- 1Department of Orthopedics, Tongji Hospital, Tongji Medical College, Huazhong University of Science and Technology, Wuhan, China
- 2Department of Digestive Surgical Oncology, Union Hospital, Tongji Medical College, Huazhong University of Science and Technology, Wuhan, China
- 3Cancer Center, Union Hospital, Tongji Medical College, Huazhong University of Science and Technology, Wuhan, China
Osteosarcoma is one of the most common types of bone sarcoma with a poor prognosis. However, genes involved in the mineral metabolism in the microenvironment of the bone affected by osteosarcoma are, to date, largely unknown. A public data series (GSE114237) was used to identify differentially expressed genes (DEGs) between osteosarcoma cells adhering to demineralized osseous surfaces and mineralized osseous surfaces. Functional enrichment analysis of DEGs and hub genes, protein-protein interaction network of DEGs and regulatory network (miRNA-mRNA network and transcription factor (TF)-mRNA network), survival analysis of hub genes was visualized. The prognostic hub genes were considered as candidate genes and their functional predictions were analyzed. A total of 207 DEGs were mainly enriched in extracellular space and thirteen hub genes were mainly enriched in the function of epithelial to mesenchymal transition. However, out of these, only one candidate gene was found to be suitable as a candidate gene. Besides that, 297 miRNAs and 349 TFs interacting with the hub genes were screened. In conclusion, the DEGs, hub genes, miRNAs and TFs screened out in this research could contribute to comprehend the latent mechanisms in osteosarcoma affected by matrix mineral and provide potential research molecular for further study.
Introduction
Osteosarcoma is one of the most common types of bone sarcoma, accounting for approximately 20% of newly diagnosed bone cancers (Klein and Siegal, 2006; Siegel et al., 2018). The prevalence of osteosarcoma is related to bone growth and is the most malignant tumors in adolescents (Mirabello et al., 2009). Unlike the other tissues, there is a lot of mineral in the microenvironment matrix of bone tissue, which may play a potential role in the tumorigenesis of osteosarcoma. When mesenchymal stem cells (MSCs) were embedded in a calcified ceramic scaffold, tumoral bone formation was observed in the surroundings scaffold (Rubio et al., 2014). In vitro experiments showed that CA2+ supplementation can provide bone mineralization (Flammini et al., 2016). Meanwhile, selenium-doped hydroxyapatite nanoparticles could induce apoptosis of tumor cells by an inherent caspase-dependent apoptosis pathway together with the generation of reactive oxygen(Wang et al., 2016). Osteosarcoma cell lines have been reported can act as osteoblasts when grown on calcified materials (Zhang et al., 2013). However, the mechanisms of the mineral in the microenvironment affected by osteosarcoma are, to date, still largely unknown.
Bioinformatics, as a new technology, has been widely developed in recent years and plays an important role in revealing the internal mechanism of tumor progression and carcinogenesis at the genome level. Till date, numerous studies have been published on different cancer types, and some researches have revealed the interesting information of the expression of key genes, microRNAs and co-expression modules in osteosarcoma(Li et al., 2019; Li et al., 2020) and drug resistance in osteosarcoma patients(Bhuvaneshwar et al., 2019).
Here we analyzed the microarray dataset obtained from osteosarcoma cells adhered to the demineralized osseous surfaces and mineralized osseous surfaces from the Gene Expression Omnibus (GEO) database to identify differentially expressed genes (DEGs). Subsequently, a protein-protein interaction (PPI) network was constructed to screen out hub genes in the DEGs. Gene Ontology (GO) and Kyoto Encyclopedia of Genes and Genomes (KEGG) pathway enrichment analyses of the DEGs and the hub genes were used to reveal the underlying mechanisms. Subsequently, we constructed regulatory networks of the hub genes. Furthermore, the hub genes survival analysis was carried out to find some candidate genes and to predict the possible function of these genes. Taken together, a total of 207 DEGs, 297 miRNAs, 349 transcription factors, 13 hub genes, and 1 candidate gene were identified.
Materials and Methods
Identification of DEGs and Construction of PPI Network
A public series submitted by Wischmann et al. in 2018, GSE114237 (Wischmann et al., 2018), was downloaded from the GEO1 (Barrett et al., 2013). The researchers had prepared 8 groups of porcine tibia slices; four groups were demineralized whereas the others were left in their native condition. Later, osteosarcoma cells (MG-63 cells) were seeded onto the osseous surfaces for 72 h. All the expression data were analyzed using the R language (version 3.5.1) BIOCONDUCTOR package, and the DEGs were screened out via the LIMMA package. The cutoff of statistical significance was p-values < 0.05 and an absolute value of fold change of gene expression greater than two. The STRING database (online Search Tool for the Retrieval of Interacting Genes,2) (Szklarczyk et al., 2017) was used to predict and construct PPI network with a statistical significance of interaction scores of >0.4 (medium confidence score), and the network was visualized by Cytoscape software (version 3.7.0, RRID: SCR_003032; the National Resource for Network Biology [NRNB], Bethesda, MD, United States) (Smoot et al., 2011). Subsequently, hub genes were selected via MCODE (Molecular Complex Detection, RRID: SCR_015828) (Bandettini et al., 2012), the app of Cytoscape, employing the following criteria: MCODE score >5.5, degree cut-off = 2, score cut-off = 0.2, max depth = 100, and k-score = 2.
Functional and Pathway Enrichment
DAVID3 (Huang et al., 2009) was used to perform the biological process (BP), cellular component (CC), molecular function (MF) analysis (Gene Ontology Consortium, 2008), and KEGG (Kanehisa and Goto, 2000) pathway enrichment analysis. The DEGs and hub genes were carried out separately with an indicated statistical significance of p-value < 0.05.
Regulatory Network of the Hub Genes
The miRDB4 (Wong and Wang, 2015) online database was used to determine the potential miRNAs that may bind to the hub genes, which were filtered by a confidence score >60. Additionally, the AnimalTFDB 3.05 (Hu et al., 2019), was used to further determine the potential transcription factors (TF) that might interact with hub genes and regulate their expression, filtered with Q-values < 0.01. The miRNA-mRNA and TF-mRNA network were visualized via Cytoscape (Smoot et al., 2011).
Survival Analysis of the Hub Genes
All the hub genes were input into the PROGgeneV26 database (Goswami and Nakshatri, 2014) separately, and the overall survival plot (Kaplan Meier, KM plot) for each gene was created. According to the median gene expression, the data were divided into high and low expression groups. PROGgeneV2 evaluates the prognostic implications of genes using the SURVIVAL package of the R language for a hypothesis test via publicly available data. The hub genes were considered as candidate genes with a p-value less than 0.05 for further analysis.
Prediction of Functions of the Candidate Genes
The candidate genes were submitted to the GeneMANIA7 (Warde-Farley et al., 2010) to illustrate the interactive functional association network among the candidate genes and their co-expression genes. The advanced statistical options used were as follows: max resultant genes = 20, max resultant attributes = 10, using the automatically selected weighing method. Subsequently, the Anatomic Viewer of the online SAGE database (Serial Analysis of Gene Expression database,8) (Boon et al., 2002) displayed the expression of candidate genes in normal and corresponding malignant human tissues. The related expression levels were ordered by different colors based on the counts of SAGE tags. Furthermore, the Open Targets Platform9 (Carvalho-Silva et al., 2019) was used to depict relationships between the candidate genes as drug targets and diseases involved in neoplasm and skeletal system disease.
The parameter selection of all these tools above is based on the developers’ references to select the most appropriate parameters.
Results
Identification of DEGs and Construction of PPI Network
The profiles of the public series from GEO had a good consistency shown in Figure 1A. In total, 207 DEGs were identified in the demineralized group; approximately 151 upregulated and 56 downregulated genes were identified (Figure 1B). As we know, the p-value calculation includes the number of genes we input for the enrichment and the total number of genes set in a specific concentration; it means that if we analyze the up-and down-regulated genes together, we will get a smaller and more significant p-value. In this way, some underestimating important significant pathways can be avoided. The PPI network constructed via Cytoscape of the DEGs as shown in Figure 1C, while the most significant module in Figure 1D with 13 nodes and 33 edges. These 13 genes were proposed as hub genes (12 upregulated genes and 1 downregulated gene). The detailed information is provided in Table 1. The network of hub genes may be an important regulatory mechanism in osteosarcoma affected by matrix mineral.
