- 1Department of Plant Breeding, Swedish University of Agricultural Sciences, Alnarp, Sweden
- 2Institute of Agriculture, Lithuanian Research Centre for Agriculture and Forestry (LAMMC), Akademija, Lithuania
- 3Nordic Genetic Resource Centre, Alnarp, Sweden
Septoria tritici blotch (STB) disease caused by Zymoseptoria tritici is one of the most damaging diseases of wheat causing significant yield losses worldwide. Identification and employment of resistant germplasm is the most cost-effective method to control STB. In this study, we characterized seedling stage resistance to STB in 175 winter wheat landraces and old cultivars of Nordic origin. The study revealed significant (p < 0.05) phenotypic differences in STB severity in the germplasm. Genome-wide association analysis (GWAS) using five different algorithms identified ten significant markers on five chromosomes. Six markers were localized within a region of 2 cM that contained seven candidate genes on chromosome 1B. Genomic prediction (GP) analysis resulted in a model with an accuracy of 0.47. To further improve the prediction efficiency, significant markers identified by GWAS were included as fixed effects in the GP model. Depending on the number of fixed effect markers, the prediction accuracy improved from 0.47 (without fixed effects) to 0.62 (all non-redundant GWAS markers as fixed effects), respectively. The resistant genotypes and single-nucleotide polymorphism (SNP) markers identified in the present study will serve as a valuable resource for future breeding for STB resistance in wheat. The results also highlight the benefits of integrating GWAS with GP to further improve the accuracy of GP.
Introduction
Septoria tritici blotch (STB) disease caused by fungal pathogen Zymoseptoria tritici is one of the devastating foliar diseases of wheat in the temperate regions worldwide. STB causes significant yield losses and additional fungicide expenses (Fones and Gurr, 2015; Torriani et al., 2015). The annual harvest losses reach 5% to 10% in the biggest EU wheat producing countries (Fones and Gurr, 2015). Cultivation of resistant cultivars in combination with fungicide application is the main strategy to control the disease. Besides, a major problem of the intensive use of fungicides is that many populations of Z. tritici have rapidly evolved resistance to its active agents (Torriani et al., 2009; Wieczorek et al., 2015; Cheval et al., 2017). Therefore, novel sources of resistance to STB and their introgression into wheat breeding programs is the most economical and environmentally friendly strategy for effective management of the disease.
So far, 21 genes are mapped for resistance to STB in wheat (Brown et al., 2015). The expression pattern and effect of these genes on resistance to STB differ in seedling and adult plant stages. For example, Stb16 is expressed and effective at the seedling and adult stages of plants while Stb17 is expressed only at the adult stage (Tabib Ghaffary et al., 2011). Stb18 is an isolate-specific resistance gene that shows variable resistance to Z. tritici at seedling and adult stages depending on the isolate (Tabib Ghaffary et al., 2011). Stb6 and Stb15 are the two most common STB resistance genes in the current European germplasm (Arraiano and Brown, 2006). Stb15 was found in about 60% of cultivars tested but, unlike Stb6, Stb15 is not known to show resistance under field conditions (Arraiano et al., 2009; Brown et al., 2015). The only qualitative gene for STB resistance Stb6 (Saintenac et al., 2018) and recently identified avirulence gene AvrStb6 of Z. tritici (Zhong et al., 2017) have been shown to be in a gene-for-gene relationship. Stb6 is among the most frequent STB genes in European wheat germplasm and suggested as the most widespread STB gene in the contemporary wheat breeding programs (Arraiano and Brown, 2017). However, this gene alone is not sufficient to provide adequate resistance to STB, and there are no other known resistance genes contributing significantly to the reduction of Z. tritici populations in Europe (Arraiano et al., 2009). The majority of variation in field resistance to STB is controlled by quantitative resistance, and the progress in breeding for STB resistance over the last 30 years presumably happened by the gradual accumulation of minor genes. Recently, it was shown that the STB disease symptoms chlorosis, necrosis, and pycnidia are under varying genetic control (Odilbekov et al., 2019). Therefore, there is a need to search for new sources of durable disease resistance to STB for marker-assisted introgression into elite wheat cultivars (Fones and Gurr, 2015; McDonald and Mundt, 2016).
Wheat landraces are a valuable source of genetic diversity. They are adapted to the environmental conditions of their place of origin and thus can provide novel sources of disease resistance for developing new cultivars adapted to the changing climate (de Carvalho et al., 2012; Lopes et al., 2015). Several useful agronomic and resistance traits have been introgressed from landraces to commercial wheat cultivars including the dwarfing gene Rht from the Japanese landrace Shiro Daruma (Dreisigacker et al., 2005) and the high grain protein content gene NAM-B1 in Fennoscandian wheat (Hagenblad et al., 2012). Valuable landraces and old cultivars of winter wheat consisting of more than 300 genotypes from Scandinavian countries is preserved at the Nordic Genetic Resource Centre (NordGen, Alnarp, Sweden), and part of this material was evaluated earlier for several agronomic traits and showed high diversity in morphological traits (Diederichsen et al., 2012), resistance to rust (Randhawa et al., 2016) and powdery mildew (Hysing et al., 2007). These studies prove that the material stored at NordGen is unique and a genetically diverse resource, which can be utilized for the improvement of wheat cultivars for Nordic and Baltic Sea Region countries (Chawade et al., 2018).
Genome-wide association studies (GWAS) and genomic selection (GS), both performed with genome-wide markers are important and effective tools for plant breeding. GWAS estimates marker effects across the whole genome on the target population based on prediction models (Desta and Ortiz, 2014). Based on linkage disequilibrium (LD), GWAS can identify new functional alleles (identify novel genes and QTLs) for many agriculturally important traits in diverse germplasm. Few GWAS studies were performed for STB resistance in European winter wheat accessions (Kollers et al., 2013; Miedaner et al., 2013; Vagndorf et al., 2017). Many regions associated with resistance to STB in the wheat genome were identified in these studies. In a study of 1,055 elite hybrids and their corresponding 87 parental lines, Miedaner et al. (2013) identified four significant single-nucleotide polymorphisms (SNP) associated with STB resistance located on chromosomes 1B, 2B, 5B, and 6A. Kollers et al. (2013) detected 39 SSR on 2A, 2D, 3A, 5B, 7A, 7D significantly associated with adult plant resistance in a panel of 372 European wheat lines. Four QTL, on chromosomes 1B, 2A, 5D, and 7A were highly associated with STB resistance in 164 North European cultivars and breeding lines (Vagndorf et al., 2017).
GS, on the other hand, enables the selection of superior genotypes based on genomic estimated breeding values (GEBV) to create models for the prediction of phenotypes in uncharacterized populations (Meuwissen et al., 2001). Previous studies have shown the feasibility of GS for predicting STB resistance in wheat. Juliana et al. (2017) achieved a mean genomic prediction (GP) accuracy of 0.45 for adult plant resistance to STB in a population of 333 and 314 advanced lines from Centro Internacional de Mejoramiento de Maíz y Trigo‘s (CIMMYT) wheat breeding program. Muqaddasi et al. (2019) investigated the potential of GP of adult stage STB infection in a European winter wheat panel of 371 elite varieties, resulting in both additive and non-additive prediction models centered around a mean GP accuracy of approximately 0.43. Spindel et al. (2016) described the new combined GS + GWAS model based only on the results of GWAS run using GS training population data. GS + GWAS has some benefits as the method does not require additional data as the same phenotype and genotype data set is used, prediction accuracy can be enhanced, and it can be more accessible to breeders as it does not require extensive knowledge on the underlying genetics of a trait of interest (Spindel et al., 2016).
Previous studies were primarily focused on resistance to STB in the adult stage of winter wheat germplasm. One of the main goals of this project was to characterize seedling stage resistance to STB in winter wheat landraces and old cultivars of Nordic origin which are well adapted to the Nordic climate. The current study relies on a collection of 175 winter wheat accessions, released between 1900 and 2012. In this work, this material was evaluated for seedling-stage resistance to STB disease. The objectives of this study were (i) to detect novel STB disease resistance loci at the seedling stage by performing GWAS analysis; (ii) to identify candidate genes to STB resistance in wheat; (iii) to evaluate GP (GP) for selection for STB resistance; and (iv) to employ GP+GWAS to further improve the accuracy of GP.
