- 1Oncogenetics Group, Vall d’Hebron Institute of Oncology, Barcelona, Spain
- 2Laboratorio de Oncología Molecular – Centro de Investigación Biomédica en Red de Cancer, Instituto de Investigación Sanitaria San Carlos, Hospital Clínico San Carlos, Madrid, Spain
- 3Hereditary Cancer Program, Catalan Institute of Oncology, Institut d’Investigació Biomédica de Bellvitge, Hospitalet de Llobregat, Barcelona, Spain
- 4Program in Molecular Mechanisms and Experimental Therapy in Oncology (Oncobell), Institut d’Investigació Biomédica de Bellvitge, Hospitalet de Llobregat, Barcelona, Spain
- 5Centro de Investigación Biomédica en Red de Cáncer, Madrid, Spain
- 6Grupo de Medicina Xenómica-USC, Fundación Pública Galega de Medicina Xenómica-SERGAS, CIBER de Enfermedades Raras, Instituto de Investigación Sanitaria, Santiago de Compostela, Spain
- 7High Risk and Cancer Prevention Group, Vall d’Hebron Institute of Oncology, Barcelona, Spain
- 8Medical Oncology Department, University Hospital Vall d’Hebron, Barcelona, Spain
- 9Area of Clinical and Molecular Genetics, University Hospital Vall d’Hebron, Barcelona, Spain
In silico tools for splicing defect prediction have a key role to assess the impact of variants of uncertain significance. Our aim was to evaluate the performance of a set of commonly used splicing in silico tools comparing the predictions against RNA in vitro results. This was done for natural splice sites of clinically relevant genes in hereditary breast/ovarian cancer (HBOC) and Lynch syndrome. A study divided into two stages was used to evaluate SSF-like, MaxEntScan, NNSplice, HSF, SPANR, and dbscSNV tools. A discovery dataset of 99 variants with unequivocal results of RNA in vitro studies, located in the 10 exonic and 20 intronic nucleotides adjacent to exon–intron boundaries of BRCA1, BRCA2, MLH1, MSH2, MSH6, PMS2, ATM, BRIP1, CDH1, PALB2, PTEN, RAD51D, STK11, and TP53, was collected from four Spanish cancer genetic laboratories. The best stand-alone predictors or combinations were validated with a set of 346 variants in the same genes with clear splicing outcomes reported in the literature. Sensitivity, specificity, accuracy, negative predictive value (NPV) and Mathews Coefficient Correlation (MCC) scores were used to measure the performance. The discovery stage showed that HSF and SSF-like were the most accurate for variants at the donor and acceptor region, respectively. The further combination analysis revealed that HSF, HSF+SSF-like or HSF+SSF-like+MES achieved a high performance for predicting the disruption of donor sites, and SSF-like or a sequential combination of MES and SSF-like for predicting disruption of acceptor sites. The performance confirmation of these last results with the validation dataset, indicated that the highest sensitivity, accuracy, and NPV (99.44%, 99.44%, and 96.88, respectively) were attained with HSF+SSF-like or HSF+SSF-like+MES for donor sites and SSF-like (92.63%, 92.65%, and 84.44, respectively) for acceptor sites.
We provide recommendations for combining algorithms to conduct in silico splicing analysis that achieved a high performance. The high NPV obtained allows to select the variants in which the study by in vitro RNA analysis is mandatory against those with a negligible probability of being spliceogenic. Our study also shows that the performance of each specific predictor varies depending on whether the natural splicing sites are donors or acceptors.
Introduction
The increasing use of massive parallel sequencing of customized multi-gene panels, for germline clinical testing of hereditary breast and ovarian cancer (HBOC) and Lynch syndrome, is leading to higher detection of genetic variants of unknown significance (VUS).
All exonic or intronic VUS can be potentially spliceogenic by disrupting the cis DNA sequences that define exons, introns, and regulatory sequences necessary for a correct RNA splicing process. Specifically, the cis DNA elements include: (i) exon–intron boundary core consensus nucleotides (GT at +1 and +2 of the 5′donor site and AG at -1 and -2 of the 3′acceptor site); (ii) intronic and exonic nucleotides adjacent to these invariable nucleotides that are also highly conserved and have been found to be critical for splice site selection: CAG/GUAAGU in donor sites and NYAG/G in acceptor sites; (iii) branch point and polypyrimidine tract sequence motifs, essential for the spliceosome complex formation; (iv) intronic and exonic sequences that act as splicing enhancers (ISE and ESE) or silencers (ISS and ESS), regulatory motifs that are usually bound by serine/arginine (SR)-rich proteins and heterogeneous nuclear ribonucleoproteins (hnRNPs), respectively (Cartegni et al., 2002; Soukarieh et al., 2016; Abramowicz and Gos, 2018). A nucleotide change in any of these elements could lead to incorrect splice site recognition, creating new ones or activating the cryptic ones, resulting in aberrant transcripts and in non-functional proteins associated with disease such as hereditary cancer.
Interestingly, it has recently been described that hereditary cancer genes (including some HBOC and Lynch genes) are enriched for spliceogenic variants (Rhine et al., 2018). This finding highlights the importance of both the identification and the functional interpretation of variants causing RNA alterations in hereditary cancer genes. In HBOC syndrome and Lynch Syndrome, the clinical classification of VUS is essential since carriers of pathogenic variants may benefit from cancer prevention and risk-reducing strategies, make informed decisions about prophylactic surgery, and benefit from targeted treatments (Moreno et al., 2016). Conversely, carriers of non-pathogenic variants can be excluded from intensive follow-ups and avoid unnecessary risk-reducing surgery (Eccles et al., 2015).
To detect splice site alterations, in vitro splicing assays with patient’s RNA or minigenes are widely used. However, testing all variants detected in the vicinity of exon–intron boundaries can be time consuming and expensive. In consequence, to select variants to be experimentally evaluated, a large number of prediction programs have been developed. These splicing computational tools are based on different premises. The most commonly used are based on Position Weight Matrix (PWM), in which each nucleotide on the splice site sequence is scored and ranked based on its frequency from its aligned consensus sequence (Shapiro and Senapathy, 1987; Desmet et al., 2009). Neural network programs use sets of sequences from databases to identify splicing sites (Reese et al., 1997). Tools based on Maximum Entropy Distribution models take into account the dependencies between nucleotide positions (Yeo and Burge, 2004). Approaches like SPANR (Xiong et al., 2015) use DNA and RNA sequence information and a machine learning method, to predict splicing alterations, enabling the identification of variants affecting cis and trans splicing factors. Another type of splicing tool has been developed using ensemble learning methods (adaptive boosting and random forest) taking advantage of individual computational tools (Jian et al., 2014a).
Several studies have analyzed the performance of these tools for genes related to cancer and other diseases and report discordant results without a consensus guideline recommending which programs should be used (Houdayer et al., 2008, 2012; Holla et al., 2009; Vreeswijk et al., 2009; Desmet et al., 2010; Théry et al., 2011; Colombo et al., 2013; Jian et al., 2014a; Tang et al., 2016) (Table 1). Here, we present an evaluation of the performance of commonly used splicing in silico tools, comparing their output with the experimental evidences obtained by RNA in vitro analysis of variants detected in HBOC and Lynch syndrome genes. In the first phase of the study, we assessed the accuracy of the splicing in silico tools with a dataset of RNA in vitro outcomes collected from four Spanish cancer genetic units. Subsequently, we validated the best algorithms obtained in the discovery phase, with findings obtained after RNA analysis extracted from different curated databases and reported literature.
