- 1Department of Biostatistics, Epidemiology, and Scientific Computing, King Faisal Specialist Hospital and Research Centre, Riyadh, Saudi Arabia
- 2AlFaisal University, College of Medicine, Riyadh, Saudi Arabia
- 3Gulf Centre for Cancer Control and Prevention, King Faisal Special Hospital and Research Centre, Riyadh, Saudi Arabia
- 4Liver and Small Bowel Transplantation and Hepatobiliary-Pancreatic Surgery Department, King Faisal Specialist Hospital and Research Centre, Riyadh, Saudi Arabia
- 5Department of Surgery, University of Almaarefa, Riyadh, Saudi Arabia
- 6Translational Genomics Department, Center for Genomic Medicine, King Faisal Specialist Hospital and Research Centre, Riyadh, Saudi Arabia
Background: Hepatocellular carcinoma (HCC) is considered the most common type of liver cancer and the fourth leading cause of cancer-related deaths in the world. Since the disease is usually diagnosed at advanced stages, it has poor prognosis. Therefore, reliable biomarkers are urgently needed for early diagnosis and prognostic assessment.
Methods: We used genome-wide gene expression profiling datasets from human and rat early HCC (eHCC) samples to perform integrated genomic and network-based analyses, and discovered gene markers that are expressed in blood and conserved in both species. We then used independent gene expression profiling datasets for peripheral blood mononuclear cells (PBMCs) for eHCC patients and from The Cancer Genome Atlas (TCGA) database to estimate the diagnostic and prognostic performance of the identified gene signature. Furthermore, we performed functional enrichment, interaction networks and pathway analyses.
Results: We identified 41 significant genes that are expressed in blood and conserved across species in eHCC. We used comprehensive clinical data from over 600 patients with HCC to verify the diagnostic and prognostic value of 41-gene-signature. We developed a prognostic model and a risk score using the 41-geneset that showed that a high prognostic index is linked to a worse disease outcome. Furthermore, our 41-gene signature predicted disease outcome independently of other clinical factors in multivariate regression analysis. Our data reveals a number of cancer-related pathways and hub genes, including EIF4E, H2AFX, CREB1, GSK3B, TGFBR1, and CCNA2, that may be essential for eHCC progression and confirm our gene signature’s ability to detect the disease in its early stages in patients’ biological fluids instead of invasive procedures and its prognostic potential.
Conclusion: Our findings indicate that integrated cross-species genomic and network analysis may provide reliable markers that are associated with eHCC that may lead to better diagnosis, prognosis, and treatment options.
Introduction
Hepatocellular carcinoma (HCC) is a leading cause of cancer-related deaths worldwide (Bray et al., 2018; Yang et al., 2019a; Villanueva, 2019). The disease is mostly diagnosed at advanced stages, and therefore has poor prognosis. Liver biopsy is an invasive technique with potential difficulties, including risk of death, and is susceptible to sampling errors (Shi et al., 2014). HCC is usually diagnosed using serum alpha-fetoprotein (AFP) and ultrasound (Schütte et al., 2014; Tsuchiya et al., 2015) (Shi et al., 2014). Since 1964, the AFP biomarker has been most commonly used biomarker for HCC evaluation (Schütte et al., 2014; Tsuchiya et al., 2015). However, AFP has low sensitivity and specificity; therefore, the measurement of serum AFP levels has been discarded from updated international surveillance guidelines (Schütte et al., 2014). Hence, there is a significant need to identify alternative or additional biomarkers that can be used in effectively diagnosing patients in an early tumor stage.