Materials and Methods
Plant Material
The material in this study comprised of 175 winter wheat cultivars and landraces (hereafter genotypes) mainly from Scandinavian countries (Supplementary Table 1). The collected genotypes were released between 1900 and 2012 and representing a century of winter wheat breeding history of the region. Four genotypes originating from Germany were also included as they have been widely grown in the Scandinavian area. The seeds were obtained from Nordic Genetic Resources Centre, Alnarp, Sweden (NordGen).
Growth Conditions
The seeds were placed on a moist filter paper in Petri dishes and kept for 4 days at +4°C in dark. Afterwards, they were transferred to room temperature conditions for two days for germination. Thereafter, the germinated seeds were sown in plastic pots (8 × 8 × 8 cm) filled with peat substrate. Two seeds of each genotype were sown per pot. Plants were grown in the Biotron greenhouse chamber at 24°C with a 16-h photoperiod and 60% humidity. The light intensity was set and controlled at 250 µmol m−2·s−1. The samples were arranged in an augmented design with eight blocks designed with the R package Agricolae (Mendiburu, 2017). Four genotypes were used as checks in each block to control block effect, namely, Nimbus (susceptible), Nelson and Target (moderately resistant), and Kranich (resistant). The entire experiment was performed twice with 1-month interval, and two replications were done at each occasion.
Inoculation and Disease Assessment
The Z. tritici strain was isolated from typical STB lesions on leaves of winter wheat collected in southern part of Sweden during 2015, and the inoculum was prepared as described previously (Odilbekov et al., 2018). Second and third leaves of the seedlings were marked close to the stem with a permanent marker before inoculation. The twentyone day old wheat seedlings were inoculated with Z. tritici inoculum using a hand sprayer with a spore concentration of 107 spores ml–1. The inoculum was applied on the leaves three times, and leaves were allowed to dry for 1 h each time. The inoculated seedlings were transferred to fully controlled daylight chamber and kept 72 h under close to 100% relative humidity at 24°C with a 16-h photoperiod and a light intensity of 250 µmol m−2·s−1. Relative humidity was reduced to 65% 72 h post-inoculation. Percentage of the necrotic area on the inoculated leaf surface (from 0% to 100%) was visually scored at 13, 16, and 19 days post-inoculation (dpi). The lesion development over the assessment period was summarized through the computation of the relative area under the disease progress curve (rAUDPC). The entire experiment was repeated twice.
Genotypic Data and Population Structure
The samples for DNA extraction were collected from 6-week-old seedling and the DNA extraction and genotyping of the samples was performed by TraitGenetics GmbH, Germany (www.traitgenetics.com/en/). The samples were genotyped with a 20K SNP wheat marker array. A total of 6,097 SNPs were used for GWAS after removing SNPs with more than 20% missing data as well as a minor allele frequency less than 5%. Principal component analysis (PCA) was done with the software Simca 14 (Umetrics, Sweden).
GWAS and GP
GWAS analysis was done with the GAPIT package (v3.0) in R (Tang et al., 2016). The primary model was constructed with the GLM algorithm (Lipka et al., 2012) with 10 principal components as covariates and MAF threshold of 0.05. A QTL was considered significant at the threshold of adjusted false discovery rate (FDR) < 0.05. New GWAS models were developed using MLM, MLMM, FarmCPU, and Super algorithm in GAPIT for verification of the QTL obtained with GLM. GP modeling was done using the R package rrBLUP (v4.6) (Endelman, 2011) for ridge-regression-based genome-wide regression. The rrBLUP model for genome-wide regression assumes the form y = Xb + Zu, where X and Z are the design matrices for fixed and random effects, respectively, b and u are vectors of fixed and random effects, and y is a vector of phenotypic values. Similar to the method proposed by Spindel et al. (2016), significant markers identified by GWAS results were included as fixed effects in the GS model and removed from the design matrix of random effects. To identify the best subset of GWAS-selected markers to include as fixed effects, all possible permutations of available GWAS-selected markers, were evaluated with respect to average model accuracy. Number of markers in the marker sets ranged from one (a single marker added as fixed effect) to five (all available markers). The GP models were validated on a set of 500 random 80/20 train/test set splits. Model accuracy was assessed by calculating Pearson’s correlation coefficient between the predicted and observed STB resistance for each of the train/test sets and estimating the average of all correlation values for each run. The best performing model was selected on the basis of the highest average model accuracy. The GP models with markers fitted as fixed effects were compared to a GP model which did not use GWAS-selected markers as fixed effects, instead fitting all available markers as random effects, and was also compared to models that mimicked the model configuration of the fixed effect models described above, but which instead sampled random markers (as opposed to selecting markers based on highest significance in a GWAS). The subset sizes used for the models using the randomly selected markers ranged from one to five. Each subset size was evaluated five times, with a new random draw of markers. The initially described model which fit all markers as random effects, and the models fitting randomly selected markers as fixed effects, were all validated against the same 500 train/test splits as the GWAS-selected marker models.
Identification of Candidate Genes
The physical positions of the significant markers from the GLM model were identified by BLASTing their sequences against the IWGSC RefSeq v1.0 genome. The physical location of flanking markers BobWhite_c42716_71 and BS00110231_51 fell into range of 623,712,765 to 623,989,423 bp in the region of chromosome 1B. The candidate genes physically located within this range were identified, and their gene annotation was extracted from IWGSC RefSeq v1.0 genome.
Results
Phenotypic Diversity
The Z. tritici isolate was evaluated on a differential set of wheat cultivars with known Stb resistance genes (Supplementary Figure 1). The evaluation of 175 genotypes showed that the phenotypic distribution of STB severity followed approximately a normal distribution (Figure 1). Highly significant (p < 0.05) phenotypic differences in STB severity were observed in the germplasm (Table S1). The mean of the rAUDPC values ranged from 0.33 for the most resistant and 2.07 for most susceptible genotypes, respectively. Tukey multiple comparison test showed that the genotypes Kranich, Starke, Galicia, and Cymbal exhibited a higher level of resistance to STB while the lower level of resistance was found in genotypes such as Penta, Sejet, Svea I, and Gluten.
Population Structure
To identify underlying genetic differences, PCA and Kinship analyses were performed on the genotypes based on 6,097 SNPs. The first and second principal components accounted for 12.3% and 10.03% of the variance, respectively. The genotypes were clustered into three major groups, and the clustering was mainly based on their geographic origin (Figure 2A). The genotypes with origin from Denmark and Finland formed two very distinct clusters, whereas the Swedish genotypes could be considered intermediate between these two clusters. The result from PCA revealed that most of the genotypes with a higher level of resistance belong to the modern wheat cultivars while most of the susceptible genotypes belonged to older released ones (Figures 2B, C). A similar result to PCA was also observed by using Kinship analysis where three different clusters were identified (Figure 3).
