- 1Zan Mitrev Clinic, Skopje, Macedonia
- 2Bio Engineering LLC, Skopje, Macedonia
To enable the implementation of precise genomics in a local healthcare system, we devised a pipeline for filtering and reporting of relevant genetic information to healthy individuals based on exome or genome data. In our analytical pipeline, the first tier of filtering is variant-centric, and it is based on the selection of annotated pathogenic, protective, risk factor, and drug response variants, and their one-by-one detailed evaluation. This is followed by a second-tier gene-centric deconstruction and filtering of virtual gene lists associated with diseases, and VUS-centric filtering according to ACMG pathogenicity criteria and pre-defined deleteriousness criteria. By applying this filtering protocol, we were able to provide valuable insights regarding the carrier status, pharmacogenetic profile, actionable cardiovascular and cancer predispositions, and potentially pathogenic variants of unknown significance to our patients. Our experience demonstrates that genomic profiling can be implemented into routine healthcare and provide information of medical significance.
Introduction
Personalized medicine is a proactive medical approach, which in general seeks to stratify patients in risk groups and tailor treatments, medical decisions, health promotion, or preventive measures according to the individual’s omics baseline profile combined with lifestyle and environmental factors (Ashley, 2016). The advent of cost-effective next-generation sequencing (NGS) technologies, such as the sequencing of whole genomes (WGS), whole and clinical exomes (WES and CES), combined with the accumulation of genetic knowledge and easy-to-use bioinformatics tools, has paved the way for genomics-based personalized medicine into clinics (Manolio et al., 2013; Goodwin et al., 2016; Doble et al., 2017; Vassy et al., 2017; Bylstra et al., 2019; Zoltick et al., 2019). Nowadays, these technologies have already started to transform healthcare by enabling precise disease screening, actionable diagnostics, treatment, and management. Despite this, precision genomics has not been fully implemented in the vast majority of healthcare systems yet. In order to facilitate its implementation, practical and user-friendly workflows and pipelines are required.
In this study, we primarily aimed to describe a pipeline for balanced CES, WES, or WGS reporting of called variants in healthy individuals interested in proactive genetic testing. A batch of datasets taken from symptomatic patients was included only as a proof-of-principle. This approach of variant filtering helped us to initialize the process of implementing precision genomics in clinical practice at a tertiary healthcare institution (Figure 1). By applying this workflow, we were expecting to find actionable variants of clinical relevance or variants that might aid reproductive decisions. Our current experience demonstrates that the implementation of genomic profiling following our filtering pipeline into real-life clinical practice can provide information of medical significance. The pipeline could be used in future systematic and longitudinal studies focusing on the translational aspects of genomic medicine.
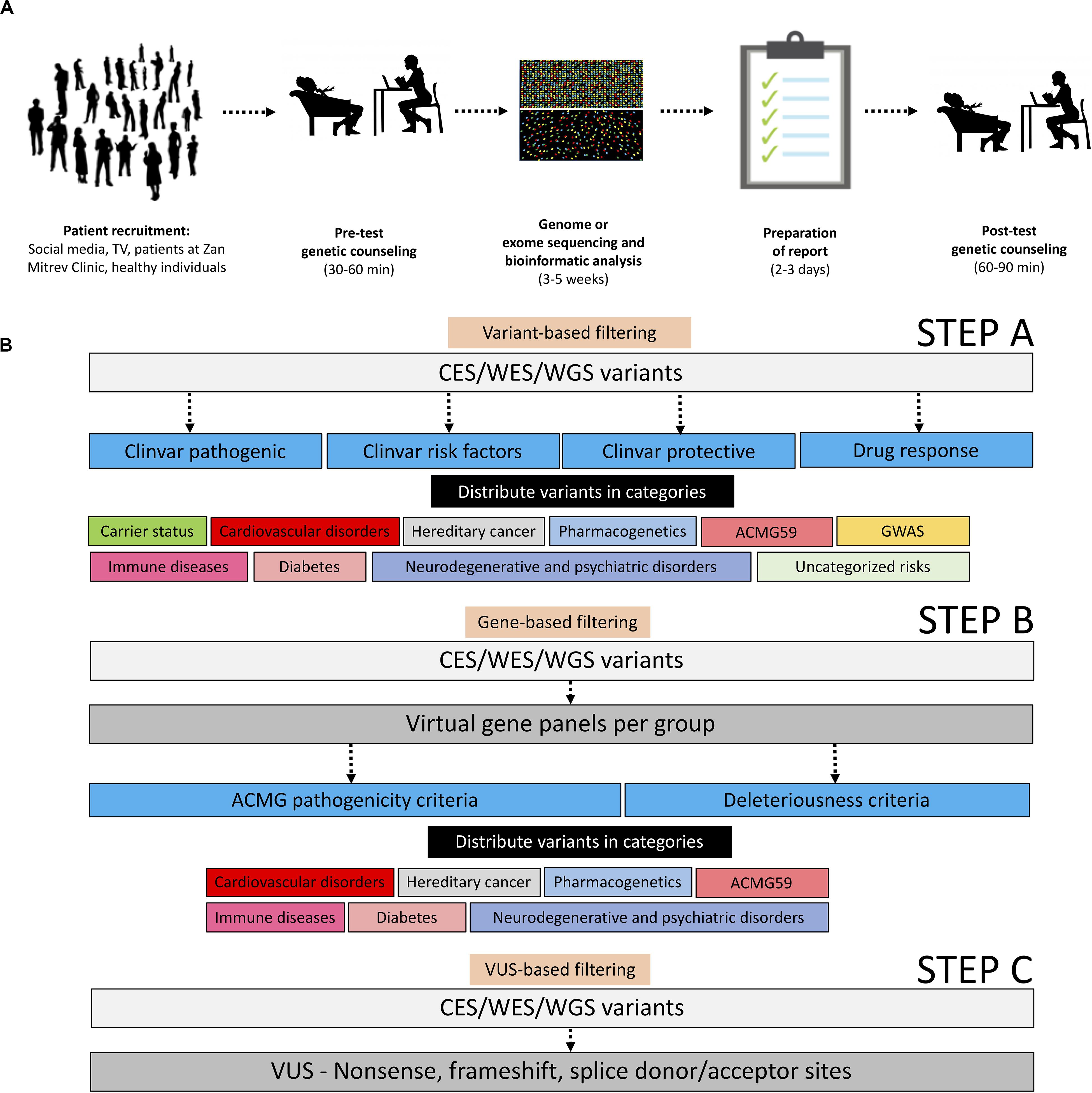
