- 1Animal Breeding and Genomics, Wageningen University and Research, Wageningen, Netherlands
- 2National Agricultural Research Organization (NARO), Entebbe, Uganda
- 3Department of Animal Breeding and Genetics, Swedish University of Agricultural Sciences, Uppsala, Sweden
- 4Department of Animal Biosciences, Centre for Genetic Improvement of Livestock (CGIL), University of Guelph, Guelph, ON, Canada
Both natural and artificial selection are among the main driving forces shaping genetic variation across the genome of livestock species. Selection typically leaves signatures in the genome, which are often characterized by high genetic differentiation across breeds and/or a strong reduction in genetic diversity in regions associated with traits under intense selection pressure. In this study, we evaluated selection signatures and genomic inbreeding coefficients, FROH, based on runs of homozygosity (ROH), in six Ugandan goat breeds: Boer (n = 13), and the indigenous breeds Karamojong (n = 15), Kigezi (n = 29), Mubende (n = 29), Small East African (n = 29), and Sebei (n = 29). After genotyping quality control, 45,294 autosomal single nucleotide polymorphisms (SNPs) remained for further analyses. A total of 394 and 6 breed-specific putative selection signatures were identified across all breeds, based on marker-specific fixation index (FST-values) and haplotype differentiation (hapFLK), respectively. These regions were enriched with genes involved in signaling pathways associated directly or indirectly with environmental adaptation, such as immune response (e.g., IL10RB and IL23A), growth and fatty acid composition (e.g., FGF9 and IGF1), and thermo-tolerance (e.g., MTOR and MAPK3). The study revealed little overlap between breeds in genomic regions under selection and generally did not display the typical classic selection signatures as expected due to the complex nature of the traits. In the Boer breed, candidate genes associated with production traits, such as body size and growth (e.g., GJB2 and GJA3) were also identified. Furthermore, analysis of ROH in indigenous goat breeds showed very low levels of genomic inbreeding (with the mean FROH per breed ranging from 0.8% to 2.4%), as compared to higher inbreeding in Boer (mean FROH = 13.8%). Short ROH were more frequent than long ROH, except in Karamojong, providing insight in the developmental history of these goat breeds. This study provides insights into the effects of long-term selection in Boer and indigenous Ugandan goat breeds, which are relevant for implementation of breeding programs and conservation of genetic resources, as well as their sustainable use and management.
Introduction
Goats are among the most important livestock species in developing countries, such as Uganda, playing a significant socio-economic, nutritional and cultural role in smallholder production systems (MAAIF, 2011). The total goat population in Uganda is estimated to consist of over14 million animals, predominantly from indigenous breeds (98%) and a small proportion (2%) from exotic breeds (MAAIF and UBOS, 2009; UBOS, 2015). Exotic breeds have been artificially selected for production traits over several generations, whereas indigenous breeds have undergone no or less intense artificial selection. While exotic breeds are subjected to more intense artificial selection, it is expected that the effect of natural selection (i.e., adaptation to the specific environment) is more apparent in the indigenous breeds and has played an important role in their development. Based on this hypothesis, it is expected that indigenous breeds will tend to exhibit resistance to gastro-intestinal parasites and local diseases, tolerance to heat, water scarcity and ability to use low quality fodder. Often high order traits like adaptation to environmental stress are influenced by several traits acting in combination. Adaptation is a complex trait that involves many biological processes and quantitative trait loci with each having a small but cumulative effect on the overall expression of the phenotype (Kim et al., 2016; Yang et al., 2016; Mwacharo et al., 2017).
Selection (both natural and artificial) is one of the main driving forces shaping genetic variation across genomes of livestock species. Under strong positive selection pressure, the frequency of favorable alleles will increase over time (Maynard and Haigh, 2007). This may result in genomic regions with high genetic differentiation across breeds and/or specific haplotypes rising to high frequencies. Such regions can thus be selection signatures. Analysis of selection signatures has the goal of identifying genomic regions or loci showing deviations from neutrality. Other forces like migration, admixture events, and population bottlenecks may have a profound effect on genomic variability, locally increasing or reducing the genetic variation.
Two well-established methods to detect selection signatures include the fixation index (FST) (Wright, 1949; Weir and Cockerham, 1984; Porto-Neto et al., 2013) and haplotype differentiation statistic – hapFLK (Fariello et al., 2013). FST is one of the most popular methods to detect selection signatures if data is available for multiple populations. The FST-approach measures population differentiation due to locus-specific allele frequencies between populations and can detect highly differentiated alleles undergoing divergent selection among populations (McRae et al., 2014; Zhao et al., 2015). A drawback of the approach is that the FST-statistic assumes that all populations are of similar effective population size and are derived independently from the same ancestral population. The hapFLK - statistic measures differences of haplotype frequencies between populations and accounts for the hierarchical structure of the populations (Fariello et al., 2013). The use of a combination of haplotype information and of the hierarchical structure of populations results in greater power for the detection of selection signatures.
Selection signature analyses using genome-wide SNPs have been widely applied in exploring the genomes of livestock species such as sheep (Kijas et al., 2012; Purfield et al., 2017; Rochus et al., 2018), cattle (Porto-Neto et al., 2014; Zhao et al., 2015; Taye et al., 2017), and goats (Burren et al., 2016; Kim et al., 2016; Brito et al., 2017). These studies have identified genes associated with a variety of traits including thermo-tolerance, immune response, reproduction functions, skin and hair structure, feed intake, and metabolism.
Ugandan indigenous goat breeds can be phenotypically categorized within three main breeds: Kigezi, Mubende, and Small East African (Mason and Maule, 1960). Other indistinct ecotypes of indigenous goat breeds also exist including Karamojong and Sebei (Nsubuga, 1996). These breeds show high genetic diversity, but weak population sub-structuring (Onzima et al., 2018). The result of the weak population structure is low levels of inbreeding and some of the breeds having similar selection signatures (Msalya et al., 2017). The indigenous breeds present a high degree of adaptation to parasites and heat tolerance, and survive on poor quality fodder, while also maintaining good reproductive rates (Mwacharo et al., 2017). However, production levels are much lower compared to specialized breeds. Therefore, Boer goats were introduced in Uganda in the early 1990s to genetically improve the growth rate and body size of the indigenous breeds (Nsubuga, 1996). Because of community-based small ruminant breeding programs and the use of limited Boer breeding males for cross breeding, the increase in inbreeding levels is a major concern to the industry.
The increase in inbreeding in livestock at a genomic level over generations leads to a reduction in genetic diversity. When an offspring is inbred, it may inherit autozygous chromosomal segments from both parents that are identical by descent (IBD), i.e., segments that are derived from a common ancestor (Broman and Weber, 1999). The result is continuous homozygous segments in the genome, also known as runs of homozygosity (ROH). The extent of ROH can be used to estimate the inbreeding coefficient (Bosse et al., 2012; Marras et al., 2015; Peripolli et al., 2018). ROH can be used to disclose the genetic relationships among individuals, usually estimating with high accuracy the autozygosity at an individual and/or population levels (Ferenčaković et al., 2011, 2013a). It can also be used to establish the level of selection pressure on the populations (Zhang et al., 2015). Length and frequency of ROHs may also be used to distinguish distant from more recent inbreeding, since the length of IBD segments follows an inverse exponential distribution with a mean of 1/2 g Morgans, where g is the number of generations from a common ancestor (Howrigan et al., 2011).
The objectives of this study were to: (1) identify unique selection signatures in the genome and the genes under selection in Ugandan goat breeds, and (2) assess the occurrence and distribution of ROH and ROH-based genomic inbreeding in Ugandan goat breeds.
Materials and Methods
Animals and Genotype Quality Control
The data used in this study were derived from 144 animals from 6 goat populations and has been described in detail previously (Onzima et al., 2018). The animals were from the five indigenous breeds, Mubende (n = 29), Kigezi (n = 29), Small East African (n = 29), Karamojong (n = 15) and Sebei (n = 29), and from the exotic Boer breed (n = 13). All animals were genotyped with the Illumina GoatSNP50 BeadChip (Tosser-Klopp et al., 2014), which features 53,347 single nucleotide polymorphisms (SNPs). Genotype quality control (QC) procedures were performed using PLINK v1.90 (Chang et al., 2015). All samples passed the quality criteria (missing genotype call rate ≥ 0.1) and were used in the analysis. The SNPs with a call rate below 0.95, a minor allele frequency (MAF) lower than 0.05, located on non-autosomal chromosomes, or not in Hardy Weinberg Equilibrium (at p < 0.001) were discarded. After QC procedures, 46,105 autosomal SNPs remained. For these SNPs, the position on the genome was obtained from the goat reference genome assembly ARS1 release 102 (Bickhart et al., 2017). After removing SNPs with unknown position on the ARS1 genome assembly, 45,294 autosomal SNPs from 144 goats remained in the final dataset.
Relatedness Within and Between Breeds
The level of relatedness between individuals (both within and between breeds) was determined using genomic similarities. For each pair of individuals, the genomic similarity (SIMSNPij) was determined according to Malécot (1948):
where nSNP is the total number of markers and Ixy,k is an indicator variable that was set to 1 when allele x of individual i and allele y of individual j at marker k were identical by state (IBS), and to 0 otherwise. Note that, as self-similarities were included, the average similarity in a breed was equivalent to the expected homozygosity in that breed.
Identifying Selection Signatures
To increase the likelihood to detect true selection signatures (i.e., no false positive results), multiple approaches can be used (Simianer, 2014). The methods adapted for analysis of selection signatures need to be robust enough to disentangle selective pressures from other effects on the population such as migration, admixture and population bottlenecks. In this study, we used allele specific population differentiation defined as FST (Wright, 1949) and a haplotype-based differentiation approach, hapFLK (Fariello et al., 2013), which accounts for haplotype structure of populations and for variable effective population sizes.
