- 1Department of Biochemistry and Molecular Biology, University of Ulsan College of Medicine, Seoul, South Korea
- 2Human Genetics, Genome Institute of Singapore, Singapore, Singapore
- 3Department of Gastroenterology, Asan Medical Center, University of Ulsan College of Medicine, Seoul, South Korea
- 4Department of Medicine, Seoul National University College of Medicine, Seoul, South Korea
Background: Expression quantitative trait loci (eQTL) datasets have extensively been used to help interpret genome-wide association study signals. Most eQTL analyses have been conducted with populations of European ancestry.
Objective: To determine the most functionally relevant genes at the Crohn’s disease (CD) loci identified in genome-wide association studies (GWAS) involving Asian populations and to find novel disease-associated genes, we conducted an eQTL analysis.
Methods: eQTL analysis was performed using whole-blood RNA-sequencing of 101 Korean patients with CD. FastQTL was used for a pair-wise genome analysis of ∼ 6.5 M SNPs and ∼ 22 K transcripts.
Results: We identified 135,164 cis-eQTL and 3,816 eGenes with a false discovery rate less than 0.05. A significant proportion of the genes identified in our study overlapped with those identified in previous studies. The significantly enriched pathways of these 3,816 eGenes included neutrophil degranulation and small molecule biosynthetic process. Integrated analysis of CD GWAS with Korean eQTL revealed two putative target genes, TNFSF15 and GPR35, at two previously reported loci, whereas TNFSF15 only with the whole blood data from the Genotype-Tissue Expression (GTEx) project, highlighting the utility of building a population-specific data set, even of modest size. The risk alleles of these genes were found to be associated with lower expression levels of TNFSF15 and GPR35, respectively. Our eQTL browser can be accessed at “http://asan.crohneqtl.com/”.
Conclusion: This resource would be useful for studies that need to employ genome-wide association analyses involving Asian populations.
Introduction
Genome-wide association studies (GWAS) have identified >200 loci that are associated with inflammatory bowel diseases (IBD) (Liu et al., 2015; de Lange et al., 2017). As GWAS variants do not necessarily identify causal genes or reveal how the variants influence traits, the effects of GWAS variants on diseases remain largely unknown. Most IBD associated variants are in the non-coding regions of the genome, suggesting that the variants may affect the trait through regulation of gene expression. Expression quantitative trait locus (eQTL) studies associate genomic and transcriptomic data sets from the same individuals to identify loci that affect mRNA expression. By linking SNPs to changes in gene expression, eQTL can be useful for annotating GWAS variants. Recently, several methods have been proposed to connect eQTL data to GWAS associations in an integrative framework often referred to as transcriptome-wide association studies (TWAS) (Gusev et al., 2016; Zhu et al., 2016). The statistical framework “eQTL and GWAS CAusal Variants Identification in Associated Regions” (eCAVIAR) estimates the probability that the same variant can be causal for both expression changes and disease status (Hormozdiari et al., 2016). Summary-data-based Mendelian Randomization (SMR) integrates GWAS with eQTL data by testing if the eQTL occurs to be associated with the disease (Zhu et al., 2016). These methods allow determination of the most functionally relevant genes at the loci and identification of novel trait-associated loci via imputation of genotype correlated gene expression levels into GWAS datasets (Gusev et al., 2016; Zhu et al., 2016). Using such methods, recent studies have suggested that a large number of candidate common trait susceptibility genes identified as eQTL are not the nearest genes to the GWAS lead SNPs, suggesting that causal genes might be distinct from the nearest genes (Hormozdiari et al., 2016). Regulatory elements can act over a long distance and in a cell-type specific manner, making the identification of the causal genes for a given pathologic condition and their roles extremely difficult.
Recently, eQTL have been identified using a broad collection of normal human tissues from diverse populations, increasing our understanding of regulation of gene expression. However, non-European ancestries are under-represented in the current eQTL databases. Comparisons of patterns of gene expression between European and African populations have revealed that more distantly related populations tend to have a greater number of differentially expressed genes, which often result from expression of different genes rather than differences in the expression levels of the same genes (Lappalainen et al., 2013). Studies on the genetic architecture of gene expression traits across three different populations found that genetic correlation of gene expression depends on shared ancestry proportions, emphasizing that a training set with ancestry similar to the test set is better at predicting gene expression in the test populations (Mogil et al., 2018).
The most recent GWAS on Asian patients with Crohn’s disease (CD) has reported 29 susceptibility loci (Yang et al., 2016); however, integration of disease association and eQTL analyses for Asian CD has never been conducted before. To determine the most functionally relevant genes at the CD loci identified in Asian GWAS and to find novel CD-associated loci, we built a Korean-CD-specific eQTL data set from whole blood of CD patients. We performed eQTL analysis using RNA-seq of whole blood in a cohort of 101 Korean CD patients and tried to integrate with the Korean CD GWAS to identify candidate causal variants at two known loci. We found not only substantial overlap between our eQTL and the blood eQTL database on a very large cohort but also additional eQTL unique to our study population. As the eQTL database was constructed using CD patients and not healthy individuals, Korean CD eQTL datasets might provide a valuable resource for link between genetic variation and gene expression and regulation not only in Asians, but also in Caucasians if new eQTL in patients become only evident when the gene is overexpressed as a result of modified inflammatory status.
Materials and Methods
Study Subjects
A total of 101 CD patients were recruited from the IBD Clinic of Asan Medical Center. All these study subjects provided written informed consent. The clinical characteristics of the 101 CD patients are shown in Supplementary Table S1. These patients had previously been genotyped as part of a previous GWAS (Yang et al., 2016), and their genotype data were included in the eQTL analysis of the study presented here.
Genotype Quality Control and Imputation
Quality control (QC) was performed as previously described GWAS (Yang et al., 2016). Briefly, individuals with a call rate ≤96% were removed. We excluded all the single nucleotide polymorphisms (SNPs) with missing rates >0.02, minor allele frequency <0.01, and Hardy-Weinberg equilibrium (HWE, P < 1.0 × 10–5). After the QC, we had 101 individual samples and 524,635 genotyped SNPs available for the downstream eQTL analyses.
To increase the power for eQTL discovery, the SNPs that passed QC were further subjected to haplotype phasing and genotype imputation using SHAPEIT (Delaneau et al., 2011) and IMPUTE2 (Howie et al., 2009), respectively, based on the 1000 Genome multi-ethnic reference panel (Feb 2012, IMPUTE v2). The imputed genotypes were subjected to a second round quality control with the imputed variants (info >0.8), missing rates <0.02, MAF > 0.01, and HWE P > 1.0 × 10–5 using SNPTEST (Marchini et al., 2007). In total, 6,451,113 SNPs including 524,635 genotyped and 5,926,478 imputed SNPs were retained.