Figure 1. Operational and analytical workflows applied in this study. (A) Operational workflow starting with patient recruitment, pre-test genetic counseling, genome, or exome sequencing and bioinformatic analyses, representative sample of reports, and post-test genetic counseling. All adapted images used in this figure have a CC license. (B) Description of the analytical workflow for variant filtration.
Materials and Methods
Patients
We evaluated 94 patients meeting our inclusion/exclusion criteria with a median age of 34.5 years (range 2 to 65) of which 51/94 (54%) were males and 43/94 (46%) were females (Table 1). All individuals/patients were recruited at the Zan Mitrev Clinic either through regular pro-active check-ups or TV/social media. The sole inclusion criterion for symptomatic patients was a referral from a medical specialist; patients who were not able to provide written informed consent and complete medical history were excluded from the study. In addition, healthy individuals who were unable to provide written informed consent were excluded from the study (Figure 1A). The analysis was done according to the workflow described in Figure 1B. The vast majority of patients were of Macedonian descent 74/94 (78.72%), followed by Albanian 11/94 (11.70%), Serbian 4/94 (4.25%), American 3/94 (3.19%), Turkish 1/94 (1.06%), and Bulgarian 1/94 (1.06%). The aforementioned protocol was used only as a proof-of-principle for analyzing genetic data from symptomatic patients (n = 15); we only communicated the mutations associated with the clinical phenotype. In contrast, full reports following this protocol were disclosed to all healthy individuals (n = 79). Symptomatic patients were informed that additional unrelated information concerning their carrier status and pharmacogenetic profile could be provided as well. Although the WGS analysis has advantages over WES and CES in the respect of providing more comprehensive and uniform coverage of the whole genome, most of the patients 89/94 (94.7%) underwent WES or CES testing, due to cost-effectiveness.
Ethics Statement
Written and signed informed consent for participation and publication of data was obtained from all subjects or their legal guardians (for patients under the age of eighteen) in this study. The ethics committee of the Zan Mitrev Clinic waived the need for IRB approval, deeming written and signed informed consent sufficient.
DNA Extraction, Library Preparation, NGS Sequencing
Around 5 ml of whole blood was collected in K2-EDTA tubes, following accepted principles for blood drawing and blood collection. DNA was extracted from 400 μl of whole blood in a SaMag-12 automatic nucleic acid extraction system (Sacace Biotechnologies, Como, Italy), yielding between 5 and 15 μg of pure DNA, measured by NanoDrop spectrometry (A260/280 ratio 1.7–1.9). Clinical exome enrichment was carried out by using the TruSight One sequencing panel (Illumina, San Diego, United States) or in-house developed CES enrichment protocol (Sophia Genetics, Saint-Sulpice, Switzerland). Whole exome enrichment was carried out by using the SureSelect Human All Exon V6 kit (Agilent Technologies, Santa Clara, United States) or Human Core Exome kit (Twist Bioscience, San Francisco, United States). The entire wet lab work (DNA QC, enrichment, library preparation, and sequencing) for CES, WES, and WGS were carried out in the Sophia Genetics, Wuxi Nextcode or DNA link, or Beijing Genomics Institute facilities, respectively.
