- 1Department of Oncology, The First Affiliated Hospital of Zhengzhou University, Zhengzhou, China
- 2Department of Surgery, Anyang Tumor Hospital, Anyang, China
- 3Institute of Medical Information, Chinese Academy of Medical Sciences, Peking Union Medical College, Beijing, China
- 4Comprehensive Cancer Center, Ohio State University, Columbus, OH, United States
- 5Department of Hematology and Oncology, Beth Israel Deaconess Medical Center, Harvard Medical School, Boston, MA, United States
- 6Department of Urology, Gunma University Graduate School of Medicine, Maebashi, Japan
- 7Department of Clinical Medicine, Xinxiang Medical University, Xinxiang, China
- 8Bioinformatics Group, Medcurius Co., Zhejiang, China
- 9Shanghai Institutes for Biological Sciences, Chinese Academy of Science, Shanghai, China
- 10Medical College, Henan Polytechnic University, Jiaozuo, China
Splicing perturbation in cancers contribute to different aspects of cancer cell progression. However, the complete functional impact of cancer-associated splicing have not been fully characterized. Comprehensive large-scale studies are essential to unravel the dominant patterns of cancer-associated splicing. Here we analyzed the genome-wide splicing data in 16 cancer types with normal samples, identified differential splicing events in each cancer type. Then we took a network-based and modularized approach to reconstruct cancer-associated splicing networks, determine the module structures, and evaluate their prognosis relevance. This approach in total identified 51 splicing modules, among which 10/51 modules are related to patient survival, 8/51 are related to progression-free interval, and 5/51 are significant in both. Most of the 51 modules show significant enrichment of important biological functions, such as stem cell proliferation, cell cycle, cell growth, DNA repair, receptor or kinase signaling, and VEGF vessel development. Module-based clustering grouped cancer types according to their tissue-of-origins, consistent with previous pan-cancer studies based on integrative clustering. Interestingly, 13/51 modules are highly common across different cancer types, suggesting the existence of pan-cancer splicing perturbations. Together, modularized perturbation of splicing represents an functionally important and common mechanism across cancer types.
Introduction
Newly transcribed messenger RNAs undergo processing steps such as capping, splicing and polyadenylation to derive mature RNAs for export and translation (Hocine et al., 2010). The splicing process, as accomplished by the spliceosome machine, can produce multiple alternative products, which is a well-known phenomena called alternative splicing (AS) (Lee and Rio, 2015). Since its first discovery in 1977, many classical studies have characterized its widespread participation in biological processes such as cell proliferation, apoptosis, angiogenesis, neuronal functions, and transcriptional regulation (Kelemen et al., 2013). Deregulation of AS also contributes to human diseases and various aspects of cancer development (David and Manley, 2010; Scotti and Swanson, 2016).
To systematically characterize the extensive cancer-associated AS perturbations, it is essential to design effective analytic strategies suitable for the ever-growing cancer genomic datasets, largely from projects such as The Cancer Genome Atlas (TCGA). Several approaches have already been taken previously. One of the most popular approaches is the event-driven approach, which aimed to detect individual events that are correlated with cancer or prognosis (Danan-Gotthold et al., 2015; Dvinge and Bradley, 2015; Shen et al., 2016). A second approach focuses on the splicing machinery side and tries to determine the deregulation of splicing factors in tumors (Sebestyén et al., 2016; Sveen et al., 2016; Seiler et al., 2018). Since these approaches emphasized different aspects of the AS perturbations, several recent studies have been linking the splicing factors and events together to identify AS deregulation and the corresponding functional impacts (Li et al., 2017; Kahles et al., 2018). However, this approach may oversee the vast majority of perturbed splicing events that are not easily explained by the few known regulatory factors (Li et al., 2017). Moreover, these studies essentially relied on single-event analysis, and have missed the inter-event linkages which could be equally important to fully understand cancer-specific AS perturbations. To complement these analyses, a fully network-based approach is needed to capture the concurrent perturbation patterns of cancer-associated AS. In addition, such an approach might also discover more robust AS patterns in one or multiple cancer types.
We carried out an extensive analysis of AS events and their interactions in different cancer types. For each cancer type, a network of cancer-associated events is reconstructed. To uncover the potential modularized control in these splicing networks, a random walk-based community identification algorithm is employed. These analyses have revealed representative splicing modules in each type of cancers, and a number of them are prognosis-relevant and involved in cancer-related functional processes. Finally, our work supports the unique value of an splicing network-based approach in understanding cancer splicing deregulation.
Materials and Methods
Data Sets and Processing
Splicing data have been downloaded from the TCGASpliceSeq database (Ryan et al., 2016). Clinical information is from the GDC TCGA project. Splicing events that failed to be quantified >10% in normal samples or >1% in cancer samples were filtered without further use. Cancer samples with >0.1% missing data were also removed. The remaining missing values were imputed with the Bioconductor impute package.
