- 1Department of Health Statistics, School of Public Health, Shanxi Medical University, Taiyuan, China
- 2Department of Epidemiology and Biostatistics, Michigan State University, East Lansing, MI, United States
While substantial progress has been made in finding genetic variants associated with nicotine dependence (ND), a large proportion of the genetic variants remain undiscovered. The current research focuses have shifted toward uncovering rare variants, gene-gene/gene-environment interactions, and structural variations predisposing to ND, the impact of genetic heterogeneity in ND has been nevertheless paid less attention. The study of genetic heterogeneity in ND not only could enhance the power of detecting genetic variants with heterogeneous effects in the population but also improve our understanding of genetic etiology of ND. As an initial step to understand genetic heterogeneity in ND, we applied a newly developed heterogeneity weighted U (HWU) method to 26 ND-related genes, investigating heterogeneous effects of these 26 genes in ND. We found no strong evidence of genetic heterogeneity in genes such as CHRNA5. However, results from our analysis suggest heterogeneous effects of CHRNA6 and CHRNB3 on nicotine dependence in males and females. Following the gene-based analysis, we further conduct a joint association analysis of two gene clusters, CHRNA5-CHRNA3-CHRNB4 and CHRNB3-CHRNA6. While both CHRNA5-CHRNA3-CHRNB4 and CHRNB3-CHRNA6 clusters are significantly associated with ND, there is a much stronger association of CHRNB3-CHRNA6 with ND when considering heterogeneous effects in gender (p-value = 2.11E-07).
Introduction
Cigarette smoking is a leading cause of preventable disease, contributing to 5 million premature deaths worldwide each year. Nicotine dependence (ND, abbreviations are summarized in Supplementary Table S1) plays a critical role in smoking behavior since it is the dependence process that (a) drives up the count of occasions of tobacco use, (b) fosters escalation of dose, and (c) generates the multiple dose-years that account for the breadth of tobacco-attributable morbidity and mortality (e.g., cancers). Family, twin, and adoption studies have shown substantial genetic influence on ND (e.g., heritability of nicotine dependence is estimated from 50 to 60%). Substantial progress has also been made through linkage, candidate gene, and genome-wide association studies (GWAS) in identifying ND-associated genetic variants (Furberg et al., 2010; Sullivan et al., 2010; Wei et al., 2012).
Among all the findings, the neuronal nicotinic acetylcholine receptors (nAChRs) subunit genes have attracted special interest. nAChRs activates the release of dopamine, playing an important role in the dopaminergic reward system and the development of ND. nAChRs are pentameric complexes assembled from a family of subunits, encoded by 9 α and 3 β genes. Studies found strong associations of the CHRNA5-CHRNA3-CHRNB4 cluster and the CHRNB3-CHRNA6 cluster (Bierut et al., 2007; Saccone et al., 2007) with ND. Especially, a non-synonymous coding single nucleotide polymorphisms (SNP) in CHRNA5, rs16969968, has been identified and confirmed in several large-scale studies and meta-analysis (Liu et al., 2010; Hancock et al., 2015). Besides nAChRs subunit genes, cytochrome P450 (CYP) genes have also received increasing attention. CYP genes play a critical role in the synthesis and metabolism of various molecules and chemicals within cells. Studies have found the potential role of CYP2A6 variants (e.g., rs4105144) in nicotine metabolism (Thorgeirsson et al., 2010).
Despite these achievements, the currently confirmed genetic variants only account for a small proportion of ND variation and a large proportion of ND loci remain undetected. While current efforts have been focused on rare variants and gene-gene interactions contributing to ND, little attention has been paid on the heterogeneous genetic etiology of ND. Traditionally, genetic heterogeneity refers to allelic/locus heterogeneity, i.e., different alleles or different loci lead to the same or similar phenotypes (Thornton-Wells et al., 2004). In the paper, genetic heterogeneity of ND is defined as a ND-associated genetic variant having different effects on individuals or on subgroups of a population (e.g., different gender groups). Compelling evidence from previous studies suggest that genetic etiology of ND could vary in subgroups (e.g., in males and females) (Li et al., 2003). When genetic heterogeneity is present, the current analysis will likely yield attenuated estimates of genetic effects, leading to low power of the study. Despite the strong evidence of heterogeneity in ND, investigating genetic variants with heterogeneous effects in ND remains a great challenge, due to issues such as multiple testing and reduced sample size in each subgroup.
