- 1Department of Genetics, Cell Biology and Anatomy, University of Nebraska Medical Center, Omaha, NE, United States
- 2Department of Pathology, University of Alabama at Birmingham, Birmingham, AL, United States
Background: Cholangiocarcinoma (CCA) is a rare disease, but it is amongst the most lethal cancers with a median survival under 1 year. Variations in DNA methylation and gene expression have been extensively studied in other cancers for their role in pathogenesis and disease prognosis, but these studies are very limited in CCA. This study focusses on the identification of DNA methylation and gene expression prognostic biomarkers using multi-omics data of CCA tumors from The Cancer Genome Atlas (TCGA).
Method: We have conducted a genome-wide analysis of differential DNA methylation and gene/miRNA expression using data from 36 CCA tumor and 9 normal samples from TCGA. The impact of DNA methylation in promoters and long-range distal enhancers on the regulation and expression of CCA-associated genes was examined using linear regression. Next, we conducted network analyses on genes which are regulated by DNA methylation as well as by miRNA. Finally, we performed Kaplan–Meier and Cox proportional hazards regression analyses in order to identify the role of selected methylation sites and specific genes and miRNAs in patient survival. We also performed real-time quantitative PCR (qPCR) to confirm the change in gene expression in CCA patients’ tumor and adjacent normal samples.
Results: Altered DNA methylation was observed on 12,259 CpGs across all chromosomes, of which 78% were hypermethylated. We observed a strong negative relationship between promoter hypermethylation and corresponding gene expression in 92% of the CpGs. Differential expression analyses revealed altered expression patterns in 3,305 genes and 101 miRNAs. Finally, we identified 17 differentially methylated promoter CpGs, 72 differentially expressed genes, and two miRNAs that are likely associated with patient survival. Pathway analysis suggested that cell division, bile secretion, amino acid metabolism, PPAR signaling, hippo signaling were highly affected by gene expression and DNA methylation alterations. The qPCR analysis further confirmed that MDK, HNF1B, PACS1, and GLUD1 are differentially expressed in CCA.
Conclusion: Based on the survival analysis, we conclude that DEPDC1, FUT4, MDK, PACS1, PIWIL4 genes, miR-22, miR-551b microRNAs, and cg27362525 and cg26597242 CpGs can strongly support their use as prognostic markers of CCA.
Introduction
Cholangiocarcinoma (CCA), commonly known as the bile duct cancer, induces tumors in epithelial cells (cholangiocytes) that line the bile ducts (Lazaridis and Gores, 2005). The bile ducts are tubes from the liver and from the gall bladder that carry a fluid called bile into the small intestine, where it is used for digesting fat. CCA tumors may originate in the ducts that are inside (intrahepatic) or outside (extrahepatic) the liver. CCA is predominantly extrahepatic with only 10% of the cases having an intrahepatic origin. About 65% of CCA cases are diagnosed in seniors over the age of 65 with equal prevalence in both males and females. CCA prevalence has been steadily rising in the United States (from 0.044 per million in 1973 to 0.118 in 2012) (Saha et al., 2016) and also in other western countries (Khan et al., 2012). Because most patients are presented with advanced CCA at the time of diagnosis, despite chemotherapy, the survival for CCA patients is under 1 year (Valle et al., 2010).
Cholangiocarcinoma presents unique challenges for therapy due to its genetic heterogeneity. An earlier report (Chaisaingmongkol et al., 2017) has identified molecular subtypes of intrahepatic and extrahepatic cholangiocarcinoma in Asian populations. A recent classification of CCA in western populations proposed four possible molecular subtypes that differ in their DNA methylation, gene expression, copy number alteration, and mutation profiles (Farshidfar et al., 2017). In both studies, the most variable genes and DNA methylation sites were used for molecular subtyping; however, the global patterns of DNA methylation and gene/miRNA expression and their association with patient survival were not considered in these studies. The focus of our study is mainly on the identification of prognostic markers that are associated with survival using the western patient population that is represented in TCGA.
We have analyzed the patterns of DNA methylation, and gene and miRNA expression in CCA and control populations using TCGA data. Detailed correlative analyses between DNA methylation and gene expression; and gene expression and miRNA expression were also performed. Pathway and gene ontology (GO) enrichment analyses of differentially methylated genes (DMGs) and differentially expressed genes (DEGs) allowed us to gain insights into how alterations in DNA methylation and gene expression affect certain biological pathways to stimulate CCA progression. CCA-specific hypermethylated and hypomethylated distal enhancer and promoter probes and the well-known transcription factor binding motifs were examined to understand the site-specific transcription factors that are expected to be involved in carcinogenesis of CCA. We also carried out a network analysis of differentially expressed genes that are affected by promoter DNA methylation and miRNA expression to explore the role of the altered regulatory network in the CCA. Finally, we carried out an extensive analysis of CCA patient survival with respect to genetic markers, including differentially expressed genes, miRNAs, and differentially methylated promoter CpG sites. We also confirmed the change in the expression of selected genes in CCA compared to adjacent normal tissues using real-time qPCR.
Materials and Methods
DNA Methylation, RNA-Seq and miRNA-Seq Data
TCGA Firehose level-3 data on DNA methylation (Illumina HumanMethylation450 BeadArray), gene expression (IlluminaHiSeq RNASeqV2), and microRNA expression (IlluminaHiSeq, miRNAseq) were downloaded using Bioconductor tool, RTCGAToolbox (Samur, 2014). The DNA methylation level-3 data includes the β values for 485,577 CpG locations with annotations for chromosomes (UCSC hg19); HUGO Gene Nomenclature Committee (HGNC) gene symbols; and CpG coordinates (UCSC hg19). These β values were calculated as (M/M + U) range from 0 to 1, where M is the frequency of methylated allele and, U is the unmethylated allele frequency; higher β values suggested elevated methylation level. The gene expression data were acquired as single RSEM (RNA-seq by Expectation-Maximization) values for 20,531 HGNC genes. The miRNA-seq data have a single expression value for 1,046 miRNAs annotated with miRBase v16.
Methylation Data Processing
To remove gender bias, β values of CpG probes that corresponded to X and Y chromosomes were discarded. We also discarded CpGs with missing β values in greater than 20% of the samples and used k-nearest neighbor-based imputation to assess the missing values with the imputeKNN module of R tool, impute data (Troyanskaya et al., 2001). Statistical analyses of DNA methylation of 45 samples (36 primary tumors and 9 normal samples) were performed at both the CpG site level and at the gene level. For distal enhancer analysis, probes that are 2 kb farther from the Transcription Start Sites (TSS) were used.
CpG probes were mapped to genes in six different sub-regions - TSS200 (the region from TSS to 200 bp upstream of TSS), TSS1500 (201–1,500 bp upstream from TSS), 5′UTR, 1st exon, gene body, and 3′UTR- and analyzed separately for each region (Supplementary Figure S1a). Similarly, DNA methylation in the CpG island regions including shore (0–2 kb from CpG islands), shelf (2–4 kb after CpG islands), and open sea regions (CpG sites anywhere in the genome without a specific designation) were also examined (Supplementary Figure S1b). We created ‘gene region collapsed data’ for dimensionality reduction of the methylation data on regions that are most appropriate for gene function. R version 3.4.2 (R Development Core Team, 2015) was used to perform the analyses. The β values for every sub-region were summarized utilizing the median, i.e., if a gene has two or more CpG sites in the same sub-region then the median of β values (by using R function ‘aggregate’) was used.
RNA-Seq and miRNA-Seq Data Processing
The level-3 RNA-seq data contain expression values for each gene obtained by normalizing the expression values for each gene in every samples. Briefly, these values were derived by mapping RNA-seq reads with MapSplice software to the human reference genome and quantifying transcripts with RSEM (Li and Dewey, 2011). TCGA level-3 has different types of RSEM output data; the expected read count data was used in this analysis. Level-3 miRNA-seq data contains raw read count for each miRBase v16 miRNA, derived from the exact mapping of miRNA-seq reads with BWA-MEM, and quantified with ShortStack (Axtell, 2013). We purged all samples and genes or miRNAs from the downstream analysis that have missing expression values (NA) for over 20% of the samples, genes or miRNAs.
Batch Effect and Unsupervised Analysis of Data
Using Mbatch (TCGA, 2010), Principal Component Analysis (PCA) was performed to identify potential batch effects among the samples for DNA methylation, gene expression, and miRNA expression data. The unsupervised clustering of epigenome and transcriptome data were performed by PCA in R using princomp function. Pearson correlation matrix, Manhattan distance matrix and heatmaps of the tumor and normal samples were generated using ggplot2 in R. Three dimensional PCA plot was generated with R packages, ggplot2, and scatterplot3d (Wickham, 2016).
Genome-Wide Analysis of Differentially Methylated CpG Probes
Methylation probes which mapped in the repeated regions of chromosomes and having a known SNP within 10 bp of interrogated CpG locations were also removed as recommended by other TCGA studies (Cancer Genome Atlas Network, 2012; Cancer Genome Atlas Research Network, 2014). Next, we normalized β values by using the Beta Mixed Integer-quantile normalization (BMIQ) (Teschendorff et al., 2013) method to correcting the bias of type II probes in Illumina HumanMethylation450K BeadChip data by utilizing the Bioconductor tool, ChAMP (Morris et al., 2014). R package, limma (Ritchie et al., 2015) was used for supervised differential methylation analysis. For a given CpG site to be considered differentially methylated, the difference between the median DNA methylation level in the primary tumor and normal samples must be at least 0.2 (Δβ ≥ 0.2) and the BH adjusted p-value ≤ 0.005. Limma was also used on summarized methylation data for differential CpG island analysis. A circular plot of differentially methylated CpGs (dm-CpGs) with differential methylation frequencies in 10 Mb sliding window for each chromosome was generated by using R tool, gtrellis (Gu et al., 2016). Then differential methylation frequency per mega base pair (Mb) was determined for each chromosome by computing the overall number of dm-CpGs for each chromosome and dividing by the length of the respective chromosome (Mb). Similarly, for each chromosome, hypermethylation and hypomethylation frequencies were also calculated. A particular chromosome considered predominantly hypermethylated if the ratio between hypermethylation to hypomethylation frequencies is ≥1.5. Similarly, if a chromosome has hypomethylation to hypermethylation frequency ratio is ≥1.5 considered as predominately hypomethylated.
Genome-Wide Analysis of Differentially Methylated Regions (DMRs)
A genomic region containing at least two dm-CpGs was considered as a differentially methylated region (DMR). A Bioconductor tool, DMRcate (TCGA, 2010) was used to analyze genome-wide DMRs. For regulatory region analysis, we mapped DMRs in super-enhancers (Pott and Lieb, 2015), Vista enhancers (Visel et al., 2007), and DNase hypersensitive sites (Consortium, 2012), which are known regulatory regions. Super-enhancers are genomic regions containing groups of transcriptionally active putative enhancers in close proximity. VISTA enhancers are individual experimentally validated human or mouse non-coding sequences with gene enhancer activity. While DNase hypersensitive regions are specific regions of the genome, where chromatin attains open structure, i.e., euchromatin, making it accessible for transcription. We mapped DMRs against these three types of regulatory regions and DMRs that show at least a 10% overlap with these regions are considered as active regulatory regions.
Differential Gene Expression Analysis
For differential gene expression analysis, 36 primary CCA and 9 normal samples expected counts’ data were used. We preprocessed the data to remove all genes that have low (≤1 count per million, CPM) or missing values in over 20% of the samples. After preprocessing, Bioconductor tools, edgeR (Robinson et al., 2010) and DESeq2 (Love et al., 2014) were used for differential gene expression analysis using an absolute logFC cutoff at 1.5 and both the raw p-value and Benjamini–Hochberg (BH) (Benjamini and Hochberg, 1995) adjusted p-value cutoffs at 0.01. For differential miRNA expression analysis, we used level-3 raw read counts data in edgeR, logFC ≥ 1, and a BH corrected p-value of 0.01.
Classification of the Tumor and Normal Samples
We used logistic regression models by applying linear models (lm) function in R to categorize tumor and normal samples using gene expression, miRNA expression, and DNA methylation data. R package ROCR (Sing et al., 2005) was used to evaluate the performance of lm for each gene, miRNA, and CpGs by using the area under the curve (AUC). R tool ROCR was used for the receiver operating characteristic (ROC) curve plot. We used only those genes, miRNAs, or CpGs that have an AUC ≥ 0.70 for further analysis.
Association Between DNA Methylation and Gene Expression
For association analysis, a total of 36 primary tumor samples that have both DNA methylation and gene expression data were examined. R package eMap (Sun, 2010) was used for the linear regression-based correlation analysis between DNA methylation and gene expression. Methylation and expression levels of genes were verified for non-zero association using Pearson’s correlation, i.e., excluding all those which have a correlation value of zero. For further analysis, probes within 100 kb from the TSS of a gene in either direction were used, and an association was taken into account as significant only if the Bonferroni corrected p-value was under 0.05. R package quantsmooth (Oosting et al., 2014) was used to visualize the genome-wide association between DNA methylation and gene expression.
The median methylation of CpGs in the ‘gene region collapsed’ regions, and gene expression of corresponding genes was assessed for non-zero correlation using Spearman correlation (R function cor.test). Correlation between DNA methylation and gene expression was regarded as noteworthy if the raw p-value and BH-corrected p-value were both below 0.05.
Enrichment Analysis
Bioconductor package clusterProfiler (Yu et al., 2012) and the desktop version of GSEA (Subramanian et al., 2005) were used for enrichment analysis of DEGs in CCA. Entrez gene ids were used with a BH multiple adjustment threshold of 0.05 with a minimum and maximum of five and 500 genes, respectively, for each pathway. KEGG, Reactome, Biocarta, and PID were utilized for the pathway enrichment analysis. Another tool, ‘camera’ in limma, was used for pathway enrichment analysis by selecting only overlapping pathways. We used ‘gometh’ module of the Bioconductor tool missMethyl (Phipson et al., 2016) for the pathway enrichment analysis of dm-CpGs. Genes associated with dmCpGs in the IlluminaHuman450K BeadChip with Δβ ≥ 0.2 were acquired from the annotation package, IlluminaHumanMethylation450kanno.ilmn12.hg19. Every GO and KEGG terms were examined using ‘gometh’, and false discovery rates (FDR) were calculated by using the BH method.
