- 1State Key Laboratory of Marine Environmental Science, College of Ocean and Earth Sciences, Xiamen University, Xiamen, China
- 2State Key Laboratory of Large Yellow Croaker Breeding, Ningde Fufa Fisheries Company Limited, Ningde, China
- 3Fujian Key Laboratory of Genetics and Breeding of Marine Organisms, College of Ocean and Earth Sciences, Xiamen University, Xiamen, China
- 4Xiamen Key Laboratory of Urban Sea Ecological Conservation and Restoration (USER), Xiamen University, Xiamen, China
Large-scale transcription studies have revealed numerous lncRNAs (long non-coding RNAs). lncRNAs have been proposed to participate in the regulation of a diverse range of biological processes, including transcriptional regulation. Although lncRNAs have attracted increasing attention, the studies in large yellow croaker (Larimichthys crocea) are still rare, and they lack systematic analysis. In this study, 101 RNA-seq datasets varied in ages, sexes, and tissues were retrieved from the NCBI database to generate a comprehensive catalog of large yellow croaker transcriptome database. A set of 14,599 high-confidence lncRNAs from 13,673 loci were identified and characterized. Furthermore, RNA-seq datasets obtained from the infection of C. irritans were employed to investigate the differential expression pattern of lncRNAs and analyze potential biological functions. A total of 77 differentially expressed lncRNAs targeting to 567 protein-coding genes were identified by using expression analysis. Several immune genes, including TLR5, CD2AP, and MMP9, were highlighted. With GO enrichment and KEGG pathway analysis, the immune-related terms or pathways were enriched. This study created a comprehensive dataset of lncRNAs for large yellow croaker, which would be helpful for the researches of functional roles of lncRNAs in large yellow croaker.
Introduction
With the rapid development of high-throughput sequencing technology, the genome-wide identification of RNAs has been performed in a large number of species and helped to discover numerous non-coding RNA genes (Kung et al., 2013; Iyer et al., 2015; Rinn and Chang, 2012). Established databases like NONCODE1 and GENCODE2 have annotated different classes of non-coding RNAs including long non-coding RNAs (lncRNAs), tRNA, rRNA, and microRNA. lncRNA is a class of non-coding transcripts with length over 200 nt. lncRNAs can affect expressions of local genes and remote genes in cis/trans ways as decoys, scaffolds, guides, and enhancers (Kung et al., 2013). Xist is a well-studied lncRNA and an ideal example to demonstrate the regulating function. Xist spreads across one X chromosome and initiates a series of events, including the deposition of repressive chromatin marks leading to expression silencing of almost the entire chromosome in female mammals (Chen et al., 2016; Carmona et al., 2018). lncRNAs also play important roles in the epigenetic process, cell cycle regulation, and cell differentiation (Hung et al., 2011; Lee, 2012; Fatica and Bozzoni, 2014; Kopp and Mendell, 2018). Because of the various functions, non-coding transcriptome profiling analysis has been performed in multiple species, such as mice (Josset et al., 2014), chicken (You et al., 2019), goat (Liu et al., 2018), sheep (Bakhtiarizadeh and Salami, 2019), silkworm (Bombyx mori) (Wu et al., 2016), and Pacific Oyster (Crassostrea gigas) (Sun and Feng, 2018). In teleost, similar works have been performed in red cusk-eel (Genypterus chilensis) (Dettleff et al., 2020), common carp (Cyprinus carpio) (Song et al., 2019), tilapia (Li et al., 2018), rainbow trout (Oncorhynchus mykiss) (Ali et al., 2018), and zebrafish (Pauli et al., 2012).
The roles of lncRNA in the immune system have attracted considerable attention (Schmitt and Chang, 2016; Fan et al., 2018). In human, mouse, and chicken, detailed function roles for specific lncRNAs in immunity have been elucidated like lincRNA-Cox, HOTTIP, H19, lincRNA-EPS, and lncRNA-CD244 (Carpenter et al., 2013; Chen et al., 2017). In teleost, studies have also been reported about profiling of lncRNAs under different immune challenges, such as salmon, trout, zebrafish, and large yellow croaker (Jiang et al., 2016; Valenzuela-Munoz et al., 2018; Liu et al., 2019; Valenzuela-Munoz et al., 2019).
Large yellow croaker (Larimichthys crocea) is the top maricultural fish according to the production amount in China (Chen et al., 2003). However, with overfishing, high-density aquaculture, and disease infestation, the L. crocea industry is encountering serious obstacles for sustainable development. A common parasitic disease caused by Cryptocaryon irritans has resulted in high mortalities and great economic losses (Zuo et al., 2012; Buchmann, 2015). It’s essential to analyze the pathogenesis of C. irritans. Based on a high-quality sequencing and assembly of L. crocea genome, we have constructed a high-density genetic linkage map and revealed temporal gene expression patterns in skin of L. crocea in response to C. irritans infection (Chen et al., 2019; Kong et al., 2019; Bai et al., 2020). However, the reported studies of L. crocea infected with C. irritans mainly focused on protein-coding transcripts or peptides on different tissues (Wang et al., 2016; Zheng et al., 2018; Bai et al., 2020). The regulation of immune functions of lncRNAs has not been conducted in L. crocea infected with C. irritans. This study focuses on the catalog of lncRNA and their immune regulatory functions in L. crocea. This work revealed an overall prospect of lncRNAs to improve the present annotation of the genome of L. crocea and also provide new insights on the response mechanisms to C. irritans infection of fish.
Materials and Methods
Data Collection
First, 81 RNA-seq datasets of L. crocea were downloaded from the NCBI database3. The transcriptome sequencing datasets varied in sexes, ages, tissues, and stress challenges such as cold, hypoxia, and different infections (Supplementary Table S1).
In addition, 20 RNA-seq datasets from skin samples of C. irritans infected L. crocea performed by our previous work were added into the dataset (Bai et al., 2020). Briefly, 448 healthy L. crocea (weight: 25.98 ± 32.72 g, body length 12.32 ± 6.18 cm) were obtained from Fufa Aquatic Products Co., Ltd. (Ningde, Fujian) to carry out a C. irritans challenge experiment. Before the challenge experiment, L. crocea were acclimated for 15 days in a cement tank (26 ± 0.2°C). A set of 20 healthy fish were randomly selected as the control group. The samples were collected at the beginning of the experiment, and four dying fish were sacrificed at each of the four time points: 24, 48, 72, and 96 h post infection. The skin tissue samples of the fish (16 infected fish and 4 control) were collected, and the RNA in the skin tissue was extracted and sequenced.
Transcription Assembly and Identification of lncRNA
All the raw data were first trimmed by Trimmomatic software (v0.38) (Bolger et al., 2014) to eliminate the low-quality data reads and adaptor sequences. Then, HISAT2 (v2.1.0) (Kim et al., 2015) was used to align the sequencing reads independently to a reference genome of L. crocea (Chen et al., 2019). The alignment results were assembled into transcripts by Cufflinks (v2.1.1) without reference. With orthoDB (v10), BUSCO (v5) was conducted to explore completeness according to conserved ortholog content and evaluate the accuracy of assembly (Simao et al., 2015).
A strict step-wise pipeline was performed to identify lncRNAs from transcripts. First, the transcripts longer than 200 nt were kept for further analysis. Next, three different softwares, CPC2, CNCI, and PLEK, were used to estimate the coding potential of transcripts. CPC2 was based on biologically meaningful sequence features (Kang et al., 2017); CNCI (v2) worked by profiling adjoining nucleotide triplets (Sun et al., 2013); PLEK (v1.2) used a computational pipeline based on an improved k-mer scheme and a support vector machine (SVM) algorithm (Li et al., 2014). Transcripts identified as coding RNAs by any of the three software were removed. Third, the remaining transcripts were searched against the annotation of L. crocea based on Swiss-Prot database and Nr database to remove known protein-coding RNAs and small non-coding RNAs classes like mRNA, tRNA, rRNA, miRNA, and snRNA (Chen et al., 2019). The survived transcripts were aligned to four open databases including Pfam4, Rfam5, Uniprot6, and miRBase7 using the program pfamscan (Madeira et al., 2019), Infernal (Nawrocki and Eddy, 2013), and BLAST8. All transcripts with alignment E-value < 1e-6 were removed. Last, the open reading frames (ORFs) of the remaining transcripts were predicted by using ORFfinder9. Any transcripts containing ORF more than 100 amino acid were filtered out. The set of remaining transcripts were considered as candidate lncRNAs in this study and used for the further analysis.
Classification of lncRNAs
lncRNAs were divided into four classes, including lincRNA, exonic lncRNA, intronic lncRNA, and overlapping lncRNA, by using the class code generated from Cuffcompare (Al-Tobasei et al., 2016). The classification was based on the position relationship between lncRNAs and protein-coding genes. lincRNAs, also known as intergenic lncRNAs that did not intersect with any protein-coding genes, were represented as class codes “u” and “p.” Exonic lncRNAs intersected with at least a protein-coding exon were represented as “j,” “e,” “o,” and “x.” Intronic lncRNAs that are not sharing any sequences with exons but exist in introns were represented as “i” or “=.” Overlapping lncRNAs whose introns contain a protein-coding gene were represented as “c.”