Total RNA Extraction From the Peripheral Blood, and Library Preparation and Sequencing
Total RNA was isolated from the peripheral blood using PAXgene Blood RNA system (PreAnalytiX, QIAGEN, Germany). Whole blood was taken and immediately store in a PAXgene Blood RNA tube at room temperature for >4 h. The total RNA was extracted using the PAXgene Blood RNA kit, following the manufacturer’s instructions. RNA quality and quantity were checked using a 2100 Bioanalyzer (Agilent Technologies, CA, United States) and the samples with an RNA integrity number ≥7 were deep-sequenced. Sequencing libraries were prepared with the Illumina TruSeq Stranded Total RNA Library Prep Kit with Ribo-Zero Globin (Illumina, CA, United States) and paired-end RNA sequencing of 101 bp reads was performed using Illumina HiSeq 2500 platform.
Quality Validation and Alignment
Paired-end RNA sequencing of 2 × 101 bp reads was performed using Illumina HiSeq 2500 platform. We evaluated the number and quality of the total reads, GC percent and adapters in the raw fastq files using FastQC v0.11.71. Every one of the 101 samples had >93 million raw reads (117 million reads on average) and passed the read quality check (average Phred quality score >36), and GC percent check (46-56%). The adapter sequences and reads with low quality were excluded using cutadapt (Martin, 2011) applying the quality Phred score cut-off <33 and read length cut-off <20 bp. We performed alignment for the trimmed reads using STAR (default settings) (Dobin et al., 2013) and GRCh37 reference genome in GENCODE release 192 (Frankish et al., 2019). For confirmation of unique mapping rate and ribosomal RNA rate, we used RNA-SeQC (DeLuca et al., 2012). As 15 CD patients showed high ribosomal RNA ratio (>40%) in sample QC, the RNA sequencing of these 15 samples were repeated and aligned to the reference genome. We confirmed high unique mapping rate (94-99%) in all the 101 samples. After alignment, we used RNA-SeQC to estimate the transcript abundance, expected read counts, and transcripts per million reads (TPM) for each gene by selecting the uniquely mapped reads with a mapping quality >255, and ≤6 mismatched bases to the reference genome.
eQTL Analysis
After removing low-expressed genes from the mRNA expression data estimated by RNA-SeQC, 21,718 genes with TPM > 0.1 and the number of reads >6 in ≥20% of the 101 CD samples were included. With the read count data of the 21,718 genes from the 101 samples, we generated a multi-dimensional scaling (MDS) plot using an R package: edgeR3 (Robinson et al., 2010) to confirm absence of batch effect in the RNA sequencing data from 15 samples that had been re-sequenced due to high rRNA contamination (Supplementary Figure S1). We used a trimmed mean of M-values (TMM) for normalization of gene expression values considering total mRNA read counts of each sample using edgeR (Robinson et al., 2010). The genomic input data of the 101 CD patients included a total of 6,451,113 SNPs from GWAS. The cis window was defined as the 1-Mb region up- and downstream of the transcription start site (TSS) (±1 Mb). Dosage was used for the association analysis for imputed SNPs. Nominal P values were calculated for each SNP-gene pair with FastQTL (Ongen et al., 2016) using the linear regression model with 27 covariates including 15 PEER (Stegle et al., 2012) factors, 3 PCs calculated using GWAS dataset of 101 CD samples, repeat or not, gender, age, age of diagnosis, follow-up year, family history, smoking or not, Montreal classification, and disease behaviors. Significance of the top associated variant per gene was estimated by adaptive permutation with the setting “–permute 1000 10000” in FastQTL (Ongen et al., 2016). The beta distribution-adjusted empirical P values were used to calculate the q-values and FDR thresholds of each gene using R package: q value4. The FDR threshold of <0.05 was applied to identify all the significant cis-eQTL.
Enrichment Analysis on eGene Set
To annotate the biological mechanisms related to the eGenes in the eQTL analysis of the 101 CD patients, we performed the Gene Ontology (GO) (Ashburner et al., 2000; The Gene Ontology Consortium, 2017) enrichment analysis in the web application, AmiGO25 (Carbon et al., 2009) using 3,816 eGenes with FDR < 0.05 in the cis-eQTL analysis. By the default setting (GO aspect: biological process, Species: Homo sapiens), the result page showed the over- or underrepresented GO terms with significant P values.
Comparison of the Direction of Allelic Effects Across the Existing Cis-eQTL Databases
We compared the allelic directions of SNP-gene associations shared among the Korean CD cis-eQTL, the existing whole blood cis-eQTL databases of Japanese (105 healthy individuals)6 (Ishigaki et al., 2017), and GTEx V7 (369 individuals)7 (Aguet et al., 2017). The number of cis-eQTL in the Korean CD, Japanese, and GTEx datasets was 135,164, 335,813 and 1,052,542, respectively. Using only significant cis-eQTLs with q value ≤ 0.05 in each dataset, we compared the slope of the overlapping SNP-gene associations between three pairs of Korean CD-Japanese, Korean CD-GTEx, and GTEx-Japanese datasets.
Colocalization Analysis Between CD GWAS and Whole Blood eQTL
For colocalization analysis between CD GWAS of 899 cases and 3,805 controls (Yang et al., 2016) and whole blood eQTL data of 101 CD patients, we used eCAVIAR (Hormozdiari et al., 2016) to estimate the probability of eQTL and GWAS sharing the same causal variants. The association test of CD GWAS was performed by frequentist association test of SNPTEST (Marchini et al., 2007) using the additive model. The eCAVIAR calculated co-localization posterior probability (CLPP) score, indicating the level of colocalization, using each Z score of eQTL and GWAS data, as well as linkage disequilibrium (LD) information. We used the LD reference of East Asians (JPT + CHB) in the 1000 genomes8 (May 2015 release). We also tried Japanese eQTL and GTEx eQTL datasets for colocalization analysis with CD GWAS. For GTEx, LD reference of Caucasian (CEU) in the 1000 genomes was used since eCAVIAR allows different LD structures for eQTL and GWAS datasets. We selected 100 SNPs upstream and downstream of the reported lead SNPs in the 29 established loci (excluding the major histocompatibility complex (MHC) region, 25 ∼ 34 Mb) in Koreans (Yang et al., 2016; Lee et al., 2018) to calculate the CLPP score. We used the default of two causal variants for locus and eCAVIAR method’s recommended significant cut-off, CLPP > 0.01, and 0.95 for total credible set posterior probability.
