- 1School of Horticulture and Plant Protection, Yangzhou University, Yangzhou, China
- 2Joint International Research Laboratory of Agriculture & Agri-Product Safety, Yangzhou University, Yangzhou, China
- 3State Key Laboratory of Vegetable Germplasm Innovation, Tianjin, China
Powdery mildew (PM) is a prevalent disease known to limit cucumber production worldwide. MicroRNAs (miRNAs) are single-stranded molecules that regulate host defense responses through posttranscriptional gene regulation. However, which specific miRNAs are involved and how they regulate cucumber PM resistance remain elusive. A PM-resistant single-segment substitution line, SSSL508-28, was developed previously using marker-assisted backcrossing of the PM-susceptible cucumber inbred D8 line. In this study, we applied small RNA and degradome sequencing to identify PM-responsive miRNAs and their target genes in the D8 and SSSL508-28 lines. The deep sequencing resulted in the identification of 156 known and 147 novel miRNAs. Among them, 32 and six differentially expressed miRNAs (DEMs) were detected in D8 and SSSL508-28, respectively. The positive correlation between DEMs measured by small RNA sequencing and stem-loop quantitative real-time reverse transcription–polymerase chain reaction confirmed the accuracy of the observed miRNA abundances. The 32 DEMs identified in the PM-susceptible D8 were all upregulated, whereas four of the six DEMs identified in the PM-resistant SSSL508-28 were downregulated. Using in silico and degradome sequencing approaches, 517 and 20 target genes were predicted for the D8 and SSSL508-28 DEMs, respectively. Comparison of the DEM expression profiles with the corresponding mRNA expression profiles obtained in a previous study with the same experimental design identified 60 and three target genes in D8 and SSSL508-28, respectively, which exhibited inverse expression patterns with their respective miRNAs. In particular, five DEMs were located in the substituted segment that contained two upregulated DEMs, Csa-miR172c-3p and Csa-miR395a-3p, in D8 and two downregulated DEMs, Csa-miR395d-3p and Csa-miR398b-3p, in SSSL508-28. One gene encoding L-aspartate oxidase, which was targeted by Csa-miR162a, was also located on the same segment and was specifically downregulated in PM-inoculated D8 leaves. Our results will facilitate the future use of miRNAs in breeding cucumber varieties with enhanced resistance to PM.
Introduction
Powdery mildew (PM) fungi are obligate biotrophic parasites that are spread worldwide and cause severe diseases in a large variety of horticultural plants, such as cucurbits, tomato, pea, and okra (Martínez-Cruz et al., 2017; Cobos et al., 2018; Fan et al., 2019). Podosphaera xanthii is the main cause of PM on cucumber under glasshouses and in open fields, which causes serious losses in crop yield and quality (Xu et al., 2016). The disease initiates as thin white spots, first on the surfaces of older leaves and then gradually spreading to younger leaves. Grayish white mycelia are visible on severely affected leaves, and such leaves finally exhibit chlorosis and senescence. The application of fungicide can effectively control the disease at present but is not environmentally friendly and is harmful to consumers’ health because cucumbers are harvested almost daily for fresh market consumption (Fukino et al., 2013; Xu et al., 2016). Genetic strategies to breed PM-resistant cultivars are currently the most promising and economical ways of controlling this disease. Thus, a deeper understanding of the molecular mechanisms that trigger cucumber plants to recognize and eventually prevent or limit PM infection is required.
Genetic mapping, RNA sequencing-based transcriptomic, and iTRAQ-based proteomic studies have revealed candidate genes and pathways associated with the genetic control and molecular responses of host immunity against cucumber PM. They include genes that encode cysteine-rich receptor-like protein kinases (Csa1G064780 and Csa1G064790) and mildew locus O (Csa5M623470), as well as molecular events involving cell wall modifications, salicylic acid metabolism, and signal transduction pathways (Berg et al., 2015; Xu et al., 2016; Xu et al., 2017). However, little is known about the coordination and regulation of host genes that affect these biological processes. Studies of microRNAs (miRNAs) in plant species have found strong evidence that some miRNAs that are responsive to pathogen infections may be critical in regulating the host defenses (Islam et al., 2017). However, more investigations are needed to understand which and how these miRNAs contribute to the dialogue between plant and pathogen attack (Carrera et al., 2009; Sanz-Carbonell et al., 2019). MiRNAs are a group of 18 to 24 long nucleotides (nt) that are endogenous small single-stranded non-coding RNAs that regulate gene expression by repressing protein translation or by targeting mRNAs for degradation (Lin et al., 2016). Genome-wide analysis of miRNAs showed that eight wheat miRNAs from six families (miR394, miR528, miR396, miR171, miR156, and miR160) were differentially expressed upon PM (Blumeria graminis f. sp. tritici Bgt) infection (Wu et al., 2015). More recently, miRNAs related to the response of barley to PM (B. graminis f. sp. hordei) were putatively identified as regulating the transcript levels of transcription factors (TFs), such as auxin response factors, NAC [for NAM (no apical meristem), ATAF, CUC (cup-shaped cotyledon)], and homeodomains, as well as several splicing factors (Hunt et al., 2019).
Because miRNAs have been shown to be involved in PM infection, we hypothesized that miRNAs may also play a role during P. xanthii parasitism of its cucumber host and that they may exhibit different expression patterns between resistant and susceptible lines. To test the hypothesis, we inoculated cucumber seedlings from the D8 and SSSL508-28 lines with P. xanthii. D8 is PM susceptible, and SSSL508-28, which has the genetic background of D8 but contains a single segment from PM-resistant line Jin5-508, is PM resistant. The extracted RNA from cucumber leaves 2 ‘s after inoculation was used for small RNA (sRNA) and degradome sequencing to capture cucumber PM-responsive miRNAs and their corresponding target genes. The results will widen our understanding of PM-responsive miRNA-mediated regulatory mechanisms in Cucurbitaceae plants.
Materials and Methods
Plant Material and Treatment
Cucumber seedlings of D8 and SSSL508-28 were grown in a growth chamber under controlled conditions (65% relative humidity, 28°C/16 h light and 20°C/8 h dark). At 21 days (three-leaf stage), homogeneous plants were selected for inoculation by spraying with a P. xanthii conidia (harvested from naturally infected D8 leaves) spore suspension of 1 × 106 conidia/mL containing 0.01% Tween-20 or sterile distilled water until runoff (Xu et al., 2017). The leaves sprayed with the sterile water served as mock controls. An average relative humidity of 90–100% was maintained after inoculation. The PM-inoculated and control leaves with three biological replicates were harvested 2 days after treatment, quickly frozen in liquid nitrogen, and maintained at −80°C until used for RNA isolation.
Small RNA Dequencing and Data Processing
Total RNA was extracted from 10 pooled cucumber leaves using Trizol reagent (Invitrogen, Carlsbad, CA, United States). RNA quality was assessed using an Agilent Bioanalyzer 2100 (Agilent Technologies, Santa Clara, CA, United States), and samples with RNA integrity ≥8 were used to generate the sRNA libraries. Twelve sRNA libraries were generated following the protocol of the TruSeq sRNA Sample Prep Kits (Illumina, San Diego, CA, United States). Briefly, the RNA fragments that were 18 to 30 nt were enriched by polyacrylamide gel electrophoresis and ligated to 5′ and 3′ adaptors using T4 RNA ligase. The ligated RNAs were reverse transcribed to generate the cDNAs libraries. Single-end sequencing (50 bp) was performed on an Illumina HiSeq 2500 platform (Illumina) by Biomarker Technologies (Beijing, China).
Clean reads were obtained from the raw reads by removing low-quality and adaptor-containing reads using the FASTAX-Toolkit. Clean reads that were 18- to 30-nt long were retained and aligned to the cucumber draft genome assembly (9930 V2.01), Rfam 14.1,2 Silva,3 GtRNAdb,4 and Repbase5 to identify non-coding RNAs using Bowtie (Langmead et al., 2009). The remaining clean reads were mapped to the miRBase database (release 226) to identify known cucumber miRNAs. Up to two mismatches were allowed in the alignments. The remaining unaligned sequences were analyzed using MTide, a probabilistic model-based miRNA prediction software especially designed for plant miRNAs (Zhang et al., 2014), to predict novel miRNAs. The cucumber genome (9930 V2.0) was used as the reference genome. The novel miRNAs that met the following stringent criteria were retained: (1) sequence length 18 to 25 nt; (2) maximal free energy of the miRNA precursor −20 kcal/mol; (3) presence of star sequences and 3′ overhang; and (4) ≤7 nucleotide mismatches between the miRNA and star miRNA. The potential novel miRNA precursors that aligned with tRNA, rRNA, snRNA, or snoRNA sequences were discarded.
The abundance of identified miRNAs in the different samples was normalized to transcripts per kilobase million (TPM). The R-based DESeq2 package (Love et al., 2014) was used to determine differential expression patterns of the identified miRNAs between PM-inoculated and control leaves. An miRNA was considered to be significantly differentially expressed if it exhibited a fold change ≥1.5 or ≤0.67 with p ≤ 0.05.
Degradome Sequencing and Data Processing
Four libraries were constructed for degradome sequencing with the RNA extracted from PM-inoculated D8, PM-inoculated SSSL508-28, and the control D8 and SSSL508-28 leaves. An Oligotex mRNA mini kit (Qiagen, Hilden, Germany) was used to separate poly(A) +RNA from the total RNA. The poly(A) +RNA was bound to the mRNACapture beads. A 5′-adaptor was ligated to the RNAs with 5′ monophosphates, followed by reverse transcription with 3′-random primers and polymerase chain reaction (PCR) amplification. The gel-purified products were sequenced using only the 5′-adaptor ligated sequences. Single-end sequencing (36 bp) of the degradome libraries also was performed on an Illumina HiSeq 2500 platform by Beijing Biomarker Technologies (Beijing, China).