Primary Bioinformatic Analysis
For CES, between 13 and 30 million reads were obtained with a NextSeq machine (Illumina, San Diego, United States), with a coverage of at least 50x for average 81% of all sequences. Sequence quality control was done with FastQC1, and sequences were mapped to hg19 with BWA (Li and Durbin, 2009). single nucleotide variant (SNV) and indel calling, together with advanced variant annotation, were done with the Sophia DDM platform (Sophia Genetics, Saint-Sulpice, Switzerland).
For WES and WGS, between 40 and 120 million reads or ∼950 million were obtained with a HiSeq X–10 machine or NovaSeq 6000 (Illumina, San Diego, United States), respectively. The coverage of WES or WGS was >75x or >40x, respectively. Alignment, variant calling, and annotation were done on the Genoox platform (Palo Alto, United States).
All CES, WES, and WGS variant lists were additionally annotated with Annovar, which provides more annotation notes than Sophia Genetics and Genoox (Yang and Wang, 2015). All detected variants were taken into consideration in the subsequent filtering steps.
Secondary Bioinformatics Analysis, Filtering, and Interpretation of Variants
The list of high confidence annotated variants was downloaded directly from the Sophia DDM/Genoox platforms in a.txt or.csv format and analyzed further in a spreadsheet program such as Microsoft Excel. In the primary variant-based selection step the entire list of variants was filtered (either in Excel or in-platform) based on ClinVar terms “pathogenic,” “protective,” “risk factor,” and “drug response” followed by manual curation, manual filtration, and manual function attribution, and then distributed in the following categories: carrier status, cardiovascular disorders, hereditary cancer, pharmacogenetics, ACMG59 (Kalia et al., 2017), immune diseases, diabetes, neurodegenerative and psychiatric disorders, uncategorized risks, and genome-wide association studies (GWAS; MacArthur et al., 2017).
As a secondary gene-based filtering approach, by using the “virtual panel” capability within the Sophia DDM/Genoox platforms, we have created an array of standardized virtual gene panels encompassing genes associated with (1) cardiovascular disorders, (2) hereditary cancer, (3) neurodegenerative and psychiatric disorders, (4) diabetes, (5) immune diseases, and (6) ACMG59 genes (Kalia et al., 2017). The virtual gene lists per panel can be found in Supplementary File S1. Variants were selected from these virtual panels based on ACMG pathogenicity criteria (Richards et al., 2015) or computationally defined deleteriousness criteria (SIFT, Polyphen2, Mutation Taster, Mutation Assessor, FATHMM, dbscSNV Ada, GERP, GeneCanyon, and fitCons), and distributed in the above-mentioned categories.
Finally, in a third filtering variants of uncertain significance (VUS)-based approach, we selected all the non-sense/frameshift VUS in exons/splice donor-acceptor sites, and we reported them in the annex without further interpretation in order to make sure their significance is reassessed in the future when their function is determined (Figure 1B).
All selected variants were evaluated (selected or discarded) according to information in ClinVar and literature2. Also, further evaluation in other databases such as the human gene mutation database3, CentoMD database (Trujillano et al., 2017), and Clinvitae4 was carried out. Pathogenic variants, especially for medium and high penetrance alleles, were interpreted according to the latest available literature and ClinGen guidelines5. Disease risk for non-Mendelian, lower-penetrance variants associated with common diseases was assessed based on GWAS. Typically, the odds ratio or relative risk was reported, or in rare cases P-value or chi-square statistic, respectively.
To increase the detection rate and minimize the rate of false positives, first, we applied the entire protocol for all detected variants (low and high confidence), and then we did the same only for high confidence retained variants. Retained variants had a quality score >100, read depth >10, and quality by depth >10. To further reduce the level of false-positive variants, we retained only variants detected by multiple variant callers (GATK Haplotype Caller and FreeBayes). Alleles with a representation of >25% of the total read coverage were defined as heterozygous. All discrepancies were solved by additional manual evaluation.
Patient Reports
Concerning the bioinformatics strategies for data analysis, filtering, and interpretation, we wanted to strike a balance between under- and over-reporting of variants; in other words, we wanted to maximize the benefit of the provided genetic analysis while reducing the costs and unnecessary follow-ups. Patient reports were divided into two sections, main report and annex, following the same line of reasoning as described previously (McLaughlin et al., 2014). The main report is typically 5–6 pages long and encompasses all the major findings (typically related to medium and high penetrance diseases), patient information, and methodology in a clear and concise language. In addition to the main report, we attached an annex consisting of 20–30 pages of all the selected (relevant) variants assigned to the above categories, in a tabular form accompanied with additional information such as:
• An in-depth reference to studies and major findings of the studies, especially for high penetrance alleles
• Odds ratio/relative risk/chi-square/p-value for common alleles (if available)
• Name of gene
• Type of variant (SNV, indel, etc.)