SMR and HEIDI Test
We performed a transcriptome-wide association study (TWAS) integrating CD GWAS of 4,704 individuals with the expression data from the whole blood tissue of the 101 CD patients using SMR software9 (Zhu et al., 2016). We used associations from SNPTEST (Marchini et al., 2007) result of the previously reported GWAS including 899 CD cases and 3,805 controls, and the whole blood cis-eQTL of the 101 CD patients as the input data of SMR and heterogeneity in dependent instruments (HEIDI) test. LD was estimated using the individual genotype data of East Asians (JPT + CHB) in the 1000 genomes (see text “footnote 8”, May 2015 release) after excluding the MHC region (25 ∼ 34 Mb) due to LD complexity. Excluding all the genes with PHEIDI < 0.05 in the HEIDI test for detection of heterogeneity, we selected the target genes with a shared causal variant between the GWAS and cis-eQTL data applying a threshold of PSMR < 2.3 × 10–6 (0.05/21,583 genes). We also performed a TWAS integrating the CD GWAS of 4,704 individuals with Japanese eQTL using SMR.
Results
Cis-eQTL
To identify the cis-eQTL variants within 1 Mb on either side of the TSS of each gene, cis-eQTL analysis was performed using the GWAS and RNA sequencing data from the peripheral blood of 101 Korean CD patients. Applying the threshold of FDR < 0.05, we found 135,164 cis-eQTL, 104,900 eSNPs, and 3,816 eGenes which had at least one cis-eQTL. The distance from an eSNP to the TSS of the target gene was ≤500 kb in 95.7% (129,333 cis-eQTL) and ≤250 kb in 86.9% (117,433 cis-eQTL) of the total 135,164 cis-eQTL, and locations of eSNPs were more likely to be near the TSS of their target genes (Supplementary Figure S2). Of the total 104,900 eSNPs, the number of the target genes was one for 83,848 eSNPs (79.9%), two for 15,508 eSNPs (14.8%), and over three for 5,544 eSNPs (5.3%) (Table 1). The proportion of the eSNPs to the total SNPs in each chromosome was 0.6-3.5% (total = 1.6%), and the ratio of the eGenes to the total genes ranged from 14.4 to 23.0% (total = 17.6%). The gene biotypes of 3,816 eGenes were composed of 2,700 protein coding genes (70.8%), 418 pseudogenes (11.0%), 272 antisense RNAs (7.1%), 270 long intergenic non-coding RNAs (7.1%), 44 sense intronic non-coding RNAs (1.2%), and 43 processed transcripts (1.1%), and 69 other gene biotypes (1.8%) (Supplementary Figure S3).
To annotate the biological processes significantly related to the 3,816 eGenes, we performed GO enrichment analysis using web-based AmiGO2 (see text “footnote 5”) (Carbon et al., 2009). Of those, 1,051 eGenes were excluded from the analysis mainly due to being non-coding genes or being absent in the reference genes of the GO dataset. A total of 2,765 eGenes were used as the input gene list. Of the significantly shared GO terms with the Bonferroni-corrected P value < 0.05, granulocyte activation (GO:0036230) and neutrophil activation (GO:0042119) showed the top two highest fold enrichment values, respectively (Supplementary Table S2).
Comparison of Direction of Allelic Effects Across Existing Cis-eQTL Databases
Using the Korean CD eQTL data and two existing cis-eQTL datasets derived from whole blood samples, including the Japanese eQTL (Ishigaki et al., 2017) and GTEx (Aguet et al., 2017), we compared the direction of allelic effects of all the common SNP-gene pairs. Of the 135,164 eQTL in the Korean CD, 335,813 in the Japanese, and 1,052,542 in the GTEx datasets, the number of shared significant cis-eQTL (a threshold of q value ≤ 0.05) in each pair was 50,848 between the Korean CD and Japanese eQTL, 58,197 between the Korean CD and GTEx, and 120,158 between the GTEx and Japanese eQTL datasets (Figure 1). In total, 96.5–98.7% of shared eGenes in each pair of the three cis-eQTL datasets showed the same direction of allelic effects. The proportion of shared eGenes with the opposite direction of allelic effects was 16 of 1,201 (1.3%) shared eGenes in the pair of Korean CD-Japanese, 44 of 1,873 (2.3%) shared eGenes in the pair of GTEx-Japanese cis-eQTL datasets and 56 of 1,581 (3.5%) shared eGenes in the pair of Korean CD-GTEx (Supplementary Table S3). Between the 16 and 56 eGenes with the opposite direction of allelic effects in the pair of Korean CD-Japanese or -GTEx, 9 eGenes overlapped. Of the SNPs with top eQTL P value of the 9 eGenes, only rs2859102 in TAP2 showed significant association with CD in Koreans (P = 8.81 × 10–11).