Regulatory Element Landscape and Transcription Factor Analysis
For regulatory element analysis, we have used the Bioconductor tool, Enhancer Linking by Methylation/Expression Relationship (ELMER) (Yao et al., 2015). ELMER uses ENCODE/REMC, ChromHMM, and FANTOM5 genomic regions for annotating enhancer regions. We used level-3 data of tumor specimens that had both DNA methylation and gene expression values. Using ELMER, we determined the distal enhancer (>2.0 kb away from known TSS) and promoter (within 2 Kb from known TSS) probes and correlated their DNA methylation states with the expression of genes in close proximity to identify transcriptional targets. First, we selected distal enhancer and promoter probes and used one-tailed t-test to identify hypermethylated and hypomethylated CpG probes. In the second step, Pearson’s correlation among the differentially methylated distal enhancer probe and 10 nearby up- and downward genes’ expression values were calculated to determine the putative target gene and distal enhancer probe pair. To achieve a high confidence correlation between distal enhancer probes and gene expression data, we used 10,000 permutations. For promoter analysis, we used the nearest single gene and calculated correlation to determine the relationship. Next, FIMO (find individual motif occurrences) tool (Grant et al., 2011) was used to locate the enriched TF binding motifs for differentially methylated distal enhancer or promoter probes, which are significantly correlated with a putative target gene, with a p-value cutoff of 1e–4. FIMO was used to scan for enriched TF binding motifs within ±100 bp region in the neighborhood of the individual probe utilizing position weight matrices (PWMs) of the human TF motif database, JASPAR-Core (Mathelier et al., 2014) and Factorbook (Wang et al., 2013). Finally, a list of upstream master regulator TFs corresponding to each of the enriched TF binding motifs whose expression has been associated with the DNA methylation of the TF binding motif region was determined by using ELMER. For each motif, the average DNA methylation of all distal enhancer/promoter probes within ±100 bp of a motif occurrence region was computed and correlated with the 1,982 known human TFs expression (Ravasi et al., 2010). Then, for each motif-gene pair, we made two separate groups of samples: M group (20% of the specimens with the highest average DNA methylation for a motif) and U group (20% of the specimens with the lowest average DNA methylation for a motif). We used the Wilcoxon rank-sum test to test the null hypothesis that M group had greater or equal overall gene expression than the U group, for each candidate motif-TF pair. All TFs were ranked by the -log10 (P), and the ones that falls within the upper 5% of this ranking were considered potential upstream master regulators.
Network Analysis for Genes Under Multiple Regulatory Control (GMRCs)
Molecular interaction networks (interactome) were used to understand the interaction between genomic and epigenomic loci associated with CCA. Gene expression correlated with both methylation and miRNA expression were used to build the gene network of interacting molecules using BisoGenet (Martin et al., 2010) plugin in Cytoscape (Shannon et al., 2003). The network was constructed by using the molecular interactions from various biological interaction databases that included BIND, BioGRID, DIP, HPRD, INTACT, MINT, ENCODE, and Microcoms. Topological parameters of the network were calculated using the CentiScaPe plugin in Cytoscape (Scardoni et al., 2009). Genes that are part of more than one association study, i.e., listed in more than one QTL analysis (DNA methylation, miRNA) were treated as genes under multiple regulatory controls (GMRCs). GMRCs and their first interactive neighbors were used to construct the network and perform a topological analysis using neighborhood connectivity and node stress parameters from CentiScaPe, to estimate the average rank (AR) score for each node. The AR score was decided by an average rank of the nodes with respect to both indices, i.e., node stress and neighborhood connectivity. The nodes with AR score cutoff ≤10 were considered as key regulatory hubs. The significance of pathways associated with sub-networks of hub nodes was decided by Fisher’s exact test p-value in Ingenuity Pathways Analysis (IPA) for a query gene-based search for pathway enrichment (Kramer et al., 2014).
Survival Analysis
To uncover the role of differentially expressed genes/miRNA in the survival of patients, CCA patients were segregated into two groups, namely high and low, using the median expression of genes as a cut-off value. To evaluate DNA methylation, we used 0.5 and 0.3 as β value cutoff for the high and the low groups. Only those promoter CpGs which were within 1000 bp downstream or 500 bp upstream of the TSS of the genes were used for survival analysis (Cooper et al., 2006; Whitfield et al., 2012). The R tool, survival was used for survival analysis, and the Kaplan–Meier survival curve plot was generated for all analyses. In addition, a log-rank or Mantel–Haenszel test was conducted to observe the difference between survival curves of two groups and to calculate the p-value.
Sample Procurement and Real-Time Quantitative PCR (qPCR) Analysis
Twenty-one snap-frozen cholangiocarcinoma (CCA) specimens, including seven samples with tumor-adjacent normal tissues, were collected from the University of Alabama at Birmingham (UAB) Tissue Biorepository, after obtaining approval from the Institutional Review Board. All samples were stored in the Invitrogen RNAlater-ICE stabilization solution (Thermo Fisher Scientific, Waltham, MA, United States) until processed for RNA extraction and real-time quantitative PCR (qPCR).
Total RNA was isolated from CCA and normal specimens with TRIzol reagent using the manufacturer’s instructions (Invitrogen, Carlsbad, CA, United States). Total RNA (2 μg) was reverse transcribed using high-capacity cDNA reverse transcription kits with RNase inhibitor (Applied Biosystems, Thermo Fisher Scientific, Waltham, MA, United States). The cDNA (10-ng) samples were used for validation and quantification of four genes (MDK, HNF1B, PACS1, and GLUD1) using PowerUp SYBR green master mix (Applied Biosystems, Thermo Fisher Scientific, Waltham, MA, United States) on an ABI real-time PCR machine and analyzed with Quant-studio real-time PCR software (Applied Biosystems, Thermo Fisher Scientific, Waltham, MA, United States). The Student’s t-test with two-tailed distribution was used to calculate the p-values for the statistical significance.
Results
TCGA level-3 DNA methylation gene expression and miRNA expression data downloaded by using the Bioconductor tool, RTCGAToolbox (Samur, 2014), and thoroughly carried out data cleaning, batch effect testing, global unsupervised analyses and comprehensive single and integrative analyses on DNA methylation, mRNA and miRNA expression datasets. To recognize the functional relevance and consequences of the differentially expressed genes in CCA, we also completed downstream analyses using clustering and pathway enrichment tools and correlated the results using AUC (Area Under the Curve) and Kaplan–Meyer survival plots.
Testing for Batch Effects in TCGA Data
High throughput data generation are prone to batch effects as a result of variations in the equipment and/or reagent kits utilized at different sites or the skill level of the handling personnel, and other factors. TCGA samples were prepared and processed in batches at various locations of the consortium; therefore, the data could be subject to batch effect vulnerability. However, our Mbatch analysis has not identified any batch effects in the DNA methylation (Supplementary Figure S2a), gene expression (Supplementary Figure S2b), or miRNA expression data (Supplementary Figure S2c).
Pre-filtering of Liver-Specific Gene Expression Data
A total of 386 liver-specific genes were removed from our analysis because the expression of these genes would likely result from the contamination of bile tissue with normal liver cells (Farshidfar et al., 2017). In addition, 80 other genes that showed at least five-fold elevated mRNA expression in the liver in comparison to all other tissues in The Human Protein Atlas (HPA) database (Uhlen et al., 2015) were also removed. In total, we removed the methylation and expression data of 466 liver-specific genes from further analysis.
Unsupervised Analysis of Epigenome and Transcriptome Data
The unsupervised analysis of epigenome and transcriptome data using PCA showed excellent separation amongst normal and tumor samples. The first principal component (PC1) data alone had the ability to separate normal and CCA samples, with differences of 19.4, 21.8, and 20.3%, for DNA methylation, gene expression or miRNA expression respectively (Figure 1). Similarly, Pearson’s correlation matrix- and Manhattan distance matrix-based heatmaps showed distinctive patterns in tumor and in normal samples (Supplementary Figures S3a,b), which indicates that these individual data types by themselves can provide the discriminating information between normal and CCA samples.
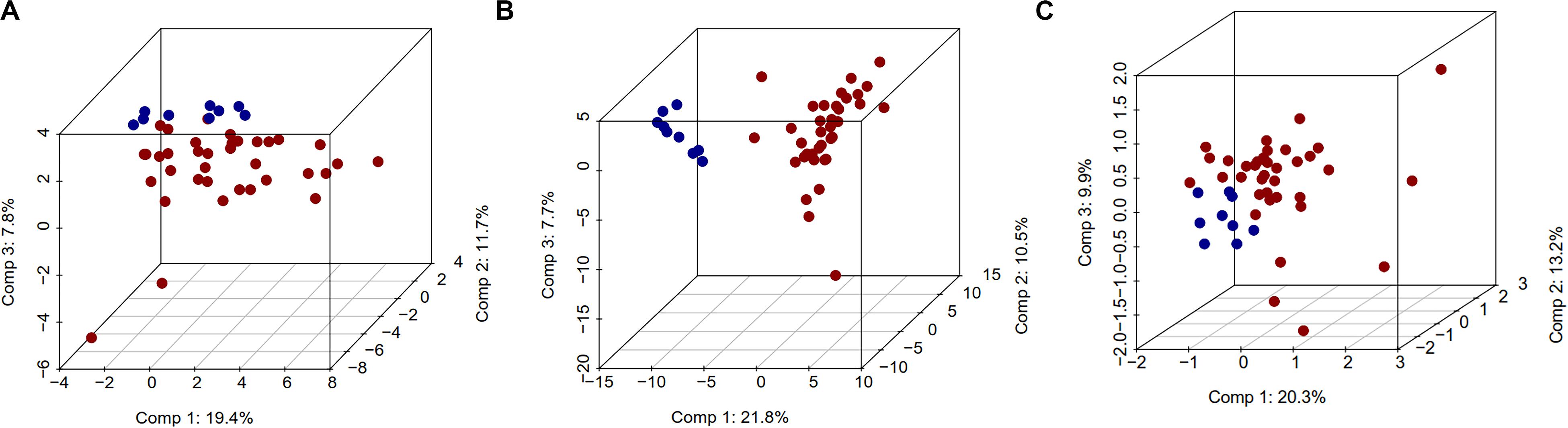
Figure 1. Three dimensional PCA plot for DNA methylation, gene expression, and miRNA expression data. In this plot, normal samples are in blue color and tumor samples in red color. For PCA analysis, we used complete DNA methylation, gene expression, and miRNA expression data. 3D PCA plots (A) DNA methylation, (B) gene expression, and (C) miRNA.
Genome-Wide Analysis of CpG Sites
To study the global DNA methylation patterns in CCA, we used the Illumina annotation file to remove all probes that are associated with liver-specific genes. A total of 12,259 differentially methylated CpGs (henceforth mentioned as dm-CpGs) were detected between tumor and normal samples; out of these 9,534 were hypermethylated and 2,725 were hypomethylated (Supplementary Figures S4a,b and Supplementary Table S1). Even at greater thresholds (Δβ ≥ 0.3), the number of dm-CpGs only drops to 9,443 showing a strong pattern of differential methylation across the genome. Figure 2A shows the global distribution of all the dm-CpGs on 22 human chromosomes. Chromosome 1 carried the maximum number of dm-CpGs, while chromosome 18 had the lowest. The two inner circles represent the density of hyper and hypomethylation in a 10 Mb sliding window throughout the genome. Distribution of dm-CpGs in ten different sub-regions of the genome is shown in Table 1 and Supplementary Figures S5a,b. List of top ten hypermethylated and hypomethylated CpG sites with corresponding gene, AUC, p-value log2 fold change and chromosome associations are available in Table 2.
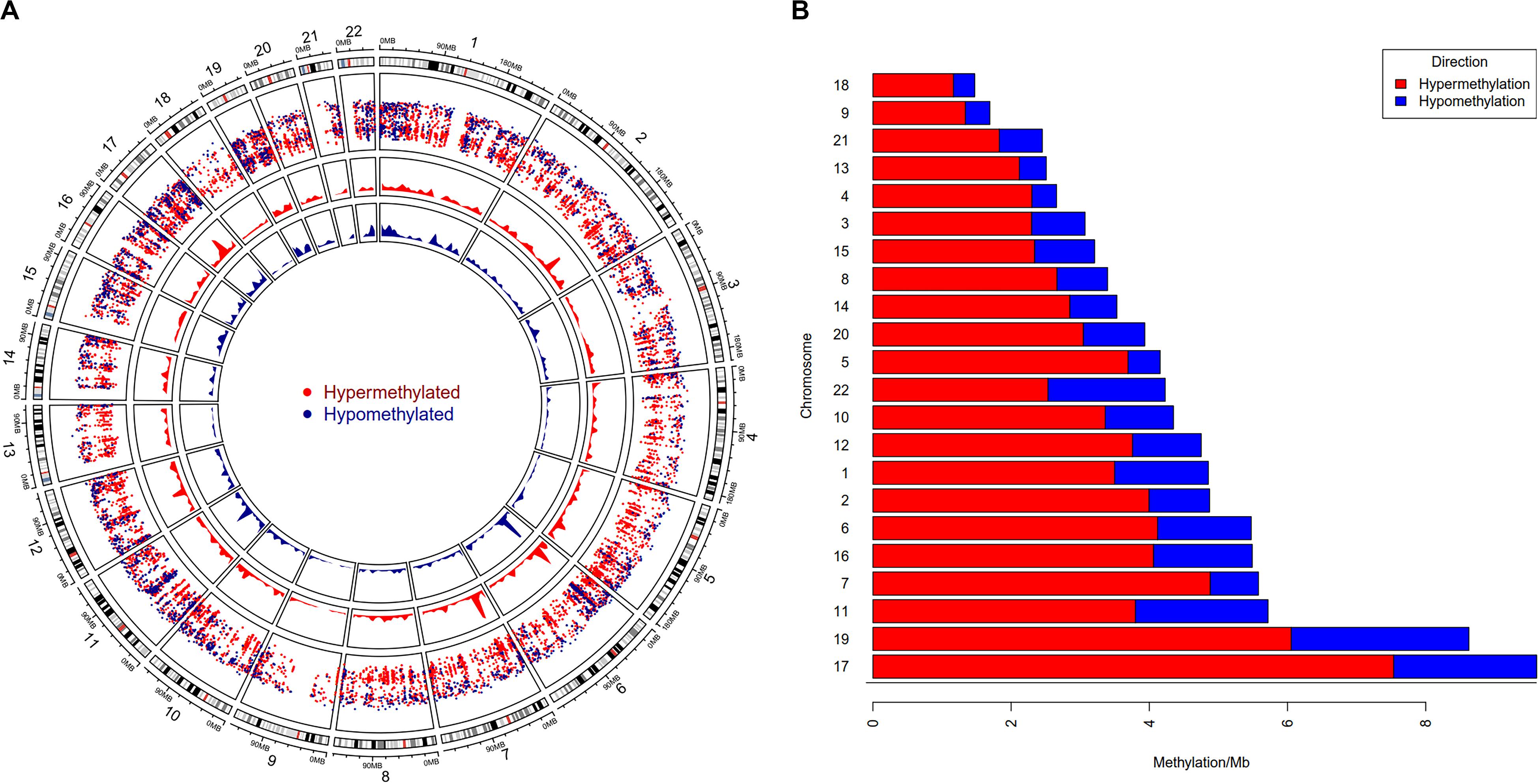
Figure 2. Genome-wide differential DNA methylation pattern in Cholangiocarcinoma. (A) Distribution of differentially methylated CpGs on each chromosome. Chromosomes are shown in a clockwise direction from 1 to 22 in the outermost circle; sex chromosomes (X or Y) were omitted from the analysis. The two innermost circles display differential hypermethylation and hypomethylation frequencies in a 10 Mb sliding window throughout the genome. The penultimate outer circle with red and blue dots correspond to hypermethylated and hypomethylated CpGs, respectively, where the distance of each dot (GpG) from the inner line represents log10 of the base-pair distance between two nearest CpGs. (B) Differential DNA methylation frequency for each chromosome (per Mb) in CCA. We assessed hypermethylation and hypomethylation frequency per Mb for each chromosome and sorted chromosomes based on their frequency.
Our results demonstrated that chromosome 17 had the highest differential methylation frequency (9.61 dm-CpGs/Mb), while chromosome 18 had the lowest (1.47 dm-CpG/Mb) (Figure 2B and Supplementary Table S1). Methylation frequency of differential CpGs were calculated within a 10 MB sliding window to identify genomic regions with high-level epigenomic perturbations. Our analysis revealed that chr7:26500001-28000000 had the highest dm-CpGs frequency, this region was mostly hypermethylated. But, there is a sub-region in this region which is hypomethylated, this sub-region contains several overexpressed genes, including HOXA3, HOXA9, HOXA10, HIABDH, LOC441204 and hsa-miR196b, with the exception of the HIABDH segment. The genomic region on chromosome 6 (30000001 – 31000000) has the highest hypomethylation frequency. This genomic region has four overexpressed genes (DDR1, NRM, RNF39, and SFTA2), and no underexpressed genes.
