- Department of Epidemiology and Biostatistics, School of Public Health, Peking University, Beijing, China
Background: Genome-wide association studies (GWASs) have identified more than 150 genetic loci that demonstrate robust association with coronary artery disease (CAD). In contrast to the success of GWAS, the translation from statistical signals to biological mechanism and exploration of causal genes for drug development remain difficult, owing to the complexity of gene regulatory and linkage disequilibrium patterns. We aim to prioritize the plausible causal genes for CAD at a genome-wide level.
Methods: We integrated the latest GWAS summary statistics with other omics data from different layers and utilized eight different computational methods to predict CAD potential causal genes. The prioritized candidate genes were further characterized by pathway enrichment analysis, tissue-specific expression analysis, and pathway crosstalk analysis.
Results: Our analysis identified 55 high-confidence causal genes for CAD, among which 15 genes (LPL, COL4A2, PLG, CDKN2B, COL4A1, FES, FLT1, FN1, IL6R, LPA, PCSK9, PSRC1, SMAD3, SWAP70, and VAMP8) ranked the highest priority because of consistent evidence from different data-driven approaches. GO analysis showed that these plausible causal genes were enriched in lipid metabolic and extracellular regions. Tissue-specific enrichment analysis revealed that these genes were significantly overexpressed in adipose and liver tissues. Further, KEGG and crosstalk analysis also revealed several key pathways involved in the pathogenesis of CAD.
Conclusion: Our study delineated the landscape of CAD potential causal genes and highlighted several biological processes involved in CAD pathogenesis. Further studies and experimental validations of these genes may shed light on mechanistic insights into CAD development and provide potential drug targets for future therapeutics.
Highlights
- Conducted an integrative analysis to prioritize the CAD potential causal genes by using eight computational methods.
- Identified 55 high-confidence causal genes for CAD, among which 15 genes ranked the highest priority.
- Plausible causal genes were enriched in lipid metabolic and extracellular region.
Introduction
With the number of all-age deaths closing to nine million in 2017, CAD is the leading cause of mortality worldwide (GBD 2017 Causes of Death Collaborators, 2018). Parallel to the high prevalence and vast number of deaths, CAD also places a crushing economic burden. According to the recent American Heart Association report, the medical costs and productivity losses of CVD are expected to grow from $555 billion in 2015 to $1.1 trillion in 2035 (Dunbar et al., 2018; Benjamin et al., 2019). Although CAD has become a major concern in global public health, there still a long way to fully understand the etiology of CAD before getting it under control.
In addition to lifestyle risk factors such as physical inactivity, unbalanced diet, smoking, alcohol, and obesity (Meier et al., 2019), genetic factors also play a pivotal role in CAD susceptibility (Musunuru and Kathiresan, 2019). Family and twin studies have demonstrated a strong genetic component of CAD, with heritability estimated between 40 and 60% (McPherson and Tybjaerg-Hansen, 2016; Khera and Kathiresan, 2017). To unravel the genetic underpinnings of CAD, multiple large-scale genetic studies have been performed. During the past decade, GWASs have identified more than 150 genetic loci at the commonly accepted genome-wide statistical significant threshold of P < 5 × 10–8 to account for multiple testing (Howson et al., 2017; Klarin et al., 2017; Nelson et al., 2017; van der Harst and Verweij, 2018). Considering the polygenic nature of CAD, more variants are expected to be identified in the near future owing to the rapid increase in sample size (Zhang et al., 2018). However, the success of GWAS has not been fully translated into an ability to find biological mechanisms and therapeutic targets behind these associations (Shu et al., 2018; Musunuru and Kathiresan, 2019).
There exist some difficulties in localizing the causal genes directly from the GWAS results. First, the lead variant identified by GWAS represents a set of variants in LD that usually spans large genomic regions (Farh et al., 2015). The complicated LD between SNPs and causative mutations is a major barrier to pinpoint the plausible causal genes. Second, the genes in the closest physical proximity to the top associated variants may be not the causal genes because of gene regulation (Smemo et al., 2014). The causal variants mediate the effect on disease risk through either a local effect on gene within the locus or action at a distance on a more remote gene. Therefore, the complexity of LD structure and distal regulation impedes our ability to identify causal genes from GWAS results. To address this issue, many GWAS-based computational methods aiming to prioritize the most likely causal genes have been developed (Rossin et al., 2011; He et al., 2013; Greene et al., 2015; Pers et al., 2015; Tasan et al., 2015; Gusev et al., 2016; Zhu et al., 2016; Shim et al., 2017). For example, Sherlock, SMR, and TWAS prioritize causal genes by combining GWAS and eQTL data; DAPPLE integrates GWAS data with PPI network to identify potential causal genes; DEPICT identifies causal genes through integrating GWAS and predicts gene functions; and prix fixe, NetWAS, and GWAB predict causal genes using co-function network, tissue-specific network, and human functional gene network, respectively.
In this study, we systematically prioritized the potential causal genes for CAD through eight cutting-edge methods (Sherlock, SMR, DAPPLE, NetWAS, prix fixe, GWAB, DEPICT, and TWAS), which are complementary with each other. Candidate causal genes were further characterized by pathway enrichment analysis, tissue-specific enrichment analysis, and pathway crosstalk analysis (Figure 1). This landscape of potential causal genes could provide information and evidence to elucidate the genetic mechanisms underlying CAD.
Materials and Methods
Genome-Wide Association Study Data of Coronary Artery Disease
We used summary statistics from a large-scale CAD GWAS in our study (Nelson et al., 2017). In brief, Nelson et al. (2017) performed a genome-wide association meta-analysis of the United Kingdom Biobank data with two published datasets (CARDIoGRAMplusC4D 1000 Genomes-imputed GWAS (Nikpay et al., 2015) and MIGen/CARDIoGRAM Exome chip study (Stitziel et al., 2016; Webb et al., 2017). The study comprised a total of 71,602 CAD cases and 260,875 controls and identified 304 independent variants, clustering 243 loci, associated with CAD at 5% FDR. More detailed information regarding sample recruitment, genotyping, quality control, and statistical analysis in provided in the original study (Nelson et al., 2017).
Blood Expression Quantitative Trait Locus Data
We used eQTL summary data from the Consortium for the Architecture of Gene Expression (CAGE, n = 2,765 in peripheral blood) and the GTEx Consortium v7 release (n = 369 from whole blood). Briefly, Lloyd-Jones et al. (2017) performed this analysis of 2,765 samples from peripheral blood, with gene expression data observed from Illumina gene expression arrays (38,624 gene expression probes) and SNP genotype data imputed to the 1000 Genomes Phase 1 Version 3 reference panel (∼8 million SNPs). Information about tissue collection, genotyping, RNA quantification, and statistical analysis can be found in the original study of Lloyd et al. (2017). The GTEx project (GTEx Consortium, 2013) contained samples from 44 healthy tissues of 20- to 70-year-old human postmortem donors. For GTEx eQTL data (v7), whole blood tissues of 369 individuals were used, and gene expression levels measured by RNA-seq. SNP genotyping was performed using the Illumina OMNI SNP Arrays.
Prioritization of Coronary Artery Disease Candidate Causal Genes
Sherlock Integrative Analysis
Based on the assumption that the expression change of a specific gene may contribute to CAD risk, we used Sherlock integrative analysis method to integrate SNP associations from CARDIoGRAMplusC4D consortium and blood eQTL from GTEx (He et al., 2013). Sherlock utilizes a Bayesian statistical framework to infer causal genes. It calculates the logarithm of Bayes factor (LBF) for each gene to represent the probability of association between specific gene and CAD. Bonferroni correction was used to correct the P value of genes identified by Sherlock integrative analysis. The corrected threshold of P value is 8.7 × 10–6 (there were 5,747 genes in the eQTL test).
Summary Data-Based Mendelian Randomization Analysis
Similar to Sherlock, SMR performs integrative analysis through integrating genetic associations from GWAS and eQTL data from CAGE (Zhu et al., 2016). Additionally, SMR uses HEIDI test to distinguish pleiotropy from linkage. Genes were considered as plausible causal gene only if they passed both SMR and HEIDI tests. The genome-wide significant threshold was defined as PSMR < 5.24 × 10–6, because we retained 9,538 gene expression probes from the CAGE eQTL analysis.
