- 1Department of Gastroenterology and Hepatology, Tianjin Medical University General Hospital, Tianjin, China
- 2General Data Technology Co., Ltd., Tianjin, China
- 3Department of Gastroenterology, Tianjin Union Medical Center, Tianjin, China
For datasets of gastric cancer collected by TCGA (The Cancer Genome Atlas) and GEO (Gene Expression Omnibus) repositories, we applied a bioinformatics approach to obtain expression data for the ISLR (immunoglobulin superfamily containing leucine-rich repeat) gene, which is highly expressed in gastric cancer tissues and closely associated with clinical prognosis. Although we did not observe an overall association of ISLR mutation, high expression or copy number variation with survival, hypomethylation of four methylated sites (assessed by the probes cg05195566, cg17258195, cg09664357, and cg07297039) of ISLR was negatively correlated with high expression levels of ISLR and was associated with poor clinical prognosis. In addition, we detected a correlation between ISLR expression and the infiltration levels of several immune cells, especially CD8+ T cells, macrophages and dendritic cells. We also identified a series of genes that were positively and negatively correlated with ISLR expression based on the TCGA-STAD, GSE13861, and GSE29272 datasets. Principal component analysis and random forest analysis were employed to further screen for six hub genes, including ISLR, COL1A2, CDH11, SPARC, COL3A1, and COL1A1, which exhibited a good ability to differentiate between tumor and normal samples. GO (Gene Ontology) and KEGG (Kyoto Encyclopedia of Genes and Genomes) pathway and gene set enrichment analysis data also suggested a potential relationship between ISLR gene expression and epithelial-mesenchymal transition (EMT). ISLR expression was negatively correlated with sensitivity to PX-12 and NSC632839. Taken together, these results show that the ISLR gene is involved in gastric carcinogenesis, and the underlying molecular mechanisms may include DNA methylation, EMT, and immune cell infiltration.
Introduction
The Cancer Genome Atlas (TCGA), a publicly funded project, archives multiple types of genomic data from various types of cancer, including gene expression, mutation, copy number variation (CNV), genome methylation, and clinical data (Cancer Genome Atlas Research Network, 2014; Tomczak et al., 2015; Wang et al., 2016). In addition, GEO (Gene Expression Omnibus) molecular datasets also offer many clinical cancer-related gene expression data (Barrett et al., 2013; Clough and Barrett, 2016). The complicated pathogenesis of gastric cancer involves multiple clinical prognosis-associated oncogenes. Previously, based on the datasets of gastric cancer within TCGA and GEO, we identified the ISLR (immunoglobulin superfamily containing leucine-rich repeat) gene by means of principal component analysis (PCA) and random forest analysis (data not shown), which showed a high expression level in gastric cancer tissues and was closely linked to clinical prognosis. The present study attempted to investigate the possible oncogenic roles of the ISLR gene in the pathogenesis and prognosis of gastric cancer.
The human ISLR gene is situated on human chromosome 15q23-q24 (Nagasawa et al., 1997). The human ISLR protein, a member of the Ig superfamily, contains a leucine-rich repeat (LRR) with conserved flanking sequences and a C2-type immunoglobulin (Ig)-like domain (Nagasawa et al., 1997). The ISLR protein has been reported to be involved in some biological events, such as cell replicative senescence of human dermal fibroblasts (Yoon et al., 2004), embryo development (Homma et al., 2009), and Gaucher disease (Lugowska et al., 2019). However, no study has mainly investigated the potential functional relationship between the ISLR gene and cancer events thus far.
In the current study, we elucidated the underlying molecular mechanisms of the ISLR gene in gastric carcinogenesis from the perspectives of genetic mutation, copy number variation, DNA methylation, immune cell infiltration, expression correlation, pathway enrichment and drug sensitivity for the first time.
Materials and Methods
Expression Analysis
We first investigated the expression level of the ISLR gene between gastric cancer and negative controls samples within the TCGA-STAD (The Cancer Genome Atlas stomach adenocarcinoma) cohort and the GTEx (Genotype Tissue Expression) database using the online tool GEPIA21 (Tang et al., 2019). A log2 (FC) (fold change) cutoff = 1, a P-value cutoff = 0.01, and a jitter size = 4 were set. Log2 [TPM (transcripts per million) + 1] values were used for log-scale. Gene expression data were visualized by the “boxplot” function of the R language (for the cancer and control samples) or the “vioplot” R package [for the pathological stage (stages I, II, III, and IV)]. Then, we obtained the expression dataset of “Chen et al. (2003),” which contains a total of 11 diffuse gastric adenocarcinoma and 24 normal control samples, by means of Oncomine2. The log2 (median-centered intensity) data were visualized by GraphPad Prism software, version 5.01 (San Diego).
Furthermore, we utilized the “GEOquery” R package to obtain the available expression and group datasets in GSE13861 and GSE29272. The difference in expression of the ISLR gene between gastric cancer cases and normal controls was analyzed by the t.test function of the compare_means and visualized by the ggviolin function of the “ggpubr” R package. We then used the wilcox.test function of the compare_means with the setting of “paired = TRUE” to analyze the difference in expression between the gastric tumor tissues and adjacent normal tissues and displayed the results using the ggdotchart function of the “ggpubr” R package. R language software [R-3.6.1, 64-bit]3 was used.
Survival Curve Analysis
We conducted OS (overall survival) and DFS (disease-free survival) analyses of gastric cancer cases in the TCGA-STAD cohort according to the expression status of the ISLR gene through GEPIA2. A group cutoff of “quartile” was set, and the Kaplan–Meier curve was plotted. We also pooled the gastric cancer cases in the GSE14210, GSE15459, GSE22377, GSE29272, GSE51105, and GSE62254 datasets for the OS, FP (first progression), and PPS (post progression survival) analyses using the Kaplan–Meier plotter tool (Szasz et al., 2016). The automatically selected best cutoff was used. We considered clinical factors including sex (female or male), pathologic stage (stages 1∼4, T2∼4, N0∼3, M0∼1), HER2 status (negative or positive), Lauren classification (intestinal, diffuse, or mixed), differentiation (poor, moderate, or well), and treatment (surgery alone, 5-Fu-based adjuvant or other adjuvant). Furthermore, we employed the Coxph (Cox proportional hazard) model to determine the correlation between ISLR expression and the clinical prognosis of gastric cancer cases in TCGA-STAD through the web-based tool TIMER (Tumor Immune Estimation Resource) (Li et al., 2016, 2017). Clinical factors, including age, sex, race, stage, and tumor purity, were included in the Coxph model.
Genetic Alteration Analysis
The alteration frequency of the ISLR gene in several studies of gastric cancer, including the TCGA pub (2014), PanCan 2018 (Ellrott et al., 2018; Gao et al., 2018; Hoadley et al., 2018; Liu J. et al., 2018; Sanchez-Vega et al., 2018; Taylor et al., 2018; Bhandari et al., 2019), TCGA cohort, Pfizer and UHK (Wang et al., 2014), UHK (Wang et al., 2011), and U Tokyo (Kakiuchi et al., 2014) studies, was analyzed via the cBioPortal database4. We provided data of genomic alteration type, mutation site profile, OS and D/PFS (disease/progression-free survival) analyses. In addition, we generated a MEXPRESS plot (Koch et al., 2015, 2019) to analyze the CNV types of the ISLR gene. The correlation between CNV and the expression level of ISLR was also analyzed by Pearson’s test. The overall survival analysis according to the CNV status of the ISLR gene (masked CNV ≥ or < −0.019) was performed through UCSC Xena5. The log-rank test was performed.
DNA Methylation Analysis
We analyzed the methylation status of ISLR DNA in the gastric cases in the TCGA-STAD cohort through MEXPRESS (Koch et al., 2015, 2019). Pearson’s test was used to determine the correlation between methylation and the expression level of the ISLR gene. We determined correlation coefficients (R) and Benjamini–Hochberg-adjusted P-values regarding different methylation probes, such as cg05195566, cg15480336, cg02077702, and cg16926502. The waterfall plot of the methylation level of the ISLR gene and Kaplan–Meir plots of the relationship between ISLR DNA hypermethylation/hypomethylation and cancer survival were generated with the MethSurv tool (Modhukur et al., 2018).
Immune Cell Infiltration Analysis
We used GEPIA2 to perform pairwise gene correlation analysis between ISLR expression and the signatures of the following immune cells: macrophages, TAMs (tumor-associated macrophages), dendritic cells, monocytes, NK (natural killer) cells; mast cells, neutrophils, eosinophils, basophils, B cells, Th1 cells, Th2 cells, Th17 cells, CD8+ T cells, Tfh (follicular helper T) cells, resting Treg cells, effector Treg cells, and exhausted T cells. Then, based on a TIMER2 approach, we calculated immune infiltration estimations for TCGA-STAD samples with the TIMER, CIBERSORT, CIBERSORT-ABS, QUANTISEQ, MCPCOUNTER, XCELL, and EPIC algorithms. A heatmap with the purity-adjusted Spearman’s rho value was obtained by the “pheatmap” R package. Specific scatter plots were provided. In addition, the correlation between ISLR SNVs and the level of infiltrating immune cells, including dendritic cells, neutrophils, CD8+ T cells, CD4+ T cells, B cells, and macrophages, was also investigated by the TIMER tool.
ISLR-Correlated Gene Cluster Analysis
We utilized the “TCGAbiolinks” R package to download the gene expression and clinical information data of TCGA-STAD cohorts from the TCGA database. Log2 [FPKM (Fragments per Kilobase Million) + 1] values were used for log-scale. The 25/75% quartile cutoff of ISLR expression in three datasets, including TCGA-STAD, GSE13861, and GSE29272, was used to define high and low groups of ISLR expression. We then analyzed the ISLR-correlated genes through the “limma” R package. The positively or negatively correlated significant genes were visualized by the “ggplot2” R package. The “VennDiagram” R package was used to identify the common genes among TCGA-STAD, GSE13861, and GSE29272. Furthermore, the “clusterProfiler” and “pathview” R packages were used for the GO (Gene Ontology) and KEGG (Kyoto Encyclopedia of Genes and Genomes) enrichment analyses. The data were visualized by the functions cnetplot and dotplot. The GOCircle and chord plots using extracellular matrix-associated terms were visualized by the “GOplot” R package.
In addition, we performed ISLR-correlated GSEA (gene set enrichment analysis) and pathway activation/inhibition analyses through a LinkedOmics approach (Vasaikar et al., 2018). The following cutoffs were used: simulations = 500, minimum number of genes = 3, and rank criteria = FDR (false discovery rate). The pathway activity module presents the difference in ISLR expression between pathway activity groups (activation and inhibition) defined by pathway scores. The pathway activity module presents the difference in gene expression between pathway activity groups (activation and inhibition) defined by pathway scores.
Principal Component Analysis
Based on the above common differentially expressed genes, we used the prcomp function for principal component analysis (PCA) to classify the normal and tumor sections in the TCGA-STAD, GSE13861, and GSE29272 datasets. A scree plot was obtained by the plot function, and a three-dimensional map [principal component 1 (PC1), PC2, and PC3] was drawn using the “scatterplot3d” package.
