- 1School of Public Health, Fudan University, Shanghai, China
- 2Nutrition and Genomics Laboratory, JM-USDA Human Nutrition Research Center on Aging at Tufts University, Boston, MA, United States
- 3Department of Cardiology, Zhongnan Hospital of Wuhan University, Wuhan, China
- 4USDA Agricultural Research Service, Nutrition and Genomics Laboratory, JM-USDA Human Nutrition Research Center on Aging at Tufts University, Boston, MA, United States
- 5IMDEA Food Institute, CEI UAM + CSIC, Madrid, Spain
- 6Centro Nacional de Investigaciones Cardiovasculares (CNIC), Madrid, Spain
Statin is the medication most widely prescribed to reduce plasma cholesterol levels. Yet, how the medication contributes to diabetes risk and impaired glucose metabolism is not clear. This study aims to examine the epigenetic mechanisms of ABCG1 through which statin use associates with risk of type 2 diabetes. We determined the association between the statin use, DNA methylation at ABCG1 and type 2 diabetes/glycemic traits in the Framingham Heart Study Offspring (FHS, n = 2741), with validation in the Women’s Health Initiative Study (WHI, n = 2020). The causal effect of statin use on the risk of type 2 diabetes was examined using a two-step Mendelian randomization approach. Next, based on transcriptome analysis, we determined the links between the medication-associated epigenetic status of ABCG1 and biological pathways on the pathogenesis of type 2 diabetes. Our results showed that DNA methylation levels at cg06500161 of ABCG1 were positively associated with the use of statin, type 2 diabetes and related traits (fasting glucose and insulin) in FHS and WHI. Two-step Mendelian randomization suggested a causal effect of statin use on type 2 diabetes and related traits through epigenetic mechanisms, specifically, DNA methylation at cg06500161. Our results highlighted that gene expression of ABCG1, ABCA1 and ACSL3, involved in both cholesterol metabolism and glycemic pathways, was inversely associated with statin use, CpG methylation, and diabetic signatures. We concluded that DNA methylation site cg06500161 at ABCG1 is a mediator of the association between statins and risk of type 2 diabetes.
Introduction
Dyslipidemia is a major risk factor for cardiovascular disease (CVD), which remains the leading cause of mortality worldwide. Lipid-lowering drugs, like statins, are the medication most widely prescribed to reduce plasma cholesterol levels (Chou et al., 2016; Zhan et al., 2018). With common and long-term use of such medications, a number of adverse effects have been reported. Specifically, statins have been associated with liver damage (Aguirre et al., 2013), muscle discomfort (Pirillo and Catapano, 2015) and diabetes (Kim D. W. et al., 2019; Kim Y. S. et al., 2019). Yet, how the medication contributes to diabetes risk and impaired glucose metabolism is not clear.
Type 2 diabetes is a complex disease that results from genetic and environmental factors and their interactions. Environmental factors, including diet, lifestyle and medications, may modify epigenetic status affecting the risk of type 2 diabetes (Vickers, 2014; Nilsson and Ling, 2017). Epigenome-wide association studies in several cohorts have shown strong associations between DNA methylation at CpG sites in ATP binding cassette subfamily G member 1 (ABCG1) and risk of type 2 diabetes (Hidalgo et al., 2014; Dayeh et al., 2016; Davegardh et al., 2018). ABCG1, a member of the ATP-binding cassette (ABC) protein family, functions in removing excess cholesterol from peripheral tissues and transporting it to the liver (Matsuo, 2010). ABCG1 expression has been reported to be regulated by statins in Caco-2 cells and COPD patients (Genvigir et al., 2011; Obeidat et al., 2015). Thus, we hypothesized statin use induces epigenetic changes that affect gene expression to increase the risk of type 2 diabetes.
This study aims to characterize the association between the use of statins, DNA methylation at ABCG1 and glycemic traits. For this purpose, we used the Framingham Heart Study Offspring (FHS) as the discovery population and validated the findings in the Women’s Health Initiative Study (WHI). We then determined if statin use had causal effects on the risk of type 2 diabetes using a two-step Mendelian randomization approach (Relton and Davey Smith, 2012; Caramaschi et al., 2017; Zhu et al., 2018). Furthermore, we determined whether altered DNA methylation at CpGs contributed to specific metabolic pathways on the pathogenesis of type 2 diabetes.
Materials and Methods
Framingham Heart Study (FHS)
The FHS follows several community dwelling generations of participants recruited in Framingham, MA, since 1948 (Dawber et al., 1951). In 1971, 5124 offspring, self-identified as European ancestry, from the original cohort and spouses were recruited (Kannel et al., 1979). In exam 8 (2005–2008) of this offspring cohort, participants completed a comprehensive set of dietary and health assessment questionnaires. The use of statins was obtained from the questionnaires. Statin users were defined as participants who took any type of statins, whereas non-statin users were those who did not use statin, but might or might not have taken other lipid-lowering drugs. LDL-C was estimated using Friedewald equation (Lindsey et al., 2004). DNA methylome and transcriptome analyses were performed on blood samples collected from 2741 participants (Marioni et al., 2015). These data were obtained from dbGaP (accession: phs000007.v29.p10; downloaded on September 27, 2017).
Women’s Health Initiative Study (WHI)
Starting from 1993, the WHI study recruited over 160,000 women into a long-term national health study that focuses on strategies for preventing heart disease, breast and colorectal cancer, and osteoporosis in postmenopausal women The Women’s Health Initiative Study Group (1998). Participants (n = 2020) from a combined case-control and pseudo-case-cohort were included in this study. Blood samples used for measurement of DNA methylation and clinical biochemistry were taken at Exam 1. Data was requested from dbGaP (accession: phs000200.v11.p3; downloaded on September 27, 2017). At Exam 1, participants also completed a comprehensive set of dietary and health assessment questionnaires. The use of statins was obtained from the questionnaires. The same definition of statin users and non-statin users in FHS was applied to WHI.
DNA Methylation Analysis
Raw IDAT files of DNA methylation analysis were quality-controlled and processed as described (Hidalgo et al., 2014; Marioni et al., 2015; Lai et al., 2018). Briefly, we used a β score to measure methylation signal as the proportion of the total methylation-specific signal, and the detection P-value as the probability that the total intensity for a given probe fell within the background signal intensity. Then, we excluded any CpG probes with a detection P-value > 0.01 and missing sample percentage > 1.5%, or > 10% of samples lacking sufficient intensity. We performed normalization on the filtered β scores using the ComBat package in R (Morris et al., 2014). Principal components were calculated on the β scores of samples that passed quality control using the prcomp function in R.
Genotyping
Genotypes for 2176 individuals from FHS were requested from dbGaP (accession: phs000342.v18.p11) as part of the NHLBI SNP Health Association Resource (SHARe) project, with initial QC and imputation having been performed previously. Briefly, approximately 500,000 SNPs were genotyped on the Affymetrix500k and Affymetrix50k chips and filtered for Hardy-Weinberg equilibrium, call rate, and minor allele frequency. These genotypes were then phased using MACH and imputed to the November 2010 release of 1000 Genomes using Minimac. Additional filters were applied to the imputed dosage data as follows: only samples associated with subjects who also had methylation data available were retained, and SNPs were filtered for an imputation quality score (R2) > 0.9. Dosages were converted to hard-calls if the dosage was within 0.2 of an integer allele count (0/1/2) and otherwise were set as missing.
Genotypes for 1966 individuals from WHI were available from dbGaP (accession: phs000746.v2.p3) as part of an imputation and harmonization effort across six GWAS sub-studies. Quality control and preprocessing steps were applied in each of the sub-studies prior to imputation (details available at dbGaP). Genotypes were then phased and aligned to the 1000 Genomes reference panel using BEAGLE and Minimac. The imputed dosages of 5,298,674 SNPs were retrieved from dbGaP and filtered for imputation quality score > 0.3, converted to hard-calls within 0.1 of an integer allele count (otherwise set as missing), and merged across sub-studies.
For both genotyped cohorts, SNP IDs, loci, and allelic information were annotated using the 1000 Genomes Phase 3 download from dbSNP (download date: April 13, 2018). Genotype processing was performed using PLINK 1.9 and 2.0 [URL1; (Chang et al., 2015)].
Transcriptome Analysis
We obtained FHS transcriptome data from dbGaP under accession phe00002.v6. Transcriptome analysis was conducted in exam 8 using the Affymetrix Human Exon 1.0 ST array for 17873 probes with mRNA from whole blood samples collected from 1616 participants from the Offspring Cohort after overnight fasting. Detailed descriptions of transcriptome analysis procedures are available (Katz et al., 2006).