Disease Association Protein–Protein Link Evaluator
Based on the “guilt by association” principle, many previous studies incorporated PPI network into GWAS analysis in order to prioritize candidate genes (Jia et al., 2012; Jia and Zhao, 2014), and DAPPLE is one of the popular approaches (Rossin et al., 2011). It requires a set of association loci as input and prioritizes genes according to connectivity in the PPI network. Genes with a corrected P value less than 0.05 will be considered as potential causal genes.
Network-Wide Association Study
Network-Wide Association Study is a machine learning method that combines gene-level association with the tissue-specific interaction network (Greene et al., 2015). The network was built using 14,000 publications and low-throughput tissue-specific expression data, which could describe the gene–gene functional interactions within a specific tissue. In this study, we used VEGAS2 (Mishra and Macgregor, 2015) to convert SNP-based summary statistics into gene-based P values. We prioritize the genes in a network built from liver tissue and set 0.05 as P value threshold, which implied that genes with P value below this cutoff were treated as positive in NetWAS.
Prix Fixe Analysis
The prix fixe strategy uses co-function networks to prioritize genes from multiple disease-associated loci (Tasan et al., 2015). It identifies genes by evaluating the significance of combinations of genes, with one gene from each GWAS candidate locus, in a gene network. In this study, the top 100 index SNPs from CAD GWAS were used as input for prix fixe (as prix fixe only accepts a maximum of 100 SNPs as input). By averaging the importance measurements, prix fixe (PF) score was obtained to prioritize candidate genes.
Genome-Wide Association Boosting
Holding the view that the genes associated with a disease tend to be functionally together, GWAB prioritizes candidate disease genes by integrating the GWAS data and human functional gene network (Shim et al., 2017). Unlike other network-based approach, GWAB has an advantage that it integrates complementary information from both population-based approach and molecular profiling approach to identify disease-associated genes. Therefore, it can make the best of information identified from functional experiments apart from the statistical association from GWAS. Shim et al. (2017) used GWAS data from Schunkert et al. (2011) as input and referenced genes from three disease gene databases (OMIM, DO, and CADgeneDB) as benchmark to predict for disease-associated genes. We therefore included Jung Eun Shim’s results into our study.
Transcriptome-Wide Association Studies
The TWAS (Gusev et al., 2016) is a powerful strategy that integrates SNP–expression correlation, GWAS summary statistics, and LD reference panels to identify genes whose cis-regulated expression is associated with disease risk. This method can be conceptualized as having imputed expression data for all cases and controls who participated in GWAS. It then correlated the imputed gene expression with traits to perform a TWAS and identified significant expression-trait associations. Mancuso et al. (2017) have integrated gene expression measurements from 45 expression panels with 30 large-scale summary GWAS data to gain insight into the role of expression in the etiology of complex traits by using TWAS. We therefore included Mancuso’s results into our study.
Data-Driven Expression Prioritized Integration for Complex Traits
The DEPICT tool predicts the most likely causal genes by integrating GWAS and data and gene functions (Pers et al., 2015). Predefined gene sets and gene-transcriptional component matrix developed from gene expression data are used to obtain gene function. van der Harst and Verweij (2018) have prioritized candidate causal genes for CAD using DEPICT. They defined sentinel SNPs as the most significant variant in a 1-Mb region that was independent from other sentinel SNPs (r2 < 0.1), which was consistent with Pers et al. (2015). Therefore, we included Van der Harst’s results (van der Harst and Verweij, 2018) (prioritized causal gene list) into our study.
Ranking the Potential Causal Genes
We used eight different approaches to predict the potential causal genes of CAD. As they are complementary with each other, we assigned equal weights to all methods. Each candidate gene will receive a score of 1 if it is identified by any of the above-mentioned approaches. A cumulative scoring strategy was performed to rank the causal genes. For example, if a gene was only identified by Sherlock analysis, the total score of this gene was 1 point. If a gene was identified by both Sherlock and SMR, the total score of this gene was 2 points. The higher the score of a gene, the more consistent its evidence for identification across the methods and thus the greater the likelihood of involvement in disease susceptibility.
Gene Ontology and Kyoto Encyclopedia of Genes and Genomes Enrichment Analysis
We performed GO analysis to test if the identified potential causal genes were significantly enriched in specific functional categories by using DAVID (Huang da et al., 2009a,b). Three GO terms, including biological process (BP), cellular component (CC), and molecular function (MF) were used. We also conducted KEGG enrichment analysis using ToppGene (Chen et al., 2009). KEGG pathways and GO terms with adjusted P values less than 0.05 [Benjamini and Hochberg (BH) method for correction of multiple testing] were considered as statistical significant.
Tissue-Specific Enrichment Analysis
In order to identify the most relevant tissue for CAD candidate causal genes, we calculated a pSI score for each gene to represent its enrichment in a specific tissue. Details of pSI score calculation can refer to the original publication (Wells et al., 2015). For each tissue, genes with pSI < 0.05 are considered as significantly enriched in the tissue. The overlap between CAD potential causal genes and the genes enriched in each tissue was estimated by Fisher’s exact test. Bonferroni correction was used to adjust for multiple testing. The significant threshold was defined as P < 0.002, because 25 tissues were tested.
Analysis of Protein–Protein Interaction and Co-Expression Network
To explore the physical interaction among the proteins encoded by the potential causal genes, we used human PPI data from STRING (Szklarczyk et al., 2017). Additionally, we further investigated the co-expressed genes of the prioritized causal genes using GeneMANIA (Montojo et al., 2014). Cytoscape was employed to visualize the network (Shannon et al., 2003). We assumed that the gene association density of CAD potential causal gene set was higher than that of random gene sets. To test this hypothesis, we generated 1,000 random gene sets, each with the same number of genes as CAD potential causal gene set, and we assessed the significance using Z-test.
Pathway Crosstalk Analysis
In order to explore the interactions among significantly enriched pathways, we further performed pathway crosstalk analysis. Two pathways were considered to crosstalk if they share a proportion of genes prioritized in our study. We employed two measurements to indicate the overlap of a pair of pathways: the Jaccard coefficient and the overlap coefficient , where A and B represent the number of candidate genes in the two pathways. In order to preclude non-specific inclusion of crosstalk, we set up the following rules: (1) only pathways with adjusted P values < 0.05 were included; (2) only pathways contained at least three candidate genes were included; and (3) pathway pairs with less than two overlapped candidate genes were removed. We visualized the results of pathway crosstalk analysis using Cytoscape (Shannon et al., 2003).
Results
Potential Causal Genes Identified by Sherlock
To identify genes whose expression level change may confer risk of CAD, we systematically integrated genetic associations from the largest GWAS of CAD so far with blood eQTL data from GTEx using Sherlock, a Bayesian statistical framework. We identified 17 potential causal genes whose expression level may affect CAD risk at P < 8.7 × 10–6 (Bonferroni corrected P < 0.05, Supplementary Table S1). For each gene, at least one SNP showed significant association with the expression of this gene and CAD risk simultaneously, implying that these SNPs may exert their effects through regulating gene expression.
Potential Causal Genes Identified by SMR
We further utilized a different integrative analysis method, SMR, to identify CAD risk genes through integrating CAD GWAS and blood eQTL data from CAGE. SMR identified six genes at P < 5.24 × 10–6. However, VAMP8 and MAT2A did not pass HEIDI test (P < 0.05), and only four genes (PSRC1, LIPA, SWAP70, and NT5C2) were retained (Supplementary Table S2). Intriguingly, three of them (PSRC1, LIPA, and NT5C2) were also identified by Sherlock, bringing further evidence to support that they might be the authentic risk genes for CAD.
Potential Causal Genes Identified by DAPPLE
Disease Association Protein–Protein Link Evaluator prioritizes disease-associated genes through using PPI data. A total of 58 genes were identified at corrected P < 0.05 (Supplementary Table S3). The top prioritized genes included SVEP1, APOB, FES, SLC22A4, PLG, ABCG5, FLT1, HNF1A, LDLR, and UBE2Z. GO analysis showed that these prioritized genes were enriched in lipid metabolism, transportation, and storage-related pathways (Supplementary Table S4).