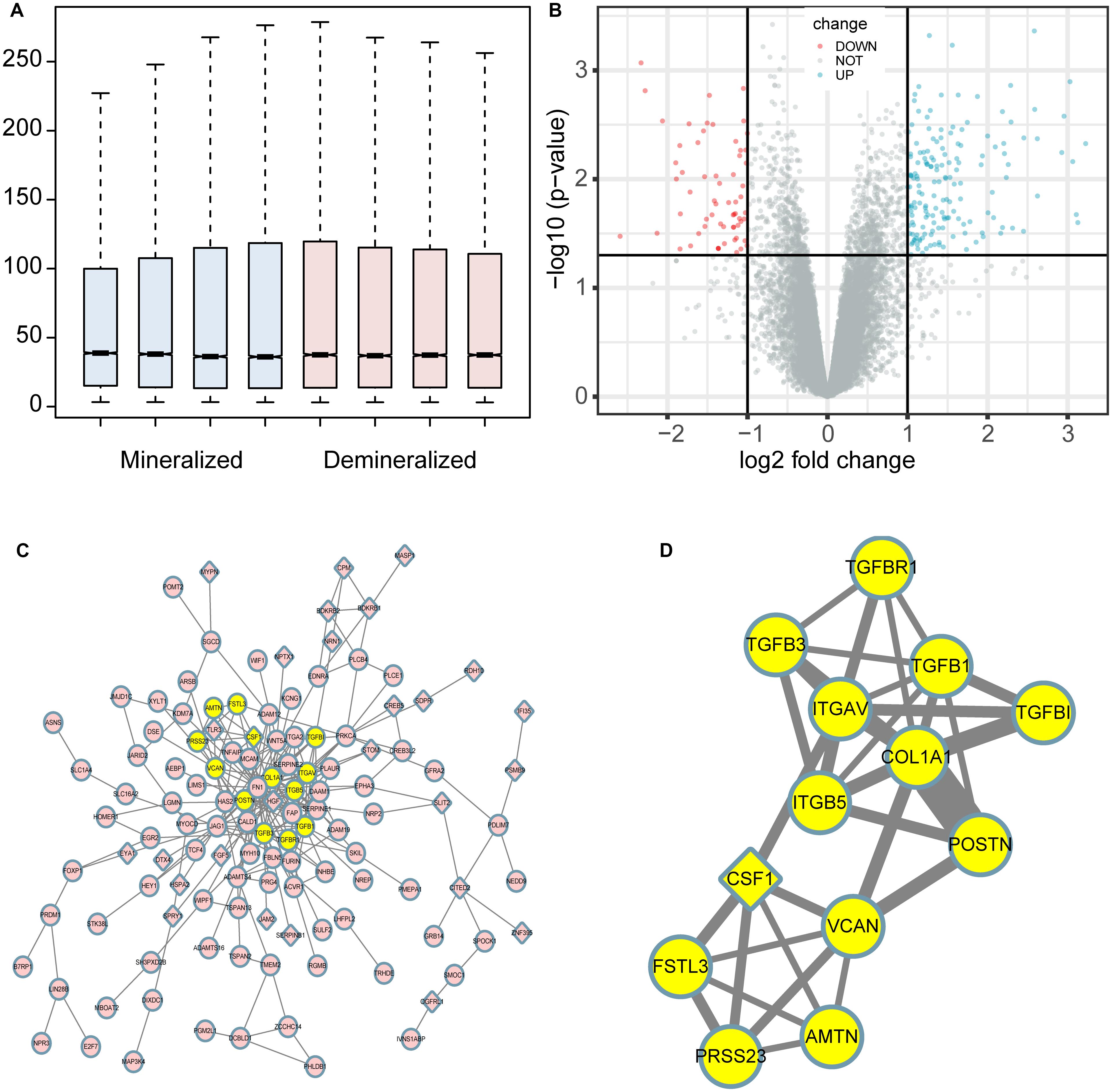
Figure 1. Boxplot, volcano plot, protein-protein interaction (PPI) network, and the most significant module of the DEGs are shown. The boxplot demonstrated that the profiles of GSE114237 had a good consistency (A). The volcano plot exhibited the expression of genes between mineralized and demineralized groups with a cut-off criterion of p-value < 0.01 and absolute value logFC (fold change) >1; the red dots represent downregulated genes, the green dots represent upregulated genes, and the gray dots represent unchanged genes (B). The PPI network of the DEGs was constructed and visualized by Cytoscape (C); the round nodes represent upregulated proteins, the diamond nodes represent downregulated proteins, and the edges represent protein-protein associations. Besides, the yellow nodes represent the most significant module obtained from the PPI network (D); the width of the edges represents the coexpression value between the nodes.
Functional and Pathway Enrichment
Results of the GO and KEGG pathway enrichment analyses of the DEGs are shown in Figure 2 (details are shown in Tables 2, 3). In the BP ontology (Figure 2A), cell adhesion (25 genes) and extracellular matrix organization (13 genes) were the most significantly enriched terms. In the CC ontology (Figure 2B), the terms were related to extracellular space (34 genes) and plasma membrane (64 genes). While the terms in the MF ontology (Figure 2C), were related to transforming growth factor-beta binding (4 genes) and protease binding (7 genes). Besides that, in the KEGG pathways (Figure 2D), the terms were revealed to be proteoglycans in cancer (11 genes) and pathways in cancer (14 genes). Particularly, GO and KEGG pathway enrichment analysis of the hub genes showed that they were mainly related to the positive regulation of epithelial to mesenchymal transition, extracellular matrix, type II transforming growth factor-beta receptor binding, and hypertrophic cardiomyopathy (Figure 3, also can be seen in Supplementary Tables 1, 2). These enrichment results reveal that transforming growth factor-beta binding function, especially in the extracellular matrix, should need more attention.
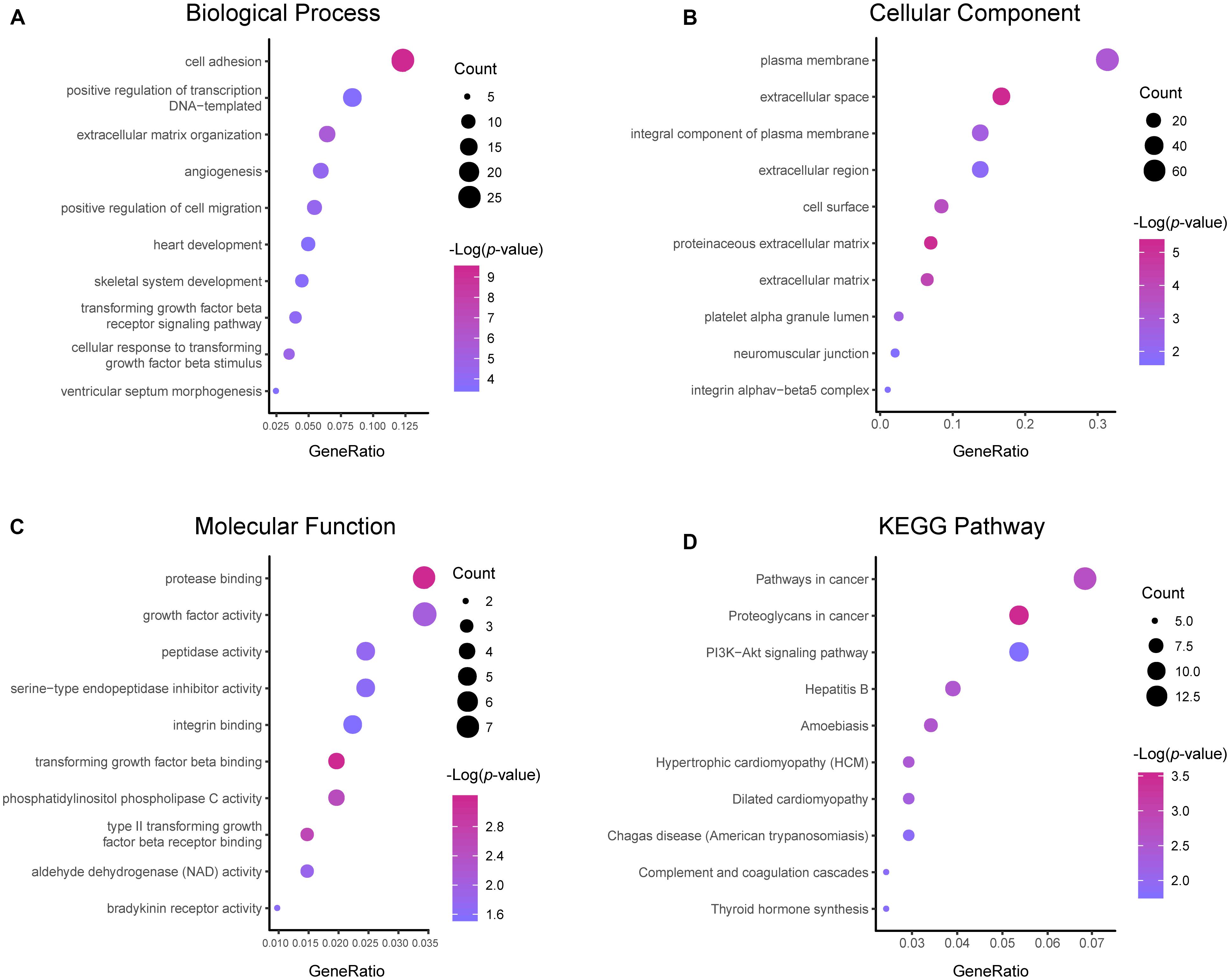
Figure 2. Functional and pathway enrichment analyses of the DEGs. These include the biological process (A), cellular component (B), molecular function (C) and KEGG pathway (D); the size of the dots represents the gene count and the color depth of the dots represents the -log (p-value).