Statistical Methods
Associations Between Statin Use and DNA Methylation of ABCG1
Focusing on ABCG1, we investigated all 31 CpG sites (cg00177237, cg00222799, cg01176028, cg01289965, cg01881899, cg02241241, cg02316713, cg02370100, cg02473680, cg05046272, cg0544165, cg05639842, cg06030219, cg06500161, cg07397296, cg07875759, cg08663969, cg08841829, cg10192877, cg11662315, cg14982472, cg16068063, cg17526396, cg20214535, cg21410080, cg23245768, cg25615529, cg26519745, cg26767954, cg26768067, cg27243685) that are in or near the ABCG1 gene. In the discovery stage in FHS, we modeled the association between statin use and methylation score at these ABCG1 CpG sites in (i) all participants and (ii) the participants who were not using antidiabetic medications using a generalized linear model, adjusting for family relationship, sex, age, smoking, alcohol and cell-type heterogeneity (basic model). Moreover, we used an LDL-C and TG adjusted model in which low density lipoprotein cholesterol (LDL-C) and triglycerides (TG) were added to the basic model. The analysis was implemented by the log link function in a GENMOD Procedure in SAS studio (University Edition). We fitted the identical model to the replication samples in WHI using the statistically significant CpG sites identified in FHS (P-value cut-off was set as 1.6E-3, Bonferroni correction), where adjustment for family relationship and gender were not needed, as WHI is an un-related female cohort.
Correlations Between DNA Methylation of ABCG1 and Diabetic Traits
In the discovery stage, we examined the association between methylation of ABCG1 CpG that were associated with statin use (P-value < 1.6E-3 in basic model) in both cohorts for diabetes-related traits. A generalized linear model was implemented with methylation measures as dependent variables and type 2 diabetes (selection criteria: fasting glucose ≥ 126 mg/dL, or use of diabetic medication) as the predictor using logit link function in a GENMOD Procedure in SAS, adjusting for family relationship, sex, age, smoking, alcohol use and cell-type heterogeneity. Participants without diabetes were included for a similar association study in the Log-Linear Model of GENMOD Procedure with methylation measures as dependent variables, while log-transformed glucose or insulin levels were used as predictors, adjusting for family relationship, sex, age, smoking, alcohol use and cell-type heterogeneity. We fitted the identical models in the replication samples in the WHI study. As noted previously, family relationship and gender were not adjusted in WHI because it is an un-related female cohort.
Two-Step Mendelian Randomization (MR) Approach
We performed a two-step MR approach to test the causal role of statin use on diabetic traits (fasting glucose and insulin) in the non-diabetic population and type 2 diabetes in the whole population. The first step MR analysis was implemented in the FHS study with the second in the WHI cohort using GSMR (Generalized Summary-data-based on Mendelian randomization) in GCTA (Zhu et al., 2018). In the first step, genome-wide SNPs were first tested for association with the statin use (exposure) and cg06500161 methylation (outcome) using a mixed linear model association (GCTA-MLMA), adjusting for sex, age, smoking, alcohol use, cell-type heterogeneity, and family relationship (Yang et al., 2014). These tests were repeated in the LDL-C and TG adjusted models. Then, the summary data from MLMA were used to estimate the causal effect of statin use on cg06500161 using GSMR. We selected the genome-wide significant SNPs (P-value < 5E-5) associated with the exposures as the instrumental variable in the basic and LDL-C and TG adjusted models, respectively. We used the HEIDI-outlier approach to remove SNPs that have effects on both the exposures and the outcomes. The remaining SNPs were then tested for the association with the outcomes for causal effect.
In the second step, SNPs were firstly tested in the identical models for association with cg06500161 methylation (exposure) as well as diabetes, log-transformed fasting glucose and insulin levels (outcomes), separately. In this step, the sex, age, smoking, and alcohol use, were adjusted. Next, the summary data from MLMA were utilized to calculate the causal effect of cg06500161 on type 2 diabetes and related traits using GSMR. We selected the genome-wide significant SNPs (P-value < 5E-5) associated with the exposures as the instrumental variable in diabetes, glucose and insulin models, respectively. We also used the HEIDI-outlier approach to remove SNPs that have effects on both the exposures and the outcomes. The remaining SNPs were then tested for the association with the outcomes for causal effect. Then the causal effects of statins on type 2 diabetes and related phenotypes through cg06500161 methylation were calculated by multiplying the causal effect of statin use on cg06500161 from Step 1 and the causal effect of cg06500161 on the outcomes (type 2 diabetes and related traits) from Step 2.
Gene Sets Analysis
To uncover the connection among statins, epigenomic modification and transcriptional network, we conducted gene set enrichment analysis (GSEA) in light of the identified methylation sites in the selected non-diabetic participants of the FHS study. Firstly, we performed separate association analyses between all transcripts and statins, cg06500161 methylation, log-transformed glucose as well as insulin levels using a default mixed linear model in OSCA (OmicS-data-based Complex Trait Analysis, version 0.42), adjusting for sex, age, smoking, alcohol, and cell-type heterogeneity in the LDL-C and TG adjusted model (Zhang et al., 2018). The CpG-associated (P-value < 0.05) genes were then evaluated for overlaps with those genes correlating (at P-value < 0.05) with statins, glucose or insulin levels. The number of overlapped genes was counted within each pathway. P-values and FDR q values were calculated for both KEGG and Reactome pathway data using GSEA with 45956 gene entries.
Results
Statin Use Is Associated With ABCG1 CpG Methylation
Clinical characteristics for all participants in both FHS and WHI cohorts are listed according to statin-use status and T2D in Table 1. Using an independent t-test or Fisher’s exact test, we examined the differences between statin and non-statin groups, excluding participants taking antidiabetic medication, and between T2D and non-T2D groups in both populations. In both cohorts (Table 1), participants who took statins or those with T2D, tended to have an increased risk for abnormal lipid profile, except for cholesterol (total cholesterol and LDL-C), compared to non-statin users or non-T2D participants (Table 1). One important observation was the percentage of T2D patients who took statin was about twice that of non-T2D participants in both FHS (56% vs 28%) and WHI (21% vs 10%). This suggests that T2D risk is strongly associated with statin use.
To examine the association of statins with ABCG1 methylation, we examined DNA methylation of ABCG1 on the Infinium 450K array using the FHS cohort as a discovery set, with the WHI cohort used for validation. Table 2 lists CpGs that were associated with statin use in all participants of FHS, adjusting for age, sex, smoking, alcohol use, cell-type heterogeneity, and family relationship. After filtering out highly correlated CpG sites based on pairwise Pearson correlation tests (r ≥ 0.5), five CpGs were significantly associated with statin use, with cg06500161 (β = 0.0320, P-value = 1.00E-32) being the most significant. After additionally adjusting for serum low density lipoprotein cholesterol (LDL-C) and total triglycerides (TG), the association remained significant for cg06500161 (β = 0.0252, P- value = 1.55E-15). We then sought replication in the WHI cohort, observing that only cg06500161 (β = 0.0143, P-value = 3.15E-3) and cg05639842 (β = 0.0484, P-value = 6.15E-3) were significantly associated with statin use (Table 2). Thus, both CpGs remained statistically significant in the LDL-C and TG adjusted model in both FHS and WHI (Table 2). We found a similar association of statin use with ABCG1 methylation in participants who were not using antidiabetic medication in both FHS and WHI cohorts (Table 3).