Potential Causal Genes Identified by NetWAS
We further mapped the gene-wide results to liver-specific network and classified by machine learning models as implemented by NetWAS. The top 50 genes were included in our further analysis (Supplementary Table S5). The most significant GO terms enriched among promising candidates genes were “protein binding” (corrected P = 7.6 × 10–8), “cytosol” (corrected P = 2.0 × 10–7), and “nucleoplasm” (corrected P = 4.3 × 10–7) (Supplementary Table S6).
Potential Causal Genes Identified by Prix Fixe
We further predicted CAD causal genes using prix fixe, which incorporates the functionally coherent subnetworks into network analysis. Ten genes were identified by prix fixe, including PCSK9, DHCR24, SOD2, LPA, PLG, MAP3K4, CDKN2B, CDKN2A, ADORA2A, and DERL3 (Supplementary Table S7). Of note, PCSK9 ranked the highest score among those genes. Genetic evidence indicated that individuals with inactivating mutations in PCSK9 have decreased levels of circulating LDL and CAD risk, which led to the two monoclonal antibodies that inhibit PCSK9 to be approved by the United States Food and Drug Administration (Cohen et al., 2006). The finding of PCSK9 further reinforced other genes identified by prix fixe as promising causal genes for CAD.
The Integrated Landscape of Causal Genes in Coronary Artery Disease
We utilized different approaches (including Sherlock, SMR, DAPPLE, NetWAS, prix fixe, GWAB, DEPICT, and TWAS) to prioritize the potential causal genes for CAD. In order to obtain the global landscape of plausible causal genes, we also integrated candidate causal genes identified by previous studies. Studies included in our further analysis are summarized as follows: (1) Causal genes predicted by Pavlides et al. using SMR (Pavlides et al., 2016). By integrating Westra blood eQTL data (Westra et al., 2013) and CARDIoGRAMplusC4D 1000 Genomes-based GWAS data (60,801 cases and 123,504 controls) (Nikpay et al., 2015), Pavlides et al. (2016) used SMR to prioritize genes and identify five candidate genes (VAMP8, SWAP70, IL6R, ATP5G1, and EIF2B2) that passed both SMR and HEIDI test (Supplementary Table S8). (2) Causal genes predicted by GWAB (Shim et al., 2017). Jung Eun Shim et al. used GWAS data from CARDIoGRAMplusC4D consortium (22,233 cases and 64,762 controls) as input and references genes from three disease gene databases (OMIM, DO, and CADgeneDB) as benchmark to predict for disease-associated genes. After network boosting, 35 genes from the largest component of the network were significantly associated with CAD (GWAB score > 7.3) (Supplementary Table S9). (3) Causal genes predicted by DEPICT (van der Harst and Verweij, 2018). Gusev et al. (2016) predicted the causal genes for CAD recently. A total of 433 genes were identified as plausible candidate genes for CAD (FDR < 0.01) (Supplementary Table S10). (4) Causal genes predicted by TWAS. Mancuso et al. (2017) integrated CARDIoGRAM consortium GWAS data (Schunkert et al., 2011) with various expression panels and identified 12 genes significantly associated with CAD (Supplementary Table S11).
Given that the results of the eight analytical methods are correlated but not identical, genes genuinely involved in disease susceptibility would be expected to show consistent results across several methods. We therefore adopted the method of cumulative scoring strategy and ranked the plausible causal genes by their frequency of occurrences in the results of different approaches. We summarized the results in Table 1 and generated the landscape of potential causal genes in CAD (Figure 2). This yielded 55 candidate genes with total score ≥2, among which 15 genes were captured by at least three analysis methods. Therefore, these 15 genes (LPL, COL4A2, PLG, CDKN2B, COL4A1, FES, FLT1, FN1, IL6R, LPA, PCSK9, PSRC1, SMAD3, SWAP70, and VAMP8) were considered as the most promising causal genes for CAD. We queried the NHGRI-EBI GWAS Catalog (Buniello et al., 2019) about the candidate genes to assess their potential pleiotropic effects. Notably, many of these genes provided evidence of pleiotropic effect of lipid traits, which confirmed the significant role of lipid metabolism in CAD.
To identify the biological roles of the 55 potential causal genes, we performed GO enrichment analysis using DAVID. We found that candidate genes were significantly enriched in the GO term such as cholesterol homeostasis (corrected P < 9.6 × 10–7), lipoprotein metabolic process (corrected P < 5.1 × 10–5), receptor complex (corrected P < 2.5 × 10–4), cholesterol transporter activity (corrected P < 2.6 × 10–5), extracellular region (corrected P < 6.8 × 10–3), and apolipoprotein binding (corrected P < 1.2 × 10–3) (Figure 3). These results indicated that lipid metabolic and extracellular region might play a critical role in the pathophysiology of CAD.
We then examined the most relevant tissue of CAD potential causal genes using tissue-specific enrichment analysis. As shown in Figure 4, these genes tended to be significantly overexpressed in liver (P = 2.2 × 10–6) and adipose tissue (P = 2.8 × 10–7). Considering genes are usually act synergistically to exert their biological function, also called as “guilt by association” principle, we further performed network analysis using PPI and expression data. As shown in Figure 5, genes in the PPI network (Figure 5A) and co-expression network (Figure 5B) demonstrated more interaction among themselves than what would be expected for a random set of genes of the same size (P < 1.0 × 10–16). Dysregulation of any gene in this highly interconnected network will affect the function of the network, which eventually lead to pathogenesis of CAD.
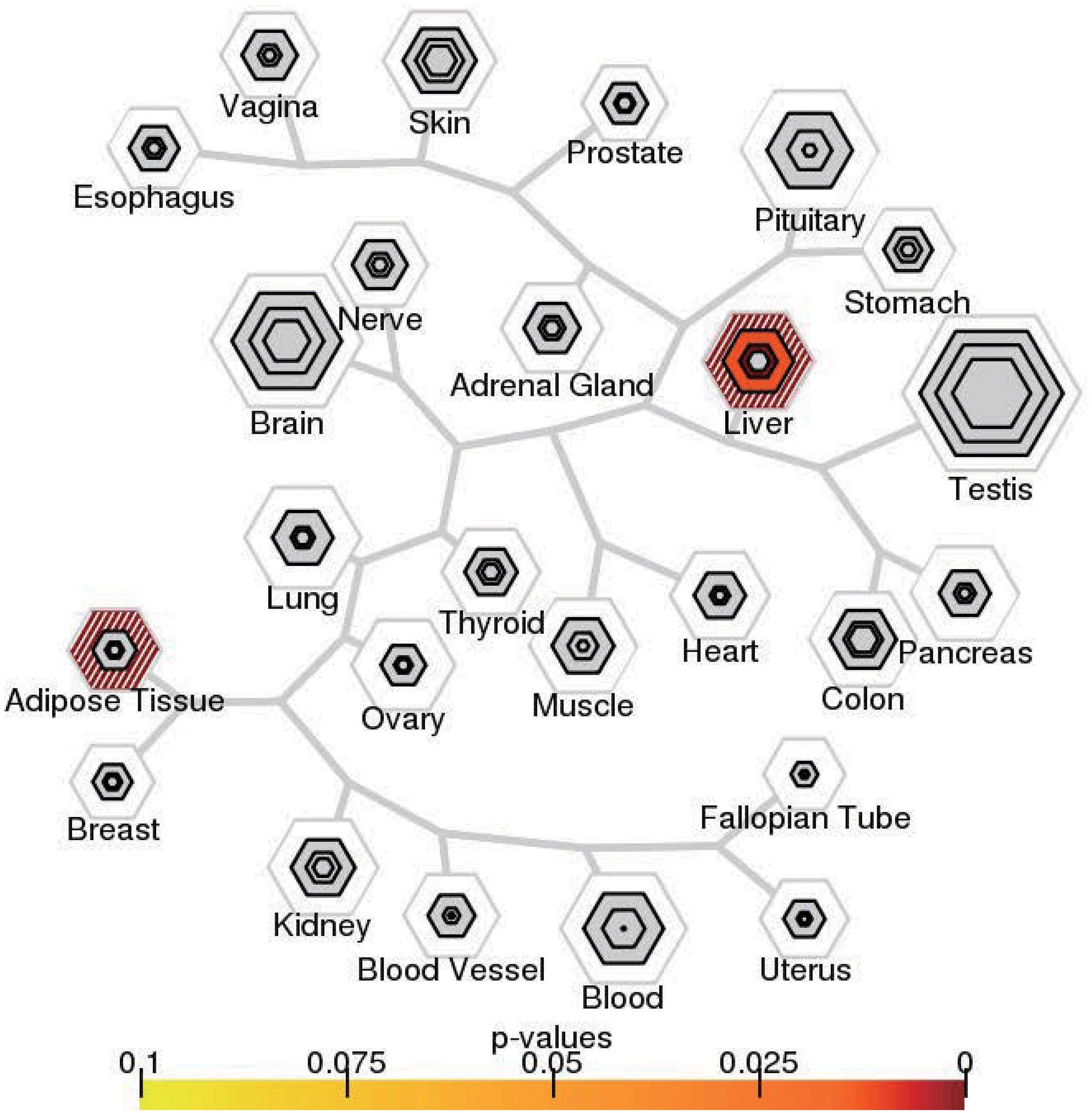
Figure 4. Tissue-specific expression enrichment of CAD potential causal genes. The 53 tissues in GTEx were grouped into 25 broad tissue types. The size of the hexagon was scaled to the number of genes meeting the pSI threshold, and its color indicates results of Fisher’s exact test.