After trimming, the clean reads that matched sequences in GenBank and Rfam 14.1 were removed. The remaining 20- or 21-nt high-quality reads were aligned to the cucumber cDNA sequences (9930 V2.0). Perfectly matched sequences were processed using the CleaveLand pipeline (v4.4) to predict potential cleavage sites (Addo-Quaye et al., 2008).
Identification and Annotation of Target Genes
The psRNATarget server7 was used to predict the miRNA-mediated target genes. We used the cucumber miRNA sequences as query sequences. The cucumber draft genome assembly (cDNA, 9930 V2.0) was used as the reference database. BLASTn hits with fewer than three mismatches were selected as potential targets. Gene ontology (GO) slim classification analysis was performed using the gene classification toolkit in CuGenDB.8
Stem-Loop Quantitative Real-Time Reverse Transcription–PCR Analysis
To validate the differential expressions of miRNA identified by sRNA sequencing (sRNA-seq), quantification of selected miRNAs was performed by stem-loop quantitative real-time reverse transcription (qRT)–PCR analysis. Synthesis of first-strand cDNA and the qRT-PCRs were performed using the miRcute fluorescent quantitative detection (SYBR Green) kit (FP401; Tiangen, Beijing, China). Primers were designed using the Vazyme miRNA designer (V1.01; Nanjing, China). The primer sequences are listed in Table 1. The PCRs were performed on an iQ5 multicolor real-time PCR detection instrument (Bio-Rad, Hercules, CA, United States). The relative expressions of the miRNAs were calculated using the 2–ΔΔCT method with U6 snRNA as the internal control. The PCRs were performed in triplicate. A correlation analysis between the expressions obtained by qRT-PCR and the expression levels obtained using the sRNA-seq data was performed using SAS 9.0 software (SAS Institute, Inc., Cary, NC, United States).
Results
Symptoms of PM Infection on SSSL508-28 and D8 Leaves
Representative symptoms to PM infection on D8 and SSSL508-28 leaves are shown in Figure 1A. The mean disease indexes of D8 and SSSL508-28 were 37.3 and 2.1, respectively (Figure 1B). The phenotypic differences between the PM-resistant and PM-susceptible lines were determined by observing the extent of PM growth on the leaf surface at 2 days post PM inoculation by scanning electron microscopy. Dense PM hyphae were seen on the surface of the D8 leaves, whereas no conidia were detected on the surface of the SSSL508-28 leaves (Figure 1C). This suggested that differences in the resistance mechanisms between SSSL508-28 and D8 may contribute to the observed difference in phenotype.
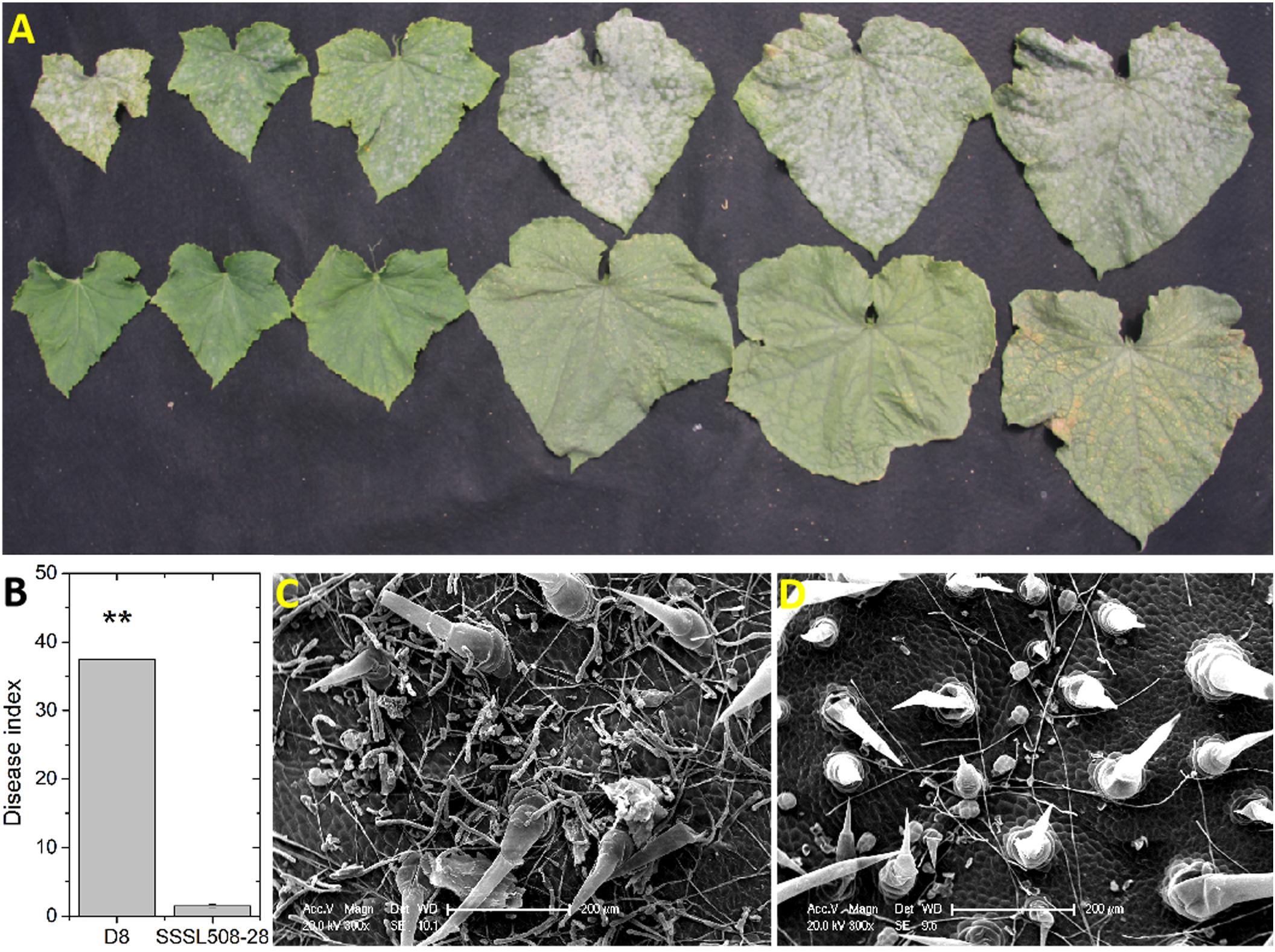
Figure 1. Performance of cucumber SSSL508-28 and D8 lines infected with PM. (A) Typical symptoms on cucumber leaves in different growth stages upon infection with the PM pathogen. Upper: D8; Lower: SSSL508-28. (B) Disease indexes of the two lines upon infection with the PM pathogen. Phenotypes of D8 (C) and SSSL508-28 (D) leaves 48 h after PM inoculation by scanning electron microscopy.
Sequencing the sRNA Libraries of Cucumber Leaves
To detect miRNAs related to PM resistance, 12 sRNA libraries with three biological replicates were constructed for sRNA-seq. They contained the RNAs extracted from non-inoculated D8 control leaves, PM-inoculated D8 leaves, non-inoculated SSSL508-28 control leaves, and PM-inoculated SSSL508-28 leaves. The numbers of raw reads from each library were between 17.9 and 35.1 million (Table 2). After filtering low-quality reads, adaptor sequences, and reads <16 or >30 nt long, more than 13.5 million clean reads remained in each library (Table 2). After the non-coding RNAs (e.g. tRNAs, rRNAs, snRNAs, and snoRNAs) were removed, the remaining >2.49 million reads in each library had at least one match to the cucumber reference genome (Table 2). The length distribution of all the miRNAs that mapped to the reference genome is presented in Figure 2A. The 24-nt miRNAs were the most abundant in all 12 libraries, which is similar to previous findings in plant species such as Arabidopsis, rice, and soybean. Analysis of nucleotide bias in the 18- to 30-nt miRNAs showed that the 19- to 22-nt mature miRNAs preferentially began with uracil (U) (Figure 2B). In addition, analysis of specific nucleotide occurrence showed a dominant bias for U at the first two positions, and the frequency of cytosine (C) was always lowest at positions 2 to 24 in the mature miRNA sequences (Figure 2C).