Fixation Index (FST)
Selection signatures for each breed were identified using an FST-statistic per SNP that compares the allele frequency in the breed to the allele frequency in a combined population of the remaining breeds, following the unbiased estimator proposed by Weir and Cockerham (1984) and implemented in PLINK (Chang et al., 2015). For example, differences between the exotic Boer breed on the one hand, and the indigenous goat breeds on the other, were investigated by calculating the FST-values between Boer and a combined population of all the indigenous breeds. We also computed FST-values for the indigenous breeds while excluding Boer from the analysis. However, as the exclusion of Boer did not influence the results for the indigenous breeds, only results with Boer included are reported in the subsequent sections.
In general, genomic regions showing high FST-values with moving averages (mas) and single SNPs indicate strong breed differentiation or selection, while low FST-values suggest no or a limited amount of population differentiation. Negative FST-values were set to zero, as they imply no genetic differentiation between the two groups.
To visualize and infer region-specific differences over the erratic pattern of individual SNPs, we computed a ma of FST (maFST) values for 5 adjacent SNPs. The maFST was computed for 5 adjacent SNPs and plotted against the chromosomal position for all goat autosomes (CHI coordinates). The SNPs with a maFST above the 95% quantile of the empirical distribution of raw FST-values were considered as putative selection signatures. The ma is a simple approach for identifying regions of interest in the genome from the erratic pattern of SNPs. This approach has been implemented successfully in analyzing systematic differences in response to genetic variation to pedigree and genome-based selection methods in chicken (Heidaritabar et al., 2014) and genome-wide genetic diversity in Dutch dairy cattle (Doekes et al., 2018). By using maFST, rather than FST for single SNPs, we aimed to reduce the influence of the small sample sizes on the results.
Haplotype Differentiation (hapFLK)
To account for haplotype structure and varying effective population sizes, we used hapFLK (Fariello et al., 2013) to detect potential selection signatures in the six goat populations. The used procedure has been described in detail by Brito et al., (2017). Briefly, hapFLK was applied to the unphased genotype data to identify putative regions under selection, by estimating the neighbor joining tree and a kinship relationship matrix based on Reynolds’ genetic distances between the breeds. The pairwise Reynolds’ distances (Reynolds et al., 1983) between populations (including an outgroup) are computed for each SNP and averaged over the genome. Using the genotype data and kinship matrix, and assuming 6 clusters in the fastPHASE model (-k, 6), the program was run and the hapFLK statistic was computed as an average of 20 expectation maximization iterations to fit the Linkage Disequilibrium (LD) model. With hapFLK values generated for each SNP, p-values were computed based on a chi-square distribution of the numerical values. The mean and variance of hapFLK distribution were estimated and used to standardize each SNP specific value. This was subsequently followed by computation of p-values from a standard normal distribution, and the (-log10) of p-values was plotted against the genomic positions. To minimize the number of false positives, a q-value threshold of 0.01 was set to control the false discovery rate (FDR) at the 1% level. Putative selective signatures were defined by the regions with a threshold of p < 0.005.
Identification of Candidate Genes Associated With Selection Signatures
Genes within putative selection signature regions were retrieved from NCBI1, using the goat reference assembly ARS1. The genes overlapping either partially or fully within the 95% threshold of the empirical distribution of the raw FST -values and within the regions with p < 0.005 for hapFLK, were putative selection candidate genes.
For each of the breeds, gene enrichment analyses were performed based on the FST, and hapFLK results with the web-based tool, Database for Annotation, Visualization, and Integrated Discovery (DAVID) v6.8 (Huang et al., 2009; Jiao et al., 2012), which allows for the investigation of the Kyoto Encyclopedia of Genes and Genomes (KEGG) pathways (Kanehisa et al., 2012) and Gene Ontology (GO) for biological processes (Ashburner et al., 2000). Fisher’s exact test (p-value = 0.05), was applied to identify significantly enriched GO biological and functional processes. More stringent settings, such as Bonferroni correction, FDR, and Fold enrichment test were not considered in the detection given the limited scope of the study. Human gene ontologies were used since the goat genome has not been properly annotated. Moreover, the human genome is highly annotated than closely related species like bovine; thereby increasing the probability of retrieving GO terms in the goat genome. Phenotypes known to be affected by the identified candidate genes were compared from literature and using the AnimalQTLdb at: https://www.animalgenome.org/cgi-bin/QTLdb/index.
Runs of Homozygosity (ROH) and Genomic Inbreeding (FROH)
For each individual, ROHs were identified using an in-house script which incorporates a set of criteria for defining regions of homozygosity.
An ROH was called if the following criteria were fulfilled: (1) 20 or more consecutive SNPs were homozygous, (2) a minimum physical length of 2 Mb, (3) a maximum gap between two consecutive SNPs of 500 Kb, and (4) maximum of 2 missing genotypes and no heterozygous calls within ROH. The rather stringent criteria were used to minimize incorrect discovery of ROH (false positives) within regions of low marker density. The minimum expected length of homozygous DNA segments was based on the time frame of approximately 25 generations, over which goats are believed to have been characterized in separate breeds in Uganda (Mason and Maule, 1960). The length of ROH derived from a common ancestor g generations ago follows an inverse exponential distribution with the mean equal to 100/2 g cM (Fisher, 1954; Thompson, 2013). A genetic distance of approximately 1 cM per Mb is often assumed in cattle (Arias et al., 2009) and assuming a similar relationship for goats, the mean length of ROH derived from common ancestor from 25 generations ago would be 2 Mb.
The proportion of ROH per animal in comparison to the whole genome SNP coverage provides a useful indication of the level of inbreeding. Genomic inbreeding coefficient based on ROHs were computed as the length of the autosome covered by ROHs divided by the overall length of the autosome covered by the SNPs (McQuillan et al., 2008):
where LROH is the sum of the total length of ROH in individual i and LAUTO is the total length of the autosomes covered by the SNPs (2.463 Gb). The number of ROHs and FROH were also evaluated for different ROH length categories. We focused on length classes from 2 to 16 Mb to investigate more ancient inbreeding (2 and 16 Mb are the expected lengths of ROH derived from common ancestors 25 and 3 generations ago, respectively) and >16 Mb to assess more recent inbreeding (expected length of ROH derived from ancestors ≤ 3 generations ago).
The ROH were estimated in each individual separately and then classified into four length categories: 2–4 Mb, 4–8 Mb, 8–16 Mb, and >16 Mb, following classification used in similar studies (Kirin et al., 2010; Ferenčaković et al., 2013a; Marras et al., 2015), specified from now on as ROH2-4 Mb, ROH4-8 Mb, ROH8-16 Mb, and ROH> 16 Mb, respectively. For each length category in each of the individuals of each breed, we computed the total number of ROH identified – nROH, mean sum of ROH coverage – SROH in Mb (defined by sum of all ROH per individual divided by the number of animals per breed) and average length of ROH (LROH, Mb).
Results
Relatedness Among Ugandan Goats
Mean genomic similarities within and between breeds are shown in Table 1. As expected, within breed similarities (diagonal) were higher than between breed similarities (off-diagonal). Within breeds, the highest mean similarity was found in Kigezi (0.643) and the lowest in Sebei (0.623). Between breeds, the indigenous Ugandan goat breeds showed higher genomic similarity with each other than the Boer breed.
Selection Signatures
Selection Signatures – FST
There was generally a high level of differentiation between Boer on the one hand, and the indigenous Ugandan breeds on the other. For Boer, the average FST across all SNPs was 0.123, while the average FST for the indigenous breeds was less than 0.050 (Supplementary Table S1).
Analysis of breed specific differentiation between each of the Ugandan goat breeds including the Boer breed resulted in several putative regions of selection as shown in Supplementary Table S2 (p < 0.05, without Bonferroni correction). In Boer, the 29 putative regions of selection were identified, which spread across 17 autosomes and overlapped with 134 genes. The regions with the highest degree of differentiation were found on CHI11, 12, 14, 18, and 24 (Figure 1). The highest ranked SNP window (maFST-value = 0.754) was found on CHI12 in a genomic region between 60.170 and 60.711 Mb and overlap with portions of the genes MAB21L1 and NBEA. Analysis of breed specific differentiation for the Ugandan indigenous goat breeds resulted in 394 putative regions of selection distributed across all the breeds showing some candidate genes for traits of economic importance. The regions varied from 66 in Mubende to 79 in the Small East African goats distributed across most of the autosomes (Supplementary Table S2). The selection signature regions in the indigenous goat breeds are on average numerous and shorter than in the Boer. There is limited overlap between the different breeds indicating signatures are mostly breed specific. Several genes were found spanning the selection signature regions across the autosomes (Supplementary Table S2). Functional analysis of some of the candidate genes (Supplementary Figure S1) shows they may be involved in tropical adaptation such as thermo-tolerance and immune response in the indigenous breeds. These include KPNA4 (CHI1), MTOR (CHI16), SH2B1 (CHI25), and MAPK3 (CHI25) in Karamojong; IL10RB, IFNAR, DNAJC13 (CHI1) in Kigezi; PPP1R36 and HSPA2 (CHI10), DNAJC24 (CHI5) in Mubende; CD80, ADPRH, IGSF11 (CHI1), IGF1 (CHI5) in Small East African goats, and HOXC12 and HOXC13 (CHI5) in Sebei. The full gene-list is found in Supplementary Table S2.