• Functional consequence (non-sense, missense, etc.)
• Genomic region (exonic, intronic, 5’UTR, etc.)
• Chromosome and chromosomal coordinates
• dbSNP or ClinVar rsID
• Population frequency (G1000, ExAC, esp5400)
• ClinVar signature (pathogenic, benign, drug response, etc.). This section reports the overall interpretation of a variation based on aggregating data from submitters.
• Inheritance (autosomal recessive, autosomal dominant, etc.)
• Level of evidence in the pharmacogenetics section (based on6 grading system)
Genetic Counseling
All the patients underwent pre- and post-test genetic counseling. At the time of pre-test genetic counseling patients were informed about the potential implications of the genetic results to themselves and their families. We discuss the pros and cons of the test, and the current state of genetic/genomic research, as well as the basic principles of inheritance and penetrance. Following the test, we held in-depth discussions where the patients were reacquainted with the basics of DNA biology, genetic variants, types of inheritance, penetrance, and implications. With regard to common medical conditions and diseases, patients were told that these conditions are multifactorial and may include other known or unknown genetic, lifestyle, or environmental components. The genetic counselors stressed the meaning of phrases “no known pathogenic mutation causing/associated with [name of condition]” and the meaning and gravity of known pathogenic mutations.
Prior to testing, all of the patients signed written informed consent. All patients having actionable variants underwent a further examination or consultation with a relevant specialist. Hence, whenever mentioned further on that the patient was advised for a specific medical procedure, the advice came from relevant specialists and not the genetic counselors alone.
Results
In our efforts to provide the most relevant genomic information to our patients, we divided variants into two categories: variants of known significance (VKS) obtained from the filtering steps A and B and VUS obtained from the filtering step C. Out of the VKS basket, we only selected variants with a defined clinical value (e.g., high penetrance, level 1a/1b pharmacogenetic association, direct involvement in a clinically relevant pathway). Out of the VUS basket, we reported only variants fulfilling ACMG pathogenicity criteria in medium and high penetrance disease-causing genes. For better reporting, every filtered variant was distributed in a fitting category (Figure 1B).
Evaluation of Known Variants
Carrier Status of Rare Diseases
By selecting genetic variants annotated as “pathogenic” or “likely pathogenic” in the ClinVar database, supplemented by further manual curation, available literature evaluation, and filtration of low-frequency variants, the rare disease carrier status of each patient for known variants was derived. The vast majority of patients (96.2%) were carriers of at least one known rare disorder/condition with some of them carrying multiple pathogenic variants, median = 4 (Supplementary File S2).
Drug Response
Regarding “drug response,” the list of variants was interpreted using information from the pharmGKB7 database. Only level 1A and level 1B clinical annotations were added to the main report, while the rest of them remained in the annex. Most of the patients were carriers of multiple Level 1A/1B variants. For example, we observed that 34/94 (36.2%) patients were “poor/intermediate CYP2D6 metabolizers,” which is relevant for the metabolism of many drugs, including anti-depressants, opioids, and tamoxifen. In order to reduce the misclassification rate of CYP2D6 metabolizers, we are currently implementing approaches for the detection of CYP2D6 (and other genes) copy number variants from WES data. In addition, 23/94 (24.5%) patients were “poor/intermediate CYP2C19 metabolizers” highly relevant for antiplatelet therapy with clopidogrel, in line with observations from our cohort of >3,000 patients (Klinceva et al., 2018). Similarly, 26/94 (27.7%) patients had a risk of statin-induced myopathy, with 2/26 (7.7%) being of a very high risk of myopathy and rhabdomyolysis as well (Supplementary File S2), in line with our internal observations from a cohort of >1,500 patients.
Actionable Variants Involved in Cardiovascular Diseases and Cancer
According to our protocol, we proceeded with the analysis of “actionable” variants (Table 2 and Supplementary File S2). Interestingly, more than one third of healthy individuals were carriers of pathogenic/potentially pathogenic variants leading to different types of arrhythmias and hereditary cancer, which are known to have incomplete penetrance.