After using the “VennDiagram” R package, common hub genes among TCGA-STAD, GSE13861, and GSE29272 were identified. Then, the cor function and “corrplot” R package were used for the Spearman correlation analysis of these hub genes. The scatter plots were then obtained by the “ggpubr” R package. The “factoextra” R package was utilized to show the principal component weight and to generate two-dimensional contribution maps of common hub genes.
Random Forest Analysis
Based on the above hub genes, we used the “randomForest” package (ntree = 500) to perform random forest modeling. The MDSplot function was used to obtain a multidimensional scale. The mean decrease accuracy and mean decrease Gini values were calculated by the ggdotchart function in the “ggpubr” package. Using the “pROC” package, the receiver operating characteristic (ROC) curve was plotted, and the area under the ROC curve (AUC) value was calculated.
Drug Sensitivity Analysis
The correlation between ISLR and sensitivity to small molecules and/or drugs was investigated using the GSCALite tool (Liu C. J. et al., 2018). Drug sensitivity and gene expression profiling data of cancer cell lines in the Cancer Therapeutics Response Portal (CTRP) were integrated for investigation (Rees et al., 2016; Liu C. J. et al., 2018). The correlation of ISLR gene expression with the small molecule/drug sensitivity (half-inhibitory concentration, IC50) was determined through a Spearman correlation analysis.
Results
Expression Analysis Data
First, the difference in ISLR gene expression between gastric cancer tissues and negative control tissues was measured. A total of 408 gastric cancer tissue samples in the TCGA-STAD cohort were included, and the adjacent tissues within TCGA-STAD and normal tissues in the GTEx database were included as negative controls (n = 211). As shown in Figure 1A, there was high expression of ISLR in the gastric cancer tumor samples (∗P < 0.05) compared with the controls. We further analyzed the difference in ISLR gene expression among different pathological stages of gastric cancer cases in the TCGA-STAD cohort and identified a positive correlation (Figure 1B, P = 2.1E-06). Then, based on the dataset reported by Chen et al. (2003), we observed that the expression level of the ISLR gene in 11 diffuse gastric adenocarcinoma cases was higher than that in 24 normal controls (Figure 1C, P = 1.8E-05). A similar expression difference between tumor and normal samples was detected in the GSE13861 dataset (Figure 1D, P = 2.4E-06). Moreover, we observed an obvious high expression level of ISLR in 164 gastric tumor tissues compared with 164 adjacent normal tissues within the GSE29272 dataset (Figure 1E, P < 2.2E-16). Collectively, these results indicated that the expression level of the ISLR gene in gastric cancer cases was higher than that in negative controls, which suggests the potential role of the ISLR gene in the etiology of gastric cancer.
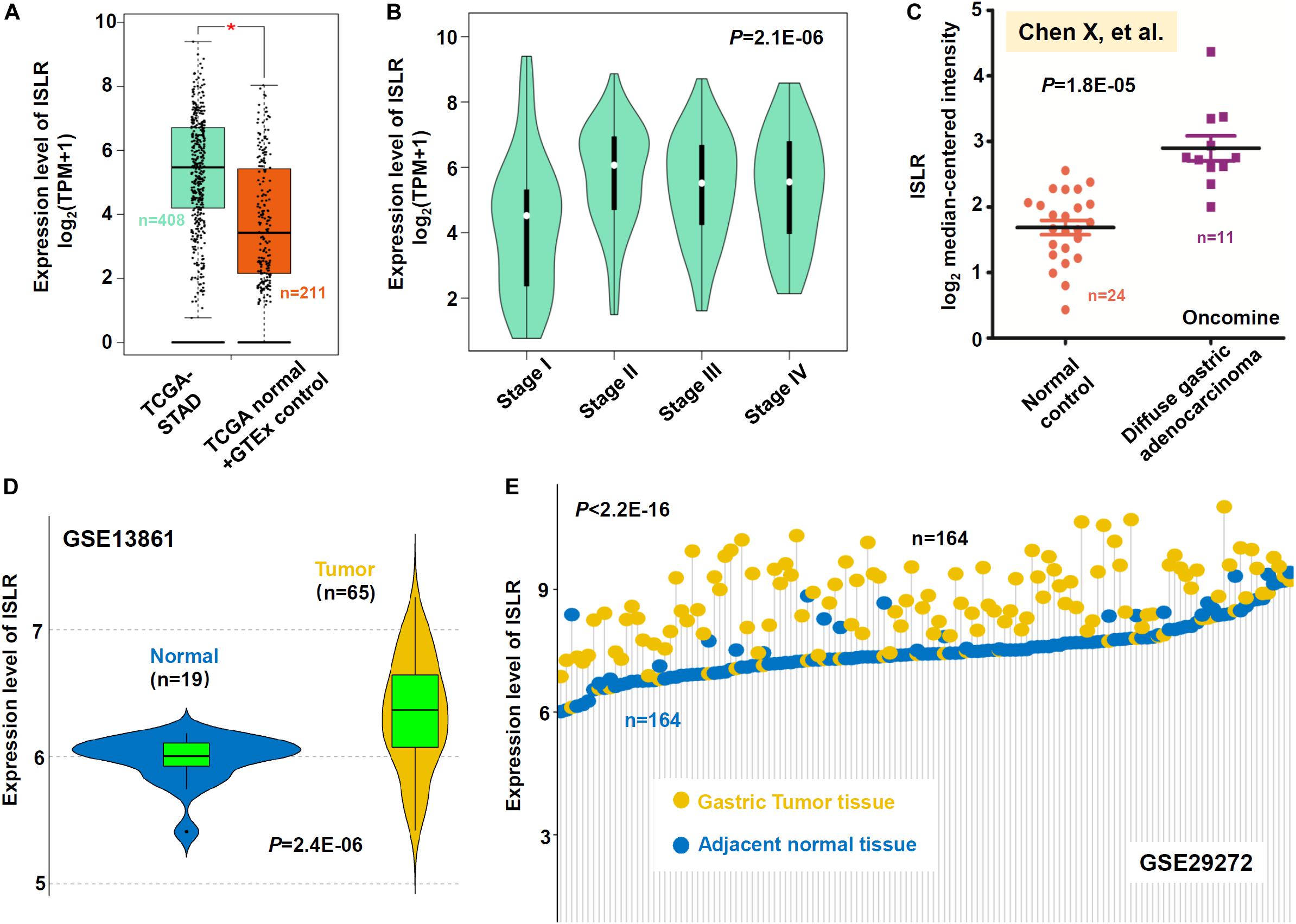
Figure 1. Expression analysis of the ISLR gene between gastric cancer and negative control samples. (A) Expression level of ISLR between gastric cancer tissues in the TCGA-STAD cohort (n = 408) and control tissues (n = 211). Normal tissues in both the TCGA and GTEx databases were included as negative controls. *P < 0.05. (B) The expression level of ISLR among different pathologic stages (stage I, II, III, and IV) was also analyzed through GEPIA2. We also compared the expression level of ISLR between diffuse gastric adenocarcinoma cases and normal controls from the studies of “Chen et al. (2003)” through the Oncomine database (C). The differences in ISLR gene expression between gastric cancer cases and normal controls in GSE13861 (D) and GSE29272 (E) were analyzed as well. Violin plots were used for GSE13861. Adjacent normal tissues were used for GSE29272, and the data were visualized by a dot plot.
Survival Curve Analysis Data
Next, we explored the correlation between ISLR expression patterns and clinical prognosis for gastric cancer cases in the TCGA-STAD cohort. As shown in Figure 2A, we observed lower rates of overall survival (P = 0.036) and disease-free survival (P = 0.016) in the high ISLR expression group than in the low ISLR expression group. We also pooled a total of six GSEA datasets for the clinical prognosis analyses. As shown in Figure 2B, there were lower overall survival (P = 3.1E-12), first progression (P = 6.2E-06), and post progression survival (P = 1.1E-16) rates in the ISLR high expression group than in the low expression group. Additionally, we fully considered the effect of different clinical factors (e.g., sex, pathologic stage, HER2 status, Lauren classification, differentiation and treatment) during the above analyses. Survival curve analyses were carried out when grouping the samples by the different clinical factors. As shown in Table 1, there was a relationship between high ISLR expression and poor overall survival (hazard ratio, HR > 1, P < 0.05) in most subgroups but not in the subgroups with poor (P = 0.21) or moderate (P = 0.086) differentiation, or stage 1 disease (P = 0.065). Surprisingly, for the 158 gastric cancer cases treated with 5-Fu-based adjuvant therapy, a high level of ISLR expression was linked to a better clinical prognosis than a low level of ISLR expression (Table 1, HR = 0.63, P = 0.013), indicating a possible connection of ISLR expression with drug sensitivity. We observed similar results in the correlation analysis of ISLR expression and first progression and post-progression survival (Supplementary Tables S1, S2). Moreover, we included the factors of tumor purity, age, sex, race, clinical stage, and ISLR expression in a Cox proportional hazard model and obtained a statistical correlation between high ISLR expression and poor clinical prognosis (Table 2, P = 0.018). These findings offer evidence regarding the relationship between ISLR expression and clinical outcomes. This led us to perform a more in-depth molecular mechanism study.
