- 1Department of Gastrointestinal Colorectal and Anal Surgery, China-Japan Union Hospital of Jilin University, Changchun, China
- 2Department of Vascular Surgery, China-Japan Union Hospital of Jilin University, Changchun, China
- 3Department of Endoscopy Center, China-Japan Union Hospital of Jilin University, Changchun, China
Colon cancer is the most commonly diagnosed malignancy and the leading cause of cancer deaths worldwide. As well as lifestyle, genetic and epigenetic changes are key factors that influence the risk of colon cancer. However, the impact of epigenetic alterations in non-coding RNAs and their consequences in colon cancer have not been fully characterized. We detected differential methylation sites (DMSs) in long non-coding RNA (lncRNA) promoters and identified lncRNA expression quantitative trait methylations (lncQTMs) by association tests. To investigate how transcription factor (TF) binding was affected by DNA methylation, we characterized the occurrence of known TFs among DMSs collected from the MEME suite. We further combined methylome and transcriptome data to construct TF–methylation–lncRNA relationships. To study the role of lncRNAs in drug response, we used pharmacological and lncRNA profiles from the Cancer Cell Line Encyclopedia (CCLE) and investigated the association between lncRNAs and drug activity. We also used combinations of TF–methylation–lncRNA relationships to stratify patient survival using a risk model. DNA methylation sites displayed global hyper-methylation in lncRNA promoters and tended to have negative relationships with the corresponding lncRNAs. Negative lncQTMs located near transcription start sites (TSSs) had more significant correlations with the corresponding lncRNAs. Some lncRNAs found to be mediated by the interplay between DNA methylation and TFs were previously identified as markers for colon cancer. We also found that the ELF1-cg05372727- LINC00460 relationship were prognostic signatures for colon cancer. These findings suggest that lncRNAs mediated by the interplay between DNA methylation and TFs are promising predictors of drug response, and that combined TF–methylation–lncRNA can serve as a prognostic signature for colon cancer.
Introduction
Colon cancer is the most commonly diagnosed malignancy and leading cause of cancer deaths worldwide (Arnold et al., 2017). The development of colon cancer is related to lifestyle and exposure to environmental risks (Kuipers et al., 2015). An accumulation of genetic and epigenetic alterations can also facilitate the development of colon cancer from normal mucosa (Hong, 2018). Early screening of colonic lesions is beneficial for colon cancer diagnosis and can reduce mortality (Suehiro et al., 2008). DNA methylation, a stable epigenetic mark, has been shown to influence a wide range of biological mechanisms (Jin and Liu, 2018). Studies have emphasized the important role of DNA methylation in the early detection of colon cancer (Ashktorab et al., 2013, 2014). However, DNA methylation in the non-coding genome and its downstream effect on colon cancer have not been fully characterized.
Human genomes are pervasively transcribed into non-coding RNAs (Yan et al., 2015). Long non-coding RNAs (lncRNAs) are capped transcripts longer than 200 nucleotides (Derrien et al., 2012). The study of lncRNAs has broadened our understanding of many biological and disease processes (Dhanoa et al., 2018; Yarani et al., 2018); for instance, lncRNAs can influence cancer progression in multiple ways, including having effects on drug sensitivity, patient prognosis, and cancer cell inhibition (Ning et al., 2017; Zhang et al., 2017; Bermudez et al., 2019). Some previous studies found that lncRNAs could be regulated by epigenetic alterations, with an impact on cancer development (Wang et al., 2018c). Therefore, systematical identification of DNA methylations on lncRNAs and their consequences in colon cancer will be helpful for cancer prevention and treatment.
TFs are cell fate controllers that are frequently involved in many diseases, including cancers (Lambert et al., 2018). A single TF can bind to thousands of sites throughout the genome, typically by recognizing DNA sequences, to guide gene transcription activity (Spitz and Furlong, 2012). Recent studies revealed that TF binding can be affected by alterations in cytosine methylation patterns (Maurano et al., 2015). For instance, DNA methylation at CpG islands of gene promoters blocks TF binding and silences gene expression (Bird, 2002). Methylation-sensitive TFs could be involved in the DNA methylation mediated transcription process in human cancers (Wang et al., 2020). DNA methylation could affect TF-target transcriptional regulation circuits across various cancer types (Liu et al., 2019). There are also cytosine methylations that can promote TF binding (Yin et al., 2017). Based on this, we studied the interactions between DNA methylation and TFs and their role in lncRNA regulation in colon cancer.
We systematically analyzed DNA methylations in lncRNA promoters and identified lncRNAs that were mediated by the interplay between DNA methylation and TFs. The lncRNAs identified in our study were associated with drug response in colon cancer. One TF–methylation–lncRNA could be used to stratify patient prognosis in colon cancer. We hope our results will provide guidance for clinical research on colon cancer in the future.
Materials and Methods
Methylation and Expression Datasets
We downloaded methylation profiles from the Infinium HumanMethylation450 BeadChip and expression profiles based on RNA sequencing in colon cancer (COAD, colon adenocarcinoma) from the UCSC Xena archive (v1.01, “Methylation450k” and “gene expression RNAseq” in the TCGA Colon Cancer cohort). Expression values were log2 transformed in UCSC Xena. In order to further explore the relationship between DNA methylation and lncRNA expression, we only kept colon samples that appeared in both the DNA methylation and the expression datasets. The resulting 306 colon cancer and 19 adjacent mucosa tissue samples were used for downstream analysis.
Construction of Methylation Profiles in lncRNAs
We omitted methylation probes that had single-nucleotide polymorphisms near the probe sequence or that were located in sex chromosomes as annotated in the Illumina sequencing platform. We obtained lncRNA annotation files from the GENCODE website (v22, GRCh38) (Frankish et al., 2019), including long intergenic non-coding RNA, antisense, process transcript, sense intronic, 3’ overlapping non-coding RNA, sense overlapping, non-coding, and macro lncRNAs. The genomic coordinates of probes were transformed from GRCh37 to GRCh38 using the UCSC liftOver tool with the default parameters (v1.0) (Zhu et al., 2009) and further mapped into lncRNA promoters [2 kb upstream to 2 kb downstream of the corresponding transcription start sites (TSSs)] to give the methylation profile of lncRNA promoters.
Identification of DMSs in lncRNAs
Methylation probes that had missing values in more than 80% of samples were excluded from the analysis; for other probes, missing values were imputed using the impute package in the R software with the default parameters. We used Wilcoxon rank-sum test (two-tailed) and differences in mean values to analyze the differences in methylation between colon cancer and corresponding mucosa tissue samples; p-values were corrected using the false discovery rate (FDR) method, probes with FDR < 0.05, and absolute methylation difference (mean methylation level in colon cancer samples—mean methylation level in normal samples) > 0.3 were regarded as DMSs. A circos plot of these sites was constructed using the circlize R package (Gu et al., 2014).
Identification of lncQTMs
The associations between these DMSs and the corresponding lncRNAs were determined by Spearman’s rank correlation test (FDR < 0.05) (Gutierrez-Arcelus et al., 2013; Bonder et al., 2017; Husquin et al., 2018). Methylation sites in the correlated methylation–lncRNA pairs were considered to be potential epigenetic regulators of the lncRNAs; we called these methylation–lncRNA pairs lncQTMs.
Identification of TF Binding Motifs Around lncQTMs
To explore the interactions between DNA methylation and TFs, we collected comprehensive human TF motif position weight matrices from the MEME suite (v5.1.1) (Bailey et al., 2015) and scanned motif occurrence in the regions ± 100 bp of methylation sites in lncQTMs using the FIMO software (v5.0.3, –verbosity 2 –thresh 0.0001) (Grant et al., 2011). Among the significant motifs (p < 0.0001), we filtered out those that did not cover the methylation sites. These motifs were expected to have more enrichment of lncQTMs than background methylation sites (all methylation sites within lncRNA promoters). For each motif, we computed the odds ratio (OR); motifs equal to or greater than 1.1 were retained for further study (Yao et al., 2015).
Establishing the TF–Methylation–lncRNA Network
For the selected motifs, we used association tests to identify significantly correlated TF–methylation pairs (Spearman’s rank correlation test, FDR < 0.05). As TFs can regulate lncRNA expression by binding to methylation sites, we also tested the relationships between TFs and their target lncRNAs (Spearman’s rank correlation test) and retained correlated TF–lncRNA pairs (FDR < 0.05). Based on these results, we constructed a TF–methylation–lncRNA relationship network for colon cancer.
Functional Annotation of lncRNAs Regulated by TFs and DNA Methylation
We collected RNA-RNA interaction data from RNAInter database (v1.0) (Lin et al., 2020). Next, we mapped lncRNAs and mRNA into RNA-RNA interaction data and obtained lncRNA-mRNA interactions. We tested expression correlations of lncRNA-mRNA interactions and identified significantly correlated lncRNA-mRNA pairs to form a network (Spearman’s rank correlation test, FDR < 0.05). After that, mRNAs in the network were used for functional annotation using the Enrichr (v2.0) web server (Kuleshov et al., 2016).