For instance, patient 2 was a carrier of a pathogenic variant (c.566G > T, p.Arg189Ile; rs199473381) in the KCNJ2 gene, which has been associated with congenital long QT syndrome (Goldenberg and Moss, 2008). Follow-up EKG revealed visible abnormalities in the heart rhythm and the patient underwent further diagnostics. Patient 20 harbored a rare pathogenic/likely pathogenic variant (c.839C > T, p.Ala280Val; rs72552291) in the GPD1L gene that has been shown to decrease inward SCN5A Na + current and cause Brugada syndrome (Pfahnl et al., 2007). The patient underwent a regular cardiac exam, and EKG showed no visible abnormalities; since the patient was taking lithium therapy (which could conceivably unmask Brugada syndrome), advice was given to discuss this with their clinical psychiatrist and cardiologist. Furthermore, in patient 32 we discovered a variant (c.253G > A, p.Asp85Asn, and rs1805128) in the KCNE1 gene that has been reported to be associated with long QT syndrome (Paulussen et al., 2004). During the post-test genetic counseling, the patient disclosed that a member of their close family had passed away “due to complications from arrhythmia.” Other patients were also found to carry variants associated with long QT or other channelopathies; patient 12 and their parent carried a pathogenic variant (c.914G > C; p.Trp305Ser; rs120074186) in the KCNQ1 gene, whilst in patient 30 we identified a potentially pathogenic variant (c.5434C > T; p.Arg1812Trp; rs121912706) in the ANK2 gene associated with sudden death of the young (Methner et al., 2016). Finally, in patient 43 and patient 56 we found a combination of variants (Marburg I and F5 Leiden, F2 and F5 Leiden, respectively) that might significantly increase the risk of thrombosis (Voorberg et al., 1994; Poort et al., 1996; Hoppe et al., 2005). All patients were advised to consult a specialist and conduct follow-up studies if deemed necessary.
In regard to cancer, in patient 52, we discovered the presence of variants (c.1437_1439delGGA, p.Glu480del, and rs587778541) in the MUTYH gene and (c.470T > C, p.Ile157Thr, and rs1787996) in the CHEK2 gene. The same CHEK2 mutation was detected in patient 92. The variant in MUTYH is pathogenic and leads to MUTYH-Associated Polyposis in a recessive manner. The presence of the variant in a heterozygous format might slightly (1.5 times) increase the risk of colorectal cancer (Nielsen et al., 1993). The CHEK2 variant has been reported to increase the risk of different types of cancer 2–3 times (Han et al., 2013). The patient was advised to consult a specialist and discuss a screening protocol. Next, patient 84 harbored the variant (c.511A > G, p.Ile171Val, and rs61754966) in the NBN gene, which is a low penetrance risk factor for cancer development (Gao et al., 2013); the patient reported having a family history of breast and pancreatic cancer. Finally, we detected a potentially pathogenic variant (c.3920T > A, p.Ile1307Lys, and rs1801155) in the APC gene in patient 91 (Leshno et al., 2016), who is currently undergoing follow-up diagnostics. All patients were advised to consult a specialist and conduct follow-up studies if deemed necessary.
Evaluation of Potentially Pathogenic Variants of Uncertain Significance (VUS)
By analyzing VUS with rare population frequency meeting ACMG pathogenicity criteria (Richards et al., 2015) we uncovered many VUS in medium- or high-penetrance genes (Supplementary File S2). For instance, patient 56 is a carrier of (c.2423A > G, p.Tyr808Cys; rs746368140) in the TGFBR3 gene. The involvement of the TGF-beta pathway has been reported in pathologies such as familial thoracic aortic aneurysm and dissection (familial TAAD; Milewicz and Regalado, 1993). The father of patient 56 was diagnosed with a thoracic and abdominal aortic aneurysm and underwent valve-sparing root replacement (Tirone-David procedure). The patient was advised to follow regular cardiovascular check-ups. Patient 75 is a carrier of VUS (c.1755dupA, p.Glu586fs, and rs751465048) in the MLH3 gene, which is part of the MMR machinery associated with Lynch syndrome (Peltomaki, 2003). The patient already had benign tumors removed from their breast and nose, in the past. The patient was advised to consult a specialist. Finally, patient 84 is a carrier of (c.3145G > A, p.Gly1049Ser, and rs778181932) in the FBN1 gene, which could be possibly associated with TAAD; the patient reported a history of sudden death in their close family.
Discussion
The central tenet of personalized medicine is proactive care of patients based on the combined information and insights provided by omics approaches, lifestyle, environmental factors, and family history. To aid the implementation of precision genomics locally into our hospital, we have outlined a workflow centered around filtering, stratification in groups, and interpretation of genetic variants that can be readily applied in any genetic lab. By applying these strategies of variant-centric, gene-centric, and VUS-centric filtering, we were able to peer into the genetic constitution of 94 patients and make initial assessments of their carrier status, pharmacogenetics profile, and genetic risk of developing rare and common disorders.
