- Quantitative Genomics, Bioinformatics and Computational Biology Group, Department of Applied Mathematics and Computer Science, Technical University of Denmark, Kongens Lyngby, Denmark
Epigenetic changes are important for understanding complex trait variation and inheritance in pigs that are also a valuable biomedical model for human health research. Testis is the main organ for reproduction and boar taint in pigs; however, there have been no studies to-date on adult pig testis epigenome. The main objective of this study was to establish a genome-wide DNA methylation map of pig testis that would help identify candidate epigenetic biomarkers and methylated genes for complex traits such as male reproduction, fertility or boar taint. Reduced Representation Bisulfite Sequencing (RRBS) was used to study methylation levels of cytosine in nine pig testis samples. The results showed that genome-wide methylation status of nine samples overlapped greatly and their variation among pigs were low. The methylation levels of promoter, exon, intron, cytosine and guanine dinucleotide (CpG) islands and CpG island shores regions were 0.15, 0.47, 0.55, 0.39, and 0.53, respectively. Cytosines binding to CpG islands showed different methylation levels between exon and intron regions. All methylation levels of CpG islands were lower than CpG island shores in different genic features. The distribution of 12,738 differentially methylated cytosines (DMCs) within CpG islands, CpG island shores and other regions was 36.86, 21.65, and 41.49%, respectively, and was 0.33, 1.71, 5.95, and 92.01% in promoter, exon, intron and intergenic regions, respectively. Methylation levels of DMCs in promoter, exon and intron regions were significantly different between CpG islands and CpG island shores (P < 0.05). A total of 898 genes with 2089 DMCs were enriched in 112 Gene Ontology (GO) terms. Fifteen methylated genes from our study were associated with fertility or boar taint traits. Our analysis revealed the methylation patterns in different genic features and CpG island regions of testis in pigs, and summarized several candidate genes associated with DMCs and the involved GO terms. These findings are helpful to understand the relationship between DNA methylation and genic CpG islands, to provide candidate epigenetic regions or biomarkers for pig production and welfare and for translational epigenomic studies that use pigs as an animal model for human research.
Introduction
Pig is a valuable biomedical model of human obesity and metabolic diseases due to the anatomic, biochemical, pharmacological, pathological, and physiological similarities to the human (Kogelman et al., 2013; Kogelman and Kadarmideen, 2016). The previous study showed that the key role of epigenetic mechanisms in male gamete could widely affect human reproduction (Stuppia et al., 2015). Testis is the reproductive gland to produce sperm, so studying epigenetics of testis in pigs could improve our understanding of epigenetic molecular mechanisms related to male fertility and semen quality. Testis epigenome is also essential for the study of inheritance of boar taint in pigs – an unpleasant smell originating from cooking pork meat from uncastrated male pigs that is inherited (Strathe et al., 2013). Epigenetics is defined as changes in gene function that are heritable and no change in DNA sequence (Wu and Morris, 2001). As a major epigenetic modification, DNA methylation has been examined to be associated with growth (Jin et al., 2014), immune response (Wang et al., 2017), and reproduction traits (Bell et al., 2011) in pigs.
With high density of DNA methylation of cytosine and guanine dinucleotides (CpGs), CpG islands play an important role in gene regulation and transcriptional repression (Goldberg et al., 2007). The genome around the CpG islands can be widely affected by the methylation levels (Long et al., 2017). CpG island shores are strongly related to a specific tissue and are involved in modulating gene expression (Doi et al., 2009; Irizarry et al., 2009b). Most variable regions in terms of methylation such as methylation differences between tissues are CpG island shores rather than CpG islands themselves (Irizarry et al., 2009a; Hansen et al., 2011). DNA methylation in promoters is usually restricted to genes in a long-term stabilization of repressed states; therefore, promoter methylation can be a methylation inhibitor of therapeutic targets to silence genes (Yang et al., 2014). Most gene bodies are CpG-poor and extensively methylated, but their methylation can be a potential therapeutic target. Since DNA demethylation of the gene bodies could cause the down-regulation, so DNA methylation inhibitors can down regulate oncogenes and metabolic genes (Jones, 2012; Yang et al., 2014).
Reduced representation bisulfite sequencing (RRBS), based on next generation sequencing (NGS) technology, has been implemented to analyze patterns of DNA methylation by reducing the portion of the genome digestion (Meissner et al., 2005). Subsequently, reduced representation CpG sites are sequenced after restriction enzyme MspI digestion in CpG islands, promoters and enhancers (Smith et al., 2009). The RRBS method primarily focuses on the enrichment of CpG-rich regions rather than the non-CpG regions (Meissner et al., 2005). In mammals, DNA methylation almost exclusively occurs at CG dinucleotides with ratios of 70–80% throughout the genome (Ehrlich et al., 1982; Law and Jacobsen, 2010). Therefore, the information of CpG islands and gene-associated CpG sites can be provided by RRBS method (Choi et al., 2015). Currently, RRBS analysis of the pigs has been presented using intestinal tissue (Gao et al., 2014), ovaries (Yuan et al., 2016), and neocortex, liver, muscle and spleen (Choi et al., 2015).
Genome-wide DNA methylation patterns in porcine ovaries and porcine prepubertal testis have been profiled (Yuan et al., 2016; Chen et al., 2018), but to the best of our knowledge, genome-wide NGS-based methylation studies on adult testis epigenome in pigs have not been reported. The main objective of this study was to develop a map of DNA methylome for porcine testis using RRBS on nine testis samples of pigs and then characterize their methylome using bioinformatics methods. We characterized porcine adult testis epigenome by reporting the methylation levels and patterns in genic features and CpG islands for each testis sample. We identified differentially methylated cytosine (DMC) in nine sample to find DMC associated genes, and their involved Gene Ontology (GO) terms and pathways in pigs. Finally, we compared our results with other similar studies and provided a list of 15 candidate epigenetic biomarkers associated with male fertility (e.g., infertility, litter size, number of stillborn, and so on), boar taint (Skatole, Androstenone) and other complex traits linked to testis of pigs.
Materials and Methods
Pig Samples
Nine commercial purebred Landrace male pigs with similar genetic background from nine different sire families were raised by the same ad libitum feeding of same feed type in the same farm/environment. All pigs were slaughtered at an age of around 22 weeks by carbon dioxide (CO2) submersion at a commercial slaughterhouse (Danish Crown, Herning, Denmark), when they reached the slaughter weight of 105 kg. Testis tissue samples were retrieved by punch biopsy into the middle part of the testis with an inner punch distance of 2 cm. Thus, all of the testis samples were collected from the same part of the testis. Each sample weighed approximately 150 mg. These pigs were not treated by immunological castration or other castrating processes during the feeding period, so they had intact testis with normal fertility and viable sperms before or at slaughter.
Tissue samples were immediately immersed into the 1.5 ml RNAlater (QIAGEN, Hilden, Germany). All samples were stored at -20°C. Restriction enzyme digestion, adaptor ligation, size selection (40–220 bp fragments), bisulfite treatment, polymerase chain reaction (PCR) amplification and library construction were performed at BGI (Beijing Genomics Institute) Co., Ltd., Shenzhen, Guangdong, China. The nine samples were sequenced by a paired-end 100 bp flow cell in an Illumina HiSeq 2500 machine (PE-100bp FC; Illumina, San Diego, CA, United States) using RRBS method.
Quality Control, Read Alignment, and Trimming
RRBS adapters and reads less than 20 bases long were trimmed by Trimmomatic software (version 0.36) (Bolger et al., 2014). Then, Bismark Bisulfite Mapper (version 0.19.0) (Krueger and Andrews, 2011) was applied to map clean reads to the porcine reference genome (Sscrofa11.1/susScr11) downloaded from the UCSC website1, and the cytosine methylation status was determined accordingly. Bismark Bisulfite Mapper includes three steps: genome preparation, alignment using Bowtie 2 (version 2.3.3.1) (Langmead and Salzberg, 2012) and methylation extractor. Bismark methylation extractor outputs read coverage and methylation percentage of detected methylated or unmethylated reads at one genomic position. The numbers of methylated and unmethylated CpG and non-CpG (CHG and CHH, H representing A/C/T) sites were also calculated for each sample. The read coverages lower than 10 counts were trimmed for discarding the unqualified reads. If an experiment suffered from PCR duplication bias, some clonal reads will impair accurate determination of methylation. Thus, cytosines with a percentile of read coverage higher than the 99.9th were also discarded for each sample.
Genome-Wide DNA Methylation Levels and Methylation Patterns
The relationships of genome-wide methylation levels with densities of CpG islands, CpG island shores and genes were calculated through regression and correlation analysis, and counted by one mega base pairs (Mb) windows for each sample. Similarities and differences of genome structure, CpG islands and methylation level between genomic intervals were visualized by R package RCircos (version 1.2.0) (Zhang et al., 2013). Genic features were divided into promoter, exon and intron regions along the porcine genome. Afterward, we localized CpG islands and CpG island shores to these three genic features and investigated methylation patterns of genic CpG islands. Methylation patterns of CpG islands located at different genic features were visualized by R package plot3D.
Differentially Methylated Cytosine (DMC) and Annotation
Methylation levels of cytosines were analyzed by the R package methylKit (version 1.4.0) (Akalin et al., 2012) based on the Bismark coverage file. Genome-wide cytosine sites were combined into one object to obtain the locations covered in all nine samples. In this study, methylation level of nine samples were considered as nine treatment levels in the logistic regression model to calculate P-values, which were then adjusted to Q-values using false discovery rate (FDR) to account for multiple hypothesis testing (Storey and Tibshirani, 2003). Chi-squared (χ2) test was used to determine the statistical significance of methylation differences between samples. Finally, we matched all DMCs into one file that included chromosomes, positions, P-values, Q-values, associated genes and their genic features, positions of CpG islands and CpG island shores and methylation levels of nine samples.