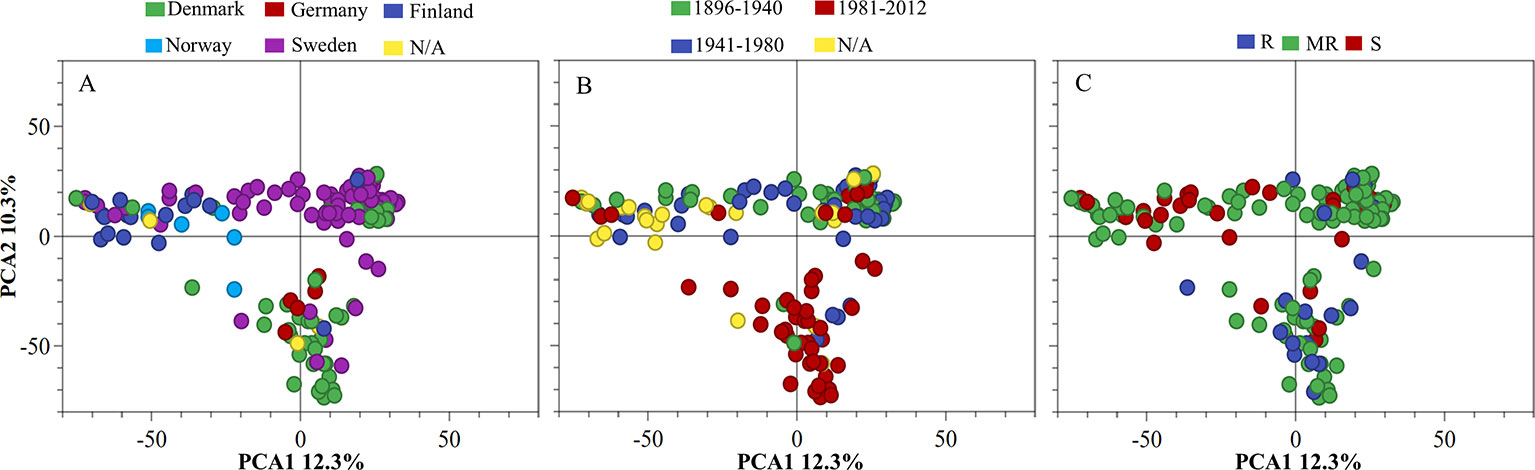
Figure 2 Principal component analysis (PCA) of 175 winter wheat cultivars/landraces coloured and labelled by (A) country of origin, (B) released year and (C) resistance/susceptibility. PCA was based on the allele frequencies of 6097 SNP markers. R, resistant; MR, moderate resistant; S, susceptible.
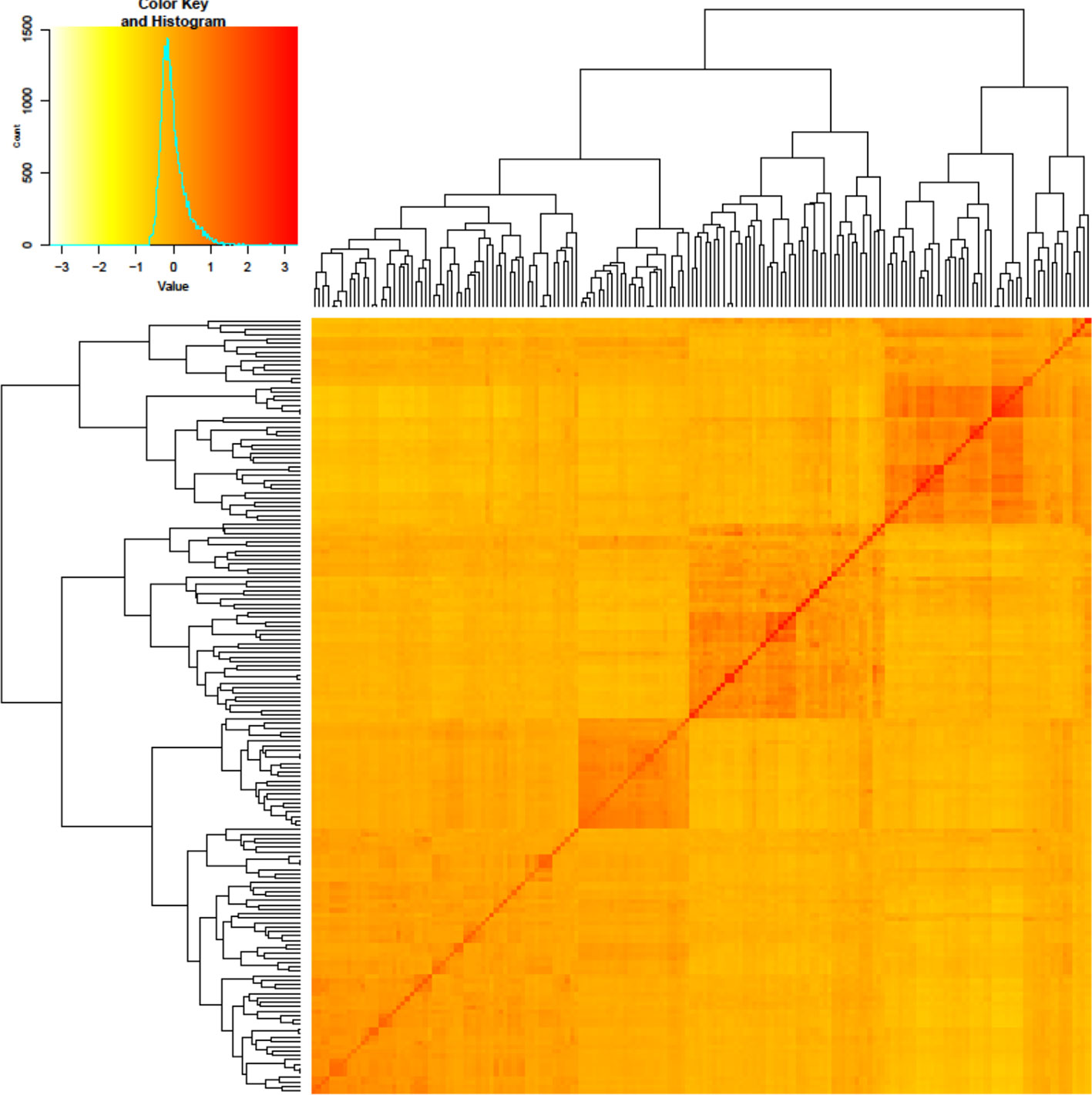
Figure 3 Heatmap and dendrogram of a kinship matrix among 175 winter wheat cultivars/landraces estimated using the SNP data.
Genome-Wide Association Analysis
The GWAS was performed using the GLM model, and both population structure and kinship (K) were taken into account to control pseudo associations (Figure 4). As is shown by the Manhattan plot and quantile-quantile plot (QQ plot) (Figures 4A, D), six significant (FDR < 0.05) SNP markers for rAUDPC of STB were detected on chromosome 1B. The identified QTL was verified using four additional GWAS models, namely, MLM, MLMM, FarmCPU, and Super and the QTL was found to be statistically significant (FDR < 0.05) in MLMM, FarmCPU, and Super results (Supplementary Figure 2). All six markers are located within a 2 cM distance on chromosome 1B (97–99 cM), thus, suggesting that it could potentially be a single QTL (Table 1, Figure 5). Additional QTL were also identified on chromosome 1A, 2B, 3A, and 5A in at least two GWAS models each (Table 1).