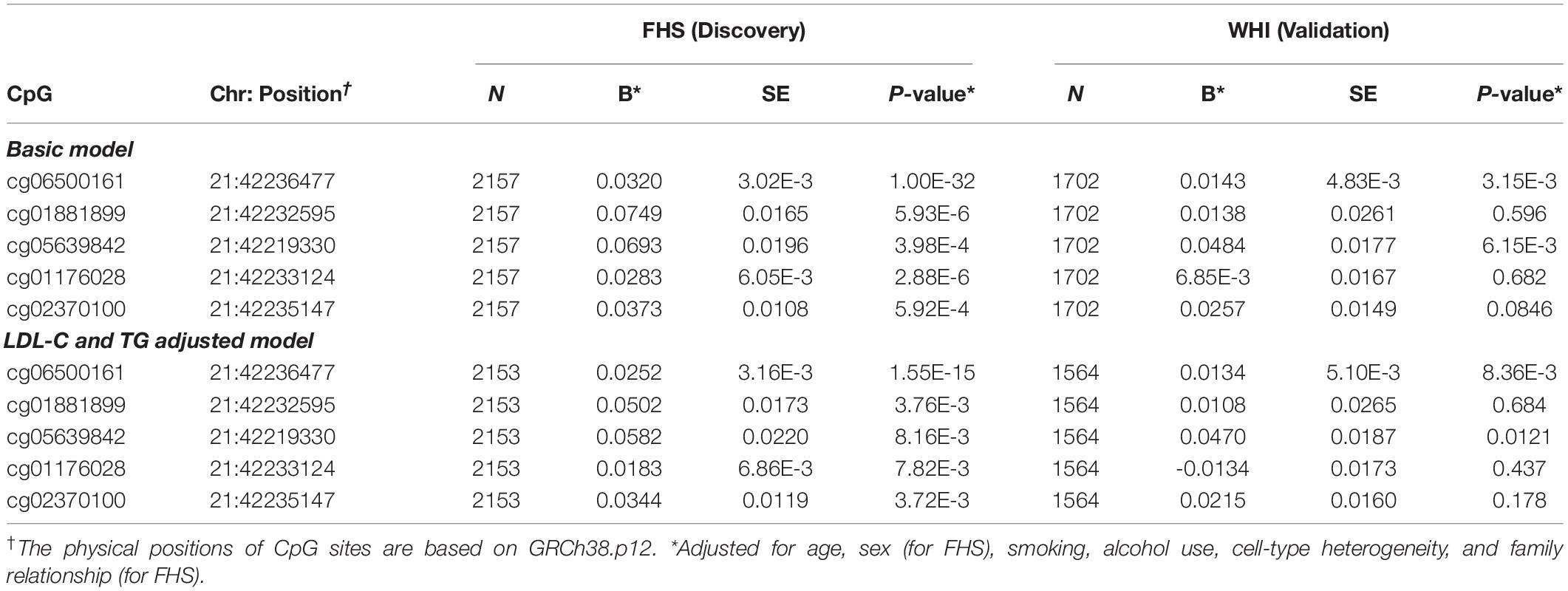
Table 2. ABCG1 CpG sites associated with statin use in all participants from the FHS and WHI studies.
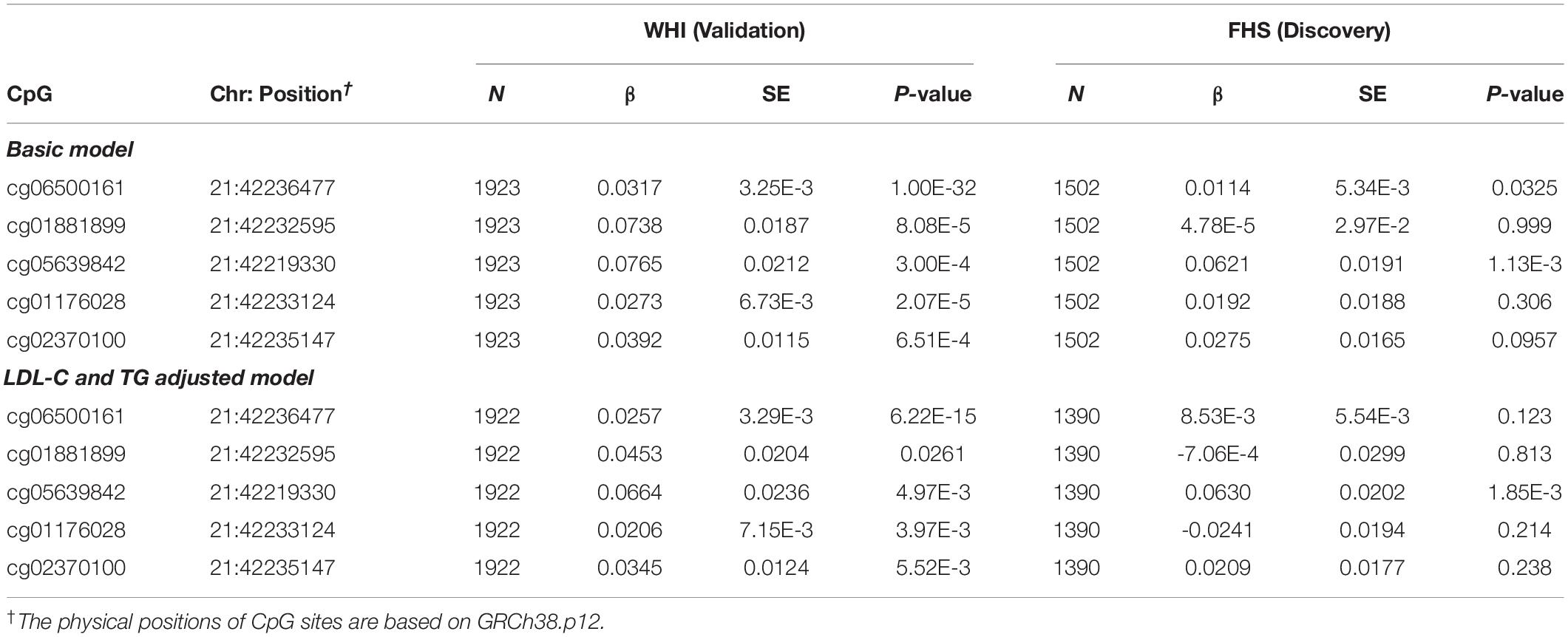
Table 3. ABCG1 CpG sites associated with statin use in participants who are not using antidiabetic medication from the FHS and WHI studies.
ABCG1 CpG Methylation Is Associated With Type 2 Diabetes and Related Traits
Because epigenetic analysis of other cohorts identified associations between DNA methylation at ABCG1 and type 2 diabetes phenotypes, we tested both cg06500161 and cg05639842 for association with type 2 diabetes in the FHS cohort. Replication was sought in the WHI cohort. We observed greater type 2 diabetes prevalence with higher DNA methylation levels at cg06500161 (β = 12.4, P-value = 1.33E-10) in FHS (Table 4). Similar results with cg06500161 were found in WHI (β = 11.7, P-value = 4.70E-11). However, cg05639842 was not associated with type 2 diabetes in either FHS or WHI (Table 4).
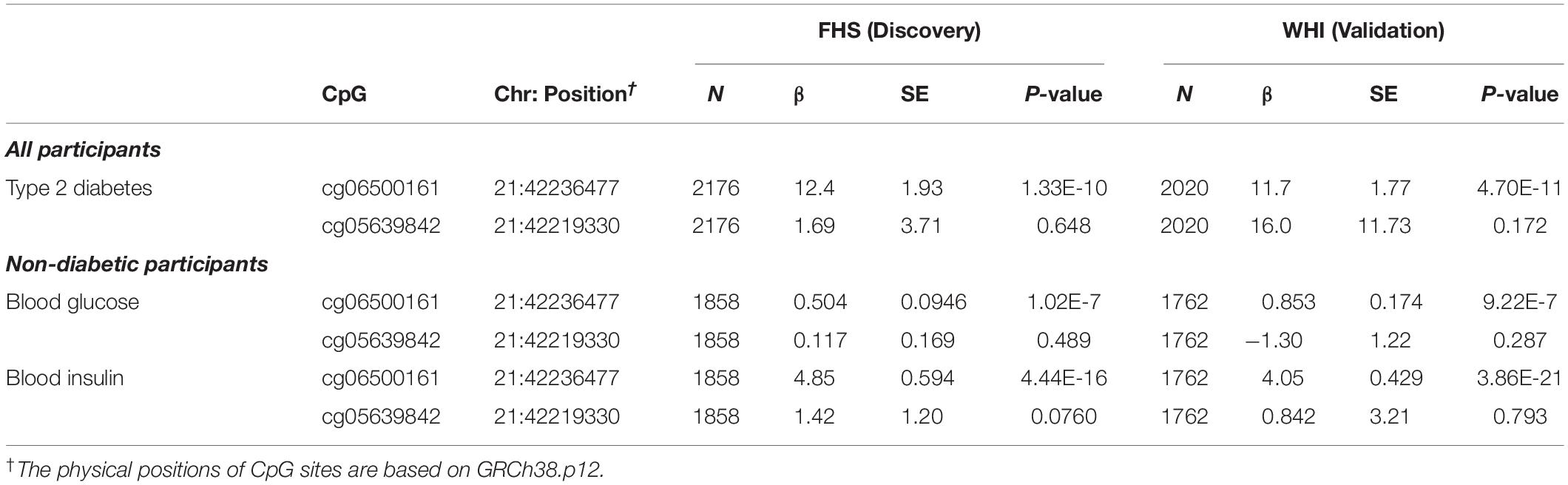
Table 4. ABCG1 CpG sites associated with type 2 diabetes, fasting glucose and fasting insulin in participants from the FHS and WHI studies.