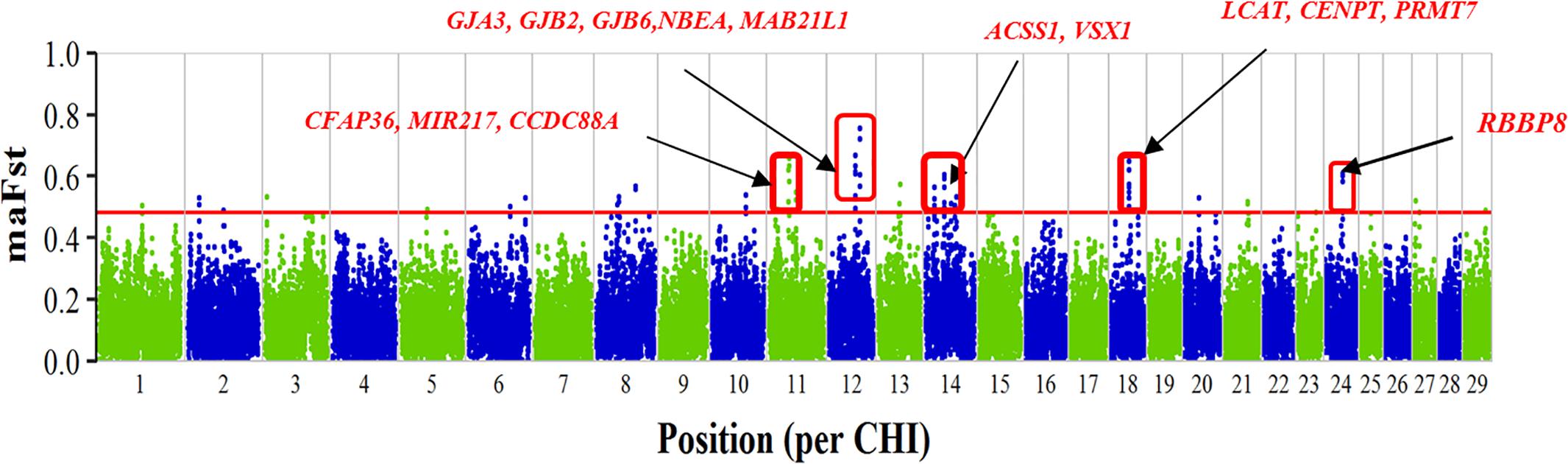
FIGURE 1. Plot of moving average FST-values of SNPs per chromosome. The plot shows comparison of Boer goats with other Ugandan goat breeds and candidate genes in putative selective signatures are shown in red. The horizontal red line (at 0.482) represents the 95%-percentile of raw FST-values.
Selection Signatures – hapFLK
The results of the haplotype-based differentiation with hapFLK are shown in Figure 2. A significance threshold of p < 0.005 was considered to identify regions under selection. The hapFLK analysis resulted in six putative selection signature regions on CHI5 (116.662–118.773 Mb), CHI6 (0.005–16.337 Mb), CHI8 (7.766–7.941 Mb), CHI13 (58.709–63.989 Mb), CHI15 (14.932–23.571 Mb), and CHI16 (40.533–45.988 Mb). Some of the candidate genes identified, which may be playing a role in tropical adaption include; CFI (CHI 6), DEFB genes (CHI 13), and ASIP (CHI 13), MTOR, PIK3CD (CHI 16), and CD44 (CHI 15) (Supplementary Table S3).
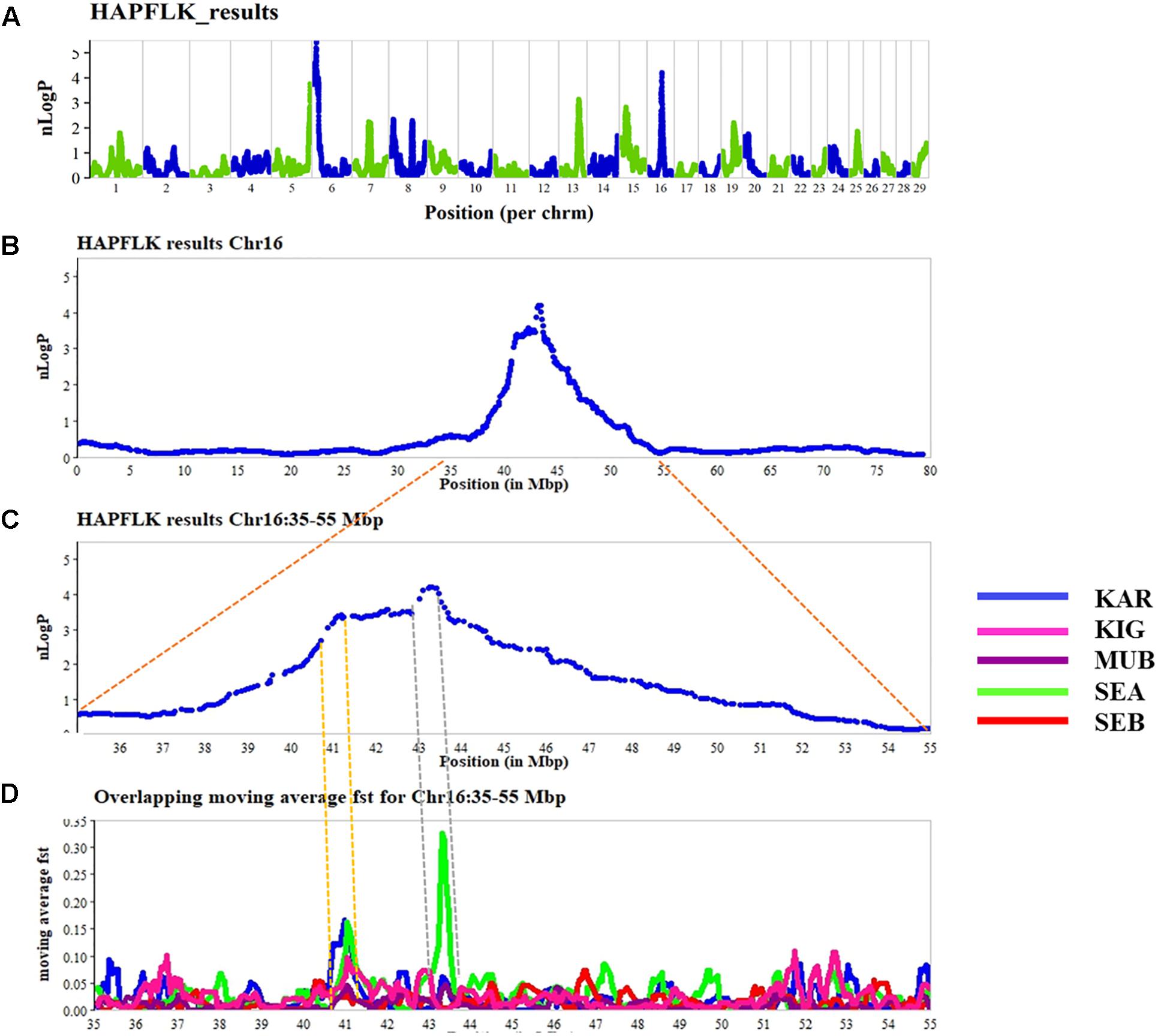
FIGURE 2. Genome-wide scan for putative signatures of selection using the hapFLK (A). The negative log of p-values is plotted in chromosomal order. The alternating colors green and blue represent the different chromosomes (on the upper panel). On the lower panels, (B–D) is a zoom into the chromosome 16 to show the regions overlapping with high FST and hapFLK values, defining putative selection signatures over four selected regions in the different breeds (KAR = Karamojong, KIG = Kigezi, MUB = Mubende, SEA = Small East African, and SEB = Sebei).
Four of the six significant regions identified by hapFLK partially overlapped with the 394 significant selective signature regions identified by FST. Several short overlapping regions were found between hapFLK and FST, with the strongest signals detected on CHI 6, 13, and 16 (Figure 2). Some of the regions were breed specific and contained several genes (Supplementary Table S4).
Gene Enrichment of Putative Selection Signatures
Within the putative selection regions identified, a list of genes was identified for each of the approaches used: FST (Supplementary Table S2) and hapFLK (Supplementary Table S3) and were used to perform separate functional analyses using DAVID with default settings on the human gene set (Huang et al., 2009; Jiao et al., 2012). Functional analysis of the FST gene-list for each of the breeds yielded 47 significant (p < 0.05) gene ontology (GO) biological process (BP) terms (Supplementary Table S5) and 15 KEGG pathways were enriched (Supplementary Table S6). The biological processes enriched were related to cell communication, male sex differentiation, microtubule cytoskeleton organization in the Boer; and negative regulation of catalytic activity, homeostasis in number of cells within the tissue, TIR-domain-containing adapter-inducing interferon-β (TRIF)-dependent toll-like receptor signaling pathway (GO:0035666), positive regulation of peptidyl-tyrosine phosphorylation (GO:0050731), cytokinesis (GO:0000910), and angiogenesis (GO:0001525) among others in the indigenous goat breeds.
The DAVID analyses based on hapFLK gene list across the breeds resulted in 18 significant (p < 0.05) biological processes (Supplementary Table S5) and nine significant (p < 0.05) KEGG pathways (Supplementary Table S6). The genes identified, were significantly involved in the defense response to bacterium (GO:0042742; p-value < 0.001), negative regulation of the apoptotic process (GO:0043066; p-value < 0.001), and positive regulation of gene expression (GO:0010628; p-value < 0.001) among others.
Analysis of ROH and Genomic Inbreeding – FROH
A total of 1,497 ROHs were detected across all individuals. The frequency of ROHs and their length-distribution differed across breeds (Figure 3). For all length categories, ROHs were generally more frequent in Boer (a breed selected for meat production) than in Ugandan indigenous goat breeds. Consequently, Boer showed the highest genomic inbreeding coefficients (Table 2). For example, the mean FROH ≥2Mb in Boer was 13.8%, while for the indigenous breeds, it ranged from 0.8% (Sebei) to 2.4% (Karamojong). Shorter ROH were more frequent than longer ROH in all breeds except for Karamojong. In the later breed, there were remarkably many ROH > 16 Mb.