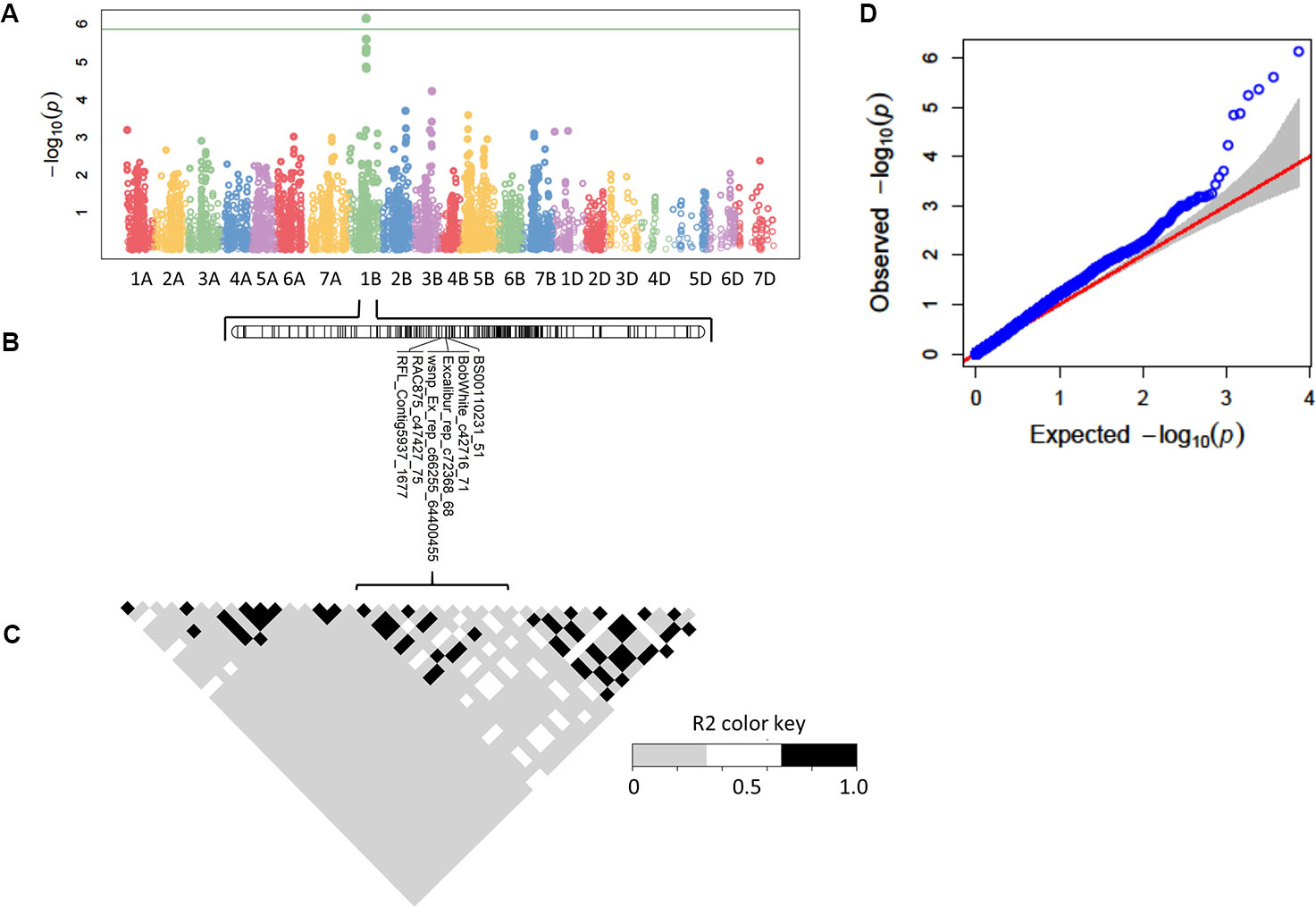
Figure 4 Single nucleotide polymorphism (SNP) significantly associated with STB resistance in winter wheat identified by genome-wide association study (GWAS) with GLM model. (A) Manhattan plot; (B) Linkage map of Chromosome 1B; (C) Linkage disequilibrium plot; (D) Quantile-quantile plot.
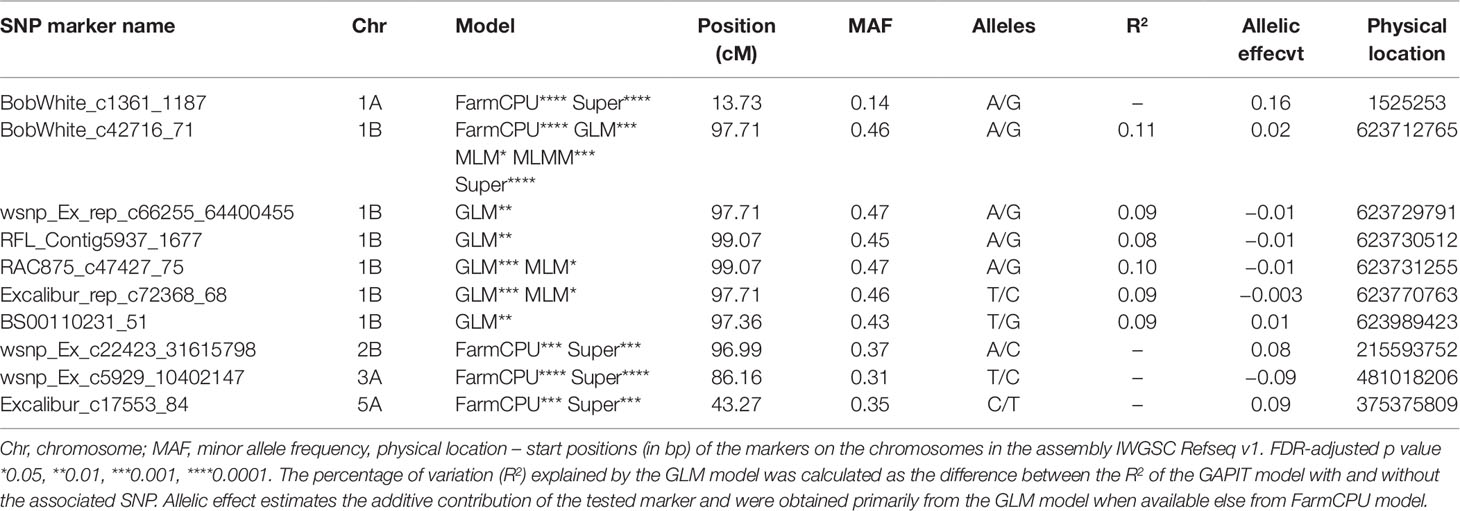
Table 1 Summary of the significant SNPs marker identified with different models which are associated with Septoria tritici blotch (STB) resistance in GWAS analysis with 175 winter wheat genotypes.
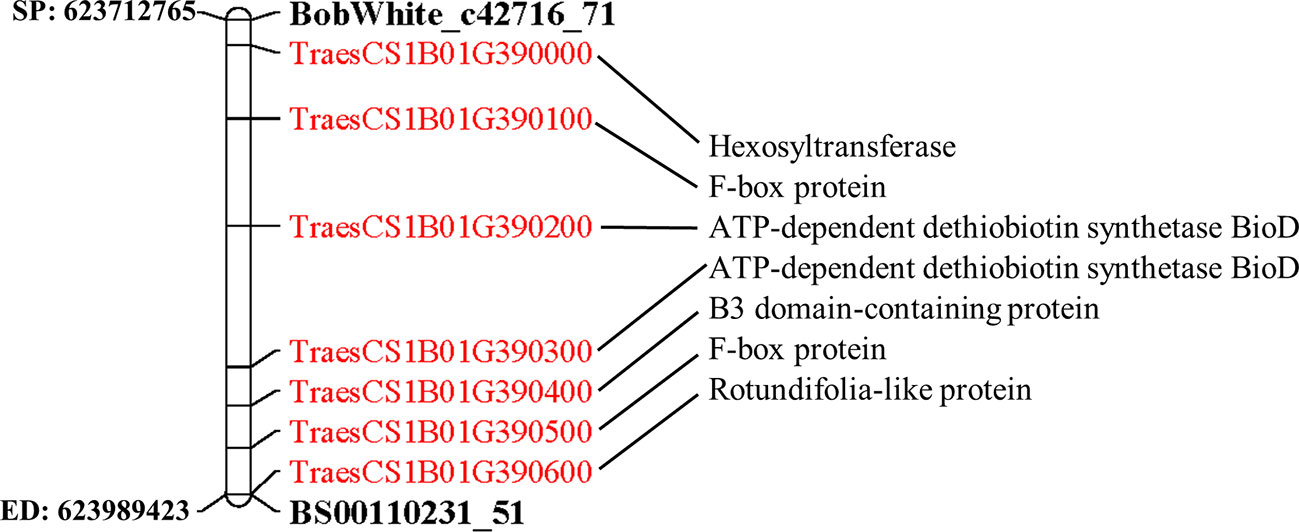
Figure 5 Wheat chromosome 1B representing the physical position (in bp) of the flanking markers and genes localized within these markers. SP, start position (BobWhite_c42716_71); ED end position (BS00110231).
Candidate Genes Located in the QTL on Chromosome 1B
In total, seven candidate genes were identified that were localized within the GWAS identified loci on chromosome 1B (Figure 5). Among these genes, two genes code for F-box protein (TraesCS1B01G390100, TraesCS1B01G390500) and two genes for ATP-dependent dethiobiotin synthetase BioD (TraesCS1B01G390200, TraesCS1B01G390300). The other three genes code for B3 domain-containing protein (TraesCS1B01G390400), Rotundifolia-like protein (TraesCS1B01G390600), and Hexosyltransferase (TraesCS1B01G390000).
Genomic Prediction
GP method was applied based on all SNPs, and the prediction of the genomic breeding value for each line was evaluated using 500 randomly generated train/test sets. The average correlation between observed tolerance to STB and predicted STB by GP was 0.47 in a model with no significant markers included as fixed effects. The GWAS results were used to select markers to fit as fixed effects. Significant markers were pooled from the GLM, MLM, MLMM, FarmCPU, and Super models. The six significant SNP markers identified in proximity to each other on chromosome 1B were reduced to the marker BobWhite_c42716_71 on the basis of the lowest FDR-adjusted p-value. In total, five significant markers were used as candidates for modeling with fixed effects (Table 1).