In the current analysis, we also performed differential methylation analysis for CpGs which are within 10Kb up- or downstream from TSS of lncRNA or within the intron/exon of lncRNA (Zhi et al., 2014). A total of 484 CpG sites (unique 435 CpGs) associated with 265 lncRNAs were differentially methylated (Supplementary Table S1); of these, 387 are hypermethylated and 97 are hypomethylated. Details of methylation in the sub-regions of lncRNAs are provided in Supplementary Table S1.
Genome-Wide Analysis of Differentially Methylated Regions (DMRs)
Methylation patterns of different sub-regions of genes may have diverse consequences on gene expression. To conduct the DMR analyses, we removed all CpGs on the sex chromosomes to remove gender bias. Only those DMRs with two or more CpGs and at least one of them with a BH-adjusted p-value < 0.01 were considered as significant. We identified a total of 6,419 DMRs across the whole genome; Chromosome 1 showed the highest (610) and Chromosome 21 showed the fewest (Hu et al., 2016) of DMRs (Supplementary Table S2). Of the 6,419, we excluded 24 short DMRs (<10 bps) from further analysis. We observed characteristic DNA methylation patterns all across the genome that distinguished the tumors from the normal samples. Examples of DMRs showing contradictory methylation patterns between normal and tumor samples on chromosome 19 (Figure 3) and chromosome 2 (Supplementary Figure S6) are displayed.
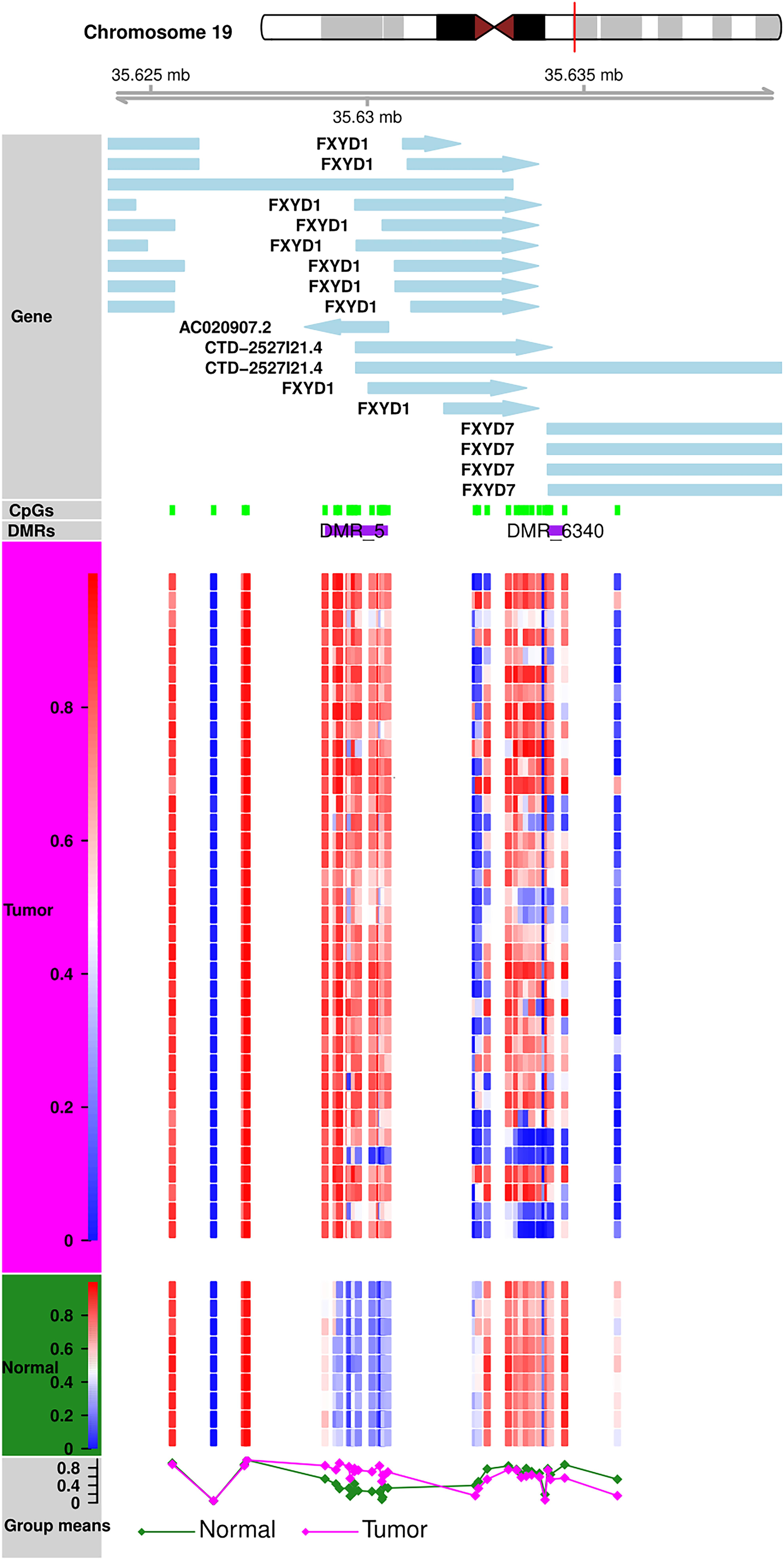
Figure 3. DMR Plot for one of the DMR. DNA methylation patterns in tumor and normal samples. Tumor and normal samples have distinct DNA methylation patterns for all CpGs in DMR.
Analysis of DMRs in the Regulatory Regions
We mapped 6,395 DMRs against 65,933 known super-enhancer regions (Khan and Zhang, 2016) and observed that about half of them (Khan et al., 2012) have at least 10% length overlap with 16,233 super-enhancers. Next, we mapped these 3,109 DMRs against 1,867,665 DNase hypersensitive site clusters (Consortium, 2012) and found that about 97% of them also overlapped with 5,432 DNase hypersensitive site clusters. Out of these, 59 DMRs also exhibited at least a 10% length overlap when mapped against 2,311 Vista enhancers (Visel et al., 2007) (Supplementary Table S2). Thus, our findings suggest that the DMRs discovered in this study have significant roles in the overall transcriptional regulation of corresponding genes in cholangiocarcinoma.
Differential Gene Expression Analysis
We observed 3,453 and 3,401 differentially expressed genes (DEGs) from DESeq2 and edgeR analyses, respectively, with 3,305 DEGs intersecting between the two sets which were forwarded for further analysis. The logistic regression analysis identified 96% of these DEGs with an ROC-AUC value of ≥0.70, which means that a normal tumor classification can be achieved by using any single gene expression value at 70% accuracy. Out of these, 2,250 and 914 DEGs were upregulated and downregulated, respectively (Supplementary Figure S7a and Supplementary Table S3).
Differential miRNA Expression Analysis
We found 101 differentially expressed miRNAs (DE-miRNAs), of which 58 were upregulated and 43 downregulated (Supplementary Figure S7b and Supplementary Table S3). Logistic regression analysis suggested that 98% of the DE-miRNAs also have an AUC of at least 0.75. Six miRNAs, i.e., miR-139, miR-187, miR-483, miR-598, miR-625, and miR-675 that were previously reported as bile duct cancer biomarkers (Wang M. et al., 2015) were also identified in our study. Apart from these, miR-21, miR-92b, miR-125a, miR-135b, miR-141, miR-196a, miR-200a, miR-200b, miR-200c, and miR-439 were upregulated, while miR-122, miR-148a, miR-152, miR-378c, miR-383, miR-483, miR-675, and miR-855 were downregulated (Supplementary Table S3). All of these miRs were earlier reported as differentially regulated in cholangiocarcinoma (Meng et al., 2006; Chen et al., 2009; Kawahigashi et al., 2009; Braconi et al., 2010; Karakatsanis et al., 2013; Wang S. et al., 2015; Zhang et al., 2015).
Clustering Analysis
Hierarchical clustering analyses were performed with hclust function in R on tumors and normal samples using dm-CpG, DEG and DE-miRNA expression values. Clustering analysis showed that tumor and normal samples have very different gene/miRNA expression and DNA methylation patterns (Figures 4A–C). All the samples were clustered along with their disease status in the clustering analysis.
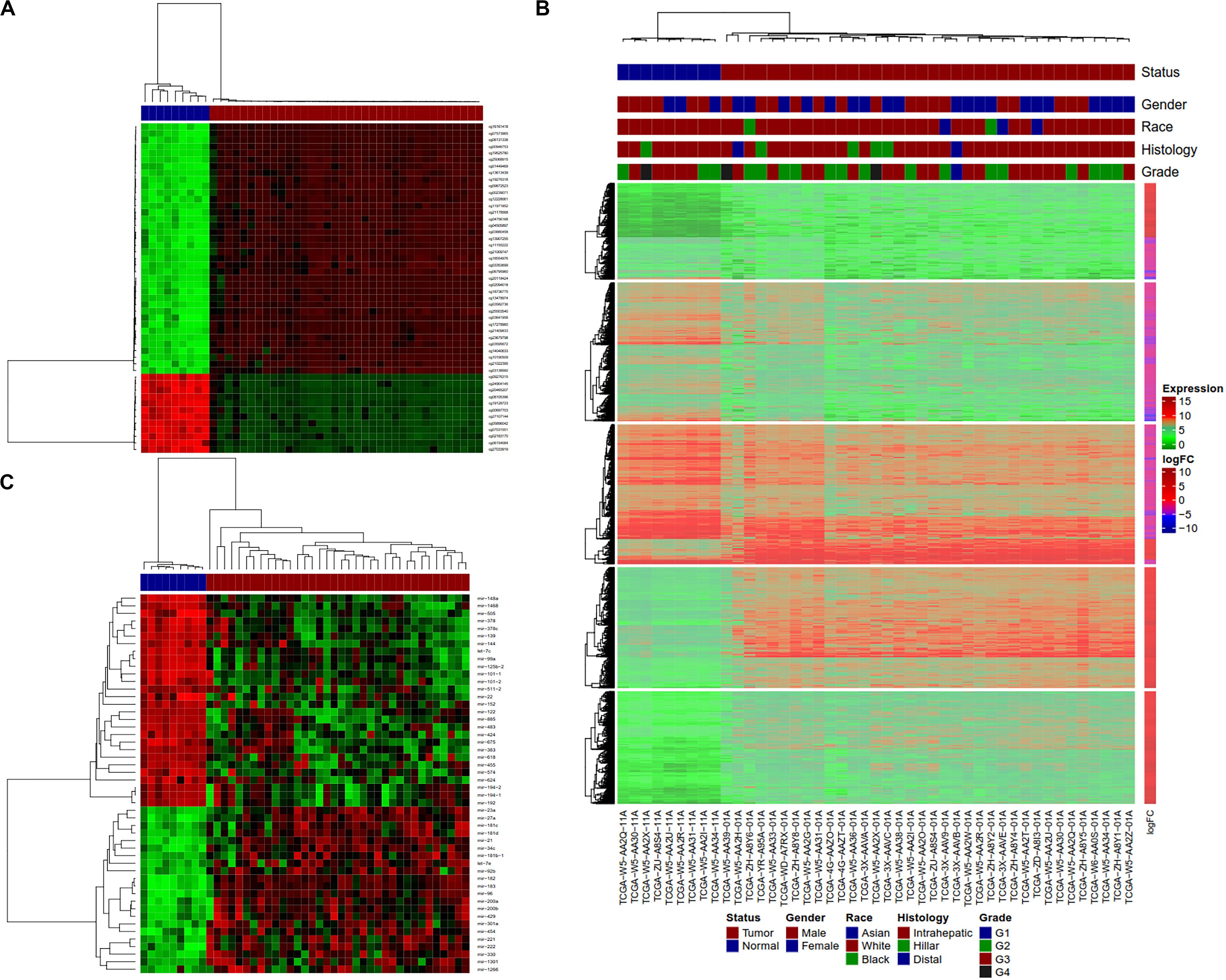
Figure 4. Clustering analysis of normal and tumor CCA samples using Dm-CpGs and DEGs. (A) Heatmap plot based on the top 50 differentially methylated CpG sites. (B) The vertical sidebar shows expression fold change in tumor Upper horizontal bars represent sample annotations of on disease status, gender, race, histology and tumor grade. (C) Heatmap plot based on the top 50 differentially expressed miRNAs.
Pathway Enrichment Analysis
Pathway enrichment analysis of differentially expressed genes was carried out using clusterProfiler (Yu et al., 2012) and GSEA (Subramanian et al., 2005). We used a weighted score (-log10 of DESeq2 FDR p-value multiplied with the sign of log2 fold change) for GSEA analysis (Davoli et al., 2017). KEGG, Biocarta, Reactome and PID pathways from MsigDB-v.5.2 (with the number of genes in the pathways ranging from 5 to 500) were used for further analyses. We identified that cell division, DNA replication, and hippo signaling pathways (Figure 5) were enriched with upregulated genes, while amino acid metabolism, glucose metabolism, drug metabolism, and autophagy pathway were enriched with downregulated genes (Supplementary Table S4 and Supplementary Figures S8a,b). Further, “camera” module in the limma tool was used for pathway enrichment analysis. DAVID and camera analyses also indicated the enrichment of nearly identical pathways as those indicated by GSEA and clusterProfiler.