To study the genetic heterogeneity of ND, we started with a gene-based association analysis of 26 ND-related genes, considering their heterogeneous effects in males and females. Following this analysis, we further investigated 2 nAChRs gene clusters, evaluating their heterogeneous effects in males and females. An advanced non-parametric method (Wei et al., 2016) has been implemented in this study to address issues of multiple testing and reduced sample size of subgroups, which facilitate our investigation of the genetic heterogeneity of ND.
Materials and Methods
Data Resource
Study of Addiction: Genetics and Environment (SAGE) is one of the largest and most comprehensive population-based studies conducted to date, aimed at discovering genetic contributions to addiction. Samples from the SAGE were selected from 3 large, complementary datasets: the Collaborative Study on the Genetics of Alcoholism (COGA), the Family Study of Cocaine Dependence (FSCD), and the Collaborative Genetic Study of Nicotine Dependence (COGEND). Multiple phenotypes were measured in the SAGE studies, including several nicotine dependence measurements (e.g., DSM IV ND). For the focus of our study, we use the Fagerstrom Test for Nicotine Dependence (FTND) item 4, the number of cigarettes smoked per day (CPD), which has been commonly used in genetic studies of nicotine use and dependence (Furberg et al., 2010). In SAGE, all subjects are classified into four categories based on participant’s lifetime score for CPD. The ordinal categorization is designed to examine the transition from nicotine use to dependence, ranging from very low dependence to very high dependence. The classes have been scored as very low (0), moderate (1), high (2) and very high (3). The SAGE studies’ assessment plans for personal characteristics (e.g., age) and for environmental conditions and processes (e.g., physical abuse) were also guided by standardized interview protocols and assessments, as described in the prior SAGE publications (Bierut et al., 2002, 2008). Table 1 summarizes demographic details of study samples (e.g., gender and ethnicity). SAGE comprises 1445 female and 1272 male samples, among which 807 samples are African-American and 1910 samples are Caucasian. The mean age from three studies ranges from 36.8 to 42.4.
Genotype and Quality Control
SAGE genotyping was performed at the Johns Hopkins University Center for Inherited Disease Research by using the Illumina Human 1M DNA Analysis BeadChip. The GENEVA Coordinating Center (CC) performed genotype imputation by using the BEAGLE software. Imputation was done separately for subjects with European and African ancestry, with references panels selected from HapMap Phase III populations. The minimum posterior probability required to call a genotype is 0.9. We merged the imputed SNPs with the genotyped SNPs, and then assembled multiple SNPs into 26 ND-related genes based on the Genome Reference Consortium release version 37 (GRCh37). Supplementary Table S2 in the Supplementary Material summarizes the 26 ND-related genes analyzed in this study. Prior to the statistical association analysis, we assessed the quality of the genotype data. As a first step in quality assessment, we examined the proportion of genotype calls for each marker (across all individuals) and for each individual (across all markers). Markers with less than 90% of successful calls were removed. Similarly, individuals with 10% missing genotypes were also excluded from the analysis. For the remaining missing genetic data, we used the average number of minor alleles of the marker to impute the missing values. Markers showing excessive deviations from Hardy-Weinberg equilibrium in the controls were marked and the individuals with unexpected relationships were removed.
Heterogeneity Weighted U Method
Compelling evidence from previous studies suggest that genetic etiology of ND could vary in males and females (Li et al., 2003). To study the heterogeneous etiology of ND, we focused on 26 ND-related genes and investigated their potential roles in ND genetic heterogeneity. Despite the strong evidence of heterogeneity in ND, it is analytically challenging to investigate heterogeneous effects of genetic variants, primarily because the commonly used subset/stratified analysis is subject to issues of reduced sample size and multiple testing. Furthermore, the ND diagnostic assessments can often be of different types (e.g., binary and continuous phenotypes), with unknown underlying distributions (Neale et al., 2006). For example, the commonly used nicotine dependence measurement, CPD, do not follow a standard distribution (e.g., Poisson distribution). Most of the existing methods are parametric-based or semi-parametric-based, which often rely on certain assumptions (e.g., a Poisson distribution assumption). When the assumptions are violated, they are subject to power loss and/or false positive results (Wei et al., 2014).