Our experience demonstrates that the implementation of genomic profiling into real-life clinical practice can provide molecular and physiological information of medical significance, although many challenges remain to be addressed (Carter and He, 2016). To begin, serious efforts should be made to improve the knowledge of physicians and raise awareness for patients and the general public about the benefits and pitfalls of pre-emptive genomic testing, especially in the context of the current genetic knowledge. Second, standardization and defined guiding principles are necessary for both the technical and interpretational side of genomics in medicine. A list of guidelines (benchmarks) should be set for the minimal quality and coverage of sequencing data. For instance, currently WES is the most cost-effective approach, but it is limited to the protein-coding regions of the genome; as WGS sequencing costs continue to plummet this will likely lead to a rise in the popularity of WGS, which generates more uniform coverage of both coding and non-coding regions of the genome, relevant for monogenic as well as polygenic disorders. In addition, standardized algorithms for variant calling in clinical settings should be recommended. Moreover, more standardized approaches for filtering and distillation of relevant information, especially methods for calculation of polygenic scores, as well as balanced reporting of valuable information and VUS, and support tools for clinical interpretation, should be designed (Carter and He, 2016). Third, our analytical workflow based on filtering and virtual gene panels is readily applicable but still has a lot of space for improvement. For example, the virtual gene lists should undergo a process of constant curation and improvements from experts in the relevant subspecialties in order to get better informed, non-redundant, and more optimal lists of genes. Another limitation is that our focused study did not provide insights in regard to the cost-effectiveness of genetic testing, as well as the perceived value by both physicians and patients in a controlled and systematic manner. In order to objectively quantify the value of proactive genetic testing, longitudinal follow-up approaches are necessary.
Finally, many complex diseases, such as diabetes, cancer, and some neurological, cardiovascular, and psychiatric disorders, likely involve a large number of different genes and environmental factors (Hindorff et al., 2009; Ashley et al., 2010; De La Vega and Bustamante, 2018; Torkamani et al., 2018). These caveats sometimes might lead to unnecessary follow-up diagnostic measures and wastefulness of resources. Currently, the greatest value of genomic approaches lies in the detection of lower frequency moderate to high penetrance variants, which are easier to interpret and are better characterized due to their more resonant effects (Doble et al., 2017).
In conclusion, by establishing a balanced filtering pipeline, we set the foundation for the integration of genomics in mainstream clinical practice. The valuable insights and experiences we have obtained can have a bearing in future systematic and longitudinal follow-up studies.
Data Availability Statement
The datasets generated for this study can be found in Zenodo 10.5281/zenodo.3830498 and url: https://doi.org/10.5281/zenodo.3830498.
Ethics Statement
Written and signed informed consent for participation and publication of data was obtained from all subjects or their legal guardians in this study. Written and signed informed consent for participation and publication of data was obtained from the legal guardians of all patients under the age of eighteen. The ethics committee of the Zan Mitrev Clinic waived the need for IRB approval, deeming written, and signed informed consent sufficient.
Author Contributions
GK conceived and designed the study. GK, SM, AS, MS, KJ, and MJ analyzed and interpreted the data. SM and AS participated in the genetic counseling process. IK and ZM contributed to the recruitment of patients and contributed intellectually. GK and RR wrote the manuscript. All authors contributed to the improvement of the manuscript and read the final version of the manuscript.
Funding
This study was carried out as part of the routine clinical work at the Zan Mitrev Clinic.
Conflict of Interest
GK, AS, MJ, and IK are currently employed by the company Bio Engineering LLC. SM, RR, and ZM are currently employed by the private Zan Mitrev Clinic. MS was formerly employed by the Zan Mitrev Clinic. KJ did an internship at the Zan Mitrev Clinic.
Supplementary Material
The Supplementary Material for this article can be found online at: https://www.frontiersin.org/articles/10.3389/fgene.2020.00619/full#supplementary-material
FILE S1 | Contains lists of genes for each category of virtual panels.
FILE S2 | Contains variant information related to patient information, methodology, rare diseases, drug-response, actionable conditions, and VUS.
Abbreviations
ACMG59, incidental findings in 59 genes recommended by American College of Medical Genetics and Genomics; CES, clinical exome sequencing; GWAS, genome-wide association studies; indel, insertion or deletion; SNV, single nucleotide variant; VUS, variants of uncertain significance; WES, whole-exome sequencing; WGS, whole-genome sequencing.