In this study, we defined CpG islands as a region with at least 200 bp, a GC fraction more than 0.5 and an observed-to-expected ratio of CpG more than 0.6. CpG island shores were then defined as regions of 2 kilo base pairs (kb) in length adjacent to CpG islands (Gardiner-Garden and Frommer, 1987). The CpG and DMC annotation within gene components of promoter, exon, intron and intergenic regions, and CpG islands, CpG island shores and other regions was performed using R package genomation (version 1.10.0) (Akalin et al., 2015). The porcine RefSeq and CpG island database (Sscrofa11.1/susScr11) for annotations were derived from the UCSC website2.
Gene Ontology (GO) Enrichment and Pathway Analysis
GO enrichment and pathway analysis were analyzed in DAVID (Database for Annotation, Visualization and Integrated Discovery) Bioinformatics Resources 6.83. NCBI reference sequences associated with DMCs were used in DAVID for the species of Sus scrofa. Significant GO terms and pathways were selected after filtering with P < 0.01. GO terms for the genes associated with DMCs were visualized by R package GOplot (version 1.0.2) (Walter et al., 2015).
Results
Statistics of Alignment With Porcine Reference Genome
In this study, bisulfite conversion efficiencies of these nine samples ranged from 98 to 99%. The RRBS sequencing generated approximately 59,328,166 read pairs per sample. On average, 58,604,646 read pairs survived the pre-processing step. The 49% of the remaining read pairs was uniquely aligned to the porcine reference genome. The reads pairs were located in 9,006,052 sites, which meant that the average depth of RRBS sequencing reads and uniquely aligned reads were approximately equal to 13 and 6.5, respectively (Table 1). A total of 871,462,976 averaged cytosines were analyzed from 28,944,768 uniquely aligned reads pairs including methylated and unmethylated cytosines in CpG/CHG/CHH contexts (Supplementary Table S1). It revealed that a paired-end 100 bp read evenly contained 30 analyzed cytosines. Additionally, a per-sample CpG methylation rate ranged from 46 to 53%. The per-sample average percentages of cytosine methylation rate in CHG and CHH sites were 0.89 and 0.63%, respectively (Table 1).
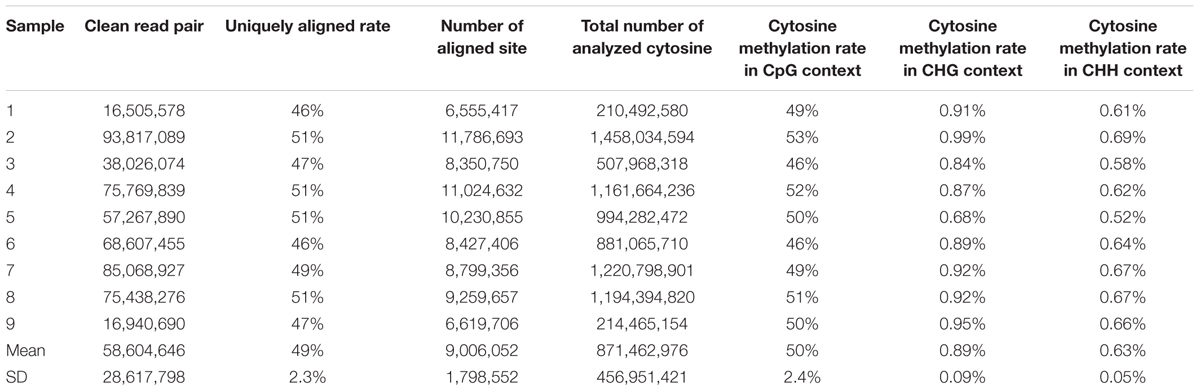
Table 1. Statistics of clean reads’ alignment with porcine reference genome (Sscrofa11.1/susScr11) and methylation rates in CpG, CHG, and CHH contexts.
It was obvious that the number of CpG sites was different at read coverage below 10, thus, the trimming criterion for read coverage was set at 10 (Figure 1A). Figure 1B revealed that the CpG site numbers of sample 1 and sample 9 were lower than the average value, while sample 5 has more CpG sites after trimming. Approximately, 9 million CpG sites were generated in each sample with read coverage equal to 21 (Figure 1C). After discarding coverage both lower than 10 and higher than 99.9th percentile, the averaged read coverage increased from 21 to 34, and the number of CpG sites reduced to a half (Figure 1C). The details of read coverages and methylation rates in CpG context of nine samples are listed in Supplementary Table S2. In addition, the coverage distributions per cytosine of nine samples after trimming are shown in Supplementary Figure S1. The percent methylation distributions per cytosine of nine samples after trimming were shown through histograms on the diagonal of Supplementary Figure S2.
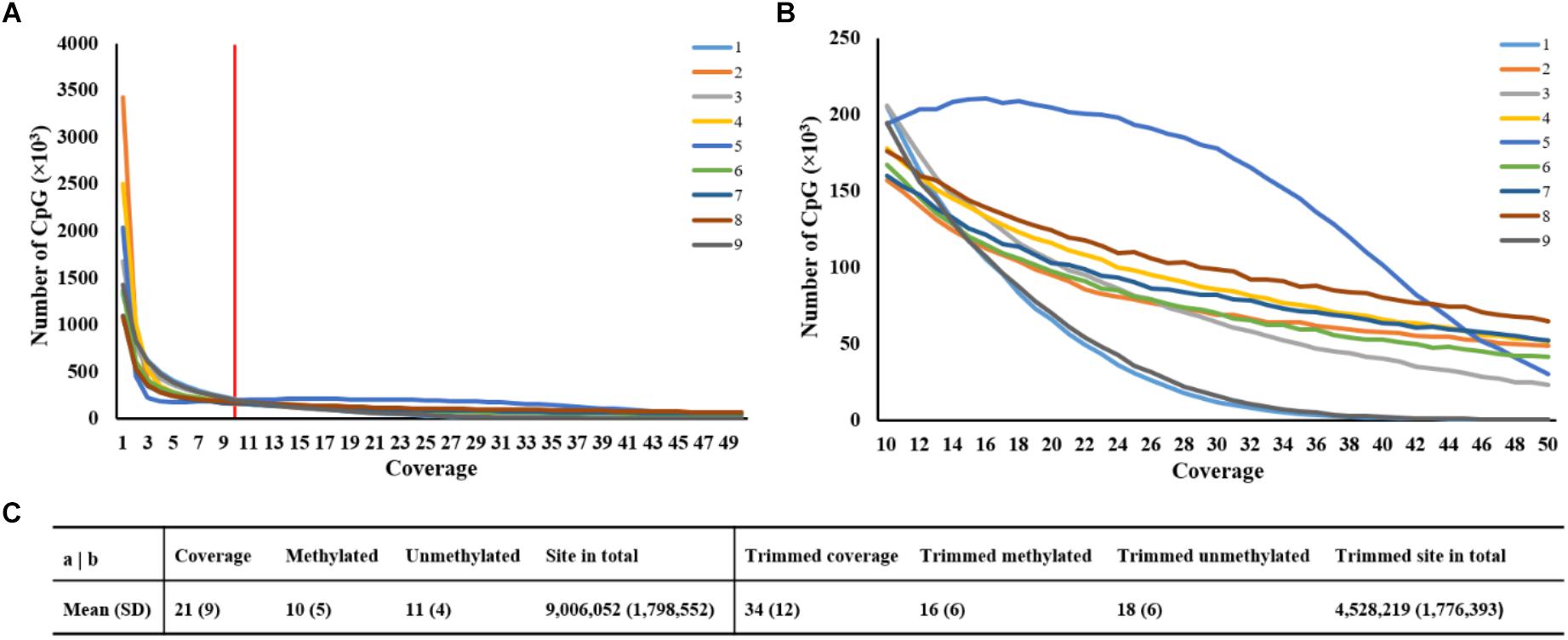
Figure 1. Statistics of averaged coverage in CpG context. (A) Number of CpG sites at different coverage of original data. Note: Red line indicated the coverage at 10. (B) Number of CpG sites at different coverage of trimmed data. (C) Comparison of statistics of averaged coverage between original and trimmed data.
Genome-Wide DNA Methylation Status
The methylation levels against densities of CpG islands, CpG island shores and genes are shown in Figure 2. The genome-wide methylation status of nine samples showed the same trends and they overlapped greatly, suggesting that the biological variation between nine samples was low. Our analysis showed that the global CpG methylation rate was similar among the nine samples with Pearson’s correlation scores ranging from 0.95 to 0.98 (Supplementary Figure S2). The methylation levels varied across the different chromosomes with higher methylation variation in regions of low gene abundance, whereas lower methylation variation in those of high gene abundance (Figure 2). The regression coefficients of densities of genes, CpG islands and CpG island shores on methylation level were -2.20 (P < 0.001), 59.04 (P < 0.001), and 73.65 (P < 0.001), respectively, on average, over nine samples (Supplementary Figure S3 and Supplementary Table S3). The correlations between methylation levels and densities of genes, CpG islands and CpG island shores were -0.12, 0.25, and 0.23, respectively (Supplementary Table S3). These results suggested that genome hypomethylation in CpG islands was beneficial for the promotion of gene transcription, but their correlations were not so high.