To address these, we used our recently developed heterogeneity weighted U (HWU) method (Wei et al., 2016) to test the association of the 26 genes with ND considering genetic heterogeneity. The method is based on a weighted U statistic, which assume no specific distribution for phenotypes and can be applied to different types of ND phenotypes (e.g., binary, ordinal, and continuous phenotypes). It is also a similarity-based method, which can be applied to high-dimensional genetic data (i.e., the number of SNPs in a gene can be great than the study sample size). HWU is constructed based on the idea that the more similar subjects are, the more similar their genetic effects are (Wei et al., 2016). According to the idea, we defined HWU as,
where Si,j is the phenotypic similarity between subjects i and j, and wi,j is a weight function measuring the genetic similarity defined as In this paper, we use cross-product kernel to measure the genetic similarity and use gender to infer the latent population structure by defining , where for male and female, respectively. Under the null hypothesis, there is no association between phenotypic similarity and genetic similarity. Under the alternative, the phenotypic similarity increases with the increase of the genetic similarity. By constructing a background similarity on variables capturing heterogeneous groups (e.g., gender groups) and incorporating it into the weighted U framework, it can consider genetic heterogeneity in the association analysis. The method was implemented in a C++ software1. By applying the software to each of 26 candidate genes, we evaluated their association with ND with the consideration of possible heterogeneous effects in gender. To consider the potential confounding effects, we adjusted the analysis for gender, race, study sites and the top four principal components calculated from the genome-wide data. Because HWU is a non-parametric method, it only evaluates the significance of association but not measures of association. To measure the association, regression-based methods, such as zero inflated Poisson model, can be used.
Results
Gene-Based Association Analysis
Previous study showed that males and females differ in ND, which was also suggested by our own data (Table 2). Considering that the genetic etiology of ND could be heterogeneous in gender (Li et al., 2003), we applied the HWU method to 26 genes previously reported to be associated with ND, investigating potential genetic heterogeneity of these genes in males and females. We started the analysis with an overall association analysis considering potential genetic heterogeneous in gender (i.e., by incorporating the gender similarity into the association test).
By considering genetic heterogeneity in gender, the analysis identified 24 genes significantly associated with CPD (Table 3). After Bonferroni correction, 17 genes remained statistically significant. The genes included 16 CHRN genes (including ATR1, IREB2, MINK1, and PTK2B), and 1 CYP genes. Four of the significant CHRN genes were on chromosome 8, 5 were on chromosome 15, 2 were on chromosome 17, and other six genes were located on different chromosomes locations. Comparatively, only one gene was significant after Bonferroni correction, while the genetic heterogeneity in gender was not considered. The detailed association results are summarized in Table 3. Additional analysis using FDR obtained similar results.
Associated Analysis of Two ND-Related Gene Clusters
Following the single-gene analysis, we also conducted a joint association analysis of two gene clusters, CHRNA5-CHRNA3-CHRNB4 and CHRNB3-CHRNA6. The CHRNA5-CHRNA3-CHRNB4 cluster is on chromosome 15 and is of particular interest for its established association with ND. The association were identified and replicated in multiple studies (Saccone et al., 2007, 2009; Wen et al., 2016a). The CHRNB3-CHRNA6 cluster is on chromosome 8. The protein produced by the gene cluster are co-localized in nAChRs in the substantia nigra, ventral tegmental area, striatum, and locus coeruleus. While it has been less studied than the CHRNA5-CHRNA3-CHRNB4, studies have found SNPs in the CHRNB3-CHRNA6 cluster are also significantly associated with ND (Culverhouse et al., 2014; Wen et al., 2016b).
The results of the joint analysis of the two gene clusters are summarized in Table 4. As expected, we found that the CHRNA5-CHRNA3-CHRNB4 cluster and CHRNB3-CHRNA6 cluster were significantly associated with ND in our study with or without considering gender heterogeneity. However, by considering gender heterogeneity, both gene clusters attained stronger associations with ND. The evidence of gender heterogeneity is particular strong in the CHRNB3-CHRNA6 cluster. Furthermore, the joint analysis of CHRNB3-CHRNA6 cluster attains a stronger association (p-value = 2.11E-07) than the single-gene analysis of CHRNB3 (p-value = 1.18E-05) and CHRNA6 (p-value = 1.98E-06).