All possible combinations of the five GWAS-selected SNP markers were evaluated, in subset sizes from one marker to all five used as fixed effects (Table 2). The highest average prediction accuracy (0.62) was obtained from a model that included all five markers as fixed effects. Among the models with reduced number of markers (1–3 markers) set as fixed effects, the models using three GWAS-selected markers performed better compared to the models using one or two markers. The prediction accuracy thus increased on average from 0.48 for one marker added as fixed effect to 0.54 for three markers. Out of the three marker models, the best performing model was a model that included the following three markers BobWhite_c1361_1187, BobWhite_c42716_71, and Excalibur_c17553_84 with a prediction accuracy of 0.59 (Supplementary Table 2). In comparison, the model that did not use GWAS-selected markers as fixed effects, and the models that used randomly selected markers (regardless of GWAS significance), performed on average worse than both the GWAS-assisted models and the model with all markers set as random effects (Table 2).
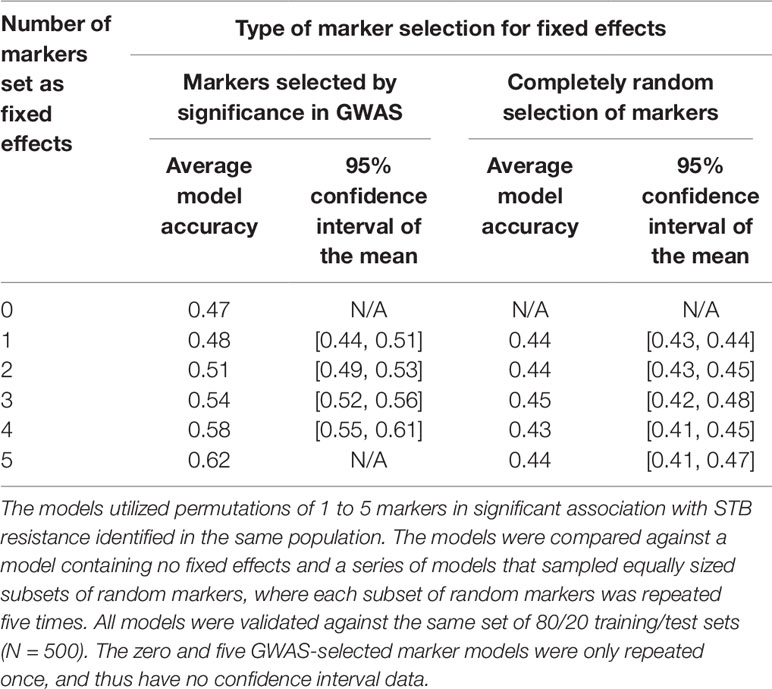
Table 2 Summary of rrBLUP-based GWAS-assisted genomic prediction models of STB resistance scored in 175 winter wheat genotypes.
Haplotype Analysis
Haplotype analysis was performed to identify haplotype variants for the QTL identified on chromosome 1B with six significant markers. Haplotype variants were detected with the software DNAsp (Rozas et al., 2017). In total, 19 haplotype variants were detected with number of genotypes ranging from 1 to 71 in each variant. Of these, 3 haplotype variants were selected with at least five or more genotypic counts/genotypes (Supplementary Table 1). Thereafter, haplotype network was constructed with the TCS algorithm in the software PopART (Leigh et al., 2015) (Figure 6). The analysis revealed that Hap_2 had the lowest mean disease score of 0.77 compared to Hap_1 (0.96) and Hap_3 (0.95). Hap_2 had 11 genotypes of which 8 originated from Denmark, 2 from Sweden, and 1 from Germany. Most of the genotypes from Denmark had high resistance while one of the two genotypes from Sweden had high resistance.
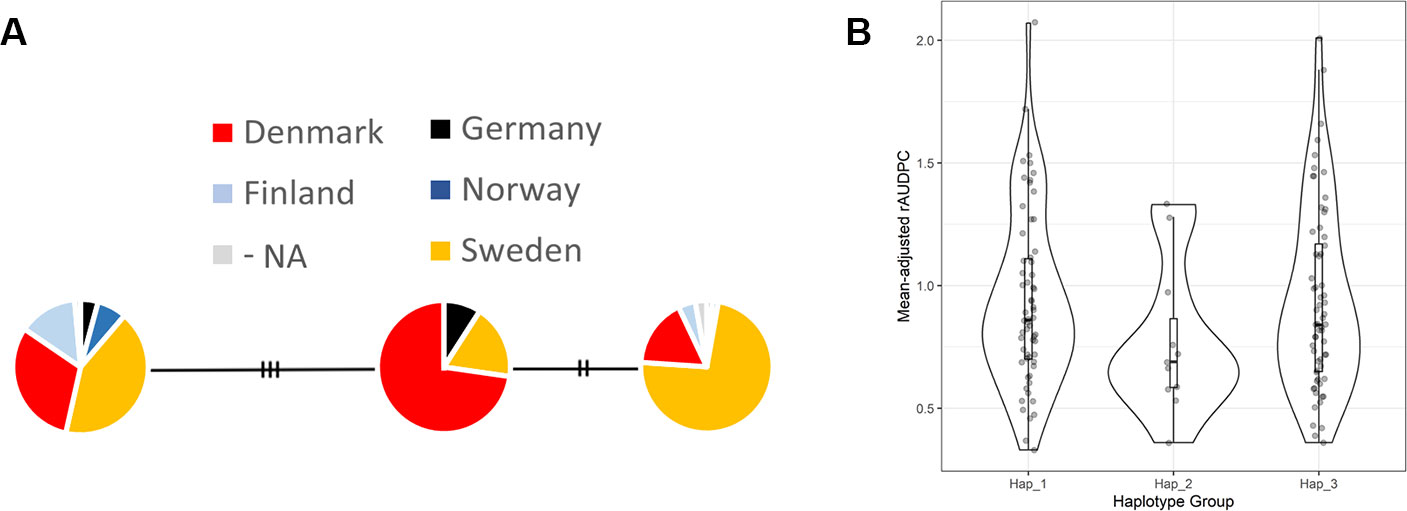
Figure 6 Haplotype variants identified from the QTL on chromosome 1B. (A) Haplotype network with nodes denoted as pie charts and (B) range of distribution of STB resistance of genotypes in each variant.
Discussion
STB is one of the most important winter wheat diseases in Northern Europe, and cultivars with higher levels of resistance which is stable and effective across environments are needed. Whereas individual Stb genes are not currently effective against Z. tritici populations in Europe (Arraiano et al., 2009), the identification of new QTL for STB resistance and incorporation of resistance into elite winter wheat cultivars is crucial. To this end, the current study analyzed 175 winter wheat genotypes of Nordic origin for STB resistance under controlled conditions at the seedling stage. Our results revealed that the NordGen genebank has a highly valuable and genetically diverse collection of germplasm comprising resistance to STB. This germplasm mainly originates from Sweden, Denmark, Finland, and Norway composing 56.2%, 25.5%, 9.6%, and 3.4% of all analyzed germplasm, respectively, and released approximately between 1900 and 2000. Population structure analysis revealed three clusters associated with geographical origin. Finish and Norwegian genotypes formed one cluster, the second cluster contains mainly Swedish genotypes while genotypes from Denmark and Germany segregated into the third cluster (Figure 2A). In addition, the result from the PCA data showed that the modern wheat cultivars exhibited a higher level of resistance in comparison to older released cultivars (Figures 2B C). This result indicated that the breeding progress for STB resistance over the last decades probably occurred by the gradual accumulation of genes with a minor effect, as is the case also in the American germplasm (Jlibene et al., 1994; Camacho-Casas et al., 1995). Similarly, the characterization of old Tunisian durum wheat accessions for resistance to STB identified resistant germplasm and four new resistant genes (Ferjaoui et al., 2015). The authors, therefore, suggested that the old Tunisian durum wheat accessions harbor novel resistance genes that can be introgressed into the modern cultivars. The results from our work highlight the potential of old germplasm as novel sources of resistance to STB for winter wheat breeding programs in Northern Europe.