Collection of Drug Response Data in Colon Cancer
The CCLE database provides comprehensive pharmacological profiles across hundreds of cell lines (Barretina et al., 2012). It also includes RNA sequencing data for cell lines, enabling quantification of lncRNAs. We downloaded drug response data from CCLE (v1.0), selecting 15 colon cancer cell lines (COAD) that were relevant to the current study. We used the activity area to evaluate drug sensitivity and kept lncRNAs that were expressed in at least 50% of cell lines.
Exploring the Association Between Drug Response and lncRNA Expression
Spearman’s rank test was used to test the correlation between lncRNA expression and drug activity area. We divided cell lines into two groups based on median expression of the lncRNA of interest, and determined the differences in activity area between the two groups (Wilcoxon rank-sum test, two-tailed). The drug activity area was normalized using the z-score method. Cell lines with an activity area at least 0.8 standard deviations (SDs) above the mean were regarded as sensitive, and those with an activity area at least 0.8 SDs below the mean were regarded as resistant; intermediate cell lines were eliminated (Dong et al., 2015). We also determined the lncRNA expression difference between sensitive and resistant groups. Considering the small size of samples used in the drug response (n = 15), p-values were not corrected using the FDR method (Albers, 2019).
Survival Analysis Based on TF–Methylation–lncRNA Relationship
We downloaded matched TCGA overall survival (OS) survival records from the TCGAbiolinks R package (Colaprico et al., 2016). In total, there were 292 patients that have the matched OS information in this study. We randomly divided the 292 patients into training (n = 146) and testing set (n = 146) to test the efficiency of TF–methylation lncRNA relationships in survival prediction. Firstly, in training set, we determine the associations between TFs, methylation sites, lncRNAs and patient OS by conducting univariate Cox regression model, respectively. Meanwhile, for each TF–methylation–lncRNA relationship, we applied a multivariate Cox proportional regression model to determine the associations between TF–methylation–lncRNA and patient OS in training set. Next, we designed risk score models that related with TFs, DNA methylation sites, lncRNAs and TF–methylation–lncRNA based on the coefficient from the Cox regression model, in which each colon cancer sample was assigned a risk score as follows:
TFs associated model: risk scorei = βtfTFi
DNA methylation sites associated model: risk scorei = βmethMethi
LncRNAs associated model: risk scorei = βlnclnci
TF–methylation–lncRNA associated model: risk scorei = βtfTFi + βmethMethi + βlnclnci
where i represents colon cancer sample i, βtf is the coefficient of the TF, βmeth is the coefficient of the methylation site, βlnc is the coefficient of the lncRNA, and TFi, Methi and lnci are the corresponding values for the TF, methylation site, and lncRNA, respectively, in colon cancer sample i.
According to the patient risk scores, we categorized colon cancer samples into two risk groups using the median risk score and applied Kaplan–Meier survival analysis (Goel et al., 2010). Differences in OS between the two groups were determined by log-rank test.
Disease free survival (DFS) records were collected from cBioPortal (v3.4.2) (Cerami et al., 2012; Gao et al., 2013); 255 patients had matched DFS records in our study. We applied the same risk model and did Kaplan–Meier survival analysis for DFS.
Statistical Analysis
Statistical analyses were performed based on R program (v3.6.1). Statistical significance is shown as ∗P < 0.05, ∗∗P < 0.01, or ∗∗∗P < 0.001.
Results
lncRNA Promoters Show Global Hyper-Methylation in Colon Cancer
Alteration of DNA methylation has been implicated in tumor progression and disease development, and studies have identified a potential role for DNA methylation in early screening of colon cancer (Chen et al., 2019). Here, we investigated DNA methylation in lncRNA promoters in colon cancer. We successfully mapped 43,881 methylation probes to lncRNA promoter regions (Figure 1A) to study DNA methylation in lncRNAs. Next, we identified 1,566 DMSs in lncRNAs (FDR < 0.05, absolute methylation difference > 0.3, Figure 1B). Most of the DMSs displayed higher methylation values in cancer samples than in normal samples (Figure 1B). We further classified DMSs as hyper-DMSs (methylation difference > 0) or hypo-DMSs (methylation difference < 0). All chromosomes had more hyper-DMSs than hypo-DMSs, and chromosome 9 had only hyper-DMSs in lncRNA promoters (Figure 1C and Supplementary Table 1). In addition, we found many more hyper-DMSs than hypo-DMSs in antisense, lincRNA, and process transcripts (8. 3-, 3. 7-, and 6.6-fold differences, respectively, Figure 1C). These hyper- and hypo-DMSs are potential regulators of the host lncRNAs and may participate in the pathogenic processes of colon cancer.
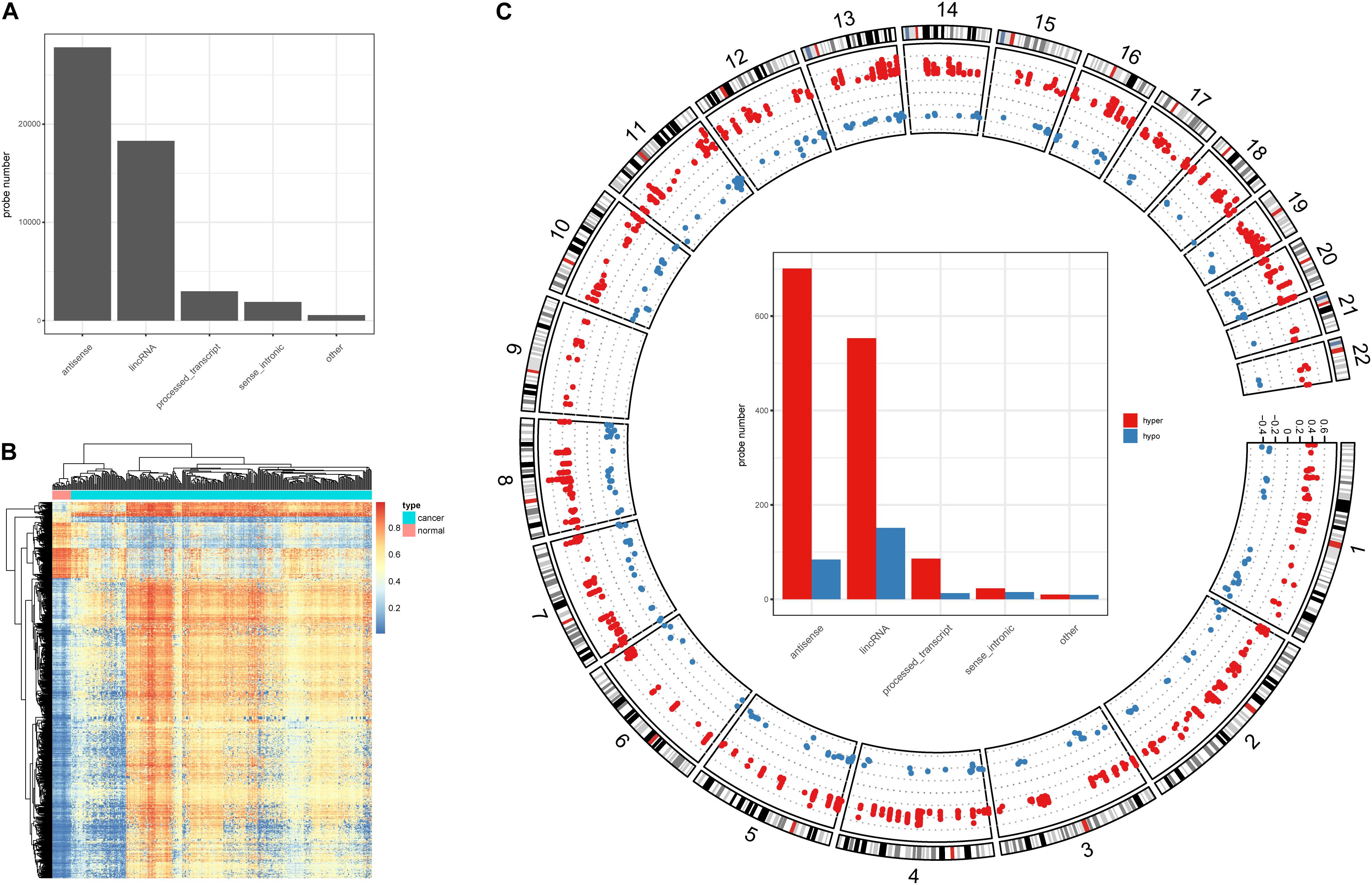
Figure 1. DNA methylation character in lncRNA promoters. (A) Probe number in each lncRNA class. (B) Heatmap of the DMSs in lncRNA promoters. (C) Circos plot of the hyper- and hypo DMSs; y-axis shows the methylation difference level, inside bar plot represent the hyper- and hypo DMSs statistics in each lncRNA class.