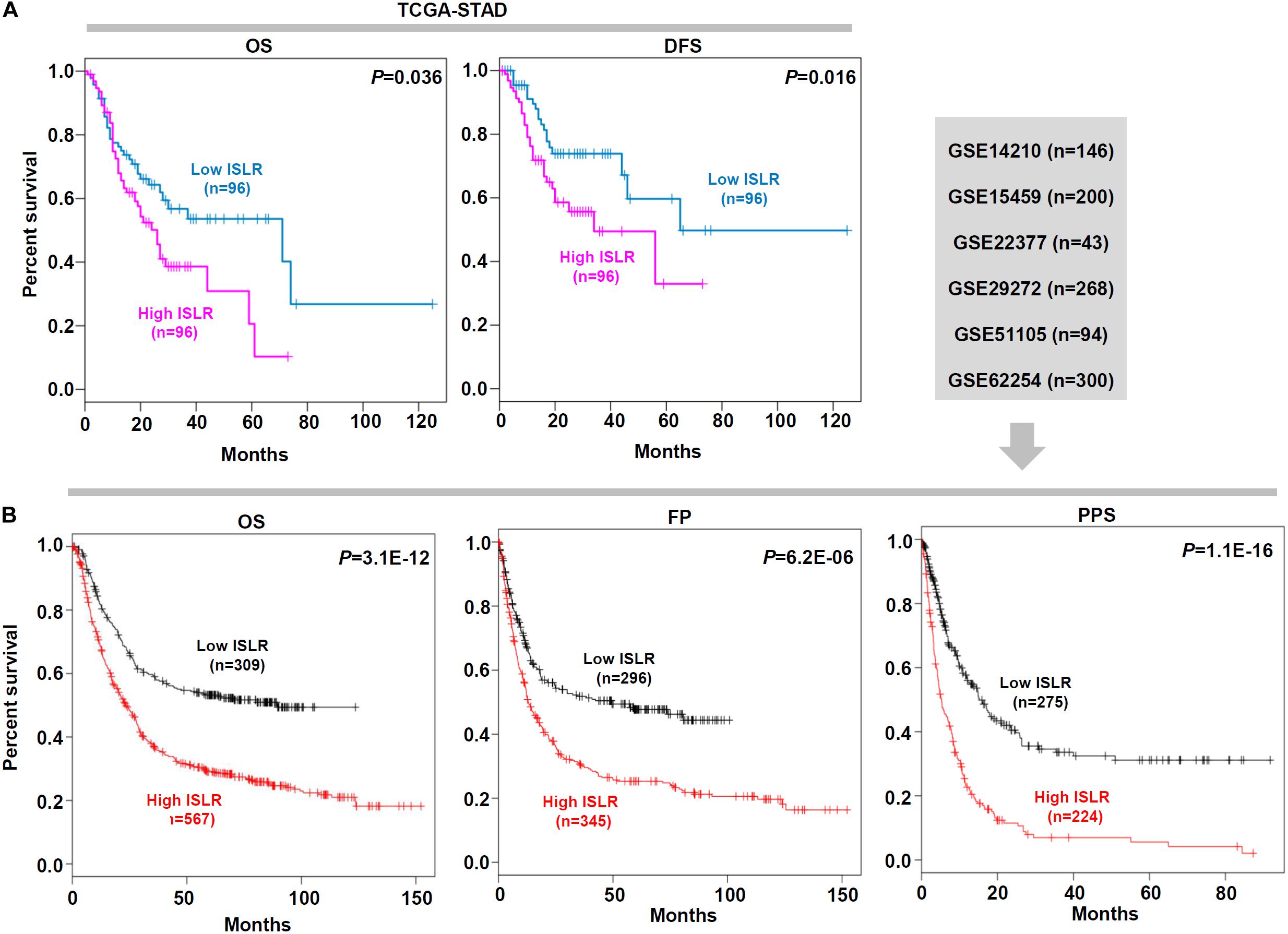
Figure 2. Survival curve analysis of the ISLR gene for gastric cancer cases. (A) Overall survival (OS) and disease-free survival (DFS) analyses according to the expression level of the ISLR gene were performed using gastric cancer cases in the TCGA-STAD cohort. (B) Based on the data of gastric cancer cases in GSE14210, GSE15459, GSE22377, GSE29272, GSE51105, and GSE62254, we also performed OS, first progression (FP), and post progression survival (PPS) analyses through Kaplan–Meier plotter.
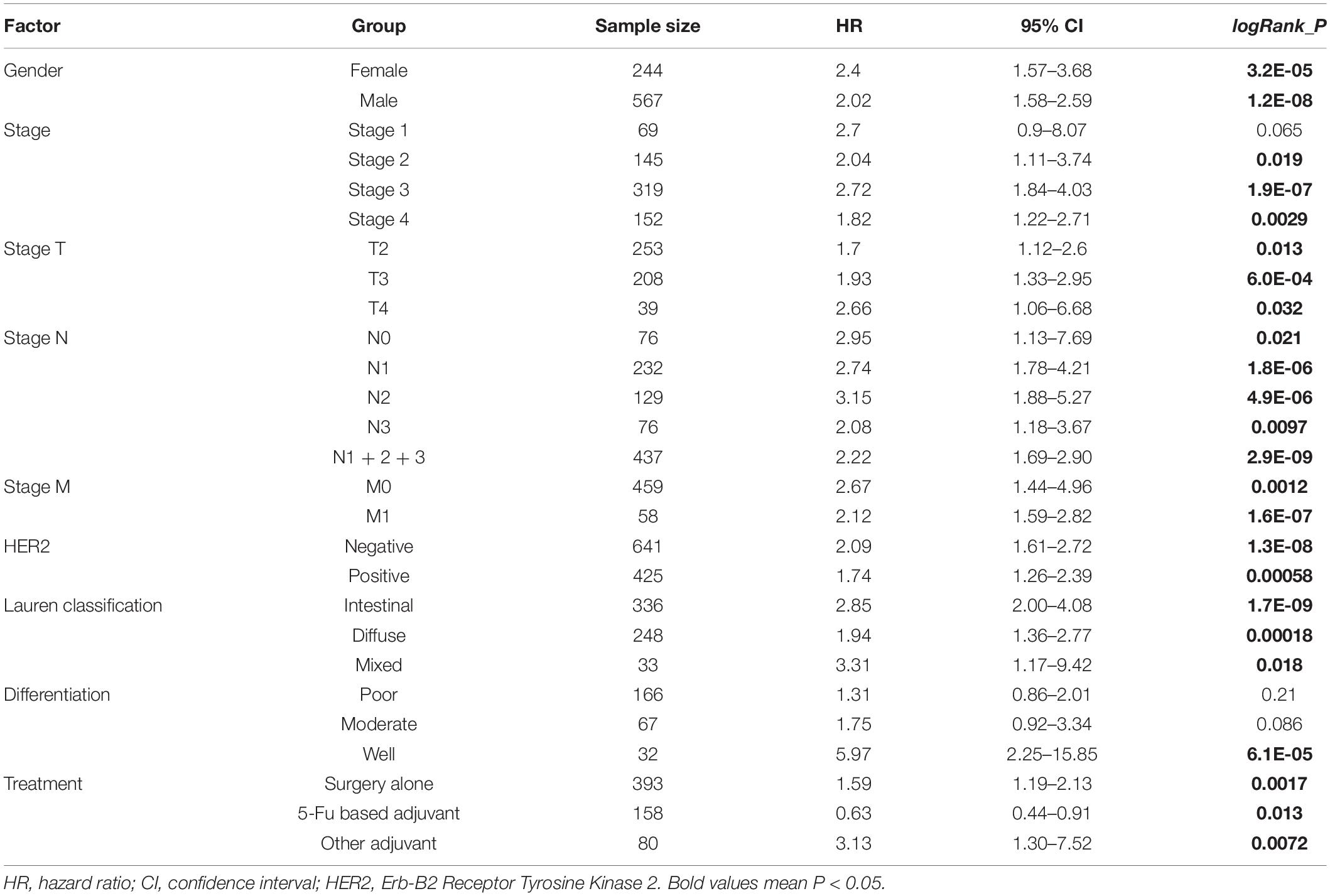
Table 1. Correlation of ISLR expression and the overall survival of gastric cancer patients in the GEO cohort (Kaplan–Meier plotter).
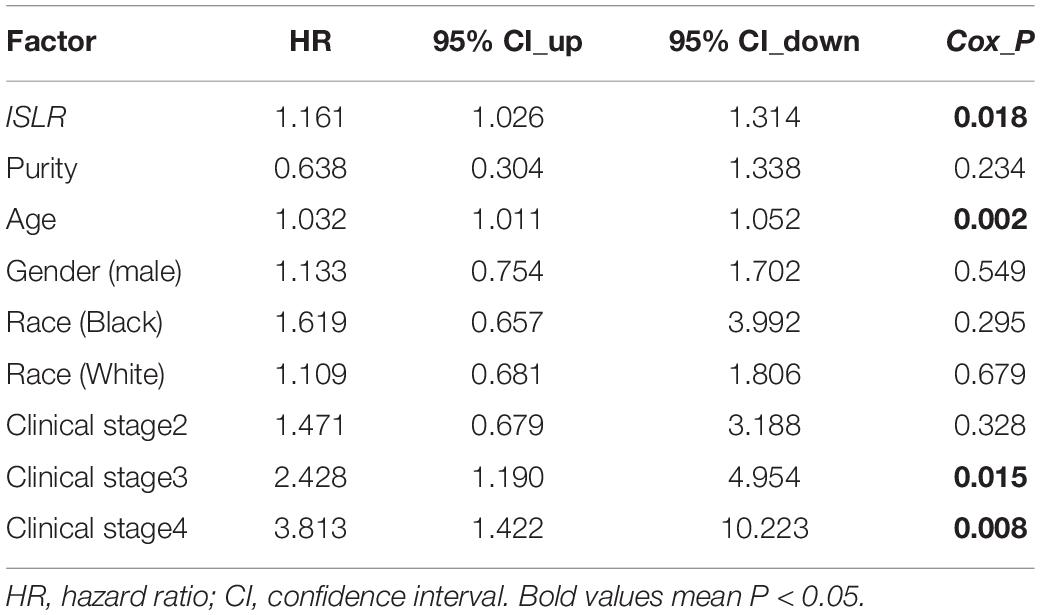
Table 2. Correlation of ISLR expression and the clinical prognosis of gastric cancer patients in the TCGA-STAD cohort (Cox proportional hazard model).
Genetic Alteration Analysis Data
We attempted to study the potential mechanism of the ISLR gene in the pathogenesis of gastric cancer in terms of gene mutation and copy number variation. As shown in Figure 3A, we detected the mutation frequency in six groups of gastric cancer cases through the cBioPortal database. There was a low mutation rate (∼2%) of ISLR in the cases in the TCGA-STAD, TCGA pub (2014), and PanCan 2018 (Ellrott et al., 2018; Gao et al., 2018; Hoadley et al., 2018; Liu J. et al., 2018; Sanchez-Vega et al., 2018; Taylor et al., 2018; Bhandari et al., 2019) cohorts and no mutation in gastric cancer cases in the Pfizer and UHK (Wang et al., 2014), UHK (Wang et al., 2011), and U Tokyo (Kakiuchi et al., 2014) cohorts. The type and location of specific mutations, with the most frequent missense mutation being R87C/H (n = 6), are shown in Figure 3B. Additionally, we did not observe a statistically significant correlation between ISLR gene mutation and the OS rate (Figure 3C, P = 0.978) or the D/PFS rate (Figure 3C, P = 0.087).