Materials and Methods
Variant Selection
Discovery Set
We restricted the study to variants located within the last 10 exonic and 20 first intronic nucleotides from the 5′ splice donor site, and the last 20 intronic and the first 10 exonic nucleotides from the 3′ splice acceptor site (-10 to +20 and -20 to +10, respectively). BRCA1, BRCA2, MLH1, MSH2, MSH6, and PMS2 variants were selected from HBOC and Lynch syndrome patients routinely analyzed for diagnostic purposes. We also included ATM, BRIP1, CDH1, PALB2, PTEN, RAD51D, STK11, and TP53 variants obtained in a research series of BRCA1 and BRCA2 negative HBOC patients. Genetic variants with unequivocal experimental evidences showing presence or absence of alterations in the mRNA, were collected from four different Spanish centers: Hospital Universitari Vall d’Hebron (HUVH), Barcelona; Hospital Clínico San Carlos (HCSC) Madrid; Fundación Pública Galega de Medicina Xenomica (FPGMX), Santiago de Compostela; Institut Català d’Oncologia (ICO), Hospital Duran i Reynals, Barcelona.
The variants included in the discovery set were analyzed in vitro in carriers and controls. RNA was isolated from whole blood leukocytes or short-term lymphocyte cultures, phytohaemagglutinin stimulated, and treated with and without puromycin. The contributing laboratories used diverse isolation protocols and/or cDNA synthesis strategies following ENIGMA recommendations (Colombo et al., 2014; Whiley et al., 2014). Briefly, the splicing products generated by reverse transcription-polymerase chain reaction (RT-PCR) assays were characterized using agarose gel or capillary electrophoresis in a QIAxcel instrument with QIAxcel DNA High Resolution Kit (QIAGEN) or an Agilent 2100 Bioanalyzer (Agilent), and Sanger sequencing. PCR primers were designed to amplify at least one whole exon 5′ and 3′ flanking the exon harboring the variant of interest. Primer sequences are available upon request.
The study was approved by the Institutional Review Board of each participating center. Patients received genetic counseling and written informed consent was obtained for further genetic and research studies.
Validation Set
At this stage, the predictors that presented the best performance alone or in combination, were applied to compare their predictions with the in vitro RNA results from the dataset obtained through literature and databases. We chose a collection of variants reported in INSIGHT, ClinVar and published works that were (i) located within the regions defined for the discovery set; (ii) identified in the set of cancer risk genes included above; (iii) experimentally confirmed as spliceogenic and non-spliceogenic in blood samples or with minigene assay at least by RT-PCR, agarose gel and Sanger Sequencing analysis; and (iv) not located at exonic splicing enhancer (ESE) regions with specific experimental evidence of causing splicing alteration.
In silico Splice Tools
A total of six splice-site prediction software programs were selected for this study. Two ensemble prediction scores constructed by Jian et al. (2014a) using adaptive boosting and random forests ensemble learning methods, were extracted from dbscSNV database1. Splicing-based Analysis of Variants (SPANR), a computational model of splicing derived from the application of “deep learning” computer algorithms (Xiong et al., 2015) was ascertained by its own web site2. Splice Site Finder (SSF-like) (based on Shapiro and Senapathy, 1987), MaxEntScan (MES) (Yeo and Burge, 2004), Splice Site Prediction by Neural Network (NNPLICE) (Reese et al., 1997), and Human Splicing Finder (HSF) (Desmet et al., 2009) accessed through Alamut Visual 2.10 (Interactive Biosoftware). The GeneSplicer program is also included in the splicing module of Alamut, but it was excluded from the study since we noticed it had an exceedingly high missing scores (no estimation was obtained for 30% of the variants analyzed; data not shown), which had also been reported by Jian et al. (2014a). SPANR and dbscSNV do not analyze insertions and deletions and dbscSNV gives estimations for variants only located from -3 to +8 at 5′ and -12 to +2 at 3′ (Supplementary Table 1).
To interrogate the splicing prediction tools, we calculated the score variation caused by the variant in the donor site or acceptor site. To do that, we compared the score computed in the wild-type sequence (WT) to the score computed in the variant sequence (VAR) as:
We calculated the % score variation for four out of the six tools (SSF-like, HSF, MES, and NNSPLICE), since dbscSNV and SPANR already provide a score change.
To consider a % score change as a positive prediction of a splicing motif disruption caused by the variant, which would lead to aberrant splicing, we adopted thresholds pre-established in the literature (Supplementary Table 1). When two programs were combined, a correct prediction of splicing alteration was considered if at least one of them scored above the threshold. When three, four, five, or six programs were combined, all tools but one had to score above the threshold to indicate splicing alteration.
Performance Assessment
In the discovery and validation phases, the experimental RNA results for each collected variant were annotated as positive splicing alteration when they unequivocally, verified by gel electrophoresis and Sanger sequencing, lead to: exon skipping, use of a new or cryptic splice site or altered alternative transcript profile. In contrast, a negative splicing alteration was annotated when the in vitro RNA result was exactly the same as that obtained in control samples.
For both stages, we calculated the overall accuracy (ratio of overall correct predictions to the total number of predictions), specificity (correct identification of non-spliceogenic variants; true negative rate), and sensitivity (correct identification of deleterious variants; true positive rate). The positive predictive values (PPV, proportion of positive predictions that were true positives), negative predictive values (NPV, proportion of negative predictions that were true negatives), false negative rates (FNR, proportion of false negative detection), and false positive rates (FPR, proportion of false positive detection) were also calculated. Matthews correlation coefficient (MCC) was used to provide a balanced comparison between in silico tools.
Results
Discovery Set
A total of 99 variants with unequivocal RNA in vitro results were studied, located within positions -10 to +20 from the 5′ donor site, and within -20 to +10 from the 3′ acceptor site (Supplementary Table 2). Forty-four of the 99 variants generated a splice defect, with 11 and 9 disrupting the canonical GT or AG dinucleotides, respectively. The 24 remaining variants with aberrant splicing were located outside invariable GT or AG positions, with 15 variants altering the 5′ splice site and nine altering the 3′ splice site. Fifty-five variants did not yield an aberrant splicing, all located outside invariant dinucleotides. Figure 1 displays the number of positive and negative splicing results relative to variant location.