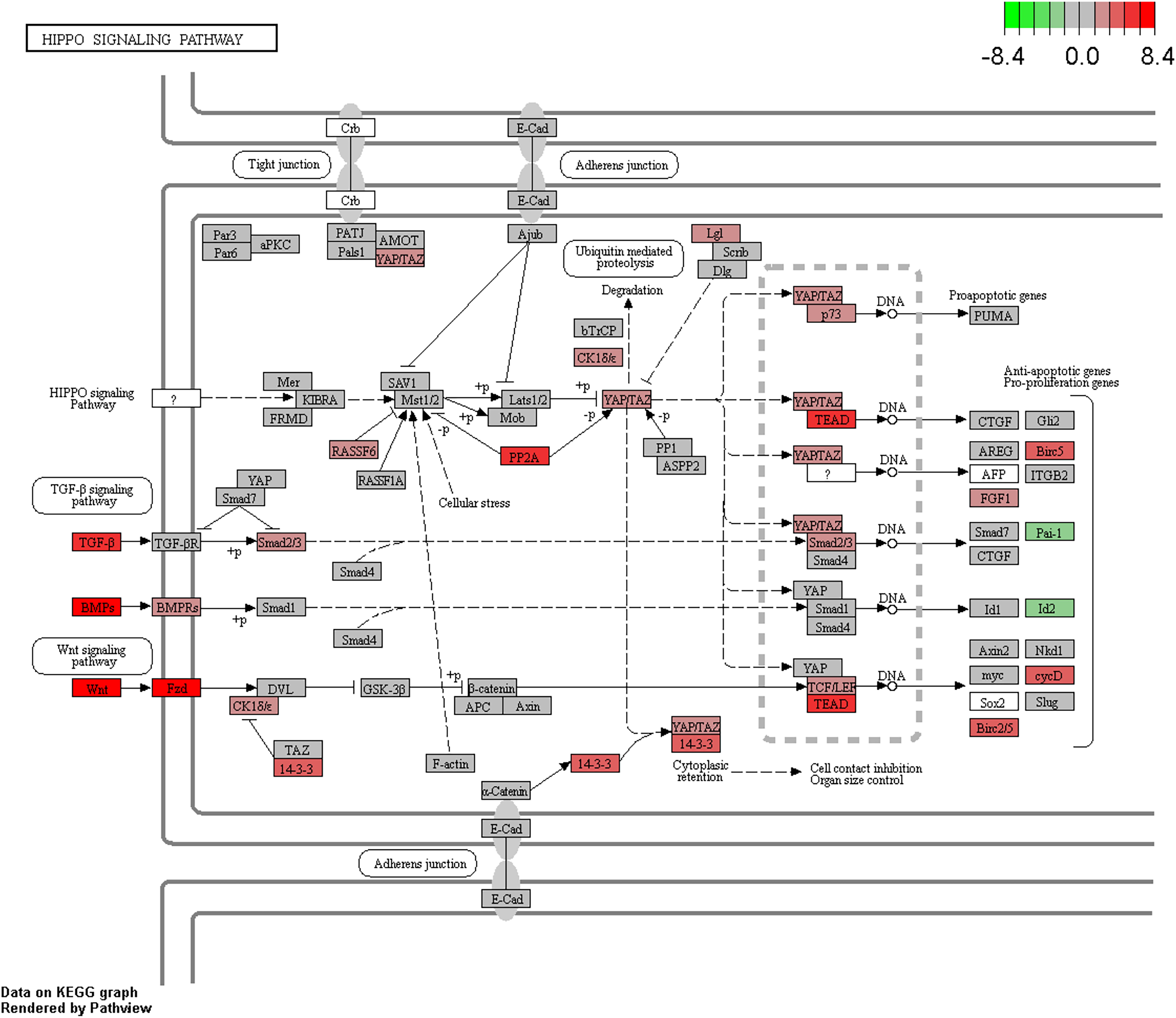
Figure 5. Enrichment of the Hippo signaling pathway in CCA. Upregulated/downregulated genes in the Hippo signaling pathway, color gradient shows the log2 fold change in tumor vs. normal analysis.
Testing for over-representation of genes that are negatively correlated between miRNA expression and gene expression using clusterProfiler showed the enrichment of pathways such as cell division, bile secretion, amino acid metabolism, PPAR signaling, fatty acid degradation, etc. Enrichment analysis of genes whose expressions were negatively correlated with miRNA expression and additionally contained differentially methylated CpGs in their promoter region also indicated the same pathways. KEGG pathway analysis of genes with dm-CpGs pointed to the enrichment of pathways related to cancer, cell division and differentiation, amino acid metabolism, degradation of xenobiotics, and immune response (Supplementary Table S4). Hippo signaling pathway is also affected by DNA methylation (FDR corrected p-value = 3.70e–18).
Correlation of DNA Methylation and Gene Expression
Pearson’s correlation was used to discover the relationship between DNA methylation and gene expression using R function, cor.test. Any correlation with a rho value of ≥0.25 and a BH adjusted p-value < 0.05 was considered significant. A significant correlation between methylated CpG sites within 1.5 kb from TSS and corresponding gene expression was observed in 5,252 CpGs, out of which 4,842 CpGs had a negative correlation and only 409 had a positive correlation (Supplementary Table S5).
Next, the correlation between locus-based DNA methylation for all dm-CpGs and the effect on gene expression within 100 kb on either side of those dm-CpGs was determined. This is to estimate the extent of multiple gene expression that is influenced by local DNA methylation in cholangiocarcinoma. Expression quantitative trait loci (eQTL) based linear regression (eMap1 function) in an R tool, eMap (Sun, 2010) was used to estimate non-zero Pearson correlation between DNA methylation and gene expression for genes that have a TSS within 100 kb of a dm-CpG. The eQTL analysis enables us to ascertain the locus of the genome (eQTL) having variation in DNA methylation that impacts the expression levels of one or more genes given that locus (within 100 kb in either direction). Finally, we observed that a total of 3,097 dm-CpGs were significantly correlated with the expression of 1,401 unique genes in cholangiocarcinoma (Supplementary Table S5).
The expression levels of 1,450 genes were positively associated with the DNA methylation levels, while 1,647 genes showed a negative correlation. Positively correlated CpG sites were quite evenly dispersed at both up and downstream of TSS (Figure 6A), while negatively correlated CpG sites were more abundant close to the TSS (2,000 bp up and downstream from TSS). The CpG sites associated with gene expression were dispersed throughout the entire genome, but chromosomes 1, 6p, 11q, 12, 16, 17, 22 are highly enriched (Supplementary Table S5).
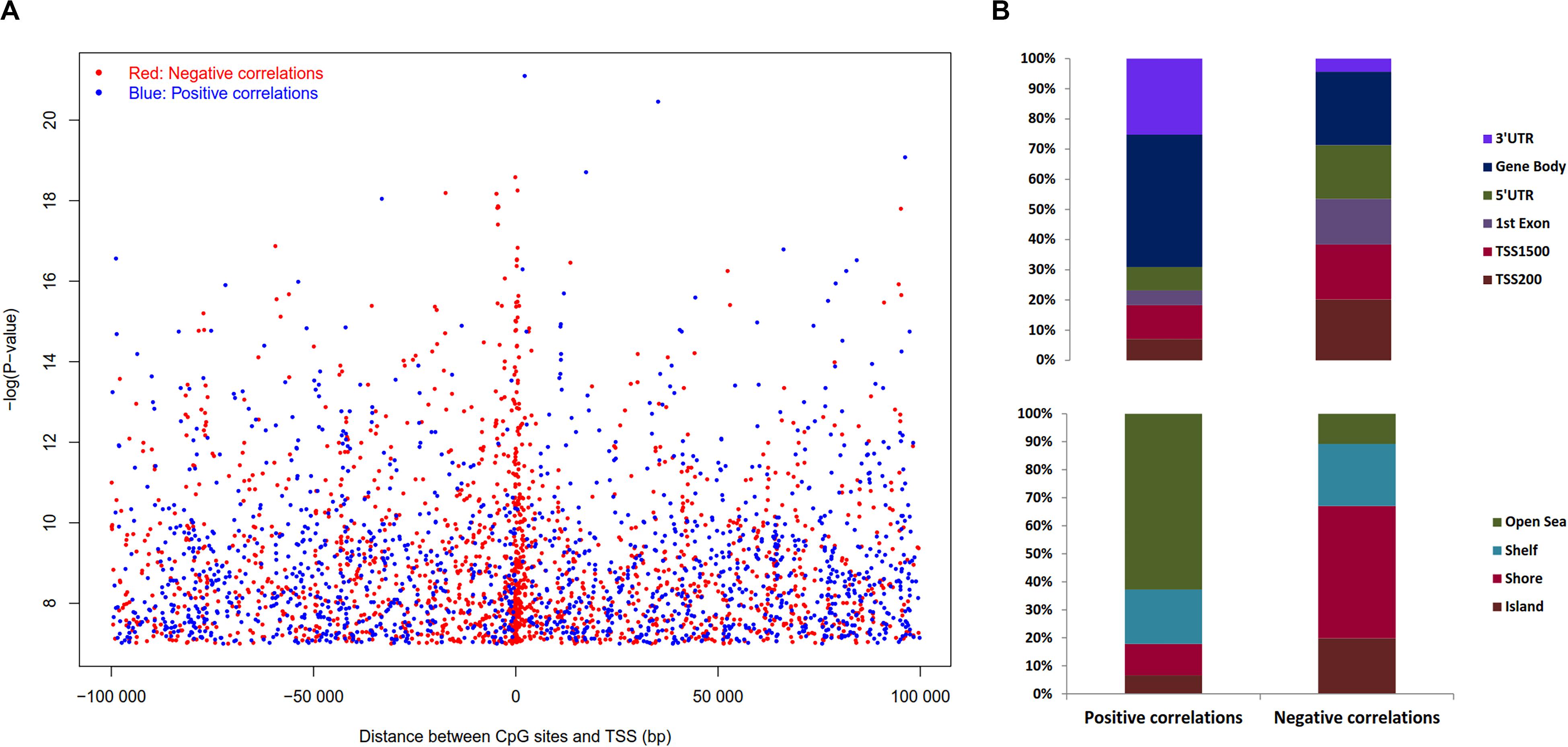
Figure 6. Distribution of the CpG sites whose DNA methylation levels were strongly associated with gene expression with BH adjusted P-value < 0.05. (A) Distribution of CpGs sites significantly correlated with corresponding gene expression. The negative logarithm of BH adjusted P-value of the correlation between DNA methylation β value and gene expression plotted against distance between CpG sites and transcription start site (TSS). Red dots denote negative correlation and blue dots denote positive correlation, sex chromosomes were excluded from the analysis. (B) Distribution of CpGs correlated with gene expression in sub-regions. Percentage stacked plot of the distribution of the negative and positive correlation corresponding to the functional regions of genes. Positive and negative correlations distribution patterns are quite different for the genomic regions.
Pearson’s correlation was assessed using R function, cor.test to estimate the association between DNA methylation in ‘gene sub-regions’ (3′ UTR, gene body, 5′ UTR, 1st exon, TSS200 and TSS1500) and the expression of corresponding genes. The expression of a total of 4,236 genes significantly correlated with DNA methylation levels in at least one subregion (Supplementary Table S5), where 3,633 genes were negatively correlated and 603 genes were positively correlated. Approximately 56% of the negative correlations were located in the upstream regulatory regions, i.e., promoter and 5′UTR, while ∼82% of the positive correlations were noticed outside of the promoter regions (TSS200 and TSS1500). In brief, upstream regulatory regions differential methylation lead to more negative correlations with gene expression as expected, and the opposite effect was observed in the case of the downstream methylation (Figure 6B). Similarly, CpG islands and its adjacent regions exhibited 89% of the negative correlations, while 63% of the positive correlations were away from islands (Figure 6B). In summary, methylation of CpG islands that are in close proximity to the TSS region was negatively associated with gene expression, while regions that are far away from islands were positively correlated. Similar trends were observed when we used Spearman’s correlations (Supplementary Figure S9).
Correlation of Micro-RNA and mRNA Expression
We used differentially expressed miRNA and mRNA for correlation analysis. Associations between miRNA-mRNA were considered significant if Pearson’s correlation was ≥| 0.8| with a BH corrected p-value cut off at 0.05, by using the Bioconductor tool MiRComb (Vila-Casadesus et al., 2016). We observed 1,200 significant miRNA-mRNA associations, which contained unique sets of 585 genes and 31 miRNAs; as expected, all of them were negatively associated (Supplementary Figure S10 plot for one correlation example). Out of these 585 genes, 146 genes were differentially methylated with Δβ ≥ 0.2 in the promoter region (within 1.5 kb from TSS). Because these genes are differentially regulated at multiple levels, we refer to these 146 genes as genes under multiple regulatory control (GMRCs).
We observed 55 GMRCs in our analysis that were both miRNA-regulated (negatively correlated with gene expression) and promoter region methylation-regulated (negatively correlated with gene expression). We hypothesize that these genes could play vital roles in cholangiocarcinoma. This is further described below by our network analysis. When we implemented the over-representation test for these genes using clusterProfiler, we observed the enrichment of bile secretion, complement and coagulation cascades and ABC transporter pathways with a BH adjusted p-value < 0.025 (Supplementary Table S5).
Network Analysis for GMRCs
Based on available interactome information, a total of 55 GMRCs were used to examine the regulatory network. The top five sub-networks were selected on the basis of the average ranking (AR) score from the network of GMRCs. To identify the molecular processes affected by these sub-networks, we performed Fisher’s exact test based overrepresentation analysis of canonical pathways using IPA software program. Among the cell cycle-related pathways, estrogen receptor (ER) signaling pathway is the most enriched. IGF-1 signaling pathway in signaling, acute phase response signaling in the inflammatory response, aryl hydrocarbon receptor signaling in development, coagulation system in immune response, and leucine degradation in amino acid metabolism stood out as the top enriched pathways (Table 3). However, enrichment of the hippo signaling pathway was observed in all the five sub-networks. The IPA canonical pathway analysis revealed that these GMRC genes were associated with critical cellular functions associated to cell cycle, growth and proliferation, cancer, amino acid metabolism, sulfonation, inflammation, and immune response (Supplementary Table S4).
Effect of Transcription Factor (TF) Binding Motif Methylation on Gene Expression
Coherence between hyper and hypomethylated CpG sites in the known TF-binding motifs was investigated to identify the master regulator transcription factors in CCA. The Bioconductor tool, ELMER was used to identify differentially methylated distal enhancers (10 kb away up or down from TSS) and promoter (within 2 kb up or down from TSS) region probes in the TF-binding motifs and their effect on gene expression in CCA. For this, we used the JASPAR and Factorbook human TF binding motif database.
Effect of Enhancer Region Methylation on Gene Expression
We used both p-value and BH adjusted p-value cutoff of 0.01 for differential methylation analysis of distal enhancer region, and observed 16,801 and 19,577 probes significantly hypermethylated and hypomethylated, respectively in CCA. In the case of the hypermethylated, 8,043 distal enhancer probes demonstrated a statistically significant correlation with gene expression (Supplementary Table S6). A total of 91 Factorbook and JASPAR TF binding sequence motifs contained a minimum of 10 hypermethylated probes each. Finally, we associated the average β value of all distal enhancer probes within ± 100 bp of an enriched motif with the 1,982 known human TF genes’ expression (Ravasi et al., 2010). Finally, we observed a total of 29 TF binding motifs whose DNA methylation is significantly associated with TF gene expression (Supplementary Table S6). All TFs that fall within the top 5% of known motif-TF pair ranking are considered potential upstream regulators of TF genes. Similarly, in the case of hypomethylation, 12,251 probe-gene pairs showed a statistically significant correlation (Supplementary Table S6) and are also enriched in 91 Factorbook and JASPAR TF binding sequence motifs with minimum 10 probes per motif. Similarly, 31 TF binding motifs having a statistically relevant association with known TF genes’ expression were observed. Our analyses suggest that SOX7/10, FOXN3, FOXM1, RXRA, RORA, STAT3, TLX1, TEAD2, MAF, and HNF4A genes are the top potential upstream master regulators of distal enhancers (Supplementary Table S6).
Effect of Promoter Region Methylation on Gene Expression
Promoter regulatory analysis showed that a total of 10,120 hypermethylated probes within 2 Kb from TSS, had statistically significant correlations with corresponding nearest gene expression. A total of 91 Factorbook and JASPAR TF binding sequence motifs were enriched with a minimum 10 significantly hypermethylated probes for each TF. We found 20 TF binding motifs whose DNA methylation has significantly associated with the corresponding TF gene’s expression (Supplementary Table S6). For hypomethylation, a total of 6,489 probe-gene pairs showed a statistically significant correlation. These probes were enriched against 91 Factorbook and JASPAR TF binding sequence motifs with at least 10 statistically significant hypomethylated probes in each TF motif region. Finally, a total of 21 TF-binding motifs showed a strong association with known TF gene expression regulators. CREB3L3, ETS2, ET4, and TEAD2 genes are the top potential upstream regulators in CCA (Supplementary Table S6).