Network Reconstruction and Module Identification
For each cancer type, Pearson correlation coefficients among splicing events were computed between each pair of the differentially spliced events (Wilcoxon signed-rank test FDR <0.1, |delta PSI|>0.1), and were used as edge weights in the reconstructed undirected graph. The Pons-Lapaty random walk algorithm (step = 4) was used to partition the weighted graph. The identified modules from each cancer type were named according to the order of module sizes (from larger to smaller). So M1 is always at least as large as M2, and M2 at least as large as M3, etc.
Overall and Progression-Free Survival Analysis
Module scores are averaged from all splicing events in each sample, with normal sample PSI values used as references and subtracted. Thus, the score measures how strong the module is perturbed in one cancer sample. To ensure robustness, both the average and median scores have been calculated. Overall survival (OS) and progression-free intervals (PFI) are respectively categorized for testing with module scores. The Kaplan-Meier survival curves are fitted and compared between samples with a higher vs. a lower module score using the Log-rank test. Hazard ratios and confidence intervals are estimated from the Cox proportional regression model. In total, 13 modules were found to be significantly correlated to either OS (10 modules) or PFI (8 modules). Of these, 5 modules were commonly significant in both OS and PFI. In addition, 2 Lung squamous cell carcinoma (LUSC) modules were nearly significant in OS and also included as candidates. Therefore, 15 modules were retained after filtering with OS and PFI analyses.
Functional Enrichment Analyses
Gene ontology (GO) enrichment was used to assess the functional properties of each module. The enrichment was determined by the Fisher's exact test method. For all significant GO terms, careful manual inspection and curation were performed to find the most relevant and biologically important functions, which is often a subset of the significant terms.
Results
Splicing Network-Based Flowchart for Identifying Prognosis-Relevant Splicing Modules
The main analytic flowchart consist of six steps (Figure 1): (1) Collection of annotated events from the TCGASpliceSeq database across 33 cancer types. The splicing classes included are exon skipping (ES), retention of introns (RI), alternative donor (AD), alternative acceptor (AA), mutually exclusive exons (ME), alternative terminator (AT). The numbers of quantified events were found in at least 99% of the samples in each cancer type range from 21129 to 43937 across the cancer types. (2) Differential splicing events between cancer samples and adjacent normal samples were identified for 16 cancer types with at least 10 normal samples. The Wilcoxon signed-rank test was used for testing. The number of differential events obtained ranges from 228 in ESCA to 1133 in LUSC. (3) Reconstruction of splicing network for each cancer type, with Pearson correlation coefficient-based similarity linkages. Pearson and Kendall correlation coefficients showed a good consistency in subsequent community detection, confirming the reliability of this procedure (Figure S1). (4) Network module identification with the Pons-Lapaty algorithm which is based on random walks in 3–5 steps to measure vertex distances for hierarchical clustering and subsequent modularity-optimized graph partition (Pons and Latapy, 2005). (5) Modules are then scored with the averaged splicing deregulation between each cancer sample and the normal samples, which provide a reasonable quantification of module-level perturbation across cancer samples. (6) Prognosis analyses for each module and its corresponding cancer type. Figure 1 shows a schematic diagram of these steps.
Cancer Splicing Networks and Modules for TCGA Cancer Types
We reconstructed splicing networks for each cancer type with at least 10 normal samples on differential events. There are 16 cancer types that bypass the above criteria, namely: Bladder urothelial carcinoma (BLCA), Breast invasive carcinoma (BRCA), Colon adenocarcinoma (COAD), Esophageal carcinoma (ESCA), Head and Neck squamous cell carcinoma (HNSC), Kidney Chromophobe (KICH), Kidney renal clear cell carcinoma (KIRC), Kidney renal papillary cell carcinoma (KIRP), Liver hepatocellular carcinoma (LIHC), Lung adenocarcinoma (LUAD), Lung squamous cell carcinoma (LUSC), Prostate adenocarcinoma (PRAD), Rectum adenocarcinoma (READ), Stomach adenocarcinoma (STAD), Thyroid carcinoma (THCA), and Uterine Corpus Endometrial Carcinoma (UCEC). The number of modules identified with the Pons-Lapaty algorithm varied between 2 and 5 for these cancer types. The visualized inspection revealed quite clear network partitions (Figure 2). In total, 51 modules were identified, and the number of events and genes in each module can be found in Table 1 and Table S1. For example, the KIRC_M1 module consisted of 710 events in 630 genes, with 41 AA events, 36 AD events, 229 AT events, 210 ES events, 3 ME events, and 191 RI events.