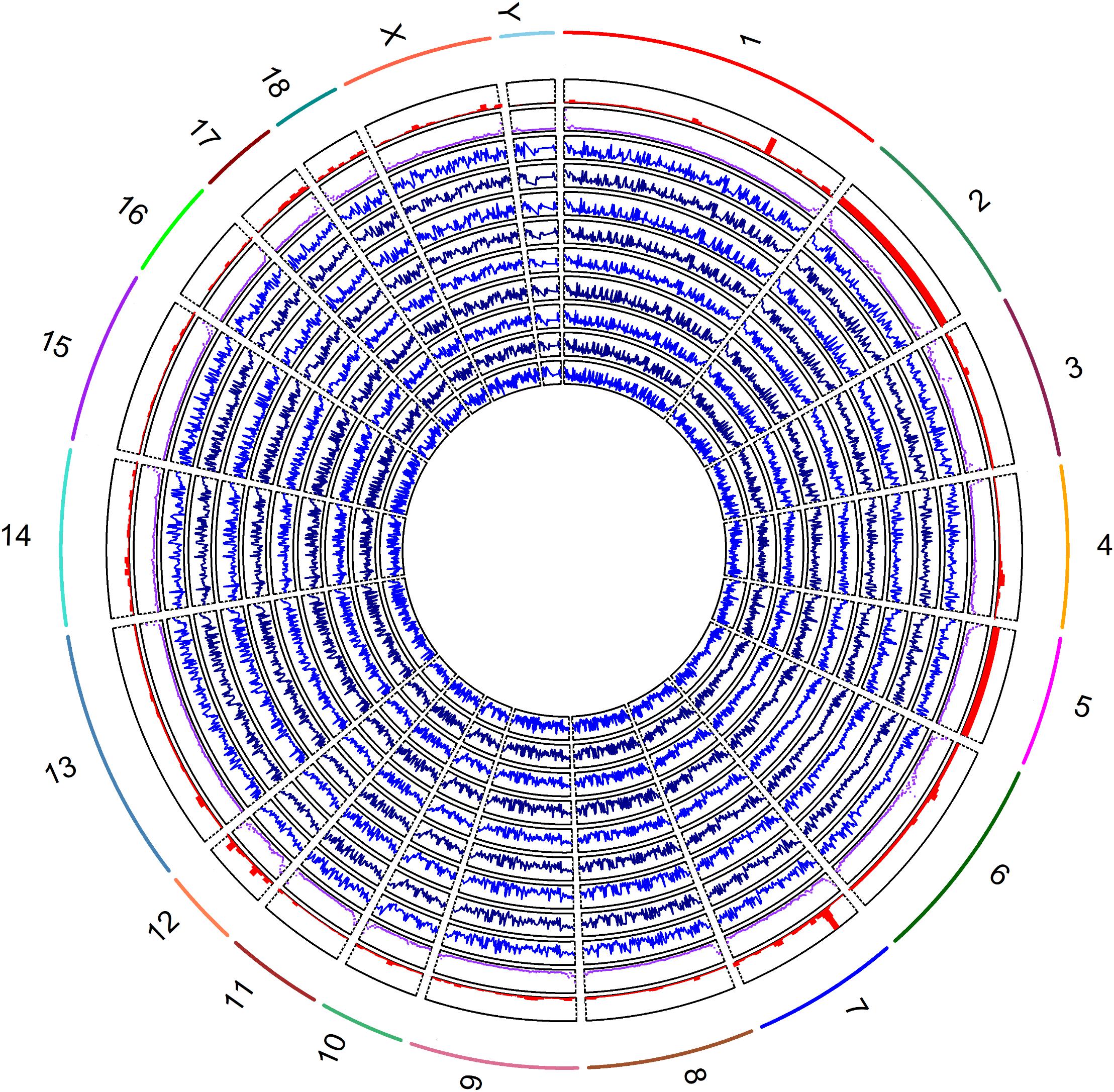
Figure 2. Global methylation levels of nine samples was shown by lines of in blue (track 1, 2, 3, 4, 5, 6 7, 8, and 9) from inside to outside. The methylation levels and the densities of CpG islands by scatter plot in purple color (track 10), and genes by histograms in red color (track 11) were counted by 1 Mb windows. The labels of outside track represented the chromosomes of the porcine genome.
Methylation Patterns of CpG Islands Located at Different Genic Features
To investigate the interaction of methylation levels between genes and CpG islands, we divided the porcine genome into three genic features (promoters, exons, and introns) and then localized CpG islands to these genic features. Methylation levels at different genic features and CpG islands displayed variously, with lowest values in the promoter regions. The methylation level were 0.15, 0.47, 0.55, 0.39, and 0.53 in the promoter, exon, intron, CpG islands, and CpG island shores regions, respectively, on average, over nine samples (Figure 3A). Comparisons of CpG islands and CpG island shores at different genic features revealed that the methylation levels of promoter regions were also the lowest. Meanwhile, CpG island shores located in intron regions showed slightly higher methylation levels than those located in exon regions, while CpG islands showed significant higher methylation levels (Figures 3B,C). Comparing with the methylation patterns in three different genic features, methylation levels of CpG islands were all lower than CpG island shores in the promoter, exon, and intron regions (Figures 3D–F).
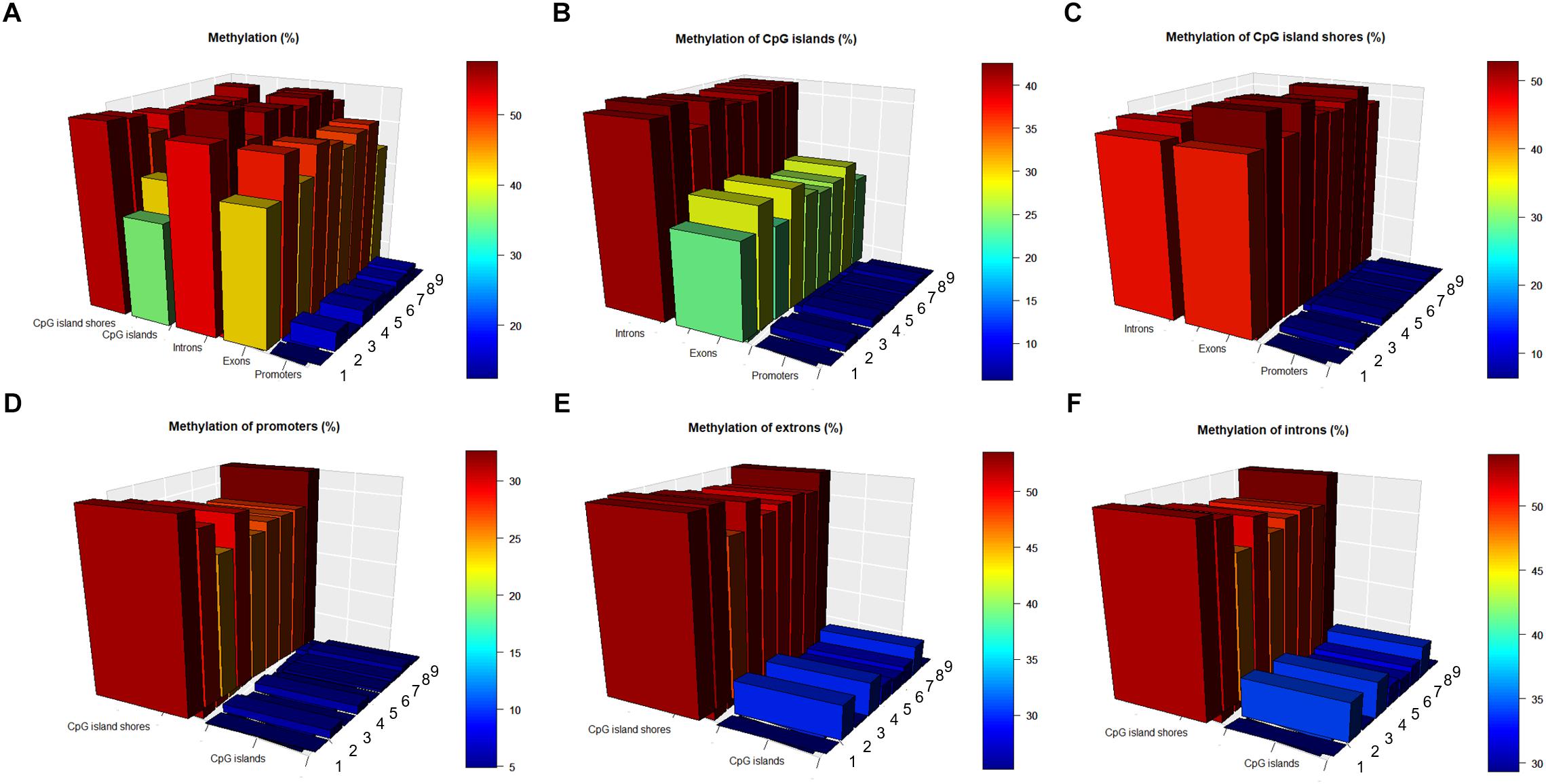
Figure 3. Methylation patterns in different genic features and CpG islands regions. (A) Methylation levels (in %) at different genic features, CpG islands and CpG island shores. (B) Methylation levels (in %) of CpG islands at different genic features. (C) Methylation levels (in %) of CpG island shores at different genic features. (D) Methylation levels (in %) of promoters in the CpG islands and CpG island shores. (E) Methylation levels (in %) of exons in the CpG islands and CpG island shores. (F) Methylation levels (in %) of introns in the CpG islands and CpG island shores.
Differentially Methylated Cytosines (DMC) and Annotations
A total of 1,244,043 CpG sites was covered in nine samples, and the number of identified DMCs was 12,738 with the level of Q < 0.01. Details of 12,738 DMCs with chromosomes, positions, P-values, Q-values, associated genes, genetic features and methylation levels are listed in Supplementary File S1. Percentages of 1,244,043 CpG sites annotated within promoter, exon, intron and intergenic regions were distributed as 5.33, 1.23, 3.80, and 89.64%, respectively. Additionally, the distribution of 1,244,043 CpG sites annotation within CpG islands, CpG island shores and other regions was 57.41, 14.71, and 27.88%, respectively. However, the distributions were 0.33, 1.71, 5.95, and 92.01% within promoter, exon, intron, and intergenic regions, respectively, when only considering the 12,738 DMCs. The distributions of DMCs annotated within CpG islands, CpG island shores and other regions were 36.86, 21.65, and 41.49%, respectively (Figure 4). The percentages of DMCs associated with CpG islands located in gene promoter, exon, intron, and intergenic regions were 69.05, 53.67, 32.32, and 36.72%, respectively. They were all higher than the DMCs associated with CpG island shores with the values of 19.05, 13.76, 24.01, and 21.66% in promoter, exon, intron, and intergenic regions, respectively (Table 2).