Relationships Between Promoter Methylation and lncRNA in Colon Cancer
To investigate the role of the identified DMSs in lncRNA transcription regulation, we systematically identified correlated DNA methylation site–lncRNA pairs (lncQTMs) in colon cancer (Figure 2A). In most chromosomes, promoter methylation sites were more likely to negatively regulate lncRNA expression; indeed, some chromosomes had no positive lncQTM pairs (Figure 2B). In total, there were 322 negative lncQTMs (correlation coefficient < 0, 87.5%) and 46 positive lncQTMs (correlation coefficient > 0, 12.5%) (Figure 2C). This indicates that promoter methylation sites are more likely to inhibit lncRNA expression in colon cancer, consistent with previous findings about DNA methylation in regulation of protein-coding genes (Schelleckes et al., 2017). In addition, we classified both positive and negative lncQTMs according to the distance between methylation sites and TSSs. For negative lncQTMs, methylation sites closer to lncRNA TSSs displayed a more significant character (smaller p-value, Figure 2D), however, for positive lncQTMs, some methylation sites located within 1,500–2,000 bp of lncRNA TSSs showed more significant character than methylation sites within 200 bp (Figure 2E). Furthermore, we analyzed correlation strengths for the lncQTMs in different distance classes: in negative lncQTMs, DNA methylation sites located within 500 bp of TSSs showed strong correlations with lncRNAs (two-tailed Wilcoxon rank-sum test, FDR < 0.05), and there was no significant difference when the distance increased (Figure 2F). For positive lncQTMs, they did not show significant differences when the distance increased (Figure 2G). This suggests that lncRNA promotor methylation sites are likely to inhibit expression, and that sites located closer to the TSSs have stronger correlations with the corresponding lncRNAs.
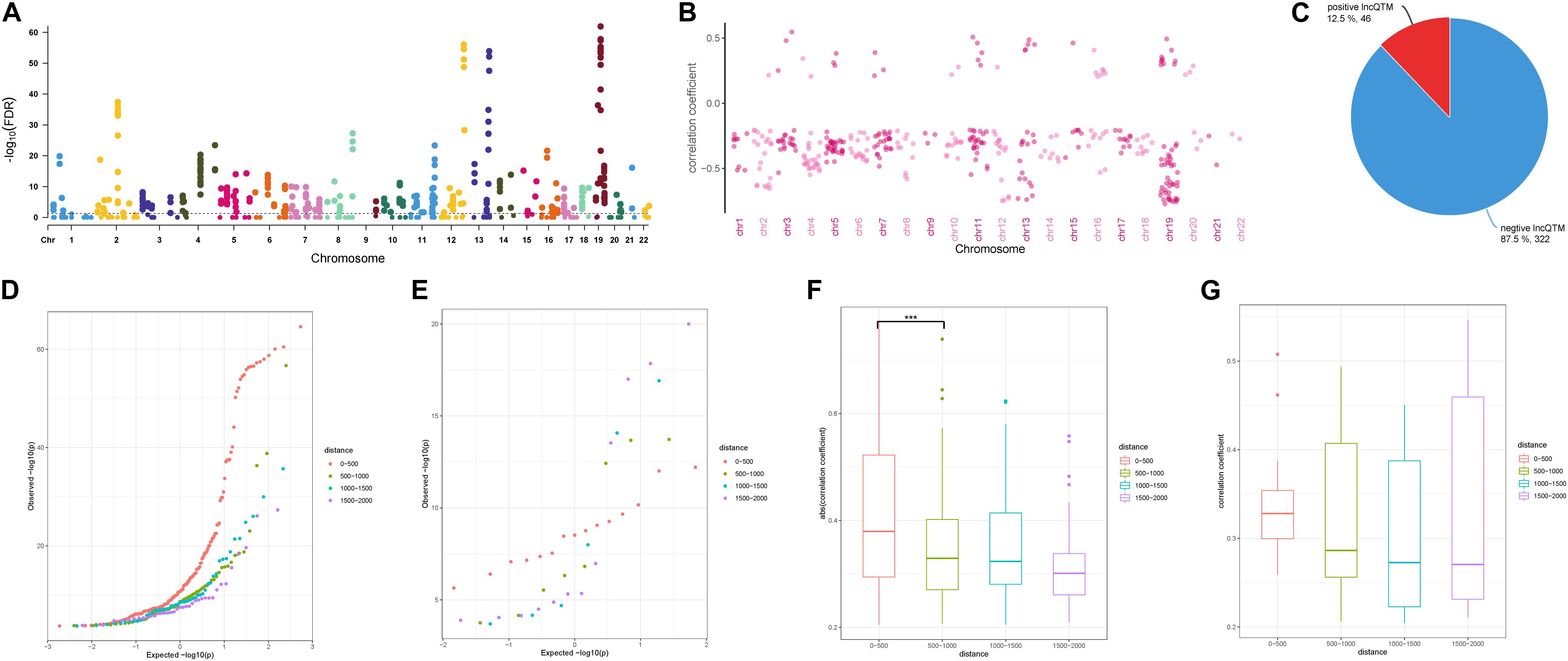
Figure 2. Character of lncQTMs in colon cancer. (A) Manhattan plot methylation-lncRNA FDR values in each chromosome (Spearman’s rank correlation test). The horizontal dotted line indicates the significant cut-off (FDR = 0.05) of lncQTMs. (B) Distribution of correlation coefficient of lncQTMs in each chromosome. (C) The number of positive and negative LncQTMs. (D) Quantile-Quantile Plot of negative lncQTMs for each distance category. (E) Quantile-Quantile Plot of positive lncQTMs for each distance category. (F) Boxplot of negative lncQTMs correlation coefficient relative to different distance. (G) Boxplot of positive lncQTMs correlation coefficient relative to different distance. ***Denote p < 0.001.
Identification of TFs Around lncQTMs in Colon Cancer
DNA methylation can modulate gene expression in multiple ways (Moore et al., 2013); specifically, it can shape TF binding events across human tissues (Hu et al., 2013; O’Malley et al., 2016). We thus speculated that human TFs might be sensitive to changes in DNA methylation in lncQTMs. Based on this speculation, we scanned TF motif occurrence in lncQTMs using the FIMO tool (Figure 3A). We expected the significant TF motifs (p < 0.0001, methylation site located within the motif) to be more enriched in lncQTMs than in all lncRNA promoter methylation sites (OR > 1.1). After filtering, we obtained 431 motifs located in lncQTMs (Figure 3B). These motifs associated with TFs are potential regulators of the corresponding lncRNAs. To confirm the relationships among TFs, methylation sites, and lncRNAs, we identified significantly correlated TF–methylation site and TF–lncRNA pairs using Spearman’s rank test (FDR < 0.05). Finally, we obtained 26 TF–methylation–lncRNA relationships in colon cancer, comprising 16 TFs, 23 methylation sites, and 20 lncRNAs (Figure 3C). DNA methylation on gene promoters generally repress transcription, whereas some researchers had reported the role of DNA methylation in promoting the transcription (Harris et al., 2018). Among the network, there were 24 methylation-lncRNA pairs; 21 pairs were negatively correlated while three pairs were positively correlated. Therefore, the hyper-DMSs in colon cancer may lead to the loss of TF-lncRNA relationship when they are negatively correlated with the corresponding lncRNAs. For the hypo-DMSs in colon cancer, they may lead to the gain of TF-lncRNA relationship when they are negatively correlated with the corresponding lncRNAs. Positively correlated pairs may have the opposite relationship with the negatively correlated pairs. Thus, these DMSs in network may lead to the gain of seven TF-lncRNA regulation relationships, and the loss of 19 TF-lncRNA regulation relationships. The regulatory relationship among TFs, DNA methylation sites, and lncRNAs is complex, and our results need to be further validated. Of the 20 lncRNAs regulated by the interplay between TF and methylation, 16 lncRNAs displayed a differential expression pattern (two-tailed Wilcoxon rank-sum test, p < 0.05, Figure 4D) between cancer and normal samples. These included lncRNA LINC00944, which has been shown to participate in the process of liver metastasis in colorectal cancer (Li et al., 2020), and lncRNA LINC00460, hypo-methylation of which can promote colon cancer and represents a potential biomarker (Zhang et al., 2019). Therefore, these TF–methylation–lncRNA events may modulate colon cancer progression and altering the interactions among them may be beneficial for therapy and prognosis.