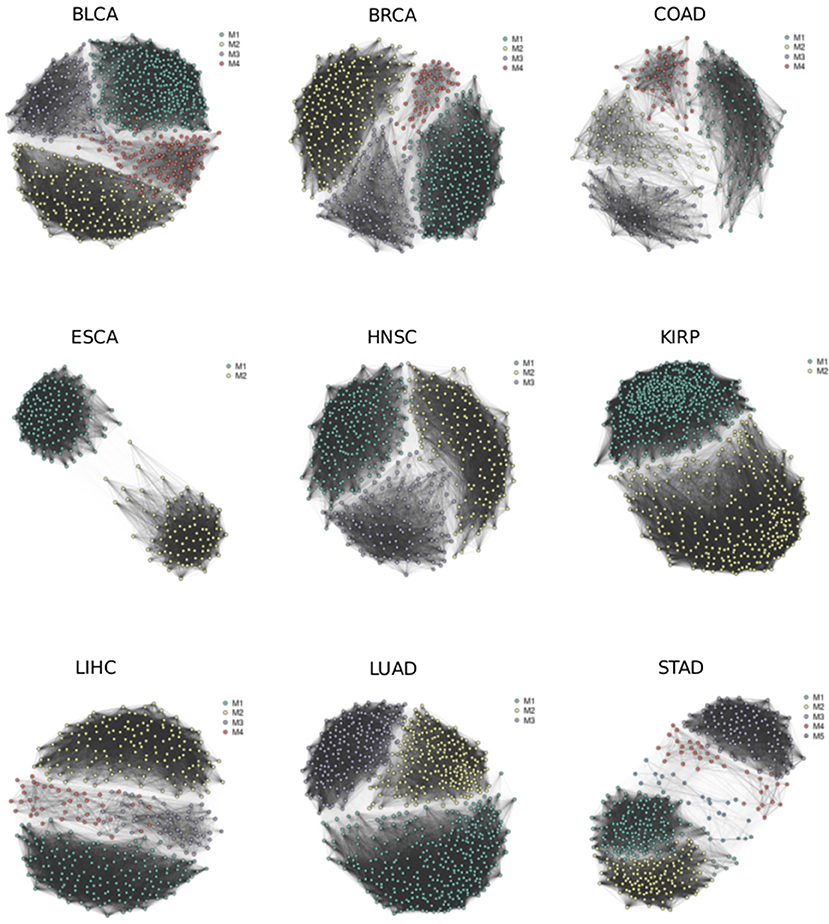
Figure 2. Splicing networks for different cancer types. Modules identified by random walks are highlighted in different colors.
Overall and Progression-Free Survival Analyses for Cancer Splicing Modules
Since the motivation of this study is to discover prognosis-related splicing modules, we quantified module scores in each cancer sample and test associations between module scores and patient survival. Both the average score and median score were computed and assessed for prognosis correlation, and a very good consistency was found (Figure S2), indicating robustness of the module scoring procedure. At a 0.05 significance level, Log-rank tests identified 10 prognosis-related modules: BLCA_M1, BLCA_M2, KIRC_M1, KIRC_M2, LIHC_M1, LIHC_M2, LUAD_M1, LUAD_M3, PRAD_M1, and UCEC_M3 (Table 2, Figure 3). Two additional modules, the LUSC_M2 (P = 0.0595, HR = 0.75 with a confidence interval 0.55–1.01) and the LUSC_M3 (P = 0.099, HR = 0.77 with a confidence interval 0.57–1.05), are close to the significance level, and therefore are still likely to be potential prognosis biomarkers (Figure 3E). Notably, LUSC_M2 contains a ME event on the known LUSC amplification gene FGFR1 (exons 12.1:12.2 vs. exon 13), which could be functionally important in LUSC (Weiss et al., 2010; Heist et al., 2012).
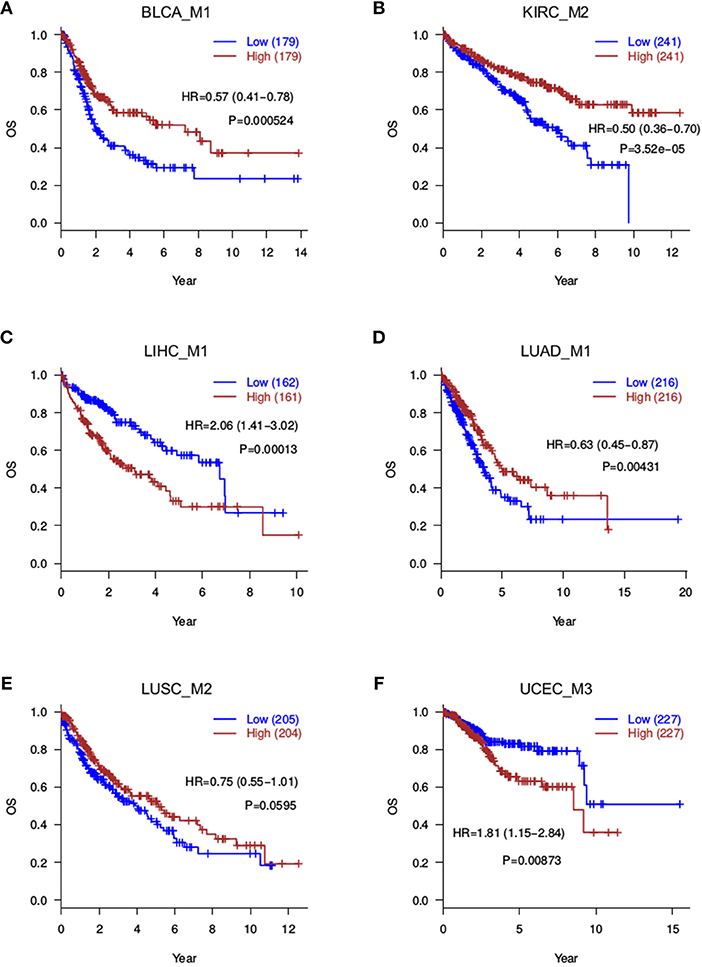
Figure 3. Overall survival analyses for splicing modules. Red curves are with a high module score, and blue curves with a low module score. (A) BLCA_M1; (B) KIRC_M2; (C) LIHC_M1; (D) LUAD_M1; (E) LUSC_M2; (F) UCEC_M3.