Analysis of Conservation
BLAST was used to evaluate conservation of lncRNAs in L. crocea with zebrafish and rainbow trout with an E-value < 1e-6 cut off. The data of zebrafish was downloaded from NOCODE database, and data of rainbow trout was retrieved from a previous lncRNAs profiling study (Al-Tobasei et al., 2016; Fang et al., 2018).
Expression Analysis of lncRNA
To investigate the expression pattern of L. crocea under the infection of C. irritans, Cuffdiff was used to identify differentially expressed lncRNAs (DElncRNAs) and differentially expressed protein-coding genes (DEgenes). Expression value of lncRNAs and protein-coding genes in each time point group (24, 48, 72, and 96 h post infection) and control group were determined in terms of FPKM. Transcripts expressing differently between any two groups and fulfilling with statistical significance criteria (|log2[foldchange]| ≥ 2 and p-value < 0.05) were regarded as DElncRNAs and DEgenes.
Target Gene Prediction of lncRNAs and Gene Clustering
The Pearson’s correlation coefficients (r) between each pair of DElncRNAs and DEgenes in L. crocea genome were calculated via in-house R scripts (v3.5.3). The DEgene with |r| > 0.99 and p-value < 0.05 was considered as the target gene of the paired DElncRNA.
Time course sequencing data analysis (TCseq) (v1.6.0) was used to cluster the genes having similar expression patterns. To identify the hub genes, all target genes were subjected to protein-protein interaction (PPI) network analysis with the Cytoscape software (v3.7.2). Three species, Oryzias latipes, Danio rerio, and Gasterosteus aculeatus, were selected as references in the STRING database (Szklarczyk et al., 2017). Genes with degree value (number of genes interacting with a specific gene) ranking top 10% were considered as the hub genes.
Functional Annotation and Co-expression Network Analysis
All the target genes were searched against the InnateDB10 to identify immune-related genes that play important roles in response to the infection of C. irritans. GO enrichment and KEGG pathway analysis of the target genes were conducted using OmicShare tools11 to get a better understanding of potential functional roles.
The 500 nt sequences located upstream of DElncRNAs and target genes were retrieved from the L. crocea genome to identify transcription factor binding sites (TFBS). The retrieved sequences were searched against the AnimalTFDB (v3.0)12. The sequences with p-value < 1e-6 were identified as potential TFBS.
Cytoscape software was applied to visualize an integrated network between DElncRNAs and target genes based on Pearson’s correlation coefficients and degree values from PPI analysis.
Quantitative Real-Time PCR (qRT-PCR) Verification
Using a SYBR Premix Ex Taq kit (Invitrogen), qRT-PCR was performed to validate the results of RNA-seq. Primer sets were designed on an online website13 (Supplementary Table S2). The thermal cycling profile consisted of 95°C for 5 min, followed by 40 cycles of 95°C for 15 s, and 60°C for 30 s. All the reactions were conducted in technical triplicates and using β-actin as an internal control. The expression levels of genes were analyzed using the comparative threshold (CT) cycle method (Livak and Schmittgen, 2001). Statistical analysis was conducted through one-way analysis of variance (ANOVA).
Results
Genome-Wide Identification of lncRNAs in L. crocea
A total of 1034.7 Gb RNA-seq data was mapped to the L. crocea genome by using HISAT2. The average mapping ratio for samples was 80.41% (Supplementary Table S3). The mapped reads were assembled into 140,508 transcripts by using Cufflinks. A set of 14,599 lncRNAs from 13,673 loci of the assembled transcripts were identified (Figure 1). BUSCO was used to evaluate the accuracy of assembly, and 78.6% complete single-copy or duplicated orthologs were identified (Supplementary Figure S1).
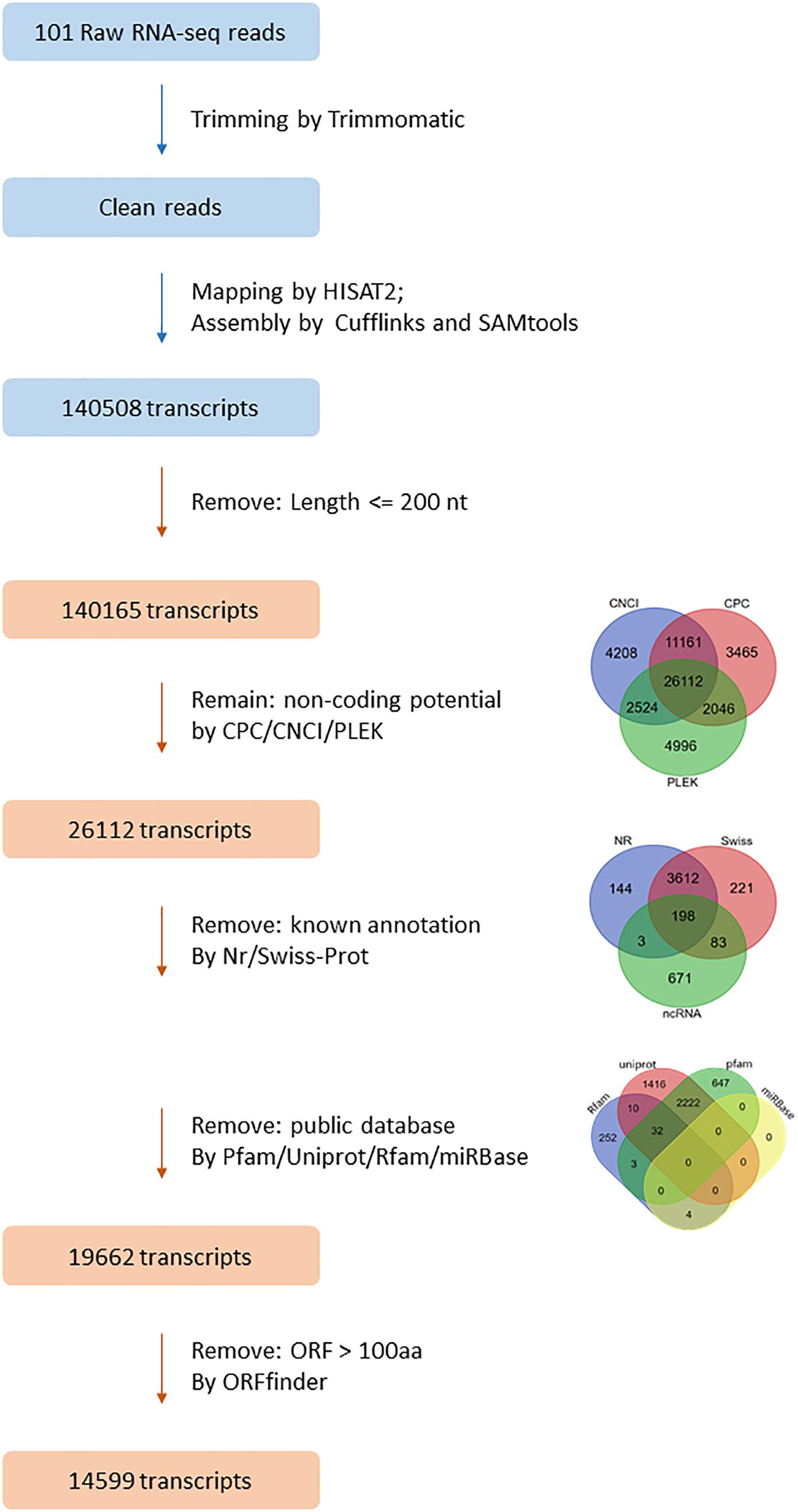
Figure 1. The filtering pipeline for identification of lncRNAs in L. crocea. Venn diagrams show the results of three coding potential prediction tools, blast against known annotation, and blast against public database. In the end, a total of 14,599 transcripts were identified as lncRNAs in L. crocea.
The length of lncRNAs ranged from 201 nt to 10,080 nt, with an average sequence length of 684 nt. The lengths of N50, N70, and N90 transcripts were 999 nt, 726 nt, and 444 nt, respectively (Table 1). The number of exons in each lncRNA ranged from 1 to 14, with an average of 1.19 exon. The majority of lncRNAs (88.06%) contained only a single exon. In contrast, the average length of mRNA was 1485, and the average number of exons in mRNA was 9.45 ranging from 1 to 143. Compared to mRNAs, lncRNAs have fewer exons and shorter transcript length, which is in accordance with the previous study (Table 2 and Figure 2) (Al-Tobasei et al., 2016; Bakhtiarizadeh and Salami, 2019).