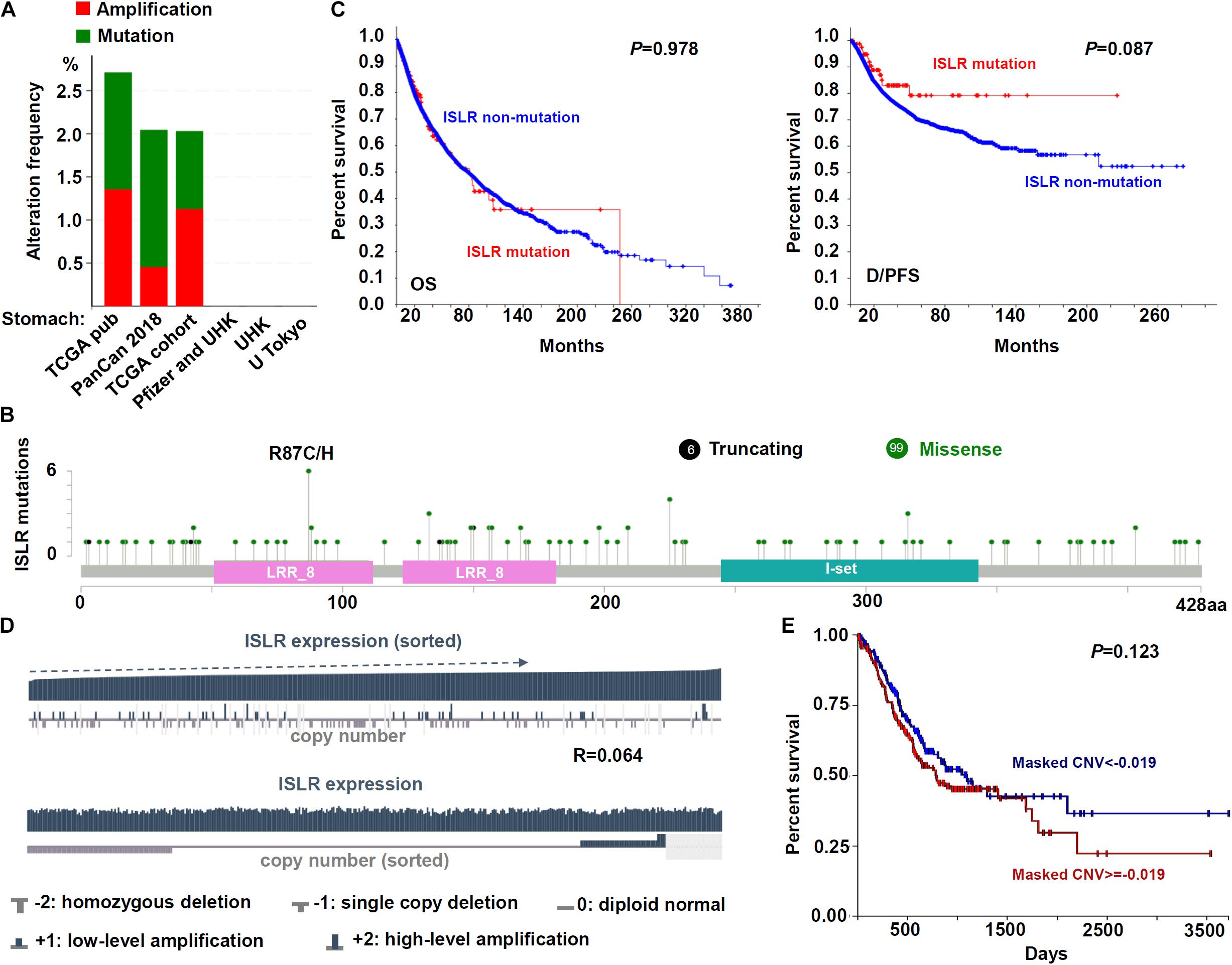
Figure 3. Mutation and CNV analyses of the ISLR gene. (A) The alteration frequency of the ISLR gene for the cases in several studies as analyzed through cBioPortal. (B) The mutation site profile of the ISLR gene is shown. (C) OS and D/PFS analyses according to the mutation status of the ISLR gene were performed. (D) Correlation between copy number variation and expression of ISLR. (E) OS analysis according to the CNV status of the ISLR gene was performed.
Next, we investigated the CNV status of the ISLR gene. As shown in Figure 3D, the ISLR gene mainly exhibited two kinds of CNVs, namely, single copy deletion and low-level amplification. However, there was no statistically significant association between ISLR CNV and gene expression (Figure 3D, R = 0.064) or the overall survival rate of gastric cancer cases (Figure 3E, P = 0.123). These results suggested that ISLR gene mutation and copy number variation may not affect gastric tumorigenesis.
DNA Methylation Analysis Data
Next, we aimed to investigate whether the ISLR gene was closely linked to ISLR DNA methylation. Based on methylation data from TCGA-STAD, we observed that the methylation values from four methylation probes, cg05195566, cg17258195, cg09664357, and cg07297039, were negatively correlated with the expression level of the ISLR gene (Figure 4, P < 0.05). Supplementary Figure S1 presents the specific information of methylation probe sites and the correlation results of ISLR gene expression with methylation level. Additionally, some methylation probes showed a correlation between ISLR hypomethylation and poor overall survival in gastric cancer (Figure 4 and Supplementary Figure S2, cg05195566, P = 9.5E-06; cg17258195, P = 0.0034; cg09664357, P = 0.0054; cg07297039, P = 0.036).
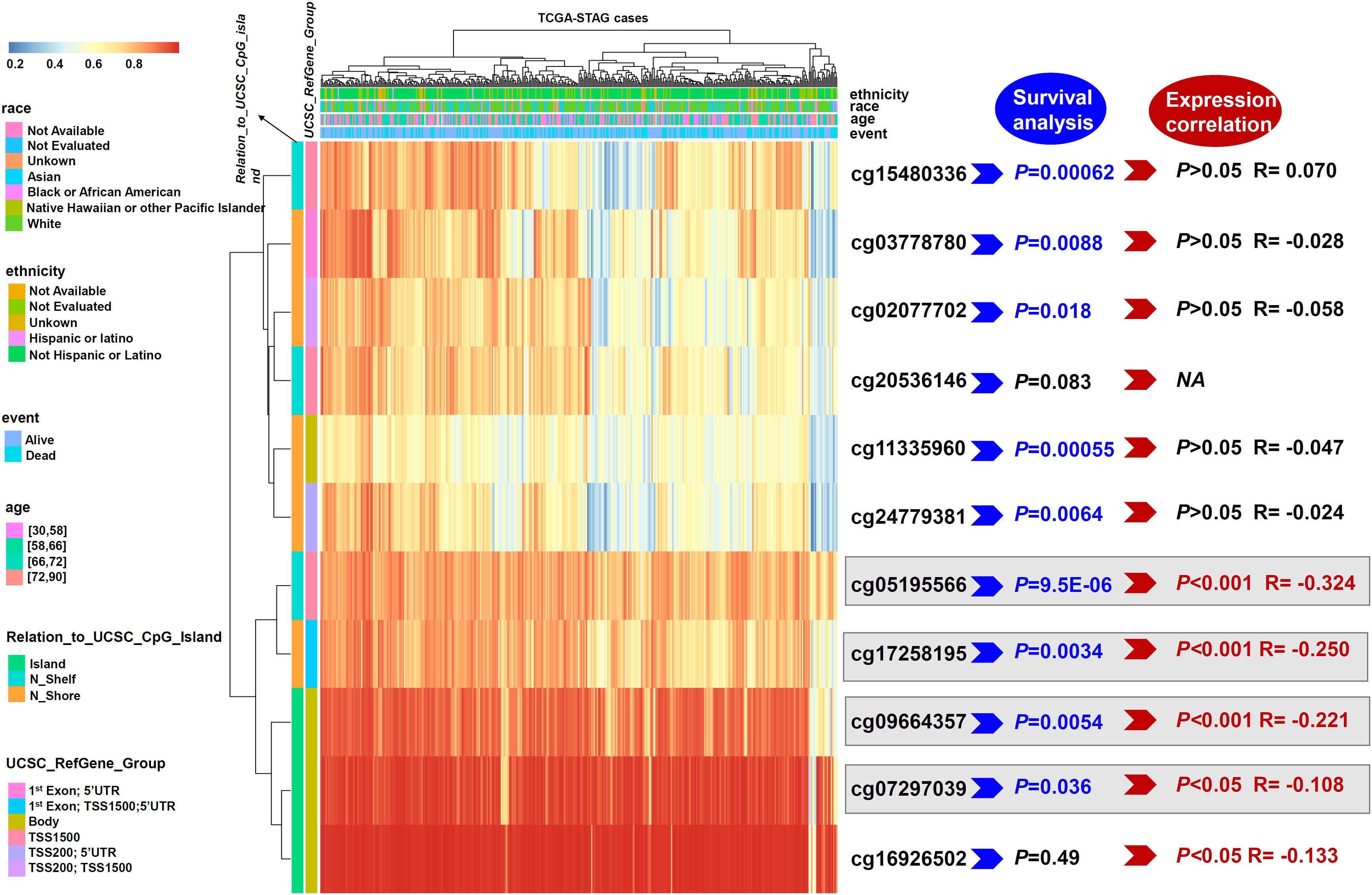
Figure 4. Waterfall plot and analysis of potential methylation probes targeting the ISLR gene. A waterfall plot of the methylation level of the ISLR gene is provided. The correlations between ISLR methylation or expression level and survival rate were also analyzed. NA, not available.
Immune Cell Infiltration Analysis Data
Herein, we sought to explore possible molecular mechanisms through immune cell infiltration during the etiology of gastric cancer. First, through GEPIA2, we analyzed the association between ISLR gene expression and immune cell infiltration status. As shown in Figure 5, we observed a positive correlation between ISLR expression and the marker genes of M1 macrophages (R = 0.48, P = 1.0E-37), M2 macrophages (R = 0.58, P = 1.2E-56), TAMs (R = 0.65, P = 4.6E-76), dendritic cells (R = 0.53, P = 6.6E-46), monocytes (R = 0.54, P = 3.6E-49), NK cells (R = 0.64, P = 5.6E-72), mast cells (R = 0.25, P = 3.3E-10), neutrophils (R = 0.55, P = 3.3E-49), and eosinophils (R = 0.42, P = 2.6E-27) but not between ISLR expression and basophils (R = 0.041, P = 0.3). We observed similar results for the different types of T and B cells, such as Tfh cells (R = 0.56, P = 2.7E-52) (R = 0.6, P = 4.0E-61), and exhausted T cells (Supplementary Figure S3).
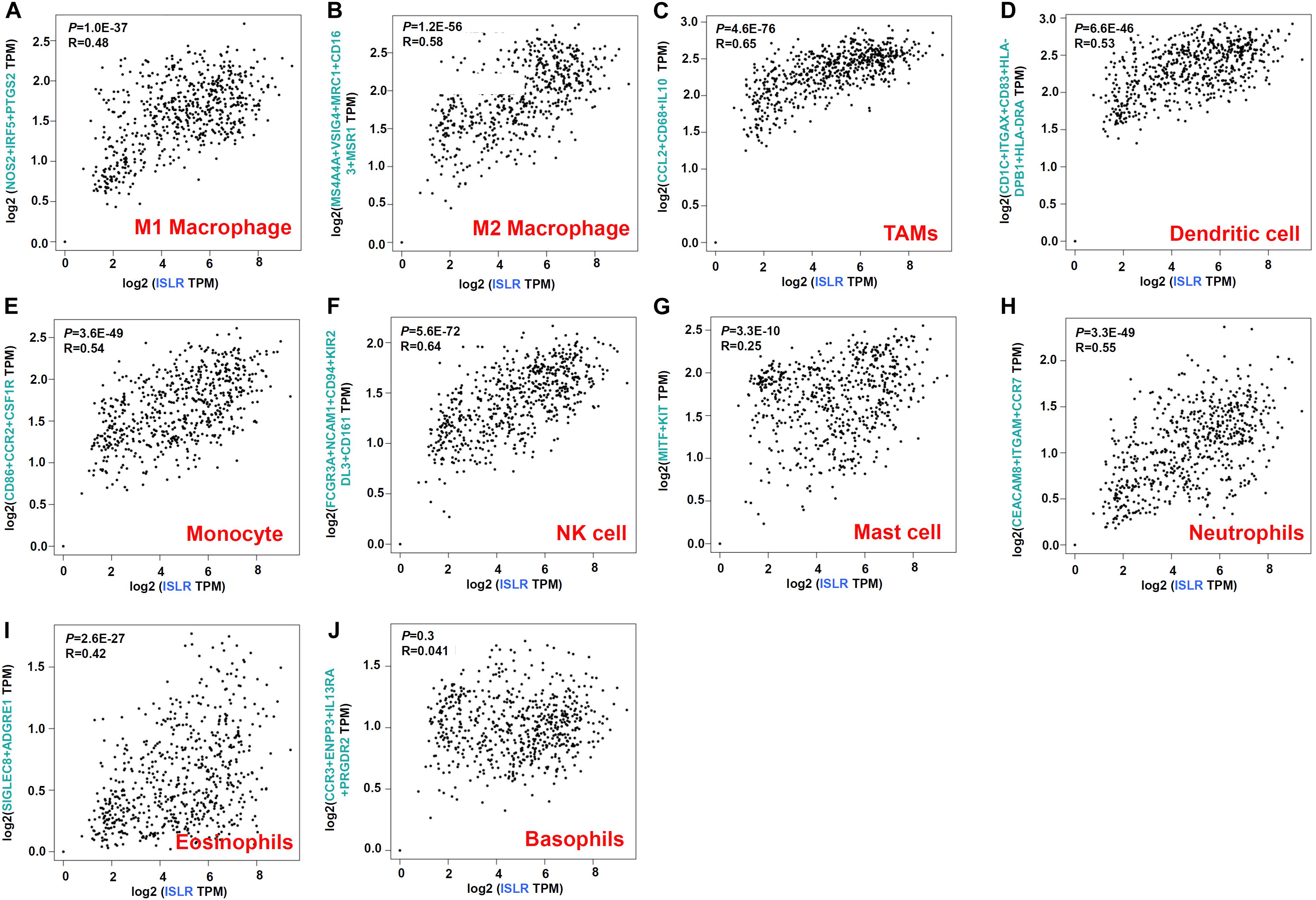
Figure 5. Correlation between ISLR expression and markers of immune cells as analyzed through GEPIA2. (A) M1 macrophages; (B) M2 macrophages; (C) TAMs; (D) dendritic cells; (E) monocytes; (F) NK cells; (G) mast cells; (H) neutrophils; (I) eosinophils; (J) basophils.