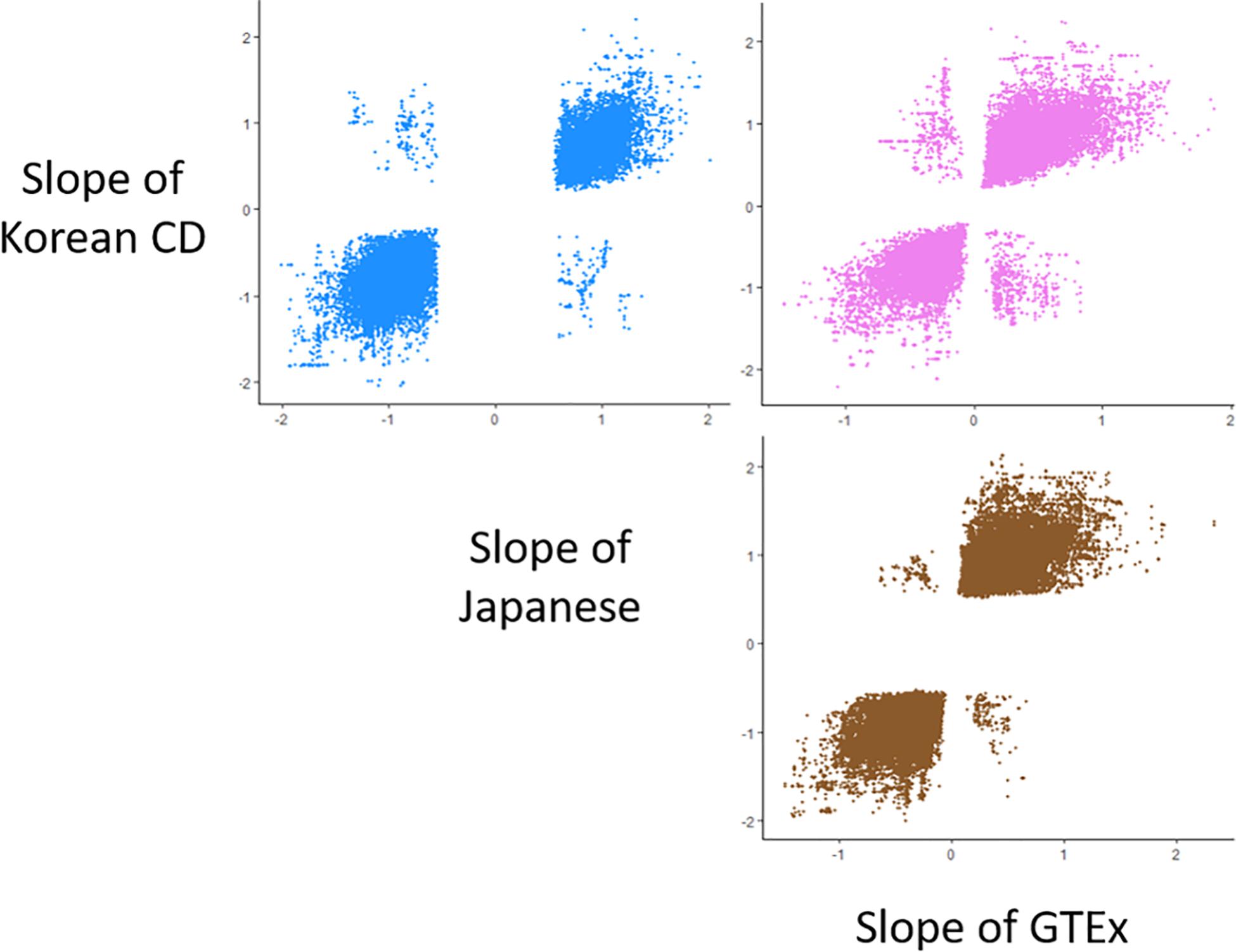
Figure 1. Scatter plots for comparison of the direction of allelic effects among the whole blood cis-eQTL data from the Korean CD, Japanese samples, and GTEx project. Each point on the scatter plots represents the allelic effect of a SNP to a gene expression. The scatter plots included 50,848 cis-eQTLs of 1,201 eGenes between the Korean CD and Japanese samples, 58,197 cis-eQTLs of 1,581 eGenes between the Korean CD and GTEx, and 120,158 cis-eQTLs of 1,873 eGenes between the GTEx and Japanese samples.
Identification of CD Susceptibility Genes From GWAS Loci Using Whole Blood eQTL
We also performed colocalization analyses of the CD GWAS and whole blood eQTL data of the Korean CD, Japanese, and GTEx datasets using eCAVIAR (Hormozdiari et al., 2016). eCAVIAR computes the CLPP score using Z statistics of GWAS and cis-eQTL data of 100 SNPs upstream and downstream of the reported lead SNP in the 29 established loci in Koreans (Yang et al., 2016; Lee et al., 2018). We identified 122 eGenes within 1 Mb window from the lead SNP in the 29 established loci as target genes for cis-eQTL data of the Korean CD dataset. Applying thresholds of CLPP > 0.01 and total credible set posterior probability >0.95, two loci including TNFSF15 (TNF Superfamily Member 15) at 9q32 and GPR35 (G-protein coupled receptor 35) at 2q37 were identified in colocalization analysis between the GWAS and cis-eQTL data of the Korean CD dataset (Table 2 and Supplementary Table S4). In the TNFSF15 locus, a previously reported SNP (rs6478109) (Yang et al., 2016) at 360 bp upstream of TNFSF15 (Figure 2A) showed a significant P value in both GWAS (3.2 × 10–45) and cis-eQTL (3.6 × 10–10) with a CLPP score of 0.23. The other causal SNP, rs7848647 at 640 bp upstream of TNFSF15 is in complete LD (r2 = 1) with rs6478109 in East Asians. Both risk allele G of rs6478109 and risk allele C of rs7848647 in CD GWAS were related to lower expression of TNFSF15 than each protective allele A or T in the whole blood tissue (Figure 2B). A reported SNP in the GPR35 locus, rs3749172 (p.Ser294Arg) (Liu et al., 2015; Yang et al., 2016) is located in exon 6 and 25,401 bp downstream of the TSS of GPR35 (Figure 2C). The CLPP score (0.17) of rs3749172 showed that rs3749172 was a shared causal variant in CD GWAS (P value = 6.7 × 10–8) and whole blood cis-eQTL (P value = 4.4 × 10–6) (Table 2). The other causal SNP, rs2953153 (CLPP = 0.20) in intron 5 and 21,164 bp downstream of the TSS of GPR35, is in high LD (r2 = 0.98) with rs3749172. Expression of GPR35 in whole blood was down-regulated at risk allele A of rs3749172 and risk allele G of rs2953153, but up-regulated at protective allele C and A, respectively (Figure 2D).
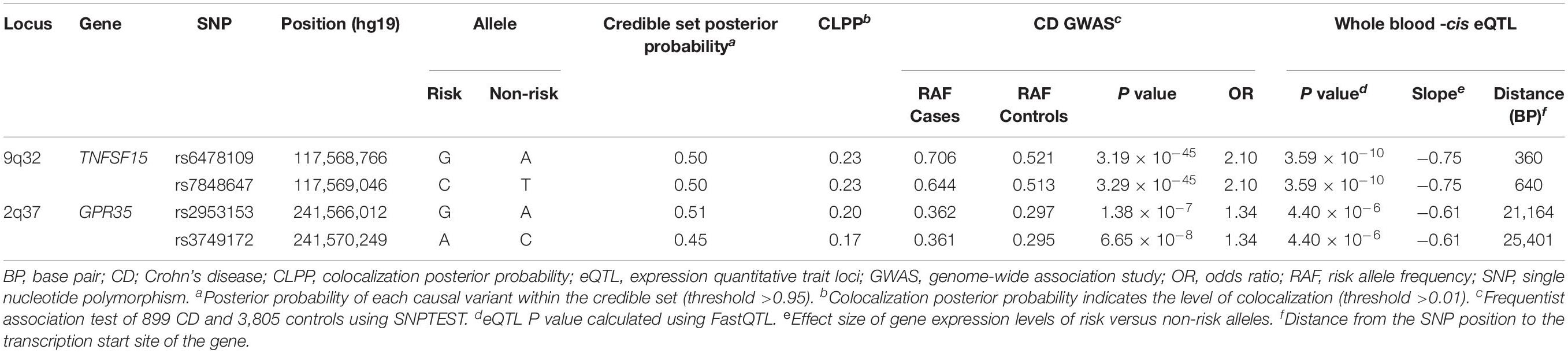
Table 2. Colocalization analysis between CD GWAS and whole blood eQTL using eCAVIAR in TNFSF15 and GPR35 locus.