Besides overall survival (OS) that reflects a long-term prognosis, it is often of interest to evaluate short-term effects on disease progression. Therefore, to further capture more prognosis-related modules, we also tested the correlation between module scores and progression-free intervals (PFI). This analysis returned 8 significant modules (P ≤ 0.05), namely, BLCA_M1, BLCA_M2, BLCA_M4, LUAD_M3, PRAD_M1, PRAD_M2, THCA_M1, and UCEC_M3 (Table 2, Figure 4). Note that BLCA_M4 is also marginally significant in OS analysis (P = 0.076, HR = 0.75 with a confidence interval of 0.54–1.03), while PRAD_M2 (OS P = 0.29) and THCA_M1 (OS P = 0.7) are only significant in PFI analysis. Five modules are strictly significant in both the OS and PFI settings (BLCA_M1, BLCA_M2, LUAD_M3, PRAD_M1, and UCEC_M3), and interestingly, their HR ratios in these two settings are in a similar trend, either both reducing or both increasing malignancy risks. BLCA_M1 lowers both the death risk (0.57, 0.41–0.78) and the disease progression risk (0.67, 0.48–0.91); BLCA_M2 increases both the death risk (1.78, 1.29–2.48) and the progression risk (1.42, 1.04–1.95); LUAD_M3 also increases both risks (1.86, 1.34–2.58 and 1.42, 1.05–1.93, respectively); PRAD_M1 also increases both risks (6.42, 0.78–52.60 and 1.80, 1.16–2.79, respectively); UCEC_M3 similarly increases both risks (1.81, 1.15–2.84 and 1.66, 1.12–2.47, respectively). These strongly indicate the consistency of splicing modules as potential prognosis biomarkers, suggesting underlying functional involvement of these modules in their corresponding cancer types.
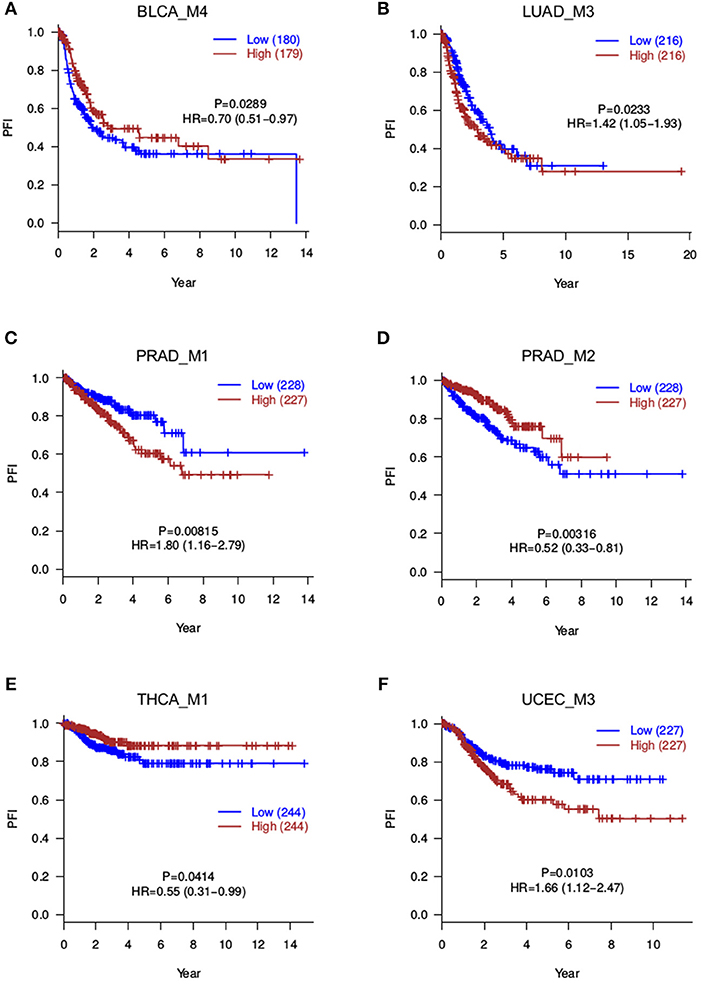
Figure 4. Progression-free survival analyses for splicing modules. Red curves are with a high module score, and blue curves with a low module score. (A) BLCA_M4; (B) LUAD_M3; (C) PRAD_M1; (D) PRAD_M2; (E) THCA_M1; (F) UCEC_M3.