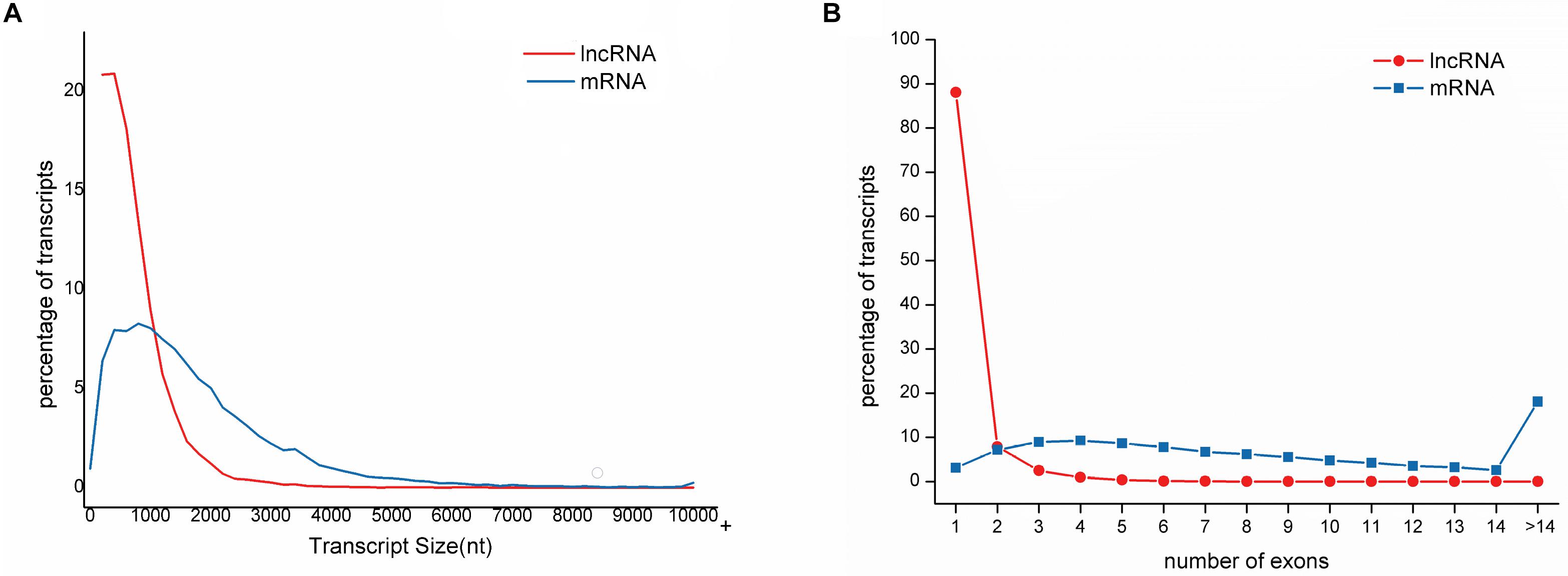
Figure 2. Distribution of sequence length and exon number of lncRNAs compared to mRNAs in large yellow croaker. (A) lncRNAs is much shorter than mRNA. The majority of lncRNAs (73.27%) is shorter than 1000 nt while only 31.45% for mRNAs. (B) lncRNAs have less exons than mRNAs. Of lncRNAs, 88.06% have only one exon while 18.06% of mRNAs have exons more than 14.
To explore the conservation of lncRNAs across different species, the 14,599 identified lncRNAs in L. crocea were blasted with lncRNAs of zebrafish and rainbow trout. Only 18 (0.12%) and 75 (0.51%) conserved lncRNAs were found in zebrafish and rainbow trout, respectively. The weak similarity proved the low level of conservation of lncRNAs between species.
Based on the position relationship with protein-coding genes, lncRNAs were divided into four classes, including lincRNA, exonic lncRNA, intronic lncRNA, and overlapping lncRNA. Among the 14,599 lncRNAs in our study, 10,008 (68.55%) lincRNAs, 1774 (12.15%) exonic lncRNAs, 2808 (19.23%) intronic lncRNAs, and 10 (0.07%) overlapping lncRNAs were identified. From the result, we can conclude that the majority of lncRNAs are lincRNAs that do not share any part with protein-coding genes.
The DElncRNAs and Target Genes Under the Infection of Cryptocaryon irritans
Using the transcript file produced in the previous step and the aligned reads from 20 RNA-seq datasets under the infection of Cryptocaryon irritans, 77 DElncRNAs were identified by Cuffdiff with the threshold of |log2FC| ≥ 2, p-value < 0.05. The 77 DElncRNAs were clustered into seven categories. Cluster1, cluster2, cluster3, cluster5, and cluster6 include lncRNAs whose expression peaks at 0, 24, 48, 72, and 96 h post-infection (Supplementary Figure S2). Cluster4 and cluster7 contain lncRNAs with continuous increase and decrease expression patterns, respectively.
Comparing the position of DElncRNAs and genes, 15 DElncRNAs were identified to locate in the genome of 46 genes of L. crocea. All genes were annotated by Swiss-Prot and 11 of them were related to immune system including C1QL4, CCL28, TNNT3, ANGPT1, BCAP29, CASQ1, S100A13, TNNI2, SERINC3, PDE1B, and STX2.
To identify target genes for DElncRNAs, we calculated the Pearson’s correlation coefficients between 77 DElncRNAs and 1,852 differential expressed genes (Supplementary Table S4). A total of 1,204 pairs with high correlation coefficient (|r| > 0.99, p-value < 0.05) including 60 DElncRNAs and 567 differential expressed genes were identified. The 567 genes were considered as the target genes in this study. Among the 1,204 high-confidence pairs, most (94.27%) were positive correlation. The number of target genes for each lncRNA ranged from 1 to 98 (TCONS_00126625 was the lncRNA that associated with the most genes), and the average number is 20. About 70% of lncRNAs have fewer than 18 target genes.
GO and KEGG Enrichment Analysis of Target Genes
All the target genes were annotated by Swiss-Prot database. The target genes were searched against the InnateDB database, which including genes involved in the innate immune response. A total of 71 genes were identified as immune-related genes, such as BCL2L1, IL17C, IL1R2, and MMP9 (Supplementary Table S5).
The target genes were clustered into five groups according to the trend of expression pattern (Figure 3). GO enrichment and KEGG pathway analysis were performed for each cluster. With the threshold of p-value < 0.01, a total of 252 GO terms were enriched in 5 clusters (Supplementary Table S6 and Supplementary Figure S3). Several GO terms related to phosphorylation and metabolic process were enriched in cluster1. Ion channel activity and transport activity were significantly enriched in cluster2, which was continually up-regulated within 96 h. Remarkably, a lot of GO terms enriched in cluster3 are related to diverse aspects of innate immune response, including toll-like receptor, cytokine, signaling pathway, and defense response. This was attributed to the up-expression of several immune-related genes, including Tlr5, MMP9, CD2AP, IL1R2, and TNFAIP3. Go terms involved in response to food were highly enriched in cluster 4 as a result of CARTPT, which encodes a preproprotein that plays a role in appetite and energy balance. The up-regulation of CARTPT in 72 h may stimulate the appetite and make response to food. The infection of C. irritans leading to the depression of movement, which may cause the enrichment of GO terms related to sarcoplasm in cluster5. The GO terms relevant to the metabolic process and respiratory chain were also enriched significantly in cluster5, which represented the down-expressed biological process after the infection of C. irritans.
