans, J. D., Nogueira, C., Wang, A., Paik, J.-H., Feng, B., et al. (2006). Comparative oncogenomics identifies NEDD9 as a melanoma metastasis gene. Cell 125, 1269–1281. doi: 10.1016/j.cell.2006.06.008
Pubmed Abstract | Pubmed Full Text | CrossRef Full Text | Google Scholar
Kircher, M., Sawyer, S., and Meyer, M. (2012). Double indexing overcomes inaccuracies in multiplex sequencing on the Illumina platform. Nucleic Acids Res. 40, e3. doi: 10.1093/nar/gkr771
Pubmed Abstract | Pubmed Full Text | CrossRef Full Text | Google Scholar
Krüger, D. H., and Bickle, T. A. (1983). Bacteriophage survival: multiple mechanisms for avoiding the deoxyribonucleic acid restriction systems of their hosts. Microbiol. Rev. 47, 345–360.
Langmead, B., Trapnell, C., Pop, M., and Salzberg, S. L. (2009). Ultrafast and memory-efficient alignment of short DNA sequences to the human genome. Genome Biol. 10:R25. doi: 10.1186/gb-2009-10-3-r25
Pubmed Abstract | Pubmed Full Text | CrossRef Full Text | Google Scholar
Leinonen, R., Akhtar, R., Birney, E., Bower, L., Cerdeno-Tárraga, A., Cheng, Y., et al. (2011). The European nucleotide archive. Nucleic Acids Res. 39, D28–D31. doi: 10.1093/nar/gkq967
Pubmed Abstract | Pubmed Full Text | CrossRef Full Text | Google Scholar
Li, J., Huang, Q., Zeng, F., Li, W., He, Z., Chen, W., et al. (2014). The prognostic value of global DNA hypomethylation in cancer: a meta-analysis. PLoS ONE 9:e106290. doi: 10.1371/journal.pone.0106290
Pubmed Abstract | Pubmed Full Text | CrossRef Full Text | Google Scholar
Lysholm, F., Wetterbom, A., Lindau, C., Darban, H., Bjerkner, A., Fahlander, K., et al. (2012). Characterization of the viral microbiome in patients with severe lower respiratory tract infections, using metagenomic sequencing. PLoS ONE 7:e30875. doi: 10.1371/journal.pone.0030875
Pubmed Abstract | Pubmed Full Text | CrossRef Full Text | Google Scholar
Marongiu, L., Godi, A., Parry, J. V., and Beddows, S. (2014). Human Papillomavirus 16, 18, 31 and 45 viral load, integration and methylation status stratified by cervical disease stage. BMC Cancer 14:384. doi: 10.1186/1471-2407-14-384
Pubmed Abstract | Pubmed Full Text | CrossRef Full Text | Google Scholar
Martin, D. H., Zozaya, M., Lillis, R., Miller, J., and Ferris, M. J. (2012). The microbiota of the human genitourinary tract: trying to see the forest through the trees. Trans. Am. Clin. Climatol. Assoc. 123, 242–256.
Niu, B., Zhu, Z., Fu, L., Wu, S., and Li, W. (2011). FR-HIT, a very fast program to recruit metagenomic reads to homologous reference genomes. Bioinformatics 27, 1704–1705. doi: 10.1093/bioinformatics/btr252
Pubmed Abstract | Pubmed Full Text | CrossRef Full Text | Google Scholar
Paulson, E. J., and Speck, S. H. (1999). Differential methylation of epstein-barr virus latency promoters facilitates viral persistence in healthy seropositive individuals. J. Virol. 73, 9959–9968.
Poreba, E., Broniarczyk, J. K., and Gozdzicka-Jozefiak, A. (2011). Epigenetic mechanisms in virus-induced tumorigenesis. Clin. Epigenetics 2, 233–247. doi: 10.1007/s13148-011-0026-6
Pubmed Abstract | Pubmed Full Text | CrossRef Full Text | Google Scholar
R Core Team (2012). R: A Language and Environment for Statistical Computing. Vienna: R Foundation for Statistical Computing.