In order to further explore the association of the CHRNB3-CHRNA6 gene cluster with ND, we performed a single-locus analysis of all SNPs located in these two gene clusters by using HWU. The results are summarized in Figure 1. From the single-locus analysis, we found six SNPs within or near CHRNA6 were in complete linkage disequilibrium (LD) and were highly associated with CPD (P-value = 4.97E-06). In addition, 5 SNPs within or near CHRNB3 reach significance level of 1.25E-04 and are also in complete LD. Additional results (e.g., coefficient estimates) from stratified analysis also suggest that there are heterogeneous effects of the two genes in males and females. For example, by using a zero inflated Poisson model, we found rs10109040 in CHRNA6 has a coefficient estimate of −0.18 with a p-value of 2.47E-13 in males and a coefficients estimate of 0.026 with a p-value of 0.23 in females; rs10958727 in gene CHRNB3 has a coefficient estimate of −0.18 with a p-value of 3.35E-14 in males and a coefficient estimate of −0.016 with a p-value of 0.45 in females.
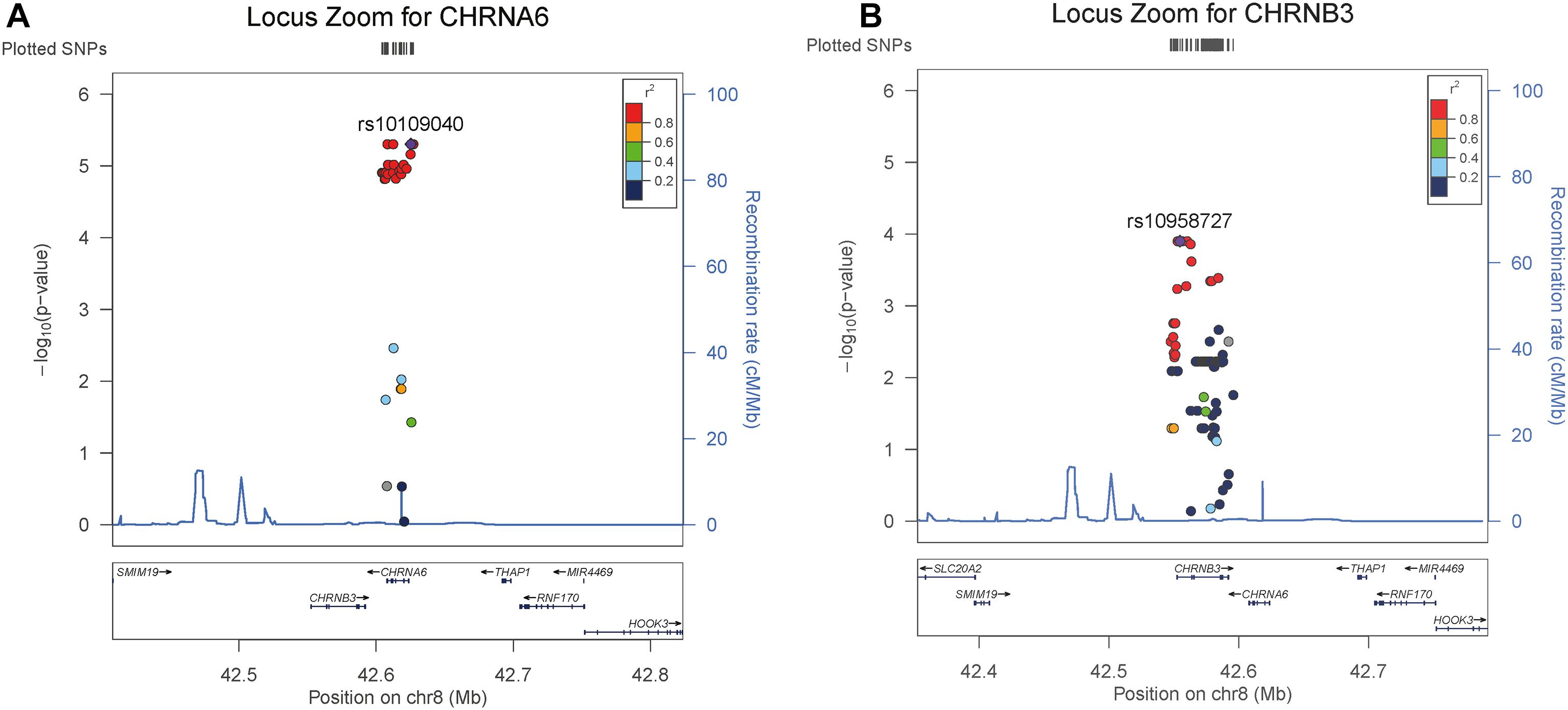
Figure 1. Summary of single-locus analysis of two interested genes by considering genetic heterogeneity; Panel (A) includes 6 SNPs within or near CHRNA6 that are in complete linkage disequilibrium (LD) and are highly associated with CPD (P-value = 4.97E-06); Panel (B) includes five SNPs within or near CHRNB3 that reach significance level of 1.25E-04 and are also in complete LD.