For the prediction of type 2 diabetes, we investigated DNA methylation at cg06500161 and cg05639842 with common risk factors for type 2 diabetes (fasting blood glucose and insulin levels) in non-diabetic participants in both cohorts. DNA methylation at cg06500161 was positively correlated with fasting glucose (β = 0.504, P-value = 1.02E-7) and insulin levels (β = 4.85, P-value = 4.44E-16) in FHS, adjusting for age, sex, smoking, alcohol, cell-type heterogeneity and family relationship, while DNA methylation at cg05639842 was not correlated with fasting glucose or insulin levels in the same model (Table 4). We found similar associations between cg06500161 methylation and fasting glucose (β = 0.853, P-value = 9.22E-7) and insulin levels (β = 4.05, P-value = 3.86E-21) in the WHI study (Table 4).
Causal Effect of Statin Use on Type 2 Diabetes and Related Traits Through Epigenetics of ABCG1 DNA Methylation
To determine the causal effect of statin use on type 2 diabetes risk/status and its related traits through DNA methylation at cg06500161, we conducted a two-step epigenetic Mendelian randomization (MR) approach in FHS and WHI consecutively. In the first step performed in FHS, SNPs were tested for associations with statin use and cg06500161 methylation, respectively, in (a) all participants and (b) non-diabetic participants. GSMR models then were implemented based on the summary results from GWAS, adjusting for sex, age, smoking, alcohol use, and cell-type heterogeneity. In this step, SNPs that were only associated with statin use were used to calculate a genetic score as the instrumental variable. For all participants, statin use was validated as a significant causal factor with cg06500161 methylation as the outcome (basic model: β = 0.0186, P-value = 2.56E-35; LDL-C and TG adjusted model: β = 0.0157, P-value = 1.05E-19; Table 5). We obtained similar results in the non-diabetic participants (basic model: β = 0.0179, P-value = 9.64E-31; LDL-C and TG adjusted model: β = 0.0142, P-value = 2.12E-19; Table 5).
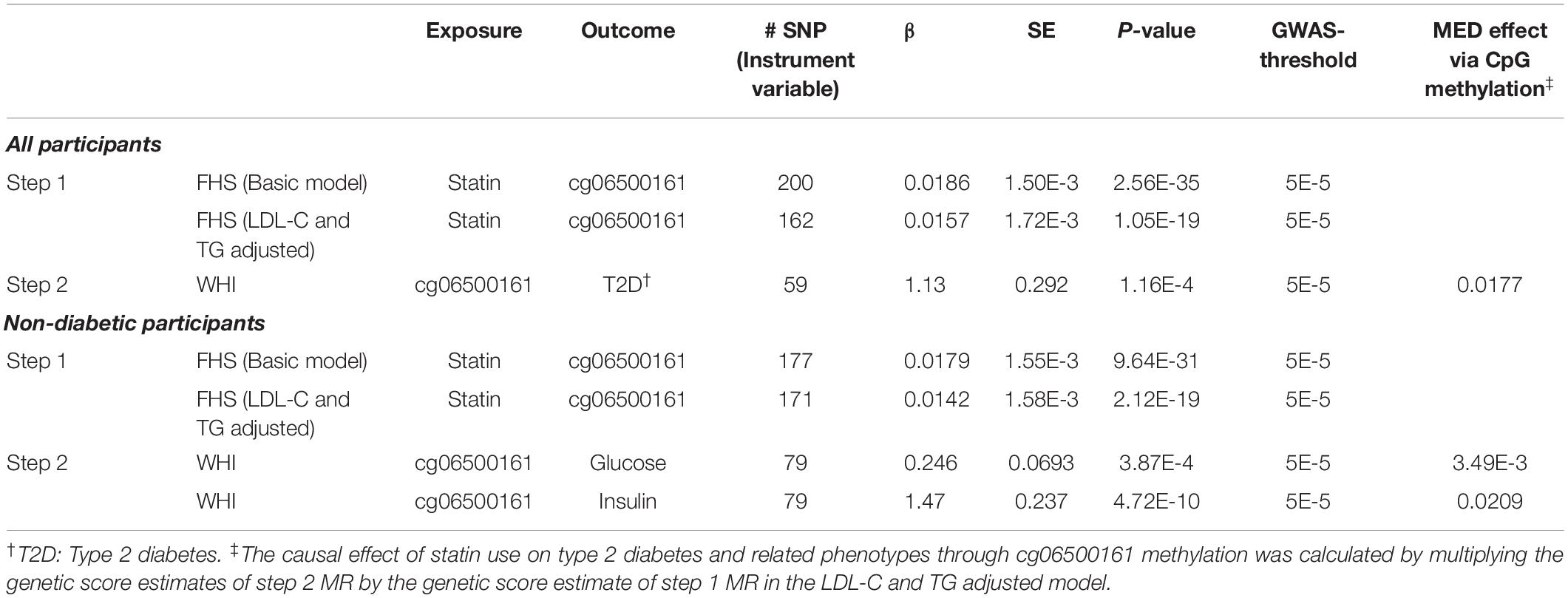
Table 5. Two-step Mendelian randomization (MR) analysis in participants from the FHS and WHI studies.
In the second step of the MR analysis, conducted in WHI, we fitted a similar basic model for associations between cg06500161 methylation and type 2 diabetes in all participants, as well as cg06500161 and fasting glucose, cg06500161 and fasting insulin in the non-diabetic participants. Similar GSMR models were also implemented (diabetes: β = 1.13, P-value = 1.16E-4; glucose: β = 0.246, P-value = 3.87E-04; insulin: β = 1.47, P-value = 4.72E-10; Table 5). In this GSMR model, we used 59, 79 and 79 SNPs (Supplementary Table S1) as the instrumental variables in diabetes, glucose and insulin models, respectively. CpG cg06500161 methylation was validated as the causal factor with type 2 diabetes, glucose and insulin as the outcomes. Combining results from the first and second steps of MR, the causal effect of statins on type 2 diabetes, glucose, and insulin through cg06500161 methylation was calculated as 0.0177, 3.49E-3 and 0.0209, respectively (Table 5).
Links Between Statin Use and DNA Methylation, and Gene Expression
To examine potential mechanisms through which statins mediated DNA methylation at cg06500161 that led to increased risk of diabetes, we separately correlated gene expression levels with statin use, cg06500161 methylation and fasting glucose and insulin levels. This was done with available gene expression data from all non-diabetic FHS participants. As shown in Supplementary Table S2, seventy-seven transcripts were correlated with both statin use and cg06500161 methylation. Fifty-six transcripts were found to be correlated with both fasting glucose and DNA methylation of cg06500161, and 80 transcripts were associated with both fasting insulin and cg06500161 methylation (Supplementary Table S2). Gene set enrichment analysis conducted with both KEGG and REACTOME gene-pathway assignments at an FDR of 10% suggested that the genes doubly associated with cg06500161 methylation and insulin are primarily involved in cholesterol and lipoprotein metabolic pathways (Table 6).
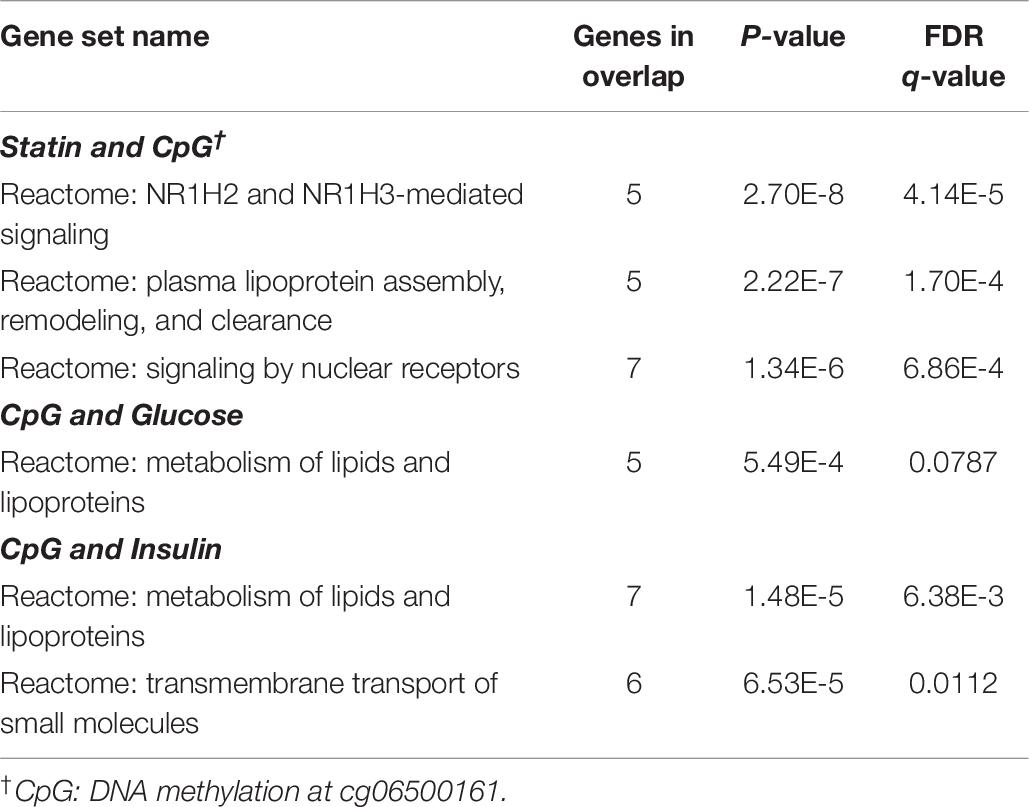
Table 6. Overlaps for doubly-associated gene expression in non-diabetic participants from the FHS study (genes in overlap ≥ 5, LDL-C and TG adjusted model).