Then, we utilized the TIMER, CIBERSORT, CIBERSORT-ABS, QUANTISEQ, MCPCOUNTER, and EPIC algorithms for further immune infiltration estimations. As shown in Figure 6, we observed a relatively obvious correlation between ISLR expression and the immune infiltration levels of CD8+ T cells, monocytes, macrophages (especially the M2 type), activated mast cells and dendritic cells when adjusted by tumor purity.
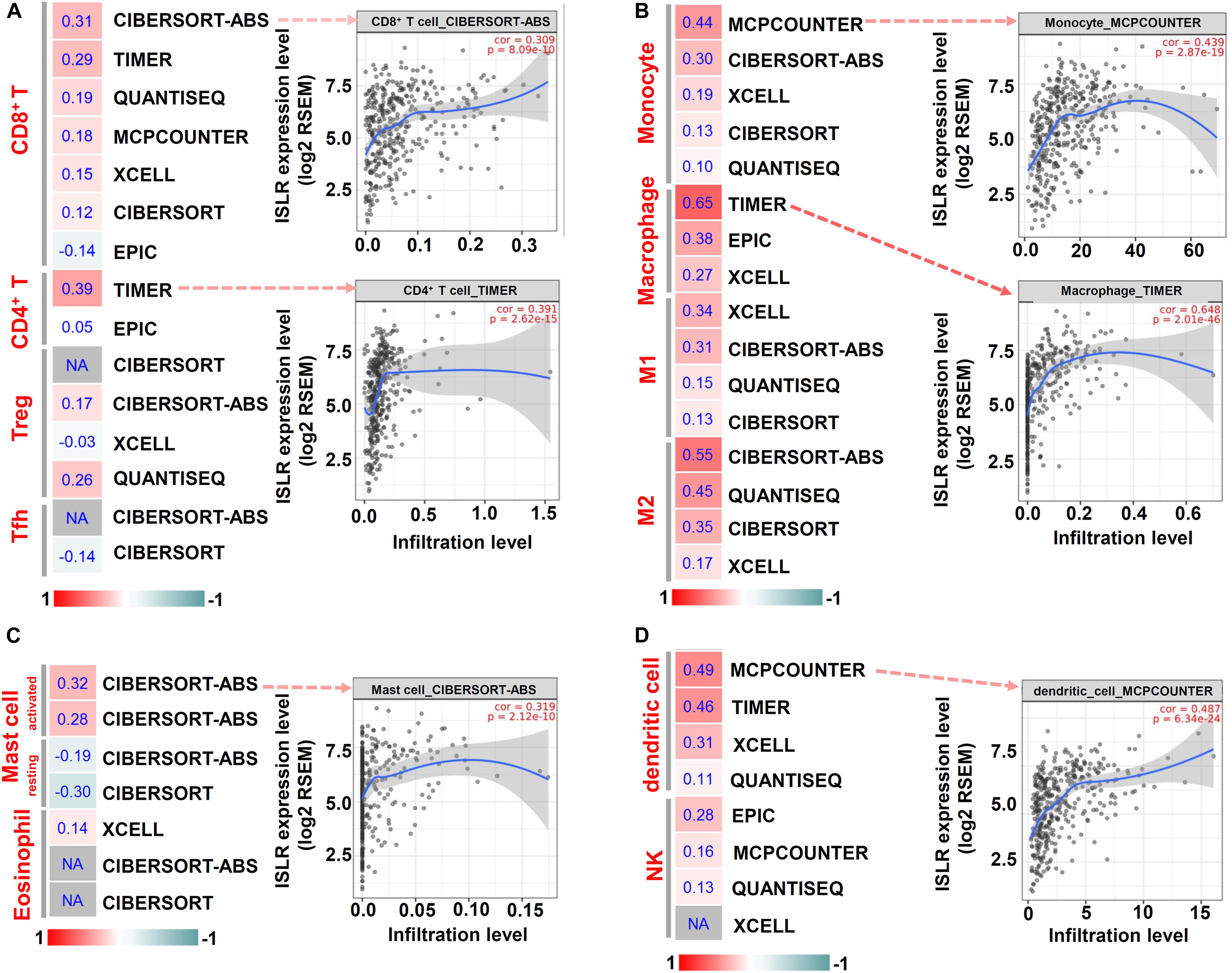
Figure 6. Correlation between ISLR expression and the infiltration level of immune cells. The TIMER, CIBERSORT, CIBERSORT.ABS, QUANTISEQ, MCPCOUNTER, XCELL, and EPIC algorithms were applied for the immune infiltration estimations of CD8+ T cells, CD4+ T cells, Treg cells, and Tfh cells (A); Monocytes and macrophages (M1, M2 type) (B); mast cells and eosinophils (C); and dendritic cells and NK cells (D). A heatmap with the purity-adjusted Spearman’s rho value and specific scatter plots are provided as examples. “NA” means a lack of an association between ISLR expression and the infiltration level of immune cells.
Additionally, we detected the correlation between ISLR CNV and the overall infiltration level of immune cells (Supplementary Figure S4). The copy deletion type of ISLR CNV was correlated with the infiltration level of dendritic cells, neutrophils, CD8+ T cells, CD4+ T cells, B cells, and macrophages (all P < 0.05), while the low-level amplification CNV was only associated with the infiltration level of dendritic cells (P < 0.001), neutrophils (P < 0.01), and CD8+ T cells (P < 0.001).
Cluster Analysis Data
Based on the “limma” R package, we obtained genes positively or negatively correlated with ISLR among three datasets: TCGA-STAD, GSE13861, and GSE29272 (Figure 7A). Then, we performed intersection analysis and identified 134 common positively correlated genes and 8 common negatively correlated genes (Figure 7B). Then, we performed GO enrichment analyses. We observed extracellular matrix-associated terms, such as extracellular structure organization and extracellular matrix structural constituents, in the GO_biological_process (Figure 7C), GO_cellular_component (Supplementary Figure S5A), and GO_molecular_function (Supplementary Figure S5B) categories. Then, we displayed the extracellular matrix-associated terms in GOCircle (Figure 7D) and chord (Figure 7E) plots.
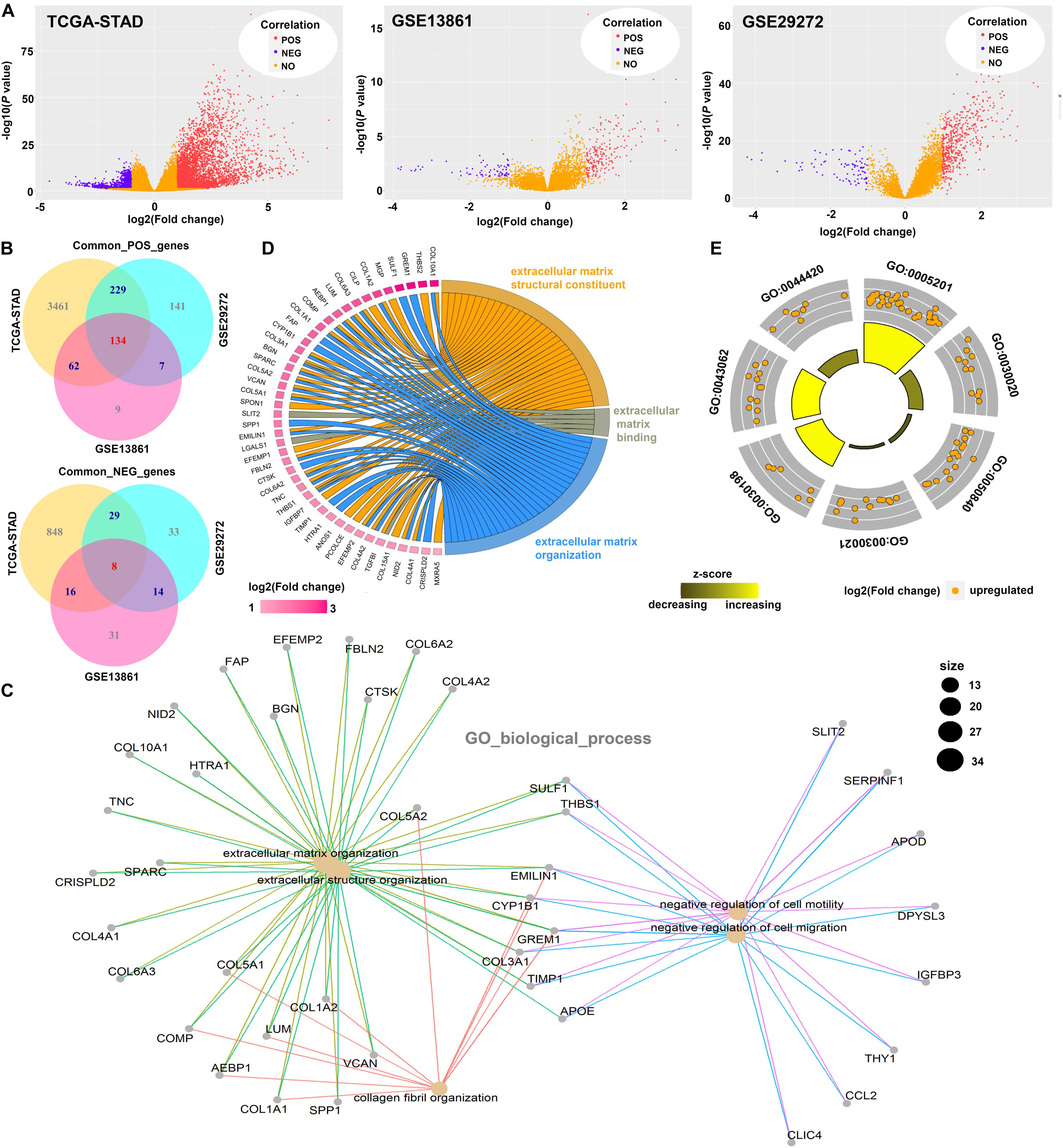
Figure 7. Cluster analysis of significant ISLR-correlated genes. (A) After expression difference analyses between the ISLR-high and -low expression groups were performed with the “limma” R package on the TCGA-STAD, GSE13861, and GSE29272 datasets, volcano plots were constructed. POS, genes positively correlated with ISLR; NEG, genes negatively correlated with ISLR; NO, genes not correlated with ISLR. (B) The “VennDiagram” R package was used for the common genes positively (POS) and negatively (NEG) correlated with ISLR. (C) The “clusterProfiler” R package was used for the GO_biological_process enrichment analysis. The GOCircle (D) and chord plots (E) using extracellular matrix-associated terms were visualized by the “GOplot” R package.