Salyakina, D., and Tsinoremas, N. F. (2013). Viral expression associated with gastrointestinal adenocarcinomas in TCGA high-throughput sequencing data. Hum. Genomics 7:23. doi: 10.1186/1479-7364-7-23
Pubmed Abstract | Pubmed Full Text | CrossRef Full Text | Google Scholar
Schiller, J. T., and Lowy, D. R. (2010). Vaccines to prevent infections by oncoviruses. Annu. Rev. Microbiol. 64, 23–41. doi: 10.1146/annurev.micro.112408.134019
Pubmed Abstract | Pubmed Full Text | CrossRef Full Text | Google Scholar
Schmieder, R., and Edwards, R. (2011). Quality control and preprocessing of metagenomic datasets. Bioinformatics 27, 863–864. doi: 10.1093/bioinformatics/btr026
Pubmed Abstract | Pubmed Full Text | CrossRef Full Text | Google Scholar
Serre, D., Lee, B. H., and Ting, A. H. (2010). MBD-isolated genome sequencing provides a high-throughput and comprehensive survey of DNA methylation in the human genome. Nucleic Acids Res. 38, 391–399. doi: 10.1093/nar/gkp992
Pubmed Abstract | Pubmed Full Text | CrossRef Full Text | Google Scholar
Smiraglia, D. J. (2001). Excessive CpG island hypermethylation in cancer cell lines versus primary human malignancies. Hum. Mol. Genet. 10, 1413–1419. doi: 10.1093/hmg/10.13.1413
Pubmed Abstract | Pubmed Full Text | CrossRef Full Text | Google Scholar
Steenbergen, R. D., Walboomers, J. M., Meijer, C. J., van der Raaij-Helmer, E. M., Parker, J. N., Chow, L. T., et al. (1996). Transition of human papillomavirus type 16 and 18 transfected human foreskin keratinocytes towards immortality: activation of telomerase and allele losses at 3p, 10p, 11q and/or 18q. Oncogene 13, 1249–1257.
Steenbergen, R. D. M., Ongenaert, M., Snellenberg, S., Trooskens, G., van der Meide, W. F., Pandey, D., et al. (2013). Methylation-specific digital karyotyping of HPV16E6E7-expressing human keratinocytes identifies novel methylation events in cervical carcinogenesis. J. Pathol. 231, 53–62. doi: 10.1002/path.4210
Pubmed Abstract | Pubmed Full Text | CrossRef Full Text | Google Scholar
Steenbergen, R. D. M., Snijders, P. J. F., Heideman, D. A. M., and Meijer, C. J. L. M. (2014). Clinical implications of (epi)genetic changes in HPV-induced cervical precancerous lesions. Nat. Rev. Cancer 14, 395–405. doi: 10.1038/nrc3728
Pubmed Abstract | Pubmed Full Text | CrossRef Full Text | Google Scholar
Szostek, S., Zawilinska, B., Kopec, J., and Kosz-Vnenchak, M. (2009). Herpesviruses as possible cofactors in HPV-16-related oncogenesis. Acta Biochim. Pol. 56, 337–342.
Tjon Pian Gi, R. E. A., San Giorgi, M. R. M., Slagter-Menkema, L., van Hemel, B. M., van der Laan, B. F. A. M., van den Heuvel, E. R., et al. (2014). The clinical course of recurrent respiratory papillomatosis: a comparison between aggressiveness of HPV6 and HPV11. Head Neck. doi: 10.1002/hed.23808. [Epub ahead of print].