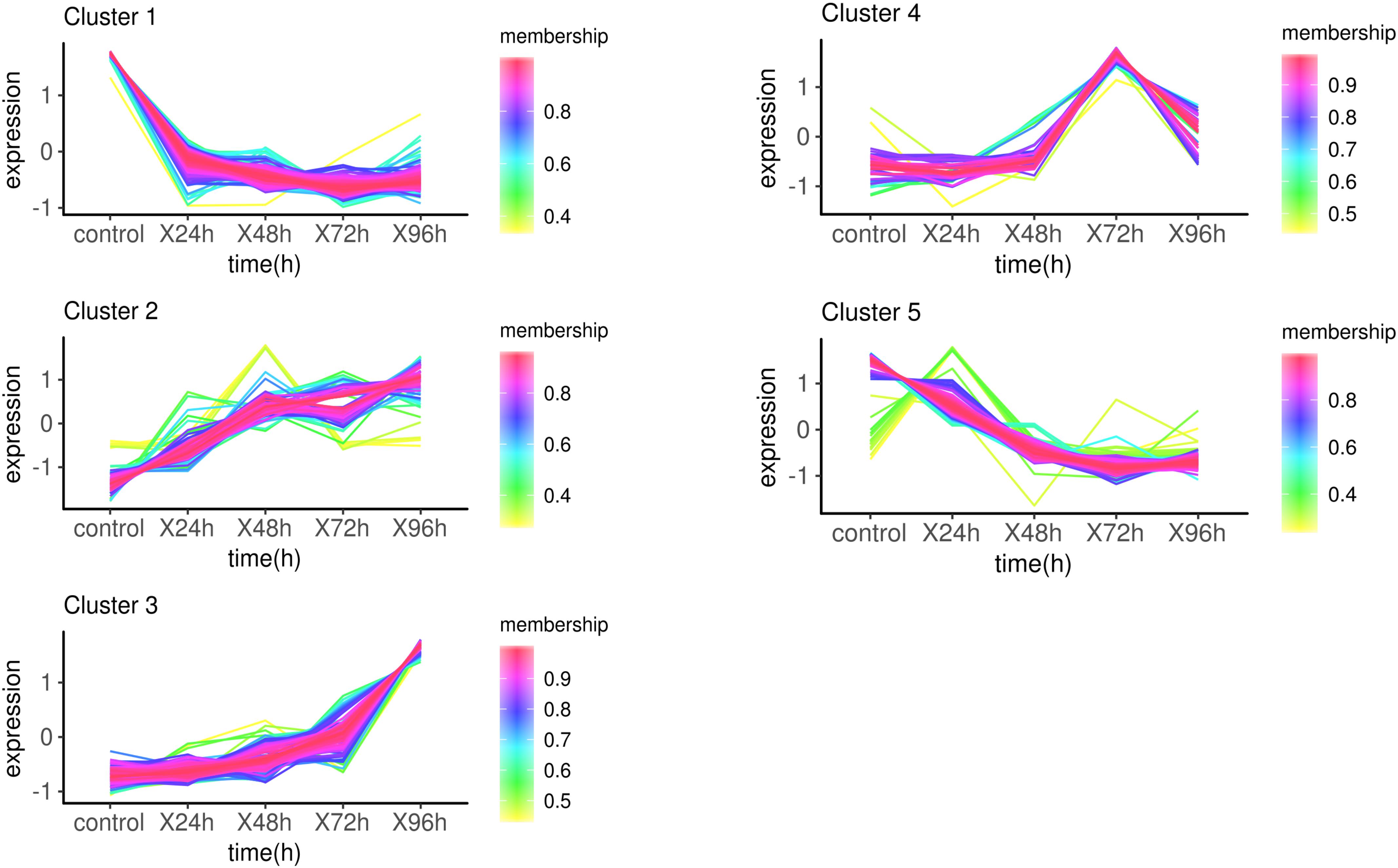
Figure 3. Expression patterns of target genes in infection analysis. Membership values indicated the degree that a transcript belonged to this cluster. The more degree, the more relevance.
With a cut-off of p-value < 0.05, there were 9 (cluster1), 7 (cluster2), 17 (cluster3), 9 (cluster4), and 33 (cluster5) KEGG pathway enriched in five groups respectively (Supplementary Table S7). Bacterial invasion of epithelial cells (ko05100) and Staphylococcus aureus infection (ko05150) were enriched in cluster1, which explained cell response to infection in the beginning. Complement and coagulation cascades (ko04610) and TGF-beta signaling pathway (ko04350) were only significantly enriched in cluster2. Cytokine-cytokine receptor interaction (ko04060) was enriched both in cluster2 and cluster3. There are several disease-related pathways that were significantly enriched in cluster3, such as amoebiasis (ko05146), transcriptional misregulation in cancers (ko05202), microRNAs in cancer (ko05206), and transcription misregulation in cancer (Supplementary Figure S4). Regulation of lipolysis in adipocyte (ko04923) and biosynthesis of amino acids (ko01230) were enriched in cluster4. Some metabolic pathways, like metabolic pathways (ko01100) and fatty acid metabolism (ko01212), were significantly enriched in cluster5.
Hub Genes and Interaction Networks Between DElncRNAs and Target Genes
A total of 466 target genes were annotated by using STRING database to predict interactions between proteins. PPI analysis was performed for each of the target clusters (Supplementary Figure S5). With the help of the PPI network, we can calculate the interaction of genes by degree method in the same cluster. Finally, a total of 21 genes ranked in the top 10% were selected as the hub genes (Table 3). The number of genes interacting with hub genes ranged from 22 (cluster4) to 58 (cluster1). Syne2 was identified as the top hub gene in cluster1. Notably, nine hub genes identified in the up-regulated cluster contained six genes related to cancer, immune cell, and immune response, including Bcl3, Rhog of cluster2, and CD2AP, RAB25, Tlr5, ZBTB7B of cluster3. Pbk involved in the activation of lymphoid cells was the only hub gene indicated in cluster4. In the hub genes of cluster5, Gp5 can encode protein that is essential for viral DNA ejection into the host cell, which may cause early viral infection in fish.
An integrated lncRNA-target genes interaction network included PPI network and co-expressed lncRNA-target genes was merged, which comprised 59 lncRNAs and 466 target genes, including 8057 interactions (Supplementary Figure S6). Except for TCONS_00002999 and PPL, all DElncRNAs and target genes merged as a complex interaction network.
Transcription Factor Binding Sites of DElncRNAs and Target Genes
It is reported that genes with strongly correlated mRNA expression profiles are more likely to have their promoter regions bound by a common transcription factor (Allocco et al., 2004). To predict TFBS in the promotor regions of DElncRNAs and target genes, 500 nts upstream of sequences were scanned. According to AnimalTFDB database, the number of potential TFBS for all DElncRNAs and target genes was 294 and 595, respectively. There were 282 transcription factors that may both bind to DElncRNAs and target genes. Indeed, among the 1,204 high-confidence pairs of DElncRNAs and target genes, 504 pairs had at least one common transcription factor, and the average number was 3.20. Over 50% (264/504) of these pairs had at least one common immune-related transcription factor. The result may infer that the co-expressed genes were likely controlled by similar regulatory mechanisms and were regulated by the same transcription factors.
Validation of DElncRNAs Using Quantitative Real-Time PCR
To validate the transcriptional expression, 4 DElncRNAs (TCONS_00012911, TCONS_00105595, TCONS_00047797, TCONS_00068543) were selected to perform real-time PCR in this study. The qRT-PCR results correlated well with the results obtained through RNA-seq (Supplementary Figure S7).
Discussion
Identification of lncRNAs in L. crocea
The ENCODE project showed that only 1–2% of the human genome encode for protein and the numerous non-coding RNAs include tRNAs, rRNAs, micro RNAs, lncRNAs, and other non-coding RNAs. With the development of RNA sequencing technologies, improved epigenomic techniques, and computational prediction methods, an increased focus was motivated to understand the roles of non-coding RNAs in biology. As an indispensable part of the genome, lncRNAs engage in diverse biological processes across every branch of life and required more analyses (Quinn and Chang, 2016). However, low conservation levels between taxa make the functional annotation difficult and block further understanding. A comparison of the lincRNAs in zebrafish, human, and mouse revealed that only 29 lincRNAs were conserved between fish and mammals (Hezroni et al., 2015). Therefore, further works call for a unique catalog of lncRNAs based on a large number of datasets for specific fish. Analysis of lncRNAs has been performed on other species in teleost. Al-Tobasei et al. (2016) analyzed more than two billion sequencing reads from four independent datasets and identified 31,195 lncRNAs of rainbow trout. Li et al. (2018) collected 103 RNAseq datasets to identify 72,276 high-confidence lncRNAs in tilapia. As an important economic aquaculture fish, no systematic analysis of lncRNAs has been conducted for L. crocea. In this study, a total of 14,599 lncRNAs were identified from 101 RNAseq datasets with a strict pipeline and divided into four classes based on the position relationship with protein-coding genes. In accordance with the previous study, lncRNAs identified in this study have some special characters compared to mRNAs like fewer exons, shorter transcript lengths, and low conversation levels (Al-Tobasei et al., 2016; Bakhtiarizadeh and Salami, 2019).
lncRNAs Play Important Roles in the Immune Response
Innate immune reactions contribute to the fundamental defense strategy of fish in response to various infection agents. It’s crucial to recognize the danger and activate subsequent signaling cascades. Previous studies have demonstrated that lncRNAs have a profound impact on the immune response in teleost (Jiang et al., 2016; Valenzuela-Munoz et al., 2018). We focused on the infection analysis of C. irritans and identified a lot of DElncRNAs with immune-related target genes. One of the important discoveries was the identification of TLRs families.