Cancer Splicing Modules Are Enriched for Critical Biological Functions
The above analyses yielded 15 modules with potential prognosis relevance (Table 2). To characterize the functional properties of each splicing module, GO enrichment analysis was performed on the 15 modules. The major functional implications of each module were manually examined and curated from the enrichment results (Table 2). Since nearly all genes transcribed in the genome, including many long non-coding genes, underwent alternative splicing, typically very few events could drive strong functional changes, and the majority of alternative splicing events at most function as weaker modifiers. Surprisingly, we found that the 15 modules, when compared to the splicing events catalog, showed very strong enrichment of important biological functions, such as stem cell proliferation and epithelial-mesenchymal transition (EMT), (BLCA_M1, LIHC_M2, LUSC_M1, THCA_M1, THCA_M2, UCEC_M2), cell cycle control (BRCA_M1, BRCA_M2, COAD_M4, KICH_M1, STAD_M1), DNA repair or regulation (COAD_M3, ESCA_M2, HNSC_M3, KICH_M1, LUAD_M3, LUSC_M3, UCEC_M3), developmental cell growth (BRCA_M4, COAD_M1, LIHC_M1, LIHC_M3, READ_M1, READ_M2, STAD_M2, STAD_M3), receptor or kinase signaling pathways (BLCA_M4, HNSC_M1, KICH_M1, KIRC_M2, KIRP_M1, KIRP_M2, LIHC_M1, LIHC_M2, LUAD_M1, LUAD_M2, LUSC_M1, LUSC_M3, READ_M2), VEGF-mediated vessel development (LUAD_M1, LUSC_M1) (Table 2). Among these major functions, EMT is required for cancer invasion and metastasis, which is closely related to cancer mortalities and prognosis (Singh and Settleman, 2010). The important EMT-related gene modulated in BLCA and LIHC is FGFR2, which regulates mesenchymal condensation in BLCA (Chaffer et al., 2006). Targeting FGFR signaling through splicing factors might expand the current toolkits (Touat et al., 2015). Vessel development controlled by VEGF signaling is another pathway directly involved in cancer metastasis and patient survival (Stacker et al., 2002; Su et al., 2006). Both VEGFA and its receptor FLT4 (VEGFR-3) were altered during splicing in lung cancers LUAD and LUSC, which might modulate angiogenesis through splicing control. In summary, these suggest that the splicing network-based module identification approach taken in this study was powerful enough to extract the few critically functional events from a much larger splicing background.
Splicing Modules Across Cancer Types Reveal Pan-Cancer Signatures
Having obtained those functionally coherent modules, we next asked whether it would be helpful to explore the pan-cancer landscape at the module level. Hierarchical clustering of cancer types with the 51 modules revealed a clear pattern that is closely related to tissue origins (Figure 5A). Lung cancers (LUAD, LUSC), colon cancers (COAD, READ), gynecological cancers (BRCA, UCEC), kidney, and prostate cancers (KIRC, KIRP, KICH, PRAD) each are clustered in a tissue origin manner. This is actually consistent with a recent pan-cancer analysis using multi-platform integrative clustering (Hoadley et al., 2018), suggesting that splicing events can also be useful for cancer classification and subtyping.