van den Brule, A. J. C., Pol, R., Fransen-Daalmeijer, N., Schouls, L. M., Meijer, C. J. L. M., and Snijders, P. J. F. (2002). GP5+/6+ PCR followed by reverse line blot analysis enables rapid and high-throughput identification of human papillomavirus genotypes. J. Clin. Microbiol. 40, 779–787. doi: 10.1128/JCM.40.3.779-787.2002
Pubmed Abstract | Pubmed Full Text | CrossRef Full Text | Google Scholar
Varley, K. E., Gertz, J., Bowling, K. M., Parker, S. L., Reddy, T. E., Pauli-Behn, F., et al. (2013). Dynamic DNA methylation across diverse human cell lines and tissues. Genome Res. 23, 555–567. doi: 10.1101/gr.147942.112
Pubmed Abstract | Pubmed Full Text | CrossRef Full Text | Google Scholar
Walboomers, J. M., Jacobs, M. V., Manos, M. M., Bosch, F. X., Kummer, J. A., Shah, K. V., et al. (1999). Human papillomavirus is a necessary cause of invasive cervical cancer worldwide. J. Pathol. 189, 12–19. doi: 10.1002/(SICI)1096-9896(199909)189:1<12::AID-PATH431>3.0.CO;2-F
Pubmed Abstract | Pubmed Full Text | CrossRef Full Text | Google Scholar
Wheeler, D. L., Barrett, T., Benson, D. A., Bryant, S. H., Canese, K., Chetvernin, V., et al. (2006). Database resources of the national center for biotechnology information. Nucleic Acids Res. 34, D173–D180. doi: 10.1093/nar/gkj158
Pubmed Abstract | Pubmed Full Text | CrossRef Full Text | Google Scholar
Willner, D., and Hugenholtz, P. (2013). From deep sequencing to viral tagging: recent advances in viral metagenomics. Bioessays 35, 436–442. doi: 10.1002/bies.201200174
Pubmed Abstract | Pubmed Full Text | CrossRef Full Text | Google Scholar
Wisman, G. B. A., Nijhuis, E. R., Hoque, M. O., Reesink-Peters, N., Koning, A. J., Volders, H. H., et al. (2006). Assessment of gene promoter hypermethylation for detection of cervical neoplasia. Int. J. Cancer 119, 1908–1914. doi: 10.1002/ijc.22060
Pubmed Abstract | Pubmed Full Text | CrossRef Full Text | Google Scholar
Yozwiak, N. L., Skewes-Cox, P., Stenglein, M. D., Balmaseda, A., Harris, E., and DeRisi, J. L. (2012). Virus identification in unknown tropical febrile illness cases using deep sequencing. PLoS Negl. Trop. Dis. 6:e1485. doi: 10.1371/journal.pntd.0001485
Pubmed Abstract | Pubmed Full Text | CrossRef Full Text | Google Scholar
Keywords: viruses, epigenomics, DNA-methylation, next generation sequencing, bioinformatics, cervical cancer, human papillomavirus, MBD-seq
Citation: Mensaert K, Van Criekinge W, Thas O, Schuuring E, Steenbergen RDM, Wisman GBA and De Meyer T (2015) Mining for viral fragments in methylation enriched sequencing data. Front. Genet. 6:16. doi: 10.3389/fgene.2015.00016
Received: 30 June 2014; Accepted: 13 January 2015;
Published online: 04 February 2015.
Edited by:
Mark D. Robinson, University of Zurich, SwitzerlandReviewed by:
Ping Ma, University of Georgia, USAMattia Pelizzola, Istituto Italiano di Tecnologia, Italy
Copyright © 2015 Mensaert, Van Criekinge, Thas, Schuuring, Steenbergen, Wisman and De Meyer. This is an open-access article distributed under the terms of the Creative Commons Attribution License (CC BY). The use, distribution or reproduction in other forums is permitted, provided the original author(s) or licensor are credited and that the original publication in this journal is cited, in accordance with accepted academic practice. No use, distribution or reproduction is permitted which does not comply with these terms.
*Correspondence: Tim De Meyer, BioBix, Department of Mathematical Modeling, Statistics and Bioinformatics, Ghent University, Coupure Links 653, Ghent 9000, Belgium e-mail:dGltLmRlbWV5ZXJAdWdlbnQuYmU=