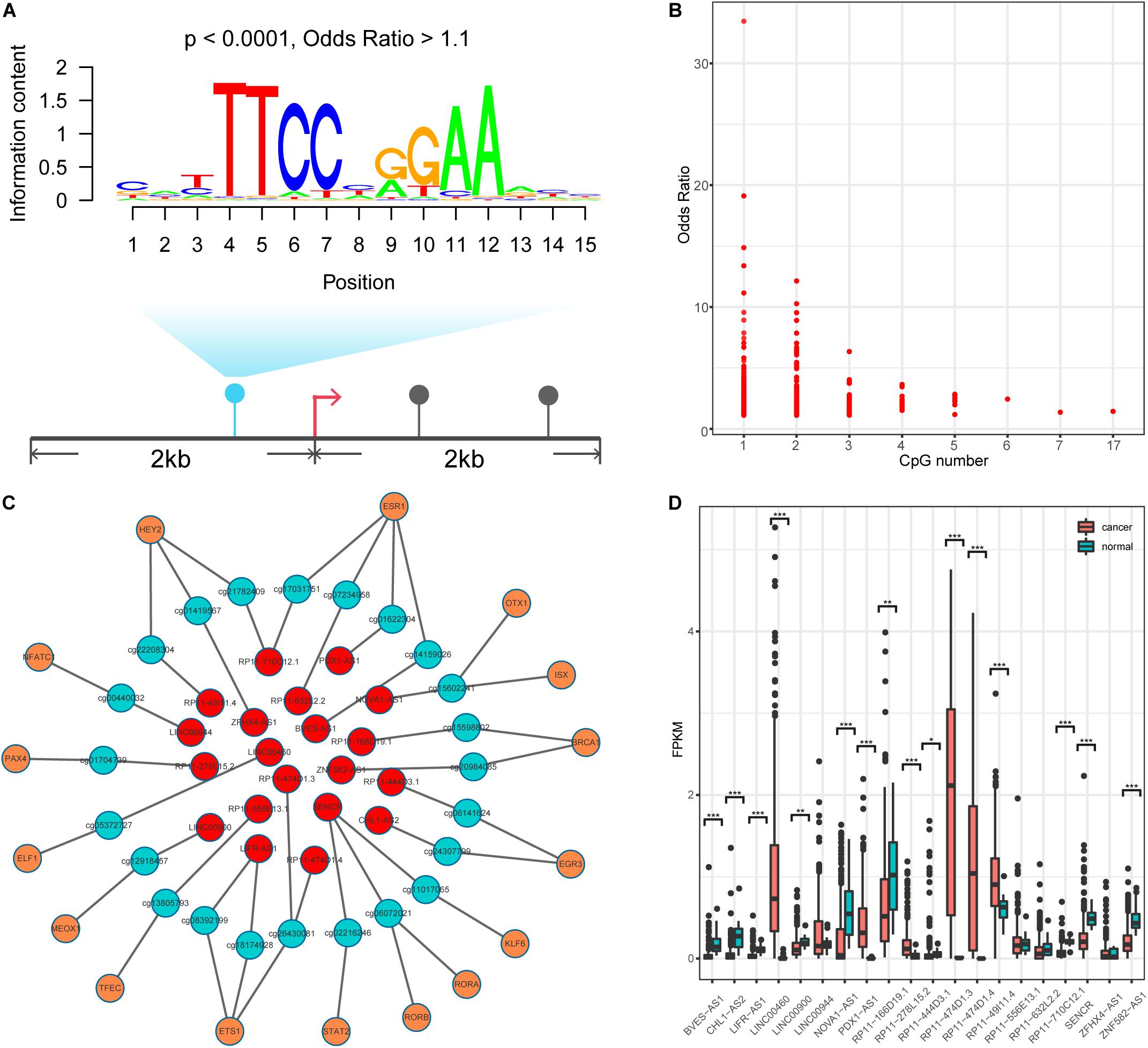
Figure 3. Identification TF-methylation-lncRNA relationships. (A) An example for illustrating the TF motif occurrence in lncQTM. (B) Lower OR value and the related CpG number. (C) An unweighted network of TF-methylation-lncRNA relationships, including 59 nodes (16 TFs, 23 methylation sites, and 20 lncRNAs), 49 edges (25 edges between TFs and DNA methylation sites, 24 edges between DNA methylation sites and lncRNAs). Red nodes represent lncRNAs, green nodes represent methylation sites, and orange nodes represent TFs. (D) LncRNA expression level for colon cancer and normal samples (Wilcoxon rank-sum test, two-tailed). *Denote p < 0.05, **denote p < 0.01, ***denote p < 0.001.
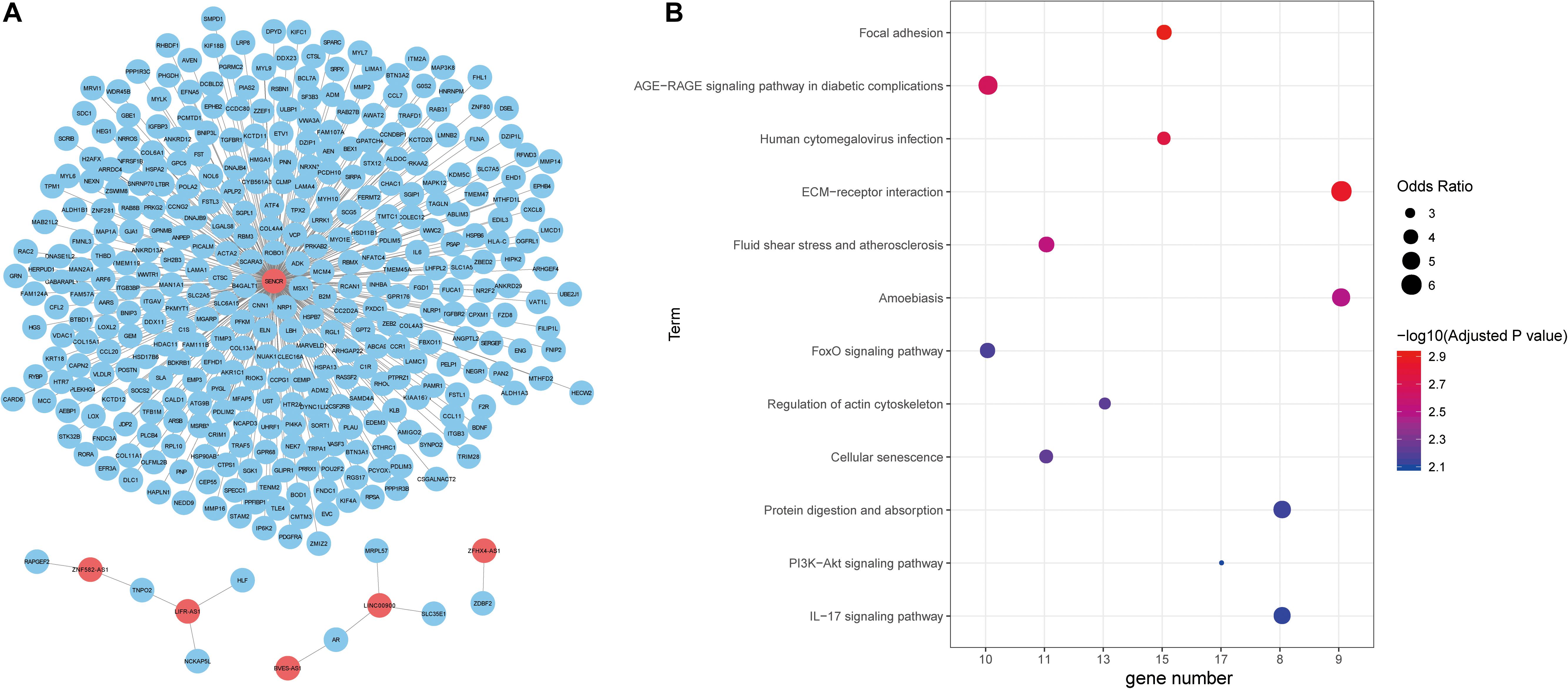
Figure 4. Functional annotation of lncRNAs. (A) The unweighted network of lncRNA-mRNA pairs, including 370 nodes (6 lncRNAs and 364 mRNAs), 366 edges. Red nodes represent lncRNAs, blue nodes represent mRNAs. (B) Significant KEGG pathways derived from the Enrichr web server.
