- 1Molecular Oncology Research Center, Barretos Cancer Hospital, Barretos, Brazil
- 2Department of Pathology, Barretos Cancer Hospital, Barretos, Brazil
- 3Department of Endoscopy, Barretos Cancer Hospital, Barretos, Brazil
- 4Translational Molecular Pathology Department, University of Texas MD Anderson Cancer Center, Houston, TX, United States
- 5Life and Health Sciences Research Institute (ICVS), School of Medicine, University of Minho, Braga, Portugal
- 6ICVS/3B's-PT Government Associate Laboratory, Guimarães, Portugal
Pyknons are specific human/primate-specific DNA motifs at least 16 nucleotides long that are repeated in blocks in intergenic and intronic regions of the genome and can be located in a new class of non-coding RNAs of variable length. Recent studies reported that pyknon deregulation could be involved in the carcinogenesis process, including colorectal cancer. We evaluated the expression profile of a set of 12 pyknons in a set of molecularly characterized colorectal cancer (CRC) patients. The pyknons (PYK10, PYK14, PYK17, PYK26, PYK27, PYK40, PYK41, PYK42, PYK43, PYK44, PYK83, and PYK90) expression was determined by qRT-PCR. A pilot analysis of 20 cases was performed, and consistent results were obtained for PYK10, PYK17, PYK42, PYK44, and PYK83. Further, the expression of the selected pyknons was evaluated in 73 CRC cases. Moreover, in 52 patients, we compared the expression profile in both tumor and normal tissues. All five pyknons analyzed showed significantly lower expression levels in the tumor compared to normal tissue. It was observed an association between expression of PYK10 with TP53 mutations (p = 0.029), PYK17 to histologic grade (p = 0.035), and PYK44 to clinical staging (p = 0.016). Moreover, levels of PYK44 were significantly associated with the patient's poor overall survival (p = 0.04). We reported the significant downregulation of pyknons motifs in tumor tissue compared with the normal counterpart, and the association of lower PYK44 expression with worse patient outcome. Further studies are needed to extend and validate these findings and determine the clinical-pathological impact.
1. Introduction
For a long time, the central dogma of molecular biology proposed RNA molecules to primarily be informational “messenger” between DNA and proteins (Sana et al., 2012; Cobb, 2015). However, only 2% of the total human genome sequence encodes genes (Mercer et al., 2009; Sana et al., 2012). Currently, it is known that the human transcriptome is more complicated than protein-coding gene collections and the intergenic regions (Pertea, 2012). Novel molecules have been discovered in the last years, and the new class of non-coding RNAs (ncRNAs) are divided into two main groups according to their nucleotide length, as small or long ncRNAs. These ncRNAs include microRNAs (miRNAs), Piwi-interacting RNAs (piRNAs), long intergenic non-coding RNAs (lincRNAs), transcription initiation RNAs (tiRNAs), sno-derived RNAs (sdRNAs), transfer RNA (t-RNA) fragments, long enhancer ncRNAs (eRNAs), and pyknon-containing transcripts among others (Pertea, 2012; Rigoutsos et al., 2017). These molecules are significant players of cellular transcription mechanisms (Cech and Steitz, 2014; Ling et al., 2015; Rigoutsos et al., 2017). The best-studied group among ncRNAs are microRNAs (miRNAs). These molecules are short RNAs of approximately 19–23 nucleotides (nt) in length that bind their target mRNAs in a sequence-dependent manner, regulating the expression of the corresponding protein-coding gene. Over the past decades, miRNAs have been found to act as mediators in molecular interactions and associated with countless diseases, including cancer (Calin et al., 2002; Bartel, 2009; Rigoutsos, 2009; Almeida et al., 2012; Tay et al., 2014; Amit et al., 2020).
Pyknons, derived from the Greek word meaning “dense, frequent, serried” was initially described by computational approaches, which aimed to investigate a high number of short sequence motifs across the human genome (Rigoutsos and Floratos, 1998; Rigoutsos et al., 2006). These motifs were posteriorly described to exhibit functional conservation in the absence of sequence conservation or synteny and at least one exonic instance in addition to the numerous identical instances in intergenic and intronic regions (Rigoutsos et al., 2006, 2017; Sana et al., 2012). Furthermore, pyknons show variable lengths and have been found in several long non-coding transcripts, suggesting that these motifs could tie together long non-coding transcripts and messenger RNAs on a broader network (Rigoutsos et al., 2017). Moreover, approximately 40% of known miRNAs show sequence similarity to pyknons, with similar transcript abundance in the cells, suggesting a putative link in such regulation process (Rigoutsos et al., 2006, 2017; Tsirigos and Rigoutsos, 2008). The previous study of pyknon sequences showed that these motifs are not syntenic, their sequences are organism-specific and are not conserved across genomes, and their intronic copies are over-represented in the same groups' protein-coding genes in primates and can be tissue-specific (Rigoutsos et al., 2006; Tsirigos and Rigoutsos, 2008; Robine et al., 2009). Despite a limited number of experiments, a possible clinical application of pyknons has been proposed as putative biomarkers due to their expression changes between health and disease, as candidates for therapeutic exploitation and also to act as a tool to discover new non-coding RNAs, that could be used to target specific transcripts in the tumor cell to increase the efficiency of immunotherapy (Rigoutsos et al., 2006; Dragomir et al., 2019). The most recent compendium of the available pyknons is organized in the pyknon database (http://cm.jefferson.edu/pyknons.html), which accounts for 209,432 distinct human pyknons at the moment and could be used to characterize these molecules and also the lncRNA interactions with other non-coding RNAs (Danis and Széll, 2018).