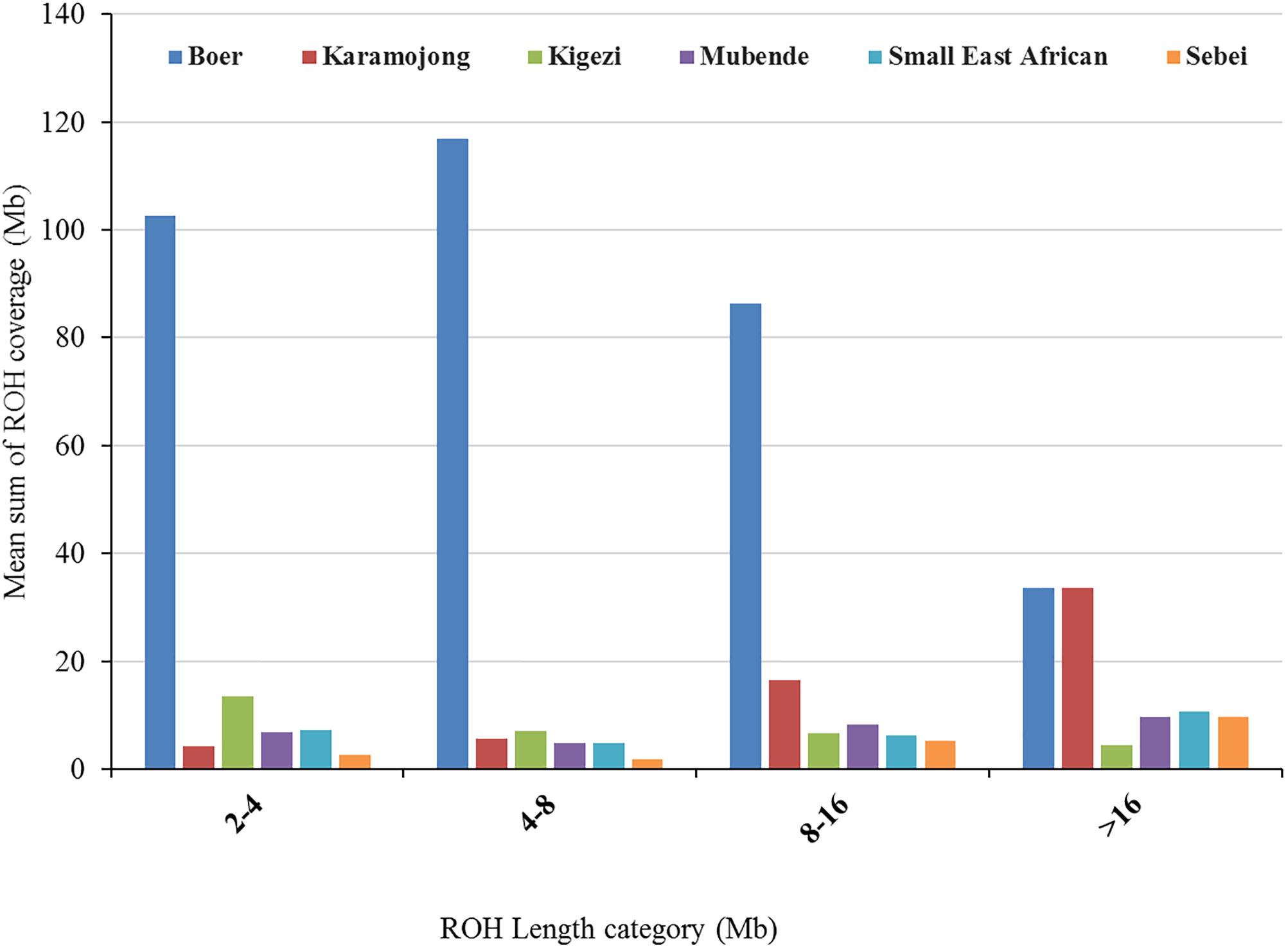
FIGURE 3. Genome-wide distribution of mean sum of ROH coverage per length category averaged per breed across six Ugandan goat breeds.
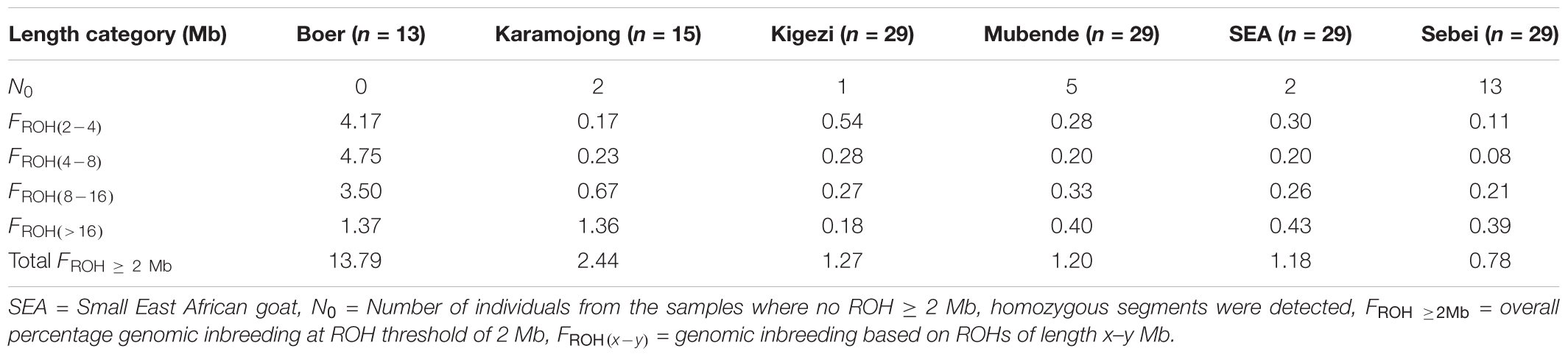
TABLE 2. Average percentage genomic inbreeding coefficient (FROH) for different length categories of ROH across six goat populations.
The ROHs were located across the whole genome, with some regions showing a higher frequency than other (Supplementary Figure S2). The mean sum of ROH segment coverage was generally higher for short ROHs than for long ROHs. The highest mean ROH coverage within the short ROH category (ROH of 2–4 Mb) was found in Boer, while Sebei had the lowest of mean ROH coverage. For instance, around 65% of the Boer mean sum of ROH segment coverage in this study (219.65 Mb) was within the shorter ROH category 2–8 Mb. However, for the other breeds, the coverage ranged from 4.52 Mb in Sebei to 20.38 Mb in Kigezi (Supplementary Table S7). In the long ROH category (ROH> 16 Mb), Boer and Karamojong showed higher ROH genome coverage (33.67 and 33.60 Mb, respectively), which indicates more recent inbreeding. In the remaining breeds, the coverage was between 4.44 Mb in Kigezi and 10.58 Mb in the Small East African breed. Boer showed high genome coverage with both short and long ROH, suggesting that the breed has experienced both recent and ancestral inbreeding compared to Ugandan indigenous breeds. The findings also suggest that among the Ugandan indigenous goat breeds, Karamojong has greater levels of inbreeding compared to the others. The proportion of the genome located on an ROH differed between breeds and chromosomes. The proportion ranged from 1.50% on CHI2 to 93.62% on CHI23 in Sebei (Supplementary Table S8). ROH segments were identified on all 29 autosomes in Boer, but the number varied in the genomes of the indigenous goat breeds with several autosomes showing no homozygous regions (Supplementary Table S9).
Discussion
In this study, we unravel selection signatures and genomic inbreeding coefficients in goat breeds of Uganda using genome-wide SNP data.
Various approaches have been implemented for the detection of selection signatures in several domestic animal species such as, cattle (Msalya et al., 2017; Taye et al., 2017), horses (Petersen et al., 2013), sheep (Kijas et al., 2012; Fariello et al., 2014; Rochus et al., 2018), and goats (Kim et al., 2016; Wang et al., 2016; Brito et al., 2017). In this study, we assessed the genome-wide differences between Ugandan indigenous goat breeds (Karamojong, Kigezi, Mubende, Small East African, and Sebei) and exotic Boer goats by using population differentiation, FST (Weir and Cockerham, 1984) and the haplotype structure in the populations, hapFLK (Fariello et al., 2013).
The statistical power to detect selection signatures may vary among the approaches. In this study, we used FST and hapFLK for detecting selection signatures. The use of different methods in detecting selection signatures boosts the accuracy of detection and eliminates unknown bias (Simianer, 2014; Ma et al., 2015).
Selection Signatures
The genomic regions potentially under selection identified in this study spanned a myriad of candidate genes with diverse biological, molecular, and cellular functions, which could be because the adaptation processes to environmental stressors is controlled by a complex network of genes acting together, other than single candidate genes. For instance, adaptation to hot and arid environments was found to be mediated by a complex network of genes in Egyptian Barki goats and sheep (Kim et al., 2016), which were directly or indirectly associated with energy and digestive metabolism, autoimmunity, thermo-tolerance (melano-genesis) and, muscular and embryonic development. Similarly, adaptation may also result from interaction of several traits under the influence of several genes (Lv et al., 2014). In this study, we found putative signatures containing a complex of genes involved directly or indirectly in immune response. Moreover, selection for complex traits may also leave limited or none of classic selection signatures due to weak selection acting on the genome (Kemper et al., 2014).
In line with expected selection signatures for such complex traits, the genomic regions identified in this study using genome-wide maker specific fixation index in the populations showed limited overlap. This suggests that the selection on genes involved in adaptation to a tropical environment were breed-specific. Moreover, the selection signatures found in our study do not display classic hard sweep characteristics, which is to be expected for complex traits. This is in contrast to the findings with Valdostana goats in Italy (Talenti et al., 2017). This may arise due to the very diverse nature of the populations and absence of hard and long selection signature regions observed within the populations at the 50K SNP marker density. Second, our study pooled genotypes from six different breeds and lending to picking out differences between the breeds, unlike in the study of Talenti et al. (2017), whose focus was on only one breed.