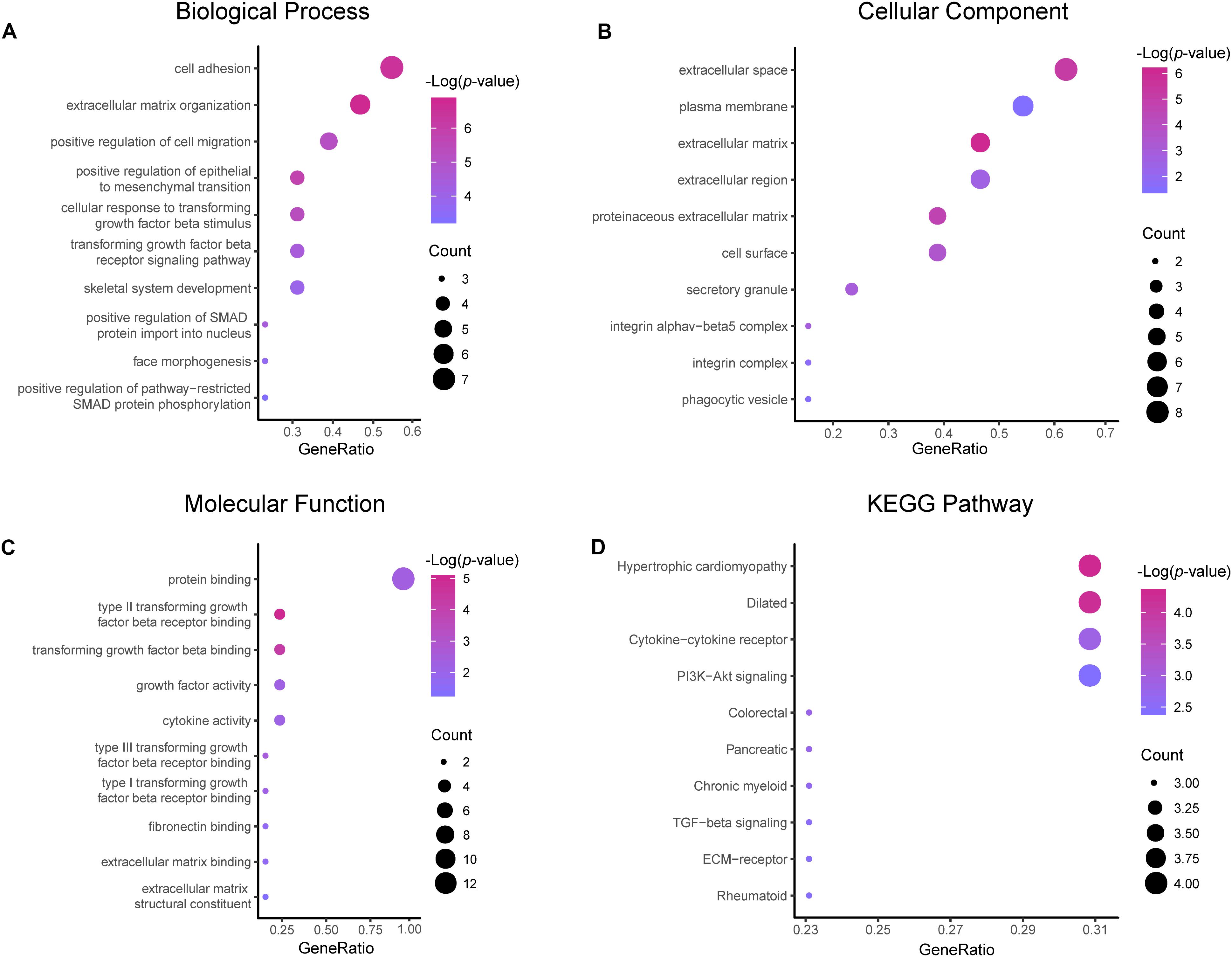
Figure 3. Functional and pathway enrichment analyses of the hub genes. These include the biological process (A), cellular component (B), molecular function (C) and KEGG pathway (D); the size of the dots represents the gene count and the color depth of the dots represents the -log (p-value).
Regulatory Network of the Hub Genes
In total, 297 miRNAs and 349 TFs were screened out employing a relative database (details in Methods). The miRNA-mRNA (Figure 4A) and TF-mRNA (Figure 4B) interaction regulatory networks were constructed and visualized with that the orange nodes represent hub genes, the purple nodes miRNAs, and the dark blue nodes TFs by Cytoscape. The top 10 miRNAs that regulated the hub genes, ranked by topological coefficients, were hsa-miR-664b-3p, hsa-miR-135a-5p, hsa-miR-548an, hsa-miR-135b-5p, hsa-miR-301a-3p, hsa-miR-301b-3p, hsa-miR-454-3p, hsa-miR-130b-3p, hsa-miR-130a-3p, and hsa-miR-128-3p. Furthermore, the top 10 TFs interacted with the hub genes, ranked by topological coefficients, were VEZF1, CHD1, POLR2A, TAF1, RUNX1T1, HCFC1, ELK4, ZEB1, CDK8, and ZNF22. The topological properties of the miRNAs and TFs are displayed in Tables 4, 5. The miRNA-mRNA network and TF-mRNA network predict the potential mechanism, which can provide evidence for subsequent experimental verification.