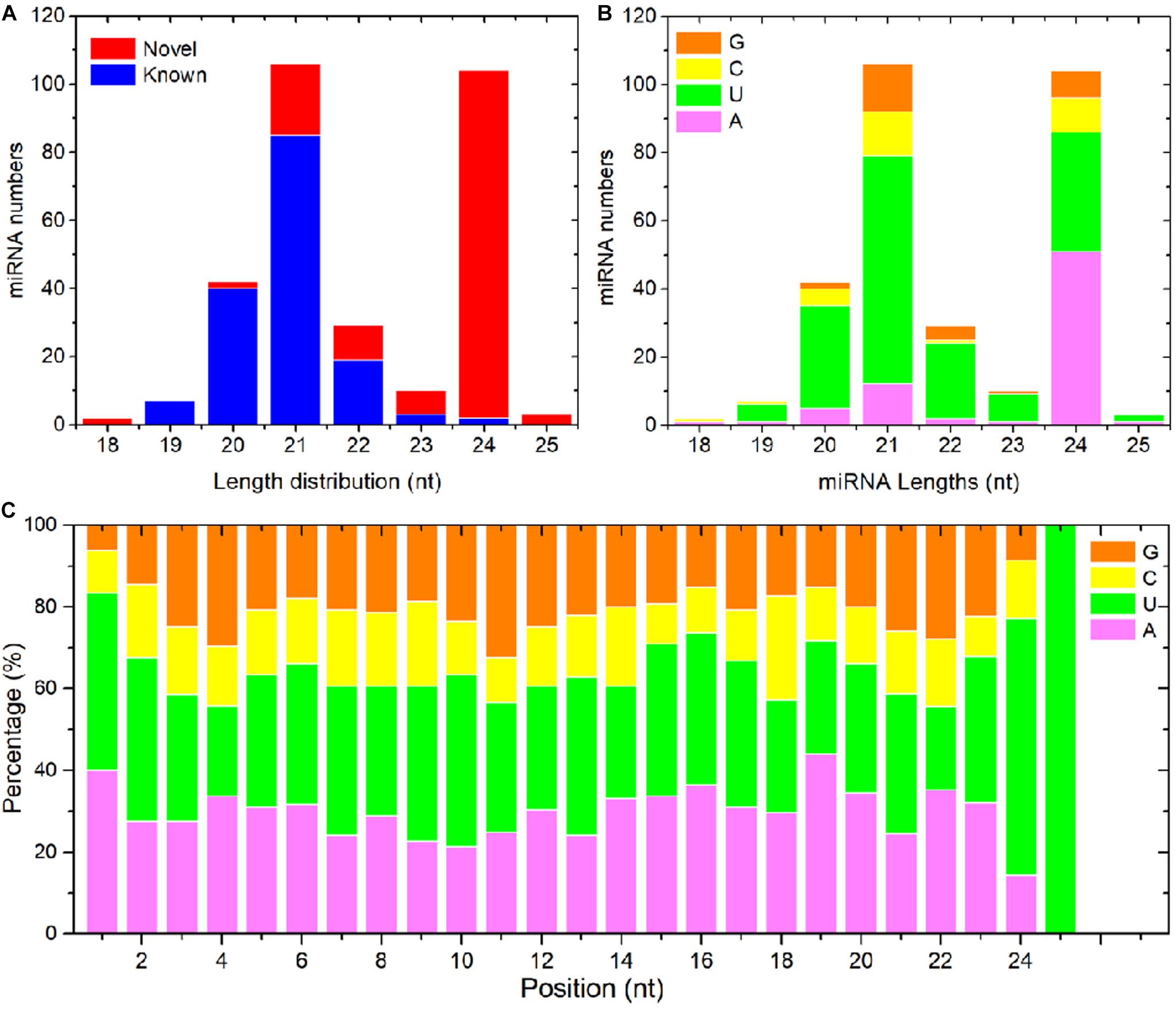
Figure 2. Length distribution and nucleotide bias of the detected cucumber miRNAs. (A) Length distribution of known and novel cucumber miRNAs. (B) Nucleotide composition of the mature miRNAs. (C) Nucleotide bias at each position of the mature miRNA sequences.
Identification of Known Cucumber miRNAs
To identify the known miRNAs, we aligned the 18- to 30-nt sRNA reads to the known plant miRNA sequences in miRBase release 22 using BLASTn. A total of 170 known miRNAs corresponding to 35 miRNA families, including miR156, miR160, miR171, miR396, and miR408, were identified from the 12 libraries (Supplementary Table 1). The miR156 family was the largest with 20 members, followed by miR166 with 16 members and miR171 with 13 members. The precursor miRNA sequences varied in length from 54 to 403 nt. Of the known miRNAs, 152 and 148 were common between the control and PM-inoculated D8 and SSSL508-28 leaves, respectively, suggesting that expression of the known miRNAs was stable in the two cucumber lines. We also found that members of the same miRNA family exhibited variations in expression levels. For example, Csa-miR156j and Csa-miR156h both belong to miR156 family, and TPM values of Csa-miR156j were 3,553 to 7,519, whereas the TPM values of Csa-miR156h were only 0 to 9.8 in the 12 libraries. These differences in expression levels among different members of the same miRNA family suggest their roles in the PM response are different. Further, six known miRNAs, Csa-miR156o-3p, Csa-miR156q-3p, Csa-miR156v, Csa-miR169k, Csa-miR172b-5p, and Csa-miR477a, were detected in only one of the 12 samples.
Identification of Novel Cucumber miRNAs
A total of 133 novel miRNAs were identified from the 12 libraries (Supplementary Table 2), and all had star sequences. The novel miRNAs were named as Csa_novel-number. The lengths of the novel miRNAs varied from 18 to 25 nt, with 24 nt being the predominant length (102/133). The minimum free energies of their pre-miRNA hairpin structures were −192.5 to −20.9 kcal⋅mol–1 with an average of −53.2 kcal⋅mol–1, which corresponds to those of other plant miRNA precursors (Zeng et al., 2018). Csa-novel_12 was the most abundant novel miRNA with a TPM value of >52,000 in all 12 libraries. Forty-nine of the novel miRNAs clustered with known miRNA families (Supplementary Table 2), suggesting they may be recently evolved members of these families.
Differentially Expressed miRNAs in PM-Inoculated Cucumber Leaves
The read counts for the detected miRNA varied from 0 to 224,965, normalized to 0 to 368,491 TPM, among the 12 libraries (Supplementary Tables 1, 2). Using a fold change ≥1.5 and p ≤ 0.05 as the threshold, we identified 32 differentially expressed miRNAs (DEMs), including 27 known and five novel miRNAs, by comparing the PM-inoculated D8 (ID) with the non-inoculated D8 (NID) libraries (Table 3). Only six DEMs, including three known and three novel miRNAs, were identified by comparing the PM-inoculated SSSL508-28 (IS) with the non-inoculated SSSL508-28 (NIS) libraries (Table 4). Interestingly, all of the 32 DEMs identified in the ID versus NID comparison were upregulated, whereas four of the six DEMs identified in the IS versus NIS comparison were downregulated. This result provides an important basis for further studies of the roles of miRNAs in cucumber PM resistance and confirms that miRNAs may have important roles during pathogen infection.
Verification of the Expression of Cucumber PM-Responsive miRNAs
To verify the identified DEMs obtained by sRNA-seq, seven known miRNAs (Csa-miR156g, Csa-miR171g, Csa-miR160b, Csa-miR162a, Csa-miR396d-3p, Csa-miR395d-3p, Csa-miR398d), and one novel miRNA (Csa-novel_71) were selected, and their expression levels were analyzed by stem-loop qRT-PCR. As shown in Figure 3, the stem-loop qRT-PCR showed that Csa-miR156g, Csa-miR171g, Csa-miR160b, Csa-miR162a, and Csa-miR396d-3p were upregulated only in PM-inoculated D8; Csa-miR398d was downregulated only in PM-inoculated SSSL508-28, and Csa-novel_71 was upregulated only in PM-inoculated SSSL508-28 compared with the corresponding non-inoculated controls. The Pearson correlation coefficient revealed a strong positive correlation (r2 = 0.611, p < 0.01) between the sRNA-seq and stem-loop qRT-PCR expression values, which confirmed the reliability of the sRNA-seq data. However, divergences between the two analyses were also observed.
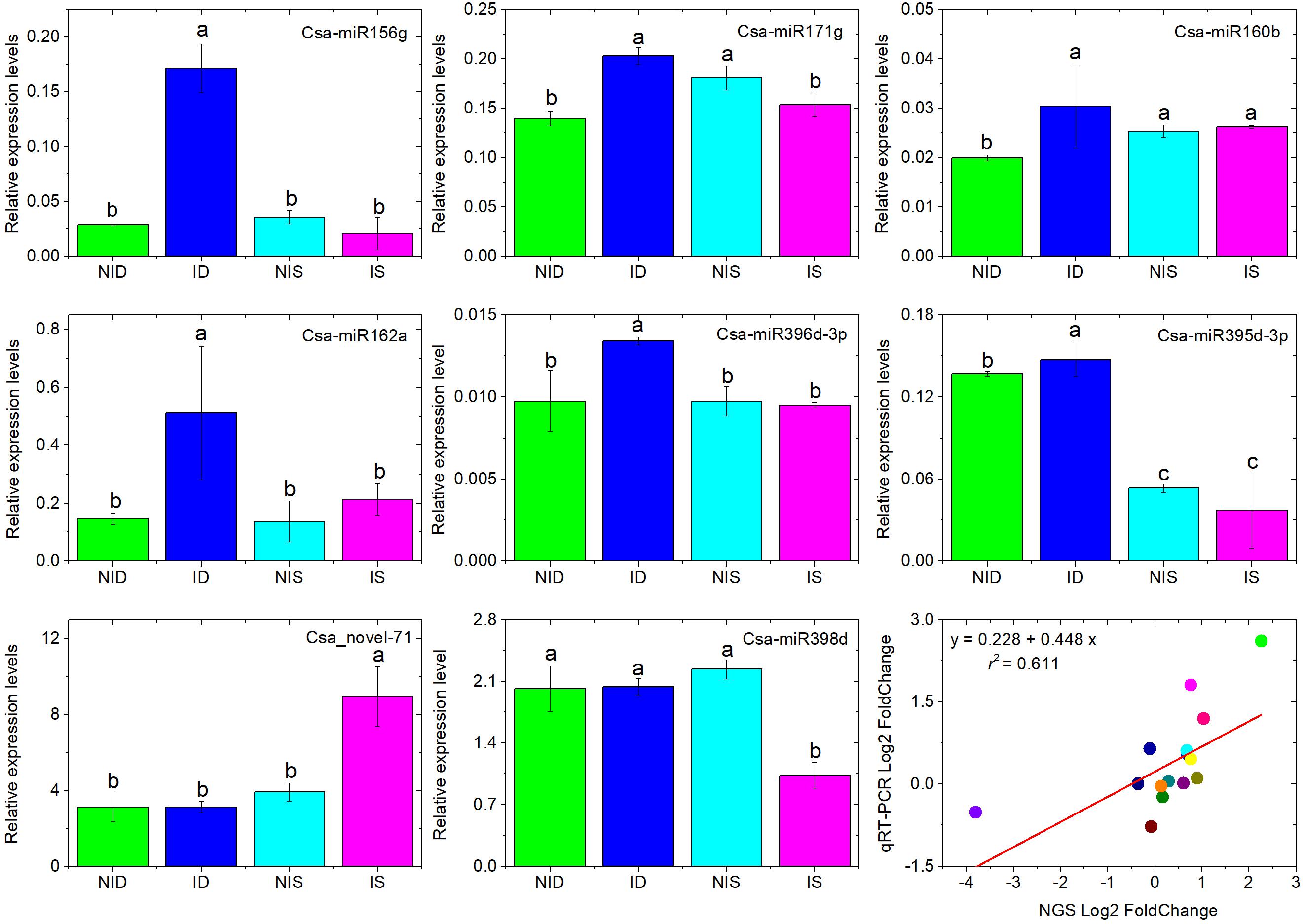
Figure 3. Analysis of eight selected miRNAs in cucumber SSSL508-28 and D8 lines 48 h after PM inoculation by stem-loop qRT-PCR. Data are the means of three replicates (±SD). The U6 gene was used as an internal control to normalize the expression data. Means with the same lowercase letter do not significantly differ by the least significant difference test at p ≤ 0.05 with a completely randomized design. Regression analysis between the eight miRNA expression levels measured by RNA sequencing and qRT-PCR indicates strong correlation between the two approaches with Pearson correlation coefficient r2 = 0.611. The gene expression values were transformed to the log2 scale.