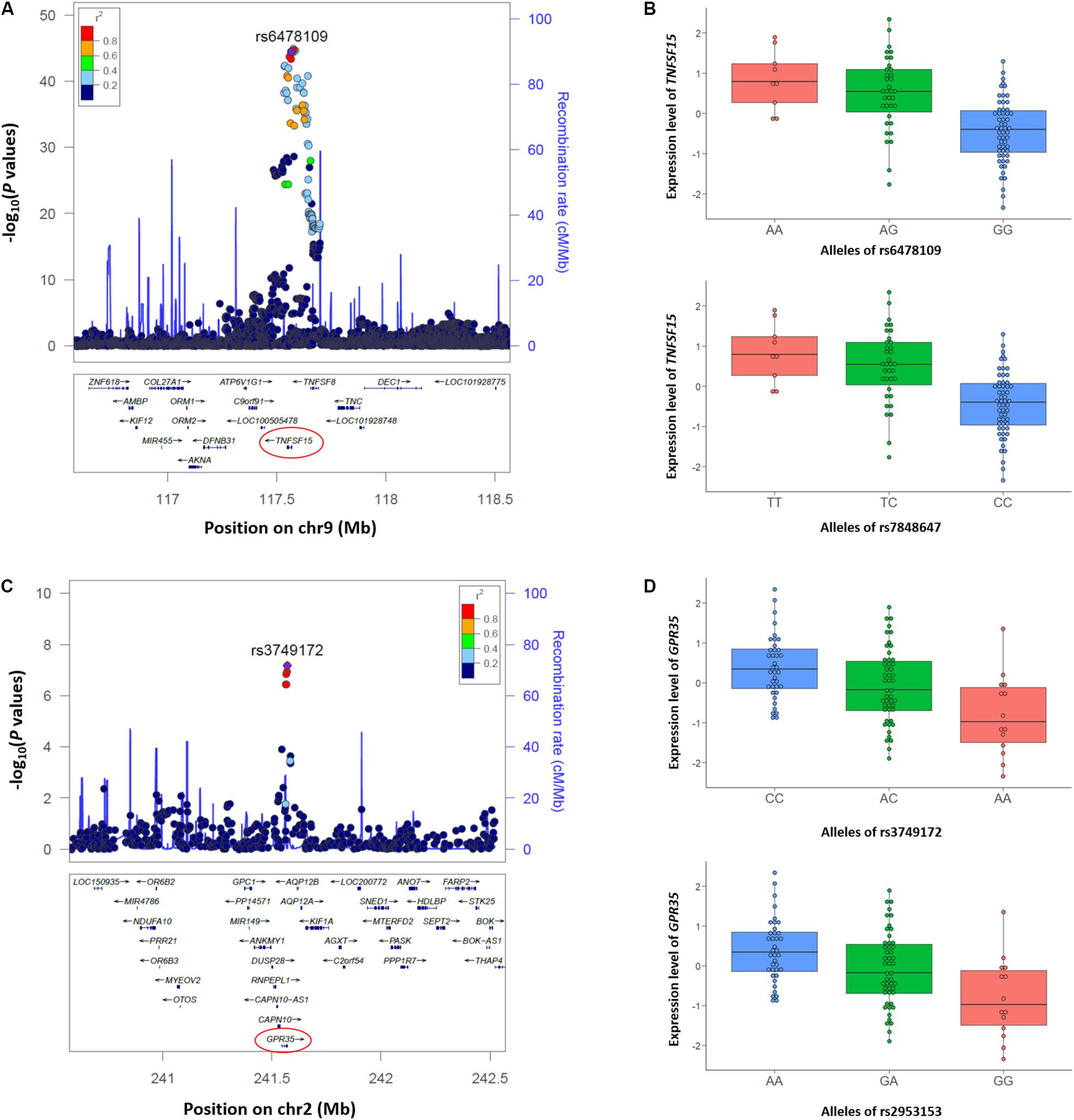
Figure 2. Two loci including TNFSF15 and GPR35 identified with colocalization analysis between CD GWAS and cis-eQTL data from the Korean CD samples. (A,C) Regional association plots of the (A) TNFSF15 locus at 9q32 using 5,956 SNPs, and (C) GPR35 locus at 2q37 using 497 SNPs in ±1 Mb from rs6478109 and rs3749172 are plotted according to their chromosomal positions (hg19) with –log10 P values from the Korean CD GWAS. Both rs6478109 and rs3749172 are shown as purple circles in each plot. LD (r2) indicated with colors was calculated using East Asian population data (JPT + CHB) for the 1000 Genomes (http://www.internationalgenome.org). Regional association plots were generated using a web browser, LocusZoom (http://locuszoom.org/genform.php?type=yourdata). (B,D) Box plots of the (B) TNFSF15 expression level according to alleles of rs6478109 and rs7848647, and (D) GRP35 expression level according to alleles of rs3749172 and rs2953153. Small circles in the box plot indicate the normalized expression level using trimmed mean of M-values (TMM).
In the colocalization analysis of the 118 target genes between the CD GWAS and Japanese cis-eQTL data, we only identified a TNFSF15 locus with two causal SNPs, rs6478109 and rs7848647 (CLPP score = 0.13, total credible set posterior probability = 1), above the threshold (Supplementary Table S5). In the GPR35 locus, rs2953153 and rs3749172 had significant CLPP scores of 0.10 and 0.39, respectively; however, a total of credible set posterior probabilities of rs2953153 and rs3749172 were below the threshold of 0.89. The colocalization analysis of the 348 target genes between the CD GWAS and GTEx identified rs6478109 and rs7848647 at the TNFSF15 locus as the most likely causal SNP, distinct from the European lead SNP rs10114470 (r2 = 0.77 with rs6478109) in the largest CD GWAS (de Lange et al., 2017; Supplementary Table S6). These two causal SNPs showed significant CLPP scores (rs6478109 = 0.06 and rs7848647 = 0.09) and a 100% credible set posterior probability. Both rs2953153 and rs3749172 in the GPR35 locus had non-significant CLPP scores < 0.01 and cis-eQTL P values with FDR > 0.05 for GPR35 expression. European lead SNP in the largest CD GWAS was rs34236350 (de Lange et al., 2017), in moderate LD with the Asian lead SNP rs3749172 (r2 = 0.34) in Europeans. The colocalization analysis of the GPR35 locus between the CD GWAS of European ancestry (de Lange et al., 2017) and GTEx failed to identify shared causal SNPs.