Survival Analysis
Fisher’s exact test and univariate Cox-regression survival analysis suggest that age, race, gender, tumor stage, tumor grade, neoplasm stage, and family history of cancer have no role in the overall survival of CCA patients (Supplementary Figure S11). Our survival analysis of promoter methylation reveals that a total of 10 differentially hypermethylated and 5 hypomethylated CpGs are linked to CCA patients’ overall survival (Supplementary Table S7). In the case of gene expression, we observed that a total of 72 DEGs and two DE-miRNAs are also associated with CCA patients’ survival (Supplementary Table S7). We found that high and low expression groups have a significant difference in the overall survival with p-value < 0.05. Even at a rigorous p-value cutoff of 0.01, we observed twelve DEG, one DE-miRNA, and two hypermethylated CpG sites associated with survival, while none of the hypomethylated CpGs qualified by this criterion. Overall, analysis of the survival-associated genes, miRNA and promoter methylations resulted in the identification of potential prognostic biomarkers of CCA.
Real-Time qPCR Based Genes Expression Validation in CCA Patients
Based on our bioinformatics analysis (Supplementary Table S7), we expanded our studies to validate the gene expression of four gene targets, MDK, HNF1B, PACS1, and GLUD1. All of these genes showed expression patterns similar to those observed in our bioinformatics analyses. There were significant differences in the expression of all four genes in CCAs as compared to adjacent normal (p-value 0.0009, 0.015, 0.001, and 0.03, respectively) (Figure 10). These results suggest that the expression of these genes would be useful in evaluating the risk for CCA.
Discussion
Alterations in DNA methylation and miRNA expression are commonplace in a variety of tumors and these changes have been perceived as causative factors of oncogenesis in several cancer types (Merlo et al., 1995; Chu et al., 2016; Ramachandran et al., 2016). Such alterations significantly affect gene expression and this information has been effectively used to identify biomarkers that could discriminate the cancerous cells from normal cells (deVos et al., 2009; Oh et al., 2013; Li et al., 2014; Wang M. et al., 2015; Chu et al., 2016; Cheerla and Gevaert, 2017). In this study, we have conducted an exhaustive analysis of global DNA methylation, mRNA and miRNA gene expression, and made correlations among all data types followed by network, pathway and survival analyses. To our knowledge, this study is the first comprehensive and integrative analysis of CCA data from TCGA.
As shown in Figure 1, the DNA methylation and mRNA/miRNA expression patterns observed in the normal versus tumor samples are sufficient to discriminate the two classes in PCA analysis (Figure 1). This suggests that the classification of the tumor and normal samples using multiple molecular data profiles could help identify accurate and reliable biomarkers in CCA. The global methylation pattern of CCA suggests that dm-CpGs were dispersed across the genome. We found that Engrailed Homeobox 1 (EN1) gene had the highest number of dm-CpGs (Kramer et al., 2014), where most of them were hypermethylated in the gene body and none in the promoter region. Engrailed 1 (EN1), is exclusively overexpressed in extremely aggressive tumors (Beltran et al., 2014), we did observe overexpression of EN1 gene in our data. This is consistent with earlier reports that gene body methylation is positively associated with expression (Yang X. et al., 2014). Due to the extensive methylation of gene body CpGs, EN1 could be a key player in CCA.
Genomic sub-region that harbors HOXA3/A9/A10, HIABDH, LOC441204, and miR196b is hypomethylated, and showed overexpression of all of these genes in CCA except HIABDH (p-value > 0.05, corroborating the fact that DNA hypomethylation in general positively affects corresponding gene expression. DMRs are distributed across the genome (Supplementary Table S2), and they overlapped with known super-enhancer regions (Khan and Zhang, 2016) and ENCODE DNase hypersensitive site clusters (Consortium, 2012). Hence, these overlapping regions of DMRs (with the DNase hypersensitive, VISTA and super-enhancer regions) could serve as transcriptional hotspots in CCA.
Differential expression analysis of transcriptome data using several orthogonal tools (DESeq2, edgeR or limma) showed a very consistent list of genes. Pathway analysis suggested the enrichment of several important cancer-related pathways. Clustering analysis showed separate clusters for the tumor and normal samples (Figure 4B). Overall, our results demonstrate that CCA tissues display a unique gene expression profile compared to their adjacent normal tissues.
Previous CCA gene expression analyses suggest the overexpression of S100A6, platelet-derived growth factor-alpha (PDGFA), neutral proliferation differentiation and control protein 1 (NPDC1), while the cytochrome P450, succinate dehydrogenase (SDHA), isocitrate dehydrogenase 2 (IDH2) and glutathione S-transferase-alpha4 (GSTA4) was downregulated (Wu et al., 2011). We also observed similar expression trends for these genes (Supplementary Table S3). Similarly, genes that encode proteins associated with cell growth and metastasis, e.g., mucin 13 (MUC13), carcinoembryonic antigen-related cell adhesion molecule 5 (CEACAM5), FXDY3, epithelial cell adhesion molecule (EPCAM), transmembrane channel-like 5 (TMC5), and ets homolog factor (ETH) are overexpressed, which corroborate previous reports in CCA (Subrungruanga et al., 2013). Cell division, DNA replication related pathways, Hippo signaling pathway were highly represented with upregulated genes, while amino acid metabolism, glucose metabolism, drug metabolism, autophagy pathways were represented by downregulated genes. Similar trends were observed in CCA (Wu et al., 2011), and more specifically, on the importance of Hippo signaling (Rizvi et al., 2016) (Figure 5) and amino acid metabolic (Murakami et al., 2015) pathways in CCA were reported. Overall, our results suggest that differential gene expression impacts cell division/differentiation, amino acid metabolism and autophagy pathways in cholangiocarcinoma (Supplementary Table S4).
From our analysis of miRNA expression data, we observed a number of differentially expressed miRNAs that include six previously identified potential biomarkers of CCA (Wang M. et al., 2015. The pattern of expression of certain of these miRNAs matches with several known CCA related miRNA (Chen et al., 2009; Kawahigashi et al., 2009; Karakatsanis et al., 2013; Wang S. et al., 2015; Zhang et al., 2015). It’s been known that miR-21 and miR-200b, both oncogenes (Meng et al., 2006; Wang L. J. et al., 2015), are overexpressed in CCA, while miR-122 (Wang L. J. et al., 2015), which is a tumor suppressor is downregulated. Another tumor suppressor, miR-1258, which is recently identified as a prognostic biomarker for several other cancers (Zhang et al., 2011; Hu et al., 2016; Shi et al., 2017) is also downregulated in CCA. Classification AUC of 1 of miR-122 and miR-1258 suggest their use as potential diagnostic biomarkers for CCA. Other known miRNAs, miR-148a and miR-152, which target DNA methyltransferases 1 (DNMT1) are also downregulated in our analysis corroborating similar reports (Braconi et al., 2010). We also observed the overexpression of miR-196b in CCA and a similar trend was observed in gastric (Shao et al., 2018) and head and neck cancers (Alvarez-Teijeiro et al., 2017). Overall, the differential expression profiles of miRNAs in CCA were distinct enough to separate normal and tumor samples using clustering analysis solely based on the miRNA expression data (Figure 4C).
Correlation among gene expression and DNA methylation of CpG sites within 100 kb of TSS showed that negatively correlated CpG sites (hypermethylated CpGs which lowered gene expression) are primarily located adjacent to TSS in the upstream region, while positively correlated ones are located in the downstream region to the TSS (Figure 6A). This result substantiates the key regulatory role of promoter DNA methylation on gene expression compared to non-promoter regions. Regarding different gene sub-regions, around 56% of the negative correlations were identified in the promoter regions (i.e., TSS200, TSS1500, and 5′UTR), and around 61% of the positive correlations were found in the non-promoter regions (Figure 6B). This supports that hypermethylation at the upstream regions of the gene predominantly results in a vital alternative biological phenomenon for gene silencing. Similar observations were also found in other cancers, including pancreatic (Mishra and Guda, 2017; Mishra et al., 2019), chronic lymphocytic leukemia (Kulis et al., 2012) and breast cancers (Fleischer et al., 2014).
The previous reports also showed that overexpression of several TFs, including FOXC1, was associated with CCA carcinogenesis (Li et al., 2013). FOXC1 is also an important biomarker of cancer which plays a central role in cell proliferation, cell differentiation, cell migration, survival and death and metastasis (Ray et al., 2010; Han et al., 2017). We also observed hypomethylation of FOXC1 TF binding motif and overexpression of FOXC1 in our study, which may have a key role in CCA. TEAD2 and TEAD4 are members of the TEAD family of transcription factors, both of which target oncogene, YAP1, which is an important protein of Hippo signaling (Diepenbruck et al., 2014; Tang et al., 2018). TEAD4 is already a known biomarker for breast cancer, colorectal cancer and prostate cancer (Zhou et al., 2016); its TF binding motif regions are hypomethylated in CCA.
Pathway analysis of the GMRC genes revealed that their functions are associated with bile secretion, ABC transporters, and complement and coagulation cascades. IPA canonical pathway analysis of the top 5 subnetworks of GMRCs and their first interactive neighbor observed several pathways related to cancer such as cell cycle and cell division, immune and inflammatory response, amino acid metabolism, etc. (Supplementary Table S4). These results suggest that GMRCs play a very important role in CCA progression.
Survival analysis of promoter methylation identified ten hypermethylated and five hypomethylated CpGs that are strongly associated with the survival of CCA patients. The majority of them are either in CpG-islands or close to CpG-island (shore) (Supplementary Table S7). Survival associated hypermethylated CpGs are mapped mostly against the HOX family (HOXA9, HOXA10, and HOXB8), GABR1, and GALNT13 genes, while CpGs in CDK18, DDR1, and SFN genes were hypomethylated. GRBRA1 encodes a gamma-aminobutyric acid (GABA) receptor and promoter hypermethylation of this gene was already reported as a biomarker in colorectal cancer (Lee et al., 2012). As shown in Figure 7, hypermethylated CpG sites in the promoter and TSS regions of GABRA1 and UPS6 (Ubiquitin Specific Peptidase 6), respectively, showed very high AUC values, hence could serve as strong biomarkers of CCA. The effect of promoter methylation of the HOX family of genes in patients’ survival has not been reported previously, but promoter hypermethylation of HOXA9 in non-smokers is associated with recurrence-free survival (RFS) in non-small cell lung cancer (Hwang et al., 2015). Our analysis reveals the role of HOXA10 promoter hypermethylation in CCA patients’ survival. Recently, Shao et al. reported the critical role of HOXA10 promoter methylation in gastric cancer (Shao et al., 2018). We also observed hypermethylation of miR-196b and hypomethylation of lncRNA MIR100HG promoter CpG, which are both associated with CCA patients’ survival. It needs to be tested how promoter methylation of HOX family genes and other genes affect CCA patient survival.
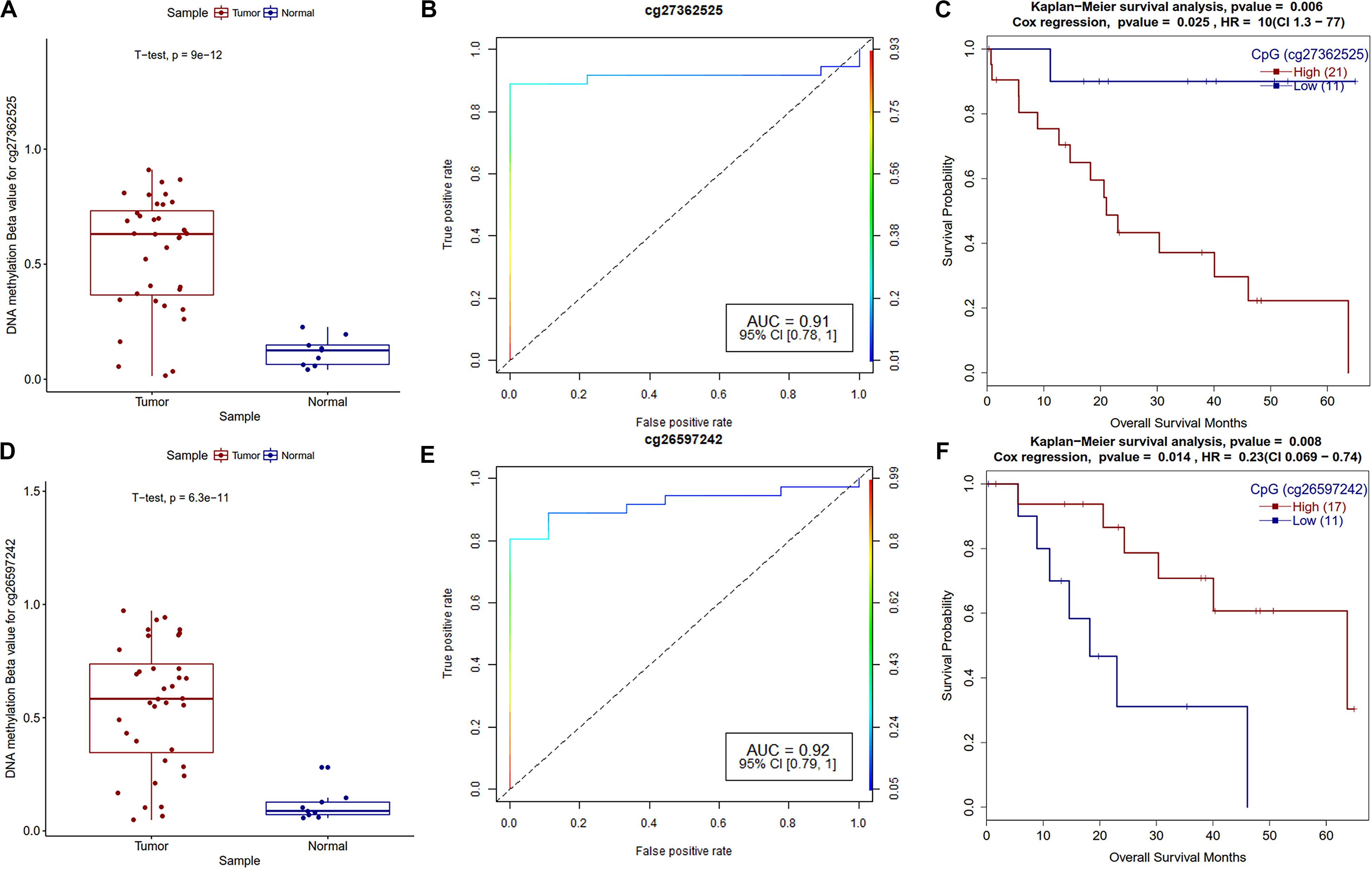
Figure 7. Survival plots of differentially methylated CpGs in CCA patients. (A) Boxplot for cg27362525 tumor and normal samples with t-test p-value. (B) ROC plot for cg27362525 for logistic regression classifier model. (C) Survival plot for high vs. low methylation group for cg27362525. (D) Boxplot for cg26597242 tumor and normal samples with t-test p-value. (E) ROC plot for cg27362525 for logistic regression classifier model. (F) Survival plot for high vs. low methylation group for cg27362525.
Further survival analysis of DEGs identified several potential biomarker genes for CCA including MLL11, MDK, DEPDC1, SLC35E4, PLXDC1, PACS1, PIWIL4, GLUD1, all have AUC ∼1 with very low p-value (Supplementary Table S6). Real-time qPCR analysis also validated the significantly altered expression of MDK, PACS1, and GLUD1 in CCA (Figure 10), which supports their potential use as CCA biomarkers. Phosphofurin acidic cluster sorting protein 1 (PACS1) is a tumor-suppressor that regulates intrinsic (mitochondrial) apoptosis with its partner ADA3. In a recent study, Brasacchio et al. (2018) reported that patients with lowered expression of PACS1 had significantly low overall survival in gastric cancer. In our analysis, we also observed low expression of PACS1 is associated with low survival in CCA. Similarly, Fucosyltransferase IV (FUT4), associated with the proliferation and metastasis, is proposed as an effective biomarker for breast cancer diagnosis (Yan et al., 2015). We also observed overexpression of FUT4 in CCA (AUC = 0.996); however, patients with lower expression showed lower overall survival (p-value 8.7e-04).