The use of high-throughput technologies together with bioinformatics approaches in cancer research gives the ability to identify molecules implicated in complex pathways in carcinogenesis. Several recent studies have used whole-genome gene expression profiling, DNA methylation profiles, copy number variations (CNVs) in HCC in order to achieve a better understanding of the processes of hepatocarcinogenesis (Colak et al., 2010; Budhu et al., 2013; Shi et al., 2014; Allain et al., 2016; Wang et al., 2017; Guan et al., 2020; Li et al., 2020). It has been reported that combining genomic and network-based analysis could lead to reliable and accurate predictive biomarkers for human diseases (Colak et al., 2013; Al-Harazi et al., 2016). The molecular characteristics of HCC are heterogeneous which results in dissimilarities in the outcome of affected patients. Detecting the early stage tumors is essential as liver resection, transplantation or local ablation are the most effective treatment option for patients at early disease stages. However, HCC is usually diagnosed at advanced stages, and therefore, has poor prognosis (Waghray et al., 2015; Llovet et al., 2016). Some of the key factors that have an effect on patient survival include number and size of nodules, vascular invasion, existence of extrahepatic metastases, and liver function (Schütte et al., 2014). However, the survival of patients with the identified tumor stages still remains heterogeneous and some patients have early recurrence of disease after treatment or liver transplantation. Therefore, it is essential to have knowledge on high-risk profiles to guide personalized treatment.
The cross-species comparative genomic approach has been shown to be a powerful approach that may lead to key driver genes involved in tumor development, invasion, and progression. Indeed, several cancer driver genes and oncogenic pathways have been identified using this approach (Sweet-Cordero et al., 2005; Paoloni et al., 2009; Colak et al., 2010; Chen et al., 2013; Colak et al., 2013; Jin et al., 2019). In this study, we performed integrated transcriptomic and network analyses using several independent genome-wide gene expression profiling of human eHCCs and a rat model that we previously developed (Colak et al., 2010). Our aim is to identify a gene signature that is conserved across species and also expressed in blood and that may be involved in development of earliest phase of the disease and disease progression. We employed independent datasets of PBMC gene expression profiling for eHCC samples as well as eHCC dataset gathered from TCGA to estimate the diagnostic and prognostic significance of the discovered gene signature. In addition, functional network and pathway analyses were performed to identify significantly altered pathways that may be critical for early HCC transformation. Multivariate Cox regression analysis demonstrated that the identified gene signature predicted the disease outcome independent of other clinical variables. Our results may provide our gene signature’s potential to detect the disease in early stages by utilizing patients’ biological fluids, rather than using invasive procedures and prognostic significance for differentiating the high-risk patient group with a poor disease outcome from the low-risk group with a more favorable outcome.
Materials and Methods
Gene Expression Analysis
Integrated whole-genome gene expression analysis is performed using a rat eHCC dataset as well as several other publically available genomic datasets for human eHCC from independent studies, such as Chiang et al. (GSE9843) (Chiang et al., 2008) (n = 65) and Wurmbach et al. (GSE6764) (Wurmbach et al., 2007) (n = 28). The rat eHCC dataset is from our previous study (Colak et al., 2010), which consisted of 22 samples (6 eHCC, 8 regenerated liver, and 8 normal samples) that were probed using Applied Biosystems Rat Genome Survey microarray. Chiang et al.‘s dataset contained 91 HCV-related HCC tumor samples, of which 65 of them were eHCC that we used in our analysis. Wurmbach et al.‘s dataset included 75 samples, 28 of which we used (18 eHCC and 10 normal samples) in this study, hence included only data from patients with eHCC. All samples were probed using Affymetrix HGU133 Plus 2.0 array. We performed ANOVA to identify significant differentially expressed genes (DEGs) in each dataset. We adjusted the p values for multiple comparisons by false discovery rate (FDR) according to Benjamini-Hochberg step-up procedure (Benjamini and Hochberg, 1995). Significantly DEGs were defined as those with adjusted p-value < 0.05, and absolute fold change >1.5. The Venn diagram approach was used to find significant genes that are conserved in both rat and human HCC datasets. The GTEx portal (https://gtexportal.org/home/) is used to identify genes that are expressed in blood. An illustration of our methodology is shown in Figure 1.