Footnotes
- ^ https://www.bioinformatics.babraham.ac.uk/projects/fastqc/
- ^ http://clinvar.com/
- ^ http://www.hgmd.cf.ac.uk/
- ^ http://clinvitae.invitae.com/
- ^ https://www.clinicalgenome.org/
- ^ www.pharmgkb.org
- ^ https://www.pharmgkb.org/
References
Ashley, E. A. (2016). Towards precision medicine. Nat. Rev. Genet. 17, 507–522. doi: 10.1038/nrg.2016.86
Ashley, E. A., Butte, A. J., Wheeler, M. T., Chen, R., Klein, T. E., Dewey, F. E., et al. (2010). Clinical assessment incorporating a personal genome. Lancet 375, 1525–1535. doi: 10.1016/S0140-6736(10)60452-7
Bylstra, Y., Davila, S., Lim, W. K., Wu, R., Teo, J. X., Kam, S., et al. (2019). Implementation of genomics in medical practice to deliver precision medicine for an Asian population. NPJ Genom. Med. 4:12. doi: 10.1038/s41525-019-0085-8
Carter, T. C., and He, M. M. (2016). Challenges of identifying clinically actionable genetic variants for precision medicine. J. Healthc Eng. 2016, 3617572. doi: 10.1155/2016/3617572
De La Vega, F. M., and Bustamante, C. D. (2018). Polygenic risk scores: a biased prediction? Genome Med. 10:100. doi: 10.1186/s13073-018-0610-x
Doble, B., Schofield, D. J., Roscioli, T., and Mattick, J. S. (2017). Prioritising the application of genomic medicine. NPJ Genom. Med. 2:35. doi: 10.1038/s41525-017-0037-0
Gao, P., Ma, N., Li, M., Tian, Q. B., and Liu, D. W. (2013). Functional variants in NBS1 and cancer risk: evidence from a meta-analysis of 60 publications with 111 individual studies. Mutagenesis 28, 683–697. doi: 10.1093/mutage/get048
Goldenberg, I., and Moss, A. J. (2008). Long QT syndrome. J. Am. Coll Cardiol. 51, 2291–2300. doi: 10.1016/j.jacc.2008.02.068
Goodwin, S., McPherson, J. D., and McCombie, W. R. (2016). Coming of age: ten years of next-generation sequencing technologies. Nat. Rev. Genet. 17, 333–351. doi: 10.1038/nrg.2016.49
Han, F. F., Guo, C. L., and Liu, L. H. (2013). The effect of CHEK2 variant I157T on cancer susceptibility: evidence from a meta-analysis. DNA Cell Biol. 32, 329–335. doi: 10.1089/dna.2013.1970
Hindorff, L. A., Sethupathy, P., Junkins, H. A., Ramos, E. M., Mehta, J. P., Collins, F. S., et al. (2009). Potential etiologic and functional implications of genome-wide association loci for human diseases and traits. Proc. Natl. Acad. Sci. U.S.A. 106, 9362–9367. doi: 10.1073/pnas.0903103106
Hoppe, B., Tolou, F., Radtke, H., Kiesewetter, H., Dorner, T., and Salama, A. (2005). Marburg I polymorphism of factor VII-activating protease is associated with idiopathic venous thromboembolism. Blood 105, 1549–1551. doi: 10.1182/blood-2004-08-3328
Kalia, S. S., Adelman, K., Bale, S. J., Chung, W. K., Eng, C., Evans, J. P., et al. (2017). Recommendations for reporting of secondary findings in clinical exome and genome sequencing, 2016 update (ACMG SF v2.0): a policy statement of the American College of Medical Genetics and Genomics. Genet. Med. 19, 249–255. doi: 10.1038/gim.2016.190
Klinceva, M., Mehandziska, S., Idoski, E., Stajkovska, A., Stavrevska, M., Jakovleva, K., et al. (2018). CYP2C19-dependent clopidogrel resistance is a possible cause for coronary stent and peripheral bypass occlusion. Eur. Heart J. 39:ehy564.961.
Leshno, A., Shapira, S., Liberman, E., Kraus, S., Sror, M., Harlap-Gat, A., et al. (2016). The APC I1307K allele conveys a significant increased risk for cancer. Int. J. Cancer 138, 1361–1367. doi: 10.1002/ijc.29876
Li, H., and Durbin, R. (2009). Fast and accurate short read alignment with burrows-wheeler transform. Bioinformatics 25, 1754–1760. doi: 10.1093/bioinformatics/btp324
MacArthur, J., Bowler, E., Cerezo, M., Gil, L., Hall, P., Hastings, E., et al. (2017). The new NHGRI-EBI Catalog of published genome-wide association studies (GWAS Catalog). Nucleic Acids Res. 45, D896–D901. doi: 10.1093/nar/gkw1133
Manolio, T. A., Chisholm, R. L., Ozenberger, B., Roden, D. M., Williams, M. S., Wilson, R., et al. (2013). Implementing genomic medicine in the clinic: the future is here. Genet. Med. 15, 258–267. doi: 10.1038/gim.2012.157
McLaughlin, H. M., Ceyhan-Birsoy, O., Christensen, K. D., Kohane, I. S., Krier, J., Lane, W. J., et al. (2014). A systematic approach to the reporting of medically relevant findings from whole genome sequencing. BMC Med. Genet. 15:134. doi: 10.1186/s12881-014-0134-1
Methner, D. N., Scherer, S. E., Welch, K., Walkiewicz, M., Eng, C. M., Belmont, J. W., et al. (2016). Postmortem genetic screening for the identification, verification, and reporting of genetic variants contributing to the sudden death of the young. Genome Res. 26, 1170–1177. doi: 10.1101/gr.195800.115
Milewicz, D. M., and Regalado, E. (1993). “Heritable thoracic aortic disease overview,” in GeneReviews®, eds M. P. Adam, H. H. Ardinger, R. A. Pagon, S. E. Wallace, L. J. H. Bean, K. Stephens, et al. (Seattle, WA: University of Washington, Seattle).