Midkine (MDK) is a heparin-binding growth factor that is overexpressed in various types of human cancers, but its clinical significance is still unknown in CCA. We also observed the overexpression of the MDK genes in the real-time qPCR analysis of CCA patients. It’s been reported that low MDK expressing patient cohorts have better survival and a similar trend was observed in our analysis (Figure 8). PIWIL4 belongs to the Argonaute family of proteins, which are involved in the development of organisms and maintenance of germline stem cells and that they are ectopically expressed in multiple forms of cancer (Hock and Meister, 2008). Wang et al. (2016) reported overexpression of PIWIL4 in breast cancer with a lowered overall patient survival. We observed a similar trend for PIWIL4 expression in CCA. DEP domain containing 1 (DEPDC1) plays a crucial role in tumor growth and metastasis (Huang et al., 2017); a previous study found that the shRNA knockdown of DEPDC1 in glioma cell lines and in mice have better survival (Kikuchi et al., 2017). Patients with high DEPDC1 expression have low survival in CCA (Figure 8).
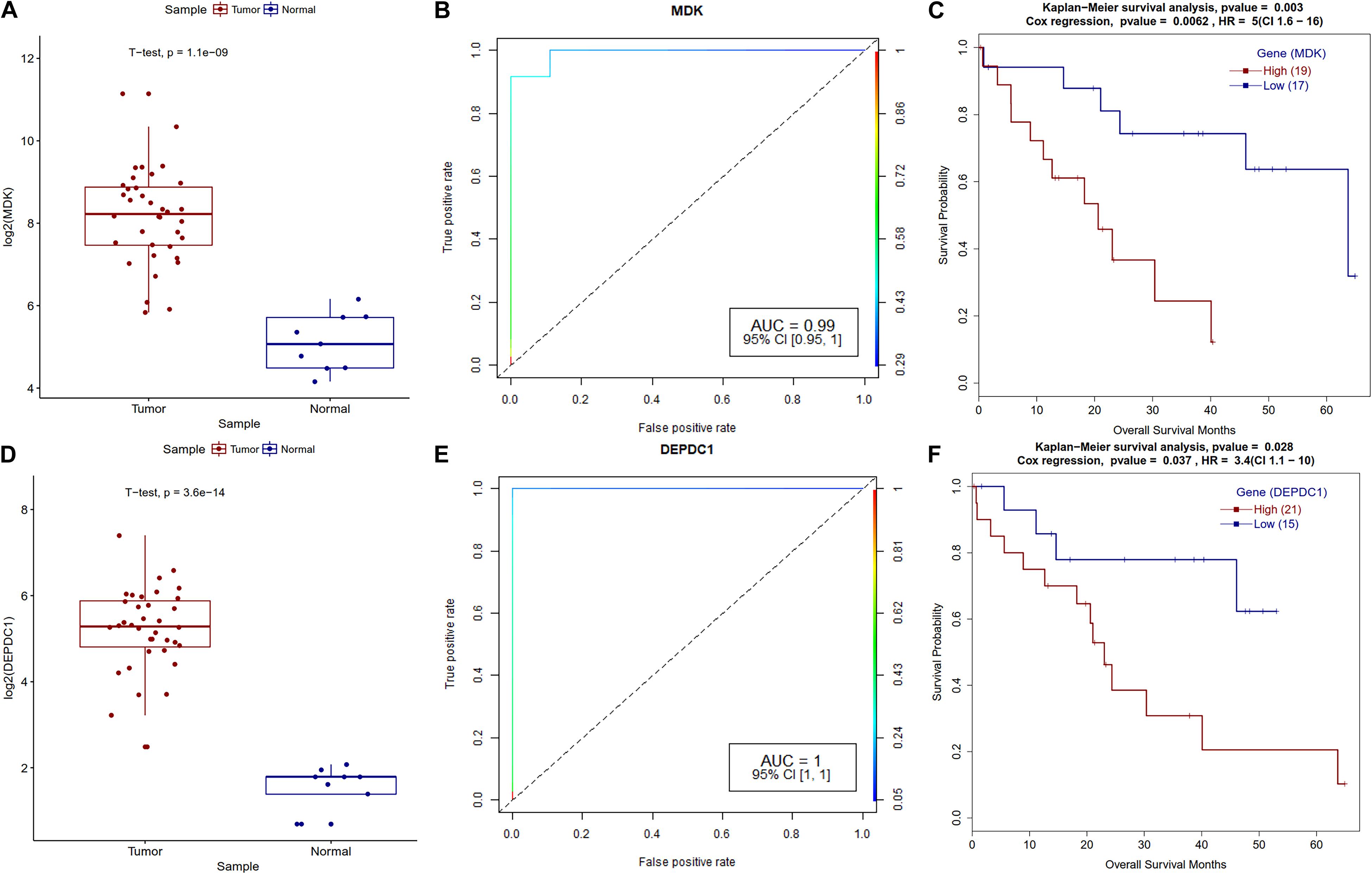
Figure 8. Survival plot for differentially expressed genes that correlate with CCA patients’ survival. (A) MDK gene expression boxplot for tumor and normal samples with t-test p-value. (B) ROC plot for MDK gene for logistic regression classifier model. (C) Kaplan–Meier plot for MDK-high and MDK-low methylation patient cohorts. (D) DEPDC1 gene expression boxplot for tumor and normal samples with t-test p-value. (E) ROC plot for the DEPDC1 gene for the logistic regression classifier model. (F) Kaplan–Meier plot for DEPDC1-high and DEPDC1-low expressing patient cohorts.
On the other hand, we observed that patients showing higher expression of ID2 have better survival in CCA, which was also observed in acute myeloid leukemia (AML) (Ghisi et al., 2016). Similarly, expression of MLLT3 and MLLT11 genes that are well studied in leukemia are also found to be strongly associated with the survival of CCA patients. Several reports from other cancers showed that patients with high GLUD1, ANXA4, and PLXDC1 expression have low overall survival, but our findings in CCA are contrasting. On similar lines, patients having high CD24 expression were shown to have low metastasis-free survival in extrahepatic CCA (Kim et al., 2013), but we observed an opposite trend in our findings (Supplementary Table S7).
MicroRNAs are known to play a vital role in cancer progression, metastasis and survival of the patients. Survival analysis of differentially expressed miRNAs revealed that miR-22 and miR-551b have a strong correlation with the survival of CCA patients. A known tumor suppressor, miR-22 (Yang C. et al., 2014) is downregulated and its high expression is correlated with poor survival of patients in CCA (Figure 9), a similar trend was observed in other types of cancers (Zuo et al., 2015; Zou et al., 2017). Regarding miR-551b, it is downregulated in most of the CCA patients, high expression is associated with better prognosis and a similar trend was observed in gastric cancer (Song et al., 2017) (Figure 9).
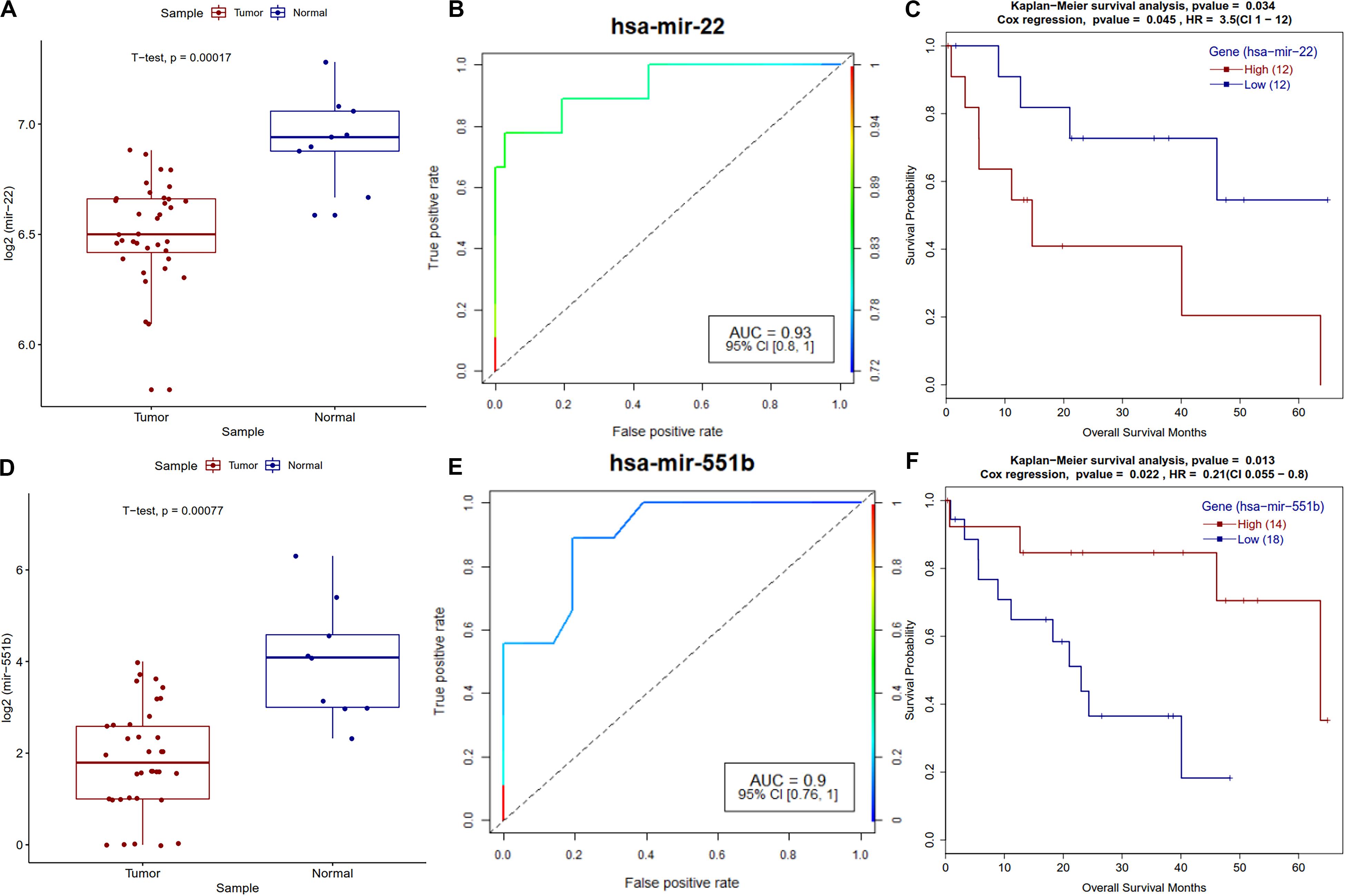
Figure 9. Survival plot of the survival associated differentially expressed miRNAs. (A) Boxplot for miR-22 expression in tumor and normal samples with t-test p-value. (B) ROC plot for miR-22 miRNA for the logistic regression classifier model. (C) Survival plot for high vs. low methylation group for miR-22 miRNA. (D) Boxplot for miR-551b expression in tumor and normal samples with t-test p-value. (E) ROC plot for miR-551b miRNA for the logistic regression classifier model. (F) Kaplan–Meier plot for miR-551b miRNA high vs. low expressing patient cohorts.
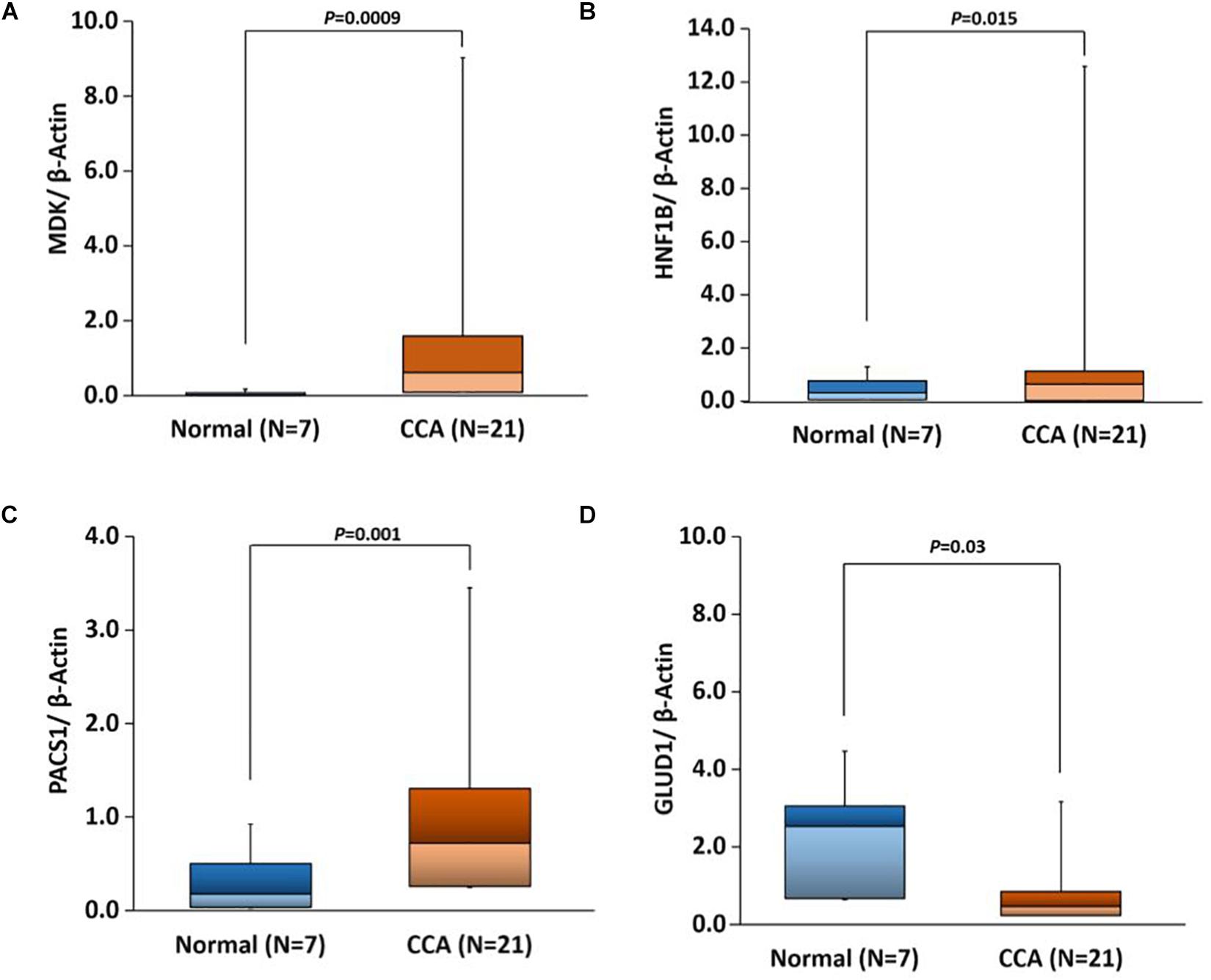
Figure 10. Box plots showing real-time quantitative PCR (qPCR) gene expression in CCA versus adjacent normal tissues with t-test p-values for the following genes. (A) MDK, (B) HNF1B, (C) PACS1, and (D) GLUD1.