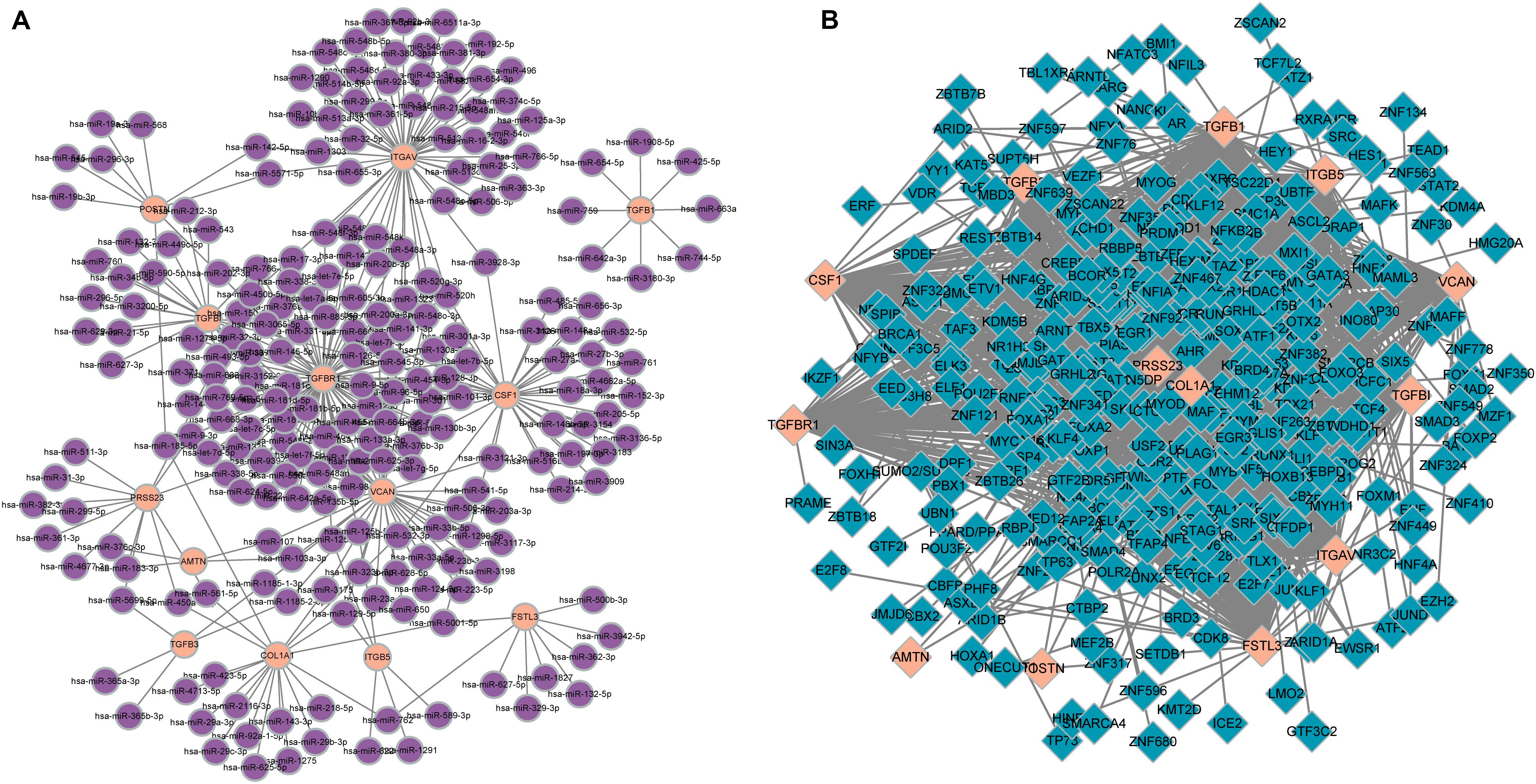
Figure 4. miRNA-mRNA (A) and transcription factor-mRNA (B) regulatory networks of the hub genes; the orange nodes represent hub genes, the purple nodes represent miRNAs, and the dark blue nodes represent transcription factors.
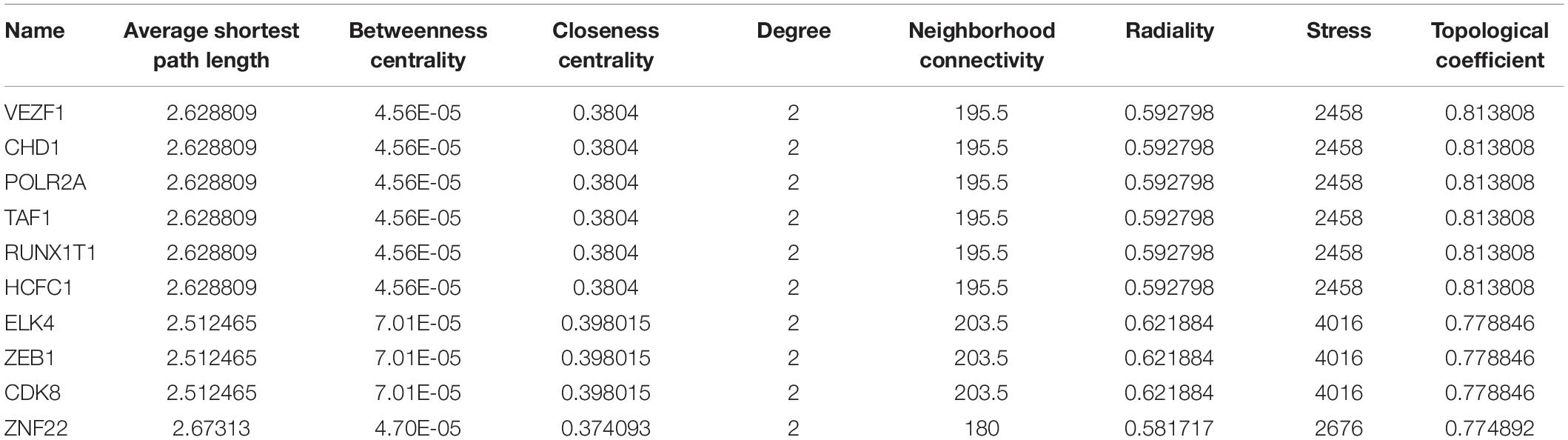
Table 5. The top 10 transcription factors that regulated the hub genes and their topological properties.
Survival Analysis of the Hub Genes
The overall survival plots could be obtained for only 11 hub genes out of 13 hub genes, as shown in Figure 5. However, most of them were not statistically significant. Unfortunately, there was no significant difference in the survival curve of COL1A1, CSF1, FSTL3, ITGAV, ITGB5, POSTN, PRSS23, TGFB1, TGFB3, and TGFBI, and no data result for AMTN and VCAN. Only one gene, namely TGFBR1, had a p-value < 0.05. High expression of TGFBR1 had better overall survival.
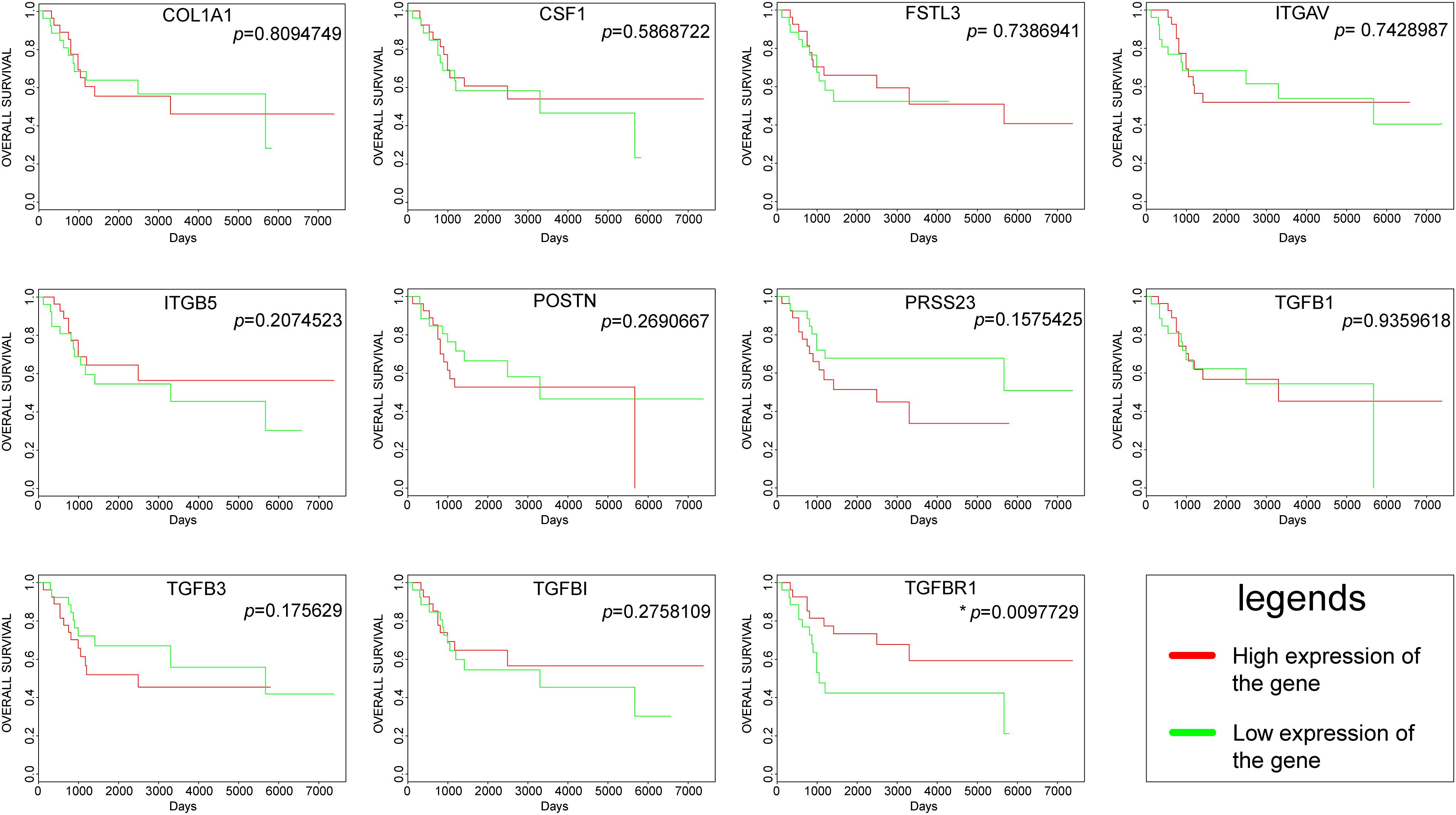
Figure 5. Survival curves of hub genes were created by the PROGgeneV2 online platform; the red line represents a high expression of the genes, and the green line represents a low expression of the genes.