Nielsen, M., Lynch, H., Infante, E., and Brand, R. (1993). “MUTYH-Associated Polyposis,” in GeneReviews®, eds M. P. Adam, H. H. Ardinger, R. A. Pagon, S. E. Wallace, L. J. H. Bean, K. Stephens, et al. (Seattle, WA: University of Washington, Seattle).
Paulussen, A. D., Gilissen, R. A., Armstrong, M., Doevendans, P. A., Verhasselt, P., Smeets, H. J., et al. (2004). Genetic variations of KCNQ1, KCNH2, SCN5A, KCNE1, and KCNE2 in drug-induced long QT syndrome patients. J. Mol. Med. 82, 182–188. doi: 10.1007/s00109-003-0522-z
Peltomaki, P. (2003). Role of DNA mismatch repair defects in the pathogenesis of human cancer. J. Clin. Oncol. 21, 1174–1179. doi: 10.1200/JCO.2003.04.060
Pfahnl, A. E., Viswanathan, P. C., Weiss, R., Shang, L. L., Sanyal, S., Shusterman, V., et al. (2007). A sodium channel pore mutation causing Brugada syndrome. Heart Rhythm. 4, 46–53. doi: 10.1016/j.hrthm.2006.09.031
Poort, S. R., Rosendaal, F. R., Reitsma, P. H., and Bertina, R. M. (1996). A common genetic variation in the 3’-untranslated region of the prothrombin gene is associated with elevated plasma prothrombin levels and an increase in venous thrombosis. Blood 88, 3698–3703. doi: 10.1182/blood.v88.10.3698.bloodjournal88103698
Richards, S., Aziz, N., Bale, S., Bick, D., Das, S., Gastier-Foster, J., et al. (2015). Standards and guidelines for the interpretation of sequence variants: a joint consensus recommendation of the American college of medical genetics and genomics and the association for molecular pathology. Genet. Med. 17, 405–424. doi: 10.1038/gim.2015.30
Torkamani, A., Wineinger, N. E., and Topol, E. J. (2018). The personal and clinical utility of polygenic risk scores. Nat. Rev. Genet. 19, 581–590. doi: 10.1038/s41576-018-0018-x
Trujillano, D., Oprea, G. E., Schmitz, Y., Bertoli-Avella, A. M., Abou Jamra, R., and Rolfs, A. (2017). A comprehensive global genotype-phenotype database for rare diseases. Mol. Genet. Genomic Med. 5, 66–75. doi: 10.1002/mgg3.262
Vassy, J. L., Christensen, K. D., Schonman, E. F., Blout, C. L., Robinson, J. O., Krier, J. B., et al. (2017). The impact of whole-genome sequencing on the primary care and outcomes of healthy adult patients: a pilot randomized trial. Ann. Intern. Med. 167, 159–169. doi: 10.7326/M17-0188
Voorberg, J., Roelse, J., Koopman, R., Buller, H., Berends, F., ten Cate, J. W., et al. (1994). Association of idiopathic venous thromboembolism with single point-mutation at Arg506 of factor V. Lancet 343, 1535–1536. doi: 10.1016/s0140-6736(94)92939-4
Yang, H., and Wang, K. (2015). Genomic variant annotation and prioritization with ANNOVAR and wANNOVAR. Nat. Protoc. 10, 1556–1566. doi: 10.1038/nprot.2015.105
Keywords: exome, genome, personalized medicine, precision genomics, clinical practice, implementation
Citation: Mehandziska S, Stajkovska A, Stavrevska M, Jakovleva K, Janevska M, Rosalia R, Kungulovski I, Mitrev Z and Kungulovski G (2020) Workflow for the Implementation of Precision Genomics in Healthcare. Front. Genet. 11:619. doi: 10.3389/fgene.2020.00619
Received: 14 October 2019; Accepted: 21 May 2020;
Published: 30 June 2020.
Edited by:
Manuel Corpas, Cambridge Precision Medicine, United KingdomReviewed by:
Chiara Fabbri, King’s College London, United KingdomKaryn Megy, University of Cambridge, United Kingdom
Copyright © 2020 Mehandziska, Stajkovska, Stavrevska, Jakovleva, Janevska, Rosalia, Kungulovski, Mitrev and Kungulovski. This is an open-access article distributed under the terms of the Creative Commons Attribution License (CC BY). The use, distribution or reproduction in other forums is permitted, provided the original author(s) and the copyright owner(s) are credited and that the original publication in this journal is cited, in accordance with accepted academic practice. No use, distribution or reproduction is permitted which does not comply with these terms.
*Correspondence: Goran Kungulovski, Z29yYW5AYmlvZW5naW5lZXJpbmcubWs=; Z29yYW4ua3VuZ3Vsb3Zza2lAem1jLm1r