We did find overlap between the selected regions identified with the hapFLK method and breed-specific FST signatures. Since hapFLK considers population stratification, the haplotypes in these regions are likely to be selected for in the corresponding breeds. The fact that these regions stand out in the hapFLK results as well as the FST results suggests that selection on those regions most closely resembles classic sweeps. Strong selection signatures were observed on CHI 6, 13, and 16, and they harbored several genes which may be important for adaptation in tropical environments, such as MTOR which is involved in heat stress response and the heat shock family of genes (Shi and Manley, 2007) and DNAJC24 involved in the first apoptosis signal (FAS) pathway and regulation of stress induction of heat shock protein (hsp) in Bos taurus (Roy and Collier, 2012). Several of the genes are involved in immune response particularly the innate immune response pathway (GO:004508). Overall, several of the genes identified in this study are associated with tropical adaptation. Moreover, in the Boer, several candidate genes identified in the putative selection signatures are involved in production related traits, reflecting a more modern selection regime. However, to pin-point the exact genes involved in tropical adaptation and production in the Ugandan goat breeds, there is need for an in-depth study at high resolution.
Generally, most of the regions under selection were subtle and breed-specific, as expected for complex traits under selection. Therefore, the forces driving selection in the genome of the indigenous goat breeds in this study may be associated with adaptation to African tropical environment, such as: thermo-tolerance, disease and parasite resistance, and the ability to perform under limited (quality and quantity) feed and water resources. The genome-wide scans identified candidate genes within the putative selective signatures associated with specific biological pathways and functions, which may be shaping the genomic architecture of Ugandan goat breeds for survival in stressful environment. Although most signatures were breed-specific, some interesting similarities could be found in the adaptive processes the genes in selected regions were involved in.
Thermo-Tolerance Genes
Several candidate genes were identified, which are associated with adaptation to thermal stress. The homeobox genes HOXC12 and HOXC13 genes identified in Sebei are involved in the anterior/posterior pattern specification (GO:0009952). The genes play a role in hair follicle differentiation, growth, and development by regulating the keratin differentiation-specific genes (Wu et al., 2009; Taye et al., 2017). The HOXC13 gene has been reported to influence skin thickness. Skin thickness and number of hair follicles impacts positively on thermoregulation. For instance, in cattle, thicker skin is associated with thermo-tolerant cattle (Bos indicus) as opposed to heat susceptible cattle (Bos taurus) breeds (Alfonzo et al., 2016). Relatedly, PPP1R36 and Heat Shock Protein A2 (HSPA2) (CHI10, 26.402–26.719 Mb) identified in Mubende are involved in heat stress response and, HSPA2, DNAJC24, and DNAJC13 are associated with the heat shock family of genes (Shi and Manley, 2007). The presence of multiple genes associated with heat stress would seem to suggest that the trait is under intense selection pressure in tropically adapted breeds. Genes such as KPNA4 (CHI1), MTOR (CHI16), SH2B1 (CHI25), and MAPK3 (CHI25) were also identified in Karamojong goats. They have been reported to be involved in the FAS pathway and regulation of stress induction of hsp in Bos taurus (Roy and Collier, 2012). Furthermore, we identified the gene IGF1 (CHI5, 64.576–65.310 Mb). IGF1 encodes a protein that is similar to insulin and it is involved in regulation of carbohydrate and lipid metabolism. IGF1 facilitates post-absorptive nutrient partitioning during heat stress and accumulation of insulin is often an adaptation mechanism to heat stress (Sanz Fernandez et al., 2015).
Adaptive and Innate Immunity Genes
Several candidate genes in the putative selection regions are involved in regulating innate and adaptive immunity in mammals. For example, we identified diacylglycerol kinase beta, DGKB gene in Small East African (CHI4 position 97.794–97.991 Mb). The gene is involved in the glycerolipid, glycerophospholipid, and phosphatidylinositol metabolic pathways and has been found to be associated with QTL for strongyles that includes Haemonchus sp (Zvinorova, 2017). Other candidate genes identified include IL10RB and IFNLR1 on CHI1(0.693–0.959 Mb) in Kigezi goats. These genes are involved in type III Interferon Signaling Pathway and confer immunity (Ferrao et al., 2016). Similarly, we also identified candidate genes in Sebei such as BCL2L1 (CHI13, 60.489–60.748 Mb), and in Small East African goats such as ERBB2 (CHI19, 39.703–40.129 Mb), and ENO1 (CHI16, 43.006–43.669 Mb). These genes are directly or indirectly associated with immunoregulation, e.g., ENO1 in humans (Ryans et al., 2017).
The identification of cytokines such as IL17RE, IL17RC, and IL23A in this study may be associated with gastrointestinal parasite resistance. Some of the cytokines have been reported to be significantly upregulated in Haemonchus contortus infected sheep and are known to be involved in adaptive immune response (GO:0002250) (Guo et al., 2016). These results would suggest that immunity genes are hotspots for natural selection in Ugandan goat breeds in response to high burden of pathogen/parasite challenge in the local environment (Thumbi et al., 2014; Bahbahani and Hanotte, 2015). Indigenous goat breeds vary in the degree of response to parasite infestation (Chiejina and Behnke, 2011; Onzima et al., 2017). We hypothesize that the variation between the breeds may be due to the genes allowing for selection on resistance traits either naturally or artificially.
One of the regions in Boer on CHI3 (84.128–84.373 Mb) harbors a gene PRMT6, which is reported to influence early embryonic development in Zebra fish (Zhao et al., 2016). The gene VAV3 (CHI3, 84.730–84.962 Mb) is also associated with the immune system (Shen et al., 2017). Interleukin 12A (IL12A) gene is another cytokine that was identified on CHI1 in Karamojong which may be associated with immune response. The gene family is reported to be involved with the immune system in humans through series of biological processes (Reitberger et al., 2017). Moreover, it is cytokine that acts as a growth factor for activated T and Natural Killer (NK) cells, enhances the NK/lymphokine activated killer cells and stimulates the production of IFN-gamma.
Genes Associated With Production Traits
The candidate gene NBEA (Neurobeachin) in the region on CHI12 (maFST = 0.754) (Supplementary Table S2) is associated with human body weight (Fox et al., 2007). Another gene of interest that we identified is VAV3 (CHI3; 84.730–84.962 Mb) on a homozygous region in Boer. The gene has been identified as a candidate gene for efficiency of food conversion in swine (Wang et al., 2015) and in goats (Brito et al., 2017). These genes are particularly significant to be identified in Boer goats, which have been extensively selected for high body weight and growth rate. Earlier studies have also identified this gene as a top candidate in Draa goats in Morocco (Benjelloun et al., 2015). However, in that study it was not conclusive if the candidate gene was associated with body weight.
Other genes identified in Boer such as the gap junction protein genes GJB2, GJB6, and GJA3 belong to the family of genes involved in cell communication (GO:0007154). They encode proteins that influence body size, skeletal and embryonic development and testicular embryogenesis, and may indirectly influence traits such as growth (Kim et al., 2016). The region (41.943–42.086 Mb) on CHI13 contains another gene ACSS1 (acyl-CoA Synthetase Short-chain Family Member 1), which has been associated with body weight, food intake, post-natal growth rate and susceptibility to weight loss among others (Liu et al., 2017).
Gene Enrichment Analysis
Our findings indicated that pathways associated with production and mechanisms of environmental adaptation, such as immune response, male reproduction, energy production and heat stress, may be under selection in Ugandan goat breeds. This is in agreement with findings in East African Short-horn Zebu cattle (Bahbahani et al., 2015, 2017, 2018), South African cattle (Makina et al., 2015), and indigenous goats in Morocco and Egypt (Benjelloun et al., 2015; Kim et al., 2016). Gene ontology analysis shows that multiple pathways are expressed in the Ugandan goat breeds, which may indicate an adaptation to varied environmental conditions. This is also confirmed by recent studies with indigenous Sudanese goats which similarly implicated several biological processes (Rahmatalla et al., 2017). The multiplicity in the number of candidate regions and genes detected in the present study confirms findings from livestock species in stressful environments (Kim et al., 2016, 2017; Mwacharo et al., 2017). These studies and the current one, reaffirm the fact that adaptation is generally a complex trait, involving several biological processes and quantitative trait loci with each contributing a small but cumulative effect to the overall phenotype.
Although, the results based on raw p-values yielded very interesting biological pathways which may be overrepresented, the results of the more stringent multiple testing corrections such as Bonferroni correction, were not significant. This may be attributed to the small sample size involved in this study. Nonetheless, these results provide a useful indication of mechanisms involved in environmental adaptation in the indigenous goat breeds.
Genomic Inbreeding Based on ROH (FROH)
In the absence of pedigree records, ROH may be useful to infer the level of inbreeding. Computing the proportion of an individual’s genome occurring as an ROH of particular length (e.g., >1, >2, or >4 Mb) provides information on the level of inbreeding relative to a population several generations ago (Curik et al., 2014; Forutan et al., 2018). At ROH threshold of >2 Mb, the indigenous breeds showed very low levels of genomic inbreeding, as compared to the higher inbreeding levels found in the exotic Boer (Table 2). The low genomic inbreeding level reported in this study is consistent with findings in Swiss goat breeds (Burren et al., 2016) and Barki goats (Kim et al., 2016). Genomic inbreeding based on ROH provides an accurate estimate of an individual’s autozygosity than pedigree based inbreeding due to either incomplete or non-existent pedigree information (Ferenčaković et al., 2013a,b; Forutan et al., 2018).