Colorectal cancers (CRC) constitute the third most diagnosed cancer worldwide and the second in mortality rates in 2018 (Bray et al., 2018). The last decades have witnessed an increase in CRC incidence, which can be justified by population aging, lifestyle factors like smoking, insufficient levels of physical activity, and poor dietary habits (Kuipers et al., 2015; Arnold et al., 2017). In Brazil, CRC is the second most common cancer type for both women and men in the Southeast region (INCA, 2020). The new Brazilian mortalities rate of CRC showed an increase for both men and women when comparing data between 1996 and 2012 (Oliveira et al., 2018). A recent study by our group characterized the CRC in the Brazilian population, showing high prevalence in the APC (71.4%), TP53 (56.0%), KRAS (52.7%), PIK3CA (15.4%), and FBXW7 (10.9%) gene drivers, together with alterations in the MAPK/ERK, PIK3/AKT, NOTCH, and receptor tyrosine kinase signaling pathways (Dos Santos et al., 2019). A better understanding of its biology and regulatory mechanisms are needed, including the understanding of the role of new transcripts.
Recently, Rigoutsos et al. (2017) performed a screening of pyknons that could be associated with colorectal cancer. They explored 11 pyknons located in regions associated with LOH and fragile-sites and identified a differential expression profile between normal and tumor tissue, reporting an association of pyknons expression with MSI status (PYK14, PYK17, PYK40, PYK41, and PYK42) and overall patient survival (PYK90) (Rigoutsos et al., 2017). Moreover, the authors showed that PYK90 is a functional pyknon within a novel lncRNA (N-BLR) that regulates epithelial-to-mesenchymal transition (EMT) and promotes migration and invasion in colorectal cancer (Rigoutsos et al., 2017).
In the present study, we aimed to assess the expression levels of five selected pyknons from those mentioned above in a series of 73 molecularly characterized Brazilian colorectal cancer patients compared to the tumor with adjacent normal tissue in a subset of 52 cases. Besides, we associated the pyknons expression with patients' clinical, molecular, and genetic ancestry features.
2. Materials and Methods
2.1. Study Population and Tissue Sample Collection
This study analyzed 73 patients with colorectal cancer surgically treated between 2009 and 2013 at the Department of Colorectal Surgery of Barretos Cancer Hospital, Barretos, Brazil. Clinical and pathological features of patients, such as age, gender, primary tumor location, clinical staging, and histological grade, are summarized in Table 1. Overall, the age ranged from 29 to 89 years old (median 59 ± 14.25) with similar gender distribution. The stratification by age showed 37 (50.7%) patients in the adult group (20–59 years) and 36 (49.3%) in the group above 59 years. The sigmoid colon and rectum were the most prevalent primary sites, with 34.2% and 43.8%, respectively, and the majority were in clinical stage II (52.1%). Most of the patients were not submitted to radiotherapy (89.0%) or adjuvant chemotherapy (60.3%). The patients were followed for 80 months, and the median global survival for this cohort at 24 months was 85.6% after diagnosis. This series was previously reported regarding the molecular portrait of 150 cancer-related genes, MSI and genetic ancestry as European (median of 83.1%), followed by Native American (4.1%), Asian (3.4%), and African (3.2%) (Dos Santos et al., 2019). The samples comprised 73 fresh-frozen tumors and a subset of 52 paired normal adjacent tissue. Tissues were immediately snap-frozen following the specimen's excision at the surgery and stored at −80°C at the Barretos Cancer Hospital Biobank until processing. Slides from all tissue specimens were carefully macrodissected and subjected to histological examination to confirm the diagnostic. Only tumor samples with the presence of at least 60% of tumor cells were included. The Barretos Cancer Hospital Institutional Review Board approved this study (project nr # 684/2013).
2.2. Nucleic Acid Isolation
Tumor nucleic acids were isolated from 25 mg of fresh-frozen tissue using Precellys ceramic beads in a lysis buffer for maceration, followed by QIAsymphony DNA Mini Kit following the Tissue 200 protocol (RNA extraction) for automated isolation in the QIAsymphony, according to the manufacturer's protocol (QIAGEN, Hilden, Germany). DNA and RNA concentration and quality were assessed by Nanodrop 2000 and Qubit (Thermo Scientific, Wilmington, DE, USA). The RNA integrity was assessed by the RNA Integrity Number (RIN) using Agilent 2100 Bioanalyzer (Agilent Technologies, Santa Clara, CA, USA), and only samples with RIN values ≥ 6.0 were considered.
2.3. Microsatellite Instability (MSI) Analyses
The MSI status was evaluated using a multiplex PCR comprising six quasi-monomorphic mononucleotide repeat markers (BAT-25, BAT-26, NR-21, NR-24, NR-27, and HSP110) and described previously (Dos Santos et al., 2019). Briefly, the analyses of MSI was performed using the GeneMapper v4.1 software (Applied Biosystems), and the status was classified as stable (MSS), when none of the markers were unstable, MSI-Low (MSI-L) when one of the markers were unstable or MSI-positive when two or more of the markers were unstable. MSS and MSI-L were considered MSI-negative (Berardinelli et al., 2018).
2.4. Mutation Status of APC, TP53, KRAS, BRAF, PIK3CA, and FBXW7
Our group recently reported the somatic mutation profile of 150 cancer-related genes in this cohort of colorectal cancer patients (Dos Santos et al., 2019). The mutation status was assessed using a Nextera Rapid Capture Custom Enrichment Kit, followed by Illumina HiSeq. Four thousand next-generation sequencing and the top mutated genes were selected to be associated with pyknons expression (Dos Santos et al., 2019). The most relevant hotspots regions of the genes KRAS (codons 12, 13, and 61) and BRAF (exons 11 and 15) were also validated by PCR followed by direct sequencing, as previously described by our group (Martinho et al., 2009; Yamane et al., 2014). The Sanger sequencing was performed in the 3500xL Genetic Analyzer (Applied Biosystems). Both sequences (forward and reverse) on electropherograms were analyzed visually, and all lesions with mutations were confirmed twice.