Transcriptome-Wide Association Study (TWAS) Using Whole Blood eQTL Data
Using summary statistics of the CD GWAS of 4,704 individuals and whole blood cis-eQTL data of the 101 CD patients, TWAS was performed using SMR (Zhu et al., 2016) to discover genes whose expression levels were related to the causal variants of the CD GWAS. Of the top 21 CD TWAS genes at 18 loci (PSMR < 0.01 and PHEIDI > 0.05), 5 TWAS genes were at four previously known CD GWAS loci (Supplementary Table S7). Applying the threshold of PHEIDI > 0.05 and PSMR < 2.31 × 10–6 (0.05/21,583 genes), SMR test identified only TNFSF15 at 9q32 with PSMR = 8.07 × 10–9 and PHEIDI = 0.13 (Supplementary Figure S4). A lead SNP of TWAS in the TNFSF15 locus was rs6478108 in intron 1 of TNFSF15 with P value = 1.6 × 10–44 in the CD GWAS, and the most significant P value in cis-eQTL (2.47 × 10–10) for TNFSF15 expression (Supplementary Table S7). rs6478108 is in high LD (r2 = 0.98) with rs6478109 discovered in the colocalization analysis, and risk allele T of rs6478108 in the CD GWAS was associated with lower expression of TNFSF15 than allele C in the whole blood of the CD patients. When we performed TWAS using Japanese eQTL, SMR test identified only TNFSF15 at 9q32 with PSMR = 5.95 × 10–10 and PHEIDI = 0.2 (Supplementary Table S8). Between the top 27 genes at 22 loci and 21 genes at 18 loci (PSMR < 0.01 and PHEIDI > 0.05) identified using the Japanese eQTL and Korean CD eQTL, respectively, 10 loci (11 genes) overlapped (Supplementary Tables 7, 8).
Discussion
In this study, we established a whole blood eQTL dataset from Korean patients with CD. Our dataset identified eQTL unique to our study population and also confirmed the eQTL signals previously identified in other populations. Using this dataset, we further identified putative target genes at two previously reported loci. We also tried to identify CD-associated genes by TWAS.
Although the eQTL signals identified in other populations were consistent with that identified in our samples, and 96.5-98.7% of the shared eGenes in each pair of three cis-eQTL datasets showed the same direction of allelic effects, we were able to colocalize the GPR35 locus using only the Korean CD or Japanese eQTL, but not GTEx whole blood. Colocalization analysis with the Korean CD or Japanese eQTL identified the same SNPs in the TNFSF15 and GPR35 loci, although the GPR35 locus was less significant in the Japanese eQTL, whereas colocalization analysis of the Korean CD GWAS and GTEx whole blood identified TNFSF15 only. Of note, TNFSF15 risk allele was found to be associated with decreased expression in the whole blood of Koreans, consistent with a recent finding involving a European population (Richard et al., 2018). The top signals of Korean and European CD GWAS at the GPR35 locus were rs3749172 and rs34236350, respectively, in moderate LD (r2 = 0.34) in Caucasians while in high LD in Asians. Rs34236350 of European CD GWAS signal was eQTL for GPR35 in GTEx sigmoid colon tissue only with up-regulation at risk allele, which is in the opposite direction relative to the whole blood eQTL of Korean and Japanese populations. Due to non-significant expression of GPR35 in GTEx whole blood (FDR > 0.05), colocalization using GTEx did not identify GPR35, indicating that population-specific eQTL effects exist. Therefore, our data highlight the utility of building a population-specific data set, even of modest size.
We found that the most significantly enriched GO terms of the 3,816 eGenes was granulocyte activation, especially neutrophil degranulation. The role of neutrophils in the pathogenesis of CD has been much better described in a theory that the common predisposition to CD is a failure of the inflammatory response to tissue damage and innate immunity (Segal, 2019). Failure of neutrophil migration to the inflammatory site is one of the mechanisms involved in granulomatous inflammation, characteristic of CD, which leads to an intense adaptive immune response and the tissues become infiltrated with large number of T cells. These cells as well as macrophages will react by producing cytokines that cause local inflammation and systemic symptoms. The GO term of the two genes identified through colocalization analysis, TNFSF15 and GPR35, was cellular process (GO:0009987) (Supplementary Table S2). GPR35, the receptor of the mucosal chemokine CXCL17 (Maravillas-Montero et al., 2015), is expressed in a subset of macrophages that are recruited to mucosal tissues by CXCL17 (Hernández-Ruiz and Zlotnik, 2017). Given the importance of macrophages in inflammation and the strong expression of both CXCL17 and GPR35 in mucosal tissues, down-regulated at CD risk allele of GPR35 can cause the failure of recruitment of myeloid cells to the site of inflammation. TNFSF15, a proinflammatory cytokine (Jin et al., 2013), can stimulate CSF2 (Colony Stimulating Factor 2) expression which can promote proliferation and maturation of granulocytes (Bhattacharya et al., 2015). Given TNFSF15 risk allele was associated with decreased expression, which can result in decrease in neutrophil activation level.
We recognize several limitations of our study. First, because of our small sample size, we had limited statistical power to detect rare or low impact eQTL, and thus we may have underestimated the number of genes overlapping with those reported in other studies. Additionally, the rather limited GWAS sample size did not allow our TWAS to identify novel candidate genes above the threshold. Second, we assessed gene expression in the whole blood of CD patient, whereas Japanese and GTEx whole blood eQTL were from healthy individuals. The Korean CD eQTL colocalized with the CD GWAS variants at two known loci, but at only one known locus with the Japanese eQTL. TWAS using the Korean CD and Japanese eQTL identified 21 and 27 genes at 18 and 22 loci (PSMR < 0.01 and PHEIDI > 0.05), respectively. TWAS using both eQTLs identified TNFSF15 only above the threshold; however, there were differences in the additional genes identified: TWAS using the Korean CD eQTL identified GPR35, whereas TWAS using the Japanese eQTL identified RNASET2. These differences might be due the differences in gene expression between the CD patient and control blood samples and/or population differences between Korean and Japanese. Although whole blood gene expression of the CD patients and healthy individuals show a high degree of similarity, we cannot exclude the possibility that new eQTL in patients become only evident when the gene is over-expressed as a result of modified inflammatory status. Third, we assessed gene expression in whole blood, which contains a heterogeneous population of cells and cannot be considered to be disease-relevant context, contributing to the low yield of causal genes identified for CD using the GWAS-eQTL integration approach. Although whole blood eQTL have been reported to be enriched with autoimmune disorder – associated SNPs (GTEx Consortium, 2015), whole blood consists of multiple distinct cell types with specific gene regulatory profiles as shown by eQTL of isolated different blood cell types (Fairfax et al., 2012). Previous GWAS have highlighted the importance of T cells in CD pathology. A recent study of single-cell RNA sequencing of blood and ileal T cells from patients with CD has shown that quiescent T cells dominate the peripheral blood (PBL) whereas cytotoxic T lymphocytes and T-helper 17 (Th 17) cells dominate intraepithelial T lymphocytes (IELs) and lamina propria T lymphocytes (LPLs), respectively (Uniken Venema et al., 2019). The authors have also noted that IELs and LPLs express significantly more CD-risk genes, whereas PBLs are not enriched for CD-risk gene expression, emphasizing importance of eQTL of disease-relevant cells.