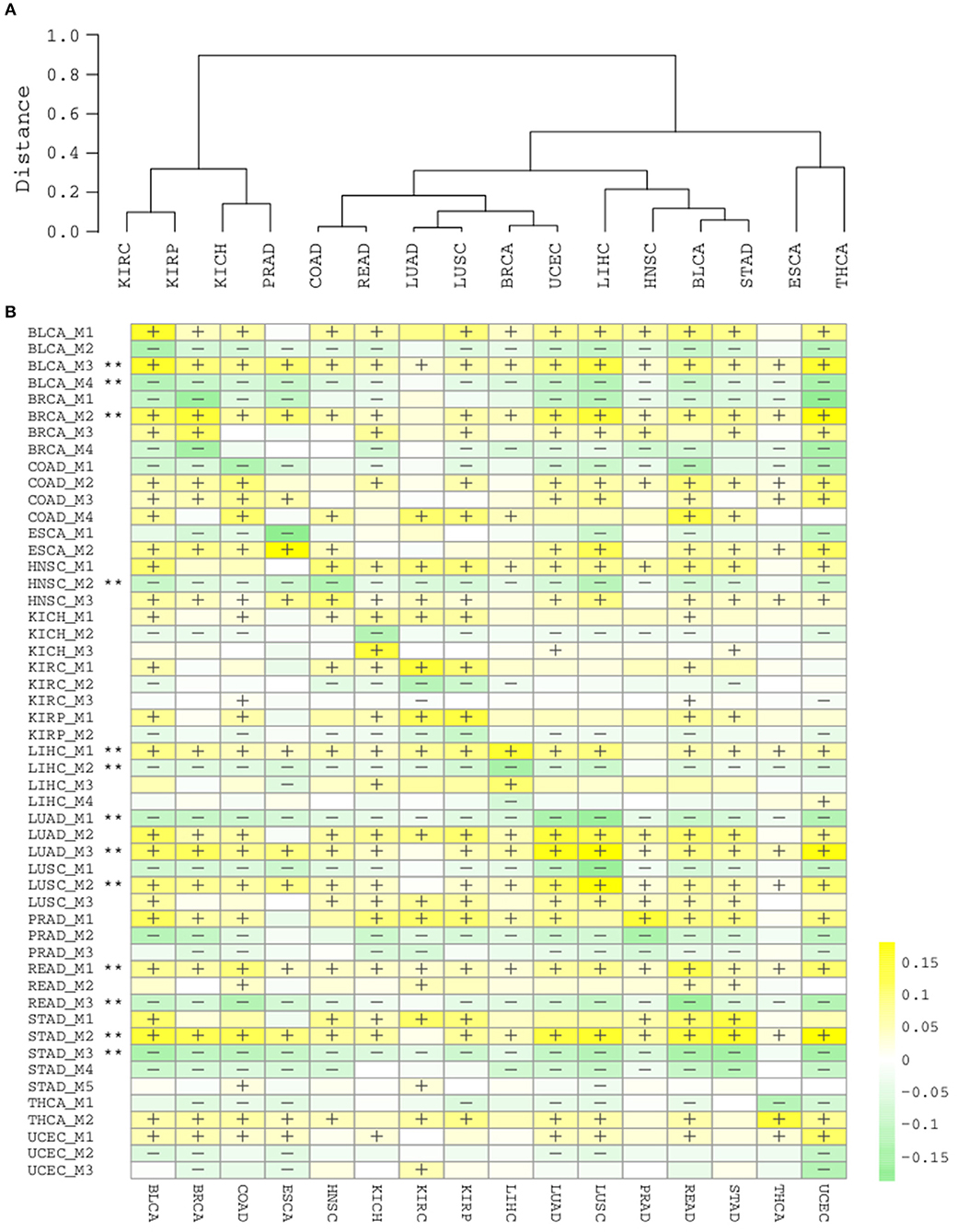
Figure 5. (A) Hierarchical clustering of cancer types by 51 splicing modules. (B) Heatmap of module scores in each cancer type. “+” and “–” respectively denote that for ≥80% samples in the cancer type are with a higher and lower module score than normal samples. “**” denotes the module are consistently “+” or “–“ in ≥15/16 cancer types.
Due to the intra-type and between-type heterogeneity of cancers, it is important to know which of the splicing modules are shared by multiple cancer types and which modulesare restricted to one or few cancer types. We summarized the scores for each module in the cancer samples and categorized them by cancer type (Figure 5B). The diagonal line here reflects the score of modules in their corresponding cancer types, while the off-diagonal regions depicts their pan-cancer potential. Although a few modules from kidney and liver cancers show a strong cancer type specificity, and largely not perturbed in other types (KICH_M3, LIHC_M3, LIHC_M4), many other modules display strong pan-cancer perturbation patterns, suggesting their wider involvement in most cancer types. With a strict criteria (perturbation found in at least 15/16 cancer types), we found that 13/51 modules are highly common across different cancer types (marked with **, Figure 5B), again suggesting that the modules identified with splicing network analysis are highly informative and important.
Discussion
Although AS has been identified and studied for many years, the full regulation pattern of these many AS events within and across cancer types are still not completely understood. Previous studies have taken advantage of single-event analyses and linked splicing to splicing factors as well as the cis-elements. Very recently, an interesting study sets out to determine the involved of spliceosome RNAs in cancer-specific AS regulation (Dvinge et al., 2018).
In this study, we have taken a novel approach that emphasizes the inter-event correlations and uncovers the modularized perturbation of splicing events in cancers. Previous studies have not emphasized the modularized control of splicing events, which according to our study is quite important. Indeed, a relatively small number of functionally important and prognosis-relevant modules have been successfully identified, with some of them being common across cancer types and others being more specific to one or few cancer types, indicating that our approach is both powerful and useful.
To focus on the more typical AS classes, we have not considered alternative promoters in this study, as their regulation are more relevant to transcriptional factors, enhancers or even epigenetic modifications (Maunakea et al., 2010; Kowalczyk et al., 2012). Nonetheless, it would be interesting to investigate the possibility of combining transcriptional events and splicing events in the future, as co-transcriptional splicing has already been proposed and supported by various studies. This might serve as a plausible framework for those interactions (Lee and Rio, 2015).
Data Availability
The datasets used for this study are publicly accessible, and are also available by contacting the corresponding authors.