2.5. Quantitative Real Time-PCR (RT-qPCR)
The reverse transcription (RT) was performed using the Superscript II reverse transcriptase (Life Technologies). Then, the synthesized cDNA was amplified by quantitative real time-PCR (RT-qPCR), using KAPA SYBR FAST qPCR Kit Master Mix (2X) Universal (KAPA Biosynthesis). Target primers' sequences specific to each pyknon were the same described by Rigoutsos et al. (2017) and are shown in Supplementary Table 1. Every single reaction included 10.4 μL KAPA SYBR FAST qPCR Kit Master Mix (2X) Universal with ROX, 0.8 μL of the PCR primer set for an individual pyknon and 7.8 μL RNase free water. In these series, it was performed two technical replicates for all the samples. Real-time PCR was carried out in StepOne Real-time PCR System (Applied Biosystems) using the following cycling conditions: 95°C for 3 min, followed by 40 cycles of 95°C for 30 s, and 60°C for 20 s, followed by a hold at 4°C. The 2-ΔCt method was used to calculate the relative amount of each pyknon compared with the expression of the endogenous controls (average of GAPDH, U6, and ACTB). Similarly to Rigoutsos et al. (2017), if the expression values for the transcript of interest were not obtained after 35 cycles of amplification, then the specific value was not considered. The expression values of PYK44 was multiplied by 10 due to its low expression. Moreover, the amplicon sequencing of this set of pyknons in several cell lines and commercial RNAs was also performed as quality control (data not shown).
2.6. Statistical Analysis
All the statistical analyzes were performed using R-environment (R Core Team, 2019) with Bioconductor packages (Huber et al., 2015) and SPSS software (SPSS for Windows version 24, SPSS Inc., Chicago, IL, USA). The relationship between the relative expression levels of selected pyknons in cancer vs. normal or paired groups was assessed using the Mann–Whitney U-test, and P ≤ 0.05 was significant. The area under the receiver operating characteristic curve (ROC), sensitivity, and specificity were obtained using the ROCR package (Sing et al., 2005). Association of clinicopathological and molecular features with pyknon expression was evaluated using Fisher's exact test. In these associations, a pyknon was dichotomized as high/low expression values according to median values of expression as a threshold. The overall survival (OS) interval was defined from diagnostic date to the time of the first event or the date on which data were censored, according to the method of Kaplan-Meier, and the curves were compared with the use of the log-rank test using survival package (Therneau and Grambsch, 2000; Therneau, 2015).
3. Results
The molecular status is summarized in Table 1. It was observed a frequency of 4.1% (3/73) of MSI-H cases. Moreover, 57.5% (42/73) of cases showed APC mutations, 50.7% (37/73) to TP53 mutations, 50.7% (37/73) to KRAS mutations, 9.6% (7/73) to PIK3CA mutations, 6.8% (5/73) to FBXW7 mutations, and none exhibited BRAF mutations (Table 1).
3.1. Pilot Cohort for Pyknon Candidate Selection
In order to validate the specificity of each PCR product and to technically select the best pyknons, i.e., those presenting ≥ 90% amplification efficiency and with potential for differential expression in colorectal cancer, a pilot study using 12 previously reported (PYK10, PYK14, PYK17, PYK26, PYK27, PYK40, PYK41, PYK42, PYK43, PYK44, PYK83, and PYK90) (Rigoutsos et al., 2017), was performed in 20 paired tumor/normal tissue cases (Supplementary Figure 1). Based on these results, some pyknons were excluded from further analyses due to the low number of samples amplified (PYK14, PYK26, PYK27, PYK41, and PYK43) (Supplementary Figure 1). PYK90 showed no amplification in all samples analyzed (Supplementary Figure 1), whereas PYK40 presented high levels of amplification but no difference between samples and controls (p = 0.81) (Supplementary Figure 1).
Therefore, five pyknons (PYK10, PYK17, PYK42, PYK44, and PYK83) could be detected in the most of samples, showed a P-value ≤ 0.1 in the pilot results (Supplementary Figure 1) and, consequently, were used for further evaluation in an expanded cohort of 73 CRC cases.
3.2. Validation Cohort: PYK10, PYK17, PYK42, PYK44, and PYK83 Are Differentially Expressed in CRC Patients
In the validation cohort, RT-qPCR results confirmed that all five (PYK10, PYK17, PYK42, PYK44, and PYK83) pyknons showed a significant down-regulation between normal (n = 52) vs. tumor groups (n = 73) and also in the paired analysis (Figure 1 and Table 2). Furthermore, we performed the ROC curve analysis for sensitivity and specificity of the five pyknons analyzed, and observed significant results for PYK17 (p = 1.26E-06, AUC = 0.77), PYK42 (p = 2.65E-07, AUC = 0.78), and PYK83 (p = 1.30E-09, AUC = 0.83) (Supplementary Figure 2). Even in paired sample analysis, all of them presented more than 0.75 of AUC and ≥70% of specificity (Table 2).