For example, our stem-loop qRT-PCR experiment showed that Csa-miR171g was downregulated in PM-inoculated SSSL508-28, whereas no significant difference was found from the sequencing results.
The discrepancies were also previously described and may be ascribed to sequence bias introduced by sRNA libraries or profiling stem-loop qRT-PCR or to different normalization approaches employed in these two strategies (Pantaleo et al., 2010; Tian et al., 2017).
Identification of miRNA Target Genes by in Silico and Degradome Approaches
To identify miRNA targets, we combined target prediction with degradome sequencing. The in silico approach predicted 517 target genes for the 32 DEMs in the ID versus NID comparison and 20 target genes for the six DEMs in the IS versus NIS comparison (see Supplementary Table 3 for details). Ten predicted targets, Csa2G423580, Csa5G497010, Csa3G144230, Csa6G298490, Csa1G153530, Csa2G215520, Csa7G447980, Csa6G510860, Csa2G076520, and Csa3G872160, were common in the two comparisons. They were targeted by Csa-miR395a-3p and Csa-miR395d-3p in the ID versus NID and IS versus NIS comparisons, respectively. The highest number of targets was identified for Csa-miR172c-3p (139 genes), followed by Csa-miR156g (53 genes) and Csa-miR396d-3p (50 genes). Four degradome cDNA libraries (NID, ID, NIS, IS) were constructed and sequenced to detect miRNA-guided cleavage products. A total of 16,479,774 degradome tags were obtained and mapped to the cucumber genome (9930 V2.0), with approximately 59.7% (over 9.8 million) matching perfectly to the genome. Based on the strength of the degradome signal at the miRNA target sites, a total of 67 target genes corresponding to 101 miRNAs were identified (p < 0.05) (Supplementary Table 4). The 67 target genes fell into three categories (0, 1, and 3). Approximately 85% (57 genes) of them were in category 0, which represents the most abundant degradome tags corresponding to the cleavage site and matching cognate transcripts (Zhu et al., 2019). The highest number of targets (60) was identified for IS, and the lowest number was identified for NIS (35). All the DEM–target pairs identified by degradome sequencing were among those predicted by psRNATarget. These two methods also showed that one target gene could be cleaved by multiply miRNAs. For example, Csa3G020600 (a GRAS TF) was cotargeted by Csa-miR171f-3p, Csa-miR171a-3p, and Csa-miR171g at the same location (Figure 4).
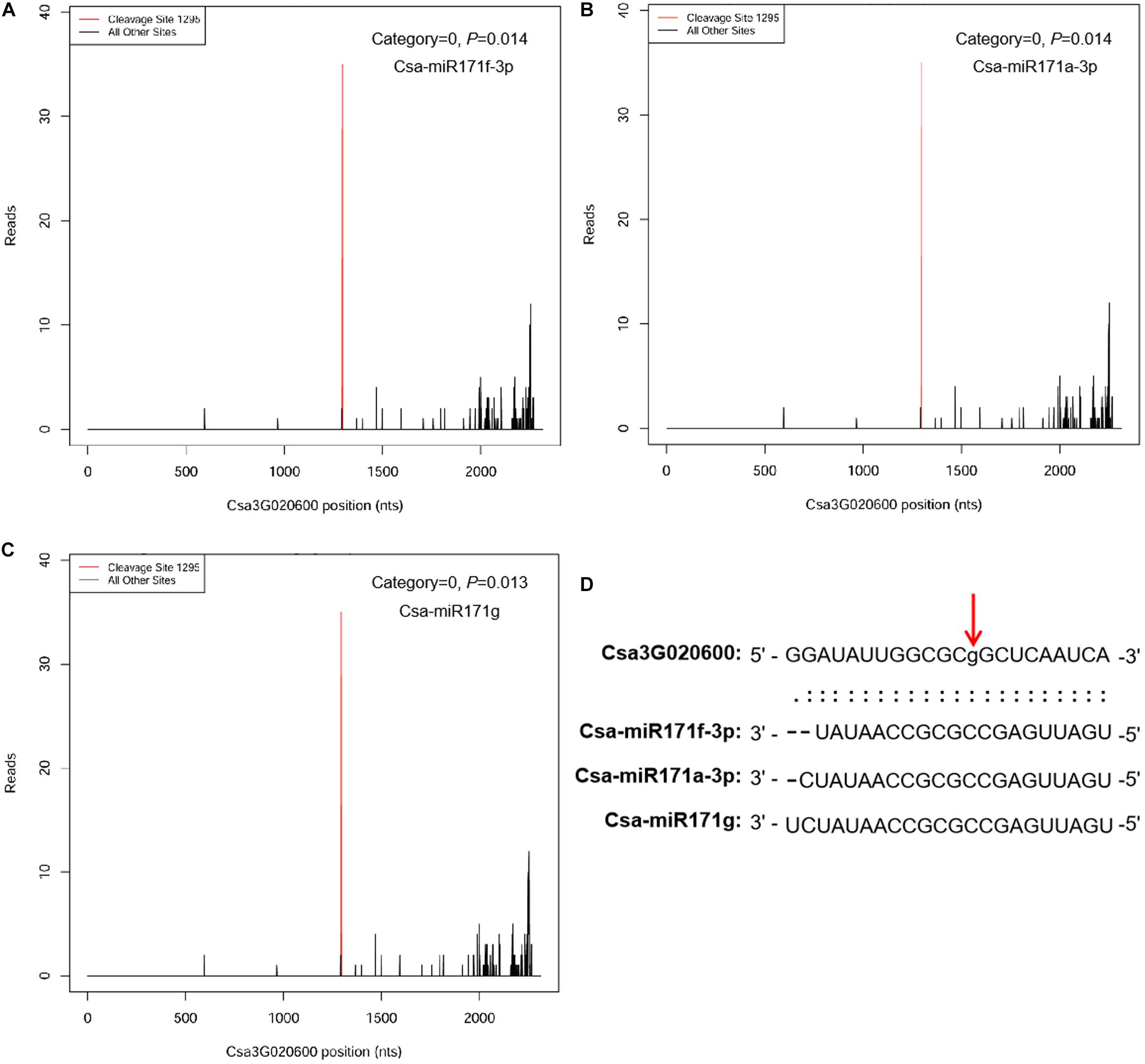
Figure 4. MicroRNAs Csa-miR171f-3p, Csa-miR171a-3p, and Csa-miR171g cotarget Csa3G020600. (A) Degradome target plot (T-plot) of Csa-miR171f-3p. (B) Degradome T-plot of Csa-miR171a-3p. (C) Degradome T-plot of Csa-miR171g. (D) Alignment of the Csa3G020600 and mature Csa-miR171f-3p, Csa-miR171a-3p, and Csa-miR171g sequences. The red vertical arrow above the aligned sequences indicates the cleavage site. The x axis indicates the positions in the Csa3G020600 cDNA, and the y axis indicates the numbers of degradome sequencing reads.
Gene ontology slim classification analysis was carried out to elucidate the potential functions of the DEM targets in response to PM inoculation. We obtained 86 different GO annotations for the target genes (Supplementary Table 5). The GO terms including carbohydrate metabolic process (GO:0005975, 31 genes), lipid metabolic process (GO:0006629, 16 genes), photosynthesis (GO:0015979, five genes), signal transduction (GO:0007165, 28 genes), and response to endogenous stimulus (GO:0009719, 13 genes) were among others (Figure 5A). It has been suggested that miRNAs may provide genetic switch mechanisms to essentially inactivate the target genes by regulation of TF functioning and TF-mediated events (Chen et al., 2011; Arora et al., 2013). In total, 77 targets belonging to 19 TF families were detected, which accounted for nearly 15% of the target genes of the 32 DEMs in ID versus NID. These TFs included members of the MYB (20 genes), NAC (nine genes), and SBP (eight genes) families (Figure 5B; see Supplementary Table 6 for details).