Conclusion
DNA methylation analysis of cholangiocarcinoma data shows significant changes in tumor methylomes compared to normal pancreatic tissues. To our understanding, this study represents the first genome-wide DNA methylation analysis of TCGA cholangiocarcinoma data. Clustering analyses based on methylation, gene or miRNA expression data show distinct clusters of the tumor and normal samples. Differential methylation of several known CCA biomarkers was observed in our study, confirming previous observations. DNA methylation region analysis reveals that several genomic regions have very high hypermethylation/hypomethylation frequencies, and expression of genes encoded from these genomic loci correlated accordingly. Pathway enrichment analysis of differentially methylated and differentially expressed genes shows that cell division, DNA replication-related pathways, and Hippo signaling pathway are the top upregulated pathways, while amino acid metabolism, glucose metabolism, drug metabolism, and autophagy pathways are the top downregulated. Our current analysis showed that Hippo signaling pathway was affected by promoter DNA methylation, gene expression, and GMRCs. This result has further confirmed the importance of hippo signaling in cholangiocarcinoma.
Differential expression of several known marker genes and miRNAs and differential methylation of promoter regions was observed in the current analysis. Real-time qPCR analysis further confirmed the change in gene expression of several genes in CCA. Most of the survival associated promoter CpGs are in the CpG-islands or proximal to CpG-islands. Survival associated promoter CpGs are mapped against genes that are either reported as a biomarker in other cancers or otherwise associated with cancers. High AUC and low survival p-value of these CpGs suggest that they can be further explored as potential biomarkers in CCA. Survival analysis of differentially expressed genes and miRNAs also identified several possible biomarkers for CCA, and a majority of these biomarkers are already identified in another type of cancers but none for CCA. Our analysis suggests that expression of DEPDC1, FUT4, MDK, PACS1, PIWIL4, miR-22, miR-551b, and DNA methylation of cg27362525 and cg26597242 could be explored further as potential biomarkers of cholangiocarcinoma.
Data Availability Statement
Publicly available datasets were analyzed in this study. This data can be found here: https://portal.gdc.cancer.gov/projects/TCGA-CHOL.
Author Contributions
NM and CG conceived and developed the study design. NM and SS performed the statistical analysis and produced the figures. MN did the DMR enrichment analysis. UM acquired samples from the University of Alabama at Birmingham (UAB) Tissue Biorepository and directed the experimental validation work. PB and AE performed real-time qPCR experiments. NM drafted the manuscript. CG edited and improved the manuscript. All the authors read and approved the final manuscript.
Funding
This work has been supported by startup funds to CG from the University of Nebraska Medical Center and the National Institutes of Health grants (P20GM103427 and P30CA036727).
Conflict of Interest
The authors declare that the research was conducted in the absence of any commercial or financial relationships that could be construed as a potential conflict of interest.
Acknowledgments
The authors would like to express their gratitude to the Bioinformatics and Systems Biology Core at UNMC for providing the computational infrastructure, the Holland Computing Center (HCC) for offering access to supercomputers. The authors are thankful to the University of Alabama at Birmingham (UAB) Tissue Biorepository for providing tissue samples for the qPCR experiments.
Supplementary Material
The Supplementary Material for this article can be found online at: https://www.frontiersin.org/articles/10.3389/fgene.2020.522125/full#supplementary-material
Supplementary Table 1 | Information of differentially methylated CpGs. Differential methylation information for lncRNAs and differential methylation frequency for each chromosome.
Supplementary Table 2 | Information of differentially methylated regions (DMRs) in CCA. Information about DMRs overlaps with VISTA and super-enhancer and DNase hypersensitive regions.
Supplementary Table 3 | Information about differentially expressed genes and miRNAs.
Supplementary Table 4 | Results for pathway enrichment analysis for differentially expressed and differentially methylated genes. Table for the list of pathways for GMRCs and the top 5 subnetworks in GMRCs analysis.
Supplementary Table 5 | Table for the correlations based on eQTL analysis and a correlation between genes and corresponding promoter CpGs. Table for the aggregate correlation of CpG in gene sub-region. Correlation between miRNA and gene expression.
Supplementary Table 6 | Correlation between gene and corresponding hypomethylated/hypomethylated distal enhancer CpGs. Transcription factor (TF) motif analysis results for hypermethylation and hypomethylation for distal enhancers and promoters.
Supplementary Table 7 | Survival analysis table for differentially expressed genes and miRNAs and, differentially methylated promoter CpGs.
References
Alvarez-Teijeiro, S., Menendez, S. T., Villaronga, M. A., Rodrigo, J. P., Manterola, L., de Villalain, L., et al. (2017). Dysregulation of Mir-196b in head and neck cancers leads to pleiotropic effects in the tumor cells and surrounding stromal fibroblasts. Sci. Rep. 7:17785.
Axtell, M. J. (2013). ShortStack: comprehensive annotation and quantification of small RNA genes. RNA 19, 740–751. doi: 10.1261/rna.035279.112
Beltran, A. S., Graves, L. M., and Blancafort, P. (2014). Novel role of Engrailed 1 as a prosurvival transcription factor in basal-like breast cancer and engineering of interference peptides block its oncogenic function. Oncogene 33, 4767–4777. doi: 10.1038/onc.2013.422
Benjamini, Y., and Hochberg, Y. (1995). Controlling the false discovery rate: a practical and powerful approach to multiple. J. R. Stat. Soc. Ser. B 57, 289–300. doi: 10.1111/j.2517-6161.1995.tb02031.x
Braconi, C., Huang, N., and Patel, T. (2010). MicroRNA-dependent regulation of DNA methyltransferase-1 and tumor suppressor gene expression by interleukin-6 in human malignant cholangiocytes. Hepatology 51, 881–890.
Brasacchio, D., Busuttil, R. A., Noori, T., Johnstone, R. W., Boussioutas, A., and Trapani, J. A. (2018). Down-regulation of a pro-apoptotic pathway regulated by PCAF/ADA3 in early stage gastric cancer. Cell Death Dis. 9:442.
Cancer Genome Atlas Network (2012). Comprehensive molecular characterization of human colon and rectal cancer. Nature 487, 330–337. doi: 10.1038/nature11252
Cancer Genome Atlas Research Network (2014). Comprehensive molecular characterization of gastric adenocarcinoma. Nature 513, 202–209. doi: 10.1038/nature13480
Chaisaingmongkol, J., Budhu, A., Dang, H., Rabibhadana, S., Pupacdi, B., Kwon, S. M., et al. (2017). Common molecular subtypes among Asian hepatocellular carcinoma and cholangiocarcinoma. Cancer Cell 32, 57–70.e3.
Cheerla, N., and Gevaert, O. (2017). MicroRNA based pan-cancer diagnosis and treatment recommendation. BMC Bioinformatics 18:32. doi: 10.1186/s12859-016-1421-y
Chen, L., Yan, H. X., Yang, W., Hu, L., Yu, L. X., Liu, Q., et al. (2009). The role of microRNA expression pattern in human intrahepatic cholangiocarcinoma. J. Hepatol. 50, 358–369. doi: 10.1016/j.jhep.2008.09.015
Chu, A., Robertson, G., Brooks, D., Mungall, A. J., Birol, I., Coope, R., et al. (2016). Large-scale profiling of microRNAs for the cancer genome Atlas. Nucleic Acids Res. 44:e3. doi: 10.1093/nar/gkv808
Consortium, E. P. (2012). An integrated encyclopedia of DNA elements in the human genome. Nature 489, 57–74. doi: 10.1038/nature11247
Cooper, S. J., Trinklein, N. D., Anton, E. D., Nguyen, L., and Myers, R. M. (2006). Comprehensive analysis of transcriptional promoter structure and function in 1% of the human genome. Genome Res. 16, 1–10. doi: 10.1101/gr.4222606
Davoli, T., Uno, H., Wooten, E. C., and Elledge, S. J. (2017). Tumor aneuploidy correlates with markers of immune evasion and with reduced response to immunotherapy. Science 355:eaaf8399. doi: 10.1126/science.aaf8399
deVos, T., Tetzner, R., Model, F., Weiss, G., Schuster, M., Distler, J., et al. (2009). Circulating methylated SEPT9 DNA in plasma is a biomarker for colorectal cancer. Clin. Chem. 55, 1337–1346. doi: 10.1373/clinchem.2008.115808
Diepenbruck, M., Waldmeier, L., Ivanek, R., Berninger, P., Arnold, P., van Nimwegen, E., et al. (2014). Tead2 expression levels control the subcellular distribution of Yap and Taz, zyxin expression and epithelial-mesenchymal transition. J. Cell Sci. 127(Pt 7), 1523–1536. doi: 10.1242/jcs.139865
Farshidfar, F., Zheng, S., Gingras, M. C., Newton, Y., Shih, J., Robertson, A. G., et al. (2017). Integrative genomic analysis of cholangiocarcinoma identifies distinct IDH-mutant molecular profiles. Cell Rep. 18, 2780–2794.
Fleischer, T., Frigessi, A., Johnson, K. C., Edvardsen, H., Touleimat, N., Klajic, J., et al. (2014). Genome-wide DNA methylation profiles in progression to in situ and invasive carcinoma of the breast with impact on gene transcription and prognosis. Genome Biol. 15:435.
Ghisi, M., Kats, L., Masson, F., Li, J., Kratina, T., Vidacs, E., et al. (2016). Id2 and E proteins orchestrate the initiation and maintenance of MLL-rearranged acute myeloid leukemia. Cancer Cell 30, 59–74. doi: 10.1016/j.ccell.2016.05.019
Grant, C. E., Bailey, T. L., and Noble, W. S. (2011). FIMO: scanning for occurrences of a given motif. Bioinformatics 27, 1017–1018. doi: 10.1093/bioinformatics/btr064
Gu, Z., Eils, R., and Schlesner, M. (2016). gtrellis: an R/Bioconductor package for making genome-level Trellis graphics. BMC Bioinformatics 17:169. doi: 10.1186/s12859-016-1051-4
Han, B., Bhowmick, N., Qu, Y., Chung, S., Giuliano, A. E., and Cui, X. (2017). FOXC1: an emerging marker and therapeutic target for cancer. Oncogene 36, 3957–3963. doi: 10.1038/onc.2017.48
Hock, J., and Meister, G. (2008). The Argonaute protein family. Genome Biol. 9:210. doi: 10.1186/gb-2008-9-2-210
Hu, M., Wang, M., Lu, H., Wang, X., Fang, X., Wang, J., et al. (2016). Loss of miR-1258 contributes to carcinogenesis and progression of liver cancer through targeting CDC28 protein kinase regulatory subunit 1B. Oncotarget 7, 43419–43431. doi: 10.18632/oncotarget.9728
Huang, L., Chen, K., Cai, Z. P., Chen, F. C., Shen, H. Y., Zhao, W. H., et al. (2017). DEPDC1 promotes cell proliferation and tumor growth via activation of E2F signaling in prostate cancer. Biochem. Biophys. Res. Commun. 490, 707–712. doi: 10.1016/j.bbrc.2017.06.105
Hwang, J. A., Lee, B. B., Kim, Y., Hong, S. H., Kim, Y. H., Han, J., et al. (2015). HOXA9 inhibits migration of lung cancer cells and its hypermethylation is associated with recurrence in non-small cell lung cancer. Mol. Carcinog. 54(Suppl. 1), E72–E80.
Karakatsanis, A., Papaconstantinou, I., Gazouli, M., Lyberopoulou, A., Polymeneas, G., and Voros, D. (2013). Expression of microRNAs, miR-21, miR-31, miR-122, miR-145, miR-146a, miR-200c, miR-221, miR-222, and miR-223 in patients with hepatocellular carcinoma or intrahepatic cholangiocarcinoma and its prognostic significance. Mol. Carcinog. 52, 297–303. doi: 10.1002/mc.21864
Kawahigashi, Y., Mishima, T., Mizuguchi, Y., Arima, Y., Yokomuro, S., Kanda, T., et al. (2009). MicroRNA profiling of human intrahepatic cholangiocarcinoma cell lines reveals biliary epithelial cell-specific microRNAs. J. Nippon Med. Sch. 76, 188–197. doi: 10.1272/jnms.76.188
Khan, A., and Zhang, X. (2016). dbSUPER: a database of super-enhancers in mouse and human genome. Nucleic Acids Res. 44, D164–D171.
Khan, S. A., Davidson, B. R., Goldin, R. D., Heaton, N., Karani, J., Pereira, S. P., et al. (2012). Guidelines for the diagnosis and treatment of cholangiocarcinoma: an update. Gut 61, 1657–1669. doi: 10.1136/gutjnl-2011-301748
Kikuchi, R., Sampetrean, O., Saya, H., Yoshida, K., and Toda, M. (2017). Functional analysis of the DEPDC1 oncoantigen in malignant glioma and brain tumor initiating cells. J. Neurooncol. 133, 297–307. doi: 10.1007/s11060-017-2457-1
Kim, K., Min, H. S., Chie, E. K., Jang, J. Y., Kim, S. W., Han, S. W., et al. (2013). CD24 expression predicts distant metastasis in extrahepatic bile duct cancer. World J. Gastroenterol. 19, 1438–1443. doi: 10.3748/wjg.v19.i9.1438
Kramer, A., Green, J., Pollard, J. Jr., and Tugendreich, S. (2014). Causal analysis approaches in ingenuity pathway analysis. Bioinformatics 30, 523–530. doi: 10.1093/bioinformatics/btt703
Kulis, M., Heath, S., Bibikova, M., Queiros, A. C., Navarro, A., Clot, G., et al. (2012). Epigenomic analysis detects widespread gene-body DNA hypomethylation in chronic lymphocytic leukemia. Nat. Genet. 44, 1236–1242.
Lee, S., Oh, T., Chung, H., Rha, S., Kim, C., Moon, Y., et al. (2012). Identification of GABRA1 and LAMA2 as new DNA methylation markers in colorectal cancer. Int. J. Oncol. 40, 889–898.
Li, B., and Dewey, C. N. (2011). RSEM: accurate transcript quantification from RNA-Seq data with or without a reference genome. BMC Bioinformatics 12:323. doi: 10.1186/1471-2105-12-323
Li, C., Shen, W., Shen, S., and Ai, Z. (2013). Gene expression patterns combined with bioinformatics analysis identify genes associated with cholangiocarcinoma. Comput. Biol. Chem. 47, 192–197. doi: 10.1016/j.compbiolchem.2013.08.010
Li, Y. S., Xie, Q., Yang, D. Y., and Zheng, Y. (2014). Role of RASSF1A promoter methylation in the pathogenesis of hepatocellular carcinoma: a meta-analysis of 21 cohort studies. Mol. Biol. Rep. 41, 3925–3933. doi: 10.1007/s11033-014-3260-0
Love, M. I., Huber, W., and Anders, S. (2014). Moderated estimation of fold change and dispersion for RNA-seq data with DESeq2. Genome Biol. 15:550.
Martin, A., Ochagavia, M. E., Rabasa, L. C., Miranda, J., Fernandez-de-Cossio, J., and Bringas, R. (2010). BisoGenet: a new tool for gene network building, visualization and analysis. BMC Bioinformatics 11:91. doi: 10.1186/1471-2105-11-91
Mathelier, A., Zhao, X., Zhang, A. W., Parcy, F., Worsley-Hunt, R., Arenillas, D. J., et al. (2014). JASPAR 2014: an extensively expanded and updated open-access database of transcription factor binding profiles. Nucleic Acids Res. 42, D142–D147.