Since TGFBR1 is one of the hub genes involved in the regulation mechanism in osteosarcoma affected by matrix mineral, and its differential expression will affect the prognosis and survival of patients. Therefore, TGFBR1 was considered as a candidate gene for further analyses.
Prediction of Functions of the Candidate Genes
An interactive functional association network constructed by GeneMANIA revealed correlations among genes for the candidate gene. The gene set enriched for TGFBR1 is responsible mainly for the pathways related to TGFB (transforming growth factor-beta) receptor binding and TGFB receptor signaling (Figure 6A). In our study, the expression profiles of the TGFBR1 in human tissue are displayed using SAGE. As shown, TGFBR1 mRNA in thyroid, lung, pancreas, colon, and prostate cancer tissues displayed higher levels than in the matched normal tissues (Figure 6B). Subsequently, the target-disease association related to TGFBR1 in neoplasm and skeletal system disease could be determined and viewed by using the Open Targets Platform. Among the diseases, the top five diseases with a high score of TGFBR1-neoplasm association were cancer, multiple keratoacanthomata, Ferguson-Smith type carcinoma, glioma, and breast carcinoma (Figure 7A). Furthermore, the top five diseases with a high score of TGFBR1-skeletal system disease association were craniosynostosis, connective tissue disease, Marfan syndrome, isolated brachycephaly, and isolated scaphocephaly (Figure 7B). All these findings predict the function of TGFBR1 and can be helpful for further research.
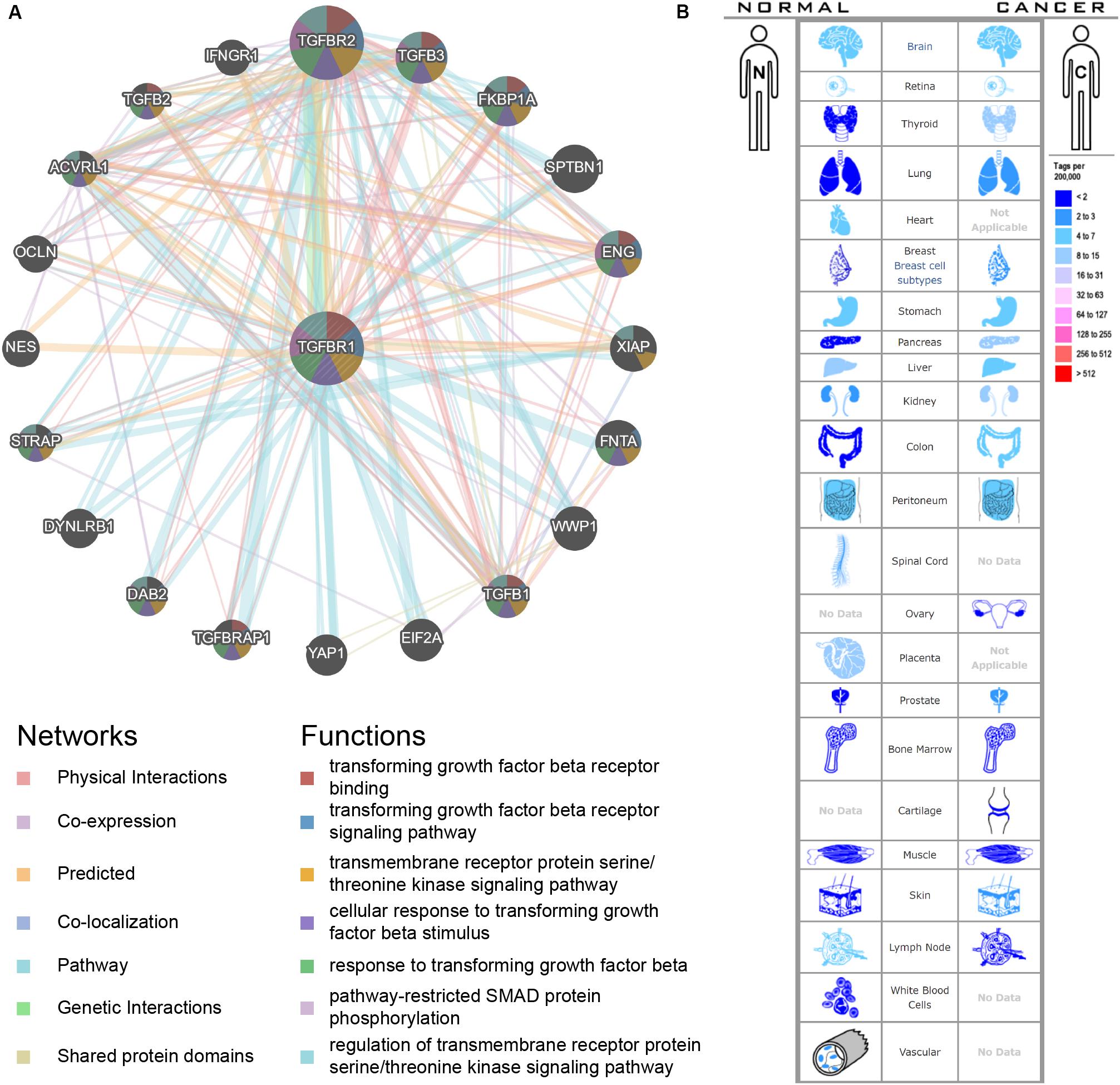
Figure 6. The functional association network (A) and expression profiles (B) of TGFBR1. In the functional association network, the edge-colors represent the bioinformatics method applied; the node-colors represent the biological functions of the set of enrichment genes. In the expression profiles, the left side represents normal tissues and the right side represents the matched cancer tissues. The related expression levels are based on analysis of the counts of SAGE tags, ordered by 10 colors.
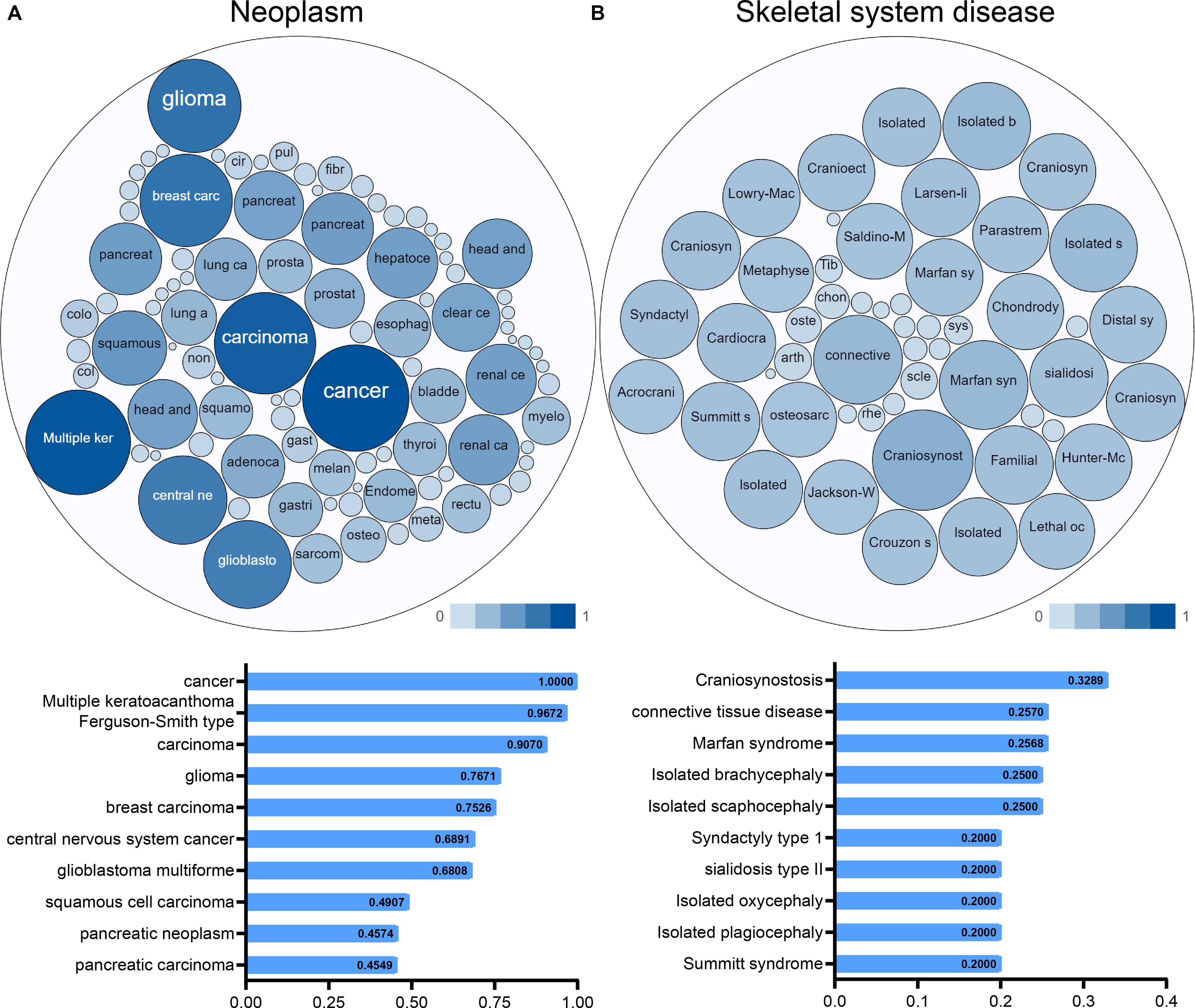
Figure 7. The target-disease association related to TGFBR1 in neoplasm (A) and skeletal system disease (B) could be determined and viewed by the Open Targets Platform; the bubbles color and bubbles size represent the association score, and the top 10 diseases with a high score value are listed below.