Author Contributions
WM, TZ, and ZF: conceived and oversaw the study, wrote the manuscript. YD, SL, RD, and YZ: performed the data analysis. NS, SA, SC, and AW: assisted in data analysis and interpretation.
Conflict of Interest Statement
Author YZ was employed by the company Medcurius Co.
The remaining authors declare that the research was conducted in the absence of any commercial or financial relationships that could be construed as a potential conflict of interest.
Acknowledgments
This study was supported by the National Natural Science Foundation of China (No. 31570917, 31400752, 81602459), the Program of China International Medical Foundation (No. CIMF-F-H001-285), the Science and Technology Project of Henan Province (No. 162102310136, 132102310148, 112102310150), the General project of Henan Medical Science and Technology Research Plan (No. 201403067), the Zhengzhou University School Joint Cultivation Fund, the Science and Technology Project of Zhengzhou City (No. 112PPTSF-317-6).
Supplementary Material
The Supplementary Material for this article can be found online at: https://www.frontiersin.org/articles/10.3389/fgene.2019.00246/full#supplementary-material
Figure S1. Comparison of Pearson and Kendall correlation coefficients on network and module detection. NMI (normalized mutual information) and ARI (adjusted Rand index) are used to evaluate consistency.
Figure S2. Consistency between average and median module scores on survival analyses. (Left) OS analysis. (Right) PFI analysis. Each circle or “+” refers to a module.
Table S1. Detailed splicing events within each of the 51 splicing modules. Each event is named as “gene::eventID::eventType”.
References
Chaffer, C. L., Brennan, J. P., Slavin, J. L., Blick, T., Thompson, E. W., and Williams, E. D. (2006). Mesenchymal-to-Epithelial transition facilitates bladder cancer metastasis: role of fibroblast growth factor receptor-2. Cancer Res. 66, 11271–11278. doi: 10.1158/0008-5472.CAN-06-2044
Danan-Gotthold, M., Golan-Gerstl, R., Eisenberg, E., Meir, K., Karni, R., and Levanon, E. Y. (2015). Identification of recurrent regulated alternative splicing events across human solid tumors. Nucl. Acids Res. 43, 5130–5144. doi: 10.1093/nar/gkv210
David, C. J., and Manley, J. L. (2010). Alternative pre-mRNA splicing regulation in cancer: pathways and programs unhinged. Genes Dev. 24, 2343–2364. doi: 10.1101/gad.1973010
Dvinge, H., and Bradley, R. K. (2015). Widespread intron retention diversifies most cancer transcriptomes. Genome Med. 7:45. doi: 10.1186/s13073-015-0168-9
Dvinge, H., Guenthoer, J., Porter, P. L., and Bradley, R. K. (2018). RNA components of the spliceosome regulate tissue- and cancer-specific alternative splicing. bioRxiv [Preprint] 326983. doi: 10.1101/326983
Heist, R. S., Mino-Kenudson, M., Sequist, L. V., Tammireddy, S., Morrissey, L., Christiani, D. C., et al. (2012). FGFR1 amplification in squamous cell carcinoma of the lung. J. Thorac. Oncol. 7, 1775–1780. doi: 10.1097/JTO.0b013e31826aed28
Hoadley, K. A., Yau, C., Hinoue, T., Wolf, D. M., Lazar, A. J., Drill, E., et al. (2018). Cell-of-origin patterns dominate the molecular classification of 10,000 tumors from 33 types of cancer. Cell 173, 291–304.e6. doi: 10.1016/j.cell.2018.03.022
Hocine, S., Singer, R. H., and Grünwald, D. (2010). RNA Processing and Export. Cold Spring Harb. Perspect. Biol. 2:a000752. doi: 10.1101/cshperspect.a000752
Kahles, A., Lehmann, K.-V., Toussaint, N. C., Hüser, M., Stark, S. G., Sachsenberg, T., et al. (2018). Comprehensive analysis of alternative splicing across tumors from 8,705 Patients. Cancer Cell 34, 211–224.e6. doi: 10.1016/j.ccell.2018.07.001
Kelemen, O., Convertini, P., Zhang, Z., Wen, Y., Shen, M., Falaleeva, M., et al. (2013). Function of alternative splicing. Gene 514, 1–30. doi: 10.1016/j.gene.2012.07.083
Kowalczyk, M. S., Hughes, J. R., Garrick, D., Lynch, M. D., Sharpe, J. A., Sloane-Stanley, J. A., et al. (2012). Intragenic enhancers act as alternative promoters. Mol. Cell 45, 447–458. doi: 10.1016/j.molcel.2011.12.021
Lee, Y., and Rio, D. C. (2015). Mechanisms and regulation of alternative pre-mRNA splicing. Ann. Rev. Biochem. 84, 291–323. doi: 10.1146/annurev-biochem-060614-034316
Li, Y., Sahni, N., Pancsa, R., McGrail, D. J., Xu, J., Hua, X., et al. (2017). Revealing the determinants of widespread alternative splicing perturbation in cancer. Cell Rep. 21, 798–812. doi: 10.1016/j.celrep.2017.09.071
Maunakea, A. K., Nagarajan, R. P., Bilenky, M., Ballinger, T. J., D'Souza, C., Fouse, S. D., et al. (2010). Conserved role of intragenic DNA methylation in regulating alternative promoters. Nature 466, 253–257. doi: 10.1038/nature09165
Pons, P., and Latapy, M. (2005). Computing Communities in Large Networks Using Random Walks (Long Version). arXiv:physics/0512106. Available online at: http://arxiv.org/abs/physics/0512106 (Accessed May 5, 2016).