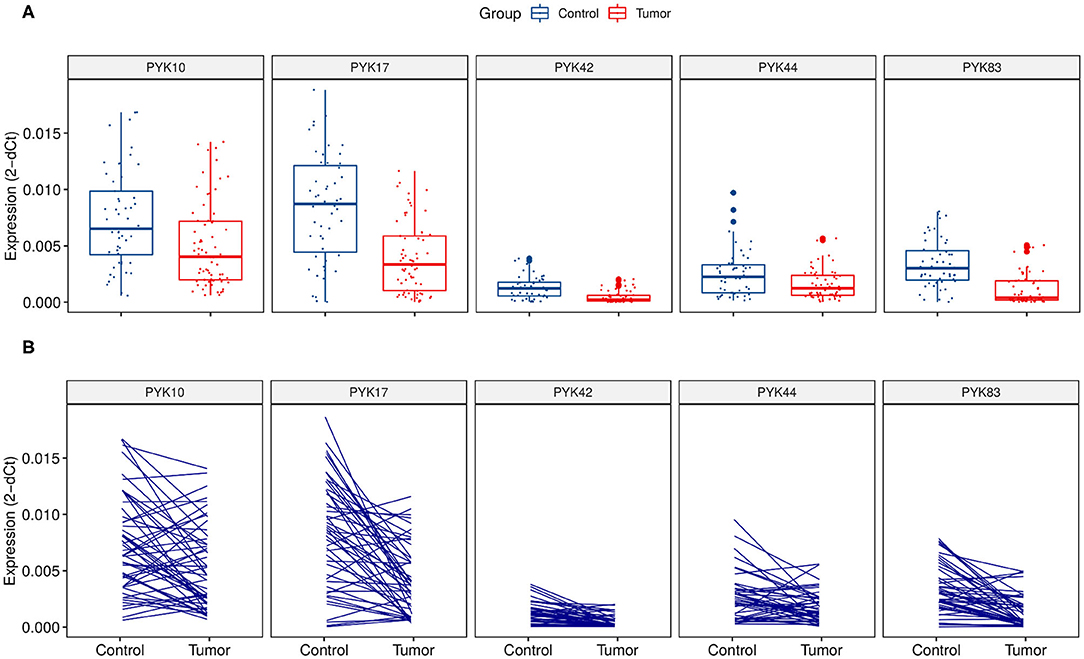
Figure 1. Relative pyknon expression in CRC samples by quantitative real-time PCR. (A) Expression and distribution of the five selected pyknons between 73 CRC patients and 52 normal samples. (B) Expression and distribution of pyknons of 52 paired samples. Y-axis values represent the ratio of each pyknon to the average of GAPDH, U6, and ACTB. The relative expression values of PYK44 were multiplied by 10 due to its low expression.
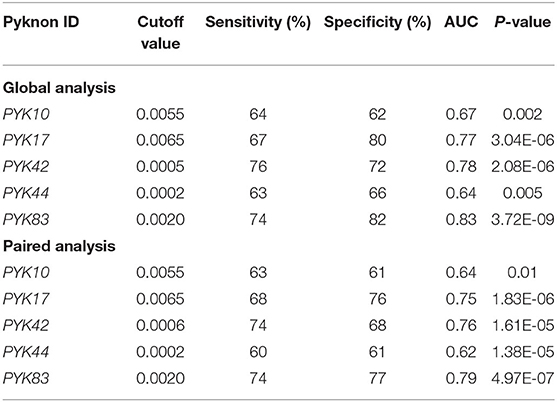
Table 2. Receiver operating characteristic (ROC) associated values for pyknons expression levels dichotomized (high/low) obtained from 73 colorectal cancer patients when compared with 52 normal tissue (global analysis) and 52 cancer/normal samples (paired analysis).
Next, we associate the differentially expressed pyknons with the patients' clinical and molecular features (Table 3). The dichotomized pyknon expression values showed that high PYK17 expression was associated with higher histologic grade (p = 0.035), low PYK44 expression was associated with higher clinical staging (p = 0.016) and high PYK10 was associated with the presence of TP53 mutations (p = 0.029) (Table 3). No significant association was observed for the PYK42 and PYK83 pyknons (Table 3).
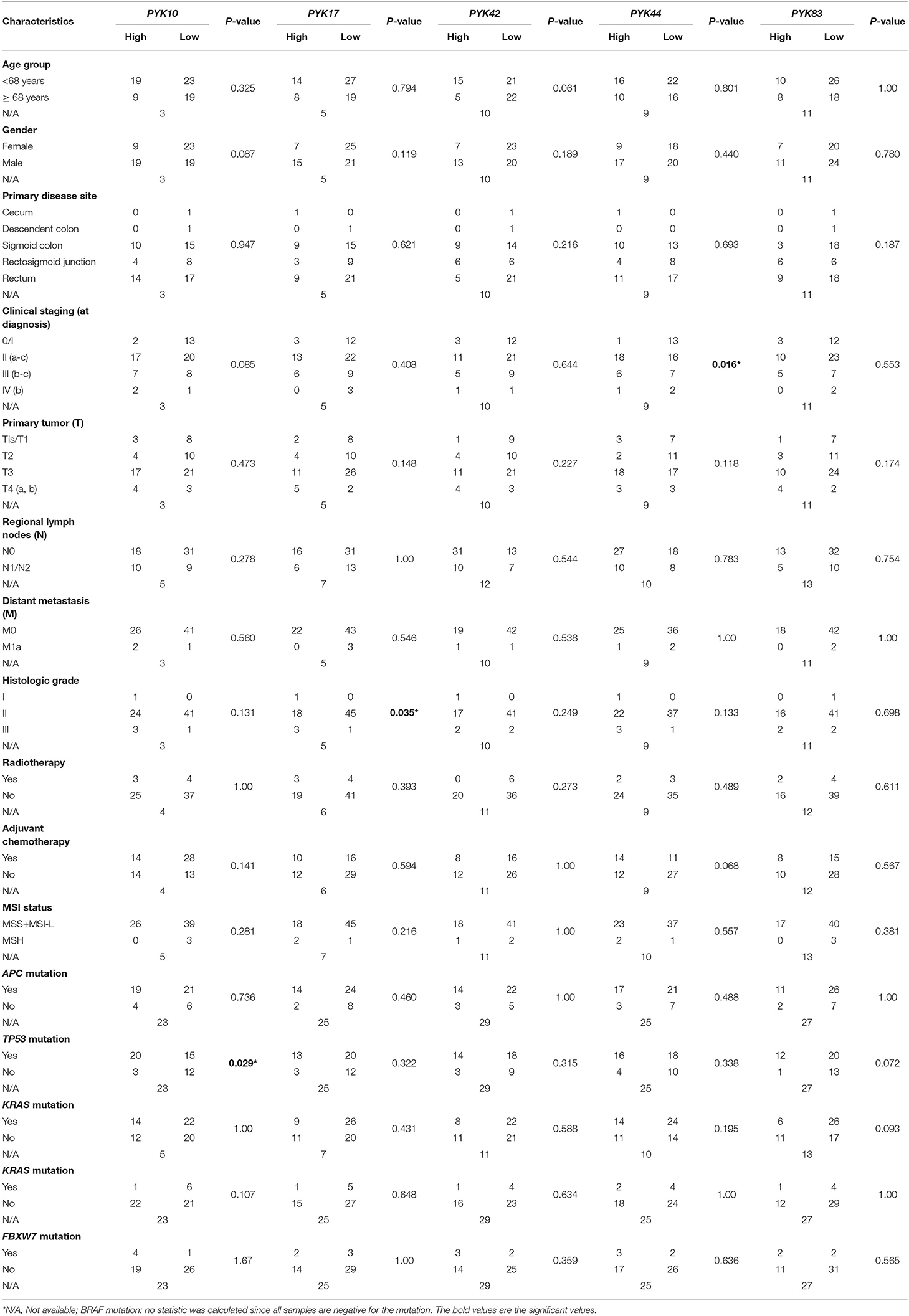
Table 3. Association of pyknons expression levels (high/low) and colorectal patients' clinicopathological and molecular features (n = 73).