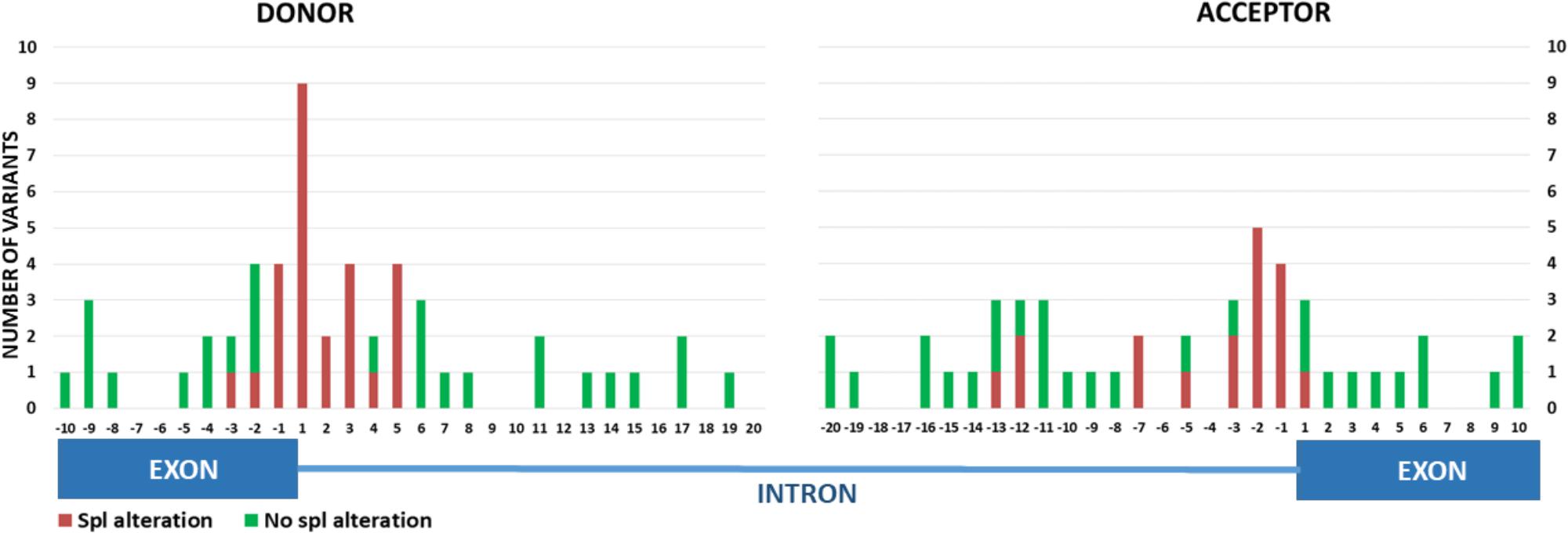
FIGURE 1. In vitro RNA results collected in the discovery set. Experimental data are displayed according to variation location. Positive splicing alterations include: exon skipping, use of a new or cryptic splice site or an altered-alternative transcript profile. Negative splicing alteration: in vitro RNA result was exactly the same as that obtained in control samples. Spl, splicing.
Six in silico tools were used to interrogate the 99 variants, and their corresponding % score variation was obtained. These outputs were compared to the experimental RNA results. The respective thresholds pre-established in the literature were adopted for each program (Supplementary Table 1).
Supplementary Table 2 lists the % score variation obtained from each splicing tool used to assess the 99 variants, highlighting which scores were in agreement with the RNA analysis outcome. Of note, seven insertions or deletions were not computed by SPANR and dbscSNV, while estimations for 33 substitutions were not provided by dbscSNV.
Table 2 shows separately, for 5′ (52 variants), 3′ (47 variants), and both splice sites (global, 99 variants), the results of performance analysis for each one of the tools. The six predictors detected wild type (WT) splice sites in reference sequences for all the genes of interest.
On average, predictions for variants located in 5′ regions have higher accuracy (90.98%), sensitivity (90.44%) and specificity (91.28%) compared to those located in 3′ regions (83.74%, 84.52%, and 82.30%, respectively) (Table 2). The predictions computed by HSF (with a score change threshold of -2%) were the most accurate and sensitive for variants at donor site, while for variants at acceptor sites or affecting either acceptor or donor sites (global), SSF-like were the most accurate (with a score change threshold of -5%). MES program (with a score change threshold of -15%) showed 100% of sensitivity on all predictions, but its specificity did not reach 87% in any case. In contrast, SPANR program showed the highest values of specificity for predictions of variants at donor site or all variants affecting either at acceptor or donor splice sites, but the lowest values of sensitivity (Table 2).
Accordingly, the lowest false negative rates for 5′splice site were reached by the HSF and MES predictors, while at 3′splice sites, the SSF-like and MES predictors obtained the lowest false negative rates (Table 2 and Figure 2). In contrast, SPANR predictor had the highest false negative and the lowest false positive rates in almost all cases (Table 2 and Figure 2). Regarding the estimation of the proportion of negative predictions that were true negatives (NPV), HSF or MES and SSF-like or MES achieved the highest values (100%) for donor and acceptor sites, respectively (Table 2).
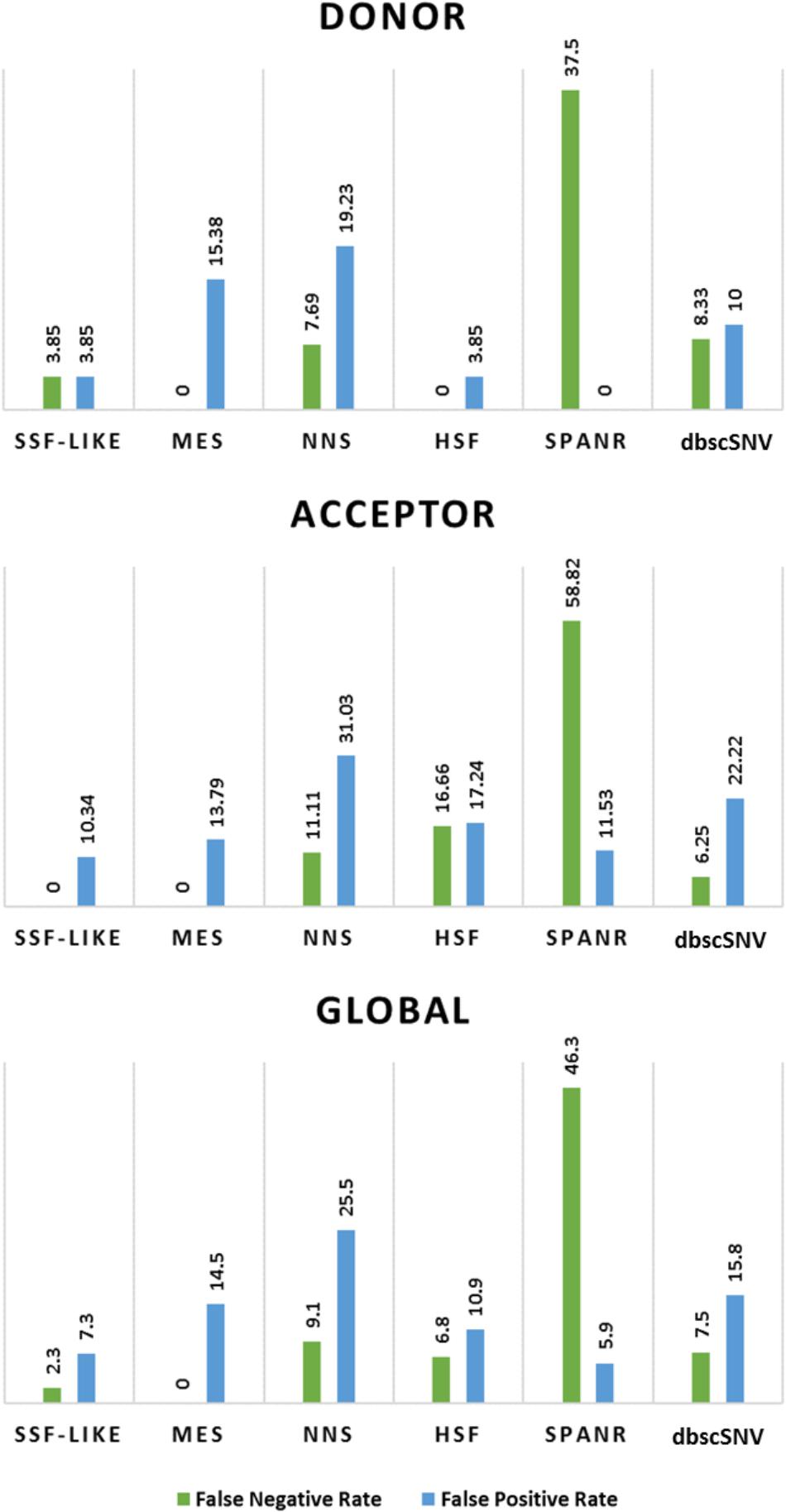
FIGURE 2. False negative and false positive rates for individual splicing prediction tools in the discovery set. dbscSNV, database consulted for extracting the adaptive boosting and random forests scores.