A QTL associated with STB resistance identified by GWAS in this study was mapped on chromosome 1B. Previous studies have mapped Stb11 on the short arm of chromosome 1B in TE9111 (Chartrain et al., 2005) and remapped Stb2 was also located close to or at Stb11 locus in Vernopolis (Liu et al., 2013). StbWW identified in three DH populations, was also mapped on chromosome 1BS at or near Stb11. Raman et al. (2009) identified eight SNPs associated with STB resistance and one was mapped on chromosome 1B in European winter wheat collection. Goudemand et al. (2013) mapped two QTL on 1B (one 1BS and one 1BL) chromosome in bi-parental crosses. Recently, Vagndorf et al. (2017) identified QTL QStb.NS-1B located on the long arm of chromosome 1B by GWAS of Danish cultivars and breeding lines that were characterized over three years in three locations in Denmark for STB. In this study, one QTL was mapped on the long arm of chromosome 1B which is in close physical proximity to the QTL QStb.NS-1B. Thus, it can be postulated that it is the same QTL as identified previously. However, our study identified this QTL for quantitative resistance at the seedling stage under controlled conditions while the study by Vagndorf et al. (2017) identified the same QTL in field trials for adult plant resistance.
The other QTL associated with STB resistance identified in this study were located on chromosomes 1A, 2B, 3A, and 5A. QTL 1A, 2B, and 5A were mapped on the short arm of the respective chromosomes and QTL on 3A was mapped on the long arm. Goudemand et al. (2013) mapped two Meta-QTL (MQTL1 and MQTL6) on chromosomes 1A and 2B and another QTL (QTL8) on chromosome 5A for STB resistance which were in close physical proximity to the QTL mapped (1A, 2B, and 5A) in this study. The MQTL1 was associated with STB resistance both in adult and seedling stages whereas QTL8 was only associated with adult and MQTL6 was only associated with seedling stage resistance.
The QTL on chromosome 3A in our study was found in close physical proximity to the previously reported QTL (QStb.risø-3A.2) which was associated with STB resistance both in adult and seedling stages (Brown et al., 2015). Thus, our study further confirms the role of the identified QTL at the seedling stage. Introgression of these QTL in winter wheat cultivars will provide both seedling and adult plant stage resistance to STB.
In the present work, we identified seven candidate genes with putative roles in resistance to STB in wheat (Figure 5). Two of the identified genes (TraesCS1B01G390100 and TraesCS1B01G390500) were associated with F-box proteins which plays a key role in plant immune responses through the involvement in hormone pathways (Yu et al., 2007). Two F-box proteins, COI1 (Xie et al., 1998) and SON1 (Kim and Delaney, 2002), have been demonstrated to have a role in plant defense in Arabidopsis plants. In our previous work, we identified candidate genes associated with STB resistance by integrating QTL mapping and transcriptome profiling, wherein, the F- box proteins were among the most represented in all identified QTL regions (Odilbekov et al., 2019). The other two genes identified in this work were related to ATP-dependent dethiobiotin synthetase BioD (TraesCS1B01G390200 and TraesCS1B01G390300). ATP-dependent dethiobiotin synthetase BioD is involved in the first step of the sub-pathway that synthesizes biotin from 7,8-diaminononanoate. Li et al. (2012) demonstrated that biotin deficiency results in light-dependent spontaneous cell death and modulates defense gene expression in Arabidopsis plants. The other putative genes identified in the present work were B3 domain-containing protein (TraesCS1B01G390400). The B3 domain has been found in several transcription factors specific to higher plant species (Waltner et al., 2005). Wang et al. (2015) found that the B3 domain of BPH29 gene was associated with insect brown planthopper resistance in rice. Also, they have shown that during the infestation, the RBPH54 triggers the salicylic acid signaling pathway and suppresses the jasmonic acid pathway, which is similar to biotrophic pathogens.
In the previous studies, prediction accuracy of GS models was found to be improved for example by increasing the training population size, testing the models on test populations genetically closely related to the training population, implementing a different GS algorithm, increasing the marker density or combining significantly associated markers as fixed effects (Solberg et al., 2008; Norman et al., 2018). In this work, we evaluated the prediction accuracy of GP models when GWAS markers were included as fixed effects. When GWAS markers obtained from different GWAS models were included as fixed effects, the accuracy of GP was significantly improved (Table 2). The results also suggest that including two or more GWAS markers as fixed effects significantly increases the accuracy of the GP models. Our results corroborate the trends in accuracy improvements seen in the previous studies integrating GWAS and GP in winter wheat (Herter et al., 2019) maize (Bian and Holland, 2017), and rice (Spindel et al., 2016).
Finally, this and the previous studies (Daetwyler et al., 2014; Crossa et al., 2016) have shown that GP can be used to obtain GEBVs for economically important traits in landraces by training models on a subset of landraces that are phenotyped. There are several hundred thousand landraces stored in genebanks worldwide, and thus, advanced methods, such as GP will enable high-throughput evaluation of landraces to identify those with superior resistance traits. The identified landraces can then be included in the wheat breeding programs to perform GP-based progeny selection.
Conclusions
This study leads to the conclusion that the wheat genotypes stored at NordGen are a genetically diverse resource. The highly resistant genotypes serve as potential donors for improving commercial cultivars in the Nordic and Baltic Sea Region countries. The significant SNP markers can be used for marker-assisted selection of STB resistance at the seedling stage in wheat breeding. The genes identified by GWAS approach can serve as candidate genes for improving STB resistance in wheat through functional studies. In addition, the results indicate that integrating GWAS with GP could facilitate further improvement of GP accuracy thereby improving the selection efficiency of the breeding program.
Data Availability Statement
The genotypic data can be assessed from the following link https://doi.org/10.6084/m9.figshare.10184468.
Author Contributions
AC conceived and planned the study and performed GWAS, haplotype and GP analysis. FO and RA performed germplasm characterization. AK performed GWAS-assisted GP analysis. JS selected genotypes and performed genotyping. FO performed statistical analysis and wrote the first draft. All authors contributed in the interpretation of the data and in writing the manuscript.
Funding
This project was funded by Lantmännen Research Foundation (2016F010), Einar Nilssons Stiftelse, and SLU Grogrund.
Conflict of Interest
The authors declare that the research was conducted in the absence of any commercial or financial relationships that could be construed as a potential conflict of interest.
Acknowledgments
We would like to thank Ganapathi Varma Saripella for the bioinformatics support.