KEGG analysis data identified the ECM-receptor interaction (Supplementary Figure S6). GSEA data also showed the extracellular matrix (ECM)-associated gene sets, including extracellular structure organization, extracellular matrix structural constituents, ECM-receptor interaction, miRNA targets in ECM and membrane receptors (Supplementary Figure S7). Based on the GSCALite pathway score analysis, we further observed activation of the epithelial-mesenchymal transition (EMT) pathway (Supplementary Figure S8).
PCA and Random Forest Analysis Data
To further identify ISLR-correlated hub genes for the differentiation of tumor from normal samples, we performed PCA. As shown in Figures 8A–C, we used PC1, PC2 and PC3 to distinguish normal from tumor samples in the three datasets: TCGA-STAD, GSE13861, and GSE29272. Then, we conducted an intersection analysis and identified six hub genes, ISLR, COL1A2 (collagen type I alpha 2 chain), CDH11 (cadherin 11), SPARC (secreted protein acidic and cysteine-rich), COL3A1 (collagen type III alpha 1 chain), and COL1A1 (collagen type I alpha 1 chain) (Figure 8D). There were strong positive correlations of expression among these genes, and all correlation coefficients were greater than 0.8 (Figure 8E). Figure 8F presents the correlation between ISLR and COL1A2 gene expression in the three datasets (R = 0.91, P < 2.2E-16). Figures 8G–I further shows the contribution of these hub genes to PC1 and PC2.
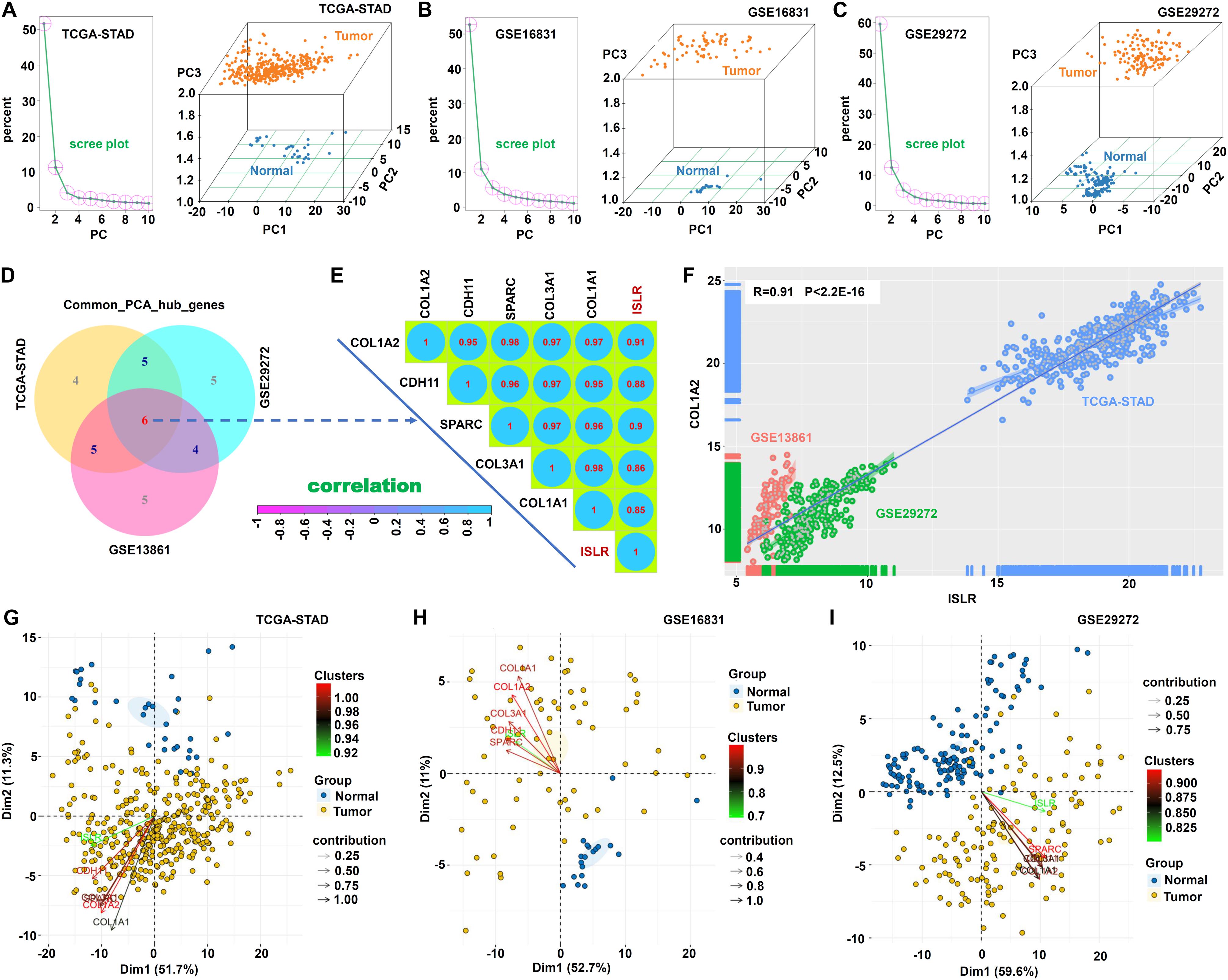
Figure 8. Principal component analysis. Based on the common_POS_genes and common_NEG_genes, the prcomp function was used for PCA to classify the normal and tumor sections in TCGA-STAD (A), GSE13861 (B), and GSE29272 (C). A scree plot and a three-dimensional map (PC1, PC2, and PC3) are provided. (D) The “VennDiagram” R package was used for the common_PCA_hub_genes. (E) The cor function and “corrplot” R package were used for the Spearman correlation analysis of these hub genes. Correlation coefficients are shown. (F) The scatter plot for the correlation between ISLR and COL1A2 gene expression is provided. (G–I) The “factoextra” R package was utilized to show the principal component weight and two-dimensional contribution maps of common hub genes.
Subsequently, we carried out a random forest analysis based on these six hub genes. The multidimensional scale plot in Figure 9A suggests the effective differentiation of normal from tumor samples in the TCGA-STAD cohort. Figure 9B shows the mean decrease accuracy and mean decrease Gini data. The AUC value of 0.869 indicated high classification accuracy (Figure 9C). Similar results were observed in the GSE16831 (Figures 9D–F) and GSE29272 (Figures 9G–I) datasets.
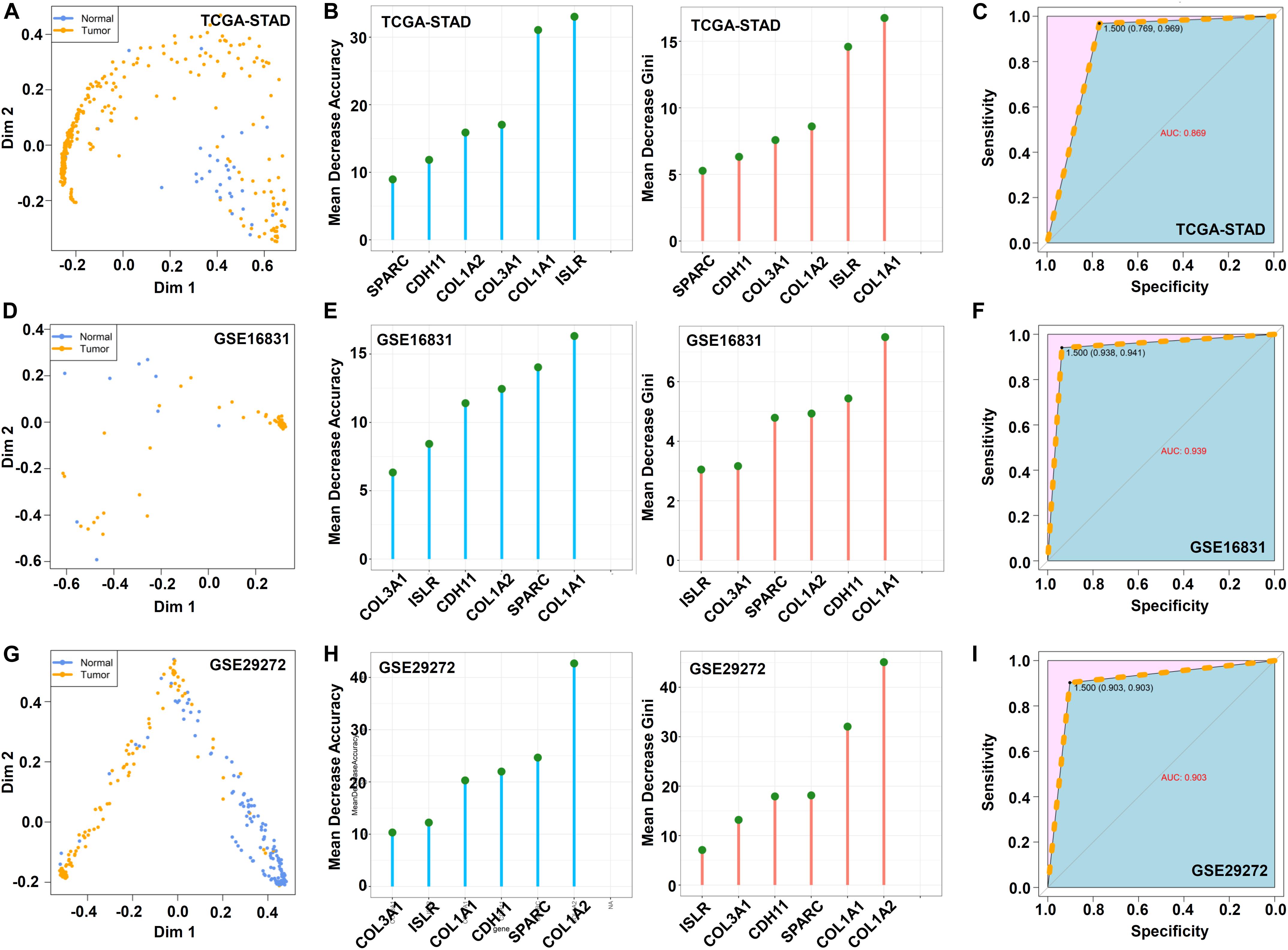
Figure 9. Random forest analysis. Based on the above hub genes, the “randomForest” package (ntree = 500) was utilized for the random forest modeling analysis. The MDSplot function was used to obtain a multidimensional scale (A,D,G). The data of mean decrease accuracy and mean decrease Gini were visualized by the ggdotchart function in the “ggpubr” package (B,E,H). The receiver operating characteristic (ROC) curve was plotted by the “pROC” package, and the area under the ROC curve (AUC) value was calculated (C,F,I).