Kyoto Encyclopedia of Genes and Genomes Enrichment Analysis and Pathway Crosstalk Analysis
Identifying KEGG pathways enriched in the candidate genes may provide further insight of the molecular mechanisms related to CAD. Table 2 shows the 24 significant enriched pathways. Among these, the top five pathways are fat digestion and absorption (PBH = 6.63 × 10–5), AGE-RAGE signaling pathway in diabetic complications (PBH = 1.92 × 10–4), pathways in cancer (PBH = 1.12 × 10–3), PI3K-Akt signaling pathway (PBH = 1.90 × 10–3), and focal adhesion (PBH = 3.96 × 10–3).
In order to gain deep understanding of how these pathways are related, we further performed a pathway crosstalk analysis. There were 22 pathways containing at least three candidate genes and met the inclusion criteria for crosstalk analysis. A total of 73 edges connected between any two of these pathways, and these edges represented the overlapping level, which was measured according to the average score of JC and OC. As shown in Figure 6, the largest crosstalk module comprised 20 pathways. By considering the topological characteristics, we identified five key pathways (pathways in cancer, AGE-RAGE signaling pathway in diabetic complications, proteoglycans in cancer, endocytosis, and PI3K-Akt signaling pathway), which had the highest betweenness centrality and degree value (Supplementary Table S12). Previous studies had provided some clues about their role in pathogenesis of CAD (Chen et al., 2016; Kay et al., 2016; Koene et al., 2016).
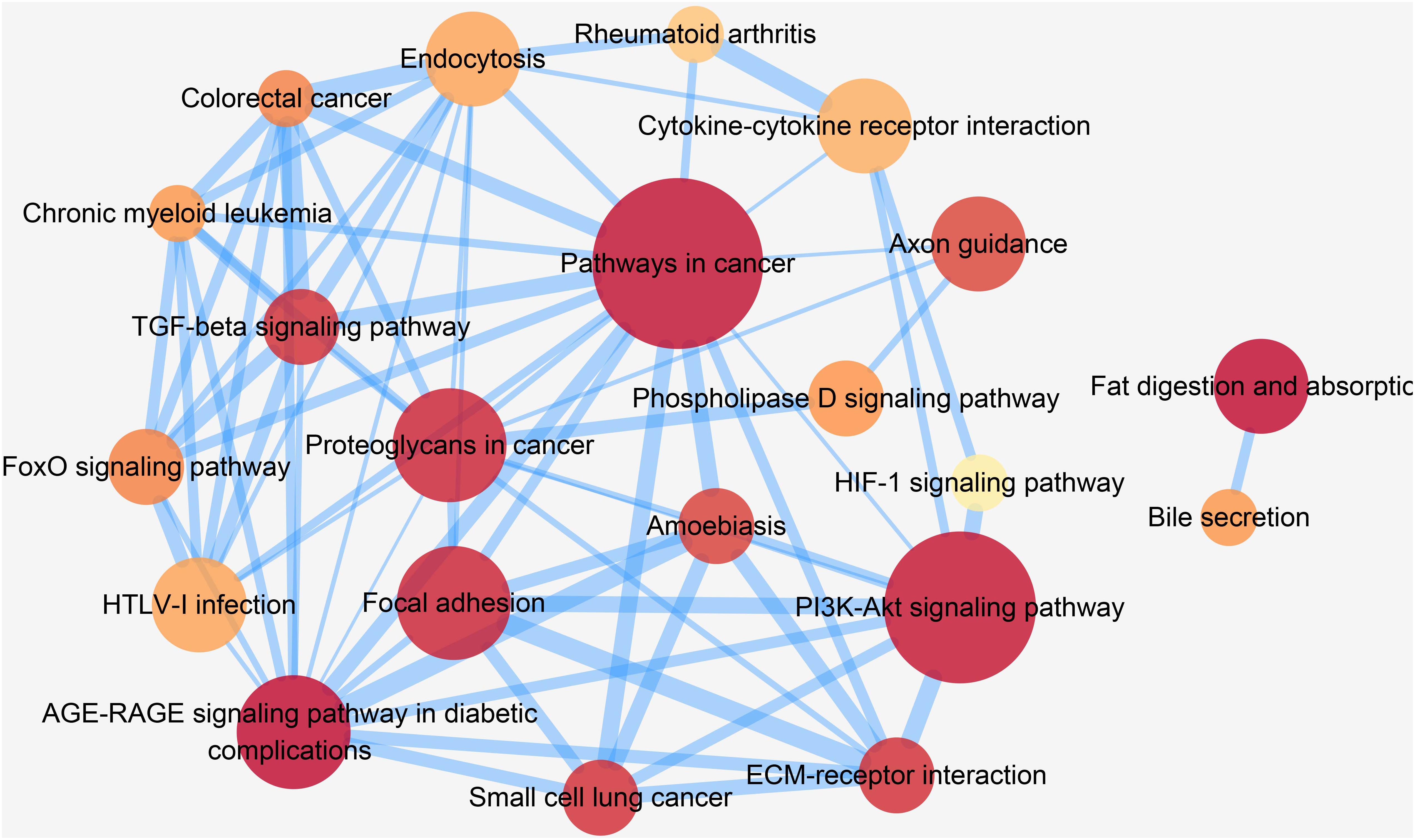
Figure 6. Crosstalk network among CAD prioritized causal genes enriched pathways. In this figure, each node represents a pathway, and each edge represents crosstalk between pathways. The color of each node is proportional to the adjusted P [Benjamini and Hochberg (BH) method] value. Darker color represents lower PBH value. The size of each node is proportional to the number of CAD prioritized causal genes in the corresponding pathway. The width of each edge is proportional to the mean value of the two coefficients (JC and OC). Larger edge width represents higher proportion of overlap between pathways.
Discussion
Although 163 loci have now been associated with CAD at a genome-wide level of significance (Erdmann et al., 2018), the reported loci usually span a large chromosomal region and contain many genes. Thus, it is difficult to pinpoint the causal gene. Besides, owing to the complexity of gene regulatory and LD pattern, this problem becomes even more complicated. Consequently, incorporating other source of prior knowledge is necessary to narrow down the CAD candidate genes. In this study, we conducted a comprehensive integrative analysis and prioritized the potential causal genes for CAD using the latest GWAS and other omics data from different layers. A total of 55 plausible causal genes were identified, among which 15 genes ranked the highest priority because of their consistent evidence from different data-driven approaches. Many of these genes were involved in in lipid metabolic and extracellular related BP. Moreover, they were enriched in liver and adipose tissue. Further, KEGG and crosstalk analysis also revealed several key pathways participating in the pathogenesis of CAD.