Meng, F., Henson, R., Lang, M., Wehbe, H., Maheshwari, S., Mendell, J. T., et al. (2006). Involvement of human micro-RNA in growth and response to chemotherapy in human cholangiocarcinoma cell lines. Gastroenterology 130, 2113–2129. doi: 10.1053/j.gastro.2006.02.057
Merlo, A., Herman, J. G., Mao, L., Lee, D. J., Gabrielson, E., Burger, P. C., et al. (1995). 5’ CpG island methylation is associated with transcriptional silencing of the tumour suppressor p16/CDKN2/MTS1 in human cancers. Nat. Med. 1, 686–692. doi: 10.1038/nm0795-686
Mishra, N. K., and Guda, C. (2017). Genome-wide DNA methylation analysis reveals molecular subtypes of pancreatic cancer. Oncotarget 8, 28990–29012. doi: 10.18632/oncotarget.15993
Mishra, N. K., Southekal, S., and Guda, C. (2019). Survival analysis of multi-omics data identifies potential prognostic markers of pancreatic ductal adenocarcinoma. Front. Genet. 10:624. doi: 10.3389/fgene.2019.00624
Morris, T. J., Butcher, L. M., Feber, A., Teschendorff, A. E., Chakravarthy, A. R., Wojdacz, T. K., et al. (2014). ChAMP: 450k chip analysis methylation pipeline. Bioinformatics 30, 428–430. doi: 10.1093/bioinformatics/btt684
Murakami, Y., Kubo, S., Tamori, A., Itami, S., Kawamura, E., Iwaisako, K., et al. (2015). Comprehensive analysis of transcriptome and metabolome analysis in intrahepatic cholangiocarcinoma and hepatocellular carcinoma. Sci. Rep. 5:16294.
Oh, T., Kim, N., Moon, Y., Kim, M. S., Hoehn, B. D., Park, C. H., et al. (2013). Genome-wide identification and validation of a novel methylation biomarker, SDC2, for blood-based detection of colorectal cancer. J. Mol. Diagn. 15, 498–507. doi: 10.1016/j.jmoldx.2013.03.004
Oosting, J., Eilers, P., and Menezes, R. (2014). quantsmooth: Quantile Smoothing and Genomic Visualization of Array Data. R package version 1.34.0.
Phipson, B., Maksimovic, J., and Oshlack, A. (2016). missMethyl: an R package for analyzing data from Illumina’s HumanMethylation450 platform. Bioinformatics 32, 286–288. doi: 10.1093/bioinformatics/btv560
Pott, S., and Lieb, J. D. (2015). What are super-enhancers? Nat. Genet. 47, 8–12. doi: 10.1038/ng.3167
R Development Core Team (2015). A Language and Environment for Statistical Computing. Viena: R Foundation for Statistical Computing.
Ramachandran, K., Speer, C., Nathanson, L., Claros, M., and Singal, R. (2016). Role of DNA methylation in cabazitaxel resistance in prostate cancer. Anticancer Res. 36, 161–168.
Ravasi, T., Suzuki, H., Cannistraci, C. V., Katayama, S., Bajic, V. B., Tan, K., et al. (2010). An atlas of combinatorial transcriptional regulation in mouse and man. Cell 140, 744–752.
Ray, P. S., Wang, J., Qu, Y., Sim, M. S., Shamonki, J., Bagaria, S. P., et al. (2010). FOXC1 is a potential prognostic biomarker with functional significance in basal-like breast cancer. Cancer Res. 70, 3870–3876. doi: 10.1158/0008-5472.can-09-4120
Ritchie, M. E., Phipson, B., Wu, D., Hu, Y., Law, C. W., Shi, W., et al. (2015). limma powers differential expression analyses for RNA-sequencing and microarray studies. Nucleic Acids Res. 43:e47. doi: 10.1093/nar/gkv007
Rizvi, S., Yamada, D., Hirsova, P., Bronk, S. F., Werneburg, N. W., Krishnan, A., et al. (2016). A hippo and fibroblast growth factor receptor autocrine pathway in cholangiocarcinoma. J. Biol. Chem. 291, 8031–8047. doi: 10.1074/jbc.m115.698472
Robinson, M. D., McCarthy, D. J., and Smyth, G. K. (2010). edgeR: a Bioconductor package for differential expression analysis of digital gene expression data. Bioinformatics 26, 139–140. doi: 10.1093/bioinformatics/btp616
Saha, S. K., Zhu, A. X., Fuchs, C. S., and Brooks, G. A. (2016). Forty-year trends in cholangiocarcinoma incidence in the U.S.: intrahepatic disease on the rise. Oncologist 21, 594–599. doi: 10.1634/theoncologist.2015-0446
Samur, M. K. (2014). RTCGAToolbox: a new tool for exporting TCGA Firehose data. PLoS One 9:e106397. doi: 10.1371/journal.pone.0106397
Scardoni, G., Petterlini, M., and Laudanna, C. (2009). Analyzing biological network parameters with CentiScaPe. Bioinformatics 25, 2857–2859. doi: 10.1093/bioinformatics/btp517
Shannon, P., Markiel, A., Ozier, O., Baliga, N. S., Wang, J. T., Ramage, D., et al. (2003). Cytoscape: a software environment for integrated models of biomolecular interaction networks. Genome Res. 13, 2498–2504. doi: 10.1101/gr.1239303
Shao, L., Chen, Z., Peng, D., Soutto, M., Zhu, S., Bates, A., et al. (2018). Methylation of the HOXA10 promoter directs miR-196b-5p-dependent cell proliferation and invasion of gastric cancer cells. Mol. Cancer Res. 16, 696–706. doi: 10.1158/1541-7786.mcr-17-0655
Shi, J., Chen, P., Sun, J., Song, Y., Ma, B., Gao, P., et al. (2017). MicroRNA-1258: an invasion and metastasis regulator that targets heparanase in gastric cancer. Oncol. Lett. 13, 3739–3745. doi: 10.3892/ol.2017.5886
Sing, T., Sander, O., Beerenwinkel, N., and Lengauer, T. (2005). ROCR: visualizing classifier performance in R. Bioinformatics 21, 3940–3941. doi: 10.1093/bioinformatics/bti623
Song, G., Zhang, H., Chen, C., Gong, L., Chen, B., Zhao, S., et al. (2017). miR-551b regulates epithelial-mesenchymal transition and metastasis of gastric cancer by inhibiting ERBB4 expression. Oncotarget 8, 45725–45735. doi: 10.18632/oncotarget.17392
Subramanian, A., Tamayo, P., Mootha, V. K., Mukherjee, S., Ebert, B. L., Gillette, M. A., et al. (2005). Gene set enrichment analysis: a knowledge-based approach for interpreting genome-wide expression profiles. Proc. Natl. Acad. Sci. U.S.A. 102, 15545–15550. doi: 10.1073/pnas.0506580102
Subrungruanga, I., Thawornkunob, C., Chawalitchewinkoon-Petmitrc, P., Pairojkul, C., Wongkham, S., and Petmitrb, S. (2013). Gene expression profiling of intrahepatic cholangiocarcinoma. Asian Pac. J. Cancer Prev. 14, 557–563. doi: 10.7314/apjcp.2013.14.1.557
Sun, W. (2010). eMap: Map Gene Expression Qtl by Linear Model. Available online at: http://www.bios.unc.edu/~weisun/software/eMap_1.2.tar.gz (accessed May 15, 2018).
Tang, J. Y., Yu, C. Y., Bao, Y. J., Chen, L., Chen, J., Yang, S. L., et al. (2018). TEAD4 promotes colorectal tumorigenesis via transcriptionally targeting YAP1. Cell Cycle 17, 102–109. doi: 10.1080/15384101.2017.1403687
TCGA (2010). TCGA Batch Effect. Available online at: http://bioinformatics.mdanderson.org/tcgambatch/ (accessed May 15, 2018).
Teschendorff, A. E., Marabita, F., Lechner, M., Bartlett, T., Tegner, J., Gomez-Cabrero, D., et al. (2013). A beta-mixture quantile normalization method for correcting probe design bias in Illumina Infinium 450 k DNA methylation data. Bioinformatics 29, 189–196. doi: 10.1093/bioinformatics/bts680
Troyanskaya, O., Cantor, M., Sherlock, G., Brown, P., Hastie, T., Tibshirani, R., et al. (2001). Missing value estimation methods for DNA microarrays. Bioinformatics 17, 520–525. doi: 10.1093/bioinformatics/17.6.520
Uhlen, M., Fagerberg, L., Hallstrom, B. M., Lindskog, C., Oksvold, P., Mardinoglu, A., et al. (2015). Proteomics. Tissue-based map of the human proteome. Science 347:1260419.
Valle, J., Wasan, H., Palmer, D. H., Cunningham, D., Anthoney, A., Maraveyas, A., et al. (2010). Cisplatin plus gemcitabine versus gemcitabine for biliary tract cancer. N. Engl. J. Med. 362, 1273–1281.
Vila-Casadesus, M., Gironella, M., and Lozano, J. J. (2016). MiRComb: an R package to analyse miRNA-mRNA interactions. Examples across five digestive cancers. PLoS One 11:e0151127. doi: 10.1371/journal.pone.0151127
Visel, A., Minovitsky, S., Dubchak, I., and Pennacchio, L. A. (2007). VISTA enhancer browser–a database of tissue-specific human enhancers. Nucleic Acids Res. 35, D88–D92.
Wang, J., Zhuang, J., Iyer, S., Lin, X. Y., Greven, M. C., Kim, B. H., et al. (2013). Factorbook.org: a wiki-based database for transcription factor-binding data generated by the ENCODE consortium. Nucleic Acids Res. 41, D171–D176.
Wang, L. J., He, C. C., Sui, X., Cai, M. J., Zhou, C. Y., Ma, J. L., et al. (2015). MiR-21 promotes intrahepatic cholangiocarcinoma proliferation and growth in vitro and in vivo by targeting PTPN14 and PTEN. Oncotarget 6, 5932–5946. doi: 10.18632/oncotarget.3465
Wang, M., Wen, T. F., He, L. H., Li, C., Zhu, W. J., and Trishul, N. M. (2015). A six-microRNA set as prognostic indicators for bile duct cancer. Int. J. Clin. Exp. Med. 8, 17261–17270.
Wang, S., Yin, J., Li, T., Yuan, L., Wang, D., He, J., et al. (2015). Upregulated circulating miR-150 is associated with the risk of intrahepatic cholangiocarcinoma. Oncol. Rep. 33, 819–825. doi: 10.3892/or.2014.3641
Wang, Z., Liu, N., Shi, S., Liu, S., and Lin, H. (2016). The role of PIWIL4, an argonaute family protein, in breast cancer. J. Biol. Chem. 291, 10646–10658. doi: 10.1074/jbc.m116.723239
Whitfield, T. W., Wang, J., Collins, P. J., Partridge, E. C., Aldred, S. F., Trinklein, N. D., et al. (2012). Functional analysis of transcription factor binding sites in human promoters. Genome Biol. 13:R50.
Wu, Z., Boonmars, T., Boonjaraspinyo, S., Nagano, I., Pinlaor, S., Puapairoj, A., et al. (2011). Candidate genes involving in tumorigenesis of cholangiocarcinoma induced by Opisthorchis viverrini infection. Parasitol. Res. 109, 657–673. doi: 10.1007/s00436-011-2298-3
Yan, X., Lin, Y., Liu, S., Aziz, F., and Yan, Q. (2015). Fucosyltransferase IV (FUT4) as an effective biomarker for the diagnosis of breast cancer. Biomed. Pharmacother. 70, 299–304. doi: 10.1016/j.biopha.2014.12.048
Yang, C., Ning, S., Li, Z., Qin, X., and Xu, W. (2014). miR-22 is down-regulated in esophageal squamous cell carcinoma and inhibits cell migration and invasion. Cancer Cell Int. 14:138.
Yang, X., Han, H., De Carvalho, D. D., Lay, F. D., Jones, P. A., and Liang, G. (2014). Gene body methylation can alter gene expression and is a therapeutic target in cancer. Cancer Cell 26, 577–590. doi: 10.1016/j.ccr.2014.07.028
Yao, L., Shen, H., Laird, P. W., Farnham, P. J., and Berman, B. P. (2015). Inferring regulatory element landscapes and transcription factor networks from cancer methylomes. Genome Biol. 16:105.
Yu, G., Wang, L. G., Han, Y., and He, Q. Y. (2012). clusterProfiler: an R package for comparing biological themes among gene clusters. OMICS 16, 284–287. doi: 10.1089/omi.2011.0118
Zhang, L., Sullivan, P. S., Goodman, J. C., Gunaratne, P. H., and Marchetti, D. (2011). MicroRNA-1258 suppresses breast cancer brain metastasis by targeting heparanase. Cancer Res. 71, 645–654. doi: 10.1158/0008-5472.can-10-1910
Zhang, M. Y., Li, S. H., Huang, G. L., Lin, G. H., Shuang, Z. Y., Lao, X. M., et al. (2015). Identification of a novel microRNA signature associated with intrahepatic cholangiocarcinoma (ICC) patient prognosis. BMC Cancer 15:64. doi: 10.1186/s12885-015-1067-6
Zhi, H., Ning, S., Li, X., Li, Y., Wu, W., and Li, X. (2014). A novel reannotation strategy for dissecting DNA methylation patterns of human long intergenic non-coding RNAs in cancers. Nucleic Acids Res. 42, 8258–8270. doi: 10.1093/nar/gku575
Zhou, Y., Huang, T., Cheng, A. S., Yu, J., Kang, W., and To, K. F. (2016). The TEAD family and its oncogenic role in promoting tumorigenesis. Int. J. Mol. Sci. 17:138. doi: 10.3390/ijms17010138
Zou, Q., Tang, Q., Pan, Y., Wang, X., Dong, X., Liang, Z., et al. (2017). MicroRNA-22 inhibits cell growth and metastasis in breast cancer via targeting of SIRT1. Exp. Ther. Med. 14, 1009–1016. doi: 10.3892/etm.2017.4590
Keywords: cholangiocarcinoma, integrative analysis, differential expression, differential methylation, prognostic biomarker, TCGA, logistic regression
Citation: Mishra NK, Niu M, Southekal S, Bajpai P, Elkholy A, Manne U and Guda C (2020) Identification of Prognostic Markers in Cholangiocarcinoma Using Altered DNA Methylation and Gene Expression Profiles. Front. Genet. 11:522125. doi: 10.3389/fgene.2020.522125
Received: 28 December 2019; Accepted: 21 August 2020;
Published: 20 October 2020.
Edited by:
Yusuf Akhter, Babasaheb Bhimrao Ambedkar University, IndiaReviewed by:
Martin Mueller, University Hospital of Bern, SwitzerlandIndranil Chattopadhyay, Central University of Tamil Nadu, India
Copyright © 2020 Mishra, Niu, Southekal, Bajpai, Elkholy, Manne and Guda. This is an open-access article distributed under the terms of the Creative Commons Attribution License (CC BY). The use, distribution or reproduction in other forums is permitted, provided the original author(s) and the copyright owner(s) are credited and that the original publication in this journal is cited, in accordance with accepted academic practice. No use, distribution or reproduction is permitted which does not comply with these terms.
*Correspondence: Nitish Kumar Mishra, bml0aXNoLm1pc2hyYUB1bm1jLmVkdQ==; Chittibabu Guda, YmFidS5ndWRhQHVubWMuZWR1