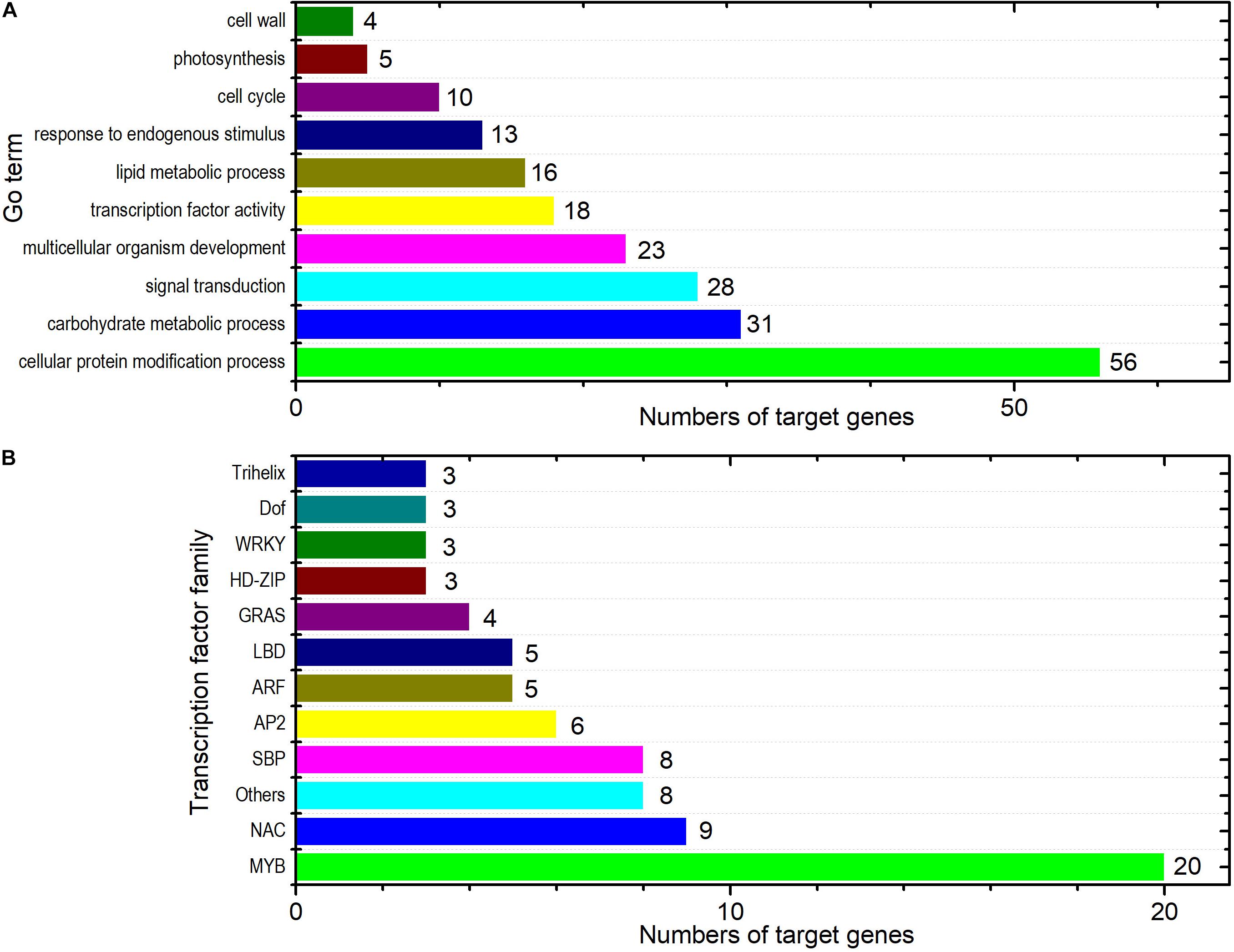
Figure 5. Functional annotation of the predicted target genes of the DEMs. (A) Selected GO terms that were significantly enriched among the predicted target genes. (B) Graphical representation of the target genes annotated as TFs under their assigned TF families. Other TF families among the target genes included BES1, bHLH, bZIP, C2H2, C3H, CO-like, FAR1, HB-PHD, Nin-like, and TALE. Detailed information about these families is available in the plant TF database V3.0 (http://planttfdb.cbi.pku.edu.cn/).
Negatively Regulated miRNA Target Genes
Given that miRNAs negatively regulate their targets, we considered that true targets will be downregulated when the miRNA is upregulated under the same PM-inoculation treatment and vice versa (Xu et al., 2019). Thus, the expression of PM-responsive DEMs (from the current sRNA-seq data) and their target genes (from the published RNA-seq study that had the same experimental design, GSE81234) were compared. We identified 92 and three miRNA–mRNA interaction pairs in D8 and SSSL508-28, respectively. A complete list of these DEMs and their negatively regulated targets (p < 0.05) with the log2 fold changes and annotations can be found in Supplementary Table 7. The identified miRNA–mRNA pairs accounted for only a small proportion of the predicted targets. The remaining targets either showed no significant change in their expression levels or had similar expression patterns as the corresponding miRNAs.
Among the 92 miRNA–mRNA interaction pairs in D8, two were located on the substituted segment (Chr5:16,676,542–23,484,079 bp; Xu et al., 2017) that includes Csa-miR172c-3p and Csa-miR395a-3p. Eight targets of Csa-miR172c-3p that were negatively regulated upon PM inoculation were classified as TFs, namely, two Dofs (Csa1G009790 and Csa1G033250), one TALE (Csa6G426360), one AP2 (Csa2G279250), one LBD (Csa5G219380), one Trihelix (Csa2G350410), one bHLH (Csa1G612950), and one CO-like (Csa7G031530) (Supplementary Table 7). Among the three miRNA–mRNA interaction pairs in SSSL508-28, three of the DEMs were located on the substituted segment (Csa-miR395d-3p, Csa-miR398d, and Csa-miR398b-3p), and their targets included a polygalacturonase (PG, Csa7G433170) and eukaryotic translation initiation factor 2α (eIF2α, Csa4G011690). A gene encoding UDP-glycosyltransferase 1 (UTG, Csa1G526840) was the target of Csa-miR398b-3p. Only one negatively regulated target of Csa-miR162a, Csa5G524850 (L-aspartate oxidase, Chr5:18,665,821–18,670,912 bp), was located on the substituted segment.
We further investigated the expression dynamics of Csa-miR172c-3p, Csa-miR395a-3p, Csa-miR395d-3p, and Csa-miR398b-3p at 12, 24, 48, and 96 h after PM inoculation by stem-loop qRT-PCR. The details are presented in Figure 6. Csa-miR172c-3p and Csa-miR395a-3p shared similar expression patterns in response to PM inoculation in D8 as compared with SSL508-28. Csa-miR395d-3p was downregulated in SSL508-28 at 48 h and upregulated in D8 at 96 h after PM inoculation. Csa-miR398b-3p was repressed congruously in PM-inoculated SSL508-28. These results further confirmed that these DEMs may be involved in the cucumber–PM interaction.