When combining the transcripts whose expression in whole blood associated significantly with statin use, cg06500161 methylation and insulin level, 16 genes were found correlated with each of three different outcomes, with the greatest responses involved in cholesterol, fatty acid and lipid transport and homeostasis (Figure 1). Specifically, it should be noted that ABCG1, ABCA1 and ACSL3 were negatively, while KAT2B positively, associated with all signatures of statin use, CpG methylation and diabetes risk/status (Supplementary Tables S3, S4).
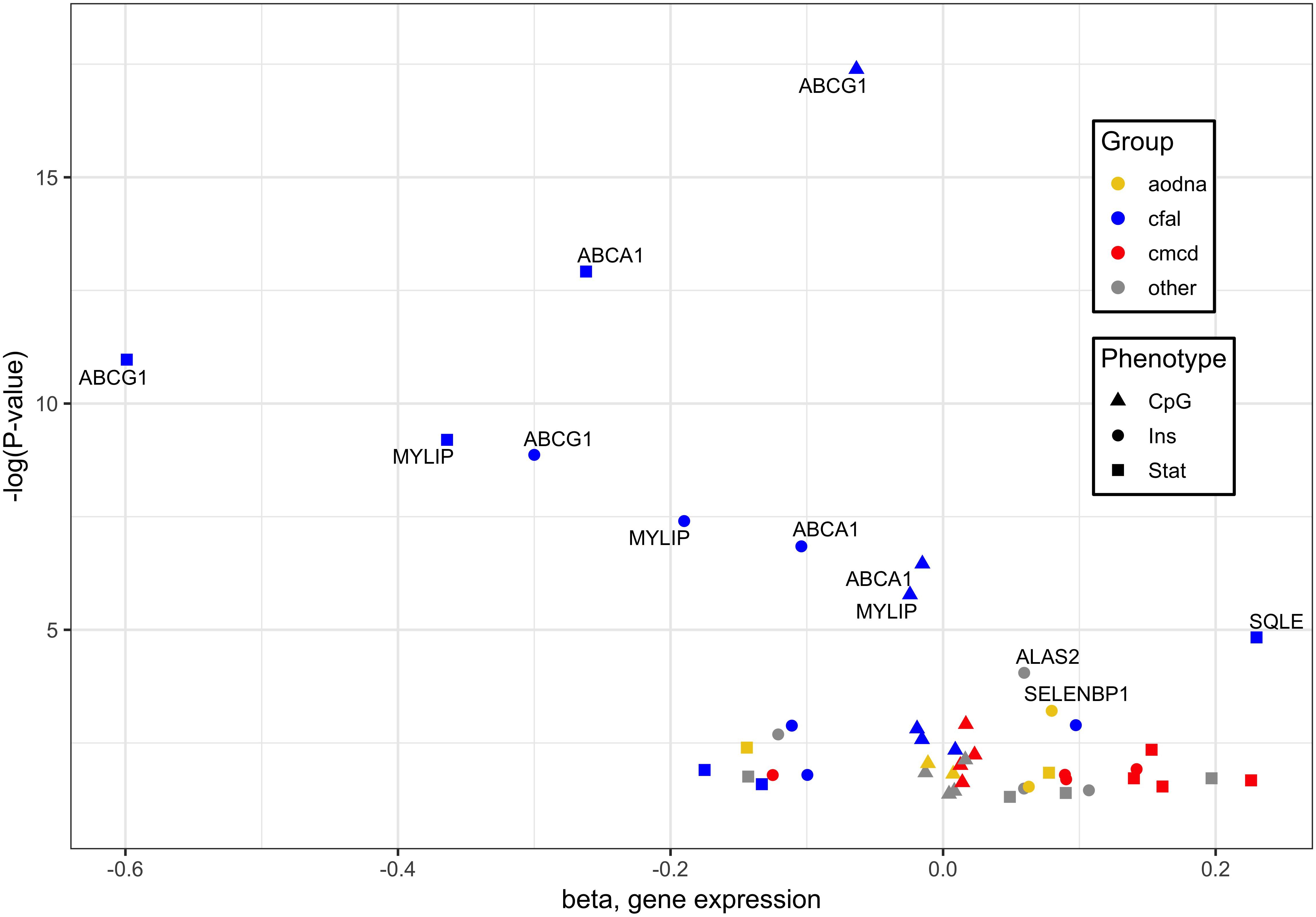
Figure 1. Genes whose expression associated significantly with cg06500161 methylation, glycemic traits and statin use. Of 16 genes whose expression in whole blood associated significantly with each of three different outcomes, those with the greatest responses are involved in cholesterol, fatty acid and lipid transport and homeostasis (blue). Associations were derived from methylation at ABCG1 CpG cg06500161, glycemic factors, and statin use, designated by triangles, circles and squares, respectively. Other notable functional groups include cell migration and cytoskeletal dysnamics (red) and antioxidant defense and DNA damage response (orange). Plotted data taken from Supplementary Table S3.
Discussion
Our findings imply that statin use mediates DNA methylation at cg06500161 of ABCG1 and this contributes to increased risk of type 2 diabetes. Firstly, we have shown that statin use was associated with DNA methylation modifications of ABCG1 and such methylation patterns are correlated with the risk of type 2 diabetes. Secondly, Mendelian randomization demonstrates that statin use has moderate causal effects on type 2 diabetes and diabetes-related traits through differential methylation at cg06500161. Moreover, our results suggest that statin use and the methylation modifications in ABCG1 are correlated with the expression of genes involved in both lipid metabolism and glycemic pathways.
This study reveals a potential epigenetic mechanism mediating the effect of statin use on glycemic signatures. The associations between epigenetic variants at ABCG1 and diabetic traits have been observed previously (Hidalgo et al., 2014; Chambers et al., 2015; Dayeh et al., 2016), but little is known about the association between lipid-lowering medications and DNA methylation of ABCG1. Because DNA methylation at cg06500161 of ABCG1 in blood has been associated positively with triglyceride levels and negatively with HDL-C levels (Dekkers et al., 2016; Braun et al., 2017), it is plausible that blood lipids have a causal effect on the epigenetic status at ABCG1. For this reason, in this study, we controlled for blood LDL cholesterol and triglyceride levels in our analyses. Importantly, our data from the LDL-C and TG adjusted model remained significant, indicating that statin use is an independent factor of higher cg06500161 methylation and consequently increased diabetic risk. Hence, the results from MR analysis have demonstrated that statin use is highly likely the causal factor of increased cg06500161 methylation and diabetic risk.
ABCG1 encodes a protein member in the ATP-binding cassette (ABC) transporters superfamily, which transports specific intracellular sterols away from the endoplasmic reticulum (Tarling and Edwards, 2011). ABCG1 has shown an allelic imbalance of expression in human pancreatic islet cells suggesting both genetic and epigenetic roles in altering the allelic expression of ABCG1 (Serre et al., 2008). Indeed, genetic variants contribute to differences in ABCG1 expression (Matsson et al., 2012). Does genetic variation impart stronger regulation of ABCG1 expression than epigenetic modification? Previous GWAS have reported negative results in the associations between ABCG1 and risk for type 2 diabetes (Vattikuti and Towler, 2004; Saxena et al., 2010). Coding SNPs in ABCG1 were found not to be associated with risks for type 2 diabetes in the Copenhagen General Population Study (Schou et al., 2012). Frisdal et al. further demonstrated that ABCG1 SNPs (rs1893590 and rs1378577) in severely obese individuals were not associated with the presence of diabetes or HOMA-IR (Frisdal et al., 2015). In the present study, we did not find any significant association between ABCG1 SNPs with T2D, fasting glucose, or insulin levels in either FHS or WHI cohorts. The mechanisms of genetic variants on ABCG1 and type 2 diabetes remain unclear and controversial, suggesting that epigenetic processes might be involved in the regulation of ABCG1 and downstream gene expression, and ultimately diabetic signatures. Our results and those of others (Dayeh et al., 2016) show that increased DNA methylation at ABCG1 was positively associated with diabetic signatures providing substantial evidence in support of these correlations between ABCG1 and type 2 diabetes from an epigenetic perspective.