Among 19 (n = 18 + 1) Sus scrofa chromosomes (SSC), DMCs occupied SSC12 (12.1%) mostly, and nearly no DMCs occupied SSC X and SSC Y with the percentages of 0.4 and 0.1%, respectively (Figure 5A). DMCs were located mostly in the shorter genes and to lesser extent in the longer genes. Similarly, most of DMCs were located in CpG islands with a short length from 200 to 1000 bp (Figure 5B). Methylation levels of DMCs in different genic features were different, with the lowest values of CpG islands in the promoter regions. Student’s t-tests showed that methylation levels of DMCs in promoter, exon and intron regions were significantly different between CpG islands and CpG island shores (P < 0.05), while those of intergenic regions were extremely significant (P < 0.001) (Figure 5C). The averaged methylation levels on different chromosomes and different individuals were similar, with values close to 50% (Figure 5D).
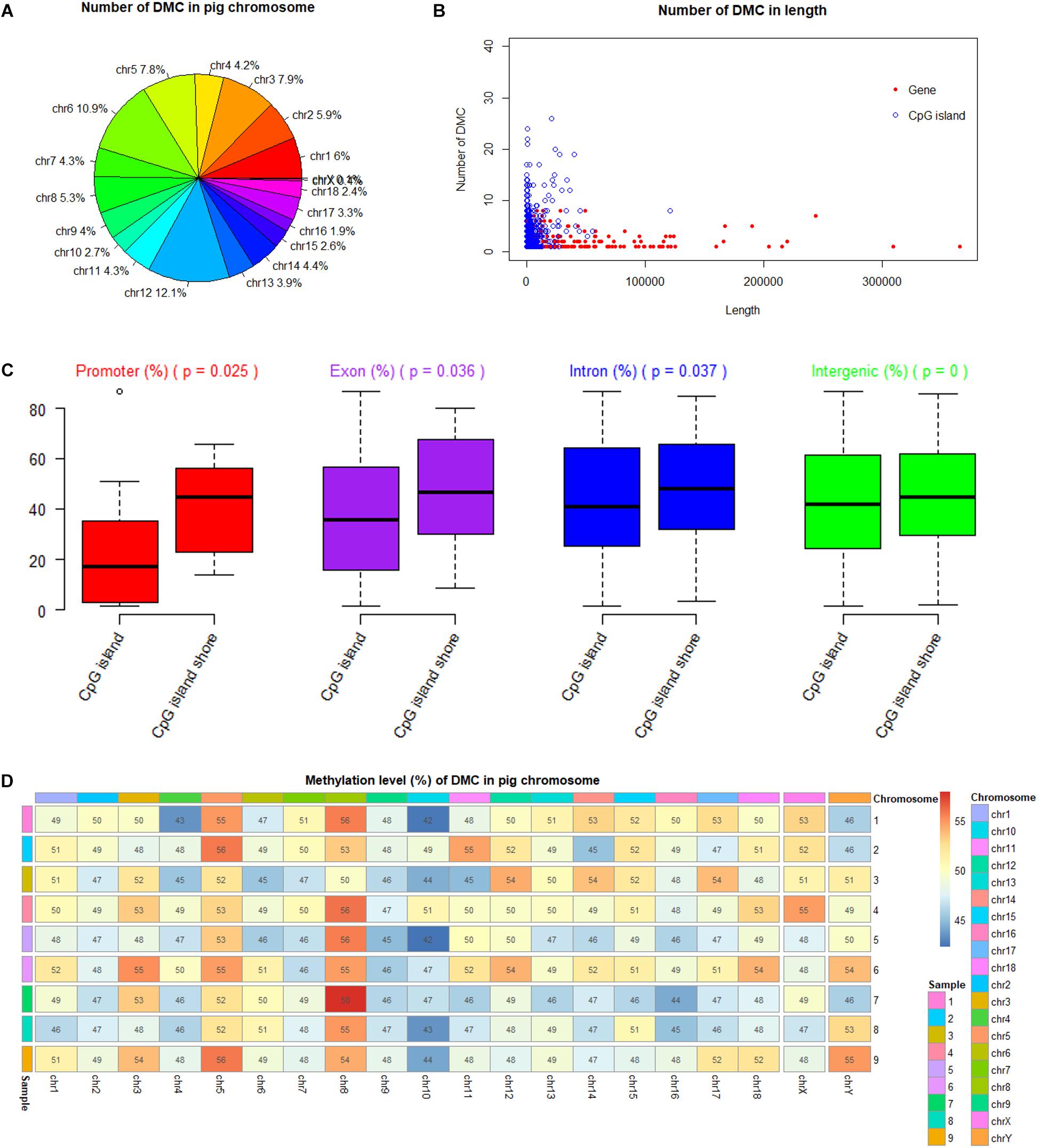
Figure 5. Methylation status of DMC in porcine chromosomes, genic features and CpG islands. (A) Number of DMC in different pig chromosome. (B) Number of DMC in the different lengths of genes and CpG islands. (C) Comparison of methylation levels between CpG islands and CpG island shores at different genic feature with Student’s t-tests. (D) Methylation levels of DMC in different pig chromosome.
Genes Associated With DMCs and Their Gene Ontology (GO) Enrichment and Pathway Analyses
We found that 976 DMCs were annotated within gene components of 415 genes after matching 12,738 DMCs to the porcine RefSeq database (Sscrofa11.1/susScr11) (Supplementary File S1). Fifteen genes associated with DMCs found to be related to fertility or boar taint traits were also reported by other studies (Table 3). Genes ACACA, CYP21A2, CYP27A1, HSD17B2, LHB, PARVG, and SERPINC1 were associated with boar taint, while genes DICER1, PCK1, SS18, and TGFB3 were associated with pig reproduction traits. In addition, the other five genes (CAPN10, FTO, HSD17B2, IGF2, and SALL4) were found to be associated with fertility traits in human, in which HSD17B2 also played a role in boar taint (Table 3).
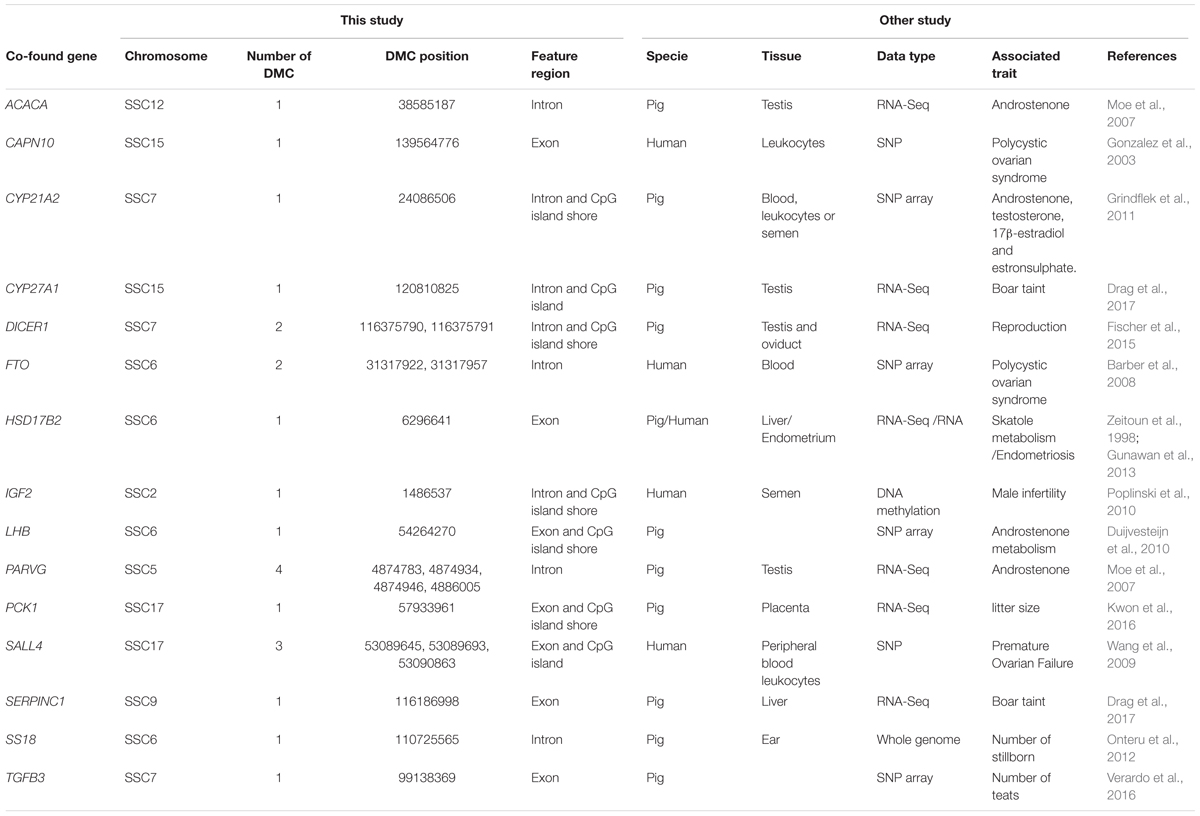
Table 3. Comparisons between DMC related genes of this study and identified genes for fertility or boat taint traits of other studies.
Hereafter, 898 genes (296 unique genes) associated with 2089 DMCs (704 unique DMCs) were enriched in 112 GO terms (Supplementary File S2). The significant GO terms (P < 0.01) are shown with the texts including 7 GO terms of biological process, 5 GO terms of cellular component and 7 GO terms of molecular function (Figure 6). Generally, as more genes were enriched in the GO terms, the number of included DMCs increased (Figure 6). Two GO terms (GO: 0005737 and GO: 0005634) in the cellular component contained the genes and DMCs mostly, that were 80 and 78 enriched genes associated with 185 and 182 DMCs, respectively (Supplementary File S2). The 23 significant pathways (P < 0.01) are listed in Supplementary Table S4. The most significant pathway was insulin signaling pathway (P = 9.89 × 10-7) containing 16 genes namely PHKG2, FASN, PHKG1, ACACA, IKBKB, FBP1, GYS1, PRKCZ, PRKAA2, PRKAG1, PCK1, ACACB, PIK3R5, SREBF1, AKT2, and MAP2K1 (Supplementary Table S4).