The family of toll-like receptors (TLRs) have been regarded as key partners in recognition of specific pathogen-associated molecular patterns (PAMPs) and activating signal pathways related to inflammatory response. TLR signaling through PAMPs recognition divided into myeloid differentiation primary response protein 88 (MyD88)-dependent and -independent signaling pathway (Li et al., 2012). Numerous studies revealed that fish TLRs have high structural similarity to the mammalian TLR system and might also be similar in the regulation of immune response (Rebl et al., 2010; Palti, 2011). In recent years, 20 TLRs, including a group of fish-specific TLRs, were identified (Zhang et al., 2013). And several studies have proved the immune-related roles of TLRs under infectious stimulation in teleost including zebrafish, fugu (Meijer et al., 2004), and orange-spotted grouper (Epinephelus coioides) (Li et al., 2012). As a significant member of TLRs, TLR5 has been shown to recognize the flagellin protein component of bacterial flagella and be responsible for flagellin-mediated NF-κB activation (Hayashi et al., 2001). Previous works also identified TLR5 in bony fish, such as zebrafish, fugu (Meijer et al., 2004), catfish (Pangasianodon hypophthalmus) (Schmitz et al., 2017), Japanese flounder (Paralichthys olivaceus) (Hwang et al., 2010), and rainbow trout (Onchorhynchus mikiss) (Tsujita et al., 2004). In this study, toll-like receptor 5 signaling pathway and MyD88-dependent toll-like receptor signaling pathway were significantly enriched in up-regulated cluster, and TLR5 is the hub genes of cluster3. With the remote position and high correlation, we speculated that lncRNA TCONS_00012911 might positively regulate TLR5 through trans way (Figure 4). Indeed, they had seven common transcription factors binding sites including five immune-related ones in the promoter region. In addition, as an important part of Toll/interleukin-1 (Il-1) receptor domain (TIR domain), IL1R2 was also indicated in cluster3. It’s well known that TIR domain was a protein–protein interaction domain that occurs in a large group of host defense associated proteins from diverse species (Meijer et al., 2004). Therefore, we speculated that the expressions of TLR5 and IL1R2 transcripts were increased under the infection of C. irritans and might be crucial for the innate immune response.
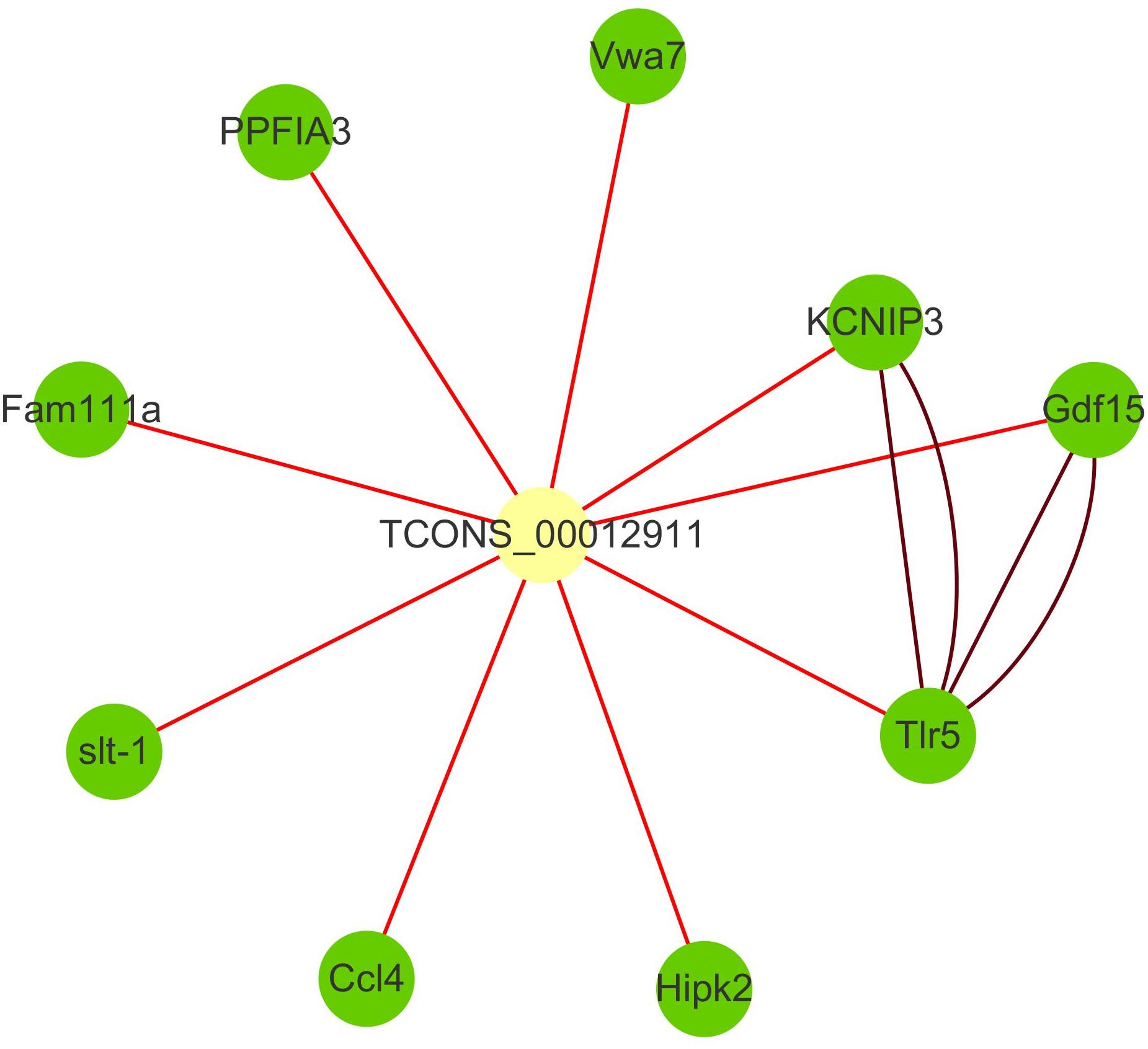
Figure 4. Interaction network of lncRNA TCONS_00012911 with putative target genes. Yellow square nodes: lncRNA TCONS_00012911; green nodes: target genes; brown lines: interactions between genes based on STRING database; red lines: positive interactions between lncRNAs and genes (Pearson’s correlation coefficient > 0.99).
Besides TLR families, other immune-related genes were identified in target genes. In cluster3, which can represent the up-regulated genes, the other immune-related enriched GO terms can be divided into three types, including the cytokine secretion (e.g., GO:0050663, GO:0050707, GO:0001819), the innate immune response (e.g., GO:0002218, GO:0002758, GO:0045088), and the defense response (e.g., GO:0031349, GO:0042742, GO:0031347). KEGG pathway also discovered several immune-related pathways, including ECM-receptor interaction (ko04512) and microRNAs in cancer (ko05206) in cluster3. The ECM functions as ligands for cell surface receptors such as integrins, dystroglycans, toll-like receptors (TLRs), and regulate cellular signaling and immune cell dynamics (Bhattacharjee et al., 2019). MicroRNAs in cancer pathway are not only indicating the function lncRNAs played in immune response but also emphasizing the interaction between microRNAs and lncRNAs. A lot of immune-related genes are involved in the enriched GO terms and pathways, including TLR5, CD2AP, and MMP9. CD2 associated protein (CD2AP) is an adaptor protein that couples endocytic proteins to the actin cytoskeleton. It can improve the protective antibody response in viral infection by tuning TCR signaling (Raju et al., 2018). Members of the matrix metalloproteinase (MMP) family are essential for the remodeling of the extracellular matrix in a number of biological processes, including a variety of immune responses. MMP9 is highly expressed in specific myeloid cell populations in which it plays a role in the innate immune response and was identified in zebrafish (Yoong et al., 2007).
In cluster2, genes that showed a continually increased pattern were collected. GO enrichment analysis showed that ion channel activity terms (e.g., GO:0005247, GO:0008308, GO:0005254) were significantly enriched. Ion channels and transporters help to establish and control cell hydrophobic lipid membranes. Analysis has revealed important roles of ionic signals in lymphocyte development and innate and adaptive immune responses (Feske et al., 2015). KEGG pathway analysis showed that the complement and coagulation cascades pathway (ko04610), cytokine-cytokine receptor interaction pathway (ko04060), and disease-related pathways (ko05020, ko05322) were significantly enriched in cluster2. Coagulation and complement are two distinct systems with unique pathophysiological roles, and both serve as innate defense against external threats (Oikonomopoulou et al., 2012). Cytokine-cytokine receptor interaction pathway plays important roles in immune response and has been identified in several previous studies of teleost under bacterial or virus infection (Peng et al., 2019; Zhang et al., 2019). In cluster1, genes highly expressed in 0 h were collected. GO enrichment showed terms related to ATP generation (e.g., GO:0006757, GO:0046031) and nucleoside diphosphate metabolic process (e.g., GO:0009135, GO:0009179, GO:0006165) were enriched. It’s well known that ATP is essential to energy support, and the decrease of ATP may be related to poor energy generation and induce the mortalities of fish in the experiment group. These results suggest that lncRNAs regulate the targeted genes and play important roles in immune response to the infection of C. irritans in L. crocea.
The Transcriptional Regulation Roles of lncRNAs in Target Genes
It’s well known that lncRNAs can regulate genes in cis or trans ways, but the mechanism underlying the transcriptional regulation has not been understood (Kopp and Mendell, 2018). Previous studies suggested that the co-expressed genes were likely regulated by the same transcription factors (Allocco et al., 2004; You et al., 2019). In this work, over 40% of high-confidence pairs had at least one common transcription factor. The similarity of DElncRNAs and target genes in transcription factor may suggest a potential function of lncRNAs to regulate target genes. For example, according to the remote position and similar expression patterns, TCONS_00040064 might positively regulate MMP9 through trans way in this study. And they have four common transcriptional factor binding sites in the promoter region, which may explain the regulation of TCONS_00040064 for MMP9.