The accuracy of all possible predictor combinations was further assessed. For 5′ donor splice sites, predictions of HSF alone or HSF together with seven different combinations, SSF-like+SPANR and SSF-like+MES+SPANR reached a 98.08% of accuracy with the highest sensitivity for all the models (100%), obtaining 96.15% of specificity, 0.96 MCC and 100% of NPV (Supplementary Table 3). For 3′ splice sites, a sequential combination recommended by Houdayer et al. (2012) using MES as first-line analysis with a cut-off of 15% followed by SSF-like with a 5% threshold achieved the best performance, with a 100% of sensitivity, 96.55% of specificity, 97.87 % of accuracy, 0.96 MCC, and 100% of NPV (Supplementary Table 4). However, SSF-like alone and two more combinations including it also showed a 100% of NPV together with 100% sensitivity and high values of accuracy (for predictions at acceptor site, Supplementary Table 4). Considering the tool combinations for predicting disruption caused by variants located in any of the two splice sites (global), MES and SSF-like sequential combination achieved the best accuracy with a 96.97% and 0.94 of MCC, followed for two combinations, including SSF-like and MES, which showed 100% sensitivity and 100% of NPV (Supplementary Table 5).
Validation Set
In order to validate the predictors with the best performance obtained in the discovery set, we analyzed a dataset of 346 variants with RNA in vitro results published or detailed in free available databases. At donor region, 210 variants were included, 177 showing in vitro splicing alterations (65 at intronic GT positions) and 33 showing no splicing effects (all outside intronic GT) (Figure 3 and Supplementary Table 6). One hundred thirty-six variants were located at the acceptor region, 95 showing splicing alterations (67 of them at intronic AG positions), and 41 with absence of alterations (40 of them outside intronic AG) (Figure 3 and Supplementary Table 7). Only SSF-like and SPANR were able to identify all WT splice sites in reference sequences for all the genes of interest.
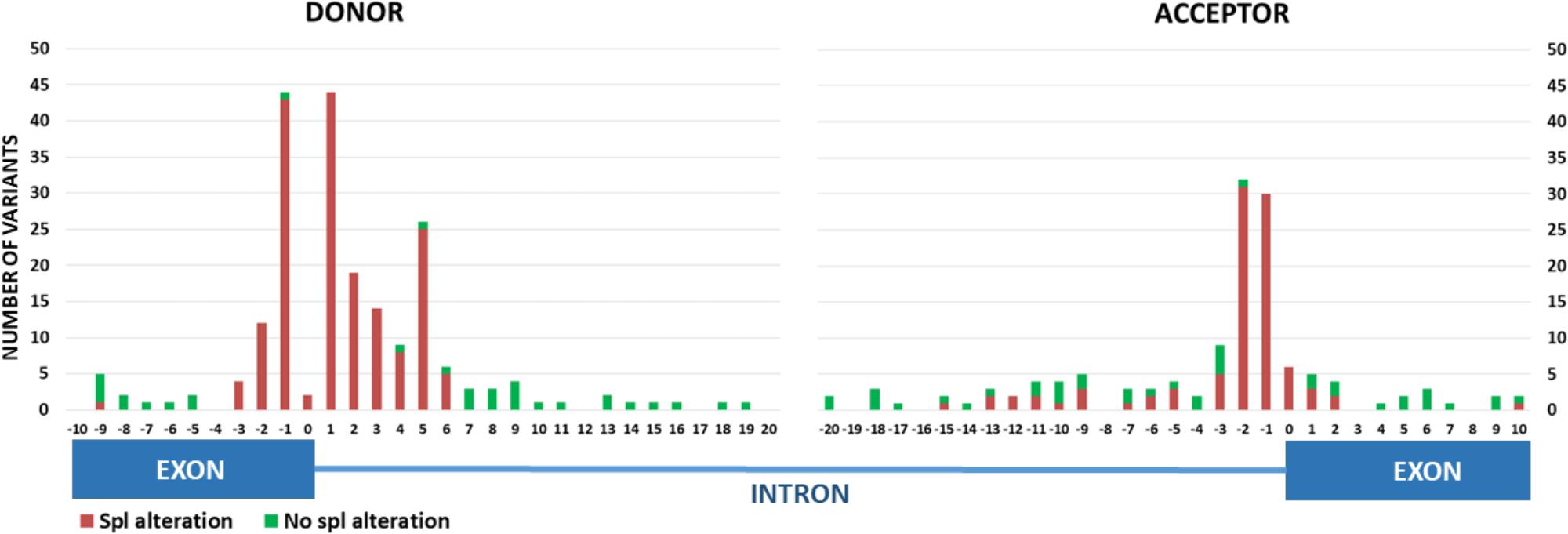
FIGURE 3. In vitro RNA results collected in the validation set. Experimental data are displayed according to variation location. Variants located at 0 position are those that affect the invariable dinucleotide positions (GT or AG) plus other contiguous nucleotides. Positive splicing alterations include: exon skipping, use of a new or cryptic splice site or an altered-alternative transcript profile. Negative splicing alteration: in vitro RNA result was exactly the same as that obtained in control samples. Spl, splicing.
We selected for validation, the HSF stand-alone and the combinations HSF+SSF-like and HSF+SSF-like+MES for 5′donor sites (Supplementary Table 3), and the SSF-like alone and the sequential MES and SSF combination for 3′acceptor sites (Supplementary Table 4), considering sensitivity, accuracy, MCC and NPV scores. We excluded the combinations including SPANR or dbscSNV since they do not provide predictions on insertions and deletions.
Overall, the in silico predictions in the validation dataset were more accurate for variants with effects on donor splice sites than acceptor sites (Table 3 and Figure 4). These findings were in agreement with those results obtained with the discovery set (Table 2).