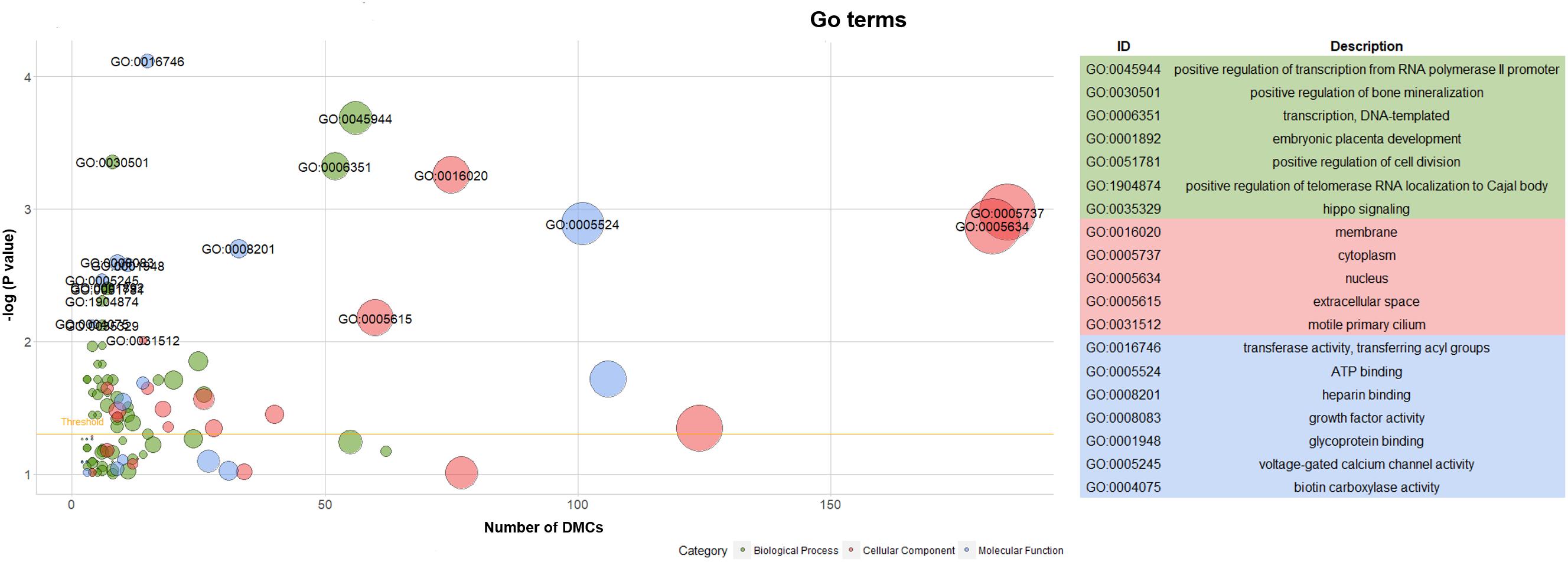
Figure 6. Go term analysis of genes associated with DMCs. Note: Yellow line in the left panel meant the threshold of significant GO terms (P < 0.05).
Discussion
Generally, the bisulfite conversion rates ranged from 90 to 100%, but some conversion rates varied between 99 and 100% depending on the commercial methods (Worm Ørntoft et al., 2017). This study showed higher bisulfite conversion efficiencies between 98 and 99%. A mapping efficiency of 38.3% was previously reported in RRBS sequencing of lamb muscle with fragment sizes of 50–150 bp, which increased to 61.4% with fragment sizes of 150–250 bp (Doherty and Couldrey, 2014). Similarly, our study revealed efficiency of 49% using 40–220 bp sizes that were uniquely mapped to the porcine reference genome (Table 1). It is consistent with 60% mapping rates using 110–220 bp sizes in RRBS sequencing for porcine ovaries (Yuan et al., 2016). We found that global CpG methylation levels ranged from 45 to 53% (50% on average), which is similar with other studies on pig methylation research using RRBS method (Gao et al., 2014; Choi et al., 2015; Schachtschneider et al., 2015), whereas non-CpG methylation levels (CHG and CHH sites) were less than 1% (Table 1). This is reasonable because CpGs within poor-CpG regions are scarcely covered based on restriction enzyme digestion by the RRBS method (Meissner et al., 2005). Our results also showed 72% of CpG methylations were mapped to CpG islands (57.41%) and to CpG island shores (14.71%), that were higher than those of Choi’s study (Choi et al., 2015). Whole genome bisulfite sequencing (WGBS) technology can produce many reads in poorly assembled non-coding DNA regions, resulting in lower mapping efficiency than RRBS method (Doherty and Couldrey, 2014). However, RRBS data sets have a somewhat lower average methylation level than WGBS data sets, because large stretches of repeat regions in non-coding DNA regions are generally highly methylated (Bird, 2002). Practically, some CpG sites had low coverage (1∼ 10) or are not even sequenced by the WGBS method, although all sites should be theoretically covered (Sun et al., 2015). Thus, average read depths of RRBS sequencing were higher than 10 in this study (Table 1 and Supplementary Table S1) and in other studies (Zhao et al., 2016; Carmona et al., 2017). Overall, RRBS method remained a better choice when considering sequencing cost, read coverage and sufficient methylation information (Choi et al., 2015).
In many cell types of different species, percentages of methylations would have a bimodal distribution, which denoted that the majority of bases has either high or low methylation to indicate a site specificity (Ehrlich et al., 1982). This bimodal pattern was a possible function to keep the factor-mediated basal transcription profile of the preimplantation embryo (Cedar and Bergman, 2012). The CpG methylation percentage distribution would be measured with two peaks at 0 and 100%, when a large number of the CpG sites were sequenced in either unmethylated or fully methylated status (Falckenhayn et al., 2013; Zhang et al., 2017). Bimodal distribution is also an important metric to help reveal whether the experiments suffer from PCR duplication bias. If there is a high degree of clonal reads from PCR, some reads will be asymmetrically amplified and read coverage distribution will have a secondary peak correspondingly on the right side. This situation will impair accurate determination of percent methylation scores for those regions. Hence, this study discarded cytosines with a percentile of read coverage higher than 99.9th, and then showed the reasonably bimodal distribution (Supplementary Figure 2) in consistency with other results using different tissues in pigs (Choi et al., 2015).
Not only did DNA methylation have a correlation with gene transcription, but also the presence of methyl moieties inhibited gene expression in vivo (Razin and Cedar, 1991). It was suggested by our study that the regression coefficients and correlation coefficients of genes and methylation levels were both negative, ranging from -1.97 to -2.46 and from -0.10 to -0.14, respectively (Supplementary Table S3). In practice, the correlation coefficient between gene expression and methylation level was approximately 0.3, negative (Bock, 2012). Methylated genes might be associated with genomic region-specific DNA methylation patterns (Raza et al., 2017), and therefore, this study investigated promoter, exon and intron regions along the porcine genome and localized CpG islands to these genic features. The interactions of methylations between three genic features and CpG islands suggested that methylation levels of promoter regions were lowest in both CpG islands and CpG island shores (Figure 3A). It was well known that DNA methylation in a promoter was correlated with the transcription of a target gene (Niesen et al., 2005). Methylation levels of CpG islands were lower than CpG island shores in the promoter, exon and intron regions in this study (Figures 3D–F). These results demonstrated that CpG islands located in different genic features displayed effects on the methylation patterns of the associated genes. Irizarry et al. (Irizarry et al., 2009b) revealed a strong relation between methylations in CpG island shores located within 2 kb of an annotated transcription start site (TSS) and expression of associated genes. Meanwhile, CpG islands located in exon regions showed different methylation level with those located in intron regions (Figures 3B,C), which suggested that exons had an effect on the methylation patterns of CpG islands. Chen et al. (2018) has profiled methylation patterns for porcine testis at three prepubertal age points (i.e., 1, 2, and 3 months). They found that the methylation levels of promoters and CpG islands decreased as the pig gradually matured, while methylation levels of gene body kept stable (Chen et al., 2018). It was suggested that lower methylations in promoters could be a specific pattern for testis tissue in adult pig, because spermatogenic cells tended to be activated for the increasing gene expression requirement at this stage. Additionally, Yuan et al. (2017) revealed that CpG islands show lower methylation levels compared to their CpG island shore regions in porcine hypothalamus-pituitary-ovary axis. Methylation levels in introns, exons, and promoters gradually decreased both in CpG islands and CpG island shores (Yuan et al., 2017). The methylation patterns of hypothalamus-pituitary-ovary axis were similar to our results except that exons located in CpG island shores of this study showed slightly higher methylations than those located in CpG islands (Figures 3B,C).
The percentages of DMCs annotation within exon, intron and intergenic regions increased, whereas DMCs annotation within promoter region decreased dramatically, when comparing DMCs with CpGs annotation within genic features. Similarly, the percentage of DMCs annotation within CpG island shores increased, while DMCs annotation within CpG islands decreased (Figure 4). As Maunakea et al. (2010) found that the methylated CpG islands in 5′ promoter regions were less than 3%, DMCs found in promoter regions were also less than 1% in this study (Figure 4). The most common promoter type in the vertebrate genome was annotated gene promoters with the CpG islands and they occupied at above 70% (Saxonov et al., 2006). We found that approximately 69% of DMCs associated with CpG islands were located in promoter regions (Table 2). Liu et al. (Liu et al., 2017) reported that the proportions of hypermethylated CpG sites located in CpG islands, CpG shores and other locations were 25.49∼34.23%, 21.57∼40.75%, and 25.02∼52.94%, respectively, during different stages of human embryonic stem cells. Genes that contained differentially methylated regions (DMRs) in their first intron were more than the genes that contained DMRs in their promoter and their first exon (Anastasiadi et al., 2018), which are the same trend as this study (Supplementary File S1).