Runs of homozygosity usually emanate from identical haplotypes being transmitted from parents to offspring (Purfield et al., 2012; Iacolina et al., 2016). The frequency of their existence provides a clue on the demographic history and management of the population over time (Kirin et al., 2010; Ceballos et al., 2018). The mean sum of ROH segment coverage was generally higher for short ROHs than for long ROHs (Figure 3). However, Karamojong showed a higher average sum of ROH> 16 Mb. The distribution of ROH coverage reported in this study is in agreement with other studies in goats (Brito et al., 2017), sheep (Purfield et al., 2017), and cattle (Ferenčaković et al., 2013a; Mastrangelo et al., 2016), in which long ROH segments were found less frequently compared to shorter ones. Although the short ROH were more frequent in the genome of the indigenous goat breeds, their absolute contribution to the genome was substantially low (except in Kigezi goats) (Supplementary Table S7). This result is consistent with findings of Bosse et al., (2012), who reported that short ROH were abundant in the porcine genome, but contributed less to the genome as compared to large ROH ( > 5 Mb). This may be due to differences in selection events in the more recent or ancestral populations. However, the short ROH in the Boer and indigenous Kigezi goats contributed more to the absolute coverage of the genome by the SNPs. The higher proportion of ROH segments within the short ROH categories indicates a relatively larger contribution of distant inbreeding, whereas the higher coverage of long ROH observed in Karamojong suggests a larger effect of more recent inbreeding. Karamojong goats are reared under pastoral production systems and may be subject to selection of best performing males by their keepers. This coupled with smaller effective population size could be contributing to the high recent inbreeding observed. On the other hand, the Kigezi goats are isolated populations that have undergone limited more recent selection, which could explain the high frequency of short ROH segments attributed to more distant inbreeding. The longer stretches of ROH in the exotic Boer goats may be due to the stringent artificial selection for production traits on few selection candidates (narrow genetic base) and may thus explain the higher levels of genomic inbreeding. Longer stretches of ROH were also observed in exotic goat breeds when compared to Barki goats in Egypt (Kim et al., 2016). Generally, shorter ROH is associated with more ancient inbreeding, while longer ROH tend to show a more recent inbreeding (Browning and Browning, 2012, 2013).
Although there are limited quantitative trait loci in goats, our study provides a basis for future research in goat genomics of tropically adapted breeds. Using medium density SNPs, we could detect selection signatures associated with adaptation to tropical environmental conditions. With the release of the caprine 50K SNP chip (Tosser-Klopp et al., 2014), several efforts are underway including improvements in the annotation of the goat genome assembly (Bickhart et al., 2017). Arguably, these developments will change the landscape of genomic research in goats, allowing for inclusion of genomic evaluations in goat breeding programs. The integration of genomic information will undoubtedly lead to better management and sustainable utilization of genetic resources. The results of this study will advance our understanding of environmentally driven adaptation and its potential application in functional genomics and selective breeding as well as in design of management programs to conserve livestock genetic diversity to cope with the current and future predicted effects of climate change.
Conclusion
Using genome-wide SNP data, we investigated for the first-time selection signatures in Ugandan goat breeds that may be shaping their adaptation to varied environmental conditions. The study identified several putative genomic regions and genes in Ugandan goat populations, which may be underlying adaptation to local environmental conditions such as heat tolerance, disease and parasite resistance, and production traits. Generally, non-classical sweeps with limited overlap were observed which is typical of complex traits.
In the absence of pedigree data, genomic information through ROH provides a useful tool for quantifying the level of genomic inbreeding in the populations.
The study provides a foundation for detailed analysis of the identified putative selection signatures in the goat genome particularly of the tropically adapted breeds and provides an avenue for a well-structured breed improvement.
Data Accessibility
Data from samples used in the present study are available from the Zenodo Digital Repository: https://doi.org/10.5281/zenodo.1184716.
Ethics Statement
The animals sampled specifically for this study had their processes evaluated and approved by the Ethics Committee of Uganda National Council of Science and Technology (UNCST; SBLS/REC/15/131).
Author Contributions
RO, MU, and MB conceived the study. RO drafted the manuscript. HD, MU, LB, and RO participated in the data analysis. MG, EK, RC, and MB supervised the study. All the authors read and approved the manuscript.
Funding
This work was financially supported by the National Agricultural Research Organization (NARO) in Uganda, through a World Bank-supported project, Agricultural Technology, and Agribusiness Advisory Services (ATAAS) (P109224).
Conflict of Interest Statement
The authors declare that the research was conducted in the absence of any commercial or financial relationships that could be construed as a potential conflict of interest.
Acknowledgments
Bert Dibbits – Wageningen University and Research, Animal Breeding, and Genomics for processing genomic DNA samples for genotyping. Paul Kashaija and Dr. Benda Kirungi from the National Livestock Resources Research Institute and Kachwekano Zonal Agricultural Research and Development Institute for support in DNA extraction and field sampling, respectively. Robert Mukiibi brainstorming discussion on the subject to shape-up the study. The smallholder farmers for allowing their animals to be sampled for the study.
Supplementary Material
The Supplementary Material for this article can be found online at: https://www.frontiersin.org/articles/10.3389/fgene.2018.00318/full#supplementary-material
Footnotes
References
Alfonzo, E. P. M., Barbosa Da Silva, M. V. G., Dos Santos Daltro, D., Stumpf, M. T., Dalcin, V. C., Kolling, G., et al. (2016). Relationship between physical attributes and heat stress in dairy cattle from different genetic groups. Int. J. Biometeorol. 60, 245–253. doi: 10.1007/s00484-015-1021-y
Arias, J. A., Keehan, M., Fisher, P., Coppieters, W., and Spelman, R. (2009). A high density linkage map of the bovine genome. BMC Genet. 10:10. doi: 10.1186/1471-2156-10-18
Ashburner, M., Ball, C. A., Blake, J. A., Botstein, D., Butler, H., Cherry, J. M., et al. (2000). Gene ontology: tool for the unification of biology. Nat. Genet. 25, 25–29. doi: 10.1038/75556
Bahbahani, H., Clifford, H., Wragg, D., Mbole-Kariuki, M. N., Van Tassell, C., Sonstegard, T., et al. (2015). Signatures of positive selection in East African Shorthorn Zebu: a genome-wide single nucleotide polymorphism analysis. Sci. Rep. 5:11729. doi: 10.1038/srep11729
Bahbahani, H., and Hanotte, O. (2015). Genetic resistance - tolerance to vector-borne diseases and the prospects and challenges of genomics. Sci. Tech. Rev. 34, 185–197.
Bahbahani, H., Salim, B., Almathen, F., Al Enezi, F., Mwacharo, J. M., and Hanotte, O. (2018). Signatures of positive selection in African Butana and Kenana dairy zebu cattle. PLoS One 13:e0190446. doi: 10.1371/journal.pone.0190446
Bahbahani, H., Tijjani, A., Mukasa, C., Wragg, D., Almathen, F., Nash, O., et al. (2017). Signatures of selection for environmental adaptation and zebu × taurine hybrid fitness in east african shorthorn zebu. Front. Genet. 8:68. doi: 10.3389/fgene.2017.00068
Benjelloun, B., Alberto, F. J., Streeter, I., Boyer, F., Coissac, E., Stucki, S., et al. (2015). Characterizing neutral genomic diversity and selection signatures in indigenous populations of moroccan goats (Capra hircus) using WGS data. Front. Genet. 6:107. doi: 10.3389/fgene.2015.00107
Bickhart, D. M., Rosen, B. D., Koren, S., Sayre, B. L., Hastie, A. R., Chan, S., et al. (2017). Single-molecule sequencing and chromatin conformation capture enable de novo reference assembly of the domestic goat genome. Nat. Genet. 49, 643–650. doi: 10.1038/ng.3802
Bosse, M., Megens, H.-J., Madsen, O., Paudel, Y., Frantz, L. A., Schook, L. B., et al. (2012). Regions of homozygosity in the porcine genome: consequence of demography and the recombination landscape. PLoS Genet. 8:e1003100. doi: 10.1371/journal.pgen.1003100
Brito, L. F., Kijas, J. W., Ventura, R. V., Sargolzaei, M., Porto-Neto, L. R., Cánovas, A., et al. (2017). Genetic diversity and signatures of selection in various goat breeds revealed by genome-wide SNP markers. BMC Genomics 18:229. doi: 10.1186/s12863-015-0220-1
Broman, K. W., and Weber, J. L. (1999). Long homozygous chromosomal segments in reference families from the centre d’étude du polymorphisme humain. Am. J. Hum. Genet. 65, 1493–1500. doi: 10.1086/302661
Browning, B., and Browning, S. (2013). Improving the accuracy and efficiency of identity by descent detection in population data. Genetics 194, 459–471. doi: 10.1534/genetics.113.150029
Browning, S. R., and Browning, B. L. (2012). Identity by descent between distant relatives: detection and applications. Annu. Rev. Genet. 46, 617–633. doi: 10.1146/annurev-genet-110711-155534
Burren, A., Neuditschko, M., Signer-Hasler, H., Frischknecht, M., Reber, I., Menzi, F., et al. (2016). Genetic diversity analyses reveal first insights into breed-specific selection signatures within Swiss goat breeds. Anim. Genet. 47, 727–739. doi: 10.1111/age.12476
Ceballos, F. C., Joshi, P. K., Clark, D. W., Ramsay, M., and Wilson, J. F. (2018). Runs of homozygosity: windows into population history and trait architecture. Nat. Rev. Genet. 19, 220–234. doi: 10.1038/nrg.2017.109
Chang, C. C., Chow, C. C., Tellier, L. C., Vattikuti, S., Purcell, S. M., and Lee, J. J. (2015). Second-generation PLINK: rising to the challenge of larger and richer datasets. GigaScience 4, 1–16. doi: 10.1186/s13742-015-0047-8
Chiejina, S. N., and Behnke, J. M. (2011). The unique resistance and resilience of the Nigerian West African Dwarf goat to gastrointestinal nematode infections. Parasites Vectors 4:12. doi: 10.1186/1756-3305-4-12
Curik, I., Ferenèakoviæ, M., and Sölkner, J. (2014). Inbreeding and runs of homozygosity: a possible solution to an old problem. Livest. Sci. 166, 26–34. doi: 10.1016/j.livsci.2014.05.034
Doekes, H. P., Veerkamp, R. F., Bijma, P., Hiemstra, S. J., and Windig, J. J. (2018). Trends in genome-wide and region-specific genetic diversity in the Dutch-Flemish holstein–friesian breeding program from 1986 to 2015. Genet. Sel. Evol. 50:15. doi: 10.1186/s12711-018-0385-y
Fariello, M. I., Boitard, S., Naya, H., Sancristobal, M., and Servin, B. (2013). Detecting signatures of selection through haplotype differentiation among hierarchically structured populations. Genetics 193, 929–941. doi: 10.1534/genetics.112.147231
Fariello, M.-I., Servin, B., Tosser-Klopp, G., Rupp, R., Moreno, C., International Sheep Genomics Consortium et al. (2014). Selection signatures in worldwide sheep populations. PLoS One 9:e103813. doi: 10.1371/journal.pone.0103813
Ferenčaković, M., Hamzic, E., Gredler, B., Curik, I., and Sölkner, J. (2011). Runs of homozygosity reveal genome-wide autozygosity in the Austrian fleckvieh cattle. Agric. Conspec. Sci. 76, 325–328.