Our comprehensive analysis predicted 55 CAD causal genes. Many of these genes are well-known CAD driver genes and participant in lipid metabolic process (LPL, LPA, PCSK9, APOA1, APOB, CETP, LDLR, and LIPA). Some of them have been translated into the targets of commercialized drugs (APOB and PCSK9). Besides, GO enrichment analysis also highlight the importance of lipid involved in CAD pathogenesis. However, targeting novel pathways instead of established CAD risk factors, such as lipid levels, may facilitate the drug development and catalyze novel CAD therapeutic options. Our GO analysis also pinpointed that the identified potential causal genes were enriched in extracellular region apart from lipid metabolic. Extracellular matrix has a critical role in cell adhesion, integrity, and communication. Changes in the extracellular space have been implicated in the pathogenesis of atherosclerosis and restenosis (Chistiakov et al., 2013). Previous network analysis also revealed the key role of extracellular region involved in CAD development and the relationship between other molecular mechanisms, such as inflammatory response and complement and coagulation (Zhao et al., 2016).
In addition to identifying the well-known CAD driver genes, our comprehensive analysis also detected novel or less well-studied plausible causal genes for CAD. For example, MAP3K4 encodes Mitogen-Activated Protein Kinase 4, which is the central core of each mitogen-activated protein (MAP) kinase pathway. Four well-characterized MAP kinase pathways, including ERK1/2, JNK, p38, and ERK5, have been reported their role in different aspects of cardiac regulation, from development to pathological remodeling (Wang, 2007). In line with our study, Ballouz et al. (2014) analyzed CARDIoGRAMplusC4D GWAS data by Gentrepid and found that MAP3K4 was one of the possible causal genes of CAD. Another candidate, CABIN1, plays a pivotal role in the T cell receptor-mediated signal transduction pathway and inhibits calcineurin-mediated signal transduction. Calcineurin lies at the intersection of protein phosphorylation and calcium signaling cascades and contributes to pathological hypertrophic remodeling (Letavernier et al., 2012; Parra and Rothermel, 2017). Another identified gene, EPOR, encodes erythropoietin (EPO) receptor. EPO has shown its effect beyond hematopoiesis, such as suppression of atherosclerosis (Ueba et al., 2013) and prevention of cardiac apoptosis (Nakamura et al., 2009). In addition, although EpoR agonists drugs are initially aimed at treating anemia, it is starting to demonstrate the sign of pleiotropic effects for treating a wide range of complex disorders, including CVD, neurodegenerative disorders, spinal cord injury, and diabetic retinopathy (Sanchis-Gomar et al., 2013). HNRNPUL1 encodes a nuclear RNA-binding protein and involved in mRNA splicing pathway. LeBlanc et al. (2016) integrated GWAS data of CAD and several CVD risk factors and used a shared polygenic signal-informed statistical framework to discover novel CAD genes. Totally, they identified 67 novel loci associated with CAD. Among these novel loci, rs12459996 near CYP2F1 also showed eQTL effect on HNRNPUL1 gene expression in blood tissue (P = 3.86 × 10–16), which indicated that this locus may exert its effect on CAD susceptibility through regulation of HNRNPUL1 expression. Superoxide Dismutase 2 (SOD2) is a well-known marker of endothelial dysfunction. Several candidate gene studies have revealed that mutations or polymorphisms of SOD2 gene are associated with CAD risk (Mollsten et al., 2009; Tian et al., 2012). Moreover, studies on Sod2-deficient mice demonstrated accelerated atherosclerosis (Zhou et al., 2012) and atherosclerotic plaque instability (Vendrov et al., 2017). These lines of evidence suggested that SOD2 might act an important role in CAD pathogenesis by regulating atherosclerosis and endothelial function.
Pathway enrichment analysis of the prioritized genes further confirmed some results of previous studies. Some key drivers of CAD identified by previous network analysis were cancer-related genes (Zhao et al., 2016). Although recognized as two separate diseases, CAD and cancer possess various similarities, from common risk factors to shared BP (Koene et al., 2016). The oxidative stress and chronic inflammation underlie both of the diseases. In addition, a growing number of evidence support a role for statins, angiotensin-converting enzyme inhibitor/angiotensin receptor blockers (ACEIs/ARBs), and aspirin in cancer prevention (Masoudkabir et al., 2017). Anti-CD47 antibody treatment may become a novel therapeutic strategy for CAD by promoting efferocytosis (Kojima et al., 2016). This convergent evidence indicated that pathways of cancer might take part in the pathogenesis of CAD. AGE-RAGE signaling pathway actively participates in inflammation and immune response. Miao et al. (2019) identified 413 differential expressed genes from two gene expression datasets of CAD cases and healthy controls, and they highlighted the role of AGE-RAGE signaling pathway in CAD pathogenesis. Extensive evidence is building to implicate AGE-RAGE in the pathogenesis of vascular perturbation, which stimulate processes that lead to the development of arterial stiffness (Senatus and Schmidt, 2017). AGE/RAGE signaling also demonstrated its role in diabetes-mediated vascular calcification (Kay et al., 2016). Calcium deposits in coronary arteries may weaken vasomotor responses and alter atherosclerotic plaque stability (Liu et al., 2015). Another two well-known pathways identified in our study were focal adhesion (hsa04510) and PI3K-Akt signaling pathway (hsa04151). Focal adhesion has an influence on both leucocyte motility within intima and interactions between platelets and endothelium through controlling cytoskeletal or adhesion dynamics (Charchar et al., 2012). Chan et al. (2014) employed integrative pathway and network analysis and revealed that focal adhesion was one of the shared molecular pathways for CAD and diabetes across diverse ethnicities. The PI3K-Akt pathway has been reported involved in cell proliferation, survival, and apoptosis. A two-stage systems genetics analysis of CAD GWAS data also found convincing association between PI3K-Akt pathway and CAD (Ghosh et al., 2015). Crosstalk analysis further demonstrated that these pathways might interconnect through overlapping genes instead of acting alone. Therefore, disruption or dysfunction of any key genes in the network may have cascading effects and result in a series of functional pathological consequences.
Our study is different from traditional fine-mapping approaches, which focus on identifying the causal variants that affect a trait of interest. Although very important, knowing which variants are causal does not equal to identifying the downstream effects of the variant on the trait. Moreover, if the causal genes affected by a locus are known, this can reduce the credible set of potential causal variants (Schaid et al., 2018; Broekema et al., 2020). Therefore, recent efforts in systems biology, integrating GWAS data and a priori knowledge from other omics, have focused on identifying such causal genes.
Unlike previous study (Braenne et al., 2015; Miller et al., 2016), which only focused on genome-wide significant region to predict causal genes, our study predicted plausible causal genes for CAD at genome-wide level by using genetic variants of both strong and subtle effects. Besides, to our knowledge, this is the first systematic analysis for CAD using comprehensive data from different layers to prioritize candidate genes. However, this study has several potential limitations. First, our study only paid attention to those genes that were prioritized by at least two computational methods. This strategy may omit some authentic CAD susceptibility genes. Besides, we assigned these methods with equal weights when combining the results. There might exist some discrepancies among the various methods because of different rationales and datasets. Further studies are needed to search the optimum weights. Second, we used eQTL data from peripheral blood samples when we conduced Sherlock and SMR analyses. Although blood tissue can be a proxy for eQTL effect in various diseases, it might be not ideal because we certainly lose power for eQTLs with tissue-specific effects (Supplementary Table S13). However, we gain power for genes with consistent effects across tissues because of the use of a very large sample size for eQTL analysis in blood. Future studies using large-scale eQTL data in pathophysiologically relevant tissues are needed to validate our results. Third, we did not consider other QTLs from different omic layers, such as DNA methylation (meQTLs), protein (pQTLs), or metabolites (mQTLs), which limited our ability to study from multi-omics perspective. In addition, the statistical methods did not perform causal inference; therefore, the genes identified in our study were only plausible candidate causal genes. However, the results still played a pivotal role in prioritizing genes for experimental follow-up. Further studies are needed to validate our results and elucidate the biological mechanisms.