Discussion
The mineralization of the bone matrix plays a potentially important role in the process of bone formation. Osteosarcoma is an intraosseous malignancy that occurs predominantly in growing skeletal regions (Alfranca et al., 2015). The extracellular mineral is the main inorganic component of the bone matrix. Zhang et al. reported that osteosarcoma cell lines altered the expression of cytokines when grown on calcified materials (Zhang et al., 2013). Meanwhile, Kingsley et al. found that calcium is released into bone microenvironment through osteoclastic bone resorption, leading to a “vicious circle” by its participation in different mechanisms of Parathyroid Hormone Related Protein (PTHRP) production (Kingsley et al., 2007). Meanwhile, Rubio et al. have demonstrated the role of microenvironment calcium matrix in the development of the osteosarcoma via embedding osteosarcoma tumor cells in calcium phosphate biomaterials (Rubio et al., 2014).
In our study, a microarray dataset was analyzed to obtain DEGs from osteosarcoma cells adhering to the demineralized osseous surfaces against mineralized osseous surfaces. Altogether, 207 DEGs comprised 151 upregulated and 56 downregulated genes were identified. Subsequently, enrichment analyses of the DEGs were used to explore some other interactions. Previous studies have reported that extracellular space in the bone microenvironment is essential for osteosarcoma development (Cai et al., 2015; Baglio et al., 2017; Cortini et al., 2017; Zheng et al., 2018). Moreover, studies have revealed that cell adhesion is a significant process of osteosarcoma, which involves a number of molecules (Gang et al., 2017; Hu et al., 2017; Villanueva et al., 2019). In other words, all these studies are consistent with the results obtained in our studies. In contrast, the functional analysis demonstrated the hub genes were mainly related to the positive regulation of epithelial to mesenchymal transition, extracellular matrix, type II transforming growth factor-beta receptor binding, and hypertrophic cardiomyopathy.
The hub genes were selected in the DEGs PPI network of the most significant module. Further survival analysis revealed TGFBR1 as a candidate gene because of its statistical significance. Meanwhile, some hub genes have been reported involved in the tumorigenesis and progression of osteosarcoma, such as ITGAV, POSTN, COL1A1, TGFB1, TGFB3, and ITGAV. These can be negatively regulated by miR-548c-3p, just like the target agents against integrin as reported in clinical trials, and can be potential tumor therapeutic targets (Luo et al., 2016). Moreover, high expression of POSTN (periostin, also known as osteoblast-specific factor two) in osteosarcoma is significantly associated with angiogenesis and is considered to be a promising prognostic factor in osteosarcoma patients (Hu et al., 2016). Similarly, COL1A1 also has a prognostic value in osteosarcoma patients (He et al., 2014). In osteosarcoma, TGFB signaling could interact with both microenvironment and tumor cells, exerting the characteristic effects of tumor precursors. Therefore, TGFBs could be considered as independent therapeutic targets for osteosarcoma (Lamora et al., 2016; Verrecchia and Redini, 2018; Xie et al., 2018).
In addition, a search of published literature revealed that some hub genes have been associated with tumors. VCAN is reported to be essential for migration and metastasis of breast cancer (Zhang et al., 2019) and could be a potential prognostic biomarker for colon cancer (Chida et al., 2016). Moreover, knockdown of PRSS23 could inhibit EIF2 signaling, thereby suppressing the tumor growth of gastric cancer (Han et al., 2019). Furthermore, one report has demonstrated that CSF1 could promote melanoma resistance to PD1 checkpoint blockade (Neubert et al., 2018), and many reports have shown that CSF1R targeting may be beneficial by showing strong anti-tumor effects (Zhu et al., 2014; Obba et al., 2015; Kumar et al., 2017; Neubert et al., 2018). Similarly, TGFBI has tumor-promoting and tumor-protective roles in certain gastrointestinal tract cancers (Han et al., 2015), and in prostate cancer (Al Shareef et al., 2018).
Particularly, the candidate gene TGFBR1 is reported to be involved in multiple biological functions in tumor. Inhibition of TGFBR1 can block the activation of fibroblasts mediated via IL1B/TGFB1 and reduce the secretion of pro-inflammatory cytokines in colorectal cancer, which would make the cancer cells more sensitive to chemotherapy. Moreover, TGFBR1 inhibitor could reduce the metastasis ability of tumor cells in vivo (Guillen Diaz-Maroto et al., 2019). It is also reported that in lung cancer TGFBR1 may be a target of tumor suppressor genes (Gao et al., 2019). However, there are few reports on the function of TGFBR1 in osteosarcoma. Upregulated TGFBR1 will induce the epithelial-to-mesenchymal transition in osteosarcoma cell lines, which is regulated by the interferon consensus sequence-binding protein, but the specific mechanism is not fully elaborated (ICSBP) (Sung et al., 2019).
This bioinformatics study provides information on DEGs and regulatory elements involved in osteosarcoma affected by matrix mineral. However, the mechanism of these molecules are still unknown, and additional well-designed experiments and analyses are required. In addition, all the results from this study were got in silico. Further, in vivo and in vitro experiments are necessary to test the biological functions of the key genes and their regulatory elements.
In conclusion, we identified 207 DEGs, 13 hub genes, 297 miRNAs, 349 TFs, and 1 candidate gene, which may be involved in the processes of matrix mineralization that may provide a functional signal to osteosarcoma cells. The molecules that we found may be potential targets for future research on the microenvironment of osteosarcoma. Furthermore, our results may contribute to the identification of the biomarkers for matrix mineralization related to osteosarcoma.
Data Availability Statement
The data that support the findings of this study were generated at GSE114237 (Wischmann et al., 2018) in GEO. Derived data supporting the findings of this study are available from the corresponding author on reasonable request.
Author Contributions
ML and XJ analyzed the data. HL provided the help of the R language. CY suggested online tools. SD and GW designed the project. SD selected the analyzed results and wrote the manuscript. All authors read and approved the final manuscript.
Conflict of Interest
The authors declare that the research was conducted in the absence of any commercial or financial relationships that could be construed as a potential conflict of interest.
Acknowledgments
We thank Lao Xinyuan, the CEO of helixlife, for his guidance and help in our scientific research work.
Supplementary Material
The Supplementary Material for this article can be found online at: https://www.frontiersin.org/articles/10.3389/fgene.2020.00533/full#supplementary-material
Abbreviations
DEGs, differentially expressed genes; GEO, gene expression omnibus; GO, gene ontology; KEGG, kyoto encyclopedia of genes and genomes; PPI, protein-protein interaction; SAGE, serial analysis of gene expression; STRING, search tool for the retrieval of interacting genes.