In humans, more than 80% of sperm cells were mainly composed in the testis (Bellve et al., 1977). The epigenetic modifications of germ cells occurring in the meiotic and post-meiotic phases of spermatogenesis are crucial for embryonic development after fertilization (Marques et al., 2010). Due to the failure of re-methylation in spermatogonia or alterations to methylation maintenance in spermatocytes, sperm cells or the mature sperm cells, the abnormal DNA methylation patterns were observed in the infertile men (Cui et al., 2016). Therefore, the methylation patterns in genic features and CpG islands of pig testis were investigated to reveal significant cytosines and associated genes for epigenetic molecular mechanisms related to male fertility. Langenstroth-Röwer et al. (2017) used the marmoset monkey as the human model for testicular methylation study. They found that cytosines were predominantly unmethylated at regulatory regions of H19, LIT1, SNRPN, MEST, and OCT4 in the germ cells. Meanwhile, DNA methylation pattern of H19, MEST, DDX-4, and MAGE-A4 did not change in germ cell fractions (Langenstroth-Röwer et al., 2017). The genome-wide promoter methylation profiles identified 367 testis and epididymis-specific hypomethylated genes and 134 hypermethylated genes, many of them were involved in the GO terms of male reproduction (Wu et al., 2013). Compared with the fertile males, it was reported that a low methylation or unmethylation pattern at the H19 was associated with hypermethylation at the MEST and a reduced sperm quality in the oligospermic patients (Niemitz and Feinberg, 2004). DMRs located in the upstream of TSS of the H19 harbored several CCCTC-binding factor (CTCF) binding sites (Takai, 2001). However, CTCF binding to the maternal unmethylated DMR could prevent IGF2 from accessing the common enhancers, and thus silencing its expression (Marques et al., 2010). Rajender et al. (2011) summarized that genes MTHFR, PAX8, NTF3, SFN, HRAS, JHM2DA, IGF2, H19, RASGRF1, GTL2, PLAG1, D1RAS3, MEST, KCNQ1, LIT1, and SNRPN were associated with male infertility. Our study also identified the DMCs located in the intron regions of IGF2 (Table 3), which was involved in GO terms of positive regulation of cell division (GO: 0051781), extracellular space (GO: 0005615), and growth factor activity (GO: 0008083) (Supplementary File S2).
Our study revealed the methylation patterns in different genic features such as promotor, exon, intron and intergenic regions, as well as CpG islands, CpG island shores regions. Furthermore, our study reported many candidate genes harboring DMCs and the involved GO terms of testis in pig. Until now, several studies have concluded the important genes associated with male fertilities using SNP array, RNA-Seq datasets for humans (Table 3), however, epigenetic studies in pigs relating to male fertility are rare. This study has reported for the first time, DNA methylome (epigenomic) architecture in adult pig testis for study of male fertility in pigs. These results will also be useful for the study of boar taint in pigs associated with sensory meat quality, as boar taint is inherited and shows complex gene regulation patterns (Strathe et al., 2013; Drag et al., 2018). Since this study is based on sequence-level resolution of transmittable epigenetic changes, we believe it may also contribute to understanding and capturing part of the genetic variation that are not captured by SNP arrays (considered missing or “missing heritability”) in genome-wide genomic prediction studies. As pig is a valuable biomedical model of human, the findings of this study are also very helpful to understand the relationship between DNA methylation and genic CpG islands, and provide candidate epigenetic biomarkers for the translational studies in human research.
Conclusion
This is the first study to report catalog of adult pig testis epigenome by developing a genome-wide DNA methylation map with the use of RRBS technology. We found that the methylation rates were lowest in promoters (0.15) and highest in introns (0.55). Cytosines binding to CpG islands showed different methylation patterns between intron and exon regions. Methylation levels of CpG islands were lower than CpG island shores in different genic features. We detected 12,738 DMCs in total. They distributions of DMCs within CpG islands, CpG island shores and other regions were 36.86, 21.65, and 41.49%, respectively. The distributions of DMCs were 0.33, 1.71, 5.95, and 92.01% in promoter, exon, intron and intergenic regions, respectively. Fifteen genes with DMCs were associated with human fertility (ACACA, CYP21A2, CYP27A1, HSD17B2, LHB, PARVG, and SERPINC1), pig reproduction (DICER1, PCK1, SS18, and TGFB3) and boar taint traits (CAPN10, FTO, HSD17B2, IGF2, and SALL4). These findings on genome-wide epigenetic signatures will be useful to understand testis-related trait inheritance in pigs (e.g., male fertility, semen quality, boar taint) for pig production and welfare. This study, based on sequence-level resolution of epigenetic changes, also contributes to understanding and capturing part of the genetic variation that are considered missing (“missing heritability”) in genome-wide genomic prediction studies. Since pigs are useful as an animal model for human research, epigenetic architecture of pigs would help in translational research.
Ethics Statement
Animal Care and Use Committee approval was not obtained for this study, because tissue samples were obtained from a commercial slaughter facility.
Author Contributions
HK conceived, designed and implemented the epigenomic experiments including collection of tissue samples and processing of samples for methylome sequencing by RRBS, and improved the manuscript. XW analyzed the data. XW and HK interpreted the results and wrote the manuscript. Both authors read and approved the final manuscript.
Funding
Ph.D. Project funded by the Department of Applied Mathematics and Computer Science, Technical University of Denmark, Denmark.
Conflict of Interest Statement
The authors declare that the research was conducted in the absence of any commercial or financial relationships that could be construed as a potential conflict of interest.
Acknowledgments
We thank staff at the Slaughter house – Danish Crown in Herning, Denmark and Dr. Ruta Skinkyté-Juskiené for assistance in tissue collection and processing of samples. We thank Dr. Markus Drag for organizing shipments of samples for sequencing. XW received Ph.D. stipends from the Technical University of Denmark, DTU Bioinformatics and DTU Compute, Denmark, and the China Scholarship Council, China.
Supplementary Material
The Supplementary Material for this article can be found online at: https://www.frontiersin.org/articles/10.3389/fgene.2019.00405/full#supplementary-material
FIGURE S1 | Histograms of log10 of read coverage per CpG site.
FIGURE S2 | Correlation analysis of the global CpG methylation patterns among nine samples. Note: Colors in the scatter plot indicate the number of CpG sites with identical methylation pattern (methylated or non-methylated): yellow denotes many correlations, blue denotes lack of correlation and green denotes different methylation patterns. Numbers in the upper right side represent the pairwise Pearson’s correlation scores. Histograms on the diagonal are methylation distribution per CpG site for each sample.
FIGURE S3 | Regression of densities of genes, CpG islands and CpG island shores on methylation levels from one sample, all counted by 1 Mb windows.
TABLE S1 | Total number of aligned cytosine methylation in different contexts.
TABLE S2 | Statistics of coverage and methylation rates in CpG context.
TABLE S3 | Regression and correlation analysis of densities of genes, CpG islands and CpG island shores on methylation levels, all counted by 1 Mb windows.
TABLE S4 | Significant pathways (P < 0.01).
FILE S1 | Details of 12,738 DMCs with chromosomes, positions, P-values, Q-values, associated genes, genetic features and methylation levels.
FILE S2 | GO terms and enriched genes associated with DMCs.
Abbreviations
BGI, Beijing Genomics Institute; bp, base pair; cm, centimetre; CO2, Carbon dioxide; CpG, Cytosine and guanine dinucleotide; CTCF, CCCTC-binding factor; DMC, Differentially methylated cytosine; DMR, Differentially methylated region; FDR, False discovery rate; GO, Gene ontology; kb, kilo base pairs; kg, kilogram; Mb, mega base pair; mg, milligram; ml, millilitre; NGS, Next generation sequencing; PCR, Polymerase chain reaction; RNA-Seq, RNA sequencing; RRBS, Reduced representation bisulfite sequencing; SNP, Single nucleotide polymorphism; SSC, Sus scrofa chromosomes; TSS, Transcription start site; WGBS, Whole genome bisulfite sequencing.