Conclusion
In summary, we integrated multi-dimensional data and depicted the landscape of plausible candidate causal genes for CAD. GO analysis further showed that these genes were enriched in lipid metabolism and extracellular region. Tissue-specific enrichment analysis revealed that these genes were significantly overexpressed in adipose and liver tissues. Further studies and experimental validations of these genes may shed light on mechanistic insights into CAD pathogenesis and provide potential drug targets for future therapeutics.
Data Availability Statement
Our datasets analyzed during the current study were derived from the following public domain resources: Summary statistics of the GWAS is available from CARDIoGRAMplusC4D Consortium (http://www.cardiogramplusc4d.org/data-downloads/). Gene expression dataset is available from GTEx (https://gtexportal.org/home/). eQTL datasets are available from Jian Yang’s lab (https://cnsgenomics.com/software/smr/#Overview).
Ethics Statement
The studies involving human participants were reviewed and approved by Peking University Institutional Review Board has evaluated the current protocol and found that no additional institutional review board approval was needed because the data are public available de-identified summary-level data. The patients/participants provided their written informed consent to participate in this study.
Author Contributions
DC conceived the study, undertook project leadership, and are guarantors of this work. QZ wrote the first draft of the manuscript. QZ and YM analyzed the data and interpreted the data. QZ, YM, SC, and QC were involved in the data collection. All authors contributed to the drafting, critical revision of the manuscript, read and approved the final version of the manuscript.
Funding
This work was supported by the National Natural Science Foundation of China (No. 81872692) and the Beijing Municipal Natural Science Foundation (No. 7182085).
Conflict of Interest
The authors declare that the research was conducted in the absence of any commercial or financial relationships that could be construed as a potential conflict of interest.
Acknowledgments
We thank the investigators of the original GWAS by CARDIoGRAMplusC4D consortium. We also thank the researchers who shared their data on the Gene Expression Omnibus, which made this study possible. The GTEx Project was supported by the Common Fund of the Office of the Director of the National Institutes of Health, and by NCI, NHGRI, NHLBI, NIDA, NIMH, and NINDS. We also acknowledge the investigators for CAGE study, who provided their eQTL dataset for public.
Supplementary Material
The Supplementary Material for this article can be found online at: https://www.frontiersin.org/articles/10.3389/fgene.2020.00320/full#supplementary-material
Abbreviations
CAD, coronary artery disease; CVD, cardiovascular disease; DAPPLE, Disease Association Protein–Protein Link Evaluator; DEPICT, Data-Driven Expression Prioritized Integration for Complex Traits; eQTL, expression quantitative trait locus; FDR, false discovery rate; GO, Gene Ontology; GTEx, Genotype-Tissue Expression; GWAB, Genome-Wide Association Boosting; GWAS, genome-wide association study; KEGG, Kyoto Encyclopedia of Genes and Genomes; LD, linkage disequilibrium; NetWAS, Network-Wide Association Study; PPI, protein–protein interaction; SMR, summary data-based Mendelian randomization; SNP, single-nucleotide polymorphism; TWAS, transcriptome-wide association studies.
References
Ballouz, S., Liu, J. Y., Oti, M., Gaeta, B., Fatkin, D., Bahlo, M., et al. (2014). Candidate disease gene prediction using gentrepid: application to a genome-wide association study on coronary artery disease. Mo.l Genet. Genomic Med. 2, 44–57. doi: 10.1002/mgg3.40
Benjamin, E. J., Muntner, P., Alonso, A., Bittencourt, M. S., Callaway, C. W., Carson, A. P., et al. (2019). Heart disease, and stroke statistics-. (2019). update: a report from the american heart association. Circulation 139, e526–e528.
Braenne, I., Civelek, M., Vilne, B., Di Narzo, A., Johnson, A. D., Zhao, Y., et al. (2015). Prediction of causal candidate genes in coronary artery disease loci. Arterioscler.Thromb.Vasc. Biol. 35, 2207–2217. doi: 10.1161/ATVBAHA.115.306108
Broekema, R. V., Bakker, O. B., and Jonkers, I. H. A. (2020). practical view of fine-mapping, and gene prioritization in the post-genome-wide association era. Open Biol. 10:190221. doi: 10.1098/rsob.190221
Buniello, A., MacArthur, J. A. L., Cerezo, M., Cerezo, M., Harris, L. W., Hayhurst, J., et al. (2019). The NHGRI-EBI GWAS Catalog of published genome-wide association studies, targeted arrays and summary statistics 2019. Nucleic Acids Res. 47, D1005–D1012. doi: 10.1093/nar/gky1120
Chan, K. H., Huang, Y. T., Meng, Q., Wu, C., Reiner, A., Sobel, E. M., et al. (2014). Shared molecular pathways, and gene networks for cardiovascular disease, and type 2 diabetes mellitus in women across diverse ethnicities. Circ. Cardiovasc Genet. 7, 911–919. doi: 10.1161/CIRCGENETICS.114.000676
Charchar, F. J., Bloomer, L. D., Barnes, T. A., Cowley, M. J., Nelson, C. P., and Wang, Y. (2012). Inheritance of coronary artery disease in men: an analysis of the role. (of)the Y chromosome. Lancet 379, 915–922. doi: 10.1016/S0140-6736(11)61453-0
Chen, J., Bardes, E. E., Aronow, B. J., and Jegga, A. G. (2009). ToppGene suite for gene list enrichment analysis, and candidate gene prioritization. Nucleic Acids Res. 37, W305–W311. doi: 10.1093/nar/gkp427
Chen, Q., Xu, T., Li, D., Pan, D., Wu, P., Luo, Y., et al. (2016). JNK/PI3K/Akt signaling pathway is involved in myocardial ischemia/reperfusion injury in diabetic rats: effects of salvianolic acid A intervention. Am. J. Transl. Res. 8, 2534–2548.
Chistiakov, D. A., Sobenin, I. A., and Orekhov, A. N. (2013). Vascular extracellular matrix in atherosclerosis. Cardiol. Rev. 21, 270–288. doi: 10.1097/CRD.0b013e31828c5ced
Cohen, J. C., Boerwinkle, E., Mosley, T. H. Jr., and Hobbs, H. H. (2006). Sequence variations in PCSK9, low LDL, and protection against coronary heart disease. N. Engl. J. Med. 354, 1264–1272. doi: 10.1056/NEJMoa054013
Dunbar, S. B., Khavjou, O. A., Bakas, T., Hunt, G., Kirch, R. A., Leib, A. R., et al. (2018). Projected Costs of Informal caregiving for cardiovascular disease:. (2015)to 2035: a policy statement from the american heart association. Circulation 137, e558–e577. doi: 10.1161/CIR.0000000000000570
Erdmann, J., Kessler, T., Munoz Venegas, L., and Schunkert, H. A. (2018). decade of genome-wide association studies for coronary artery disease: the challenges ahead. Cardiovasc. Res.114, 1241–1257. doi: 10.1093/cvr/cvy084
Farh, K. K., Marson, A., Zhu, J., Kleinewietfeld, M., Housley, W. J., Beik, S., et al. (2015). Genetic, and epigenetic fine mapping of causal autoimmune disease variants. Nature 518, 337–343. doi: 10.1038/nature13835
GBD 2017 Causes of Death Collaborators (2018). Global, regional, and national age-sex-specific mortality for 282 causes of death in 195 countries and territories, 1980–2017: a systematic analysis for the global burden of disease study 2017. Lancet 392, 1736–1788.
Ghosh, S., Vivar, J., Nelson, C. P., Willenborg, C., Segrè, A. V., Mäkinen, V. P., et al. (2015). Systems genetics analysis of genome-wide association study reveals novel associations between key biological processes, and coronary artery disease. Arteriosc. Thromb. Vasc. Biol.. 35, 1712–1722. doi: 10.1161/ATVBAHA.115.305513
Greene, C. S., Krishnan, A., Wong, A. K., Ricciotti, E., Zelaya, R. A., Himmelstein, D. S., et al. (2015). Understanding multicellular function, and disease with human tissue-specific networks. Nature genetics 47, 569–576. doi: 10.1038/ng.3259
Gusev, A., Ko, A., Shi, H., Bhatia, G., Chung, W., Penninx, B. W., et al. (2016). Integrative approaches for large-scale transcriptome-wide association studies. Nat. Genet.48, 245–252. doi: 10.1038/ng.3506
He, X., Fuller, C. K., Song, Y., Meng, Q., Zhang, B., Yang, X., et al. (2013). Sherlock: detecting gene-disease associations by matching patterns of expression QTL, and GWAS. Am. J. Hum. Genet. 92, 667–680. doi: 10.1016/j.ajhg.2013.03.022
Howson, J. M. M., Zhao, W., Barnes, D. R., Ho, W. K., Young, R., Paul, D. S., et al. (2017). Fifteen new risk loci for coronary artery disease highlight arterial-wall-specific mechanisms. Nat. Genet. 49:1113.