3.3. Lower Tumor PYK44 Expression Is Associated With a Worse Prognosis
Furthermore, we evaluated the association of pyknon expression status (high/low) with overall patient survival (OS) (Figure 2). We found that low levels of PYK44 were significantly associated with inferior OS (p = 0.04, Figure 2D). Although its relative expression values are lower than the other pyknons, PYK44 presented a fold change of 1.6 between normal and tumor samples.
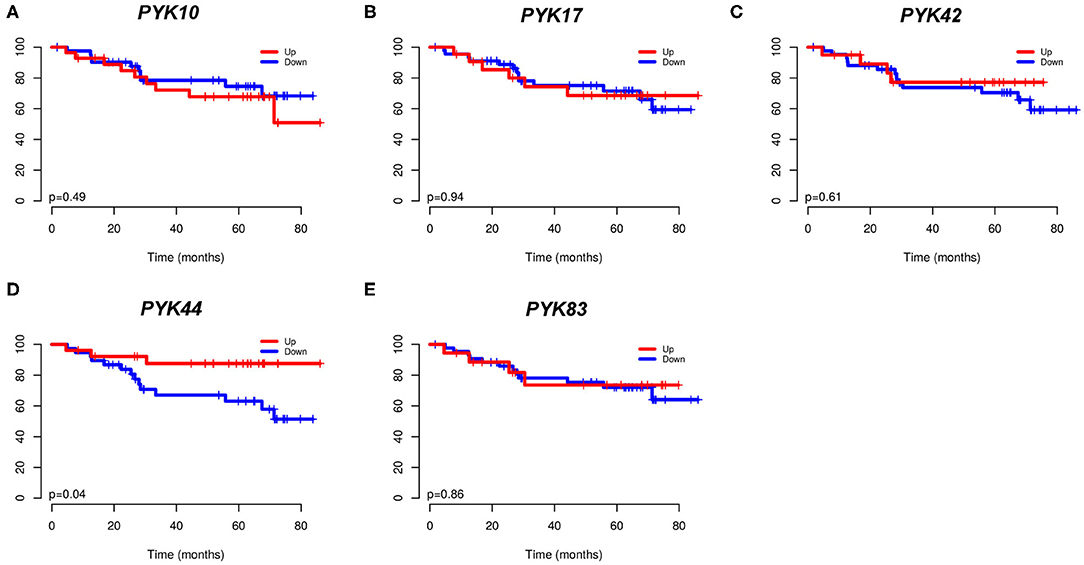
Figure 2. Kaplan-Meier curves from 73 CRC patients. In (A) PYK10; (B) PYK17; (C) PYK42; (D) PYK44; and (E) PYK83. The expression was dichotomized (high/low) according to a cutoff value corresponding to the median value of all patients.
4. Discussion
The present study intended to continue a recent study that reported the role of pyknons, a novel type of ncRNAs, in colorectal tumorigenesis (Rigoutsos et al., 2017). We evaluated the relative expression levels of 12 pyknons motifs (PYK10, PYK14, PYK17, PYK26, PYK27, PYK40, PYK41, PYK42, PYK43, PYK44, PYK83, and PYK90) in a series of 73 CRC Brazilian patients compared to 52 normal tissue counterpart and associated these results with clinicopathological, molecular and genetic ancestry features. We observed that PYK10, PYK17, PYK42, PYK44, and PYK83 exhibited significantly lower expression in tumors compared with adjacent normal tissue. Moreover, we found that high PYK10 expression was associated with TP53 mutations, and PYK44 downregulation was associated with poor prognosis in CRC.
The present results do not validate the results obtained by Rigoutsos et al. (2017), which report an overall overexpression of pyknons in tumor tissue. Notably, the authors reported that PYK14, PYK40, PYK41, PYK44, and PYK90 were significantly upregulated (Rigoutsos et al., 2017). The PYK90 was also found to be upregulated in gastric cancer (Youn et al., 2019). In our series, the PYK44 was significantly downregulated in tumor tissue, the PYK40 did not change between tumor and normal tissue, PYK41 exhibited a shallow expression level, and PYK90 had an absence of expression. However, Rigoutsos et al. (2017) did not report expression level alterations between the groups evaluating either association of PYK44 with OS. Despite the use of the same primer set and methodology, some reasons can explain these results. In the present study, we performed a paired analysis of tumor and adjacent normal tissue, at variance with Rigoutsos et al. (2017), in which tumor and normal tissue were derived from distinct patients. Moreover, the distinct geographic location of patients and distinct exposure could explain such differences since it has been suggested that race disparities could contribute to ncRNA expression (Huang et al., 2011). A previous study by our group characterized the genetic ancestry in the same study patients, showing the prevalence of European ancestry in our samples in 83.1% of the samples (Dos Santos et al., 2019). Also, Dragomir et al. (2019) recently showed downregulation of several pyknons after evaluating non-infection complications of splenectomy, including PYK10, PYK14, and PYK17. These findings show that pyknon expression could change in another context.