Next, we explored the function of lncRNAs that were regulated by the interplay between TFs and DNA methylation. By integrating RNA-RNA interaction and gene expression profiles, we established a lncRNA-mRNA network (Figure 4A). Noticeably, lncRNA SENCR could interact with 356 mRNA in colon cancer. Previous studies had reported the role of SENCR in multiple human cancers (Jiang et al., 2017; Xu et al., 2019; Zheng et al., 2019). DANCR had also been proven to promote the proliferation and metastasis of colorectal cancer via miR-577 sponging (Wang et al., 2018a). By conducting functional enrichment analysis, we observed some pathways had been elaborated to play vital roles in colon cancer (Figure 4B). For example, cellular senescence could predict the treatment outcome for metastasized colorectal cancer (Haugstetter et al., 2010). Interleukin-17 (IL-17) acts as an important promoter to affect the initiation and progression of colorectal cancer (Wu et al., 2013). Collectively, functional enrichment results showed lncRNAs in network potentially affect colon cancer by interacting with the corresponding mRNAs.
lncRNAs Are Associated With Drug Response in Colon Cancer
Recently, lncRNAs have been used to shed light on drug responses in human cancer (Wang et al., 2018b). Here, we also investigate the associations between lncRNA and anticancer drug response. We downloaded pharmacological and lncRNA profiles for colon cancer from the CCLE database and obtained a total of 19 drug components used in colon cancer. Among the colon cancer cell lines in CCLE, eight lncRNAs (from the TF–methylation–lncRNA network) are expressed in 50% of cell lines. The expression level of four lncRNAs are positively or negatively correlated with the activity of seven drugs in colon cancer cell lines (Figure 5A), suggesting lncRNAs could reflect the drug response in colon cancer. We divided cancer samples into two groups based on the median lncRNA expression value and investigated the differences in drug response (activity area) between the two groups. Of the correlated lncRNA-drug pairs, three lncRNAs could distinguish the responses of three drugs (LINC00460 and AZD6244, LINC00460 and PD-0325901, and RP11-278L15.2 and PLX4720) in the cancer samples (Figure 5B, Wilcoxon rank-sum test, two tailed, p < 0.05). Next, we further stratified colon cancer samples into resistant and sensitive groups for each drug (Supplementary Table 2) and tested the lncRNA expression difference between two groups (methods). Noticeably, lncRNA RP11-278L15.2 showed significantly different expression levels between the resistant and sensitive groups of the drug PLX4720 (Figure 5C). In addition, the expression level of RP11-278L15.2 was positively correlated with the activity of drug PLX4720 (Figure 5D, p = 0.02, correlation coefficient = 0.59). Therefore, RP11-278L15.2 could potentially serve as a biomarker to estimate the PLX4720 effect in clinical research.
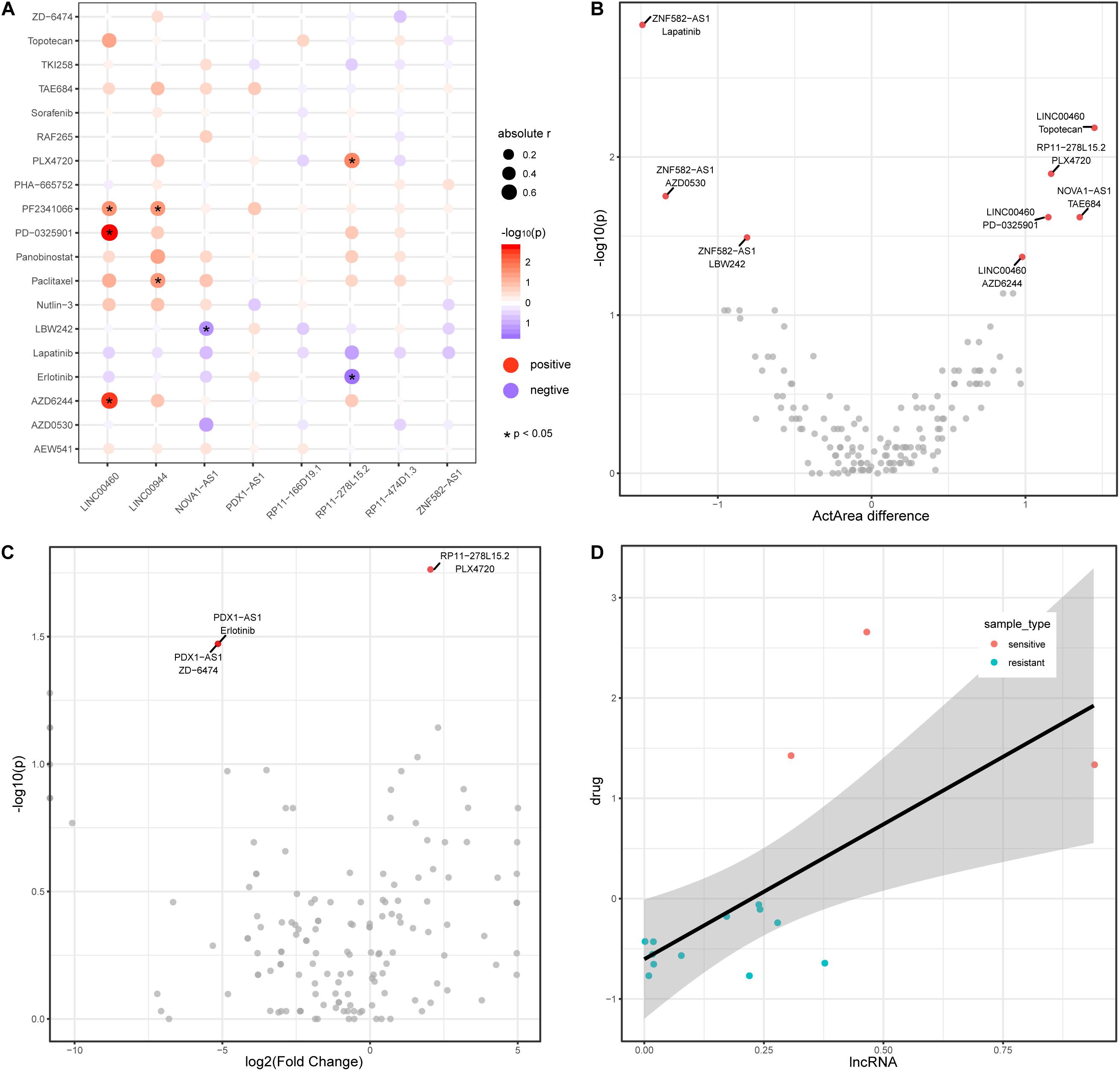
Figure 5. LncRNAs are associated with drug activity in colon cancer. (A) Correlation between lncRNAs (x-axis) and drug activity (y-axis, activity area) by Spearman’s rank correlation test. Circle size represents the absolute correlation coefficient between lncRNAs and drugs, red indicates positive correlation, and blue indicates negative correlation. *Denote p < 0.05. (B) Scatter plot of Activity area difference (x-axis) and –log10(p-value) derived from Wilcoxon rank-sum test (two-tailed, y-axis) for each lncRNA-drug pair. (C) Scatter plot of log2(Fold Change) of lncRNA expression level between cancer and normal samples and -log10(p-value) derived from Wilcoxon rank-sum test (two-tailed, y-axis) for each lncRNA-drug pair. (D) Scatter plot of the expression between lncRNA RP11-278L15.2 and the activity of drug PLX4720.
Mining TF–Methylation–lncRNA Prognostic Signatures in Colon Cancer
The TF–methylation–lncRNA regulatory events detected in our research might affect patient prognosis in colon cancer. In the training set, we designed risk models related with the individual TFs, DNA methylation sites, lncRNAs, and the combination of TF–methylation–lncRNA relationship by Cox proportional regression method (methods). Patients were divided into low- and high-groups based on the obtained risk score. Next, we performed survival analysis using the Kaplan–Meier estimate method and obtained the corresponding p-value (log rank test). Results showed TF–methylation–lncRNA had better efficiency (lower p values) than individual components (Supplementary Figure 1A). Therefore, we used the risk model derived from TF–methylation–lncRNA relationships. One of the identified TF–methylation–lncRNA relationships (ELF1-cg05372727- LINC00460) was significantly associated with survival in the training set (log rank p < 0.05, Figures 6A,B) and could also be validated in the testing set (log rank p < 0.05, Figures 6C,D and Supplementary Table 3), which is the potential prognostic biomarker for colon cancer. We applied our risk model in DFS and divided patients into low- and high-groups. Results showed our risk model is not applicable for DFS (Supplementary Figure 1B and Supplementary Table 3). This result emphasizes the important role of TF–methylation–lncRNA in OS survival for colon cancer.