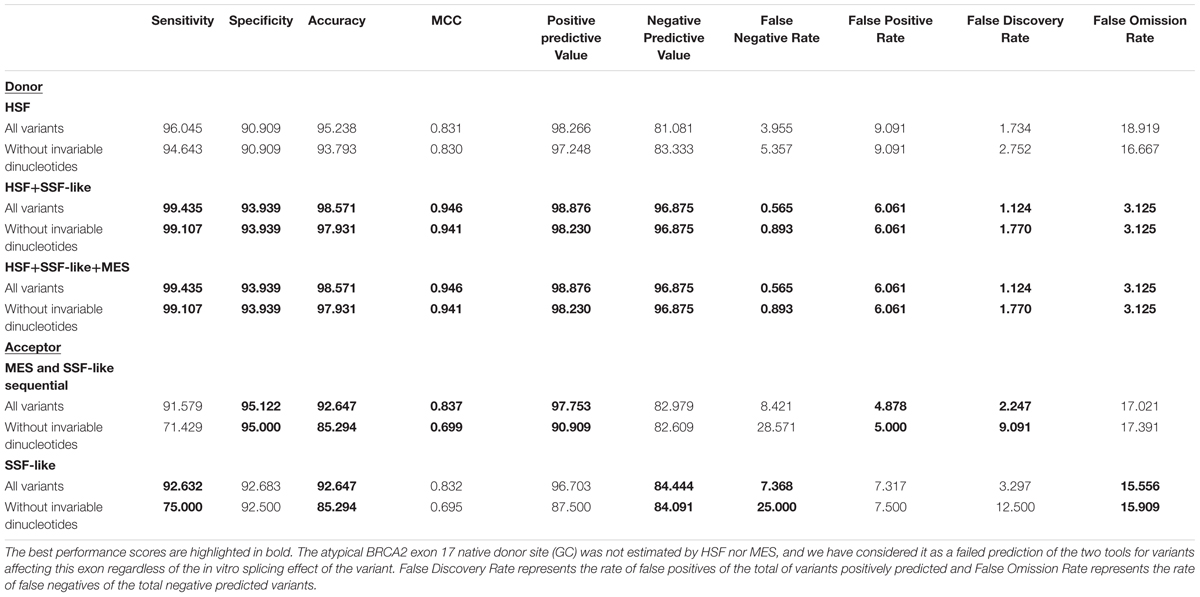
TABLE 3. Performance with the validation dataset of the best in silico tools previously selected from the results at discovery stage.
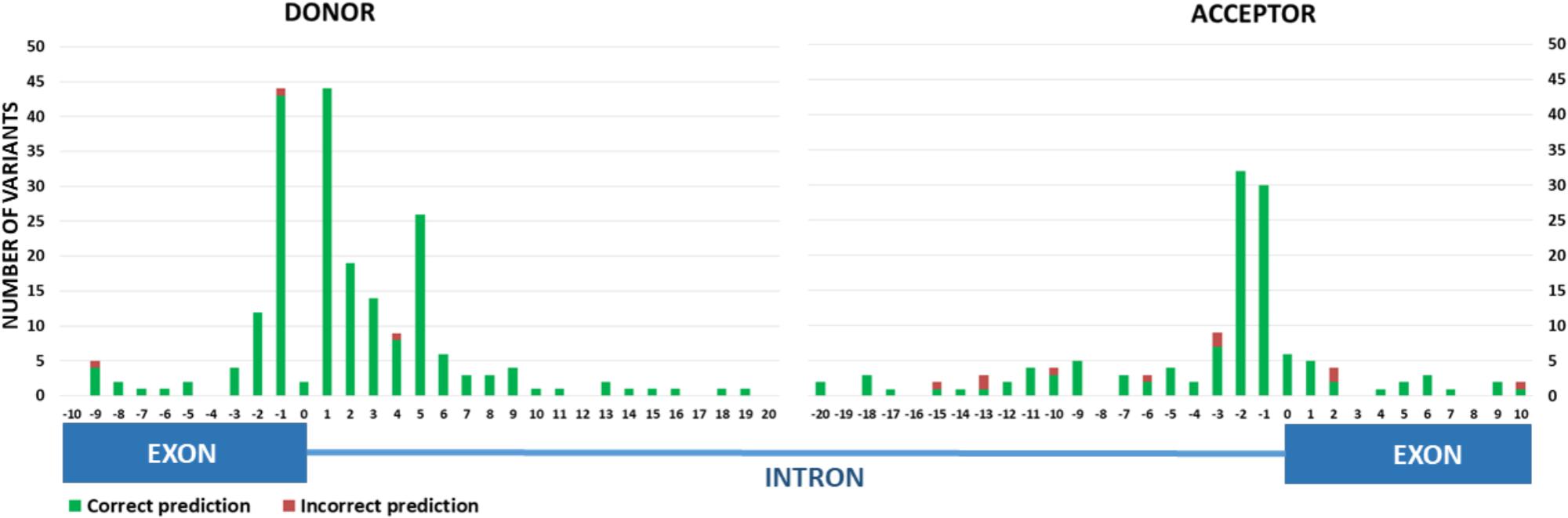
FIGURE 4. Prediction performance of HSF+SSF-like for donor sites and SSF-like for acceptor sites with variants collected in the validation set. Correct prediction: in silico and in vitro results are concordant. Incorrect prediction: in silico and in vitro results are discordant.
The data analysis indicated that for 5′ donor sites the best combinations, with 98.57% accuracy, 99.44% of sensitivity and 96.88% of NPV, are HSF+SSF-like or HSF+SSF-like+MES (Table 3) with very slight differences in performance, between the estimations of splicing effects for all variants (including variants placed at invariable dinucleotides) and for the group of variants located outside the two invariable nucleotides. For acceptor sites, the sequential combination of MES and SSF-like (Houdayer et al., 2012) and SSF-like stand-alone reached a performance with the same score of accuracy, 92.65%, but SSF-like showed a highest NPV (Table 3). Unlike the donor site, the accuracy of these predictors decreased (to 85.29%) when the variants analyzed did not include those at the two nucleotide invariables (AG) of the 3′ acceptor splice site (Table 3). For predictions of variants outside these dinucleotides, the rate of false negatives showed by SSF-like is slightly lower than those rates of MES and SSF-like sequential combination (25% versus 28.57%, respectively, Table 3).
Discussion
The use of massive parallel sequencing in clinical diagnostics is leading to a significant increase in data and the detection of a high number of variants of uncertain significance (VUS) with potential effect on splicing which need interpretation. Therefore, prediction of the effect of DNA sequence variations on splicing using in silico tools has become a common approach. Several studies have been published on the performance and reliability of in silico predictions of the splicing impact of variants (Jian et al., 2014b). Table 1 details the results obtained in these studies and shows that the recommendations provided about the most appropriate to be used are not concordant. However, the studies that give clear recommendations, always include one of the HSF, SSF, or MES programs, alternatively.
We have evaluated the reliability of in silico splicing effect predictions of six programs (MES, HSF, SSF-like, SPANR, NNSplice, and dbscSNV) comparing their scores with splicing in vitro analysis outcomes of variants identified in hereditary cancer related genes. We elaborated the study in two stages, discovery and validation, to identify the best predictors or the best combination for their application in routine clinical testing, taking into account the percentages reached for sensitivity, specificity, accuracy and NPV as well as the score of Mathews Coefficient Correlation (MCC).
In the discovery stage, significant performance differences were appreciated among individual tools (Table 2). For global, as well as for 5′, and 3′ splice sites, low accuracies of SPANR and NNSplice contrasted with the high performance achieved by SSF, MES, and HSF, while dbscSNV demonstrated an intermediate accuracy.