Huang da, W., Sherman, B. T., and Lempicki, R. A. (2009a). Bioinformatics enrichment tools: paths toward the comprehensive functional analysis of large gene lists. Nucleic Acids Res. 37, 1–13. doi: 10.1093/nar/gkn923
Huang da, W., Sherman, B. T., and Lempicki, R. A. (2009b). Systematic, and integrative analysis of large gene lists using DAVID bioinformatics resources. Nat. Protoc. 4, 44–57. doi: 10.1038/nprot.2008.211
Jia, P., Wang, L., Fanous, A. H., Pato, C. N., Edwards, T. L., and Zhao, Z. (2012). Network-assisted investigation of combined causal signals from genome-wide association studies in schizophrenia. PLoS Comput. Biol. 8:e1002587. doi: 10.1371/journal.pcbi.1002587
Jia, P., and Zhao, Z. (2014). Network. assisted analysis to prioritize GWAS results: principles, methods, and perspectives. Hum. Genet. 133, 125–138. doi: 10.1007/s00439-013-1377-1
Kay, A. M., Simpson, C. L., and Stewart, J. A. Jr. (2016). The role of AGE/RAGE signaling in diabetes-mediated vascular calcification. J. Diabetes Res. 2016, 6809703–6809703. doi: 10.1155/2016/6809703
Khera, A. V., and Kathiresan, S. (2017). Genetics of coronary artery disease: discovery, biology, and clinical translation. Nat. Rev. Genet. 18, 331–344. doi: 10.1038/nrg.2016.160
Klarin, D., Zhu, Q. M., Emdin, C. A., Chaffin, M., Horner, S., McMillan, B. J., et al. (2017). Genetic analysis in UK biobank links insulin resistance, and transendothelial migration pathways to coronary artery disease. Nat. Genet. 49:1392. doi: 10.1038/ng.3914
Koene, R. J., Prizment, A. E., Blaes, A., and Konety, S. H. (2016). shared risk factors in cardiovascular disease, and cancer. Circulation 133, 1104–1114. doi: 10.1161/CIRCULATIONAHA.115.020406
Kojima, Y., Volkmer, J. P., McKenna, K., Civelek, M., Lusis, A. J., Miller, C. L., et al. (2016). CD47-blocking antibodies restore phagocytosis, and prevent atherosclerosis. Nature 536, 86–90. doi: 10.1038/nature18935
LeBlanc, M., Zuber, V., Andreassen, B. K., Witoelar, A., Zeng, L., Bettella, F., et al. (2016). Identifying novel gene variants in coronary artery disease, and shared genes with several cardiovascular risk factors. Circ. Res. 118, 83–94. doi: 10.1161/CIRCRESAHA.115.306629
Letavernier, E., Zafrani, L., Perez, J., Letavernier, B., Haymann, J. P., and Baud, L. (2012). The role of calpains in myocardial remodelling, and heart failure. Cardiovasc. Res. 96, 38–45. doi: 10.1093/cvr/cvs099
Liu, W., Zhang, Y., Yu, C.-M., Ji, Q. W., Cai, M., Zhao, Y. X., et al. (2015). Current understanding of coronary artery calcification. J. Geriatr. Cardiol. 12, 668–675.
Lloyd-Jones, L. R., Holloway, A., McRae, A., Yang, J., Small, K., Zhao, J., et al. (2017). The genetic architecture of gene expression in peripheral blood. Am. J. Hum. Genet. 100, 228–237. doi: 10.1016/j.ajhg.2016.12.008
Mancuso, N., Shi, H., Goddard, P., Kichaev, G., Gusev, A., and Pasaniuc, B. (2017). Integrating gene expression with summary association statistics to identify genes associated with 30 complex traits. Am. J. Hum. Genet. 100, 473–487. doi: 10.1016/j.ajhg.2017.01.031
Masoudkabir, F., Sarrafzadegan, N., Gotay, C., Ignaszewski, A., Krahn, A. D., Davis, M. K., et al. (2017). Cardiovascular disease, and cancer: Evidence for shared disease pathways, and pharmacologic prevention. Atherosclerosis 263, 343–351. doi: 10.1016/j.atherosclerosis.2017.06.001
McPherson, R., and Tybjaerg-Hansen, A. (2016). Genetics of coronary artery disease. Circ. Res. 118, 564–578. doi: 10.1161/CIRCRESAHA.115.306566
Meier, T., Grafe, K., Senn, F., Sur, P., Stangl, G. I., Dawczynski, C., et al. (2019). Cardiovascular mortality attributable to dietary risk factors in 51 countries in the WHO European region from (1990) to 2016: a systematic analysis of the global burden of disease study. Eur. J. Epidemiol. 34, 37–55. doi: 10.1007/s10654-018-0473-x
Miao, L., Yin, R.-X., Huang, F., Yang, S., Chen, W.-X., and Wu, J.-Z. (2019). Integrated analysis of gene expression changes associated with coronary artery disease. Lipids Health Dis. 18, 92–92. doi: 10.1186/s12944-019-1032-5
Miller, C. L., Pjanic, M., Wang, T., Nguyen, T., Cohain, A., Lee, J. D., et al. (2016). Integrative functional genomics identifies regulatory mechanisms at coronary artery disease loci. Nat. Commun. 7, 12092–12092. doi: 10.1038/ncomms12092
Mishra, A., and Macgregor, S. (2015). VEGAS2: software for more flexible gene-based testing. Twin Res. Hum. Genet. 18, 86–91. doi: 10.1017/thg.2014.79
Mollsten, A., Jorsal, A., Lajer, M., Vionnet, N., and Tarnow, L. (2009). The V16A polymorphism in SOD2 is associated with increased risk of diabetic nephropathy, and cardiovascular disease in type 1 diabetes. Diabetologia 52, 2590–2593. doi: 10.1007/s00125-009-1550-1
Montojo, J., Zuberi, K., Rodriguez, H., Bader, G. D., and Morris, Q. (2014). GeneMANIA: fast gene network construction, and function prediction for Cytoscape. F1000Res 3, 153–153. doi: 10.12688/f1000research.4572.1
Musunuru, K., and Kathiresan, S. (2019). Genetics of common. Complex coronary artery disease. Cell 177, 132–145. doi: 10.1016/j.cell.2019.02.015
Nakamura, R., Takahashi, A., Yamada, T., Miyai, N., Irie, H., Kinoshita, N., et al. (2009). Erythropoietin in patients with acute coronary syndrome, and its cardioprotective action after percutaneous coronary intervention. Circ. J. 73, 1920–1926. doi: 10.1253/circj.CJ-09-0219
Nelson, C. P., Goel, A., Butterworth, A. S., Kanoni, S., Webb, T. R., Marouli, E., et al. (2017). Association analyses based on false discovery rate implicate new loci for coronary artery disease. Nat. Genet. 49, 1385–1391. doi: 10.1038/ng.3913
Nikpay, M., Goel, A., Won, H. H., Hall, L. M., Willenborg, C., Kanoni, S., et al. (2015). A comprehensive 1,. (??)Genomes-based genome-wide association meta-analysis of coronary artery disease. Nat. Genet. 47, 1121–1130. doi: 10.1038/ng.3396
Parra, V., and Rothermel, B. A. (2017). Calcineurin signaling in the heart: the importance of time, and place. J. Mol. Cell Cardiol. 103, 121–136. doi: 10.1016/j.yjmcc.2016.12.006
Pavlides, J. M., Zhu, Z., Gratten, J., McRae, A. F., Wray, N. R., and Yang, J. (2016). Predicting gene targets from integrative analyses of summary data from GWAS and eQTL studies for 28 human complex traits. Genome Med. 8, 84. doi: 10.1186/s13073-016-0338-4
Pers, T. H., Karjalainen, J. M., Chan, Y., Westra, H. J., Wood, A. R., Yang, J., et al. (2015). Biological interpretation of genome-wide association studies using predicted gene functions. Nat. Commun. 6:5890. doi: 10.1038/ncomms6890