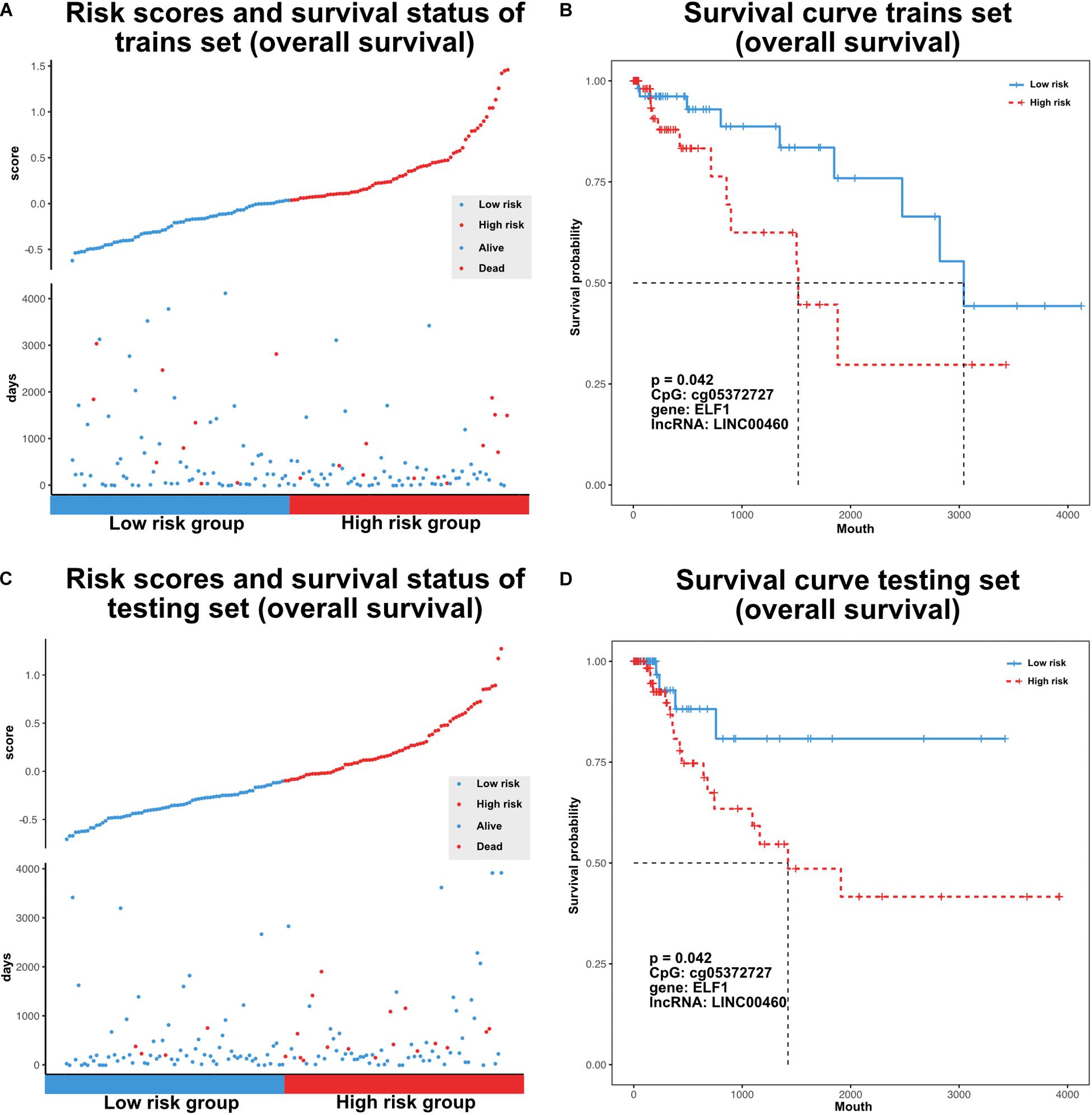
Figure 6. Characterize prognostic related TF-methylation-lncRNA in colon cancer. (A) Scatter plot of risk scores and OS status in TCGA training set. (B) OS curve for two risk groups in TCGA training set, p-value is calculated from log-rank test. (C) Scatter plot of risk scores and OS status in TCGA testing set. (D) OS curve for two risk groups in TCGA testing set, p-value is calculated from log-rank test.
Discussion
Colon cancer remains one of the most commonly diagnosed cancers worldwide. An accumulation of genetic and epigenetic alterations can result in the progression of colon cancer (Fadda et al., 2018). DNA methylation is a stable epigenetic marker, and its alteration has been shown to influence a range of diseases, including cancer (Kim and Costello, 2017). Some studies have reported the vital role of DNA methylation in protein-coding genes (Gonzalo et al., 2010; Ashktorab et al., 2013, 2014), however, its impact in non-coding genomes has not been widely understood. Here, we systematically characterized DMSs associated with lncRNAs and found that most DMSs in lncRNA promoters displayed a hyper-methylation pattern. These DMSs might modulate the lncRNA transcription process and further affect downstream biological responses triggered by the corresponding lncRNAs.
To gain insight into the relationships between DMSs and lncRNAs, we identified lncQTMs by association analysis in colon cancer. Of the 368 lncQTMs, 322 (87.5%) showed negative regulation. This suggests that DNA methylation in lncRNA promoters is likely to repress lncRNA expression in colon cancer. Negative lncQTMs that were closer to lncRNA TSSs had a greater and more significant impact on the expression level. DNA methylation alterations can influence chromatin status and TF binding events. Sequence-specific TFs can recognize typical DNA sequence (motifs) in regulatory elements and regulate the corresponding genes (Stormo, 2013). We thus made use of the known TF motifs and investigated motif occurrence around lncQTMs. We also considered the correlations among TFs, methylation sites, and lncRNAs to establish TF–methylation–lncRNA relationships. Of the lncRNAs that were regulated by the interplay between DNA methylation and TFs, lncRNAs HAND2-AS1 and LINC00460 have been shown to play a part in colon cancer development. Thus, regulation of lncRNA transcription by abnormal DNA methylation sites has a potential role in colon cancer progression.
lncRNAs have multiple functions in cancer drug sensitivity and patient prognosis (Tian et al., 2018; Jiang et al., 2019; Zhao et al., 2019). We thus investigated whether the lncRNAs shown to be mediated by DNA methylation and TFs in our study had an impact on drug efficacy and prognosis in colon cancer. Based on pharmacological and lncRNA profiles from the CCLE database, we observed that some lncRNAs are significantly correlated with drug activity. Colon cancer cell lines showed significant differences in drug response when stratified by the expression levels of lncRNAs. We divided samples into sensitive and resistant groups and found lncRNA RP11-278L15.2 display significant expression differences between the two groups of drug PLX4720, which potentially reflects the drug response of PLX4720 in clinical research. However, due to the limited cell lines analyzed in this study, we could not accurately evaluate the lncRNA efficiency in drug response prediction. With the increasement of datasets, we will continue to explore it.
We also performed survival analysis by combining TF–methylation–lncRNA relationships and found ELF1-cg05372727-LINC00460 could be used to categorize colon cancer patients into low- and high-risk groups. Furthermore, we tend to validate the prognostic efficiency of ELF1-cg05372727-LINC00460 in the independent datasets. However, there is no other public datasets that contained DNA methylation, gene expression, and OS data. By using the Gene Expression Omnibus (GEO) database, we did survival analysis based on the risk score model on two datasets that only contained expression and OS time (GSE17536, GSE59582). Results showed ELF1-LINC00460 was not associated with OS time in these two datasets (Supplementary Figures 1C,D, p = 0.26 in GSE17536, p = 0.1 in GSE59582). This suggests the importance of combining DNA methylation level of cg05372727 and the expression level of ELF1 and LINC00460 in survival prediction. With the generation of the appropriate data, we will continue to validate it.
In summary, our results provide a comprehensive view of DNA methylation in lncRNA promoters, and further demonstrate that the lncRNA transcription process is mediated by the interplay between DNA methylation and TFs. In addition, we highlight the roles of lncRNAs in drug response and patient prognosis. However, further studies are required to investigate the downstream biological mechanisms in colon cancer regulated by the lncRNAs identified in our study.
Data Availability Statement
The public datasets can be downloaded from UCSC Xena archive (https://xenabrowser.net/datapages/), TCGA (https://portal.gdc.cancer.gov/), cBioPortal (https://www. cbioportal.org/), GEO (https://www.ncbi.nlm.nih.gov/geo/). All other data presented in this study are included in the article/Supplementary Table.
Author Contributions
TZ and YL conceived and designed the experiments. JZ conducted most of the experiments. ZSh, ZSo, and JL give advices and supervised some experiments. JZ and ZSh wrote this manuscript. All authors read and approved the final manuscript.
Conflict of Interest
The authors declare that the research was conducted in the absence of any commercial or financial relationships that could be construed as a potential conflict of interest.
Acknowledgments
This manuscript has been released as a pre-print at Research Square (Zhang et al., 2020).
Supplementary Material
The Supplementary Material for this article can be found online at: https://www.frontiersin.org/articles/10.3389/fgene.2020.554833/full#supplementary-material
Supplementary Figure 1 | Exploring the association between TF-methylation-lncRNA and OS in colon cancer. (A) Box plots of lncRNAs, DNA methylation sites, lncRNAs, TF-methylation-lncRNA associated p-values (−log10 transformed, log-rank test). (B) DFS curve for two risk groups in TCGA colon cancer, p-value is calculated from log-rank test. (C) OS curve for two risk groups in GSE17536 dataset, p-value is calculated from log-rank test. (D) OS curve for two risk groups in GSE39582 dataset, p-value is calculated from log-rank test.
Abbreviations
DMSs, differential methylation sites; lncRNA, long non-coding RNA; lncQTMs, lncRNA expression quantitative trait methylations; TF, transcription factor; CCLE, Cancer Cell Line Encyclopedia; COAD, colon adenocarcinoma; OR, odds ratio; OS, overall survival; DFS, Disease free survival; GEO, Gene Expression Omnibus.