ABCG1 is a central regulator of cellular lipid homeostasis (Schmitz et al., 2001). Recently, several reports also suggest that ABCG1 may be involved in glucose metabolism. ABCG1 has been reported to mediate cholesterol transport and thus influence insulin secretion in pancreatic β-cells (Sturek et al., 2010; Kruit et al., 2012). Decreased ABCG1 expression has been found in skeletal muscle from subjects with type 2 diabetes (Dayeh et al., 2016). In order to investigate possible methylation-driven gene expression changes and uncover the potential mechanism of diabetes risk mediated by statins, we examined the correlation of the transcriptome with statins, cg06500161 methylation status, and glucose and insulin levels in all non-diabetic participants in FHS. The expressions of ABCG1, ABCA1, ACSL3 were negatively, while KAT2B positively, associated with the medication, cg06500161 methylation, and glucose and insulin levels. KAT2B (lysine acetyltransferase 2B), functions as a histone acetyltransferase to promote transcriptional activation (Ogryzko et al., 1996), has been reported to stimulate hepatic gluconeogenesis and increase circulating blood glucose concentrations, whereas inhibition of KAT2B improves glucose balance in insulin-resistant mice (Ravnskjaer et al., 2013). ABCA1 (ATP binding cassette subfamily A member 1), which normally moves cholesterol and phospholipids across cell membranes, could increase insulin secretion in pancreatic β-cells (Kruit et al., 2012). Additionally, silencing ACSL3 and ACSL4 (Long-chain acyl-CoA synthetases), which are responsible for activation of long-chain fatty acids, have been found to inhibit glucose-stimulated insulin release in pancreatic β-cells (Ansari et al., 2017). Meanwhile, previous findings, as illustrated in Figure 2, also have shown that reduced ABCA1 and ACSL3 expression impairs the phosphorylation of AKT, resulting in insulin resistance (de Haan et al., 2014; Li et al., 2018). Thus, decreased expression of ABCG1, ABCA1, and ACSL3 could impair insulin secretion and lead to insulin resistance. All this supports the positive correlations observed here between statin use and insulin levels as well as glucose levels, which result in an increased risk of type 2 diabetes. It is also worth noting that other mechanisms such as epigenetic changes on non-coding RNAs and histone modifications might also affect the association between the statin use and T2D risk (Muka et al., 2016; Sun and Wong, 2016), and further studies are warranted to elucidate these mechanisms.
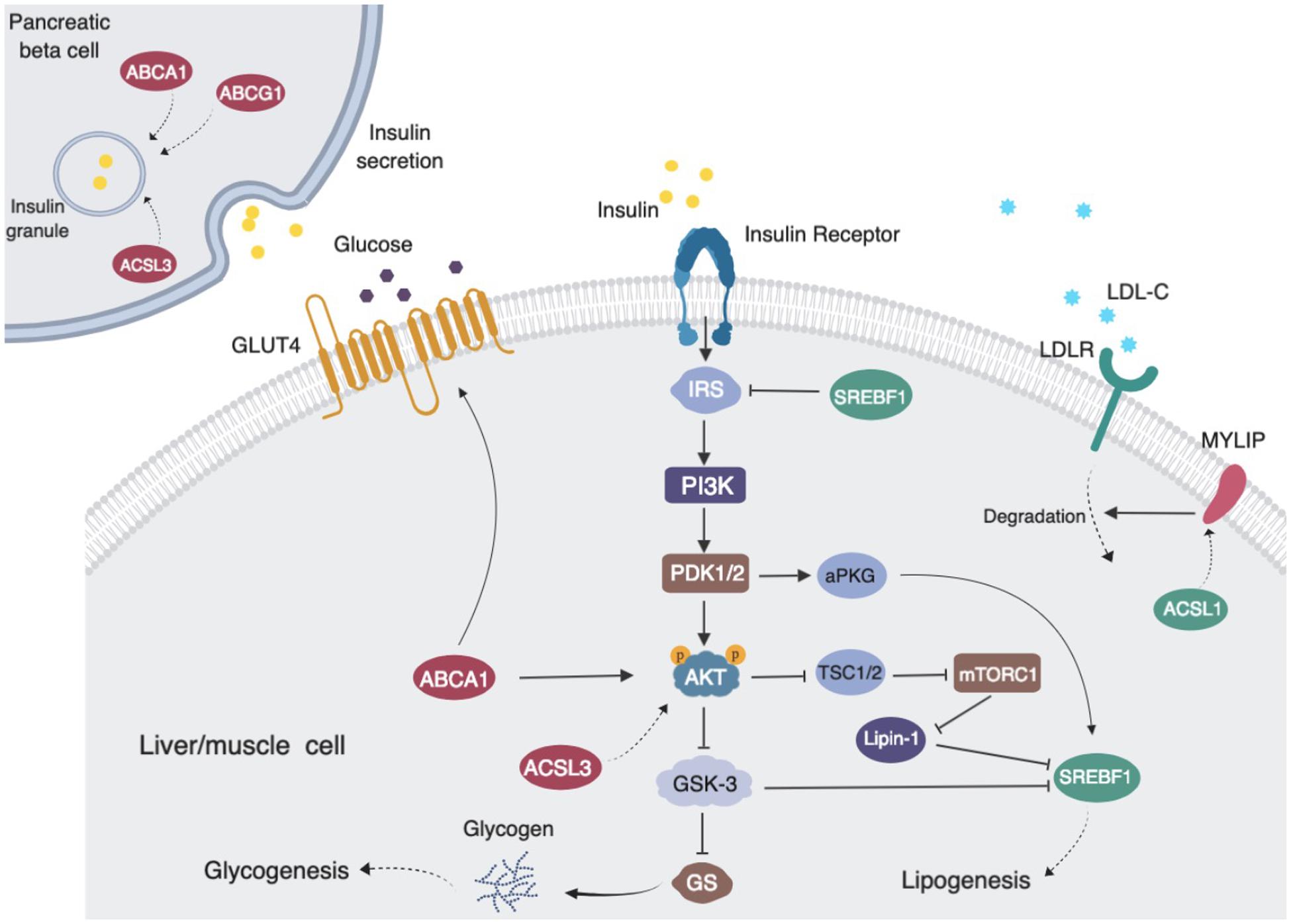
Figure 2. Proposed pathways of statin use associating with cg06500161 methylation, fasting blood glucose and fasting blood insulin levels (LDL-C and TG adjusted model). ABCG1, ABCA1, ACSL3 and MYLIP (dark red) expression levels were negatively associated with medication use, CpG methylation and diabetic signatures. SREBF1 and ACSL3 (dark green) expression levels were inversely associated with medication use and CpG methylation. Statin use induced ABCG1 methylation that leads to decreased expression of ABCG1, then altered insulin sensitivity and insulin secretion and increased risk of diabetes.
Some limitations should be acknowledged when interpreting the results of this study. First, there were differences in gender distribution between the two cohorts, in particular, WHI includes females only, which might contribute to slight inconsistencies in associations between statin use and methylation at ABCG1. Second, some glucose and insulin metabolism take place in the liver, pancreatic islet, adipose tissue, and skeletal muscle, but the available data permitted investigation of the mechanisms only in blood. This limitation in the sample type should not undermine the link between statin use and diabetes risk, as DNA methylation status in blood often reflects the epigenetic status in other tissues (Bysani et al., 2016; Crujeiras et al., 2017). Nevertheless, our findings suggest that further investigation is warranted in order to further characterize the molecular mechanism of increased diabetes risk with specific statin use.
In summary, we report DNA methylation site cg06500161 at ABCG1 as a mediator of the association between statins and type 2 diabetes. These findings provide insights into the epigenetic mechanisms of statins influencing glycemic traits and further, type 2 diabetes pathogenesis. We show evidence for cg06500161 as a potential predictor or therapeutic target for prevention of type 2 diabetes. Implementation of functional studies of cg06500161 will uncover the precise molecular mechanisms. The eventual goal is to translate this information into clinical use, specifically, to develop useful prediction and prevention strategies for type 2 diabetes.