Drug Sensitivity Analysis Data
Finally, based on the CTRP database, we conducted a small molecule/drug sensitivity (IC50) evaluation and further detected that the expression of the ISLR gene was negatively related to sensitivity to PX-12 and NSC632839 (Supplementary Figure S9).
Discussion
Based on the available datasets of gastric cancer cases collected by TCGA and GEO, for the first time, we found a statistical correlation between high expression of the ISLR gene and poor overall survival, disease-free survival, first progression, and post-progression survival. There were significant differences in ISLR expression among different pathological stages (stages 1–4). When gastric cancer samples were divided by clinical information, a positive correlation between ISLR expression and gastric cancer prognosis existed in most subgroups, such as subgroups based on different Lauren classifications (intestinal or diffuse). Notably, we only observed a correlation between ISLR gene expression and OS in the well-differentiated subgroup but not in the poorly or moderately differentiated subgroup. In addition, we detected a positive effect of ISLR expression on survival in the pathological stage 3 subgroup but not the stage 1, 2, or 4 subgroups. These results implied that the prognostic ability of high ISLR gene expression may increase with tumor differentiation or pathological grade.
Upon integrated analysis, we observed that high expression of the ISLR gene showed a correlation with low sensitivity to PX-12 (an irreversible inhibitor of thioredoxin-1) (Metcalfe et al., 2016) and NSC632839 (a non-selective isopeptidase inhibitor) (Nicholson et al., 2008), indicating that high ISLR gene expression may be associated with chemoresistance in gastric cancer. Unexpectedly, during the survival analysis of gastric cancer patients treated with a 5-Fu-based adjuvant, high expression of the ISLR gene was linked to a better prognosis than low expression of the ISLR gene. It is possible that 5-Fu treatment interferes with the expression of the ISLR gene in patients, which leads to changes in survival outcomes. Additionally, although we did not detect a correlation between ISLR gene expression and 5-Fu drug sensitivity through our preliminary assessment, more clinical gastric cancer samples specifically under the treatment of 5-Fu and comprehensive analysis are needed to validate the relationship between ISLR expression and 5-Fu chemotherapy resistance.
DNA methylation status is closely associated with the carcinogenesis or drug resistance of gastric cancer (Tahara and Arisawa, 2015; Choi et al., 2017). Although we failed to detect a correlation between ISLR gene mutations or CNVs and the clinical prognosis of gastric cancer, the hypomethylation status of several sites within ISLR (cg05195566, cg17258195, cg09664357, and cg0729703) was linked to high expression of ISLR and clinically poor survival outcomes. We noted that the cg05195566 and cg17258195 sites are situated in the promoter region, while cg09664357 and cg0729703 are outside the promoter region. It is worthwhile to further investigate how methylation of different sites within ISLR affects the expression level and survival outcomes of gastric cancer patients.
Considering the structure of the ISLR protein as a member of the Ig superfamily (Nagasawa et al., 1997) and the functional links between immune infiltration and gastric cancer (Kim et al., 2016; Liu et al., 2016; Pan et al., 2019; Xu et al., 2019), we first investigated the correlation between ISLR gene expression and macrophage, neutrophil, dendritic cell, B cell, T cell and other immune cell infiltration levels based on gene expression correlations and the TIMER, CIBERSORT, CIBERSORT-ABS, QUANTISEQ, MCPCOUNTER, XCELL, and EPIC algorithms. The results were adjusted for tumor purity. We observed a positive correlation between ISLR gene expression and several immune cells, especially CD8+ T cells, macrophages and dendritic cells. We also detected a correlation between ISLR CNV and immune infiltration. These results indicated that the tumor microenvironment may be key in the complex molecular mechanism by which the ISLR gene affects carcinogenesis of gastric cancer.
The extracellular matrix (ECM) and epithelial-mesenchymal transition (EMT) have been reported to be associated with the invasion and migration of gastric cancer (Lukaszewicz-Zajac et al., 2011; Peng et al., 2014; Huang et al., 2015). After pooling the ISLR expression-associated genes, we detected significantly enriched ECM-related pathways, including miRNA targets in the ECM and membrane receptors. Our PCA and random forest analysis further identified six extracellular matrix-associated hub genes, which were able to distinguish between gastric cancer and normal control samples. We also found that ISLR gene expression was associated with the activation of the EMT pathway. Considering the connection between miRNAs and EMT in gastric cancer (Bure et al., 2019), we performed GSCALite mRNA-miRNA regulation network analysis to identify several potential ISLR-binding miRNAs, including hsa-miR16-5p, hsa-miR-3116, hsa-mir-934, hsa-miR98-5p, and hsa-miR-339-5p (data not shown). It is meaningful to evaluate the relationship between ISLR expression and EMT from the perspective of miRNA and to investigate the mechanism underlying the progression of gastric cancer. In addition, the chief aim of our research was only to examine the potential mechanism by which the ISLR gene participates in gastric carcinogenesis. It should be noted that ISLR does not show specificity for gastric cancer tissue (data not shown), and its role in other cancers cannot be ruled out. Most likely, ISLR works as an effective prognostic marker during gastric carcinogenesis because it forms functional protein-protein and protein-nucleic acid complexes.
Conclusion
After our bioinformatics and biostatistics analyses of gastric cancer cases within the TCGA and GEO cohorts, high ISLR expression was identified as a potential prognostic biomarker for gastric cancer. DNA hypomethylation of ISLR is linked to high expression of the ISLR gene and overall clinical prognosis. ISLR expression was also correlated with the infiltration of several immune cells (e.g., CD8+ T cells, macrophages and dendritic cells), EMT pathway activity and sensitivity to PX-12 and NSC632839. Our findings are of great significance for conducting ISLR-based cell or animal experimental validation.
Data Availability Statement
The datasets generated for this study are available on request to the corresponding author.
Author Contributions
SL conceived and designed the study and drafted the manuscript. SL and WZ performed the ISLR expression, survival curve analysis, and genetic alteration analysis. SL and MS performed the DNA methylation, immune cell infiltration analysis, ISLR-correlated pathway, PCA, random forest, and drug sensitivity analysis. All authors reviewed the manuscript before submission and approved the final version of the manuscript.
Conflict of Interest
WZ was employed by the General Data Technology Co., Ltd, Tianjin.
The remaining authors declare that the research was conducted in the absence of any commercial or financial relationships that could be construed as a potential conflict of interest.
Supplementary Material
The Supplementary Material for this article can be found online at: https://www.frontiersin.org/articles/10.3389/fgene.2020.00620/full#supplementary-material
FIGURE S1 | Correlation between methylation status and expression of ISLR in gastric cases in the TCGA-STAD cohort. Detailed information on the methylation probe is provided. Pearson correlation coefficients (R) and Benjamini–Hochberg-adjusted P-values (∗P < 0.05, ∗∗∗P < 0.001) for the comparison are shown as well.
FIGURE S2 | Correlation between the methylation status of ISLR DNA and the survival rate of gastric patients in the TCGA-STAD cohort. A total of eleven methylation probes, including cg15480336 (A), cg03778780 (B), cg02077702 (C), cg20536146 (D), cg11335960 (E), cg24779381 (F), cg05195566 (G), cg177258195 (H), cg09664357 (I), cg07297039 (J), and cg16926502 (K), were used.
FIGURE S3 | Correlation between ISLR and markers of T or B cells as analyzed through GEPIA2. (A) B cells; (B) Th1 cells; (C) Th2 cells; (D) Th17 cells; (E) CD8+ T cells; (F) Tfh cells; (G) resting Treg cells; (H) effector Treg cells; (I) exhausted T cells.
FIGURE S4 | Correlation between ISLR CNV and the infiltration level of immune cells as analyzed through TIMER. (A) dendritic cells; (B) neutrophils; (C) CD8+ T cells; (D) CD4+ T cells; (E) B cells; (F) macrophages. (∗P < 0.05, ∗∗P < 0.01, ∗∗∗P < 0.001).
FIGURE S5 | GO_biological_process and GO_molecular_function enrichment analysis. The “clusterProfiler” R package was used for the GO_biological_process (A) and GO_molecular_function (B) enrichment analyses.
FIGURE S6 | KEGG enrichment analysis. The “pathview” R package was used for the KEGG enrichment analysis.
FIGURE S7 | GSEA. A LinkedOmics approach was utilized for the ISLR-correlated GSEA profiles. Four extracellular matrix (ECM)-associated gene sets, including genes involved in extracellular structural organization (A), extracellular matrix structural constituents (B), ECM-receptor interactions (C), and miRNA targets in the ECM and membrane receptors (D), were identified.
FIGURE S8 | Correlation between ISLR expression and pathway activation or inhibition.
FIGURE S9 | Correlation between ISLR expression and small molecule/drug sensitivity (IC50).
TABLE S1 | Correlation of ISLR expression and the first progression status of gastric cancer patients in the GEO cohort (Kaplan–Meier plotter).
TABLE S2 | Correlation of ISLR expression and the PPS of gastric cancer patients in the GEO cohort (Kaplan–Meier plotter).