Footnotes
References
Albers, C. (2019). The problem with unadjusted multiple and sequential statistical testing. Nat. Commun. 10:1921. doi: 10.1038/s41467-019-09941-0
Arnold, M., Sierra, M. S., Laversanne, M., Soerjomataram, I., Jemal, A., and Bray, F. (2017). Global patterns and trends in colorectal cancer incidence and mortality. Gut 66, 683–691. doi: 10.1136/gutjnl-2015-310912
Ashktorab, H., Daremipouran, M., Goel, A., Varma, S., Leavitt, R., Sun, X., et al. (2014). DNA methylome profiling identifies novel methylated genes in African American patients with colorectal neoplasia. Epigenetics 9, 503–512. doi: 10.4161/epi.27644
Ashktorab, H., Rahi, H., Wansley, D., Varma, S., Shokrani, B., Lee, E., et al. (2013). Toward a comprehensive and systematic methylome signature in colorectal cancers. Epigenetics 8, 807–815. doi: 10.4161/epi.25497
Bailey, T. L., Johnson, J., Grant, C. E., and Noble, W. S. (2015). The MEME Suite. Nucl. Acids Res. 43, W39–W49. doi: 10.1093/nar/gkv416
Barretina, J., Caponigro, G., Stransky, N., Venkatesan, K., Margolin, A. A., Kim, S., et al. (2012). The Cancer Cell Line Encyclopedia enables predictive modelling of anticancer drug sensitivity. Nature 483, 603–607. doi: 10.1038/nature11003
Bermudez, M., Aguilar-Medina, M., Lizarraga-Verdugo, E., Avendano-Felix, M., Silva-Benitez, E., Lopez-Camarillo, C., et al. (2019). LncRNAs as Regulators of Autophagy and Drug Resistance in Colorectal Cancer. Front. Oncol. 9:1008. doi: 10.3389/fonc.2019.01008
Bird, A. (2002). DNA methylation patterns and epigenetic memory. Genes Dev. 16, 6–21. doi: 10.1101/gad.947102
Bonder, M. J., Luijk, R., Zhernakova, D. V., Moed, M., Deelen, P., Vermaat, M., et al. (2017). Disease variants alter transcription factor levels and methylation of their binding sites. Nat. Genet. 49, 131–138. doi: 10.1038/ng.3721
Cerami, E., Gao, J., Dogrusoz, U., Gross, B. E., Sumer, S. O., Aksoy, B. A., et al. (2012). The cBio cancer genomics portal: an open platform for exploring multidimensional cancer genomics data. Cancer Discov. 2, 401–404. doi: 10.1158/2159-8290.cd-12-0095
Chen, J., Sun, H., Tang, W., Zhou, L., Xie, X., Qu, Z., et al. (2019). DNA methylation biomarkers in stool for early screening of colorectal cancer. J. Cancer 10, 5264–5271. doi: 10.7150/jca.34944
Colaprico, A., Silva, T. C., Olsen, C., Garofano, L., Cava, C., Garolini, D., et al. (2016). TCGAbiolinks: an R/Bioconductor package for integrative analysis of TCGA data. Nucl. Acids Res. 44:e71. doi: 10.1093/nar/gkv1507
Derrien, T., Johnson, R., Bussotti, G., Tanzer, A., Djebali, S., Tilgner, H., et al. (2012). The GENCODE v7 catalog of human long noncoding RNAs: analysis of their gene structure, evolution, and expression. Genome Res. 22, 1775–1789. doi: 10.1101/gr.132159.111
Dhanoa, J. K., Sethi, R. S., Verma, R., Arora, J. S., and Mukhopadhyay, C. S. (2018). Long non-coding RNA: its evolutionary relics and biological implications in mammals: a review. J. Anim. Sci. Technol. 60:25. doi: 10.1016/b978-1-78548-265-6.50002-2
Dong, Z., Zhang, N., Li, C., Wang, H., Fang, Y., Wang, J., et al. (2015). Anticancer drug sensitivity prediction in cell lines from baseline gene expression through recursive feature selection. BMC Cancer 15:489. doi: 10.1186/s12885-015-1492-6
Fadda, A., Gentilini, D., Moi, L., Barault, L., Leoni, V. P., Sulas, P., et al. (2018). Colorectal cancer early methylation alterations affect the crosstalk between cell and surrounding environment, tracing a biomarker signature specific for this tumor. Int. J. Cancer 143, 907–920. doi: 10.1002/ijc.31380
Frankish, A., Diekhans, M., Ferreira, A. M., Johnson, R., Jungreis, I., Loveland, J., et al. (2019). GENCODE reference annotation for the human and mouse genomes. Nucl. Acids Res. 47, D766–D773. doi: 10.1093/nar/gky955
Gao, J., Aksoy, B. A., Dogrusoz, U., Dresdner, G., Gross, B., Sumer, S. O., et al. (2013). Integrative analysis of complex cancer genomics and clinical profiles using the cBioPortal. Sci. Signal 6:pl1. doi: 10.1126/scisignal.2004088
Goel, M. K., Khanna, P., and Kishore, J. (2010). Understanding survival analysis: Kaplan-Meier estimate. Int. J. Ayurveda Res. 1, 274–278. doi: 10.4103/0974-7788.76794
Gonzalo, V., Lozano, J. J., Munoz, J., Balaguer, F., Pellise, M., De Miguel, C., et al. (2010). Aberrant gene promoter methylation associated with sporadic multiple colorectal cancer. PLoS One 5:e8777. doi: 10.1371/journal.pone.0008777
Grant, C. E., Bailey, T. L., and Noble, W. S. (2011). FIMO: scanning for occurrences of a given motif. Bioinformatics 27, 1017–1018. doi: 10.1093/bioinformatics/btr064
Gu, Z., Gu, L., Eils, R., Schlesner, M., and Brors, B. (2014). circlize Implements and enhances circular visualization in R. Bioinformatics 30, 2811–2812. doi: 10.1093/bioinformatics/btu393
Gutierrez-Arcelus, M., Lappalainen, T., Montgomery, S. B., Buil, A., Ongen, H., Yurovsky, A., et al. (2013). Passive and active DNA methylation and the interplay with genetic variation in gene regulation. Elife 2:e00523. doi: 10.7554/eLife.00523.024
Harris, C. J., Scheibe, M., Wongpalee, S. P., Liu, W., Cornett, E. M., Vaughan, R. M., et al. (2018). A DNA methylation reader complex that enhances gene transcription. Science 362, 1182–1186. doi: 10.1126/science.aar7854
Haugstetter, A. M., Loddenkemper, C., Lenze, D., Grone, J., Standfuss, C., Petersen, I., et al. (2010). Cellular senescence predicts treatment outcome in metastasised colorectal cancer. Br. J. Cancer 103, 505–509. doi: 10.1038/sj.bjc.6605784
Hong, S. N. (2018). Genetic and epigenetic alterations of colorectal cancer. Intest. Res. 16, 327–337. doi: 10.5217/ir.2018.16.3.327
Hu, S., Wan, J., Su, Y., Song, Q., Zeng, Y., Nguyen, H. N., et al. (2013). DNA methylation presents distinct binding sites for human transcription factors. Elife 2:e00726. doi: 10.7554/eLife.00726.028
Husquin, L. T., Rotival, M., Fagny, M., Quach, H., Zidane, N., Mcewen, L. M., et al. (2018). Exploring the genetic basis of human population differences in DNA methylation and their causal impact on immune gene regulation. Genome Biol. 19:222. doi: 10.1186/s13059-018-1601-3
Jiang, M. C., Ni, J. J., Cui, W. Y., Wang, B. Y., and Zhuo, W. (2019). Emerging roles of lncRNA in cancer and therapeutic opportunities. Am. J. Cancer Res. 9, 1354–1366.