Footnotes
- ^ http://hgdownload.cse.ucsc.edu/goldenPath/susScr11/bigZips/susScr11.fa.gz
- ^ http://genome.ucsc.edu/cgi-bin/hgTables
- ^ https://david.ncifcrf.gov/
References
Akalin, A., Franke, V., Vlahoviček, K., Mason, C. E., and Schübeler, D. (2015). Genomation: a toolkit to summarize, annotate and visualize genomic intervals. Bioinformatics 31, 1127–1129. doi: 10.1093/bioinformatics/btu775
Akalin, A., Kormaksson, M., Li, S., Garrett-Bakelman, F. E., Figueroa, M. E., Melnick, A., et al. (2012). MethylKit: a comprehensive R package for the analysis of genome-wide DNA methylation profiles. Genome Biol. 13:R87. doi: 10.1186/gb-2012-13-10-R87
Anastasiadi, D., Esteve-Codina, A., and Piferrer, F. (2018). Consistent inverse correlation between DNA methylation of the first intron and gene expression across tissues and species. Epigenetics Chromatin 11:37. doi: 10.1186/s13072-018-0205-1
Barber, T. M., Bennett, A. J., Groves, C. J., Sovio, U., Ruokonen, A., Martikainen, H., et al. (2008). Association of variants in the fat mass and obesity associated (FTO) gene with polycystic ovary syndrome. Diabetologia 51, 1153–1158. doi: 10.1007/s00125-008-1028-6
Bell, J. T., Pai, A. A., Pickrell, J. K., Gaffney, D. J., Pique-Regi, R., Degner, J. F., et al. (2011). DNA methylation patterns associate with genetic and gene expression variation in HapMap cell lines. Genome Biol. 12:R10. doi: 10.1186/gb-2011-12-1-r10
Bellve, A. R., Cavicchia, J. C., Millette, C. F., O’Brien, D. A., Bhatnagar, Y. M., and Dym, M. (1977). Spermatogenic cells of the prepuberal mouse. Isolation and morphological characterization. J. Cell Biol. 74, 68–85. doi: 10.1083/jcb.74.1.68
Bird, A. (2002). DNA methylation patterns and epigenetic memory. Genes Dev. 16, 6–21. doi: 10.1101/gad.947102
Bock, C. (2012). Analysing and interpreting DNA methylation data. Nat. Rev. Genet. 13, 705–719. doi: 10.1038/nrg3273
Bolger, A. M., Lohse, M., and Usadel, B. (2014). Trimmomatic: A flexible trimmer for Illumina sequence data. Bioinformatics 30, 2114–2120. doi: 10.1093/bioinformatics/btu170
Carmona, J. J., Accomando, W. P., Binder, A. M., Hutchinson, J. N., Pantano, L., Izzi, B., et al. (2017). Empirical comparison of reduced representation bisulfite sequencing and Infinium BeadChip reproducibility and coverage of DNA methylation in humans. npj Genomic Med. 2:13. doi: 10.1038/s41525-017-0012-9
Cedar, H., and Bergman, Y. (2012). Programming of DNA methylation patterns. Annu. Rev. Biochem. 81, 97–117. doi: 10.1146/annurev-biochem-052610-091920
Chen, X., Shen, L. H., Gui, L. X., Yang, F., Li, J., Cao, S. Z., et al. (2018). Genome-wide DNA methylation profile of prepubertal porcine testis. Reprod. Fertil. Dev. 30, 349–358. doi: 10.1071/RD17067
Choi, M., Lee, J., Le, M. T., Nguyen, D. T., Park, S., Soundrarajan, N., et al. (2015). Genome-wide analysis of DNA methylation in pigs using reduced representation bisulfite sequencing. DNA Res. 22, 343–355. doi: 10.1093/dnares/dsv017
Cui, X., Jing, X., Wu, X., Yan, M., Li, Q., Shen, Y., et al. (2016). DNA methylation in spermatogenesis and male infertility. Exp. Ther. Med. 12, 1973–1979. doi: 10.3892/etm.2016.3569
Doherty, R., and Couldrey, C. (2014). Exploring genosme wide bisulfite sequencing for DNA methylation analysis in livestock: a technical assessment. Front. Genet. 5:126. doi: 10.3389/fgene.2014.00126
Doi, A., Park, I.-H., Wen, B., Murakami, P., Aryee, M. J., Irizarry, R., et al. (2009). Differential methylation of tissue- and cancer-specific CpG island shores distinguishes human induced pluripotent stem cells, embryonic stem cells and fibroblasts. Nat. Genet. 41, 1350–1353. doi: 10.1038/ng.471
Drag, M., Hansen, M. B., and Kadarmideen, H. N. (2018). Systems genomics study reveals expression quantitative trait loci, regulator genes and pathways associated with boar taint in pigs. PLoS One 13:e0192673. doi: 10.1371/journal.pone.0192673
Drag, M., Skinkyté-Juskiené, R., Do, D. N., Kogelman, L. J. A., and Kadarmideen, H. N. (2017). Differential expression and co-expression gene networks reveal candidate biomarkers of boar taint in non-castrated pigs. Sci. Rep. 7:12205. doi: 10.1038/s41598-017-11928-0
Duijvesteijn, N., Knol, E. F., Merks, J. W. M., Crooijmans, R. P. M. A., Groenen, M. A. M., Bovenhuis, H., et al. (2010). A genome-wide association study on androstenone levels in pigs reveals a cluster of candidate genes on chromosome 6. BMC Genet. 11:42. doi: 10.1186/1471-2156-11-42
Ehrlich, M., Gama-Sosa, M. A., Huang, L. H., Midgett, R. M., Kuo, K. C., Mccune, R. A., et al. (1982). Amount and distribution of 5-methylcytosine in human DNA from different types of tissues or cells. Nucleic Acids Res. 10, 2709–2721. doi: 10.1093/nar/10.8.2709
Falckenhayn, C., Boerjan, B., Raddatz, G., Frohme, M., Schoofs, L., and Lyko, F. (2013). Characterization of genome methylation patterns in the desert locust Schistocerca gregaria. J. Exp. Biol. 216(Pt 8), 1423–1429. doi: 10.1242/jeb.080754
Fischer, D., Laiho, A., Gyenesei, A., and Sironen, A. (2015). Identification of reproduction-related gene polymorphisms using whole transcriptome sequencing in the large white pig population. G3 5, 1351–1360. doi: 10.1534/g3.115.018382
Gao, F., Zhang, J., Jiang, P., Gong, D., Wang, J. W., Xia, Y., et al. (2014). Marked methylation changes in intestinal genes during the perinatal period of preterm neonates. BMC Genomics 15:716. doi: 10.1186/1471-2164-15-716
Gardiner-Garden, M., and Frommer, M. (1987). CpG Islands in vertebrate genomes. J. Mol. Biol. 196, 261–282. doi: 10.1016/0022-2836(87)90689-9
Goldberg, A. D., Allis, C. D., and Bernstein, E. (2007). Epigenetics: a landscape takes shape. Cell 128, 635–638. doi: 10.1016/j.cell.2007.02.006
Gonzalez, A., Abril, E., Roca, A., Aragón, M. J., Figueroa, M. J., Velarde, P., et al. (2003). Specific CAPN10 gene haplotypes influence the clinical profile of polycystic ovary patients. J. Clin. Endocrinol. Metab. 88, 5529–5536. doi: 10.1210/jc.2003-030322
Grindflek, E., Lien, S., Hamland, H., Hansen, M. H. S., Kent, M., van Son, M., et al. (2011). Large scale genome-wide association and LDLA mapping study identifies QTLs for boar taint and related sex steroids. BMC Genomics 12:362. doi: 10.1186/1471-2164-12-362
Gunawan, A., Sahadevan, S., Cinar, M. U., Neuhoff, C., Große-Brinkhaus, C., Frieden, L., et al. (2013). Identification of the novel candidate genes and variants in boar liver tissues with divergent skatole levels using RNA deep sequencing. PLoS One 8:e72298. doi: 10.1371/journal.pone.0072298
Hansen, K. D., Timp, W., Bravo, H. C., Sabunciyan, S., Langmead, B., McDonald, O. G., et al. (2011). Increased methylation variation in epigenetic domains across cancer types. Nat. Genet. 43, 768–775. doi: 10.1038/ng.865
Irizarry, R. A., Ladd-Acosta, C., Wen, B., Wu, Z., Montano, C., Onyango, P., et al. (2009a). Genome-wide methylation analysis of human colon cancer reveals similar hypo-and hypermethylation at conserved tissue-specific CpG island shores. Nat. Genet. 41:178. doi: 10.1038/ng.298.Genome-wide
Irizarry, R. A., Ladd-Acosta, C., Wen, B., Wu, Z., Montano, C., Onyango, P., et al. (2009b). The human colon cancer methylome shows similar hypo- and hypermethylation at conserved tissue-specific CpG island shores. Nat. Genet. 41, 178–186. doi: 10.1038/ng.298
Jin, L., Jiang, Z., Xia, Y., Lou, P., Chen, L., Wang, H., et al. (2014). Genome-wide DNA methylation changes in skeletal muscle between young and middle-aged pigs. BMC Genomics 15:653. doi: 10.1186/1471-2164-15-653
Jones, P. A. (2012). Functions of DNA methylation: Islands, start sites, gene bodies and beyond. Nat. Rev. Genet. 13, 484–492. doi: 10.1038/nrg3230
Kogelman, L. J. A., and Kadarmideen, H. N. (2016). Applications of systems genetics and biology for obesity using pig models. Syst. Biol. Anim. Prod. Heal. 1, 25–42. doi: 10.1007/978-3-319-43335-6_2
Kogelman, L. J. A., Kadarmideen, H. N., Mark, T., Karlskov-Mortensen, P., Bruun, C. S., Cirera, S., et al. (2013). An F2 pig resource population as a model for genetic studies of obesity and obesity-related diseases in humans: design and genetic parameters. Front. Genet. 4:29. doi: 10.3389/fgene.2013.00029
Krueger, F., and Andrews, S. R. (2011). Bismark: a flexible aligner and methylation caller for bisulfite-seq applications. Bioinformatics 27, 1571–1572. doi: 10.1093/bioinformatics/btr167
Kwon, S. G., Hwang, J. H., Park, D. H., Kim, T. W., Kang, D. G., Kang, K. H., et al. (2016). Identification of differentially expressed genes associated with litter size in Berkshire pig placenta. PLoS One 11:e0153311. doi: 10.1371/journal.pone.0153311
Langenstroth-Röwer, D., Gromoll, J., Wistuba, J., Tröndle, I., Laurentino, S., Schlatt, S., et al. (2017). De novo methylation in male germ cells of the common marmoset monkey occurs during postnatal development and is maintained in vitro. Epigenetics 12, 527–539. doi: 10.1080/15592294.2016.1248007