The regulation and expression of genes are intricate and are affected by complicated factors. In this study, an integrated network comprised of 59 lncRNAs and 481 target genes, including 8057 interactions, was merged and visualized the complicated relationship between lncRNAs and protein-coding genes that differentially expressed infected by C. irritans. The further studies and experiments about interactions of lncRNA, miRNA, and protein-coding RNA are required to understand the regulatory process in immune and other biological processes.
Conclusion
To generate a comprehensive transcriptome of L. crocea, 101 RNAseq datasets were used, and a total of 14,599 lncRNAs were identified through a strict pipeline. DE analysis identified 77 DElncRNAs in response to the infection of C. irritans, and 567 differentially expressed protein-coding genes were regarded as target genes. A set of immune-related GO terms and KEGG pathways were enriched, in which immune genes including TLR5, MMP9, CD2AP, and IL1R2 were up-regulated significantly. This study provides a new insight for lncRNAs annotation in L. crocea genome and helps to deepen the understanding of the roles of lncRNAs in innate immune response to C. irritans.
Data Availability Statement
All datasets generated for this study are included in the article/Supplementary Material.
Author Contributions
PX and WZ conceived and supervised the study. XZ, TZ, and PX designed and managed the experiments, and wrote the manuscript. XZ, BC, HB, and YB performed the analysis and designed the charts and tables. XZ, YB, JZ, FP, YW, LC, YS, QK, and JC conducted the experiments. All authors have read and approved the manuscript.
Funding
This study was supported by the Industry-University Collaboration Project of Fujian Province (No. 2019N5001), the Independent Research Project for State Key Laboratory of Large Yellow Croaker Breeding (No. LYC2017ZY01), the Open Research Fund Project of State Key Laboratory of Large Yellow Croaker Breeding (Nos. LYC2019RS02 and LYC2019RS03), the Science and Technology Platform Construction of Fujian Province (No. 2018N2005), the Local Science and Technology Development Project Guide by the Central Government (Nos. 2017L3019 and 2019L3032), the National Key Research and Development Program of China (No. 2018YFC1406305), China Agriculture Research System (No. CARS-47), the Foreign Cooperation Project of Fujian Province (No. 2019I1008), the Fundamental Research Funds for the Central Universities (Nos. 20720180123 and 20720160110), the Principal Fund for Xiamen University (No. 20720200110), the Student Innovation and Entrepreneurship Training Program for Xiamen University (No. 2020X0584), and the Project of Industry-College-Institute Cooperation between Ningde City and Xiamen University (Nos. 2018C003 and 2019C002).
Conflict of Interest
QK, WZ, JC, and PX were employed by the State Key Laboratory of Large Yellow Croaker Breeding, which was constructed by the Ningde Fufa Fisheries Company Limited.
The remaining authors declare that the research was conducted in the absence of any commercial or financial relationships that could be construed as a potential conflict of interest.
Acknowledgments
We thank the Ningde Fufa Fisheries Company Limited (Ningde, China) for the help of fish husbandry.
Supplementary Material
The Supplementary Material for this article can be found online at: https://www.frontiersin.org/articles/10.3389/fgene.2020.590475/full#supplementary-material
Supplementary Figure 1 | The results of BUSCO softwares.
Supplementary Figure 2 | Expression patterns of DElncRNAs in infection analysis. Membership values indicated the degree that a transcript belonged to this cluster. The more degree, the more relevance.
Supplementary Figure 3 | The top 20 GO terms enriched in each clusters.
Supplementary Figure 4 | Significantly differentially expressed genes identified in diverse KEGG pathways in cluster3. Red boxes indicate significantly differential expression and black boxes indicate unchanged expression. (A) Cytokine-cytokine receptor interaction pathway. (B) ECM-receptor interaction pathway. (C) MicroRNAs in cancer pathway. (D) Transcription misregulation in cancer pathway.
Supplementary Figure 5 | The PPI networks of target genes. Nodes represent target genes. The bigger node and the darker color mean the higher degree value. Line represent interactions between two genes. The wider line and darker color mean the closer interaction.
Supplementary Figure 6 | Integrated lncRNA-target genes interaction network constructed based on co-expressed genes and STRING-derived PPI. Yellow square nodes: DElncRNAs; green nodes: target genes; brown lines: interactions between genes; red lines: positive interactions between lncRNAs and genes (Pearson’s correlation coefficient > 0.99); blue lines: negative interactions between lncRNAs and genes (Pearson’s correlation coefficient < −0.99). The Pearson’s coefficient or interaction value (based on STRING database) of each pairwise was shown in Supplementary Tables 4, 8, respectively.
Supplementary Figure 7 | Validation of RNA-seq data by qRT-PCR. Orange bar: RT-PCR; green bar: RNA-seq. (A) TCONS_00012911. (B) TCONS_00105595. (C) TCONS_00047797. (D) TCONS_00068543.
Supplementary Table 1 | Data collection.
Supplementary Table 2 | Primer sets for qRT-PCR.
Supplementary Table 3 | The mapping ratio for samples.
Supplementary Table 4 | Interaction between DElncRNAs and target genes.
Supplementary Table 5 | Target genes identified by the InnateDB as immune genes.
Supplementary Table 6 | The top 20 significantly enriched GO term of target genes.
Supplementary Table 7 | The significantly enriched KEGG pathways of target genes.
Supplementary Table 8 | Interaction between target genes.
Footnotes
- ^ http://www.noncode.org/
- ^ https://www.gencodegenes.org/
- ^ https://www.ncbi.nlm.nih.gov/
- ^ http://pfam.xfam.org/
- ^ http://rfam.xfam.org/
- ^ https://www.uniprot.org/
- ^ http://www.mirbase.org/
- ^ https://blast.ncbi.nlm.nih.gov/Blast.cgi
- ^ https://indra.mullins.microbiol.washington.edu/sms2/orf_find.html
- ^ https://www.innatedb.ca/
- ^ https://www.omicshare.com/tools/Home?l=en-us
- ^ http://bioinfo.life.hust.edu.cn/AnimalTFDB/
- ^ https://www.genscript.com/tools/real-time-pcr-taqman-primer-design-tool
References
Ali, A., Al-Tobasei, R., Kenney, B., Leeds, T. D., and Salem, M. (2018). Integrated analysis of IncRNA and mRNA expression in rainbow trout families showing variation in muscle growth and fillet quality traits. Sci. Rep. 8:12111. doi: 10.1038/s41598-018-30655-8
Allocco, D. J., Kohane, I. S., and Butte, A. J. (2004). Quantifying the relationship between co-expression, co-regulation and gene function. BMC Bioinformatics 5:18. doi: 10.1186/1471-2105-5-18
Al-Tobasei, R., Paneru, B., and Salem, M. (2016). Genome-wide discovery of long non-coding RNAs in rainbow trout. PLoS One 11:e0148940. doi: 10.1371/journal.pone.0148940
Bai, H., Zhou, T., Zhao, J., Chen, B., Pu, F., Bai, Y., et al. (2020). Transcriptome analysis reveals the temporal gene expression patterns in skin of large yellow croaker (Larimichthys crocea) in response to Cryptocaryon irritans infection. Fish Shellfish Immunol. 99, 462–472. doi: 10.1016/j.fsi.2020.02.024
Bakhtiarizadeh, M. R., and Salami, S. A. (2019). Identification and expression analysis of long noncoding RNAs in fat-tail of sheep breeds. G3 Genes Genomes Genetics 9, 1263–1276. doi: 10.1534/g3.118.201014
Bhattacharjee, O., Ayyangar, U., Kurbet, A. S., Ashok, D., and Raghavan, S. (2019). Unraveling the ECM-immune cell crosstalk in skin diseases. Front. Cell Dev. Biol. 7:68. doi: 10.3389/fcell.2019.00068