Discussion
In this study, we investigated the heterogeneous effects of 26 ND-associated genes in males and females. We found 17 genes significantly associated with ND by considering genetic heterogeneity in males and females. The most significant findings were from two nAChRs subunit genes, CHRNA6 and CHRNB3, of which we found strong evidence of gender heterogeneity. The Human Protein Atlas2 shows that CHRNB3 is expressed mostly in the brain, pancreas, and testis, and is a male-tissue-enriched gene (Fagerberg et al., 2014). On the other hand, CHRNA6 is expressed specifically in the female tissues (e.g., the fallopian tube) and has almost no expression in male tissues. Our analysis indicated that variants in CHRNA6 and CHRNB3 (i.e., rs10109040 and rs10958727) had significant effects on ND in males but not in females. While the finding from CHRNB3 is consistent with previous literature, the one from CHRNA6 is not supported by previous findings. Significant association of CHRNA6 with ND in males has also been reported in a recent study (Wen et al., 2017), which could be explained by the high linkage disequilibrium between CHRNA6 and CHRNB3 (i.e., it may not be a real association signal).
Another group of interesting genes were from the CHRNA5-CHRNA3-CHRNB4 cluster. Numerous studies suggested that the CHRNA5-CHRNA3-CHRNB4 genes cluster contributed to ND, which was also confirmed by our analysis. Several studies showed that the rare variants in CHRNA3 and CHRNB4 were associated with decreased risk for ND. Furthermore, the carriers of missenses variants in the CHRNB4 were found to smoke less cigarettes per day (Haller et al., 2012; Wen et al., 2016a). The non-synonymous SNP rs16969968 is a well-known risk variant in CHRNA5, which has been replicated in many studies (Saccone et al., 2009; Wen et al., 2016a). While genes in the CHRNA5-CHRNA3-CHRNB4 cluster are well-known for their role in ND, our study shows there is limited evidence of gender heterogeneity of these genes, which is consistent with findings from previous studies.
Biological studies showed that CHRNA4 and CHRNB2 could be related to ND (Marks et al., 1992; Whiteaker et al., 1998). Previous studies found that CHRNA4 and CHRNB2 were over-expressed under chronic nicotine exposure. Finding from another study also suggested that functional rare variants in CHRNA4 might reduce ND risk (Xie et al., 2011). While existing literatures have been controversial on the association of CHRNA4 and CHRNB2 with ND, results from our association analysis supports the association of these two genes with ND.
CHRNA1, CHRNA7, and CHRNB1 have been less studied for their role in ND. However, there is evidence that genetic variants in these genes contribute to ND (Philibert et al., 2009). Experiment studies reported that the CHRNA7 gene within the ventral tegmental area was directly involved in nicotine tolerance and withdrawal (Nomikos et al., 2000; Ryan and Loiacono, 2001; Grabus et al., 2005). Moreover, a study showed the association of CHRN genes (e.g., CHRNA7) with nicotine dependence differed in males and females (Philibert et al., 2009).
In this study, we also studied the relationship between nicotine dependence and two CYP genes, CYP2A6 and CYP2B6. CYP genes encode the enzyme for the metabolism of xenobiotics in the body. Studies showed that CYP2A6 influenced nicotine metabolism (Malaiyandi et al., 2005), while there was also evidence that this gene is not related with ND (Bandiera et al., 2015). Our finding is in line with the latter study. CYP2B6 is associated with smoking behaviors and ND in human brain, and relapse could occur due to the rise of nicotine level in brain with CYP2B6 activity dropping (Garcia et al., 2015).
The ND measurement, such as CPD, does not have a known underlying distribution. This complexity, however, has not been carefully considered in the existing analytic method. In our analysis, we adopted a new non-parametric method, HWU, which made no assumptions on phenotype distribution and therefore provided a robust and powerful performance for association analysis. Moreover, in HWU, we integrated the latent population structure (e.g., inferred from gender) into a weight function and test heterogeneous effects without stratifying the sample, which addressed the issues of reduced sample size and multiple testing. By using the new method, we were able to identify genes, such as CHRNA6 and CHRNB3, associated with ND. To show the evidence of gender heterogeneity, we also performed an analysis without considering the heterogeneous effects. We found that most genes were not significantly associated with ND in this additional analysis, which could suggest the importance of considering gender heterogeneity. If the genetic mechanisms differ in male and female, performing the analysis without considering genetic heterogeneity will likely lead to low power of the study.