Footnotes
- ^ http://www.ncbi.nlm.nih.gov/geo, RRID: SCR_005012
- ^ http://string-db.org, RRID: SCR_005223
- ^ https://david.ncifcrf.gov/, RRID: SCR_001881
- ^ http://mirdb.org/mining.html, RRID: SCR_010848
- ^ http://www.bioguo.org/AnimalTFDB/, RRID: SCR_001624
- ^ http://genomics.jefferson.edu/proggene
- ^ http://www.genemania.org, RRID: SCR_005709
- ^ http://www.ncbi.nlm.nih.gov/SAGE, RRID: SCR_000796
- ^ https://www.targetvalidation.org/
References
Al Shareef, Z., Kardooni, H., Murillo-Garzon, V., Domenici, G., Stylianakis, E., Steel, J. H., et al. (2018). Protective effect of stromal Dickkopf-3 in prostate cancer: opposing roles for TGFBI and ECM-1. Oncogene 37, 5305–5324. doi: 10.1038/s41388-018-0294-0
Alfranca, A., Martinez-Cruzado, L., Tornin, J., Abarrategi, A., Amaral, T., De Alava, E., et al. (2015). Bone microenvironment signals in osteosarcoma development. Cell Mol. Life Sci. 72, 3097–3113. doi: 10.1007/s00018-015-1918-y
Baglio, S. R., Lagerweij, T., Perez-Lanzon, M., Ho, X. D., Leveille, N., Melo, S. A., et al. (2017). Blocking tumor-educated MSC paracrine activity halts osteosarcoma progression. Clin. Cancer Res. 23, 3721–3733. doi: 10.1158/1078-0432.CCR-17-3198
Bandettini, W. P., Kellman, P., Mancini, C., Booker, O. J., Vasu, S., Leung, S. W., et al. (2012). MultiContrast delayed enhancement (MCODE) improves detection of subendocardial myocardial infarction by late gadolinium enhancement cardiovascular magnetic resonance: a clinical validation study. J. Cardiovasc. Magn. Reson. 14:83. doi: 10.1186/1532-429X-14-83
Barrett, T., Wilhite, S. E., Ledoux, P., Evangelista, C., Kim, I. F., Tomashevsky, M., et al. (2013). NCBI GEO: archive for functional genomics data sets–update. Nucleic Acids Res. 41, D991–D995. doi: 10.1093/nar/gks1193
Bhuvaneshwar, K., Harris, M., Gusev, Y., Madhavan, S., Iyer, R., Vilboux, T., et al. (2019). Genome sequencing analysis of blood cells identifies germline haplotypes strongly associated with drug resistance in osteosarcoma patients. BMC Cancer 19:357. doi: 10.1186/s12885-019-5474-y
Boon, K., Osorio, E. C., Greenhut, S. F., Schaefer, C. F., Shoemaker, J., Polyak, K., et al. (2002). An anatomy of normal and malignant gene expression. Proc. Natl. Acad. Sci. U.S.A. 99, 11287–11292. doi: 10.1073/pnas.152324199
Cai, R., Kawazoe, N., and Chen, G. (2015). Influence of surfaces modified with biomimetic extracellular matrices on adhesion and proliferation of mesenchymal stem cells and osteosarcoma cells. Colloids Surf. B Biointerfaces 126, 381–386. doi: 10.1016/j.colsurfb.2014.11.050
Carvalho-Silva, D., Pierleoni, A., Pignatelli, M., Ong, C., Fumis, L., Karamanis, N., et al. (2019). Open targets platform: new developments and updates two years on. Nucleic Acids Res. 47, D1056–D1065. doi: 10.1093/nar/gky1133
Chida, S., Okayama, H., Noda, M., Saito, K., Nakajima, T., Aoto, K., et al. (2016). Stromal VCAN expression as a potential prognostic biomarker for disease recurrence in stage II-III colon cancer. Carcinogenesis 37, 878–887. doi: 10.1093/carcin/bgw069
Cortini, M., Avnet, S., and Baldini, N. (2017). Mesenchymal stroma: role in osteosarcoma progression. Cancer Lett. 405, 90–99. doi: 10.1016/j.canlet.2017.07.024
Flammini, L., Martuzzi, F., Vivo, V., Ghirri, A., Salomi, E., Bignetti, E., et al. (2016). Hake fish bone as a calcium source for efficient bone mineralization. Int. J. Food Sci. Nutr. 67, 265–273. doi: 10.3109/09637486.2016.1150434
Gang, L., Qun, L., Liu, W. D., Li, Y. S., Xu, Y. Z., and Yuan, D. T. (2017). MicroRNA-34a promotes cell cycle arrest and apoptosis and suppresses cell adhesion by targeting DUSP1 in osteosarcoma. Am. J. Transl. Res. 9, 5388–5399.
Gao, L., Hu, Y., Tian, Y., Fan, Z., Wang, K., Li, H., et al. (2019). Lung cancer deficient in the tumor suppressor GATA4 is sensitive to TGFBR1 inhibition. Nat. Commun. 10:1665. doi: 10.1038/s41467-019-09295-7
Gene Ontology Consortium, (2008). The Gene Ontology project in 2008. Nucleic Acids Res. 36, D440–D444. doi: 10.1093/nar/gkm883
Goswami, C. P., and Nakshatri, H. (2014). PROGgeneV2: enhancements on the existing database. BMC Cancer 14:970. doi: 10.1186/1471-2407-14-970
Guillen Diaz-Maroto, N., Sanz-Pamplona, R., Berdiel-Acer, M., Cimas, F. J., Garcia, E., Goncalves-Ribeiro, S., et al. (2019). Noncanonical TGFbeta pathway relieves the blockade of IL1beta/TGFbeta-mediated crosstalk between tumor and stroma: TGFBR1 and TAK1 inhibition in colorectal cancer. Clin. Cancer Res. 25, 4466–4479. doi: 10.1158/1078-0432.CCR-18-3957
Han, B., Cai, H., Chen, Y., Hu, B., Luo, H., Wu, Y., et al. (2015). The role of TGFBI (betaig-H3) in gastrointestinal tract tumorigenesis. Mol. Cancer 14:64. doi: 10.1186/s12943-015-0335-z
Han, B., Yang, Y., Chen, J., He, X., Lv, N., and Yan, R. (2019). PRSS23 knockdown inhibits gastric tumorigenesis through EIF2 signaling. Pharmacol. Res. 142, 50–57. doi: 10.1016/j.phrs.2019.02.008
He, M., Wang, Z., Zhao, J., Chen, Y., and Wu, Y. (2014). COL1A1 polymorphism is associated with risks of osteosarcoma susceptibility and death. Tumour Biol. 35, 1297–1305. doi: 10.1007/s13277-013-1172-6
Hu, C., Chen, X., Wen, J., Gong, L., Liu, Z., Wang, J., et al. (2017). Antitumor effect of focal adhesion kinase inhibitor PF562271 against human osteosarcoma in vitro and in vivo. Cancer Sci. 108, 1347–1356. doi: 10.1111/cas.13256
Hu, F., Shang, X. F., Wang, W., Jiang, W., Fang, C., Tan, D., et al. (2016). High-level expression of periostin is significantly correlated with tumour angiogenesis and poor prognosis in osteosarcoma. Int. J. Exp. Pathol. 97, 86–92. doi: 10.1111/iep.12171
Hu, H., Miao, Y. R., Jia, L. H., Yu, Q. Y., Zhang, Q., and Guo, A. Y. (2019). AnimalTFDB 3.0: a comprehensive resource for annotation and prediction of animal transcription factors. Nucleic Acids Res. 47, D33–D38. doi: 10.1093/nar/gky822
Huang, D. W., Sherman, B. T., and Lempicki, R. A. (2009). Systematic and integrative analysis of large gene lists using DAVID bioinformatics resources. Nat. Protoc. 4, 44–57.
Kanehisa, M., and Goto, S. (2000). KEGG: kyoto encyclopedia of genes and genomes. Nucleic Acids Res. 28, 27–30. doi: 10.1038/gene.2015.7
Kingsley, L. A., Fournier, P. G., Chirgwin, J. M., and Guise, T. A. (2007). Molecular biology of bone metastasis. Mol. Cancer Ther. 6, 2609–2617.
Klein, M. J., and Siegal, G. P. (2006). Osteosarcoma: anatomic and histologic variants. Am. J. Clin. Pathol. 125, 555–581.