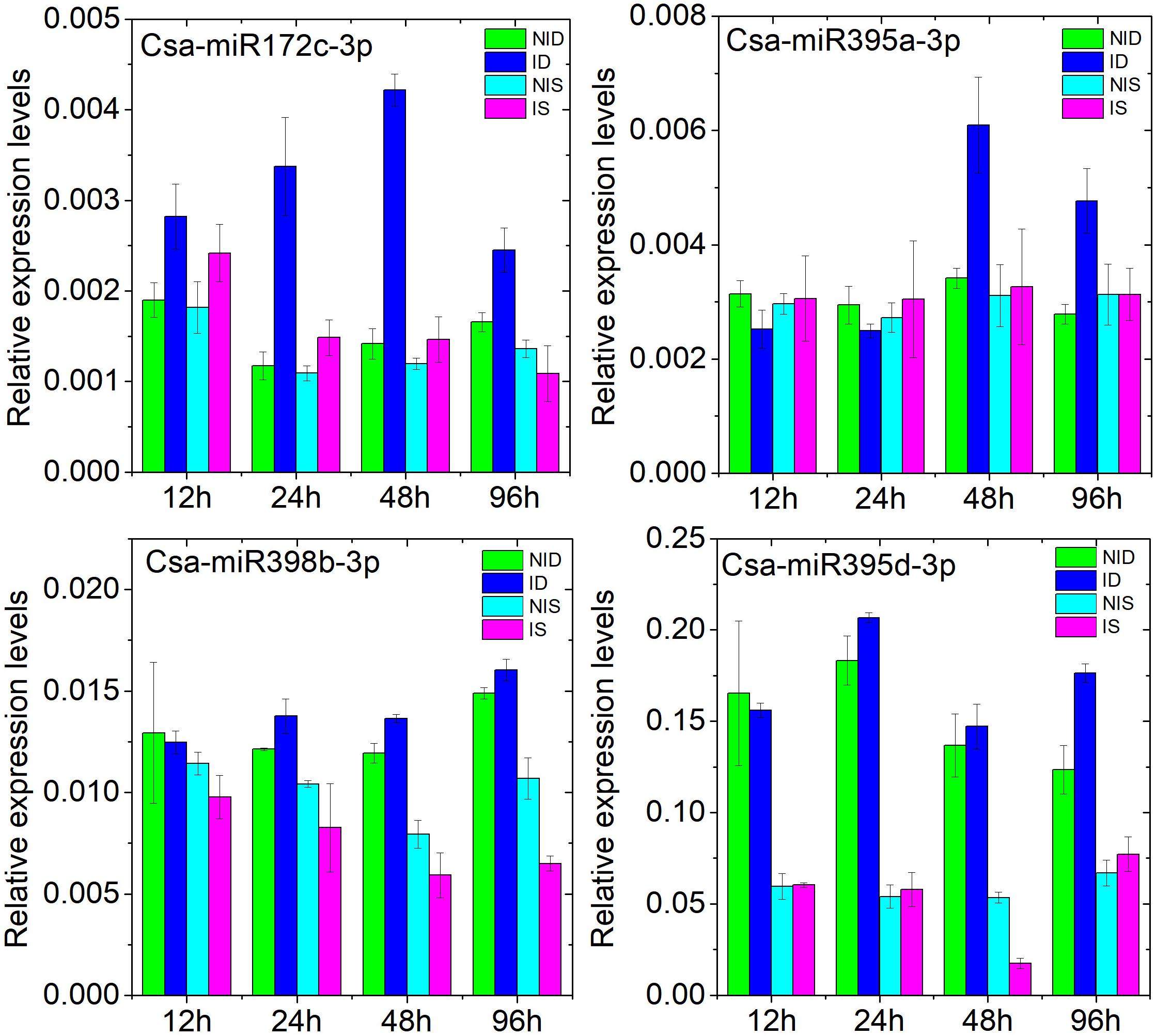
Figure 6. Analysis of Csa-miR172c-3p, Csa-miR395a-3p, Csa-miR395d-3p, and Csa-miR398b-3p at 12, 24, 48, and 96 h after PM inoculation in cucumber SSSL508-28 and D8 lines by stem-loop qRT-PCR. NID, non-inoculated D8 control leaves; ID, PM-inoculated D8 leaves; NIS, non-inoculated SSL508-28 control leaves; IS, PM-inoculated SSL508-28 leaves. Data are the means of three replicates (± SD). The U6 gene was used as an internal control to normalize the expression data.
Discussion
The role of miRNAs in the PM response has been reported in wheat (Xin et al., 2010; Wu et al., 2015) and barely (Hunt et al., 2019), but the relationship between miRNAs and cucumber PM resistance is still not clear. The scanning electron microscopy results showed a higher density of PM hyphae on the leaf surface of D8 than on SSSL508-28 48 h after inoculation (Figure 1), which reflected their different responses to PM. To better understand the molecular mechanisms behind PM resistance in cucumber, we sequenced sRNA and degradome libraries constructed from the PM-infected leaves of D8 and SSSL508-28 at 48 h after treatment and the corresponding non-infected controls. The comparative analysis identified 32 and six PM-responsive DEMs in D8 and SSSL508-28, respectively. More DEMs were identified in the ID versus NID comparison (approximately 5.4-fold) than in the IS versus NIS comparison (Tables 3, 4), which suggested the miRNA expression levels may vary greatly depending on the lines/varieties. All of the 32 DEMs identified in the PM-susceptible D8 were upregulated, whereas most of the DEMs identified in the PM-resistant SSSL508-28 were downregulated. Interestingly, several of these PM-related miRNAs have been reported previously. For instances, Han et al. (2016) found the accumulation of vvi-miR156f in PM-inoculated Chinese wild Vitis pseudoreticulata leaves, and Jagadeeswaran et al. (2009) found miR398 expression decreased in Arabidopsis leaves infiltrated with a virulent strains of Pseudomonas syringae pv. tomato, Pst DC3000. In the current study, we found that several miR156 variants (Cs-miR156j, Cs-miR156k, Cs-miR156, and Cs-miR156 g) were upregulated in PM-inoculated cucumber D8 leaves, which is consistent with the previous studies. In addition, Csa-miR398b-3p and Csa-miR398c were downregulated upon PM inoculation only in SSSL508-28. These results suggested some miRNAs may have similar expression patterns in disease resistance among different plant species. However, many of the DEMs identified in our study were not significantly affected or showed opposite trends to those reported by Xin et al. (2010) and Han et al. (2016), including members of the miR398 (Csa-miR398a-3p and Csa-miR398d) and miR858 (Csa-miR858a and Csa-miR858b) families. These discrepancies suggest that some miRNAs may be species-specific in response to PM inoculation or are expressed at specific developmental stages or in specific tissues. Thus, the expression patterns of these miRNAs need to be studied individually.
MicroRNAs respond to environmental stresses by degrading or inhibiting the translation of their target genes. We obtained more than 500 target genes with diverse functions for the identified DEMs using computational and degradome approaches. The enrichment of GO terms such as regulation of RNA biosynthetic process (GO:2001141) and defense response (GO:0006952) highlighted the importance of these targets in the PM response (Figure 5A). Generally, the accumulation of miRNAs silences their target genes and vice versa (Xu et al., 2019). To reduce the number of false positives and obtain a more mechanistic insight about the regulatory activity of the cucumber DEMs, we focused on those that targeted negatively expressed genes with transcriptomic evidence. The DEMs and/or target genes within the substituted segment of SSL508-28 might be used to prioritize genetic factors because they directly affect phenotypic variation. Csa-miR172c-3p, which is located on the substituted segment, is of particular interest because it accumulated in PM-inoculated D8 leaves and targeted TFs. It is conceivable that miRNAs that target TFs will have an extensive influence on gene expression. We found that Csa-miR172c-3p regulated TFs such as AP2 (Csa2G279250), bHLH (Csa1G612950), and Dofs (Csa1G009790 and Csa1G033250), which have been shown to play important roles in disease responses in plants (Samad et al., 2017; Kessens et al., 2018). Further, expression profiling studies have shown that some of these TFs positively regulate plant immune responses. For example, in barley, several AP2 TFs were upregulated at 12 h after PM inoculation (Molitor et al., 2011), and in the pumpkin resistant line “112-2”, bHLH61 (c71304_g1) was found to be induced at 3, 6, 24, and 48 h after PM infection (Guo et al., 2018). Moreover, seven Dofs (CsDof27, CsDof29, CsDof03, CsDof18, CsDof28, CsDof35, and CsDof36) from cucumber and one from grape (VvDOF3) were found to be induced by inoculation with the downy mildew pathogen and PM, respectively (Wen et al., 2016; Yu et al., 2019). Thus, we hypothesized that, in D8 leaves, the specific downregulation of the TFs targeted by Csa-miR172c-3p may at least partially account for the low PM resistance of the cucumber D8 line. Besides the TFs, Csa-miR172c-3p also targets Csa6G062300, which encodes a type 2c protein phosphatase (PP2C). Protein phosphatases are the obligate partners of protein kinases in the cellular control circuitry (DeLong, 2006). Biological functions have been assigned to several PP2Cs, including organ development (Lita et al., 2003), MAPK signaling (Schweighofer et al., 2004), ABA perception (Park et al., 2009), and disease resistance (Widjaja et al., 2010). Hu et al. (2009) found that ectopic expression of a rice PP2C gene OsBIPP2C2a in tobacco plants resulted in enhanced disease resistance to Phytophthora parasitica var. nicotianae and mosaic virus and constitutive expression of defense-related genes. The PP2C identified in this work, Csa6G062300, was downregulated in D8 after PM inoculation, implying that phosphatase levels regulated by Csa-miR172c may be important for cucumber PM resistance. However, little is known about the two negatively regulated targets of Csa-miR395a-3p, Csa3G020600 (encoding a zinc finger protein), and Csa4G664250 (encoding a coiled-coil domain-containing protein).
Despite the limited number of miRNA–mRNA interaction pairs that were identified in SSL508-28, two of the DEMs (Csa-miR395d-3p and Csa-miR398b-3p) were located on the substituted segment, and many predicted targets have been reported previously to be involved in disease resistance. An inverse expression pattern was found for Csa-miR395d-3p and its target gene Csa4G011690, which encodes the eukaryotic translation initiation factor 2α (eIF2α). eIF2α is required in the initiation of the translation process because it regulates the rate of global protein synthesis (Chambers et al., 2015; Sesma et al., 2017). Enhanced global protein synthesis is cytoprotective in the early response to many biotic stresses. The downregulation of Csa-miR395d-3 and upregulation of eIF2α in SSSL508-28 suggested that the resistant genotype could activate protein synthesis of, for example, receptor-like kinases and receptor-like proteins, at an early stage of infection to restrict the growth of PM pathogens as was reported previously (Tang et al., 2017). Csa-miR398b-3p was found to negatively regulate its target Csa1G526840, which encodes UGT, in response to PM inoculation. This role of Csa-miR398b has not been reported previously. UGTs encode cytosol-localized enzymes that catalyze the conjugation of a range of diverse small lipophilic compounds with sugars to generate water-soluble glycosides (Ahn et al., 2012). The importance of UGTs in plant defense responses against infections has been well characterized. For example, overexpression of UGT74F2 and UGT76B1, or knockout of ugt73b3 and ugt73b5, significantly altered plant resistance to pathogen infection (Langlois-Meurinne et al., 2005; Song et al., 2008; von Saint Paul et al., 2011). Xing et al. (2018) found that wheat overexpressing UGT3 exhibited significantly enhanced resistance to Fusarium head blight and increased the endogenous salicylic acid and jasmonic acid content in the grains compared with in the untransformed control. Therefore, the enhanced expression of the UGT (Csa1G526840) genes in the resistant SSSL508-28 genotype compared with their expression in the susceptible D8 genotype might be associated with PM resistance.