In summary, our findings are consistent with existing biological lectures or previous association analysis. While this study reveals potential heterogeneous effects of several ND-associated genes in gender, this is an initial effort to study genetic heterogeneity in ND. Future studies are required to replicate the findings from our analysis and further investigate genetic heterogeneity in ND. Because HWU is a non-parametric method, it is not straightforward to incorporate a variable selection algorithm into HWU. Alternatively, we could screen SNPs based on information theory, such as using an diSNP pre-selection method (Cen et al., 2012; Wu and Cui, 2014), which could potentially improve the power of association analysis. In addition to HWU, other high-dimensional statistical methods, especially robust variable selection methods considering genetic heterogeneity (Wu and Ma, 2015), can be adopted for association analysis considering genetic heterogeneity.
Author Contributions
QL directed the study and contributed to the data interpretation. XT conducted the quality control process and modified the HWU method. XZ and TL performed the analysis. XZ and TM were involved in the result interpretation. XZ drafted the manuscript. TW, WX, and GL provided the suggestions and revised the manuscript. All authors reviewed and improved the submitted manuscript.
Funding
This research was supported, in part, by the National Institute on Drug Abuse under Award Number R01DA043501 and the National Library of Medicine under Award Number R01LM012848, and also support for the study of addiction: Genetics and Environment (SAGE) was provided through the NIH Genes, Environment and Health Initiative (GEI) (U01 HG004422).
Conflict of Interest Statement
The authors declare that the research was conducted in the absence of any commercial or financial relationships that could be construed as a potential conflict of interest.
Acknowledgments
We thank Jim Anthony and Melanie Noell Bernard for helpful discussions.
Supplementary Material
The Supplementary Material for this article can be found online at: https://www.frontiersin.org/articles/10.3389/fgene.2019.00448/full#supplementary-material
Footnotes
References
Bandiera, F. C., Ross, K. C., Taghavi, S., Delucchi, K., Tyndale, R. F., and Benowitz, N. L. (2015). Nicotine dependence, nicotine metabolism, and the extent of compensation in response to reduced nicotine content cigarettes. Nicotine Tob. Res. 17, 1167–1172. doi: 10.1093/ntr/ntu337
Bierut, L. J., Madden, P. A. F., Breslau, N., Johnson, E. O., Hatsukami, D., Pomerleau, O. F., et al. (2007). Novel genes identified in a high-density genome wide association study for nicotine dependence. Hum. Mol. Genet. 16, 24–35. doi: 10.1093/hmg/ddl441
Bierut, L. J., Saccone, N. L., Rice, J. P., Goate, A., Foroud, T., Edenberg, H., et al. (2002). Defining alcohol-related phenotypes in humans: the collaborative study on the genetics of alcoholism. Alcohol Res. Health 26, 208–213.