Rossin, E. J., Lage, K., Raychaudhuri, S., Xavier, R. J., Tatar, D., Benita, Y., et al. (2011). Proteins encoded in genomic regions associated with immune-mediated disease physically interact, and suggest underlying biology. PLoS Genetics 7:e1001273. doi: 10.1371/journal.pgen.1001273
Sanchis-Gomar, F., Perez-Quilis, C., and Lippi, G. (2013). Erythropoietin receptor (EpoR) agonism is used to treat a wide range of disease. Mol. Med. 19, 62–64. doi: 10.2119/molmed.2013.00025
Schaid, D. J., Chen, W., and Larson, N. B. (2018). From genome-wide associations to candidate causal variants by statistical fine-mapping. Na.t Rev. Genet. 19, 491–504. doi: 10.1038/s41576-018-0016-z
Schunkert, H., Konig, I. R., Kathiresan, S., Reilly, M. P., Assimes, T. L., Holm, H., et al. (2011). Large-scale association analysis identifies 13 new susceptibility loci for coronary artery disease. Nat. Genet. 43, 333–338. doi: 10.1038/ng.784
Senatus, L. M., and Schmidt, A. M. (2017). The AGE-RAGE axis: implications for age-associated arterial diseases. Front. Genet. 8:187. doi: 10.3389/fgene.2017.00187
Shannon, P., Markiel, A., Ozier, O., Baliga, N. S., Wang, J. T., and Ramage, D. (2003). et al. Genome Res. 13, 2498–2504. doi: 10.1101/gr.1239303
Shim, J. E., Bang, C., Yang, S., Lee, T., Hwang, S., Kim, C. Y., et al. (2017). GWAB: a web server for the network-based boosting of human genome-wide association data. Nucleic Acids Res 45, W154–W161. doi: 10.1093/nar/gkx284
Shu, L., Blencowe, M., and Yang, X. (2018). Translating GWAS findings to novel therapeutic targets for coronary artery disease. Front. Cardiovasc. Med. 5:56. doi: 10.3389/fcvm.2018.00056
Smemo, S., Tena, J. J., Kim, K. H., Gamazon, E. R., Sakabe, N. J., Gómez-Marín, C., et al. (2014). Obesity-associated variants within FTO form long-range functional connections with IRX3. Nature 507, 371–375. doi: 10.1038/nature13138
Stitziel, N. O., Stirrups, K. E., Masca, N. G., Erdmann, J., Ferrario, P. G., König, I. R., et al. (2016). Coding variation in ANGPTL4, LPL, and SVEP1, and the risk of coronary disease. N. Engl. J. Med. 374, 1134–1144. doi: 10.1056/NEJMoa1507652
Szklarczyk, D., Morris, J. H., Cook, H., Kuhn, M., Wyder, S., Simonovic, M., et al. (2017). The STRING database in. (2017): quality-controlled protein-protein association networks, made broadly accessible. Nucleic Acids Res. 45, D362–D368. doi: 10.1093/nar/gkw937
Tasan, M., Musso, G., Hao, T., Vidal, M., MacRae, C. A., and Roth, F. P. (2015). Selecting causal genes from genome-wide association studies via functionally coherent subnetworks. Nat. Methods 12, 154–159. doi: 10.1038/nmeth.3215
Tian, C., Liu, T., Fang, S., Du, X., and Jia, C. (2012). Association of C47T polymorphism in SOD2 gene with coronary artery disease: a case-control study, and a meta-analysis. Mol. Biol. Rep. 39, 5269–5276. doi: 10.1007/s11033-011-1324-y
Ueba, H., Shiomi, M., Brines, M., Yamin, M., Kobayashi, T., Ako, J., et al. (2013). Suppression of coronary atherosclerosis by helix B surface Peptide, a. (nonerythropoietic), tissue-protective compound derived from erythropoietin. Mol. Med. 19, 195–202. doi: 10.2119/molmed.2013.00037
van der Harst, P., and Verweij, N. (2018). Identification of 64 novel genetic loci provides an expanded view on the genetic architecture of coronary artery disease. Circ. Res. 122, 433–443. doi: 10.1161/CIRCRESAHA.117.312086
Vendrov, A. E., Stevenson, M. D., Alahari, S., Pan, H., Wickline, S. A., and Madamanchi, N. R. (2017). Attenuated superoxide dismutase 2 activity induces atherosclerotic plaque instability during aging in hyperlipidemic mice. J. Am. Heart Assoc. 6: e006775. doi: 10.1161/JAHA.117.006775
Wang, Y. (2007). Mitogen-activated protein kinases in heart development, and diseases. Circulation 116, 1413–1423. doi: 10.1161/CIRCULATIONAHA.106.679589
Webb, T. R., Erdmann, J., Stirrups, K. E., Stitziel, N. O., Masca, N. G., Jansen, H., et al. (2017). Systematic evaluation of pleiotropy identifies 6 further loci associated with coronary artery disease. J. Am. Coll. Cardiol. 69, 823–836. doi: 10.1016/j.jacc.2016.11.056
Wells, A., Kopp, N., Xu, X., O’Brien, D. R., Yang, W., Nehorai, A., et al. (2015). The anatomical distribution of genetic associations. Nucleic Acids Res. 43, 10804–10820. doi: 10.1093/nar/gkv1262
Westra, H. J., Peters, M. J., Esko, T., Yaghootkar, H., Schurmann, C., Kettunen, J., et al. (2013). Systematic identification of trans eQTLs as putative drivers of known disease associations. Nat. Gen. 45, 1238–1243. doi: 10.1038/ng.2756
Zhang, Y., Qi, G., Park, J.-H., and Chatterjee, N. (2018). Estimation of complex effect-size distributions using summary-level statistics from genome-wide association studies across 32 complex traits. Nat. Genet. 50, 1318–1326. doi: 10.1038/s41588-018-0193-x
Zhao, Y., Chen, J., Freudenberg, J. M., Meng, Q., Rajpal, D. K., and Yang, X. (2016). Network-based identification, and prioritization of key regulators of coronary artery disease loci. Arteriosc. Thromb. Vasc. Bio. 36, 928–941. doi: 10.1161/ATVBAHA.115.306725
Zhou, R. H., Vendrov, A. E., Tchivilev, I., Niu, X. L., Molnar, K. C., Rojas, M., et al. (2012). Mitochondrial oxidative stress in aortic stiffening with age: the role of smooth muscle cell function. Arteriosc. Thromb.Vasc. Biol. 32, 745–755. doi: 10.1161/ATVBAHA.111.243121
Keywords: coronary artery disease, genome-wide association studies, prioritize, causal genes, expression quantitative trait locus, protein–protein interaction, network, integration analysis
Citation: Zheng Q, Ma Y, Chen S, Che Q and Chen D (2020) The Integrated Landscape of Biological Candidate Causal Genes in Coronary Artery Disease. Front. Genet. 11:320. doi: 10.3389/fgene.2020.00320
Received: 07 December 2019; Accepted: 18 March 2020;
Published: 21 April 2020.
Edited by:
Yi Zhao, Beijing University of Chinese Medicine, ChinaReviewed by:
Matteo D’Antonio, University of California, San Diego, United StatesRicha Gupta, DNAnexus, Inc., United States
Copyright © 2020 Zheng, Ma, Chen, Che and Chen. This is an open-access article distributed under the terms of the Creative Commons Attribution License (CC BY). The use, distribution or reproduction in other forums is permitted, provided the original author(s) and the copyright owner(s) are credited and that the original publication in this journal is cited, in accordance with accepted academic practice. No use, distribution or reproduction is permitted which does not comply with these terms.
*Correspondence: Dafang Chen, ZGFmYW5nY2hlbkBiam11LmVkdS5jbg==