Ferenčaković, M., Hamziæ, E., Gredler, B., Solberg, T. R., Klemetsdal, G., Curik, I., et al. (2013a). Estimates of autozygosity derived from runs of homozygosity: empirical evidence from selected cattle populations. J. Anim. Breeding Genet. 130, 286–293. doi: 10.1111/jbg.12012
Ferenčaković, M., Sölkner, J., and Curik, I. (2013b). Estimating autozygosity from high-throughput information: effects of SNP density and genotyping errors. Genet. Sel. Evol. 45:42. doi: 10.1186/1297-9686-45-42
Ferrao, R., Wallweber, H. J., Ho, H., Tam, C., Franke, Y., Quinn, J., et al. (2016). The structural basis for class II cytokine receptor recognition by JAK1. Structure 24, 897–905. doi: 10.1016/j.str.2016.03.023
Fisher, R. A. (1954). A fuller theory of “Junctions” in inbreeding. Heredity 8, 187–197. doi: 10.1038/hdy.1954.17
Forutan, M., Ansari Mahyari, S., Baes, C., Melzer, N., Schenkel, F. S., and Sargolzaei, M. (2018). Inbreeding and runs of homozygosity before and after genomic selection in North American holstein cattle. BMC Genomics 19:98. doi: 10.1186/s12864-018-4453-z
Fox, C. S., Heard-Costa, N., Cupples, L. A., Dupuis, J., Vasan, R. S., and Atwood, L. D. (2007). Genome-wide association to body mass index and waist circumference: the framingham Heart Study 100K project. BMC Med. Genet. 8:S18. doi: 10.1186/1471-2350-8-S1-S18
Guo, Z., González, J. F., Hernandez, J. N., Mcneilly, T. N., Corripio-Miyar, Y., Frew, D., et al. (2016). Possible mechanisms of host resistance to Haemonchus contortus infection in sheep breeds native to the Canary Islands. Sci. Rep. 6:26200. doi: 10.1038/srep26200
Heidaritabar, M., Vereijken, A., Muir, W. M., Meuwissen, T., Cheng, H., Megens, H. J., et al. (2014). Systematic differences in the response of genetic variation to pedigree and genome-based selection methods. Heredity 113, 503–513. doi: 10.1038/hdy.2014.55
Howrigan, D., Simonson, M., and Keller, M. (2011). Detecting autozygosity through runs of homozygosity: a comparison of three autozygosity detection algorithms. BMC Genomics 12:460. doi: 10.1186/1471-2164-12-460
Huang, D. W., Sherman, B. T., and Lempicki, R. A. (2009). Systematic and integrative analysis of large gene lists using David bioinformatics resources. Nat. Protoc. 4, 44–57. doi: 10.1038/nprot.2008.211
Iacolina, L., Stronen, A. V., Pertoldi, C., Tokarska, M., Nørgaard, L. S., Muñoz, J., et al. (2016). Novel graphical analyses of runs of homozygosity among species and livestock breeds. Int. J. Genom. 2016:8. doi: 10.1155/2016/2152847
Jiao, X., Sherman, B. T., Huang, D. W., Stephens, R., Baseler, M. W., Lane, H. C., et al. (2012). DAVID-WS: a stateful web service to facilitate gene/protein list analysis. Bioinformatics 28, 1805–1806. doi: 10.1093/bioinformatics/bts251
Kanehisa, M., Goto, S., Sato, Y., Furumichi, M., and Tanabe, M. (2012). KEGG for integration and interpretation of large-scale molecular data sets. Nucleic Acids Res. 40, D109–D114. doi: 10.1093/nar/gkr988
Kemper, K. E., Saxton, S. J., Bolormaa, S., Hayes, B. J., and Goddard, M. E. (2014). Selection for complex traits leaves little or no classic signatures of selection. BMC Genomics 15:246. doi: 10.1186/1471-2164-15-246
Kijas, J. W., Lenstra, J. A., Hayes, B., Boitard, S., Porto Neto, L. R., San Cristobal, M., et al. (2012). Genome-wide analysis of the world’s sheep breeds reveals high levels of historic mixture and strong recent selection. PLoS Biol. 10:e1001258. doi: 10.1371/journal.pbio.1001258
Kim, E. S., Elbeltagy, A. R., Aboul-Naga, A. M., Rischkowsky, B., Sayre, B., Mwacharo, J. M., et al. (2016). Multiple genomic signatures of selection in goats and sheep indigenous to a hot arid environment. Heredity 116, 255–264. doi: 10.1038/hdy.2015.94
Kim, J., Hanotte, O., Mwai, O. A., Dessie, T., Bashir, S., Diallo, B., et al. (2017). The genome landscape of indigenous African cattle. Genome Biol. 18:34. doi: 10.1186/s13059-017-1153-y
Kirin, M., McQuillan, R., Franklin, C. S., Campbell, H., Mckeigue, P. M., and Wilson, J. F. (2010). Genomic runs of homozygosity record population history and consanguinity. PLoS One 5:e13996. doi: 10.1371/journal.pone.0013996
Liu, L., Cui, H., Fu, R., Zheng, M., Liu, R., Zhao, G., et al. (2017). The regulation of IMF deposition in pectoralis major of fast- and slow- growing chickens at hatching. J. Anim. Sci. Biotechnol. 8, 77. doi: 10.1186/s40104-017-0207-z
Lv, F.-H., Agha, S., Kantanen, J., Colli, L., Stucki, S., Kijas, J. W., et al. (2014). Adaptations to climate-mediated selective pressures in sheep. Mol. Biol. Evol. 31, 3324–3343. doi: 10.1093/molbev/msu264
Ma, Y., Wei, J., Zhang, Q., Chen, L., Wang, J., Liu, J., et al. (2015). A genome scan for selection signatures in pigs. PLoS One 10:e0116850. doi: 10.1371/journal.pone.0116850
MAAIF (2011). Statistical Abstrac. Entebbe: Ministry of Agriculture Animal Industry and Fisheries (MAAIF).
MAAIF and UBOS (2009). The NAtional Livestock Census Report 2008. Entebbe: Ministry of Agriculture, Animal Industry and Fisheries (MAAIF).
Makina, S. O., Muchadeyi, F. C., Van Marle-Köster, E., Taylor, J. F., Makgahlela, M. L., and Maiwashe, A. (2015). Genome-wide scan for selection signatures in six cattle breeds in South Africa. Genet. Sel. Evol. 47:92. doi: 10.1186/s12711-015-0173-x
Marras, G., Gaspa, G., Sorbolini, S., Dimauro, C., Ajmone-Marsan, P., Valentini, A., et al. (2015). Analysis of runs of homozygosity and their relationship with inbreeding in five cattle breeds farmed in Italy. Anim. Genet. 46, 110–121. doi: 10.1111/age.12259
Mason, I. L., and Maule, J. P. (1960). “The indigenous livestock of eastern and Southern Africa,” in Commonwealth Bureau of Animal Breeding and Genetics, ed. N. C. Wright [Farnham Royal: CAB (Commonwealth Agricultural Bureaux)], 151.
Mastrangelo, S., Tolone, M., Di Gerlando, R., Fontanesi, L., Sardina, M. T., and Portolano, B. (2016). Genomic inbreeding estimation in small populations: evaluation of runs of homozygosity in three local dairy cattle breeds. Animal 10, 746–754. doi: 10.1017/s1751731115002943
Maynard, J., and Haigh, J. (2007). The hitch-hiking effect of a favourable gene. Genet. Res. 89, 391–403. doi: 10.1017/s0016672308009579
McQuillan, R., Leutenegger, A.-L., Abdel-Rahman, R., Franklin, C. S., Pericic, M., Barac-Lauc, L., et al. (2008). Runs of homozygosity in European populations. Am. J. Hum. Genet. 83, 359–372. doi: 10.1016/j.ajhg.2008.08.007
McRae, K. M., McEwan, J. C., Dodds, K. G., and Gemmell, N. J. (2014). Signatures of selection in sheep bred for resistance or susceptibility to gastrointestinal nematodes. BMC Genomics 15:637. doi: 10.1186/1471-2164-15-637
Msalya, G., Kim, E. S., Laisser, E. L., Kipanyula, M. J., Karimuribo, E. D., Kusiluka, L. J., et al. (2017). Determination of genetic structure and signatures of selection in three strains of tanzania shorthorn zebu, boran and friesian cattle by genome-wide SNP analyses. PLoS One 12:e0171088. doi: 10.1371/journal.pone.0171088
Mwacharo, J. M., Kim, E.-S., Elbeltagy, A. R., Aboul-Naga, A. M., Rischkowsky, B. A., and Rothschild, M. F. (2017). Genomic footprints of dryland stress adaptation in Egyptian fat-tail sheep and their divergence from East African and western Asia cohorts. Sci. Rep. 7:17647. doi: 10.1038/s41598-017-17775-3
Nsubuga, H. S. K. (1996). “Small ruminant: goats and sheep in Uganda,” in Small Ruminant Research and Development in Africa: Proceedings of the Third Biennial Conference of the African Small Ruminant Research Network, (Kampala: UICC), 326.