Data Availability Statement
Publicly available datasets were analyzed in this study. These data can be found here: dbGaP: phs000200.v11.p3, phs000746.v2.p3, phs000342.v18.p11, phs000007.v29.p10, and phe00002.v6.
Ethics Statement
The studies involving human participants were reviewed and approved by the Institutional Review Board (IRB) at Tufts University. The patients/participants provided their written informed consent to participate in this study.
Author Contributions
C-QL and YL conceptualized and designed the study. YL, YS, TG, and LP contributed to data analysis. KW contributed to the processing and quality control of imputed genotypes of acquired through dbGaP. LP, JO, YS, TG, and CS critically evaluated the manuscript. JO is responsible for the funding and supervision of the study. YL and C-QL wrote and edited the manuscript. All authors read and approved the final manuscript.
Funding
This work was partially supported by grant 201806105018 from the China Scholarship Council (CSC), and by the United States Department of Agriculture, under agreement no. 8050-51000-098-00D.
Conflict of Interest
The authors declare that the research was conducted in the absence of any commercial or financial relationships that could be construed as a potential conflict of interest.
Acknowledgments
The Genotype-Tissue Expression (GTEx) Project was supported by the Common Fund of the Office of the Director of the National Institutes of Health, and by NCI, NHGRI, NHLBI, NIDA, NIMH, and NINDS. Any opinions, findings, conclusion, or recommendations expressed in this publication are those of the authors and do not necessarily reflect the view of the United States Department of Agriculture. None of the authors had a conflict of interest. Mention of trade names or commercial products in this publication is solely for the purpose of providing specific information and does not imply recommendation or endorsement by the United States Department of Agriculture. The USDA is an equal opportunity provider and employer.
Supplementary Material
The Supplementary Material for this article can be found online at: https://www.frontiersin.org/articles/10.3389/fgene.2020.00622/full#supplementary-material
Footnotes
References
Aguirre, L., Hijona, E., Macarulla, M. T., Gracia, A., Larrechi, I., Bujanda, L., et al. (2013). Several statins increase body and liver fat accumulation in a model of metabolic syndrome. J. Physiol. Pharmacol. 64, 281–288.
Ansari, I. H., Longacre, M. J., Stoker, S. W., Kendrick, M. A., O’Neill, L. M., Zitur, L. J., et al. (2017). Characterization of Acyl-CoA synthetase isoforms in pancreatic beta cells: gene silencing shows participation of ACSL3 and ACSL4 in insulin secretion. Arch. Biochem. Biophys. 618, 32–43. doi: 10.1016/j.abb.2017.02.001
Braun, K. V. E., Dhana, K., de Vries, P. S., Voortman, T., van Meurs, J. B. J., Uitterlinden, A. G., et al. (2017). Epigenome-wide association study (EWAS) on lipids: the Rotterdam Study. Clin. Epigenet. 9:15.
Bysani, M., Perfilyev, A., de Mello, V. D., Rönn, T., Nilsson, E., Pihlajamäki, J., et al. (2016). Epigenetic alterations in blood mirror age-associated DNA methylation and gene expression changes in human liver. Epigenomics 9, 105–122. doi: 10.2217/epi-2016-0087
Caramaschi, D., Sharp, G. C., Nohr, E. A., Berryman, K., Lewis, S. J., Davey Smith, G., et al. (2017). Exploring a causal role of DNA methylation in the relationship between maternal vitamin B12 during pregnancy and child’s IQ at age 8, cognitive performance and educational attainment: a two-step Mendelian randomization study. Hum. Mol. Genet. 26, 3001–3013. doi: 10.1093/hmg/ddx164
Chambers, J. C., Loh, M., Lehne, B., Drong, A., Kriebel, J., Motta, V., et al. (2015). Epigenome-wide association of DNA methylation markers in peripheral blood from Indian Asians and Europeans with incident type 2 diabetes: a nested case-control study. Lancet Diabetes Endocrinol. 3, 526–534.
Chang, C. C., Chow, C. C., Tellier, L. C., Vattikuti, S., Purcell, S. M., and Lee, J. J. (2015). Second-generation PLINK: rising to the challenge of larger and richer datasets. Gigascience 4:7.
Chou, R., Dana, T., Blazina, I., Daeges, M., and Jeanne, T. L. (2016). Statins for prevention of cardiovascular disease in adults: evidence report and systematic review for the US preventive services task force. JAMA 316, 2008–2024. doi: 10.1001/jama.2015.15629
Crujeiras, A. B., Diaz-Lagares, A., Sandoval, J., Milagro, F. I., Navas-Carretero, S., Carreira, M. C., et al. (2017). DNA methylation map in circulating leukocytes mirrors subcutaneous adipose tissue methylation pattern: a genome-wide analysis from non-obese and obese patients. Sci. Rep. 7:41903. doi: 10.1038/srep41903
Davegardh, C., Garcia-Calzon, S., Bacos, K., and Ling, C. (2018). DNA methylation in the pathogenesis of type 2 diabetes in humans. Mol. Metab. 14, 12–25. doi: 10.1016/j.molmet.2018.01.022
Dawber, T. R., Meadors, G. F., and Moore, F. E. Jr. (1951). Epidemiological approaches to heart disease: the Framingham Study. Am. J. Public Health 41, 279–281.
Dayeh, T., Tuomi, T., Almgren, P., Perfilyev, A., Jansson, P. A., de Mello, V. D., et al. (2016). DNA methylation of loci within ABCG1 and PHOSPHO1 in blood DNA is associated with future type 2 diabetes risk. Epigenetics 11, 482–488. doi: 10.1080/15592294.2016.1178418
de Haan, W., Bhattacharjee, A., Ruddle, P., Kang, M. H., and Hayden, M. R. (2014). ABCA1 in adipocytes regulates adipose tissue lipid content, glucose tolerance, and insulin sensitivity. J. Lipid Res. 55, 516–523. doi: 10.1194/jlr.M045294
Dekkers, K. F., van Iterson, M., Slieker, R. C., Moed, M. H., Bonder, M. J., van Galen, M., et al. (2016). Blood lipids influence DNA methylation in circulating cells. Genome Biol. 17:138.
Frisdal, E., Le Lay, S., Hooton, H., Poupel, L., Olivier, M., Alili, R., et al. (2015). Adipocyte ATP-binding cassette G1 promotes triglyceride storage, fat mass growth, and human obesity. Diabetes 64, 840–855. doi: 10.2337/db14-0245
Genvigir, F. D., Rodrigues, A. C., Cerda, A., Hirata, M. H., Curi, R., and Hirata, R. D. (2011). ABCA1 and ABCG1 expressions are regulated by statins and ezetimibe in Caco-2 cells. Drug Metabol. Drug Interact. 26, 33–36. doi: 10.1515/DMDI.2011.101
Hidalgo, B., Irvin, M. R., Sha, J., Zhi, D., Aslibekyan, S., Absher, D., et al. (2014). Epigenome-wide association study of fasting measures of glucose, insulin, and HOMA-IR in the genetics of lipid lowering drugs and diet network study. Diabetes 63, 801–807. doi: 10.2337/db13-1100
Kannel, W. B., Feinleib, M., McNamara, P. M., Garrison, R. J., and Castelli, W. P. (1979). An investigation of coronary heart disease in families: the Framingham offspring study. Am. J. Epidemiol. 110, 281–290. doi: 10.1093/oxfordjournals.aje.a112813
Katz, S., Irizarry, R. A., Lin, X., Tripputi, M., and Porter, M. W. (2006). A summarization approach for Affymetrix GeneChip data using a reference training set from a large, biologically diverse database. BMC Bioinformatics 7:464. doi: 10.1186/1471-2105-7-464
Kim, D.-W., Kim, D.-H., Park, J.-H., Choi, M., Kim, S., Kim, H., et al. (2019). Association between statin treatment and new-onset diabetes mellitus: a population based case–control study. Diabetol. Metab. Syndr. 11:30.