In the present study, we found that the downregulation of the PYK17 was associated with histologic grade II, suggesting a putative biological role for this pyknon. Interestingly, we found an association between high PYK10 with TP53 mutation. This finding is in line with previous studies since several non-coding RNAs are reported as associated with the regulation of cancer pathways, including the p53 pathway, a master regulator of the cell cycle, and survival (Huarte et al., 2010). A recent study reported that genes regulated by FLANC, a novel lncRNA co-localized with a pyknon motif, could regulate genes that are key components of the p53 pathway (Pichler et al., 2020).
Rigoutsos et al. (2017) also reported down-regulation of PYK42 and associated the lower expression of this pyknon with the MSI-H phenotype, suggesting that the PYK42 expression could be associated with most aggressive colorectal tumors. Likewise, we also observed down-regulation of PYK42 in the tumor when compared with normal adjacent tissue; however, only three cases exhibited MSI-H, hampering any meaningful conclusion.
Our results also showed down-regulation of all pyknons analyzed. These molecules are new players between the non-coding regulation molecules, and further studies are necessary to understand their modulation patterns and functional role (Dragomir et al., 2020). Considering that pyknons loci show sequence similarity with several known miRNAs that are frequently located in fragile sites of the genome associated with cancer (Calin et al., 2004; Durkin and Glover, 2007; Bartel, 2009; Hansji et al., 2014; Ling et al., 2015), recent studies have suggested that genomic events in these regions could lead to the down-regulation of miRNAs in a broad range of tumors (Lu et al., 2005; Garzon et al., 2006; Juan et al., 2010). Additionally, down-regulation of long non-coding RNAs is reported in CRC, and therefore other non-coding RNAs, such as pyknons, could show this behavior as well (Schetter et al., 2012; Mohammadi et al., 2016).
The deeper understanding of the possible regulatory role of pyknons as tumor suppressors or oncogenic non-coding RNAs is still in its infancy. Pyknons dysregulation (high or lower expression in tumors) could also be associated with transcriptional and epigenetic regulation, imprinting, splicing, subcellular transport, a scaffold for protein–protein interactions, kinase function regulation of metabolic checkpoints, following the same pattern observed in other long non-coding RNAs (Inamura, 2017). Finally, new evidence has pointed out the role of pyknons to locate functional other classes of non-coding RNAs, as a method to distinguish between functional and “junk” transcripts (Danis and Széll, 2018).
Concluding, we reported the expression profile of pyknons motifs and showed its significant downregulation in tumor tissue when compared with the normal counterpart, and the association of PYK44 lower expression with worse patient outcomes. Further studies are needed to extend and validate these findings and determine their clinical-pathological impact.
Data Availability Statement
The raw data supporting the conclusions of this article will be made available by the authors, without undue reservation.
Ethics Statement
The studies involving human participants were reviewed and approved by Barretos Cancer Hospital Ethical Committee. The patients/participants provided their written informed consent to participate in this study.
Author Contributions
AE and WM were responsible for collecting data, sample preparation, and qRT-PCR experiments. WD and GB were responsible for MSI, ancestry, and sequencing experiments. DG and CS-N were the medical doctors involved in the selection of samples. AE analyzed the data and wrote the original paper in English. GC was responsible for the initial idea, protocols, and discussion of the results. RR supervised the study, made revisions, edited the use of language, and ultimately wrote the final version of the manuscript. All authors contributed to the article and approved the submitted version.
Funding
This work was supported by Brazilian agencies FAPESP (process nr# 2013/15515-6), Fellowships from CAPES, FINEP (MCTI/FINEP/MS/SCTIE/DECIT), Public Ministry of Labor Campinas (Research, Prevention and Education of Occupational Cancer, Brazil), and MD Anderson Cancer Center (USA) SINF (Sister Institution Network Fund) grant.
Conflict of Interest
The authors declare that the research was conducted in the absence of any commercial or financial relationships that could be construed as a potential conflict of interest.
Acknowledgments
We thank Barretos Cancer Hospital Biobank for technical assistance for nucleic acid isolation.
Supplementary Material
The Supplementary Material for this article can be found online at: https://www.frontiersin.org/articles/10.3389/fgene.2020.581454/full#supplementary-material
Supplementary Table 1. Primer sequences used for quantitative real time PCR in this study.
Supplementary Figure 1. Pyknon's relative expression in 20 paired CRC samples of the pilot study was done by quantitative real-time PCR. Y-axis values represent the ratio of each pyknon to the average of GAPDH, U6, and ACTB. The relative expression values of PYK26, PYK27, PYK41, PYK43, PYK44, and PYK90 were multiplied by ten due to its low expression.
Supplementary Figure 2. Receiver operating characteristic (ROC) curve for dichotomized expression values of pyknons obtained from 73 CRC patients and 52 normal samples. The area under the ROC curve (AUC) is shown to (A) PYK10; (B) PYK17; (C) PYK42; (D) PYK44 and (E) PYK83.