Bierut, L. J., Strickland, J. R., Thompson, J. R., Afful, S. E., and Cottler, L. B. (2008). Drug use and dependence in cocaine dependent subjects, community-based individuals, and their siblings. Drug Alcohol Depend. 95, 14–22. doi: 10.1016/j.drugalcdep.2007.11.023
Cen, W., Shaoyu, L., and Yuehua, C. (2012). Genetic association studies: an information content perspective. Curr. Genomics 13, 566–573. doi: 10.2174/138920212803251382
Culverhouse, R. C., Johnson, E. O., Breslau, N., Hatsukami, D. K., Sadler, B., Brooks, A. I., et al. (2014). Multiple distinct CHRNB3-CHRNA6 variants are genetic risk factors for nicotine dependence in African Americans and European Americans. Addiction 109, 814–822. doi: 10.1111/add.12478
Fagerberg, L., Hallström, B. M., Oksvold, P., Kampf, C., Djureinovic, D., Odeberg, J., et al. (2014). Analysis of the human tissue-specific expression by genome-wide integration of transcriptomics and antibody-based proteomics. Mol. Cell. Proteomics 13, 397–406. doi: 10.1074/mcp.M113.035600
Furberg, H., Kim, Y., Dackor, J., Boerwinkle, E., Franceschini, N., Ardissino, D., et al. (2010). Genome-wide meta-analyses identify multiple loci associated with smoking behavior. Nat. Genet. 42, 441–447. doi: 10.1038/ng.571
Garcia, K. L., Coen, K., Miksys, S., Le, A. D., and Tyndale, R. F. (2015). Effect of brain CYP2B inhibition on brain nicotine levels and nicotine self-administration. Neuropsychopharmacology 40, 1910–1918. doi: 10.1038/npp.2015.40
Grabus, S. D., Martin, B. R., and Imad Damaj, M. (2005). Nicotine physical dependence in the mouse: involvement of the alpha7 nicotinic receptor subtype. Eur. J. Pharmacol. 515, 90–93. doi: 10.1016/j.ejphar.2005.03.044
Haller, G., Druley, T., Vallania, F. L., Mitra, R. D., Li, P., Akk, G., et al. (2012). Rare missense variants in CHRNB4 are associated with reduced risk of nicotine dependence. Hum. Mol. Genet. 21, 647–655. doi: 10.1093/hmg/ddr498
Hancock, D. B., Wang, J. C., Gaddis, N. C., Levy, J. L., Saccone, N. L., Stitzel, J. A., et al. (2015). A multiancestry study identifies novel genetic associations with CHRNA5 methylation in human brain and risk of nicotine dependence. Hum. Mol. Genet. 24, 5940–5954. doi: 10.1093/hmg/ddv303
Li, M. D., Cheng, R., Ma, J. Z., and Swan, G. E. (2003). A meta-analysis of estimated genetic and environmental effects on smoking behavior in male and female adult twins. Addiction 98, 23–31.
Liu, J. Z., Tozzi, F., Waterworth, D. M., Pillai, S. G., Muglia, P., Middleton, L., et al. (2010). Meta-analysis and imputation refines the association of 15q25 with smoking quantity. Nat. Genet. 42, 436–440. doi: 10.1038/ng.572
Malaiyandi, V., Sellers, E. M., and Tyndale, R. F. (2005). Implications of CYP2A6 genetic variation for smoking behaviors and nicotine dependence. Clin. Pharmacol. Ther. 77, 145–158. doi: 10.1016/j.clpt.2004.10.011
Marks, M. J., Pauly, J. R., Gross, S. D., Deneris, E. S., Hermans-Borgmeyer, I., Heinemann, S. F., et al. (1992). Nicotine binding and nicotinic receptor subunit RNA after chronic nicotine treatment. J. Neurosci. 12, 2765–2784.
Neale, M. C., Aggen, S. H., Maes, H. H., Kubarych, T. S., and Schmitt, J. E. (2006). Methodological issues in the assessment of substance use phenotypes. Addict. Behav. 31, 1010–1034.
Nomikos, G. G., Schilström, B., Hildebrand, B. E., Panagis, G., Grenhoff, J., and Svensson, T. H. (2000). Role of alpha7 nicotinic receptors in nicotine dependence and implications for psychiatric illness. Behav. Brain Res. 113, 97–103.
Philibert, R. A., Todorov, A., Andersen, A., Hollenbeck, N., Gunter, T., Heath, A., et al. (2009). Examination of the nicotine dependence (NICSNP) consortium findings in the Iowa adoption studies population. Nicotine Tob. Res. 11, 286–292. doi: 10.1093/ntr/ntn034
Ryan, R. E., and Loiacono, R. E. (2001). Nicotine regulates alpha7 nicotinic receptor subunit mRNA: implications for nicotine dependence. Neuroreport 12, 569–572.
Saccone, N. L., Wang, J. C., Breslau, N., Johnson, E. O., Hatsukami, D., Saccone, S. F., et al. (2009). The CHRNA5-CHRNA3-CHRNB4 nicotinic receptor subunit gene cluster affects risk for nicotine dependence in African-Americans and in European-Americans. Cancer Res. 69, 6848–6856. doi: 10.1158/0008-5472.CAN-09-0786
Saccone, S. F., Hinrichs, A. L., Saccone, N. L., Chase, G. A., Konvicka, K., Madden, P. A. F., et al. (2007). Cholinergic nicotinic receptor genes implicated in a nicotine dependence association study targeting 348 candidate genes with 3713 SNPs. Hum. Mol. Genet. 16, 36–49.