One of the negatively regulated targets of Csa-miR162a, L-aspartate oxidase (LASPO, Csa5G524850), also was located on the substituted segment and was specifically downregulated in PM-inoculated D8 leaves (Supplementary Table 7). Although LASPO is the first enzyme in the de novo biosynthesis of NAD+ pathway in plants, little is known about its biochemical properties in plants. Pétriacq et al. (2012) reported the upregulation of AtLASPO (At5g14760) transcripts in Arabidopsis leaves infected with the hemibiotrophic bacterial plant pathogen P. syringae. Macho et al. (2012) observed that the impaired stomatal immunity against P. syringa was caused by mutations in the gene encoding AtLASPO. Hao et al. (2018) found that AtLASPO expression was clearly correlated with LASPO activity and NAD+ levels. These results suggested a pivotal role for LASPO in NAD+ production upon pathogen infection. We speculated that downregulation of cucumber LASPO by Csa-miR162a in D8 may be another reason for its sensitivity to PM.
Conclusion
Key miRNA–target regulatory pairs were identified in cucumber in response to PM infection by sRNA transcriptomics and degradome sequencing. A total of 32 and six DEMs were identified in the ID versus NID and IS versus NIS comparisons, respectively. The integrated analysis of the expression data identified a total of 92 and three miRNA–mRNA interaction pairs in D8 and SSSL508-28, respectively, which showed inverse expression patterns. The comparative study highlighted an extensive genotype-specific response to PM infection that was different from the common responses in the resistant SSSL508-28 and susceptible D8 genotypes. The miRNAs Csa-miR172c-3p, Csa-miR395a-3p, Csa-miR395d-3p, and Csa-miR398b-3p and the target genes AP2, bHLH, Dof, UGT, and LASPO were found to play critical roles in the PM-inoculated cucumber leaves. Further studies of these miRNAs and target genes will further expand our understanding of the molecular mechanisms underlying PM resistance in cucumber.
Data Availability Statement
The raw small RNA sequencing reads were deposited into NCBI Sequence Read Archive (SRA) under the accession number PRJNA563503. The raw degradome RNA sequencing reads were deposited into NCBI SRA under the accession number PRJNA574048.
Author Contributions
XC conceived and designed the study. XX analyzed the sequencing data and wrote the manuscript. CZ collected the samples and performed the qRT-PCR study. MT, YS, XX, XQ, and QX helped analyze the data and revise the manuscript. All authors reviewed and approved this submission.
Funding
This research was supported by the National Natural Science Foundation of China (Grant Nos. 31171978, 31672176, and 31801883) and the Natural Science Foundation of the Jiangsu Higher Education Institutions of China (Grant No. 18KJB210014).
Conflict of Interest
The authors declare that the research was conducted in the absence of any commercial or financial relationships that could be construed as a potential conflict of interest.
Acknowledgments
We thank Margaret Biswas, Ph.D., from Liwen Bianji, Edanz Group China (www.liwenbianji.cn/ac), for editing the English text of a draft of this manuscript.
Supplementary Material
The Supplementary Material for this article can be found online at: https://www.frontiersin.org/articles/10.3389/fgene.2020.00246/full#supplementary-material
Footnotes
- ^ http://cucurbitgenomics.org/
- ^ http://rfam.xfam.org/
- ^ https://www.arb-silva.de/
- ^ http://gtrnadb.ucsc.edu/
- ^ https://www.girinst.org/
- ^ http://www.mirbase.org/
- ^ http://plantgrn.noble.org/psRNATarget
- ^ http://cucurbitgenomics.org/funcat
References
Addo-Quaye, C., Miller, W., and Axtell, M. (2008). CleaveLand: a pipeline for using degradome data to find cleaved small RNA targets. Bioinformatics 25, 130–131. doi: 10.1093/bioinformatics/btn604
Ahn, S., Vogel, H., and Heckel, D. (2012). Comparative analysis of the UDP-glycosyltransferase multigene family in insects. Insect Biochem. Mol. 42, 133–147. doi: 10.1016/j.ibmb.2011.11.006
Arora, S., Rana, R., Chhabra, A., Jaiswal, A., and Rani, V. (2013). miRNA–transcription factor interactions: a combinatorial regulation of gene expression. Mol. Genet. Genomics 288, 77–87. doi: 10.1007/s00438-013-0734-z
Berg, J. A., Appiano, M., Santillán Martínez, M., Hermans, F. W., Vriezen, W. H., Visser, R. G., et al. (2015). A transposable element insertion in the susceptibility gene CsaMLO8 results in hypocotyl resistance to powdery mildew in cucumber. BMC Plant Biol. 15:243. doi: 10.1186/s12870-015-0635-x
Carrera, J., Rodrigo, G., Jaramillo, A., and Elena, S. (2009). Reverse-engineering the Arabidopsis thaliana transcriptional network under changing environmental conditions. Genome Biol. 10:R96. doi: 10.1186/gb-2009-10-9-r96
Chambers, J. E., Dalton, L. E., Clarke, H. J., Malzer, E., Dominicus, C. S., Patel, V., et al. (2015). Actin dynamics tune the integrated stress response by regulating eukaryotic initiation factor 2α dephosphorylation. eLife 4:e04872.
Chen, C., Chen, S., Fuh, C., Juan, H., and Huang, H. (2011). Coregulation of transcription factors and microRNAs in human transcriptional regulatory network. BMC Bioinformatics 12:S41. doi: 10.1186/1471-2105-12-S1-S41
Cobos, M., Satovic, Z., Rubiales, D., and Fondevilla, S. (2018). Er3 gene, conferring resistance to powdery mildew in pea, is located in pea LGIV. Euphytica 214:203.
DeLong, A. (2006). Switching the flip: protein phosphatase roles in signaling pathways. Curr. Opin. Plant Biol. 9, 470–477. doi: 10.1016/j.pbi.2006.07.015
Fan, C., Cui, H., Ding, Z., Gao, P., and Luan, F. (2019). First report of powdery mildew caused by Podosphaera xanthii on okra in China. Plant Dis. 103, 1027–1027. doi: 10.1094/pdis-09-18-1543-pdn
Fukino, N., Yoshioka, Y., Sugiyama, M., Sakata, Y., and Matsumoto, S. (2013). Identification and validation of powdery mildew (Podosphaera xanthii)-resistant loci in recombinant inbred lines of cucumber (Cucumis sativus L.). Mol. Breed. 32, 267–277. doi: 10.1007/s11032-013-9867-3
Guo, W., Chen, B., Chen, X., Guo, Y., Yang, H., Li, X., et al. (2018). Transcriptome profiling of pumpkin (Cucurbita moschata Duch.) leaves infected with powdery mildew. PLoS One 13:e0190175. doi: 10.1371/journal.pone.0190175
Han, L., Weng, K., Ma, H., Xiang, G., Li, Z., Wang, Y., et al. (2016). Identification and characterization of Erysiphe necator-responsive microRNAs in Chinese wild Vitis pseudoreticulata by high-throughput sequencing. Front. Plant Sci. 7:621. doi: 10.3389/fpls.2016.00621
Hao, J., Pétriacq, P., de Bont, L., Hodges, M., and Gakière, B. (2018). Characterization of l-aspartate oxidase from Arabidopsis thaliana. Plant Sci. 271, 133–142. doi: 10.1016/j.plantsci.2018.03.016
Hunt, M., Banerjee, S., Surana, P., Liu, M., Fuerst, G., Mathioni, S., et al. (2019). Small RNA discovery in the interaction between barley and the powdery mildew pathogen. BMC Genomics 20:610. doi: 10.1186/s12864-019-5947-z
Hu, X., Zhang, H., Li, G., Yang, Y., Zheng, Z., and Song, F. (2009). Ectopic expression of a rice protein phosphatase 2C gene OsBIPP2C2 in tobacco improves disease resistance. Plant Cell Rep. 28, 985–995. doi: 10.1007/s00299-009-0701-7
Islam, W., Islam, S., Qasim, M., and Wang, L. (2017). Host-pathogen interactions modulated by small RNAs. RNA Biol. 14, 891–904. doi: 10.1080/15476286.2017.1318009
Jagadeeswaran, G., Saini, A., and Sunkar, R. (2009). Biotic and abiotic stress down-regulate miR398 expression in Arabidopsis. Planta 229, 1009–1014. doi: 10.1007/s00425-009-0889-3
Kessens, R., Sorensen, N., and Kabbage, M. (2018). An inhibitor of apoptosis (SfIAP) interacts with SQUAMOSA promoter-binding protein (SBP) transcription factors that exhibit pro-cell death characteristics. Plant Direct 2:e00081. doi: 10.1002/pld3.81
Langlois-Meurinne, M., Gachon, C., and Saindrenan, P. (2005). Pathogen-responsive expression of glycosyltransferase genes UGT73B3 and UGT73B5 is necessary for resistance to Pseudomonas syringae pv tomato in Arabidopsis. Plant Physiol. 139, 1890–1901.