References
Almeida, M. I., Nicoloso, M. S., Zeng, L., Ivan, C., Spizzo, R., Gaf, R., et al. (2012). Strand-specific miR-28-5p and miR-28-3p have distinct effects in colorectal cancer cells. Gastroenterology 142, 886–896.e9. doi: 10.1053/j.gastro.2011.12.047
Amit, M., Takahashi, H., Dragomir, M. P., Lindemann, A., Gleber-Netto, F. O., Pickering, C. R., et al. (2020). Loss of p53 drives neuron reprogramming in head and neck cancer. Nature 578, 449–454. doi: 10.1038/s41586-020-1996-3
Arnold, M., Sierra, M. S., Laversanne, M., Soerjomataram, I., Jemal, A., and Bray, F. (2017). Global patterns and trends in colorectal cancer incidence and mortality. Gut 66, 683–691. doi: 10.1136/gutjnl-2015-310912
Bartel, D. P. (2009). MicroRNAs: target recognition and regulatory functions. Cell 136, 215–233. doi: 10.1016/j.cell.2009.01.002
Berardinelli, G. N., Scapulatempo-Neto, C., Dures, R., Antnio de Oliveira, M., Guimares, D., and Reis, R. M. (2018). Advantage of HSP110 (T17) marker inclusion for microsatellite instability (MSI) detection in colorectal cancer patients. Oncotarget 9, 28691–28701. doi: 10.18632/oncotarget.25611
Bray, F., Ferlay, J., Soerjomataram, I., Siegel, R. L., Torre, L. A., and Jemal, A. (2018). Global cancer statistics 2018: GLOBOCAN estimates of incidence and mortality worldwide for 36 cancers in 185 countries. Cancer J. Clin. 68, 394–424. doi: 10.3322/caac.21492
Calin, G. A., Dumitru, C. D., Shimizu, M., Bichi, R., Zupo, S., Noch, E., et al. (2002). Frequent deletions and down-regulation of micro- RNA genes miR15 and miR16 at 13q14 in chronic lymphocytic leukemia. Proc. Natl. Acad. Sci. U.S.A. 99, 15524–15529. doi: 10.1073/pnas.242606799
Calin, G. A., Sevignani, C., Dumitru, C. D., Hyslop, T., Noch, E., Yendamuri, S., et al. (2004). Human microRNA genes are frequently located at fragile sites and genomic regions involved in cancers. Proc. Natl. Acad. Sci. U.S.A. 101, 2999–3004. doi: 10.1073/pnas.0307323101
Cech, T. R., and Steitz, J. A. (2014). The noncoding RNA revolution-trashing old rules to forge new ones. Cell 157, 77–94. doi: 10.1016/j.cell.2014.03.008
Cobb, M. (2015). Who discovered messenger RNA? Curr. Biol. 25, R526–R532. doi: 10.1016/j.cub.2015.05.032
Danis, J., and Széll, M. (2018). Functional relevance of pyknons in tumor formation. Non-coding RNA Invest. 2:3. doi: 10.21037/ncri.2017.12.05
Dos Santos, W., Sobanski, T., de Carvalho, A. C., Evangelista, A. F., Matsushita, M., Berardinelli, G. N., et al. (2019). Mutation profiling of cancer drivers in Brazilian colorectal cancer. Sci. Rep. 9:13687. doi: 10.1038/s41598-019-49611-1
Dragomir, M. P., Kopetz, S., Ajani, J. A., and Calin, G. A. (2020). Non-coding RNAs in GI cancers: from cancer hallmarks to clinical utility. Gut 69, 748–763. doi: 10.1136/gutjnl-2019-318279
Dragomir, M. P., Tudor, S., Okubo, K., Shimizu, M., Chen, M., Giza, D. E., et al. (2019). The non-coding RNome after splenectomy. J. Cell. Mol. Med. 23, 7844–7858. doi: 10.1111/jcmm.14664
Durkin, S. G., and Glover, T. W. (2007). Chromosome fragile sites. Annu. Rev. Genet. 41, 169–192. doi: 10.1146/annurev.genet.41.042007.165900
Garzon, R., Fabbri, M., Cimmino, A., Calin, G. A., and Croce, C. M. (2006). MicroRNA expression and function in cancer. Trends Mol. Med. 12, 580–587. doi: 10.1016/j.molmed.2006.10.006
Hansji, H., Leung, E. Y., Baguley, B. C., Finlay, G. J., and Askarian-Amiri, M. E. (2014). Keeping abreast with long non-coding RNAs in mammary gland development and breast cancer. Front. Genet. 5:379. doi: 10.3389/fgene.2014.00379
Huang, R. S., Gamazon, E. R., Ziliak, D., Wen, Y., Im, H. K., Zhang, W., et al. (2011). Population differences in microRNA expression and biological implications. RNA Boil. 8, 692–701. doi: 10.4161/rna.8.4.16029
Huarte, M., Guttman, M., Feldser, D., Garber, M., Koziol, M. J., Kenzelmann-Broz, D., et al. (2010). A large intergenic noncoding RNA induced by p53 mediates global gene repression in the p53 response. Cell 142, 409–419. doi: 10.1016/j.cell.2010.06.040
Huber, W., Carey, V. J., Gentleman, R., Anders, S., Carlson, M., Carvalho, B. S., et al. (2015). Orchestrating high-throughput genomic analysis with Bioconductor. Nat. Methods 12, 115–121. doi: 10.1038/nmeth.3252
Inamura, K. (2017). Major tumor suppressor and oncogenic non-coding RNAs: clinical relevance in lung cancer. Cells 6:12. doi: 10.3390/cells6020012
Juan, D., Alexe, G., Antes, T., Liu, H., Madabhushi, A., Delisi, C., et al. (2010). Identification of a microRNA panel for clear-cell kidney cancer. Urology 75, 835–841. doi: 10.1016/j.urology.2009.10.033
Kuipers, E. J., Grady, W. M., Lieberman, D., Seufferlein, T., Sung, J. J., Boelens, P. G., et al. (2015). Colorectal cancer. Nat. Rev. Dis. Primers 1:15065. doi: 10.1038/nrdp.2015.65
Ling, H., Vincent, K., Pichler, M., Fodde, R., Berindan-Neagoe, I., Slack, F. J., et al. (2015). Junk DNA and the long non-coding RNA twist in cancer genetics. Oncogene 34, 5003–5011. doi: 10.1038/onc.2014.456
Lu, J., Getz, G., Miska, E. A., Alvarez-Saavedra, E., Lamb, J., Peck, D., et al. (2005). MicroRNA expression profiles classify human cancers. Nature 435, 834–838. doi: 10.1038/nature03702
Martinho, O., Gouveia, A., Viana-Pereira, M., Silva, P., Pimenta, A., Reis, R. M., et al. (2009). Low frequency of MAP kinase pathway alterations in KIT and PDGFRA wild-type GISTs. Histopathology 55, 53–62. doi: 10.1111/j.1365-2559.2009.03323.x
Mercer, T. R., Dinger, M. E., and Mattick, J. S. (2009). Long non-coding RNAs: insights into functions. Nat. Rev. Genet. 10, 155–159. doi: 10.1038/nrg2521
Mohammadi, A., Mansoori, B., and Baradaran, B. (2016). The role of microRNAs in colorectal cancer. Biomed. Pharmacother. 84, 705–713. doi: 10.1016/j.biopha.2016.09.099
Oliveira, M. M. d., Latorre, M. d. R. D. d. O., Tanaka, L. F., Rossi, B. M., and Curado, M. P. (2018). Disparities in colorectal cancer mortality across Brazilian States. Braz. J. Epidemiol. 21:e180012. doi: 10.1590/1980-549720180012
Pertea, M. (2012). The human transcriptome: an unfinished story. Genes 3, 344–360. doi: 10.3390/genes3030344
Pichler, M., Rodriguez-Aguayo, C., Nam, S. Y., Dragomir, M. P., Bayraktar, R., Anfossi, S., et al. (2020). Therapeutic potential of FLANC, a novel primate-specific long non-coding RNA in colorectal cancer. Gut. doi: 10.1136/gutjnl-2019-318903
R Core Team (2019). R: A Language and Environment for Statistical Computing. Vienna: R Foundation for Statistical Computing.