Ryan, M., Wong, W. C., Brown, R., Akbani, R., Su, X., Broom, B., et al. (2016). TCGASpliceSeq a compendium of alternative mRNA splicing in cancer. Nucleic Acids Res. 44, D1018–D1022. doi: 10.1093/nar/gkv1288
Scotti, M. M., and Swanson, M. S. (2016). RNA mis-splicing in disease. Nat. Rev. Genet. 17, 19–32. doi: 10.1038/nrg.2015.3
Sebestyén, E., Singh, B., Miñana, B., Pagès, A., Mateo, F., Pujana, M. A., et al. (2016). Large-scale analysis of genome and transcriptome alterations in multiple tumors unveils novel cancer-relevant splicing networks. Genome Res.115:gr.199935. doi: 10.1101/gr.199935.115
Seiler, M., Peng, S., Agrawal, A. A., Palacino, J., Teng, T., Zhu, P., et al. (2018). somatic mutational landscape of splicing factor genes and their functional consequences across 33 cancer types. Cell Rep. 23, 282–296.e4. doi: 10.1016/j.celrep.2018.01.088
Shen, S., Wang, Y., Wang, C., Wu, Y. N., and Xing, Y. (2016). SURVIV for survival analysis of mRNA isoform variation. Nat. Commun. 7:11548. doi: 10.1038/ncomms11548
Singh, A., and Settleman, J. (2010). EMT, cancer stem cells and drug resistance: an emerging axis of evil in the war on cancer. Oncogene 29, 4741–4751. doi: 10.1038/onc.2010.215
Stacker, S. A., Achen, M. G., Jussila, L., Baldwin, M. E., and Alitalo, K. (2002). Metastasis: lymphangiogenesis and cancer metastasis. Nat. Rev. Cancer 2, 573–583. doi: 10.1038/nrc863
Su, J.-L., Yang, P.-C., Shih, J.-Y., Yang, C.-Y., Wei, L.-H., Hsieh, C.-Y., et al. (2006). The VEGF-C/Flt-4 axis promotes invasion and metastasis of cancer cells. Cancer Cell 9, 209–223. doi: 10.1016/j.ccr.2006.02.018
Sveen, A., Kilpinen, S., Ruusulehto, A., Lothe, R. A., and Skotheim, R. I. (2016). Aberrant RNA splicing in cancer; expression changes and driver mutations of splicing factor genes. Oncogene 35, 2413–2427. doi: 10.1038/onc.2015.318
Touat, M., Ileana, E., Postel-Vinay, S., Andr,é, F., and Soria, J.-C. (2015). Targeting FGFR signaling in cancer. Clin Cancer Res. 21, 2684–2694. doi: 10.1158/1078-0432.CCR-14-2329
Keywords: alternative splicing, splicing network, splicing modules, cancer splicing, prognosis
Citation: Du Y, Li S, Du R, Shi N, Arai S, Chen S, Wang A, Zhang Y, Fang Z, Zhang T and Ma W (2019) Modularized Perturbation of Alternative Splicing Across Human Cancers. Front. Genet. 10:246. doi: 10.3389/fgene.2019.00246
Received: 20 November 2018; Accepted: 05 March 2019;
Published: 03 April 2019.
Edited by:
Chuan Lu, Aberystwyth University, United KingdomReviewed by:
Igor B. Rogozin, National Institutes of Health (NIH), United StatesSandeep Kumar Dhanda, La Jolla Institute for Immunology (LJI), United States
Copyright © 2019 Du, Li, Du, Shi, Arai, Chen, Wang, Zhang, Fang, Zhang and Ma. This is an open-access article distributed under the terms of the Creative Commons Attribution License (CC BY). The use, distribution or reproduction in other forums is permitted, provided the original author(s) and the copyright owner(s) are credited and that the original publication in this journal is cited, in accordance with accepted academic practice. No use, distribution or reproduction is permitted which does not comply with these terms.
*Correspondence: Zhaoyuan Fang, ZmFuZ3poYW95dWFuQHNpYnMuYWMuY24=
Tengfei Zhang, ZmNjemhhbmd0ZkB6enUuZWR1LmNu
Wang Ma, ZG9jdG9ybWF3YW5nQDEyNi5jb20=
†These authors have contributed equally to this work