Onzima, R. B., Mukiibi, R., Ampaire, A., Benda, K. K., and Kanis, E. (2017). Between-breed variations in resistance/resilience to gastrointestinal nematodes among indigenous goat breeds in Uganda. Trop. Anim. Health Prod. 49, 1763–1769. doi: 10.1007/s11250-017-1390-9
Onzima, R. B., Upadhyay, M. R., Mukiibi, R., Kanis, E., Groenen, M. A. M., and Crooijmans, R. P. M. A. (2018). Genome-wide population structure and admixture analysis reveals weak differentiation among Ugandan goat breeds. Anim. Genet. 49, 59–70. doi: 10.1111/age.12631
Peripolli, E., Stafuzza, N. B., Munari, D. P., Lima, A. L. F., Irgang, R., Machado, M. A., et al. (2018). Assessment of runs of homozygosity islands and estimates of genomic inbreeding in Gyr (Bos indicus) dairy cattle. BMC Genomics 19:34. doi: 10.1186/s12864-017-4365-3
Petersen, J. L., Mickelson, J. R., Rendahl, A. K., Valberg, S. J., Andersson, L. S., Axelsson, J., et al. (2013). Genome-wide analysis reveals selection for important traits in domestic horse breeds. PLoS Genet. 9:e1003211. doi: 10.1371/journal.pgen.1003211
Porto-Neto, L. R., Lee, S. H., Lee, H. K., and Gondro, C. (2013). “Detection of signatures of selection using FST,” in Genome-Wide Association Studies and Genomic Prediction, eds C. Gondro, J. Van Der Werf, and B. Hayes (Totowa, NJ: Humana Press), 423–436. doi: 10.1007/978-1-62703-447-0-19
Porto-Neto, L. R., Lee, S. H., Sonstegard, T. S., Tassell, C. P., Lee, H. K., and Gibson, J. P. (2014). Genome-wide detection of signatures of selection in Korean Hanwoo cattle. Anim. Genet. 45, 180–190. doi: 10.1111/age.12119
Purfield, D. C., Berry, D. P., McParland, S., and Bradley, D. G. (2012). Runs of homozygosity and population history in cattle. BMC Genet. 13:70. doi: 10.1186/1471-2156-13-70
Purfield, D. C., McParland, S., Wall, E., and Berry, D. P. (2017). The distribution of runs of homozygosity and selection signatures in six commercial meat sheep breeds. PLoS One 12:e0176780. doi: 10.1371/journal.pone.0176780
Rahmatalla, S. A., Arends, D., Reissmann, M., Said Ahmed, A., Wimmers, K., Reyer, H., et al. (2017). Whole genome population genetics analysis of sudanese goats identifies regions harboring genes associated with major traits. BMC Genet. 18:92. doi: 10.1186/s12863-017-0553-z
Reitberger, S., Haimerl, P., Aschenbrenner, I., Esser-Von Bieren, J., and Feige, M. J. (2017). Assembly-induced folding regulates interleukin 12 biogenesis and secretion. J. Biol. Chem. 292, 8073–8081. doi: 10.1074/jbc.M117.782284
Reynolds, J., Weir, B. S., and Cockerham, C. C. (1983). estimation of the coancestry coefficient: basis for a short-term genetic distance. Genetics 105, 767–779.
Rochus, C. M., Tortereau, F., Plisson-Petit, F., Restoux, G., Moreno-Romieux, C., Tosser-Klopp, G., et al. (2018). Revealing the selection history of adaptive loci using genome-wide scans for selection: an example from domestic sheep. BMC Genomics 19:71. doi: 10.1186/s12864-018-4447-x
Roy, K. S., and Collier, R. J. (2012). “Regulation of Acclimation to Environmental Stress,” in Environmental Physiology of Livestock. Hoboken, NJ: Wiley-Blackwell, 49–63. doi: 10.1002/9781119949091.ch4
Ryans, K., Omosun, Y., Mckeithen, D. N., Simoneaux, T., Mills, C. C., Bowen, N., et al. (2017). The immunoregulatory role of alpha enolase in dendritic cell function during Chlamydia infection. BMC Immunol. 18:27. doi: 10.1186/s12865-017-0212-1
Sanz Fernandez, M. V., Stoakes, S. K., Abuajamieh, M., Seibert, J. T., Johnson, J. S., Horst, E. A., et al. (2015). Heat stress increases insulin sensitivity in pigs. Physiol. Rep. 3:e12478. doi: 10.14814/phy2.12478
Shen, Y., Zhang, Y., Han, Y., Su, P., Gou, M., Pang, Y., et al. (2017). A novel Vav3 homolog identified in lamprey, lampetra japonica, with roles in lipopolysaccharide-mediated immune response. Int. J. Mol. Sci. 18:2035. doi: 10.3390/ijms18102035
Shi, Y., and Manley, J. L. (2007). A complex signaling pathway regulates SRp38 phosphorylation and pre-mRNA splicing in response to heat shock. Mol. Cell 28, 79–90. doi: 10.1016/j.molcel.2007.08.028
Simianer, H. (2014). “Statistical problems in livestock population genomics,” in Proceedings of the 10th World Congress on Genetics Applied to Livestock Production, Vancouver.
Talenti, A., Bertolini, F., Pagnacco, G., Pilla, F., Ajmone-Marsan, P., Rothschild, M. F., et al. (2017). The valdostana goat: a genome-wide investigation of the distinctiveness of its selective sweep regions. Mamm. Genome 28, 114–128. doi: 10.1007/s00335-017-9678-7
Taye, M., Lee, W., Caetano-Anolles, K., Dessie, T., Hanotte, O., Mwai, O. A., et al. (2017). Whole genome detection of signature of positive selection in African cattle reveals selection for thermotolerance. Anim. Sci. J. 88, 1889–1901. doi: 10.1111/asj.12851
Thompson, E. A. (2013). Identity by descent: variation in meiosis. across genomes, and in populations. Genetics 194, 301–326. doi: 10.1534/genetics.112.148825
Thumbi, S. M., Bronsvoort, B. M., Poole, E. J., Kiara, H., Toye, P. G., Mbole-Kariuki, M. N., et al. (2014). Parasite Co-infections and their impact on survival of indigenous cattle. PLoS One 9:e76324. doi: 10.1371/journal.pone.0076324
Tosser-Klopp, G., Bardou, P., Bouchez, O., Cabau, C., Crooijmans, R., Dong, Y., et al. (2014). Design and characterization of a 52K SNP chip for goats. PLoS One 9:e86227. doi: 10.1371/journal.pone.0086227
Wang, M. S., Li, Y., Peng, M. S., Zhong, L., Wang, Z. J., Li, Q. Y., et al. (2015). Genomic analyses reveal potential independent adaptation to high altitude in tibetan chickens. Mol. Biol. Evol. 32, 1880–1889. doi: 10.1093/molbev/msv071
Wang, X., Liu, J., Zhou, G., Guo, J., Yan, H., Niu, Y., et al. (2016). Whole-genome sequencing of eight goat populations for the detection of selection signatures underlying production and adaptive traits. Sci. Rep. 6:38932. doi: 10.1038/srep38932
Weir, B. S., and Cockerham, C. C. (1984). Estimating F-statistics for the analysis of population structure. Evolution 38, 1358–1370. doi: 10.2307/2408641
Wright, S. (1949). The genetical structure of populations. Ann. Eugen. 15, 323–354. doi: 10.1111/j.1469-1809.1949.tb02451.x
Wu, J.-H., Zhang, W.-G., Li, J.-Q., Yin, J., and Zhang, Y.-J. (2009). Hoxc13 expression pattern in cashmere goat skin during hair follicle development. Agric. Sci. China 8, 491–496. doi: 10.1016/S1671-2927(08)60237-0
Yang, J., Li, W.-R., Lv, F.-H., He, S.-G., Tian, S.-L., Peng, W.-F., et al. (2016). Whole-genome sequencing of native sheep provides insights into rapid adaptations to extreme environments. Mol. Biol. Evol. 33, 2576–2592. doi: 10.1093/molbev/msw129
Zhang, Q., Guldbrandtsen, B., Bosse, M., Lund, M. S., and Sahana, G. (2015). Runs of homozygosity and distribution of functional variants in the cattle genome. BMC Genomics 16:542. doi: 10.1186/s12864-015-1715-x
Zhao, F., McParland, S., Kearney, F., Du, L., and Berry, D. P. (2015). Detection of selection signatures in dairy and beef cattle using high-density genomic information. Genet. Sel. Evol. 47, 49. doi: 10.1186/s12711-015-0127-3
Zhao, X.-X., Zhang, Y.-B., Ni, P.-L., Wu, Z.-L., Yan, Y.-C., and Li, Y.-P. (2016). Protein arginine methyltransferase 6 (Prmt6) is essential for early zebrafish development through the direct suppression of gadd45αa stress sensor gene. J. Biol. Chem. 291, 402–412. doi: 10.1074/jbc.M115.666347
Keywords: Capra hircus, homozygosity, adaptation, genomic inbreeding, genetic diversity, selective sweeps, candidate genes
Citation: Onzima RB, Upadhyay MR, Doekes HP, Brito LF, Bosse M, Kanis E, Groenen MAM and Crooijmans RPMA (2018) Genome-Wide Characterization of Selection Signatures and Runs of Homozygosity in Ugandan Goat Breeds. Front. Genet. 9:318. doi: 10.3389/fgene.2018.00318
Received: 27 February 2018; Accepted: 25 July 2018;
Published: 14 August 2018.
Edited by:
Max F. Rothschild, Iowa State University, United StatesReviewed by:
Heather Jay Huson, Cornell University, United StatesGábor Mészáros, Universität für Bodenkultur Wien, Austria
Copyright © 2018 Onzima, Upadhyay, Doekes, Brito, Bosse, Kanis, Groenen and Crooijmans. This is an open-access article distributed under the terms of the Creative Commons Attribution License (CC BY). The use, distribution or reproduction in other forums is permitted, provided the original author(s) and the copyright owner(s) are credited and that the original publication in this journal is cited, in accordance with accepted academic practice. No use, distribution or reproduction is permitted which does not comply with these terms.
*Correspondence: Robert B. Onzima, cm9iZXJ0Lm9uemltYUB3dXIubmw=; cm9iZXJ0b256aW1hQGdtYWlsLmNvbQ==