Supplementary Material
The Supplementary Material for this article can be found online at: https://www.frontiersin.org/articles/10.3389/fgene.2019.01224/full#supplementary-material
References
Arraiano, L., Brown, J. (2006). Identification of isolate-specific and partial resistance to septoria tritici blotch in 238 European wheat cultivars and breeding lines. Plant Pathol. 55 (6), 726–738. doi: 10.1111/j.1365-3059.2006.01444.x
Arraiano, L. S., Brown, J. K. M. (2017). Sources of resistance and susceptibility to Septoria tritici blotch of wheat. Mol. Plant Pathol. 18 (2), 276–292. doi: 10.1111/mpp.12482
Arraiano, L. S., Balaam, N., Fenwick, P. M., Chapman, C., Feuerhelm, D., Howell, P., et al. (2009). Contributions of disease resistance and escape to the control of septoria tritici blotch of wheat. Plant Pathol. 58 (5), 910–922. doi: 10.1111/j.1365-3059.2009.02118.x
Bian, Y., Holland, J. B. (2017). Enhancing genomic prediction with genome-wide association studies in multiparental maize populations. Heredity 118 (6), 585–593. doi: 10.1038/hdy.2017.4
Brown, J. K., Chartrain, L., Lasserre-Zuber, P., Saintenac, C. (2015). Genetics of resistance to Zymoseptoria tritici and applications to wheat breeding. Fungal Genet. Biol. 79, 33–41. doi: 10.1016/j.fgb.2015.04.017
Camacho-Casas, M., Kronstad, W., Scharen, A. (1995). Septoria tritici resistance and associations with agronomic traits in a wheat cross. Crop Sci. 35 (4), 971–976. doi: 10.2135/cropsci1995.0011183X003500040006x
Chartrain, L., Brading, P., Brown, J. (2005). Presence of the Stb6 gene for resistance to Septoria tritici blotch (Mycosphaerella graminicola) in cultivars used in wheat-breeding programmes worldwide. Plant Pathol. 54 (2), 134–143. doi: 10.1111/j.1365-3059.2005.01164.x
Chawade, A., Armoniene, R., Berg, G., Brazauskas, G., Frostgard, G., Geleta, M., et al. (2018). A transnational and holistic breeding approach is needed for sustainable wheat production in the Baltic Sea region. Physiol. Plant. 164 (4), 442–451. doi: 10.1111/ppl.12726
Cheval, P., Siah, A., Bomble, M., Popper, A. D., Reignault, P., Halama, P. (2017). Evolution of QoI resistance of the wheat pathogen Zymoseptoria tritici in Northern France. Crop Prot. 92, 131–133. doi: 10.1016/j.cropro.2016.10.017
Crossa, J., Jarquín, D., Franco, J., Pérez-Rodríguez, P., Burgueño, J., Saint-Pierre, C., et al. (2016). Genomic Prediction of Gene Bank Wheat Landraces. G3. Genes|Genomes|Genetics 6 (7), 1819–1834. doi: 10.1534/g3.116.029637
Daetwyler, H. D., Bansal, U. K., Bariana, H. S., Hayden, M. J., Hayes, B. J. (2014). Genomic prediction for rust resistance in diverse wheat landraces. Theor. Appl. Genet. 127 (8), 1795–1803. doi: 10.1007/s00122-014-2341-8
de Carvalho, M. A. A. P., Bebeli, P. J., Bettencourt, E., Costa, G., Dias, S., Dos Santos, T. M. M., et al. (2012). Cereal landraces genetic resources in worldwide GeneBanks. A review. Agron. Sustain. Dev. 33 (1), 177–203. doi: 10.1007/s13593-012-0090-0
Desta, Z. A., Ortiz, R. (2014). Genomic selection: genome-wide prediction in plant improvement. Trends In Plant Sci. 19 (9), 592–601. doi: 10.1016/j.tplants.2014.05.006
Diederichsen, A., Solberg, S.Ø., Jeppson, S. (2012). Morphological changes in Nordic spring wheat (Triticum aestivum L.) landraces and cultivars released from 1892 to 1994. Genet. Resour. Crop Evol. 60 (2), 569–585. doi: 10.1007/s10722-012-9858-y
Dreisigacker, S., Zhang, P., Warburton, M. L., Skovmand, B., Hoisington, D., Melchinger, A. E. (2005). Genetic Diversity among and within CIMMYT Wheat Landrace Accessions Investigated with SSRs and Implications for Plant Genetic Resources Management. Crop Sci. 45 (2), 653–661. doi: 10.2135/cropsci2005.0653
Endelman, J. B. (2011). Ridge Regression and Other Kernels for Genomic Selection with R Package rrBLUP. Plant Genome 4 (3), 250–255. doi: 10.3835/plantgenome2011.08.0024
Ferjaoui, S., M’Barek, S. B., Bahri, B., Slimane, R. B., Hamza, S. (2015). Identification of resistance sources to Septoria Tritici blotch in old Tunisian durum wheat germplasm applied for the analysis of the Zymoseptoria triticidurum wheat interaction. J. Plant Pathol. 97 (3), 1–11. doi: 10.4454/JPP.V97I3.028
Fones, H., Gurr, S. (2015). The impact of Septoria tritici Blotch disease on wheat: An EU perspective. Fungal Genet. Biol. 79, 3–7. doi: 10.1016/j.fgb.2015.04.004
Goudemand, E., Laurent, V., Duchalais, L., Ghaffary, S. M. T., Kema, G. H., Lonnet, P., et al. (2013). Association mapping and meta-analysis: two complementary approaches for the detection of reliable Septoria tritici blotch quantitative resistance in bread wheat (Triticum aestivum L.). Mol. Breed. 32 (3), 563–584. doi: 10.1007/s11032-013-9890-4
Hagenblad, J., Asplund, L., Balfourier, F., Ravel, C., Leino, M. W. (2012). Strong presence of the high grain protein content allele of NAM-B1 in Fennoscandian wheat. Theor. Appl. Genet. 125 (8), 1677–1686. doi: 10.1007/s00122-012-1943-2
Herter, C. P., Ebmeyer, E., Kollers, S., Korzun, V., Würschum, T., Miedaner, T. (2019). Accuracy of within- and among-family genomic prediction for Fusarium head blight and Septoria tritici blotch in winter wheat. Theor. Appl. Genet. 132 (4), 1121–1135. doi: 10.1007/s00122-018-3264-6
Hysing, S. C., Merker, A., Liljeroth, E., Koebner, R. M., Zeller, F. J., Hsam, S. L. (2007). Powdery mildew resistance in 155 Nordic bread wheat cultivars and landraces. Hereditas 144 (3), 102–119. doi: 10.1111/j.2007.0018-0661.01991.x
Jlibene, M., Gustafson, J., Rajaram, S. (1994). Inheritance of resistance to Mycosphaerella graminicola in hexaploid wheat. Plant Breed. 112 (4), 301–310. doi: 10.1111/j.1439-0523.1994.tb00688.x
Juliana, P., Singh, R. P., Singh, P. K., Crossa, J., Rutkoski, J. E., Poland, J. A., et al. (2017). Comparison of Models and Whole-Genome Profiling Approaches for Genomic-Enabled Prediction of Septoria Tritici Blotch, Stagonospora Nodorum Blotch, and Tan Spot Resistance in Wheat. Plant Genome 10 (2). doi: 10.3835/plantgenome2016.08.0082
Kim, H. S., Delaney, T. P. (2002). Arabidopsis SON1 is an F-box protein that regulates a novel induced defense response independent of both salicylic acid and systemic acquired resistance. Plant Cell 14 (7), 1469–1482. doi: 10.1105/tpc.001867
Kollers, S., Rodemann, B., Ling, J., Korzun, V., Ebmeyer, E., Argillier, O., et al. (2013). Genetic architecture of resistance to Septoria tritici blotch (Mycosphaerella graminicola) in European winter wheat. Mol. Breed. 32 (2), 411–423. doi: 10.1007/s11032-013-9880-6
Leigh, J. W., Bryant, D., Nakagawa, S. (2015). popart: full-feature software for haplotype network construction. Methods In Ecol. Evol. 6 (9), 1110–1116. doi: 10.1111/2041-210x.12410
Li, J., Brader, G., Helenius, E., Kariola, T., Palva, E. T. (2012). Biotin deficiency causes spontaneous cell death and activation of defense signaling. Plant J. 70 (2), 315–326. doi: 10.1111/j.1365-313X.2011.04871.x
Lipka, A. E., Tian, F., Wang, Q., Peiffer, J., Li, M., Bradbury, P. J., et al. (2012). GAPIT: genome association and prediction integrated tool. Bioinformatics 28 (18), 2397–2399. doi: 10.1093/bioinformatics/bts444
Liu, Y., Zhang, L., Thompson, I. A., Goodwin, S. B., Ohm, H. W. (2013). Molecular mapping re-locates the Stb2 gene for resistance to Septoria tritici blotch derived from cultivar Veranopolis on wheat chromosome 1BS. Euphytica 190 (1), 145–156. doi: 10.1007/s10681-012-0796-8
Lopes, M. S., El-Basyoni, I., Baenziger, P. S., Singh, S., Royo, C., Ozbek, K., et al. (2015). Exploiting genetic diversity from landraces in wheat breeding for adaptation to climate change. J. Exp. Bot. 66 (12), 3477–3486. doi: 10.1093/jxb/erv122
McDonald, B. A., Mundt, C. C. (2016). How Knowledge of Pathogen Population Biology Informs Management of Septoria Tritici Blotch. Phytopathology 106 (9), 948–955. doi: 10.1094/phyto-03-16-0131-rvw
Mendiburu, F. D. (2017). agricolae: Statistical Procedures for Agricultural Research. R package version 1.2-8. Available: https://CRAN.R-project.org/package=agricolae.