Sullivan, P. F., Neale, B. M., van den Oord, E., Miles, M. F., Neale, M. C., Bulik, C. M., et al. (2010). Candidate genes for nicotine dependence via linkage, epistasis, and bioinformatics. Am. J. Med. Genet. B Neuropsychiatr. Genet. 126B, 23–36.
Thorgeirsson, T. E., Gudbjartsson, D. F., Surakka, I., Vink, J. M., Amin, N., Geller, F., et al. (2010). Sequence variants at CHRNB3-CHRNA6 and CYP2A6 affect smoking behavior. Nat. Genet. 42, 448–453. doi: 10.1038/ng.573
Thornton-Wells, T. A., Moore, J. H., and Haines, J. L. (2004). Genetics, statistics and human disease: analytical retooling for complexity. Trends Genet. 20, 640–647.
Wei, C., Elston, R. C., and Lu, Q. (2016). A weighted u statistic for association analyses considering genetic heterogeneity. Stat. Med. 35, 2802–2814. doi: 10.1002/sim.6877
Wei, C., Li, M., He, Z., Vsevolozhskaya, O., Schaid, D. J., and Lu, Q. (2014). A weighted u statistic for genetic association analyses of sequencing data. Genet. Epidemiol. 38, 699–708. doi: 10.1002/gepi.21864
Wei, J., Chu, C., Wang, Y., Yang, Y., Wang, Q., Li, T., et al. (2012). Association study of 45 candidate genes in nicotine dependence in Han Chinese. Addict. Behav. 37, 622–626. doi: 10.1016/j.addbeh.2012.01.009
Wen, L., Han, H., Liu, Q., Su, K., Yang, Z., Cui, W., et al. (2017). Significant association of the CHRNB3-CHRNA6 gene cluster with nicotine dependence in the Chinese Han population. Sci. Rep. 7:9745. doi: 10.1038/s41598-017-09492-8
Wen, L., Jiang, K., Yuan, W., Cui, W., and Li, M. D. (2016a). Contribution of variants in CHRNA5/A3/B4 gene cluster on chromosome 15 to tobacco smoking: from genetic association to mechanism. Mol. Neurobiol. 53, 472–484. doi: 10.1007/s12035-014-8997-x
Wen, L., Yang, Z., Cui, W., and Li, M. D. (2016b). Crucial roles of the CHRNB3-CHRNA6 gene cluster on chromosome 8 in nicotine dependence: update and subjects for future research. Transl. Psychiatry 6:e843. doi: 10.1038/tp.2016.103
Whiteaker, P., Sharples, C. G., and Wonnacott, S. (1998). Agonist-induced up-regulation of alpha4beta2 nicotinic acetylcholine receptors in M10 cells: pharmacological and spatial definition. Mol. Pharmacol. 53, 950–962.
Wu, C., and Cui, Y. (2014). Boosting signals in gene-based association studies via efficient SNP selection. Brief. Bioinform. 15, 279–291. doi: 10.1093/bib/bbs087
Wu, C., and Ma, S. (2015). A selective review of robust variable selection with applications in bioinformatics. Brief. Bioinform. 16, 873–883. doi: 10.1093/bib/bbu046
Keywords: nicotine dependence, genetic heterogeneity, nAChRs genes, SAGE, weighted U
Citation: Zhang X, Lan T, Wang T, Xue W, Tong X, Ma T, Liu G and Lu Q (2019) Considering Genetic Heterogeneity in the Association Analysis Finds Genes Associated With Nicotine Dependence. Front. Genet. 10:448. doi: 10.3389/fgene.2019.00448
Received: 01 January 2019; Accepted: 30 April 2019;
Published: 17 May 2019.
Edited by:
Mogens Fenger, Capital Region of Denmark, DenmarkReviewed by:
Alexandre Bureau, Laval University, CanadaCen Wu, Kansas State University, United States
Copyright © 2019 Zhang, Lan, Wang, Xue, Tong, Ma, Liu and Lu. This is an open-access article distributed under the terms of the Creative Commons Attribution License (CC BY). The use, distribution or reproduction in other forums is permitted, provided the original author(s) and the copyright owner(s) are credited and that the original publication in this journal is cited, in accordance with accepted academic practice. No use, distribution or reproduction is permitted which does not comply with these terms.
*Correspondence: Guifen Liu, TGl1Z2Y2NkAxMjYuY29t Qing Lu, cWx1QGVwaS5tc3UuZWR1