Jiang, N., Wang, X., Xie, X., Liao, Y., Liu, N., Liu, J., et al. (2017). lncRNA DANCR promotes tumor progression and cancer stemness features in osteosarcoma by upregulating AXL via miR-33a-5p inhibition. Cancer Lett. 405, 46–55. doi: 10.1016/j.canlet.2017.06.009
Jin, Z., and Liu, Y. (2018). DNA methylation in human diseases. Genes Dis. 5, 1–8. doi: 10.1016/j.gendis.2018.01.002
Kim, M., and Costello, J. (2017). DNA methylation: an epigenetic mark of cellular memory. Exp. Mol. Med. 49:e322. doi: 10.1038/emm.2017.10
Kuipers, E. J., Grady, W. M., Lieberman, D., Seufferlein, T., Sung, J. J., Boelens, P. G., et al. (2015). Colorectal cancer. Nat. Rev. Dis. Primers 1:15065. doi: 10.1038/nrdp.2015.65
Kuleshov, M. V., Jones, M. R., Rouillard, A. D., Fernandez, N. F., Duan, Q., and Wang, Z. (2016). Enrichr: a comprehensive gene set enrichment analysis web server 2016 update. Nucl. Acids Res. 44, W90–W97. doi: 10.1093/nar/gkw377
Lambert, S. A., Jolma, A., Campitelli, L. F., Das, P. K., Yin, Y., Albu, M., et al. (2018). The Human Transcription Factors. Cell 172, 650–665. doi: 10.1016/j.cell.2018.01.029
Li, Y., Jiang, T., Zhou, W., Li, J., Li, X., Wang, Q., et al. (2020). Pan-cancer characterization of immune-related lncRNAs identifies potential oncogenic biomarkers. Nat. Commun. 11:1000. doi: 10.1038/s41467-020-14802-2
Lin, Y., Liu, T., Cui, T., Wang, Z., Zhang, Y., Tan, P., et al. (2020). RNAInter in 2020: RNA interactome repository with increased coverage and annotation. Nucl. Acids Res. 48, D189–D197. doi: 10.1093/nar/gkz804
Liu, Y., Liu, Y., Huang, R., Song, W., Wang, J., Xiao, Z., et al. (2019). Dependency of the Cancer-Specific Transcriptional Regulation Circuitry on the Promoter DNA Methylome. Cell Rep. 26:e3465. doi: 10.1016/j.celrep.2019.02.084
Maurano, M. T., Wang, H., John, S., Shafer, A., Canfield, T., Lee, K., et al. (2015). Role of DNA Methylation in Modulating Transcription Factor Occupancy. Cell Rep. 12, 1184–1195. doi: 10.1016/j.celrep.2015.07.024
Moore, L. D., Le, T., and Fan, G. (2013). DNA methylation and its basic function. Neuropsychopharmacology 38, 23–38. doi: 10.1038/npp.2012.112
Ning, L., Li, Z., Wei, D., Chen, H., and Yang, C. (2017). LncRNA, NEAT1 is a prognosis biomarker and regulates cancer progression via epithelial-mesenchymal transition in clear cell renal cell carcinoma. Cancer Biomark 19, 75–83. doi: 10.3233/cbm-160376
O’Malley, R. C., Huang, S. C., Song, L., Lewsey, M. G., Bartlett, A., Nery, J. R., et al. (2016). Cistrome and Epicistrome Features Shape the Regulatory DNA Landscape. Cell 165, 1280–1292. doi: 10.1016/j.cell.2016.04.038
Schelleckes, K., Schmitz, B., Ciarimboli, G., Lenders, M., Pavenstadt, H. J., Herrmann, E., et al. (2017). Promoter methylation inhibits expression of tumor suppressor KIBRA in human clear cell renal cell carcinoma. Clin. Epigenet. 9:109. doi: 10.1186/s13148-017-0415-6
Spitz, F., and Furlong, E. E. (2012). Transcription factors: from enhancer binding to developmental control. Nat. Rev. Genet. 13, 613–626. doi: 10.1038/nrg3207
Stormo, G. D. (2013). Modeling the specificity of protein-DNA interactions. Quant. Biol. 1, 115–130. doi: 10.1007/s40484-013-0012-4
Suehiro, Y., Wong, C. W., Chirieac, L. R., Kondo, Y., Shen, L., Webb, C. R., et al. (2008). Epigenetic-genetic interactions in the APC/WA. Clin. Cancer Res. 14, 2560–2569. doi: 10.1158/1078-0432.CCR-07-1802
Tian, T., Wang, M., Lin, S., Guo, Y., Dai, Z., Liu, K., et al. (2018). The Impact of lncRNA Dysregulation on Clinicopathology and Survival of Breast Cancer: A Systematic Review and Meta-analysis. Mol. Ther. Nucl. Acids 12, 359–369. doi: 10.1016/j.omtn.2018.05.018
Wang, Y., Lu, Z., Wang, N., Feng, J., Zhang, J., Luan, L., et al. (2018a). Long noncoding RNA DANCR promotes colorectal cancer proliferation and metastasis via miR-577 sponging. Exp. Mol. Med. 50, 1–17. doi: 10.1038/s12276-018-0082-5
Wang, Y., Wang, Z., Xu, J., Li, J., Li, S., Zhang, M., et al. (2018b). Systematic identification of non-coding pharmacogenomic landscape in cancer. Nat. Commun. 9:3192. doi: 10.1038/s41467-018-05495-9
Wang, Z., Yang, B., Zhang, M., Guo, W., Wu, Z., Wang, Y., et al. (2018c). lncRNA Epigenetic Landscape Analysis Identifies EPIC1 as an Oncogenic lncRNA that Interacts with MYC and Promotes Cell-Cycle Progression in Cancer. Cancer Cell 33:e709.
Wang, Z., Yin, J., Zhou, W., Bai, J., Xie, Y., Xu, K., et al. (2020). Complex impact of DNA methylation on transcriptional dysregulation across 22 human cancer types. Nucl. Acids Res. 48, 2287–2302. doi: 10.1093/nar/gkaa041
Wu, D., Wu, P., Huang, Q., Liu, Y., Ye, J., and Huang, J. (2013). Interleukin-17: a promoter in colorectal cancer progression. Clin. Dev. Immunol. 2013:436307. doi: 10.1155/2013/436307
Xu, Y. D., Shang, J., Li, M., and Zhang, Y. Y. (2019). LncRNA DANCR accelerates the development of multidrug resistance of gastric cancer. Eur. Rev. Med. Pharmacol. Sci. 23, 2794–2802.
Yan, X., Hu, Z., Feng, Y., Hu, X., Yuan, J., Zhao, S. D., et al. (2015). Comprehensive Genomic Characterization of Long Non-coding RNAs across Human Cancers. Cancer Cell 28, 529–540. doi: 10.1016/j.ccell.2015.09.006
Yao, L., Shen, H., Laird, P. W., Farnham, P. J., and Berman, B. P. (2015). Inferring regulatory element landscapes and transcription factor networks from cancer methylomes. Genome Biol. 16:105.
Yarani, R., Mirza, A. H., Kaur, S., and Pociot, F. (2018). The emerging role of lncRNAs in inflammatory bowel disease. Exp. Mol. Med. 50, 1–14. doi: 10.1038/s12276-018-0188-9
Yin, Y., Morgunova, E., Jolma, A., Kaasinen, E., Sahu, B., Khund-Sayeed, S., et al. (2017). Impact of cytosine methylation on DNA binding specificities of human transcription factors. Science 356:eaaj2239. doi: 10.1126/science.aaj2239
Zhang, H., Lu, Y., Wu, J., and Feng, J. (2019). LINC00460 Hypomethylation Promotes Metastasis in Colorectal Carcinoma. Front. Genet. 10:880. doi: 10.3389/fgene.2019.00880
Zhang, J., Shen, Z., Song, Z., Luan, J., Li, Y., and Zhao, T. (2020). Dissecting drug and prognostic lncRNA signature mediated by DNA methylation and transcription factor in colon cancer. Research Square Preprint. doi: 10.21203/rs.3.rs-23145/v1
Zhang, W., Yuan, W., Song, J., Wang, S., and Gu, X. (2017). LncRna CPS1-IT1 Suppresses Cell Proliferation, Invasion and Metastasis in Colorectal Cancer. Cell Physiol. Biochem. 44, 567–580. doi: 10.1159/000485091
Zhao, W., Shan, B., He, D., Cheng, Y., Li, B., Zhang, C., et al. (2019). Recent Progress in Characterizing Long Noncoding RNAs in Cancer Drug Resistance. J. Cancer 10, 6693–6702. doi: 10.7150/jca.30877
Zheng, Y., Zheng, B., Meng, X., Yan, Y., He, J., and Liu, Y. (2019). LncRNA DANCR promotes the proliferation, migration, and invasion of tongue squamous cell carcinoma cells through miR-135a-5p/KLF8 axis. Cancer Cell Int. 19:302. doi: 10.1186/s12935-019-1016-6
Keywords: colon cancer, DNA methylation, transcription factor, lncRNA, drug response, survival
Citation: Zhang J, Shen Z, Song Z, Luan J, Li Y and Zhao T (2020) Drug Response Associated With and Prognostic lncRNAs Mediated by DNA Methylation and Transcription Factors in Colon Cancer. Front. Genet. 11:554833. doi: 10.3389/fgene.2020.554833
Received: 30 April 2020; Accepted: 09 September 2020;
Published: 04 November 2020.
Edited by:
Yunyan Gu, Harbin Medical University, ChinaReviewed by:
Kumardeep Chaudhary, Icahn School of Medicine at Mount Sinai, United StatesYongsheng Kevin Li, Hainan Medical University, China
Copyright © 2020 Zhang, Shen, Song, Luan, Li and Zhao. This is an open-access article distributed under the terms of the Creative Commons Attribution License (CC BY). The use, distribution or reproduction in other forums is permitted, provided the original author(s) and the copyright owner(s) are credited and that the original publication in this journal is cited, in accordance with accepted academic practice. No use, distribution or reproduction is permitted which does not comply with these terms.
*Correspondence: Yezhou Li, bGl5ZXpob3VAamx1LmVkdS5jbg==; Tiancheng Zhao, emhhb3RpYW5jaGVuZ0BqbHUuZWR1LmNu