Conclusion
In conclusion, we made the results of our eQTL study involving Korean CD patients available. Our study confirmed the eQTL signals previously identified in other populations, while also detecting additional eQTL unique to our study population. Considering that data from non-European ancestries are limited, our dataset will be useful in furthering our understanding of pathomechanisms of CD. Our results contribute to the understanding of gene expression regulation in Koreans, and also complement existing eQTL databases in other ethnic groups. eQTL loci identified in the current study will help prioritize follow-up of findings from other GWAS of complex diseases.
Data Availability Statement
The RNA sequencing datasets generated for this study can be found in the Gene Expression Omnibus (GEO) database GSE143507. Our eQTL browser can be accessed at “http://asan.crohneqtl.com/”.
Ethics Statement
This study was approved by the Institutional Review Board of the Asan Medical Center. All samples were obtained with written informed consent under Institutional Review Board-approved protocols.
Author Contributions
KS obtained financial support and conceived the study. S-KY, BY, SP, and H-SL recruited the study subjects and participated in the diagnostic evaluation. JB and JM performed the experiments. SJ and WL analyzed the data. KS, JL, and BH supervised the data analysis and interpretation. SJ and KS drafted the manuscript. WL, JL, and BH revised the manuscript.
Funding
This work was supported by a Mid-Career Researcher Program grant from the National Research Foundation of Korea to KS (2017R1A2A1A05001119 and 2020R1A2C2003275), funded by the Ministry of Science, Information & Communication Technology, and Future Planning of the South Korea. JL was supported by the Agency for Science, Technology and Research (A∗STAR), Singapore. This work was also supported by the PLSI supercomputing resources of the Korea Institute of Science and Technology Information.
Conflict of Interest
BY: Grant/research support from CELLTRION, Inc.; Lecture fees from Abbvie Korea, CELLTRION, Inc., Janssen Korea, Shire Korea, Takeda Korea, and IQVIA; Consultant fees from Abbvie Korea, Ferring Korea, Janssen Korea, Kangstem Biotech, Kuhnil Pharm., Shire Korea, Takeda Korea, IQVIA, Cornerstones Health, and Robarts Clinical Trials Inc. S-KY: Grant/research support from Janssen Korea Ltd.
The remaining authors declare that the research was conducted in the absence of any commercial or financial relationships that could be construed as a potential conflict of interest.
Acknowledgments
The authors would like to thank all participating patients and healthy donors who provided the DNA, RNA, and clinical information necessary for this study.
Supplementary Material
The Supplementary Material for this article can be found online at: https://www.frontiersin.org/articles/10.3389/fgene.2020.00486/full#supplementary-material
Footnotes
- ^ https://www.bioinformatics.babraham.ac.uk/projects/fastqc/
- ^ https://www.gencodegenes.org/human/release_19.html
- ^ http://bioconductor.org/packages/release/bioc/html/edgeR.html
- ^ https://github.com/StoreyLab/qvalue
- ^ http://amigo.geneontology.org/amigo
- ^ 1https://humandbs.biosciencedbc.jp/en/hum0099-v1#hum0099.v1.eqtl.v1
- ^ https://gtexportal.org/home/datasets
- ^ http://www.1000genomes.org
- ^ http://cnsgenomics.com/software/smr/
References
Aguet, F., Brown, A. A., Castel, S. E., Davis, J. R., He, Y., Jo, B., et al. (2017). Genetic effects on gene expression across human tissues. Nature 550, 204–213.
Ashburner, M., Ball, C. A., Blake, J. A., Botstein, D., Butler, H., Cherry, J. M., et al. (2000). Gene ontology: tool for the unification of biology. Nat. Genet. 25, 25–29.
Bhattacharya, P., Thiruppathi, M., Elshabrawy, H. A., Alharshawi, K., Kumar, P., and Prabhakar, B. S. (2015). GM-CSF: an immune modulatory cytokine that can suppress autoimmunity. Cytokine 75, 261–271.
Carbon, S., Ireland, A., Mungall, C. J., Shu, S., Marshall, B., Lewis, S., et al. (2009). AmiGO: online access to ontology and annotation data. Bioinformatics 25, 288–289.
de Lange, K. M., Moutsianas, L., Lee, J. C., Lamb, C. A., Luo, Y., Kennedy, N. A., et al. (2017). Genome-wide association study implicates immune activation of multiple integrin genes in inflammatory bowel disease. Nat. Genet. 49, 256–261.
Delaneau, O., Marchini, J., and Zagury, J. F. (2011). A linear complexity phasing method for thousands of genomes. Nat. Methods 9, 179–181.
DeLuca, D. S., Levin, J. Z., Sivachenko, A., Fennell, T., Nazaire, M., Williams, C., et al. (2012). RNA-SeQC: RNA-seq metrics for quality control and process optimization. Bioinformatics 28, 1530–1532.
Dobin, A., Davis, C. A., Schlesinger, F., Drenkow, J., Zaleski, C., Jha, S., et al. (2013). STAR: ultrafast universal RNA-seq aligner. Bioinformatics 29, 15–21.
Fairfax, B. P., Makino, S., Radhakrishnan, J., Plant, K., Leslie, S., Dilthey, A., et al. (2012). Genetics of gene expression in primary immune cells identifies cell-type specific master regulators and roles of HLA alleles. Nat. Genet. 44, 502–510.
Frankish, A., Diekhans, M., Ferreira, A. M., Johnson, R., Jungreis, I., Loveland, J., et al. (2019). GENCODE reference annotation for the human and mouse genomes. Nucleic Acids Res. 47, D766–D773.
GTEx Consortium (2015). Human genomics. the genotype-tissue expression (GTEx) pilot analysis: multitissue gene regulation in humans. Science 348, 648–660.