Footnotes
- ^ http://gepia2.cancer-pku.cn/#analysis
- ^ https://www.oncomine.org/resource/main.html
- ^ https://www.r-project.org/
- ^ https://www.cbioportal.org/
- ^ https://xenabrowser.net/
References
Barrett, T., Wilhite, S. E., Ledoux, P., Evangelista, C., Kim, I. F., Tomashevsky, M., et al. (2013). NCBI GEO: archive for functional genomics data sets–update. Nucleic Acids Res. 41, D991–D995. doi: 10.1093/nar/gks1193
Bhandari, V., Hoey, C., Liu, L. Y., Lalonde, E., Ray, J., Livingstone, J., et al. (2019). Molecular landmarks of tumor hypoxia across cancer types. Nat. Genet. 51, 308–318. doi: 10.1038/s41588-018-0318-2
Bure, I. V., Nemtsova, M. V., and Zaletaev, D. V. (2019). Roles of E-cadherin and noncoding RNAs in the epithelial-mesenchymal transition and progression in gastric cancer. Int. J. Mol. Sci. 20:2870. doi: 10.3390/ijms20122870
Cancer Genome Atlas Research Network (2014). Comprehensive molecular characterization of gastric adenocarcinoma. Nature 513, 202–209. doi: 10.1038/nature13480
Chen, X., Leung, S. Y., Yuen, S. T., Chu, K. M., Ji, J., Li, R., et al. (2003). Variation in gene expression patterns in human gastric cancers. Mol. Biol. Cell 14, 3208–3215. doi: 10.1091/mbc.e02-12-0833
Choi, S. J., Jung, S. W., Huh, S., Chung, Y. S., Cho, H., and Kang, H. (2017). Alteration of DNA methylation in gastric cancer with chemotherapy. J. Microbiol. Biotechnol. 27, 1367–1378. doi: 10.4014/jmb.1704.04035
Clough, E., and Barrett, T. (2016). The gene expression omnibus database. Methods Mol. Biol. 1418, 93–110. doi: 10.1007/978-1-4939-3578-9_5
Ellrott, K., Bailey, M. H., Saksena, G., Covington, K. R., Kandoth, C., Stewart, C., et al. (2018). Scalable open science approach for mutation calling of tumor exomes using multiple genomic pipelines. Cell Syst. 6, 271–281.e7. doi: 10.1016/j.cels.2018.03.002
Gao, Q., Liang, W. W., Foltz, S. M., Mutharasu, G., Jayasinghe, R. G., Cao, S., et al. (2018). Driver fusions and their implications in the development and treatment of human cancers. Cell Rep. 23, 227–238.e3. doi: 10.1016/j.celrep.2018.03.050
Hoadley, K. A., Yau, C., Hinoue, T., Wolf, D. M., Lazar, A. J., Drill, E., et al. (2018). Cell-of-origin patterns dominate the molecular classification of 10,000 tumors from 33 types of cancer. Cell 173, 291–304.e6. doi: 10.1016/j.cell.2018.03.022
Homma, S., Shimada, T., Hikake, T., and Yaginuma, H. (2009). Expression pattern of LRR and Ig domain-containing protein (LRRIG protein) in the early mouse embryo. Gene Expr. Patterns 9, 1–26. doi: 10.1016/j.gep.2008.09.004
Huang, L., Wu, R. L., and Xu, A. M. (2015). Epithelial-mesenchymal transition in gastric cancer. Am. J. Transl. Res. 7, 2141–2158.
Kakiuchi, M., Nishizawa, T., Ueda, H., Gotoh, K., Tanaka, A., Hayashi, A., et al. (2014). Recurrent gain-of-function mutations of RHOA in diffuse-type gastric carcinoma. Nat. Genet. 46, 583–587. doi: 10.1038/ng.2984
Kim, J. W., Nam, K. H., Ahn, S. H., Park, D. J., Kim, H. H., Kim, S. H., et al. (2016). Prognostic implications of immunosuppressive protein expression in tumors as well as immune cell infiltration within the tumor microenvironment in gastric cancer. Gastric Cancer 19, 42–52. doi: 10.1007/s10120-014-0440-5
Koch, A., De Meyer, T., Jeschke, J., and Van Criekinge, W. (2015). MEXPRESS: visualizing expression, DNA methylation and clinical TCGA data. BMC Genomics 16:636. doi: 10.1186/s12864-015-1847-z
Koch, A., Jeschke, J., Van Criekinge, W., van Engeland, M., and De Meyer, T. (2019). MEXPRESS update 2019. Nucleic Acids Res. 47, W561–W565. doi: 10.1093/nar/gkz445
Li, B., Severson, E., Pignon, J. C., Zhao, H., Li, T., Novak, J., et al. (2016). Comprehensive analyses of tumor immunity: implications for cancer immunotherapy. Genome Biol. 17:174.
Li, T., Fan, J., Wang, B., Traugh, N., Chen, Q., Liu, J. S., et al. (2017). TIMER: a web server for comprehensive analysis of tumor-infiltrating immune cells. Cancer Res. 77, e108–e110. doi: 10.1158/0008-5472.can-17-0307
Liu, C. J., Hu, F. F., Xia, M. X., Han, L., Zhang, Q., and Guo, A. Y. (2018). GSCALite: a web server for gene set cancer analysis. Bioinformatics 34, 3771–3772. doi: 10.1093/bioinformatics/bty411
Liu, J., Lichtenberg, T., Hoadley, K. A., Poisson, L. M., Lazar, A. J., Cherniack, A. D., et al. (2018). An integrated TCGA pan-cancer clinical data resource to drive high-quality survival outcome analytics. Cell 173, 400–416.e11. doi: 10.1016/j.cell.2018.02.052
Liu, J. Y., Yang, X. J., Geng, X. F., Huang, C. Q., Yu, Y., and Li, Y. (2016). Prognostic significance of tumor-associated macrophages density in gastric cancer: a systemic review and meta-analysis. Minerva Med. 107, 314–321.
Lugowska, A., Hetmanczyk-Sawicka, K., Iwanicka-Nowicka, R., Fogtman, A., Ciesla, J., Purzycka-Olewiecka, J. K., et al. (2019). Gene expression profile in patients with Gaucher disease indicates activation of inflammatory processes. Sci. Rep. 9:6060.
Lukaszewicz-Zajac, M., Mroczko, B., and Szmitkowski, M. (2011). Gastric cancer - The role of matrix metalloproteinases in tumor progression. Clin. Chim. Acta 412, 1725–1730. doi: 10.1016/j.cca.2011.06.003
Metcalfe, C., Ramasubramoni, A., Pula, G., Harper, M. T., Mundell, S. J., and Coxon, C. H. (2016). Thioredoxin inhibitors attenuate platelet function and thrombus formation. PLoS One 11:e0163006. doi: 10.1371/journal.pone.0163006
Modhukur, V., Iljasenko, T., Metsalu, T., Lokk, K., Laisk-Podar, T., and Vilo, J. (2018). MethSurv: a web tool to perform multivariable survival analysis using DNA methylation data. Epigenomics 10, 277–288. doi: 10.2217/epi-2017-0118
Nagasawa, A., Kubota, R., Imamura, Y., Nagamine, K., Wang, Y., Asakawa, S., et al. (1997). Cloning of the cDNA for a new member of the immunoglobulin superfamily (ISLR) containing leucine-rich repeat (LRR). Genomics 44, 273–279. doi: 10.1006/geno.1997.4889
Nicholson, B., Leach, C. A., Goldenberg, S. J., Francis, D. M., Kodrasov, M. P., Tian, X., et al. (2008). Characterization of ubiquitin and ubiquitin-like-protein isopeptidase activities. Protein Sci. 17, 1035–1043. doi: 10.1110/ps.083450408
Pan, J. H., Zhou, H., Cooper, L., Huang, J. L., Zhu, S. B., Zhao, X. X., et al. (2019). LAYN is a prognostic biomarker and correlated with immune infiltrates in gastric and colon cancers. Front. Immunol. 10:6. doi: 10.3389/fimmu.2019.00006
Peng, Z., Wang, C. X., Fang, E. H., Wang, G. B., and Tong, Q. (2014). Role of epithelial-mesenchymal transition in gastric cancer initiation and progression. World J. Gastroenterol. 20, 5403–5410. doi: 10.3748/wjg.v20.i18.5403
Rees, M. G., Seashore-Ludlow, B., Cheah, J. H., Adams, D. J., Price, E. V., Gill, S., et al. (2016). Correlating chemical sensitivity and basal gene expression reveals mechanism of action. Nat. Chem. Biol. 12, 109–116. doi: 10.1038/nchembio.1986
Sanchez-Vega, F., Mina, M., Armenia, J., Chatila, W. K., Luna, A., La, K. C., et al. (2018). Oncogenic signaling pathways in the cancer genome atlas. Cell 173, 321–337.e10. doi: 10.1016/j.cell.2018.03.035
Szasz, A. M., Lanczky, A., Nagy, A., Forster, S., Hark, K., Green, J. E., et al. (2016). Cross-validation of survival associated biomarkers in gastric cancer using transcriptomic data of 1,065 patients. Oncotarget 7, 49322–49333. doi: 10.18632/oncotarget.10337
Tahara, T., and Arisawa, T. (2015). DNA methylation as a molecular biomarker in gastric cancer. Epigenomics 7, 475–486. doi: 10.2217/epi.15.4
Tang, Z., Kang, B., Li, C., Chen, T., and Zhang, Z. (2019). GEPIA2: an enhanced web server for large-scale expression profiling and interactive analysis. Nucleic Acids Res. 47, W556–W560. doi: 10.1093/nar/gkz430
Taylor, A. M., Shih, J., Ha, G., Gao, G. F., Zhang, X., Berger, A. C., et al. (2018). Genomic and functional approaches to understanding cancer aneuploidy. Cancer Cell 33, 676–689.e3. doi: 10.1016/j.ccell.2018.03.007
Tomczak, K., Czerwinska, P., and Wiznerowicz, M. (2015). The cancer genome atlas (TCGA): an immeasurable source of knowledge. Contemp. Oncol. 19, A68–A77. doi: 10.5114/wo.2014.47136
Vasaikar, S. V., Straub, P., Wang, J., and Zhang, B. (2018). LinkedOmics: analyzing multi-omics data within and across 32 cancer types. Nucleic Acids Res. 46, D956–D963. doi: 10.1093/nar/gkx1090
Wang, K., Kan, J., Yuen, S. T., Shi, S. T., Chu, K. M., Law, S., et al. (2011). Exome sequencing identifies frequent mutation of ARID1A in molecular subtypes of gastric cancer. Nat. Genet. 43, 1219–1223. doi: 10.1038/ng.982
Wang, K., Yuen, S. T., Xu, J., Lee, S. P., Yan, H. H., Shi, S. T., et al. (2014). Whole-genome sequencing and comprehensive molecular profiling identify new driver mutations in gastric cancer. Nat. Genet. 46, 573–582. doi: 10.1038/ng.2983
Wang, Z., Jensen, M. A., and Zenklusen, J. C. (2016). A practical guide to the cancer genome atlas (TCGA). Methods Mol. Biol. 1418, 111–141. doi: 10.1007/978-1-4939-3578-9_6
Xu, J., Yu, Y., He, X., Niu, N., Li, X., Zhang, R., et al. (2019). Tumor-associated macrophages induce invasion and poor prognosis in human gastric cancer in a cyclooxygenase-2/MMP9-dependent manner. Am. J. Transl. Res. 11, 6040–6054.
Keywords: ISLR, expression, methylation, immune cell infiltration, gastric cancer
Citation: Li S, Zhao W and Sun M (2020) An Analysis Regarding the Association Between the ISLR Gene and Gastric Carcinogenesis. Front. Genet. 11:620. doi: 10.3389/fgene.2020.00620
Received: 12 December 2019; Accepted: 22 May 2020;
Published: 16 June 2020.
Edited by:
Min Zhang, Purdue University, United StatesReviewed by:
Jian Li, Tulane University, United StatesJun Li, University of Notre Dame, United States
Copyright © 2020 Li, Zhao and Sun. This is an open-access article distributed under the terms of the Creative Commons Attribution License (CC BY). The use, distribution or reproduction in other forums is permitted, provided the original author(s) and the copyright owner(s) are credited and that the original publication in this journal is cited, in accordance with accepted academic practice. No use, distribution or reproduction is permitted which does not comply with these terms.
*Correspondence: Shu Li, dGpfbGlzaHVAMTYzLmNvbQ==