Bolger, A. M., Lohse, M., and Usadel, B. (2014). Trimmomatic: a flexible trimmer for Illumina sequence data. Bioinformatics 30, 2114–2120. doi: 10.1093/bioinformatics/btu170
Buchmann, K. (2015). Impact and control of protozoan parasites in maricultured fishes. Parasitology 142, 168–177. doi: 10.1017/s003118201300005x
Carmona, S., Lin, B., Chou, T., Arroyo, K., and Sun, S. (2018). LncRNA Jpx induces Xist expression in mice using both trans and cis mechanisms. PLoS Genet. 14:e1007378. doi: 10.1371/journal.pgen.1007378
Carpenter, S., Aiello, D., Atianand, M. K., Ricci, E. P., Gandhi, P., Hall, L. L., et al. (2013). A long noncoding RNA mediates both activation and repression of immune response genes. Science 341, 789–792. doi: 10.1126/science.1240925
Chen, B., Zhou, Z., Ke, Q., Wu, Y., Bai, H., Pu, F., et al. (2019). The sequencing and de novo assembly of the Larimichthys crocea genome using PacBio and Hi-C technologies. Sci. Data 6:188. doi: 10.1038/s41597-019-0194-3
Chen, C.-K., Blanco, M., Jackson, C., Aznauryan, E., Ollikainen, N., Surka, C., et al. (2016). Xist recruits the X chromosome to the nuclear lamina to enable chromosome-wide silencing. Science 354, 468–472. doi: 10.1126/science.aae0047
Chen, X. H., Lin, K. B., and Wang, X. W. (2003). Outbreaks of an iridovirus disease in maricultured large yellow croaker, Larimichthys crocea (Richardson), in China. J. Fish Dis. 26, 615–619. doi: 10.1046/j.1365-2761.2003.00494.x
Chen, Y. G., Satpathy, A. T., and Chang, H. Y. (2017). Gene regulation in the immune system by long noncoding RNAs. Nat. Immunol. 18, 962–972. doi: 10.1038/ni.3771
Dettleff, P., Hormazabal, E., Aedo, J., Fuentes, M., Meneses, C., Molina, A., et al. (2020). Identification and evaluation of long noncoding RNAs in response to handling stress in red Cusk-Eel (Genypterus chilensis) via RNA-seq. Mar. Biotechnol. 22, 94–108. doi: 10.1007/s10126-019-09934-6
Fan, Q., Yang, L., Zhang, X., Peng, X., Wei, S., Su, D., et al. (2018). The emerging role of exosome-derived non-coding RNAs in cancer biology. Cancer Lett. 414, 107–115. doi: 10.1016/j.canlet.2017.10.040
Fang, S., Zhang, L., Guo, J., Niu, Y., Wu, Y., Li, H., et al. (2018). NONCODEV5: a comprehensive annotation database for long non-coding RNAs. Nucleic Acids Res. 46, D308–D314. doi: 10.1093/nar/gkx1107
Fatica, A., and Bozzoni, I. (2014). Long non-coding RNAs: new players in cell differentiation and development. Nat. Rev. Genet. 15, 7–21. doi: 10.1038/nrg3606
Feske, S., Wulff, H., and Skolnik, E. Y. (2015). Ion channels in innate and adaptive immunity. Annu. Rev. Immunol. 33, 291–353. doi: 10.1146/annurev-immunol-032414-112212
Hayashi, F., Smith, K. D., Ozinsky, A., Hawn, T. R., Yi, E. C., Goodlett, D. R., et al. (2001). The innate immune response to bacterial flagellin is mediated by Toll-like receptor 5. Nature 410, 1099–1103. doi: 10.1038/35074106
Hezroni, H., Koppstein, D., Schwartz, M. G., Avrutin, A., Bartel, D. P., and Ulitsky, I. (2015). Principles of long noncoding RNA evolution derived from direct comparison of transcriptomes in 17 species. Cell Reports 11, 1110–1122. doi: 10.1016/j.celrep.2015.04.023
Hung, T., Wang, Y., Lin, M. F., Koegel, A. K., Kotake, Y., Grant, G. D., et al. (2011). Extensive and coordinated transcription of noncoding RNAs within cell-cycle promoters. Nat. Genet. 43, 621–629. doi: 10.1038/ng.848
Hwang, S. D., Asahi, T., Kondo, H., Hirono, I., and Aoki, T. (2010). Molecular cloning and expression study on Toll-like receptor 5 paralogs in Japanese flounder, Paralichthys olivaceus. Fish Shellfish Immunol. 29, 630–638. doi: 10.1016/j.fsi.2010.06.011
Iyer, M. K., Niknafs, Y. S., Malik, R., Singhal, U., Sahu, A., Hosono, Y., et al. (2015). The landscape of long noncoding RNAs in the human transcriptome. Nat. Genet. 47, 199–208. doi: 10.1038/ng.3192
Jiang, L. H., Liu, W., Zhu, A. Y., Zhang, J. S., Zhou, J. J., and Wu, C. W. (2016). Transcriptome analysis demonstrate widespread differential expression of long noncoding RNAs involve in Larimichthys crocea immune response. Fish Shellfish Immunol. 51, 1–8. doi: 10.1016/j.fsi.2016.02.001
Josset, L., Tchitchek, N., Gralinski, L. E., Ferris, M. T., Eisfeld, A. J., Green, R. R., et al. (2014). Annotation of long non-coding RNAs expressed in collaborative cross founder mice in response to respiratory virus infection reveals a new class of interferon-stimulated transcripts. RNA Biol. 11, 875–890. doi: 10.4161/rna.29442
Kang, Y.-J., Yang, D.-C., Kong, L., Hou, M., Meng, Y.-Q., Wei, L., et al. (2017). CPC2: a fast and accurate coding potential calculator based on sequence intrinsic features. Nucleic Acids Res. 45, W12–W16.
Kim, D., Langmead, B., and Salzberg, S. L. (2015). HISAT: a fast spliced aligner with low memory requirements. Nature Methods 12, 357–360. doi: 10.1038/nmeth.3317
Kong, S. N., Ke, Q. Z., Chen, L., Zhou, Z. X., Pu, F., Zhao, J., et al. (2019). Constructing a high-density genetic linkage map for large yellow croaker (Larimichthys crocea) and mapping resistance trait against ciliate parasite Cryptocaryon irritans. Mar. Biotechnol. 21, 262–275. doi: 10.1007/s10126-019-09878-x
Kopp, F., and Mendell, J. T. (2018). Functional classification and experimental dissection of long noncoding RNAs. Cell 172, 393–407. doi: 10.1016/j.cell.2018.01.011
Kung, J. T. Y., Colognori, D., and Lee, J. T. (2013). Long noncoding RNAs: past, present, and future. Genetics 193, 651–669. doi: 10.1534/genetics.112.146704
Lee, J. T. (2012). Epigenetic regulation by long noncoding RNAs. Science 338, 1435–1439. doi: 10.1126/science.1231776
Li, A., Zhang, J., and Zhou, Z. (2014). PLEK: a tool for predicting long non-coding RNAs and messenger RNAs based on an improved k-mer scheme. BMC Bioinformatics 15:311. doi: 10.1186/1471-2105-15-311
Li, B. J., Jiang, D. L., Meng, Z. N., Zhang, Y., Zhu, Z. X., Lin, H. R., et al. (2018). Genome-wide identification and differentially expression analysis of lncRNAs in tilapia. BMC Genomics 19:729. doi: 10.1186/s12864-018-5115-x
Li, Y.-W., Luo, X.-C., Dan, X.-M., Qiao, W., Huang, X.-Z., and Li, A.-X. (2012). Molecular cloning of orange-spotted grouper (Epinephelus coioides) TLR21 and expression analysis post Cryptocaryon irritans infection. Fish Shellfish Immunol. 32, 476–481. doi: 10.1016/j.fsi.2011.11.021
Liu, X., Li, W., Jiang, L., Lu, Z., Liu, M., Gong, L., et al. (2019). Immunity-associated long non-coding RNA and expression in response to bacterial infection in large yellow croaker (Larimichthys crocea). Fish Shellfish Immunol. 94, 634–642. doi: 10.1016/j.fsi.2019.09.015
Liu, Y., Qi, B., Xie, J., Wu, X., Ling, Y., Cao, X., et al. (2018). Filtered reproductive long non-coding RNAs by genome-wide analyses of goat ovary at different estrus periods. BMC Genomics 19:866. doi: 10.1186/s12864-018-5268-7
Livak, K. J., and Schmittgen, T. D. (2001). Analysis of relative gene expression data using real-time quantitative PCR and the 2(T)(-Delta Delta C) method. Methods 25, 402–408. doi: 10.1006/meth.2001.1262
Madeira, F., Park, Y. M., Lee, J., Buso, N., Gur, T., Madhusoodanan, N., et al. (2019). The EMBL-EBI search and sequence analysis tools APIs in 2019. Nucleic Acids Res. 47, W636–W641. doi: 10.1093/nar/gkz268
Meijer, A. H., Krens, S. F. G., Rodriguez, I. A. M., He, S. N., Bitter, W., Snaar-Jagalska, B. E., et al. (2004). Expression analysis of the Toll-like receptor and TIR domain adaptor families of zebrafish. Mol. Immunol. 40, 773–783. doi: 10.1016/j.molimm.2003.10.003
Nawrocki, E. P., and Eddy, S. R. (2013). Infernal 1.1: 100-fold faster RNA homology searches. Bioinformatics 29, 2933–2935. doi: 10.1093/bioinformatics/btt509