At the second stage of our study, we validated the combinations of HSF with SSF-like or HSF+SSF-like+MES as the highest performance for splicing aberrations at donor sites, and SSF-like stand-alone at acceptor sites (Table 3). All these results are in agreement with the trend observed in the previous published results, where HSF or SSF or MES outperformed other methods (Table 1). Of note, besides high accuracy and sensitivity, these validated tools, combined or as stand-alone, also had high NPV. This is relevant in a clinical setting, since it allows to separate the variants with an extremely low or non-existent probability of being abnormally spliceogenic from those variants in which in vitro RNA studies are of interest, with the consequent saving of resources in the laboratory.
All of the three predictors are available through Alamut Visual 2.10 (Interactive Biosoftware, Rouen), allowing a high throughput analysis, which is essential in a massive parallel sequencing annotation pipeline. Yet, in the newest version of Alamut Visual (2.11) the HSF predictor is not included in its splicing module, it is freely available at Human Splice Finder website3 or through VarAFT software4, which allows the annotation of a large batch of variants. MES program is also freely accessible via web5,6 , although caution should be taken when obtaining predictions via Alamut or via web, since differences have been reported (Tang et al., 2016). SSF-like tool is currently only accessible through Alamut, yet it has been recently published a free program named Splicing Prediction in Consensus Elements (SPiCE7) that combines predictions from SSF-like and MES (Leman et al., 2018). On the other hand, SPANR and dbscSNV are free and could be easily implemented in a pipeline (Xiong et al., 2015; Liu et al., 2017), but these tools are not able to interpret splicing alterations caused by insertion or deletions (6.36% of validation set variants), which represents a limitation for their use compared to the other tools.
Non-canonical GC-AG and AT-AC sequences at the splice site invariant positions occur in 0.56 and 0.09% of the splice site pairs, respectively (Abramowicz and Gos, 2018). In the list of the genes that we analyzed, only six splice sites vary from the canonical splice site GT-AG: ATM exon 50 donor site (GC), BRCA2 exon 17 donor site (GC), MUTYH exon 14 donor site (GC), PALB2 exon 12 donor site (GC), STK11 exon 2 donor site (AT) and exon 3 acceptor site (AC). In our validation dataset, we only had variants at atypical BRCA2 exon 17 donor site (GC), and among the studied tools, only SSF-like and SPANR were able to identify these atypical splicing sites and made a prediction for variants located nearby. As the performance of SSF-like is better than SPANR, we suggest the use of SSF-like to analyze these non-canonical splicing sites.
The tools analyzed in this article have only been interrogated to predict alteration at donor and acceptor splice sites. However, alterations in RNA may be produced by variant effects on other factors in cis (branch points, polypyrimidine tract, intronic and exonic splicing silencers and enhancers) or create new splice sites or activate cryptic ones. At the stage of validation, the rate of false negative predictions is significantly higher for acceptor sites than for donor sites (Table 3). This difference may be due to the greater complexity of the sequence adjacent to the 3′, with the presence of the branch point and the polypyrimidine tract. Therefore, variants located in these two last elements could alter RNA and not be detected as changes in the scores of the splicing sites computed by the predictors. For example, the variant c.1066-6T>G at ATM (included in the validation set), which is not predicted correctly by MES and SSF-like sequential combination (Supplementary Table 7), alters the polypyrimidine tract causing an aberrant transcript (Dörk et al., 2001).
Likewise, the BRCA2 exonic variant c.467A>G, located nine nucleotides upstream from the 5′ donor site, causes the loss of these last nine nucleotides, while the HSF and SSF-like predicts that their scores for the native donor splice site of 88.9 and 84.5, respectively, are not changed by the variant, which it is misinterpreted as a false negative (Supplementary Table 6). Using some of the tools analyzed in our study to identify enhanced cryptic sites or creation of new splice sites, the variant is predicted to cause a new donor site at nine nucleotides from 5′, in concordance with in vitro results: SSF-like indicates a new donor site with a score of 96.9 against 84.5 of the natural splice site, MES 11.1 vs. 9.5 and HSF 98.2 vs. 88.9.
Furthermore, variants located in the exonic regions collected in our study could affect enhancer elements (ESEs) leading to an exon skipping, but they would not be correctly predicted by the analyzed tools. Although variants with specific experimental evidence of suffering this type of alteration were not included in our study, most articles consulted do not explicitly describe or exhaustively exclude the effect of ESEs. As an example, the BRCA1 c.557C>A altering splicing variant gathered at validation set is not predicted to affect native acceptor site by SSF-like, but specific tools to predict splicing defect caused by regulatory sequence disruption indicates an ESE disturbance: ESRseq score of -1.567 (Ke et al., 2011) and HEXplorer ΔHZEI = -30.24 (Erkelenz et al., 2014).
Computational tools or programs able to perform predictions on the disruption of all cis DNA elements would cover the whole landscape of aberrant RNA splicing yielded by spliceogenic VUS. Theoretically, SPANR is able to detect exon skipping caused by all of the elements above mentioned, although our study indicated that this program has a low performance for at least to predict correctly alterations of donor and acceptor sites (Table 2). The HSF predictor accessed via its website8, also predicts the impact of genetic variations on branch point elements and has been improved for the identification of natural non-canonical splice sites (Oetting et al., 2018). The breast cancer genes PRIORS probabilities program9, gives MES estimations of disruption of natural splice sites and also computes the creation of new donor and acceptor splice sites using NNSplice, yet only for BRCA1 and BRCA2 genes (Vallée et al., 2016). However, the accuracy and performance of SPANR, HSF, and PRIORS predictions of variants placed in elements other than natural splice sites has not yet been evaluated.
To our knowledge, our study is the only that evaluates the accuracy of different tools separately for donor and acceptor sites, resulting in different recommendations for each one with high performance (Table 1).
One limitation of our study is the use of splicing in silico tools through a non-free commercial program, Alamut Visual 2.10, with the uncertainty of whether the predictions obtained through Visual Alamut are the same as those estimated directly by the tools in their respective free access websites. We have confirmed that HSF via web (see footnote 8; data not shown) and MES via SPICE (see footnote 7; Supplementary Table 8), at least for native splice sites, provide the same estimations than those provided by Alamut Visual 2.10. However, SSF-like predictions obtained through Alamut Visual 2.10 slightly differ from the predictions ascertained through SPICE (Supplementary Table 8). Therefore and considering our findings, we recommend as a free pipeline to use HSF accessed via web and MES via SPICE for donor and acceptor site predictions, respectively.
Another limitation is the higher number of variants causing splicing defects compared to the number of variants causing no splicing alteration in our validation dataset. This bias is due to a tendency to report only variants that cause splicing defects. Some studies, in order to avoid this bias, have included common single nucleotide polymorphisms (SNPs) from control dataset, assuming that they do not cause alterations (Table 1). Likewise, reports of RNA in vitro effects of variants in the two invariable dinucleotides GT-AG are overrepresented, while those located further from splice junctions are less frequently analyzed.