Kim, Y. S., Han, Y. E., Choi, E. A., You, N. Y., Lee, J. W., You, H. S., et al. (2019). Statin use increased new-onset diabetes in hypercholesterolemic individuals: data from the Korean National Health Insurance Service-National Health Screening Cohort database (NHIS-HEALS). Prim Care Diabetes 14, 246–253. doi: 10.1016/j.pcd.2019.08.005
Kruit, J. K., Wijesekara, N., Westwell-Roper, C., Vanmierlo, T., de Haan, W., Bhattacharjee, A., et al. (2012). Loss of both ABCA1 and ABCG1 results in increased disturbances in islet sterol homeostasis, inflammation, and impaired β-cell function. Diabetes 61, 659–664. doi: 10.2337/db11-1341
Lai, C.-Q., Smith, C. E., Parnell, L. D., Lee, Y.-C., Corella, D., Hopkins, P., et al. (2018). Epigenomics and metabolomics reveal the mechanism of the APOA2-saturated fat intake interaction affecting obesity. Am. J. Clin. Nutr. 108, 188–200. doi: 10.1093/ajcn/nqy081
Li, K., Mao, Y.-H., Qiu, W.-H., He, J.-W., Wang, D.-J., Hu, C., et al. (2018). Acyl-CoA synthetase long-chain 3 regulates AKT phosphorylation and the functional activity of human prostate cancer cells. J. BioX Res. 1, 56–61. doi: 10.1097/jbr.0000000000000009
Lindsey, C. C., Graham, M. R., Johnston, T. P., Kiroff, C. G., and Freshley, A. (2004). A clinical comparison of calculated versus direct measurement of low-density lipoprotein cholesterol level. Pharmacotherapy 24, 167–172. doi: 10.1592/phco.24.2.167.33142
Marioni, R. E., Shah, S., McRae, A. F., Chen, B. H., Colicino, E., Harris, S. E., et al. (2015). DNA methylation age of blood predicts all-cause mortality in later life. Genome Biol. 16:25.
Matsson, P., Yee, S. W., Markova, S., Morrissey, K., Jenkins, G., Xuan, J., et al. (2012). Discovery of regulatory elements in human ATP-binding cassette transporters through expression quantitative trait mapping. Pharmacogenomics J. 12, 214–226. doi: 10.1038/tpj.2011.8
Matsuo, M. (2010). ATP-binding cassette proteins involved in glucose and lipid homeostasis. Biosci. Biotechnol. Biochem. 74, 899–907. doi: 10.1271/bbb.90921
Morris, T. J., Butcher, L. M., Feber, A., Teschendorff, A. E., Chakravarthy, A. R., Wojdacz, T. K., et al. (2014). ChAMP: 450k chip analysis methylation pipeline. Bioinformatics 30, 428–430. doi: 10.1093/bioinformatics/btt684
Muka, T., Nano, J., Voortman, T., Braun, K. V. E., Ligthart, S., Stranges, S., et al. (2016). The role of global and regional DNA methylation and histone modifications in glycemic traits and type 2 diabetes: a systematic review. Nutr. Metab. Cardiovasc. Dis. 26, 553–566. doi: 10.1016/j.numecd.2016.04.002
Nilsson, E., and Ling, C. (2017). DNA methylation links genetics, fetal environment, and an unhealthy lifestyle to the development of type 2 diabetes. Clin. Epigenetics 9:105.
Obeidat, M., Fishbane, N., Nie, Y., Chen, V., Hollander, Z., Tebbutt, S. J., et al. (2015). The effect of statins on blood gene expression in COPD. PLoS One 10:e0140022. doi: 10.1371/journal.pone.0140022
Ogryzko, V. V., Schiltz, R. L., Russanova, V., Howard, B. H., and Nakatani, Y. (1996). The transcriptional coactivators p300 and CBP are histone acetyltransferases. Cell 87, 953–959. doi: 10.1016/s0092-8674(00)82001-2
Pirillo, A., and Catapano, A. L. (2015). Statin intolerance: diagnosis and remedies. Curr. Cardiol. Rep. 17:27. doi: 10.1007/s11886-015-0582-z
Ravnskjaer, K., Hogan, M. F., Lackey, D., Tora, L., Dent, S. Y., Olefsky, J., et al. (2013). Glucagon regulates gluconeogenesis through KAT2B- and WDR5-mediated epigenetic effects. J. Clin. Invest. 123, 4318–4328. doi: 10.1172/jci69035
Relton, C. L., and Davey Smith, G. (2012). Two-step epigenetic Mendelian randomization: a strategy for establishing the causal role of epigenetic processes in pathways to disease. Int. J. Epidemiol. 41, 161–176. doi: 10.1093/ije/dyr233
Saxena, R., Hivert, M. F., Langenberg, C., Tanaka, T., Pankow, J. S., Vollenweider, P., et al. (2010). Genetic variation in GIPR influences the glucose and insulin responses to an oral glucose challenge. Nat. Genet. 42, 142–148. doi: 10.1038/ng.521
Schmitz, G., Langmann, T., and Heimerl, S. (2001). Role of ABCG1 and other ABCG family members in lipid metabolism. J. Lipid Res. 42, 1513–1520.
Schou, J., Tybjaerg-Hansen, A., Moller, H. J., Nordestgaard, B. G., and Frikke-Schmidt, R. (2012). ABC transporter genes and risk of type 2 diabetes: a study of 40,000 individuals from the general population. Diabetes Care 35, 2600–2606. doi: 10.2337/dc12-0082
Serre, D., Gurd, S., Ge, B., Sladek, R., Sinnett, D., Harmsen, E., et al. (2008). Differential allelic expression in the human genome: a robust approach to identify genetic and epigenetic cis-acting mechanisms regulating gene expression. PLoS Genet. 4:e1000006. doi: 10.1371/journal.pgen.1000006
Sturek, J. M., Castle, J. D., Trace, A. P., Page, L. C., Castle, A. M., Evans-Molina, C., et al. (2010). An intracellular role for ABCG1-mediated cholesterol transport in the regulated secretory pathway of mouse pancreatic beta cells. J. Clin. Invest. 120, 2575–2589. doi: 10.1172/JCI41280
Sun, X., and Wong, D. (2016). Long non-coding RNA-mediated regulation of glucose homeostasis and diabetes. Am. J. Cardiovasc. Dis. 6, 17–25.
Tarling, E. J., and Edwards, P. A. (2011). ATP binding cassette transporter G1 (ABCG1) is an intracellular sterol transporter. Proc. Natl. Acad. Sci. U.S.A. 108, 19719–19724. doi: 10.1073/pnas.1113021108
The Women’s Health Initiative Study Group (1998). Design of the Women’s Health Initiative clinical trial and observational study. The Women’s Health Initiative Study Group. Control. Clin. Trials 19, 61–109. doi: 10.1016/s0197-2456(97)00078-0
Vattikuti, R., and Towler, D. A. (2004). Osteogenic regulation of vascular calcification: an early perspective. Am. J. Physiol. Endocrinol. Metab. 286, E686–E696. doi: 10.1152/ajpendo.00552.2003
Vickers, M. H. (2014). Early life nutrition, epigenetics and programming of later life disease. Nutrients 6, 2165–2178. doi: 10.3390/nu6062165
Yang, J., Zaitlen, N. A., Goddard, M. E., Visscher, P. M., and Price, A. L. (2014). Advantages and pitfalls in the application of mixed-model association methods. Nat. Genet. 46, 100–106. doi: 10.1038/ng.2876
Zhan, S., Tang, M., Liu, F., Xia, P., Shu, M., and Wu, X. (2018). Ezetimibe for the prevention of cardiovascular disease and all-cause mortality events. Cochrane Database Syst. Rev. 11:Cd012502. doi: 10.1002/14651858.CD012502.pub2
Zhang, F., Chen, W., Zhu, Z., Zhang, Q., Deary, I. J., Wray, N. R., et al. (2018). OSCA: a tool for omic-data-based complex trait analysis. bioRxiv [Preprint] doi: 10.1101/445163
Keywords: statin, ABCG1, methylation, type 2 diabetes, cg06500161
Citation: Liu Y, Shen Y, Guo T, Parnell LD, Westerman KE, Smith CE, Ordovas JM and Lai C-Q (2020) Statin Use Associates With Risk of Type 2 Diabetes via Epigenetic Patterns at ABCG1. Front. Genet. 11:622. doi: 10.3389/fgene.2020.00622
Received: 18 February 2020; Accepted: 22 May 2020;
Published: 16 June 2020.
Edited by:
Suowen Xu, University of Science and Technology of China, ChinaReviewed by:
Wilfried Le Goff, Institut National de la Santé et de la Recherche Médicale (INSERM), FranceLine Hjort, Rigshospitalet, Denmark
Michinori Matsuo, Kyoto Women’s University, Japan
Copyright © 2020 Liu, Shen, Guo, Parnell, Westerman, Smith, Ordovas and Lai. This is an open-access article distributed under the terms of the Creative Commons Attribution License (CC BY). The use, distribution or reproduction in other forums is permitted, provided the original author(s) and the copyright owner(s) are credited and that the original publication in this journal is cited, in accordance with accepted academic practice. No use, distribution or reproduction is permitted which does not comply with these terms.
*Correspondence: Chao-Qiang Lai, Y2hhb3FpYW5nLmxhaUB1c2RhLmdvdg==