Langmead, B., and Salzberg, S. L. (2012). Fast gapped-read alignment with Bowtie 2. Nat. Methods 9, 357–359. doi: 10.1038/nmeth.1923
Law, J. A., and Jacobsen, S. E. (2010). Establishing, maintaining and modifying DNA methylation patterns in plants and animals. Nat. Rev. Genet. 11, 204–220. doi: 10.1038/nrg2719
Liu, Z., Feng, Q., Sun, P., Lu, Y., Yang, M., Zhang, X., et al. (2017). Genome-wide DNA methylation drives human embryonic stem cell erythropoiesis by remodeling gene expression dynamics. Epigenomics 9, 1543–1558. doi: 10.2217/epi-2017-0039
Long, M. D., Smiraglia, D. J., and Campbell, M. J. (2017). The genomic impact of DNA CpG methylation on gene expression; relationships in prostate cancer. Biomolecules 7:E15. doi: 10.3390/biom7010015
Marques, C. J., Francisco, T., Sousa, S., Carvalho, F., Barros, A., and Sousa, M. (2010). Methylation defects of imprinted genes in human testicular spermatozoa. Fertil. Steril. 94, 585–594. doi: 10.1016/j.fertnstert.2009.02.051
Maunakea, A. K., Nagarajan, R. P., Bilenky, M., Ballinger, T. J., Dsouza, C., Fouse, S. D., et al. (2010). Conserved role of intragenic DNA methylation in regulating alternative promoters. Nature 466, 253–257. doi: 10.1038/nature09165
Meissner, A., Gnirke, A., Bell, G. W., Ramsahoye, B., Lander, E. S., and Jaenisch, R. (2005). Reduced representation bisulfite sequencing for comparative high-resolution DNA methylation analysis. Nucleic Acids Res. 33, 5868–5877. doi: 10.1093/nar/gki901
Moe, M., Meuwissen, T., Lien, S., Bendixen, C., Wang, X., Conley, L. C., et al. (2007). Gene expression profiles in testis of pigs with extreme high and low levels of androstenone. BMC Genomics 8:405. doi: 10.1186/1471-2164-8-405
Niemitz, E. L., and Feinberg, A. P. (2004). Epigenetics and assisted reproductive technology: a call for investigation. Am. J. Hum. Genet. 74, 599–609. doi: 10.1086/382897
Niesen, M. I., Osborne, A. R., Yang, H., Rastogi, S., Chellappan, S., Cheng, J. Q., et al. (2005). Activation of a methylated promoter mediated by a sequence-specific DNA-binding protein, RFX. J. Biol. Chem. 280, 38914–38922. doi: 10.1074/jbc.M504633200
Onteru, S. K., Fan, B., Du, Z. Q., Garrick, D. J., Stalder, K. J., and Rothschild, M. F. (2012). A whole-genome association study for pig reproductive traits. Anim. Genet. 43, 18–26. doi: 10.1111/j.1365-2052.2011.02213.x
Poplinski, A., Tüttelmann, F., Kanber, D., Horsthemke, B., and Gromoll, J. (2010). Idiopathic male infertility is strongly associated with aberrant methylation of MEST and IGF2/H19 ICR1. Int. J. Androl. 33, 642–649. doi: 10.1111/j.1365-2605.2009.01000.x
Rajender, S., Avery, K., and Agarwal, A. (2011). Epigenetics, spermatogenesis and male infertility. Mutat. Res. Rev. Mutat. Res. 727, 62–71. doi: 10.1016/j.mrrev.2011.04.002
Raza, M. A., Yu, N., Wang, D., Cao, L., Gan, S., and Chen, L. (2017). Differential DNA methylation and gene expression in reciprocal hybrids between Solanum lycopersicum and S. pimpinellifolium. DNA Res. 24, 597–607. doi: 10.1093/dnares/dsx028
Razin, A., and Cedar, H. (1991). DNA methylation and gene expression. Microbiol. Rev. 55, 451–458. doi: 10.1002/wsbm.64
Saxonov, S., Berg, P., and Brutlag, D. L. (2006). A genome-wide analysis of CpG dinucleotides in the human genome distinguishes two distinct classes of promoters. Proc. Natl. Acad. Sci. U.S.A. 103, 1412–1417. doi: 10.1073/pnas.0510310103
Schachtschneider, K. M., Madsen, O., Park, C., Rund, L. A., Groenen, M. A. M., and Schook, L. B. (2015). Adult porcine genome-wide DNA methylation patterns support pigs as a biomedical model. BMC Genomics 16:743. doi: 10.1186/s12864-015-1938-x
Smith, Z. D., Gu, H., Bock, C., Gnirke, A., and Meissner, A. (2009). High-throughput bisulfite sequencing in mammalian genomes. Methods 48, 226–232. doi: 10.1016/j.ymeth.2009.05.003
Storey, J. D., and Tibshirani, R. (2003). Statistical significance for genomewide studies. Proc. Natl. Acad. Sci. U.S.A. 100, 9440–9445. doi: 10.1073/pnas.1530509100
Strathe, A. B., Velander, I. H., Mark, T., and Kadarmideen, H. N. (2013). Genetic parameters for androstenone and skatole as indicators of boar taint and their relationship to production and litter size traits in Danish Landrace. J. Anim. Sci. 91, 2587–2595. doi: 10.2527/jas.2012-6107
Stuppia, L., Franzago, M., Ballerini, P., Gatta, V., and Antonucci, I. (2015). Epigenetics and male reproduction: the consequences of paternal lifestyle on fertility, embryo development, and children lifetime health. Clin. Epigenet. 7:120. doi: 10.1186/s13148-015-0155-4
Sun, Z., Cunningham, J., Slager, S., and Kocher, J. P. (2015). Base resolution methylome profiling: considerations in platform selection, data preprocessing and analysis. Epigenomics 7, 813–828. doi: 10.2217/epi.15.21
Takai, D. (2001). Large scale mapping of methylcytosines in CTCF-binding sites in the human H19 promoter and aberrant hypomethylation in human bladder cancer. Hum. Mol. Genet. 10, 2619–2626. doi: 10.1093/hmg/10.23.2619
Verardo, L. L., Silva, F. F., Lopes, M. S., Madsen, O., Bastiaansen, J. W. M., Knol, E. F., et al. (2016). Revealing new candidate genes for reproductive traits in pigs: combining bayesian GWAS and functional pathways. Genet. Sel. Evol. 48:9. doi: 10.1186/s12711-016-0189-x
Walter, W., Sánchez-Cabo, F., and Ricote, M. (2015). GOplot: An R package for visually combining expression data with functional analysis. Bioinformatics 31, 2912–2914. doi: 10.1093/bioinformatics/btv300
Wang, B., Li, L., Ni, F., Song, J., Wang, J., Mu, Y., et al. (2009). Mutational analysis of SAL-Like 4 (SALL4) in Han Chinese women with premature ovarian failure. Mol. Hum. Reprod. 15, 557–562. doi: 10.1093/molehr/gap046
Wang, H., Wang, J., Ning, C., Zheng, X., Fu, J., Wang, A., et al. (2017). Genome-wide DNA methylation and transcriptome analyses reveal genes involved in immune responses of pig peripheral blood mononuclear cells to poly I:C. Sci. Rep. 7:9707. doi: 10.1038/s41598-017-10648-9
Worm Ørntoft, M. B., Jensen, S. Ø, Hansen, T. B., Bramsen, J. B., and Andersen, C. L. (2017). Comparative analysis of 12 different kits for bisulfite conversion of circulating cell-free DNA. Epigenetics 12, 626–636. doi: 10.1080/15592294.2017.1334024
Wu, C., Ding, X., Li, H., Zhu, C., and Xiong, C. (2013). Genome-wide promoter methylation profile of human testis and epididymis: Identified from cell-free seminal DNA. BMC Genomics 14:288. doi: 10.1186/1471-2164-14-288
Wu, C. T., and Morris, J. R. (2001). Genes, genetics, and epigenetics: a correspondence. Science 293, 1103–1105. doi: 10.1126/science.293.5532.1103
Yang, X., Han, H., DeCarvalho, D. D., Lay, F. D., Jones, P. A., and Liang, G. (2014). Gene body methylation can alter gene expression and is a therapeutic target in cancer. Cancer Cell 26, 577–590. doi: 10.1016/j.ccr.2014.07.028
Yuan, X. L., Gao, N., Xing, Y., Zhang, H. B., Zhang, A. L., Liu, J., et al. (2016). Profiling the genome-wide DNA methylation pattern of porcine ovaries using reduced representation bisulfite sequencing. Sci. Rep. 6:22138. doi: 10.1038/srep22138
Yuan, X. L., Zhang, Z., Li, B., Gao, N., Zhang, H., Sangild, P. T., et al. (2017). Genome-wide DNA methylation analysis of the porcine hypothalamus-pituitary-ovary axis. Sci. Rep. 7:4277. doi: 10.1038/s41598-017-04603-x
Zeitoun, K., Takayama, K., Sasano, H., Suzuki, T., Moghrabi, N., Andersson, S., et al. (1998). Deficient 17β-hydroxysteroid dehydrogenase type 2 expression in endometriosis: failure to metabolize 17β-estradiol. J. Clin. Endocrinol. Metab. 83, 4474–4480. doi: 10.1210/jc.83.12.4474
Zhang, H., Meltzer, P., and Davis, S. (2013). RCircos: an R package for Circos 2D track plots. BMC Bioinformatics 14:244. doi: 10.1186/1471-2105-14-244
Zhang, L., Gu, C., Yang, L., Tang, F., and Gao, Y. Q. (2017). The sequence preference of DNA methylation variation in mammalians. PLoS One 12:e0186559. doi: 10.1371/journal.pone.0186559
Keywords: pig, testis, epigenome, DNA methylation, RRBS, DMC
Citation: Wang X and Kadarmideen HN (2019) An Epigenome-Wide DNA Methylation Map of Testis in Pigs for Study of Complex Traits. Front. Genet. 10:405. doi: 10.3389/fgene.2019.00405
Received: 24 October 2018; Accepted: 12 April 2019;
Published: 30 April 2019.
Edited by:
David E. MacHugh, University College Dublin, IrelandReviewed by:
Zhe Zhang, South China Agricultural University, ChinaRicardo Zanella, The University of Passo Fundo, Brazil
Copyright © 2019 Wang and Kadarmideen. This is an open-access article distributed under the terms of the Creative Commons Attribution License (CC BY). The use, distribution or reproduction in other forums is permitted, provided the original author(s) and the copyright owner(s) are credited and that the original publication in this journal is cited, in accordance with accepted academic practice. No use, distribution or reproduction is permitted which does not comply with these terms.
*Correspondence: Haja N. Kadarmideen, aGFqYWtAZHR1LmRr