Conclusion
In conclusion, to perform in silico analysis of VUS potentially affecting natural splice sites in hereditary cancer genes, we recommend the use of the HSF+SSF-like combination (with Δ-2% and Δ-5% as thresholds, respectively) for donor sites and SSF-like (Δ-5%) stand-alone for acceptor sites. These tools have shown in the validation stage a high sensitivity and especially a high NPV. Although the in vitro study of RNA remains the gold standard to evaluate the process of splicing, and it is not recommended to use these predictions as the sole source of evidence to make clinical assertions (Richards et al., 2015), our results indicate that these combined tools can be used to filter out VUS with a very low probability of altering splicing without losing true spliceogenic variants that will need deeper experimental validation. Complementing the analysis using specific predictors to identify variants that could affect elements other than splice sites (such as branch points or ESEs), may be useful for the screening of the whole RNA defect landscape. Lastly, it is worth stating that (i) the aim of this work was not to classify variants but to provide an in silico algorithm with the highest performance to predict an altered in vitro splicing regardless of whether the variants are benign or pathogenic; and (ii) the detection of splicing defect does not automatically denote the pathogenicity of the variant for which a comprehensive qualitative and quantitative RNA analysis is warranted as highlighted in ENIGMA10 or ACGM guidelines (Richards et al., 2015) for variant classification.
Author Contributions
AM-F, LD-L, SG-E, and OD: conception or design of the work. AM-F, LD-L, GM, SB, IL-P, MM, MS, RB, AB, EC, AL-F, NS, and MP: acquisition of data for the work. AM-F, AV, CL, MP, GC, MdH, JB, SG-E, and OD: data analysis and interpretation. AMF, SG-E, and OD: drafting the work. All authors: critical revision of the article and final approval of the version to be published.
Funding
This work was supported by Spanish Instituto de Salud Carlos III (ISCIII) funding, an initiative of the Spanish Ministry of Economy and Innovation partially supported by European Regional Development FEDER Funds: PI15/00355 (to OD), PI16/01218 (to SG-E), PI15/00059 (to MdH), PI16/00563 (to CL), SAF2015-68016-R (to GC and MP), CIBERONC (to GC), INT15/00070, INT16/00154, and INT17/00133 (to AV). The Catalan Institute of Oncology (ICO) work was supported by the Government of Catalonia [Pla estratègic de recerca i innovació en salut (PERIS), 2017SGR1282 and 2017SGR496]; and the Scientific Foundation Asociación Española Contra el Cáncer. ICO thanks CERCA Program/Generalitat de Catalunya for institutional support. This work was partially funded by CIBERER (ER17P1AC7112/2017) and Xunta de Galicia (IN607B) funds given to AV. SG-E and SB were supported by the Miguel Servet Program (CP10/00617) and Asociación Española Contra el Cáncer (AECC) contract, respectively. RB was supported by European Union’s Horizon 2020 research and innovation program under grant agreement No 634935.
Conflict of Interest Statement
The authors declare that the research was conducted in the absence of any commercial or financial relationships that could be construed as a potential conflict of interest.
Acknowledgments
We thank Xavier de la Cruz for helpful discussions and Leo Judkins for English language-editing. We acknowledge the Cellex Foundation for providing research facilities and equipment. We also thank the participating patients and families and all the members of the Units of Genetic Counselling and Genetic Diagnostic the Hereditary Cancer Program of the Catalan Institute of Oncology (ICO-IDIBELL).
Supplementary Material
The Supplementary Material for this article can be found online at: https://www.frontiersin.org/articles/10.3389/fgene.2018.00366/full#supplementary-material
Footnotes
- ^ https://sites.google.com/site/jpopgen/dbNSFP
- ^ http://tools.genes.toronto.edu/
- ^ http://www.umd.be/HSF3/
- ^ https://varaft.eu/
- ^ http://genes.mit.edu/burgelab/maxent/Xmaxentscan_scoreseq.html
- ^ http://genes.mit.edu/burgelab/maxent/Xmaxentscan_scoreseq_acc.html
- ^ https://sourceforge.net/projects/spicev2-1/
- ^ http://www.umd.be/HSF3/
- ^ http://priors.hci.utah.edu/PRIORS/index.php
- ^ https://enigmaconsortium.org/
References
Abramowicz, A., and Gos, M. (2018). Splicing mutations in human genetic disorders: examples, detection, and confirmation. J. Appl. Genet. 59, 253–268. doi: 10.1007/s13353-018-0444-7
Cartegni, L., Chew, S. L., and Krainer, A. R. (2002). Listening to silence and understanding nonsense: exonic mutations that affect splicing. Nat. Rev. Genet. 3, 285–298. doi: 10.1038/nrg775
Colombo, M., Blok, M. J., Whiley, P., Santamariña, M., Gutiérrez-Enríquez, S., Romero, A., et al. (2014). Comprehensive annotation of splice junctions supports pervasive alternative splicing at the BRCA1 locus: a report from the ENIGMA consortium. Hum. Mol. Genet. 23, 3666–3680. doi: 10.1093/hmg/ddu075
Colombo, M., de Vecchi, G., Caleca, L., Foglia, C., Ripamonti, C. B., Ficarazzi, F., et al. (2013). Comparative in vitro and in silico analyses of variants in splicing regions of BRCA1 and BRCA2 genes and characterization of novel pathogenic mutations. PLoS One 8:e57173. doi: 10.1371/journal.pone.0057173
Desmet, F. O., Hamroun, D., Collod-Béroud, G., Claustres, M., and Béroud, C. (2010). “Bioinformatics identification of splice site signals and prediction of mutation effects,” in Research Advances in Nucleic Acids Research, ed. R. M. Mohan (Kerala: Global Research Network), 1–16.
Desmet, F.-O., Hamroun, D., Lalande, M., Collod-Béroud, G., Claustres, M., and Béroud, C. (2009). Human Splicing Finder: an online bioinformatics tool to predict splicing signals. Nucleic Acids Res. 37, 1–14. doi: 10.1093/nar/gkp215
Dörk, T., Bendix, R., Bremer, M., Rades, D., Klöpper, K., Bremer, M., et al. (2001). Spectrum of ATM gene mutations in a hospital-based series of unselected breast cancer patients. Cancer Res. 61, 7608–7615.
Eccles, E. B., Mitchell, G., Monteiro, A. N. A., Schmutzler, R., Couch, F. J., Spurdle, A. B., et al. (2015). BRCA1 and BRCA2 genetic testing-pitfalls and recommendations for managing variants of uncertain clinical significance. Ann. Oncol. 26, 2057–2065. doi: 10.1093/annonc/mdv278
Erkelenz, S., Theiss, S., Otte, M., Widera, M., Peter, J. O., and Schaal, H. (2014). Genomic HEXploring allows landscaping of novel potential splicing regulatory elements. Nucleic Acids Res. 42, 10681–10697. doi: 10.1093/nar/gku736
Holla,ØL., Nakken, S., Mattingsdal, M., Ranheim, T., Berge, K. E., Defesche, J. C., et al. (2009). Effects of intronic mutations in the LDLR gene on pre-mRNA splicing: comparison of wet-lab and bioinformatics analyses. Mol. Genet. Metab. 96, 245–252. doi: 10.1016/j.ymgme.2008.12.014
Houdayer, C., Caux-Moncoutier, V., Krieger, S., Barrois, M., Bonnet, F., Bourdon, V., et al. (2012). Guidelines for splicing analysis in molecular diagnosis derived from a set of 327 combined in silico/in vitro studies on BRCA1 and BRCA2 variants. Hum. Mutat. 33, 1228–1238. doi: 10.1002/humu.22101
Houdayer, C., Dehainault, C., Mattler, C., Michaux, D., Caux-Moncoutier, V., Pagès-Berhouet, S., et al. (2008). Evaluation of in silico splice tools for decision-making in molecular diagnosis. Hum. Mutat. 29, 975–982. doi: 10.1002/humu.20765
Jian, X., Boerwinkle, E., and Liu, X. (2014a). In silico prediction of splice-altering single nucleotide variants in the human genome. Nucleic Acids Res. 42, 13534–13544. doi: 10.1093/nar/gku1206
Jian, X., Boerwinkle, E., and Liu, X. (2014b). In silico tools for splicing defect prediction: a survey from the viewpoint of end users. Genet. Med. 16, 497–503. doi: 10.1038/gim.2013.176
Ke, S., Shang, S., Kalachikov, S. M., Morozova, I., Yu, L., Russo, J. J., et al. (2011). Quantitative evaluation of all hexamers as exonic splicing elements. Genome Res. 21, 1360–1374. doi: 10.1101/gr.119628.110
Leman, R., Gaildrat, P., Gac, G. L., Ka, C., Fichou, Y., Audrezet, M., et al. (2018). Novel diagnostic tool for prediction of variant spliceogenicity derived from a set of 395 combined in silico / in vitro studies: an international collaborative effort. Nucleic Acids Res. doi: 10.1093/nar/gky372 [Epub ahead of print].