Rigoutsos, I. (2009). New tricks for animal microRNAS: targeting of amino acid coding regions at conserved and nonconserved sites. Cancer Res. 69, 3245–3248. doi: 10.1158/0008-5472.CAN-09-0352
Rigoutsos, I., and Floratos, A. (1998). Combinatorial pattern discovery in biological sequences: the TEIRESIAS algorithm. Bioinformatics 14, 55–67. doi: 10.1093/bioinformatics/14.1.55
Rigoutsos, I., Huynh, T., Miranda, K., Tsirigos, A., McHardy, A., and Platt, D. (2006). Short blocks from the noncoding parts of the human genome have instances within nearly all known genes and relate to biological processes. Proc. Natl. Acad. Sci. U.S.A. 103, 6605–6610. doi: 10.1073/pnas.0601688103
Rigoutsos, I., Lee, S. K., Nam, S. Y., Anfossi, S., Pasculli, B., Pichler, M., et al. (2017). N-BLR, a primate-specific non-coding transcript leads to colorectal cancer invasion and migration. Genome Biol. 18:98. doi: 10.1186/s13059-017-1224-0
Robine, N., Lau, N. C., Balla, S., Jin, Z., Okamura, K., Kuramochi-Miyagawa, S., et al. (2009). A broadly conserved pathway generates 3'UTR-directed primary piRNAs. Curr. Biol. 19, 2066–2076. doi: 10.1016/j.cub.2009.11.064
Sana, J., Faltejskova, P., Svoboda, M., and Slaby, O. (2012). Novel classes of non-coding RNAs and cancer. J. Transl. Med. 10:103. doi: 10.1186/1479-5876-10-103
Schetter, A. J., Okayama, H., and Harris, C. C. (2012). The role of microRNAs in colorectal cancer. Cancer J. 18, 244–252. doi: 10.1097/PPO.0b013e318258b78f
Sing, T., Sander, O., Beerenwinkel, N., and Lengauer, T. (2005). ROCR: visualizing classifier performance in R. Bioinformatics 21:7881. doi: 10.1093/bioinformatics/bti623
Tay, Y., Rinn, J., and Pandolfi, P. P. (2014). The multilayered complexity of ceRNA crosstalk and competition. Nature 505, 344–352. doi: 10.1038/nature12986
Therneau, T. M., and Grambsch, P. M. (2000). Modeling Survival Data: Extending the Cox Model. New York, NY: Springer. doi: 10.1007/978-1-4757-3294-8
Tsirigos, A., and Rigoutsos, I. (2008). Human and mouse introns are linked to the same processes and functions through each genome's most frequent non-conserved motifs. Nucleic Acids Res. 36, 3484–3493. doi: 10.1093/nar/gkn155
Yamane, L. S., Scapulatempo-Neto, C., Alvarenga, L., Oliveira, C. Z., Berardinelli, G. N., Almodova, E., et al. (2014). KRAS and BRAF mutations and MSI status in precursor lesions of colorectal cancer detected by colonoscopy. Oncol. Rep. 32, 1419–1426. doi: 10.3892/or.2014.3338
Keywords: pyknons, non-coding RNAs, gene expression regulation, TP53 mutations, colorectal cancer, Brazilian population
Citation: Evangelista AF, de Menezes WP, Berardinelli GN, Dos Santos W, Scapulatempo-Neto C, Guimarães DP, Calin GA and Reis RM (2020) Pyknon-Containing Transcripts Are Downregulated in Colorectal Cancer Tumors, and Loss of PYK44 Is Associated With Worse Patient Outcome. Front. Genet. 11:581454. doi: 10.3389/fgene.2020.581454
Received: 08 June 2020; Accepted: 14 September 2020;
Published: 12 November 2020.
Edited by:
Pantelis Hatzis, Alexander Fleming Biomedical Sciences Research Center, GreeceReviewed by:
Giovanni Blandino, Regina Elena National Cancer Institute (IRCCS), ItalyJingying Zhou, The Chinese University of Hong Kong, China
Copyright © 2020 Evangelista, de Menezes, Berardinelli, Dos Santos, Scapulatempo-Neto, Guimarães, Calin and Reis. This is an open-access article distributed under the terms of the Creative Commons Attribution License (CC BY). The use, distribution or reproduction in other forums is permitted, provided the original author(s) and the copyright owner(s) are credited and that the original publication in this journal is cited, in accordance with accepted academic practice. No use, distribution or reproduction is permitted which does not comply with these terms.
*Correspondence: Rui Manuel Reis, cnVpcmVpcy5oY2JAZ21haWwuY29t
†These authors have contributed equally to this work