Meuwissen, T. H., Hayes, B. J., Goddard, M. E. (2001). Prediction of total genetic value using genome-wide dense marker maps. Genetics 157 (4), 1819–1829.
Miedaner, T., Zhao, Y., Gowda, M., Longin, C. F. H., Korzun, V., Ebmeyer, E., et al. (2013). Genetic architecture of resistance to Septoria tritici blotch in European wheat. BMC Genomics 14 (1), 858. doi: 10.1186/1471-2164-14-858
Muqaddasi, Q. H., Zhao, Y., Rodemann, B., Plieske, J., Ganal, M. W., Röder, M. S. (2019). Genome-wide Association Mapping and Prediction of Adult Stage Blotch Infection in European Winter Wheat via High-Density Marker Arrays. Plant Genome 12 (1). doi: 10.3835/plantgenome2018.05.0029
Norman, A., Taylor, J., Edwards, J., Kuchel, H. (2018). Optimising Genomic Selection in Wheat: Effect of Marker Density, Population Size and Population Structure on Prediction Accuracy. G3:. Genes|Genomes|Genetics 8 (9), 2889–2899. doi: 10.1534/g3.118.200311
Odilbekov, F., Armoniené, R., Henriksson, T., Chawade, A. C. (2018). Proximal phenotyping and machine learning methods to identify Septoria tritici blotch disease symptoms in wheat. Front. In Plant Sci. 9, 685. doi: 10.3389/fpls.2018.00685
Odilbekov, F., He, X., Armoniené, R., Saripella, G. V., Henriksson, T., Singh, P. K., et al. (2019). QTL Mapping and Transcriptome Analysis to Identify Differentially Expressed Genes Induced by Septoria Tritici Blotch Disease of Wheat. Agronomy 9 (9), 510. doi: 10.3390/agronomy9090510
Raman, R., Milgate, A. W., Imtiaz, M., Tan, M. K., Raman, H., Lisle, C., et al. (2009). Molecular mapping and physical location of major gene conferring seedling resistance to Septoria tritici blotch in wheat. Mol. Breed. 24 (2), 153–164. doi: 10.1007/s11032-009-9280-0
Randhawa, M., Bansal, U., Lillemo, M., Miah, H., Bariana, H. (2016). Postulation of rust resistance genes in Nordic spring wheat genotypes and identification of widely effective sources of resistance against the Australian rust flora. J. Appl. Genet. 57 (4), 453–465. doi: 10.1007/s13353-016-0345-6
Rozas, J., Ferrer-Mata, A., Sánchez-DelBarrio, J. C., Guirao-Rico, S., Librado, P., Ramos-Onsins, S. E., et al. (2017). DnaSP 6: DNA Sequence Polymorphism Analysis of Large Data Sets. Mol. Biol. Evol. 34 (12), 3299–3302. doi: 10.1093/molbev/msx248
Saintenac, C., Lee, W. S., Cambon, F., Rudd, J. J., King, R. C., Marande, W., et al. (2018). Wheat receptor-kinase-like protein Stb6 controls gene-for-gene resistance to fungal pathogen Zymoseptoria tritici. Nat. Genet. 50 (3), 368–374. doi: 10.1038/s41588-018-0051-x
Solberg, T. R., Sonesson, A. K., Woolliams, J. A., Meuwissen, T. H. E. (2008). Genomic selection using different marker types and densities. J. Anim. Sci. 86 (10), 2447–2454. doi: 10.2527/jas.2007-0010
Spindel, J. E., Begum, H., Akdemir, D., Collard, B., Redoña, E., Jannink, J. L., et al. (2016). Genome-wide prediction models that incorporate de novo GWAS are a powerful new tool for tropical rice improvement. Heredity 116 (4), 395–408. doi: 10.1038/hdy.2015.113
Tabib Ghaffary, S. M., Faris, J. D., Friesen, T. L., Visser, R. G. F., van der Lee, T. A. J., Robert, O., et al. (2011). New broad-spectrum resistance to septoria tritici blotch derived from synthetic hexaploid wheat. Theor. Appl. Genet. 124 (1), 125–142. doi: 10.1007/s00122-011-1692-7
Tang, Y., Liu, X., Wang, J., Li, M., Wang, Q., Tian, F., et al. (2016). GAPIT Version 2: an enhanced integrated tool for genomic association and prediction. Plant Genome 9 (2). doi: 10.3835/plantgenome2015.11.0120
Torriani, S. F. F., Brunner, P. C., McDonald, B. A., Sierotzki, H. (2009). QoI resistance emerged independently at least 4 times in European populations of Mycosphaerella graminicola. Pest Manage. Sci. 65 (2), 155–162. doi: 10.1002/ps.1662
Torriani, S. F., Melichar, J. P., Mills, C., Pain, N., Sierotzki, H., Courbot, M. (2015). Zymoseptoria tritici: A major threat to wheat production, integrated approaches to control. Fungal Genet. Biol. 79, 8–12. doi: 10.1016/j.fgb.2015.04.010
Vagndorf, N., Nielsen, N. H., Edriss, V., Andersen, J. R., Orabi, J., Jørgensen, L. N., et al. (2017). Genomewide association study reveals novel quantitative trait loci associated with resistance towards Septoria tritici blotch in North European winter wheat. Plant Breed. 136 (4), 474–482. doi: 10.1111/pbr.12490
Waltner, J. K., Peterson, F. C., Lytle, B. L., Volkman, B. F. (2005). Structure of the B3 domain from Arabidopsis thaliana protein At1g16640. Protein Sci. 14 (9), 2478–2483. doi: 10.1110/ps.051606305
Wang, Y., Cao, L., Zhang, Y., Cao, C., Liu, F., Huang, F., et al. (2015). Map-based cloning and characterization of BPH29, a B3 domain-containing recessive gene conferring brown planthopper resistance in rice. J. Exp. Bot. 66 (19), 6035–6045. doi: 10.1093/jxb/erv318
Wieczorek, T. M., Berg, G., Semaškienė, R., Mehl, A., Sierotzki, H., Stammler, G., et al. (2015). Impact of DMI and SDHI fungicides on disease control and CYP51 mutations in populations of Zymoseptoria tritici from Northern Europe. Eur. J. Plant Pathol. 143 (4), 861–871. doi: 10.1007/s10658-015-0737-1
Xie, D.-X., Feys, B. F., James, S., Nieto-Rostro, M., Turner, J. G. (1998). COI1: an Arabidopsis gene required for jasmonate-regulated defense and fertility. Science 280 (5366), 1091–1094. doi: 10.1126/science.280.5366.1091
Yu, H., Wu, J., Xu, N., Peng, M. (2007). Roles of F-box proteins in plant hormone responses. Acta Biochim. Biophys. Sin. 39 (12), 915–922. doi: 10.1111/j.1745-7270.2007.00358.x
Keywords: GWAS - genome-wide association study, genomic prediction (GP), genomic selection (GS), wheat, Septoria tritici blotch (STB), Quantitative trait loci (QTL)
Citation: Odilbekov F, Armoniené R, Koc A, Svensson J and Chawade A (2019) GWAS-Assisted Genomic Prediction to Predict Resistance to Septoria Tritici Blotch in Nordic Winter Wheat at Seedling Stage. Front. Genet. 10:1224. doi: 10.3389/fgene.2019.01224
Received: 01 June 2019; Accepted: 05 November 2019;
Published: 26 November 2019.
Edited by:
Alison Bentley, National Institute of Agricultural Botany (NIAB), United KingdomReviewed by:
Thomas Miedaner, University of Hohenheim, GermanyMatthew Rouse, United States Department of Agriculture, United States
Morten Lillemo, Norwegian University of Life Sciences, Norway
Copyright © 2019 Odilbekov, Armoniené, Koc, Svensson and Chawade. This is an open-access article distributed under the terms of the Creative Commons Attribution License (CC BY). The use, distribution or reproduction in other forums is permitted, provided the original author(s) and the copyright owner(s) are credited and that the original publication in this journal is cited, in accordance with accepted academic practice. No use, distribution or reproduction is permitted which does not comply with these terms.
*Correspondence: Aakash Chawade, aakash.chawade@slu.se