Liu, X., Wu, C., Li, C., and Boerwinkle, E. (2017). dbNSFP v3.0: a one-stop database of functional predictions and annotations for human non-synonymous and splice site SNVs. Hum. Mutat. 37, 235–241. doi: 10.1002/humu.22932
Moreno, L., Linossi, C., Esteban, I., Gadea, N., Carrasco, E., Bonache, S., et al. (2016). Germline BRCA testing is moving from cancer risk assessment to a predictive biomarker for targeting cancer therapeutics. Clin. Transl. Oncol. 18, 981–987. doi: 10.1007/s12094-015-1470-0
Oetting, W. S., Béroud, C., Brenner, S. E., Greenblatt, M. S., Karchin, R., and Mooney, S. D. (2018). Methods and tools for assessing the impact of genetic variations. Hum. Mutat. 39, 454–458. doi: 10.1002/humu.23393
Reese, M. G., Eeckman, F. H., Kulp, D., and Haussler, D. (1997). Improved splice site detection in genie. J. Comput. Biol. 4, 311–323. doi: 10.1089/cmb.1997.4.311
Rhine, C. L., Cygan, K. J., Soemedi, R., Maguire, S., Murray, M. F., Monaghan, S. F., et al. (2018). Hereditary cancer genes are highly susceptible to splicing mutations. PLoS Genet. 14:e1007231. doi: 10.1371/journal.pgen.1007231
Richards, S., Aziz, N., Bale, S., Bick, D., Das, S., and Gastier-Foster, J. (2015). Standards and guidelines for the interpretation of sequence variants: a joint consensus recommendation of the American College of Medical Genetics and Genomics and the association for molecular pathology. Genet. Med. 17, 405–424. doi: 10.1038/gim.2015.30
Shapiro, M. B., and Senapathy, P. (1987). RNA splice junctions of different classes of eukaryotes: sequence statistics and functional implications in gene expression. Nucleic Acids Res. 15, 7155–7174. doi: 10.1093/nar/15.17.7155
Soukarieh, O., Gaildrat, P., Hamieh, M., Drouet, A., Baert-Desurmont, S., Frébourg, T., et al. (2016). Exonic splicing mutations are more prevalent than currently estimated and can be predicted by using in silico tools. PLoS Genet. 12:e1005756. doi: 10.1371/journal.pgen.1005756
Tang, R., Prosser, D. O., and Love, D. R. (2016). Evaluation of bioinformatic programmes for the analysis of variants within splice site consensus regions. Adv. Bioinformatics 2016:5614058. doi: 10.1155/2016/5614058
Théry, J. C., Krieger, S., Gaildrat, P., Révillion, F., Buisine, M. P., Killian, A., et al. (2011). Contribution of bioinformatics predictions and functional splicing assays to the interpretation of unclassified variants of the BRCA genes. Eur. J. Hum. Genet. 19, 1052–1058. doi: 10.1038/ejhg.2011.100
Vallée, M. P., Di Sera, T. L., Nix, D. A., Paquette, A. M., Parsons, M. T., Bell, R., et al. (2016). Adding in silico assessment of potential splice aberration to the integrated evaluation of BRCA gene unclassified variants. Hum. Mutat. 37, 627–639. doi: 10.1002/humu.22973
Vreeswijk, M. P., Kraan, J. N., Van Der Klift, H. M., Vink, G. R., Cornelisse, C. J., Wijnen, J. T., et al. (2009). Intronic variants in BRCA1 and BRCA2 that affect RNA splicing can be reliably selected by splice-site prediction programs. Hum. Mutat. 30, 107–114. doi: 10.1002/humu.20811
Whiley, P. J., de la Hoya, M., Thomassen, M., Becker, A., Brandao, R., Pedersen, I. S., et al. (2014). Comparison of mRNA splicing assay protocols across multiple laboratories: recommendations for best practice in standardized clinical testing. Clin. Chem. 60, 341–352. doi: 10.1001/jamasurg.2014.1086
Xiong, H. Y., Alipanahi, B., Lee, L. J., Bretschneider, H., Merico, D., Yuen, R. K. C., et al. (2015). The human splicing code reveals new insights into the genetic determinants of disease. Science 347:1254806. doi: 10.1126/science.1254806
Keywords: hereditary cancer genes, NGS of gene-panel, VUS classification, in silico tools, splicing, RNA alteration
Citation: Moles-Fernández A, Duran-Lozano L, Montalban G, Bonache S, López-Perolio I, Menéndez M, Santamariña M, Behar R, Blanco A, Carrasco E, López-Fernández A, Stjepanovic N, Balmaña J, Capellá G, Pineda M, Vega A, Lázaro C, de la Hoya M, Diez O and Gutiérrez-Enríquez S (2018) Computational Tools for Splicing Defect Prediction in Breast/Ovarian Cancer Genes: How Efficient Are They at Predicting RNA Alterations? Front. Genet. 9:366. doi: 10.3389/fgene.2018.00366
Received: 23 May 2018; Accepted: 22 August 2018;
Published: 05 September 2018.
Edited by:
Paolo Peterlongo, IFOM - The FIRC Institute of Molecular Oncology, ItalyReviewed by:
Rachid Karam, Ambry Genetics, United StatesLogan Walker, University of Otago, New Zealand
Copyright © 2018 Moles-Fernández, Duran-Lozano, Montalban, Bonache, López-Perolio, Menéndez, Santamariña, Behar, Blanco, Carrasco, López-Fernández, Stjepanovic, Balmaña, Capellá, Pineda, Vega, Lázaro, de la Hoya, Diez and Gutiérrez-Enríquez. This is an open-access article distributed under the terms of the Creative Commons Attribution License (CC BY). The use, distribution or reproduction in other forums is permitted, provided the original author(s) and the copyright owner(s) are credited and that the original publication in this journal is cited, in accordance with accepted academic practice. No use, distribution or reproduction is permitted which does not comply with these terms.
*Correspondence: Orland Diez, b2RpZXpAdmhpby5uZXQ= orcid.org/0000-0001-7339-0570 Sara Gutiérrez-Enríquez, c2d1dGllcnJlekB2aGlvLm5ldA== orcid.org/0000-0002-1711-6101
†These authors have contributed equally to this work