Langmead, B., Trapnell, C., Pop, M., and Salzberg, S. (2009). Ultrafast and memory-efficient alignment of short DNA sequences to the human genome. Genome Biol. 10:R25. doi: 10.1186/gb-2009-10-3-r25
Lin, R., He, L., He, J., Qin, P., Wang, Y., Deng, Q., et al. (2016). Comprehensive analysis of microRNA-Seq and target mRNAs of rice sheath blight pathogen provides new insights into pathogenic regulatory mechanisms. DNA Res. 23, 415–425. doi: 10.1093/dnares/dsw024
Lita, P., Miller, A., and Clark, S. (2003). POLTERGEIST encodes a protein phosphatase 2C that regulates CLAVATA pathways controlling stem cell identity at Arabidopsis shoot and flower meristems. Curr. Biol. 13, 179–188. doi: 10.1016/s0960-9822(03)00042-3
Love, M., Huber, W., and Anders, S. (2014). Moderated estimation of fold change and dispersion for RNA-seq data with DESeq2. Genome Biol. 15:550.
Macho, A. P., Boutrot, F., Rathjen, J. P., and Zipfel, C. (2012). Aspartate oxidase plays an important role in Arabidopsis stomatal immunity. Plant Physiol. 159, 1845–1856. doi: 10.1104/pp.112.199810
Martínez-Cruz, J., Romero, D., de Vicente, A., and Pérez-García, A. (2017). Transformation of the cucurbit powdery mildew pathogen Podosphaera xanthii by Agrobacterium tumefaciens. New Phytol. 213, 1961–1973. doi: 10.1111/nph.14297
Molitor, A., Zajic, D., Voll, L., Pons-Kühnemann, J., Samans, B., Kogel, K., et al. (2011). Barley leaf transcriptome and metabolite analysis reveals new aspects of compatibility and Piriformospora indica–mediated systemic induced resistance to powdery mildew. Mol. Plant Microbe Interact. 24, 1427–1439. doi: 10.1094/MPMI-06-11-0177
Pantaleo, V., Szittya, G., Moxon, S., Miozzi, L., Moulton, V., Dalmay, T., et al. (2010). Identification of grapevine microRNAs and their targets using high-throughput sequencing and degradome analysis. Plant J. 62, 960–976. doi: 10.1111/j.0960-7412.2010.04208.x
Park, S. Y., Fung, P., Nishimura, N., Jensen, D. R., Fujii, H., Zhao, Y., et al. (2009). Abscisic acid inhibits type 2C protein phosphatases via the PYR/PYL family of START proteins. Science 324, 1068–1071. doi: 10.1126/science.1173041
Pétriacq, P., de Bont, L., Hager, J., Didierlaurent, L., Mauve, C., Guérard, F. et al. (2012). Inducible NAD overproduction in Arabidopsis alters metabolic pools and gene expression correlated with increased salicylate content and resistance to Pst-AvrRpm1. Plant J. 70, 650–665. doi: 10.1111/j.1365-313X.2012.04920.x
Samad, A. F., Sajad, M., Nazaruddin, N., Fauzi, I. A., Murad, A., Zainal, Z., et al. (2017). MicroRNA and transcription factor: key players in plant regulatory network. Front. Plant Sci. 8:565. doi: 10.3389/fpls.2017.00565
Sanz-Carbonell, A., Marques, M., Bustamante, A., Fares, M. A., Rodrigo, G., and Gomez, G. (2019). Inferring the regulatory network of the miRNA-mediated response to biotic and abiotic stress in melon. BMC Plant Biol. 19:78. doi: 10.1186/s12870-019-1679-0
Schweighofer, A., Hirt, H., and Meskiene, I. (2004). Plant PP2C phosphatases: emerging functions in stress signaling. Trends Plant Sci. 9, 236–243. doi: 10.1016/j.tplants.2004.03.007
Sesma, A., Castresana, C., and Castellano, M. (2017). Regulation of translation by TOR, eIF4E and eIF2α in plants: current knowledge, challenges and future perspectives. Front. Plant Sci. 8:644. doi: 10.3389/fpls.2017.00644
Song, J. T., Koo, Y. J., Seo, H. S., Kim, M. C., Choi, Y. D., and Kim, J. H. (2008). Overexpression of AtSGT1, an Arabidopsis salicylic acid glucosyltransferase, leads to increased susceptibility to Pseudomonas syringae. Phytochemistry 69, 1128–1134. doi: 10.1016/j.phytochem.2007.12.010
Tang, D., Wang, G., and Zhou, J. (2017). Receptor kinases in plant-pathogen interactions: more than pattern recognition. Plant Cell 29, 618–637. doi: 10.1105/tpc.16.00891
Tian, B., Wang, S., Todd, T., Johnson, C., Tang, G., and Trick, H. (2017). Genome-wide identification of soybean microRNA responsive to soybean cyst nematodes infection by deep sequencing. BMC Genomics 18:572. doi: 10.1186/s12864-017-3963-4
von Saint Paul, V., Zhang, W., Kanawati, B., Geist, B., Faus-Keßler, T., Schmitt-Kopplin, P., et al. (2011). The Arabidopsis glucosyltransferase UGT76B1 conjugates isoleucic acid and modulates plant defense and senescence. Plant Cell 23, 4124–4145. doi: 10.1105/tpc.111.088443
Wen, C. L., Cheng, Q., Zhao, L., Mao, A., Yang, J., Yu, S., et al. (2016). Identification and characterisation of Dof transcription factors in the cucumber genome. Sci. Rep. 6:23072. doi: 10.1038/srep23072
Widjaja, I., Lassowskat, I., Bethke, G., Eschen-Lippold, L., Long, H. H., Naumann, K., et al. (2010). A protein phosphatase 2C, responsive to the bacterial effector AvrRpm1 but not to the AvrB effector, regulates defense responses in Arabidopsis. Plant J. 61, 249–258. doi: 10.1111/j.1365-313X.2009.04047.x
Wu, F., Guo, Q., Zhang, W., and Jin, W. (2015). Identification and analysis of powdery mildew-responsive miRNAs in Wheat. J. Phytopathol. 163, 264–270. doi: 10.1111/jph.12315
Xin, M., Wang, Y., Yao, Y., Xie, C., Peng, H., Ni, Z., et al. (2010). Diverse set of microRNAs are responsive to powdery mildew infection and heat stress in wheat (Triticum aestivum L.). BMC Plant Biol. 10:123. doi: 10.1186/1471-2229-10-123
Xing, L., Gao, L., Chen, Q., Pei, H., Di, Z., Xiao, J., et al. (2018). Over-expressing a UDP-glucosyltransferase gene (Ta-UGT3) enhances Fusarium head blight resistance of wheat. Plant Growth Regul. 84, 561–571. doi: 10.1007/s10725-017-0361-5
Xu, Q., Xu, X., Shi, Y., Qi, X., and Chen, X. (2017). Elucidation of the molecular responses of a cucumber segment substitution line carrying Pm5.1 and its recurrent parent triggered by powdery mildew by comparative transcriptome profiling. BMC Genomics 18:21. doi: 10.1186/s12864-016-3438-z
Xu, X., Wang, K., Pan, J., and Chen, X. (2019). Small RNA sequencing identifies cucumber miRNA roles in Waterlogging-triggered adventitious root primordia formation. Mol. Biol. Rep. 46, 6381–6389. doi: 10.1007/s11033-019-05084-z
Xu, X., Yu, T., Xu, R., Shi, Y., Lin, X., Xu, Q., et al. (2016). Fine mapping of a dominantly inherited powdery mildew resistance major-effect QTL, Pm1.1, in cucumber identifies a 41.1 kb region containing two tandemly arrayed cysteine-rich receptor-like protein kinase genes. Theor. Appl. Genet. 129, 507–516. doi: 10.1007/s00122-015-2644-4
Yu, Y. H., Bian, L., Wan, Y. T., Jiao, Z. L., Yu, K. K., Zhang, G. H., et al. (2019). Grape (Vitis vinifera) VvDOF3 functions as a transcription activator and enhances powdery mildew resistance. Plant Physiol. Biochem. 143, 183–189. doi: 10.1016/j.plaphy.2019.09.010
Zeng, X., Xu, Y., Jiang, J., Zhang, F., Ma, L., Wu, D., et al. (2018). Identification of cold stress responsive microRNAs in two winter turnip rape (Brassica rapa L.) by high throughput sequencing. BMC Plant Biol. 18:52. doi: 10.1186/s12870-018-1242-4
Zhang, Z., Jiang, L., Wang, J., Gu, P., and Chen, M. (2014). MTide: an integrated tool for the identification of miRNA-target interaction in plants. Bioinformatics 31, 290–291. doi: 10.1093/bioinformatics/btu633
Keywords: cucumber, powdery mildew, miRNA, target genes, comparative analysis
Citation: Xu X, Zhong C, Tan M, Song Y, Qi X, Xu Q and Chen X (2020) Identification of MicroRNAs and Their Targets That Respond to Powdery Mildew Infection in Cucumber by Small RNA and Degradome Sequencing. Front. Genet. 11:246. doi: 10.3389/fgene.2020.00246
Received: 08 October 2019; Accepted: 02 March 2020;
Published: 26 March 2020.
Edited by:
Meixiang Zhang, Nanjing Agricultural University, ChinaReviewed by:
Weibo Jin, Zhejiang Sci-Tech University, ChinaJing Fan, Sichuan Agricultural University, China
Copyright © 2020 Xu, Zhong, Tan, Song, Qi, Xu and Chen. This is an open-access article distributed under the terms of the Creative Commons Attribution License (CC BY). The use, distribution or reproduction in other forums is permitted, provided the original author(s) and the copyright owner(s) are credited and that the original publication in this journal is cited, in accordance with accepted academic practice. No use, distribution or reproduction is permitted which does not comply with these terms.
*Correspondence: Xuehao Chen, xhchen@yzu.edu.cn