Kumar, V., Donthireddy, L., Marvel, D., Condamine, T., Wang, F., Lavilla-Alonso, S., et al. (2017). Cancer-associated fibroblasts neutralize the anti-tumor effect of CSF1 receptor blockade by inducing PMN-MDSC infiltration of tumors. Cancer Cell 32, 654.e5–668.e5. doi: 10.1016/j.ccell.2017.10.005
Lamora, A., Talbot, J., Mullard, M., Brounais-Le Royer, B., Redini, F., and Verrecchia, F. (2016). TGF-beta signaling in bone remodeling and osteosarcoma progression. J. Clin. Med. 5:96. doi: 10.3390/jcm5110096
Li, M., Jin, X., Guo, F., Wu, G., Wu, L., and Deng, S. (2019). Integrative analyses of key genes and regulatory elements in fluoride-affected osteosarcoma. J. Cell. Biochem. 120, 15397–15409. doi: 10.1002/jcb.28807
Li, M., Jin, X., Li, H., Wu, G., Wang, S., Yang, C., et al. (2020). Key genes with prognostic values in suppression of osteosarcoma metastasis using comprehensive analysis. BMC Cancer 20:65. doi: 10.1186/s12885-020-6542-z
Luo, Z., Li, D., Luo, X., Li, L., Gu, S., Yu, L., et al. (2016). Decreased expression of miR-548c-3p in osteosarcoma contributes to cell proliferation via targeting ITGAV. Cancer Biother. Radiopharm. 31, 153–158. doi: 10.1089/cbr.2016.1995
Mirabello, L., Troisi, R. J., and Savage, S. A. (2009). Osteosarcoma incidence and survival rates from 1973 to 2004: data from the surveillance, epidemiology, and end results program. Cancer 115, 1531–1543. doi: 10.1002/cncr.24121
Neubert, N. J., Schmittnaegel, M., Bordry, N., Nassiri, S., Wald, N., Martignier, C., et al. (2018). T cell-induced CSF1 promotes melanoma resistance to PD1 blockade. Sci. Transl. Med. 10:eaan3311. doi: 10.1126/scitranslmed.aan3311
Obba, S., Hizir, Z., Boyer, L., Selimoglu-Buet, D., Pfeifer, A., Michel, G., et al. (2015). The PRKAA1/AMPKalpha1 pathway triggers autophagy during CSF1-induced human monocyte differentiation and is a potential target in CMML. Autophagy 11, 1114–1129. doi: 10.1080/15548627.2015.1034406
Rubio, R., Abarrategi, A., Garcia-Castro, J., Martinez-Cruzado, L., Suarez, C., Tornin, J., et al. (2014). Bone environment is essential for osteosarcoma development from transformed mesenchymal stem cells. Stem Cells 32, 1136–1148. doi: 10.1002/stem.1647
Siegel, R. L., Miller, K. D., and Jemal, A. (2018). Cancer statistics, 2018. CA Cancer J. Clin. 68, 7–30.
Smoot, M. E., Ono, K., Ruscheinski, J., Wang, P. L., and Ideker, T. (2011). Cytoscape 2.8: new features for data integration and network visualization. Bioinformatics 27, 431–432. doi: 10.1093/bioinformatics/btq675
Sung, J. Y., Yoon, K., Ye, S. K., Goh, S. H., Park, S. Y., Kim, J. H., et al. (2019). Upregulation of transforming growth factor-beta type I receptor by interferon consensus sequence-binding protein in osteosarcoma cells. Biochim. Biophys. Acta Mol. Cell. Res. 1866, 761–772. doi: 10.1016/j.bbamcr.2019.01.015
Szklarczyk, D., Morris, J. H., Cook, H., Kuhn, M., Wyder, S., Simonovic, M., et al. (2017). The STRING database in 2017: quality-controlled protein-protein association networks, made broadly accessible. Nucleic Acids Res. 45, D362–D368. doi: 10.1093/nar/gkw937
Verrecchia, F., and Redini, F. (2018). Transforming growth factor-beta signaling plays a pivotal role in the interplay between osteosarcoma cells and their microenvironment. Front. Oncol. 8:133. doi: 10.3389/fonc.2018.00133
Villanueva, F., Araya, H., Briceno, P., Varela, N., Stevenson, A., Jerez, S., et al. (2019). The cancer-related transcription factor RUNX2 modulates expression and secretion of the matricellular protein osteopontin in osteosarcoma cells to promote adhesion to endothelial pulmonary cells and lung metastasis. J. Cell Physiol. 234, 13659–13679. doi: 10.1002/jcp.28046
Wang, Y., Wang, J., Hao, H., Cai, M., Wang, S., Ma, J., et al. (2016). In vitro and in vivo mechanism of bone tumor inhibition by selenium-doped bone mineral nanoparticles. ACS Nano 10, 9927–9937. doi: 10.1021/acsnano.6b03835
Warde-Farley, D., Donaldson, S. L., Comes, O., Zuberi, K., Badrawi, R., Chao, P., et al. (2010). The GeneMANIA prediction server: biological network integration for gene prioritization and predicting gene function. Nucleic Acids Res. 38, W214–W220. doi: 10.1093/nar/gkq537
Wischmann, J., Lenze, F., Thiel, A., Bookbinder, S., Querido, W., Schmidt, O., et al. (2018). Matrix mineralization controls gene expression in osteoblastic cells. Exp. Cell Res. 372, 25–34. doi: 10.1016/j.yexcr.2018.09.005
Wong, N., and Wang, X. (2015). miRDB: an online resource for microRNA target prediction and functional annotations. Nucleic Acids Res. 43, D146–D152. doi: 10.1093/nar/gku1104
Xie, L., Yao, Z., Zhang, Y., Li, D., Hu, F., Liao, Y., et al. (2018). Deep RNA sequencing reveals the dynamic regulation of miRNA, lncRNAs, and mRNAs in osteosarcoma tumorigenesis and pulmonary metastasis. Cell Death Dis. 9:772. doi: 10.1038/s41419-018-0813-5
Zhang, H., Wu, H., Zheng, J., Yu, P., Xu, L., Jiang, P., et al. (2013). Transforming growth factor beta1 signal is crucial for dedifferentiation of cancer cells to cancer stem cells in osteosarcoma. Stem Cells 31, 433–446. doi: 10.1002/stem.1298
Zhang, Y., Zou, X., Qian, W., Weng, X., Zhang, L., Zhang, L., et al. (2019). Enhanced PAPSS2/VCAN sulfation axis is essential for Snail-mediated breast cancer cell migration and metastasis. Cell Death Differ. 26, 565–579. doi: 10.1038/s41418-018-0147-y
Zheng, Y., Wang, G., Chen, R., Hua, Y., and Cai, Z. (2018). Mesenchymal stem cells in the osteosarcoma microenvironment: their biological properties, influence on tumor growth, and therapeutic implications. Stem Cell Res. Ther. 9:22. doi: 10.1186/s13287-018-0780-x
Zhu, Y., Knolhoff, B. L., Meyer, M. A., Nywening, T. M., West, B. L., Luo, J., et al. (2014). CSF1/CSF1R blockade reprograms tumor-infiltrating macrophages and improves response to T-cell checkpoint immunotherapy in pancreatic cancer models. Cancer Res. 74, 5057–5069. doi: 10.1158/0008-5472.CAN-13-3723
Keywords: osteosarcoma, matrix mineral, protein-protein interaction network, differentially expressed genes, regulatory elements
Citation: Li M, Jin X, Li H, Yang C, Deng S and Wu G (2020) Comprehensive Analysis of Key Genes and Regulatory Elements in Osteosarcoma Affected by Bone Matrix Mineral With Prognostic Values. Front. Genet. 11:533. doi: 10.3389/fgene.2020.00533
Received: 03 February 2020; Accepted: 04 May 2020;
Published: 03 June 2020.
Edited by:
Chun Liang, Miami University, United StatesReviewed by:
Jin Chen, University of Kentucky, United StatesFiroz Ahmed, Jeddah University, Saudi Arabia
Copyright © 2020 Li, Jin, Li, Yang, Deng and Wu. This is an open-access article distributed under the terms of the Creative Commons Attribution License (CC BY). The use, distribution or reproduction in other forums is permitted, provided the original author(s) and the copyright owner(s) are credited and that the original publication in this journal is cited, in accordance with accepted academic practice. No use, distribution or reproduction is permitted which does not comply with these terms.
*Correspondence: Sisi Deng, ZGVuZ3Npc2lAaHVzdC5lZHUuY24=; Gang Wu, eGh6bHdnQDE2My5jb20=
†These authors have contributed equally to this work and share first authorship