Oikonomopoulou, K., Ricklin, D., Ward, P. A., and Lambris, J. D. (2012). Interactions between coagulation and complement-their role in inflammation. Semin. Immunopathol. 34, 151–165. doi: 10.1007/s00281-011-0280-x
Palti, Y. (2011). Toll-like receptors in bony fish: from genomics to function. Dev. Comp. Immunol. 35, 1263–1272. doi: 10.1016/j.dci.2011.03.006
Pauli, A., Valen, E., Lin, M. F., Garber, M., Vastenhouw, N. L., Levin, J. Z., et al. (2012). Systematic identification of long noncoding RNAs expressed during zebrafish embryogenesis. Genome Res. 22, 577–591. doi: 10.1101/gr.133009.111
Peng, H., Yang, B., Li, B., Cai, Z., Cui, Q., Chen, M., et al. (2019). Comparative transcriptomic analysis reveals the gene expression profiles in the liver and spleen of Japanese pufferfish (Takifugu rubripes) in response to Vibrio harveyi infection. Fish Shellfish Immunol. 90, 308–316. doi: 10.1016/j.fsi.2019.04.304
Quinn, J. J., and Chang, H. Y. (2016). Unique features of long non-coding RNA biogenesis and function. Nat. Rev. Genet. 17, 47–62. doi: 10.1038/nrg.2015.10
Raju, S., Kometani, K., Kurosaki, T., Shaw, A. S., and Egawa, T. (2018). The adaptor molecule CD2AP in CD4 T cells modulates differentiation of follicular helper T cells during chronic LCMV infection. PLoS Pathog. 14:e1007053. doi: 10.1371/journal.ppat.1007053
Rebl, A., Goldammer, T., and Seyfert, H.-M. (2010). Toll-like receptor signaling in bony fish. Veterinary Immunol. Immunopathol. 134, 139–150. doi: 10.1016/j.vetimm.2009.09.021
Rinn, J. L., and Chang, H. Y. (2012). Genome regulation by long noncoding RNAs. Annu. Rev. Immunol. 81, 145–166. doi: 10.1146/annurev-biochem-051410-092902
Schmitt, A. M., and Chang, H. Y. (2016). Long noncoding RNAs in cancer pathways. Cancer Cell 29, 452–463. doi: 10.1016/j.ccell.2016.03.010
Schmitz, M., Baekelandt, S., Bequet, S., and Kestemont, P. (2017). Chronic hyperosmotic stress inhibits renal Toll-Like Receptors expression in striped catfish (Pangasianodon hypophthalmus, Sauvage) exposed or not to bacterial infection. Dev. Comp. Immunol. 73, 139–143. doi: 10.1016/j.dci.2017.03.020
Simao, F. A., Waterhouse, R. M., Ioannidis, P., Kriventseva, E. V., and Zdobnov, E. M. (2015). BUSCO: assessing genome assembly and annotation completeness with single-copy orthologs. Bioinformatics 31, 3210–3212. doi: 10.1093/bioinformatics/btv351
Song, F., Wang, L., Zhu, W., and Dong, Z. (2019). Long noncoding RNA and mRNA expression profiles following igf3 knockdown in common carp, Cyprinus carpio. Sci. Data 6:190024.
Sun, L., Luo, H., Bu, D., Zhao, G., Yu, K., Zhang, C., et al. (2013). Utilizing sequence intrinsic composition to classify protein-coding and long non-coding transcripts. Nucleic Acids Res. 41:e166. doi: 10.1093/nar/gkt646
Sun, W., and Feng, J. (2018). Differential lncRNA expression profiles reveal the potential roles of lncRNAs in antiviral immune response of Crassostrea gigas. Fish Shellfish Immunol. 81, 233–241. doi: 10.1016/j.fsi.2018.07.032
Szklarczyk, D., Morris, J. H., Cook, H., Kuhn, M., Wyder, S., Simonovic, M., et al. (2017). The STRING database in 2017: quality-controlled protein-protein association networks, made broadly accessible. Nucleic Acids Res. 45, D362–D368. doi: 10.1093/nar/gkw937
Tsujita, T., Tsukada, H., Nakao, M., Oshiumi, H., Matsumoto, M., and Seya, T. (2004). Sensing bacterial flagellin by membrane and soluble orthologs of toll-like receptor 5 in rainbow trout (Onchorhynchus mikiss). J. Biol. Chem. 279, 48588–48597. doi: 10.1074/jbc.m407634200
Valenzuela-Munoz, V., Pereiro, P., Alvarez-Rodriguez, M., Gallardo-Escarate, C., Figueras, A., and Novoa, B. (2019). Comparative modulation of lncRNAs in wild-type and rag1-heterozygous mutant zebrafish exposed to immune challenge with spring viraemia of carp virus (SVCV). Sci. Rep. 9, 1–13. doi: 10.1038/s41598-019-50766-0
Valenzuela-Munoz, V., Valenzuela-Miranda, D., and Gallardo-Escarate, C. (2018). Comparative analysis of long non-coding RNAs in Atlantic and Coho salmon reveals divergent transcriptome responses associated with immunity and tissue repair during sea lice infestation. Dev. Comp. Immunol. 87, 36–50. doi: 10.1016/j.dci.2018.05.016
Wang, P., Wang, J., Su, Y.-Q., Mao, Y., Zhang, J.-S., Wu, C. W., et al. (2016). Transcriptome analysis of the Larimichthys crocea liver in response to Cryptocaryon irritans. Fish Shellfish Immunol. 48, 1–11. doi: 10.1016/j.fsi.2015.11.011
Wu, Y., Cheng, T., Liu, C., Liu, D., Zhang, Q., Long, R., et al. (2016). Systematic identification and characterization of long non-coding RNAs in the silkworm, Bombyx mori. PLoS One 11:e0147147. doi: 10.1371/journal.pone.0147147
Yoong, S., O’Connell, B., SoaneS, A., Crowhurst, M. O., Lieschke, G. J., and Ward, A. C. (2007). Characterization of the zebrafish matrix metalloproteinase 9 gene and its developmental expression pattern. Gene Exp. Patterns 7, 39–46. doi: 10.1016/j.modgep.2006.05.005
You, Z., Zhang, Q., Liu, C., Song, J., Yang, N., and Lian, L. (2019). Integrated analysis of lncRNA and mRNA repertoires in Marek’s disease infected spleens identifies genes relevant to resistance. BMC Genomics 20:245. doi: 10.1186/s12864-019-5625-1
Zhang, J., Liu, S., Rajendran, K. V., Sun, L., Zhang, Y., Sun, F., et al. (2013). Pathogen recognition receptors in channel catfish: III Phylogeny and expression analysis of Toll-like receptors. Dev. Comp. Immunol. 40, 185–194. doi: 10.1016/j.dci.2013.01.009
Zhang, L., Wang, C., Liu, H., and Fu, P. (2019). The important role of phagocytosis and interleukins for nile tilapia (Oreochromis niloticus) to defense infection of Aeromonas hydrophila based on transcriptome analysis. Fish Shellfish Immunol. 92, 54–63. doi: 10.1016/j.fsi.2019.05.041
Zheng, L.-B., Mao, Y., Wang, J., Chen, R.-N., Su, Y.-Q., Hong, Y.-Q., et al. (2018). Excavating differentially expressed antimicrobial peptides from transcriptome of Larimichthys crocea liver in response to Cryptocaryon irritans. Fish Shellfish Immunol. 75, 109–114. doi: 10.1016/j.fsi.2018.01.028
Zuo, R., Ai, Q., Mai, K., Xu, W., Wang, J., Xu, H., et al. (2012). Effects of dietary n-3 highly unsaturated fatty acids on growth, nonspecific immunity, expression of some immune related genes and disease resistance of large yellow croaker (Larmichthys crocea) following natural infestation of parasites (Cryptocaryon irritans). Fish Shellfish Immunol. 32, 249–258. doi: 10.1016/j.fsi.2011.11.005
Keywords: Larimichthys crocea, Cryptocaryon irritans, lncRNAs, infection, immune response
Citation: Zhang X, Zhou T, Chen B, Bai H, Bai Y, Zhao J, Pu F, Wu Y, Chen L, Shi Y, Ke Q, Zheng W, Chen J and Xu P (2020) Identification and Expression Analysis of Long Non-coding RNA in Large Yellow Croaker (Larimichthys crocea) in Response to Cryptocaryon irritans Infection. Front. Genet. 11:590475. doi: 10.3389/fgene.2020.590475
Received: 01 August 2020; Accepted: 18 September 2020;
Published: 12 November 2020.
Edited by:
Shikai Liu, Ocean University of China, ChinaReviewed by:
Jie Mei, Huazhong Agricultural University, ChinaJun Hong Xia, Sun Yat-sen University, China
Copyright © 2020 Zhang, Zhou, Chen, Bai, Bai, Zhao, Pu, Wu, Chen, Shi, Ke, Zheng, Chen and Xu. This is an open-access article distributed under the terms of the Creative Commons Attribution License (CC BY). The use, distribution or reproduction in other forums is permitted, provided the original author(s) and the copyright owner(s) are credited and that the original publication in this journal is cited, in accordance with accepted academic practice. No use, distribution or reproduction is permitted which does not comply with these terms.
*Correspondence: Peng Xu, xupeng77@xmu.edu.cn; xupeng77@gmail.com