Gusev, A., Ko, A., Shi, H., Bhatia, G., Chung, W., Penninx, B. W., et al. (2016). Integrative approaches for large-scale transcriptome-wide association studies. Nat. Genet. 48, 245–252.
Hernández-Ruiz, M., and Zlotnik, A. (2017). Mucosal chemokines. J. Interferon Cytokine Res. 37, 62–70.
Hormozdiari, F., van de Bunt, M., Segrè, A. V., Li, X., Joo, J. W. J., Bilow, M., et al. (2016). Colocalization of GWAS and eQTL signals detects target genes. Am. J. Hum. Genet. 99, 1245–1260.
Howie, B. N., Donnelly, P., and Marchini, J. (2009). A flexible and accurate genotype imputation method for the next generation of genome-wide association studies. PLoS Genet. 5:e1000529. doi: 10.1371/journal.pgen.1000529
Ishigaki, K., Kochi, Y., Suzuki, A., Tsuchida, Y., Tsuchiya, H., Sumitomo, S., et al. (2017). Polygenic burdens on cell-specific pathways underlie the risk of rheumatoid arthritis. Nat. Genet. 49, 1120– 1125.
Jin, S., Chin, J., Seeber, S., Niewoehner, J., Weiser, B., Beaucamp, N., et al. (2013). TL1A/TNFSF15 directly induces proinflammatory cytokines, including TNF?, from CD3+CD161+ T cells to exacerbate gut inflammation. Mucosal Immunol. 6, 886–899.
Lappalainen, T., Sammeth, M., Friedländer, M. R., t Hoen, P. A. C., Monlong, J., Rivas, M. A., et al. (2013). Transcriptome and genome sequencing uncovers functional variation in humans. Nature 501, 506–511.
Lee, H.-S., Lee, S. B., Kim, B. M., Hong, M., Jung, S., Hong, J., et al. (2018). Association of CDKN2A/CDKN2B with inflammatory bowel disease in Koreans. J. Gastroenterol. Hepatol. 33, 887–893.
Liu, J. Z., van Sommeren, S., Huang, H., Ng, S. C., Alberts, R., Takahashi, A., et al. (2015). Association analyses identify 38 susceptibility loci for inflammatory bowel disease and highlight shared genetic risk across populations. Nat. Genet. 47, 979–986.
Maravillas-Montero, J. L., Burkhardt, A. M., Hevezi, P. A., Carnevale, C. D., Smit, M. J., and Zlotnik, A. (2015). Cutting edge: GPR35/CXCR8 is the receptor of the mucosal chemokine CXCL17. J. Immunol. 194, 29–33.
Marchini, J., Howie, B., Myers, S., McVean, G., and Donnelly, P. (2007). A new multipoint method for genome-wide association studies by imputation of genotypes. Nat. Genet. 39, 906–913.
Martin, M. (2011). Cutadapt removes adapter sequences from high-throughput sequencing reads. EMBnet. journal 17, 1–12.
Mogil, L. S., Andaleon, A., Badalamenti, A., Dickinson, S. P., Guo, X., Rotter, J. I., et al. (2018). Genetic architecture of gene expression traits across diverse populations. PLoS Genet. 14:e1007586. doi: 10.1371/journal.pgen.10007586
Ongen, H., Buil, A., Brown, A. A., Dermitzakis, E. T., and Delaneau, O. (2016). Fast and efficient QTL mapper for thousands of molecular phenotypes. Bioinformatics 32, 1479–1485.
Richard, A. C., Peters, J. E., Savinykh, N., Lee, J. C., Hawley, E. T., Meylan, F., et al. (2018). Reduced monocyte and macrophage TNFSF15/TL1A expression is associated with susceptibility to inflammatory bowel disease. PLoS Genet. 14:e1007458. doi: 10.1371/journal.pgen.10007458
Robinson, M. D., McCarthy, D. J., and Smyth, G. K. (2010). edgeR: a Bioconductor package for differential expression analysis of digital gene expression data. Bioinformatics 26, 139–140.
Segal, A. W. (2019). Studies on patients establish Crohn’s disease as a manifestation of impaired innate immunity. J. Intern. Med. 286, 373–388.
Stegle, O., Parts, L., Piipari, M., Winn, J., and Durbin, R. (2012). Using probabilistic estimation of expression residuals (PEER) to obtain increased power and interpretability of gene expression analyses. Nat. Protoc. 7, 500–507.
The Gene Ontology Consortium (2017). Expansion of the gene ontology knowledgebase and resources. Nucleic Acids Res. 45, D331–D338.
Uniken Venema, W. T., Voskuil, M. D., Vila, A. V., van der Vries, G., Jansen, B. H., Jabri, B., et al. (2019). Single-cell RNA sequencing of blood and ileal T cells from patients with Crohn disease reveals tissue-specific characteristics and drug targets. Gastroenterology 156, 812–815.
Yang, S. K., Hong, M., Oh, H., Low, H. Q., Jung, S., Ahn, S., et al. (2016). Identification of loci at 1q21 and 16q23 that affect susceptibility to inflammatory bowel disease in Koreans. Gastroenterology 151, 1096–1099.
Keywords: Crohn’s disease, gene expression, eQTL, Asian, blood
Citation: Jung S, Liu W, Baek J, Moon JW, Ye BD, Lee H-S, Park SH, Yang S-K, Han B, Liu J and Song K (2020) Expression Quantitative Trait Loci (eQTL) Mapping in Korean Patients With Crohn’s Disease and Identification of Potential Causal Genes Through Integration With Disease Associations. Front. Genet. 11:486. doi: 10.3389/fgene.2020.00486
Received: 15 January 2020; Accepted: 20 April 2020;
Published: 14 May 2020.
Edited by:
Ahmed Rebai, Centre of Biotechnology of Sfax, TunisiaReviewed by:
Kais Ghedira, Institut Pasteur de Tunis, TunisiaFakher Frikha, Faculty of Science of Sfax, Tunisia
Copyright © 2020 Jung, Liu, Baek, Moon, Ye, Lee, Park, Yang, Han, Liu and Song. This is an open-access article distributed under the terms of the Creative Commons Attribution License (CC BY). The use, distribution or reproduction in other forums is permitted, provided the original author(s) and the copyright owner(s) are credited and that the original publication in this journal is cited, in accordance with accepted academic practice. No use, distribution or reproduction is permitted which does not comply with these terms.
*Correspondence: Kyuyoung Song, a3lzb25nQGFtYy5zZW91bC5rcg==
†These authors have contributed equally to this work