Functional Pathway, Gene Ontology Enrichment and Network Analyses
Functional pathway, gene ontology (GO) enrichment, and gene interaction network analyses were performed using (QIAGEN Inc., https://www.qiagenbioinformatics.com/products/ingenuity-pathway-analysis), (DAVID) (Dennis et al., 2003), Protein Analysis Through Evolutionary Relationships (PANTHER™) classification systems (Mi et al., 2017), and Network Analyst. (Xia et al., 2014; Xia et al., 2015). The DEGs were mapped to its corresponding gene object in the Ingenuity pathway knowledge base and gene interaction networks. A right-tailed Fisher’s exact test was used to calculate a p-value determining the probability that the biological function (or pathway) assigned to the data set is explained by chance alone (Colak et al., 2020).
Independent Gene Expression Datasets for Validation
In order to validate the diagnostic value of our gene signature, we used two independently performed microarray and RNAseq datasets for early human HCCs. The first dataset was a PBMC microarray (Affymetrix Human Genome U133 U Plus 2.0 array) from Shi et al’s study (10 eHCCs and 10 normal controls) (Shi et al., 2014). The second dataset was RNAseq primary solid tumors from The Cancer Genome Atlas (TCGA) database that contained data from 421 samples (371 HCCs and 50 normal controls), including cases with HBV-infected, HCV-infected, and no virus infected HCCs. Among those 371 HCC samples, 171 were diagnosed as early HCC with stage 1 (TCGA-eHCC), which we also used for validation. We performed unsupervised PCA and two-dimensional hierarchical clustering by Pearson correlation with average linkage clustering using PARTEK Genomics Suite (Partek Inc., St. Louis, MO, United States). Moreover, we obtained an independent microarray gene expression profiling dataset from Gene Expression Omnibus database (GSE25097) (Ivanovska et al., 2011; Lamb et al., 2011) to validate the classifier built using our identified gene signature. The dataset includes 511 samples (268 hepatitis B virus (HBV) related HCC tumors and 243 adjacent normals). In order to identify the association of our gene signature with HCC tumor growth rate, we retrieved and reanalyzed a whole genome microarray expression dataset (GSE54236) (Villa et al., 2016). It contains 161 samples of HCC (n = 81) and normal (n = 80) tissue. Samples were probed using Agilent Whole Human Genome Oligo Microarrays. Tumor samples were divided into fast-growing (n = 20) and slow-growing tumors (n = 61) according to tumor doubling time, where the first quartile is considered the fast-growing tumors and the other three quartiles are the slow-growing tumors.
Multivariate and Survival Analyses
Univariate and multivariate Cox regression analyses were used to investigate the prognostic value of our gene signature along with other clinical variables. A prognosis risk score for each patient was calculated (Aguirre-Gamboa et al., 2013), which is a linear combination of expression levels of our genes multiplied by a regression coefficient (β) of each gene extracted from the multivariate Cox proportional hazards regression model, using the following formula: prognosis risk score = expression of gene1 × β1+ expression of gene2 × β2 + … expression of genen × βn. We used an independent liver cancer dataset with detailed clinical data from TCGA RNAseq dataset that consisted of samples from 361 liver cancer patients. We used the median as a cutoff value for classifying patients into high and low risk groups.
Overall and recurrence free survival analyses were also performed. Survival curves were plotted using the Kaplan-Meier method, and significance between survival curves was calculated by the log-rank test. The Cox proportional-hazards regression for survival data was used to calculate hazard ratios. A p-value < 0.05 was considered statistically significant.
HCC Classifier Model and Performance Evaluation
We used different machine learning algorithms to develop a predictive model for HCC using the 41 geneset. Several classifiers were built using K-Nearest Neighbor (KNN), Linear Support Vector Machine (SVM), Linear Discriminant Analysis (LDA) and Naïve Bayes (NB) to achieve the optimal classifier. The standardized gene expression values of our gene set were used as feature values. We estimated the classification performance on TCGA with 10-fold cross validation as well as training with TCGA (early stage HCC samples only, n = 221) and testing on an independent dataset (GSE25097) (n = 511 samples) (Ivanovska et al., 2011; Lamb et al., 2011). Hence, we tested the classifer performance on datasets that were not used for signature identification to confirm if our gene set is able to distinguish patients from normal controls. Four statistics measures were used: accuracy, specificity, sensitivity, and area under curve (AUC) that are defined as:
The TP, TN, FP, FN indicate true positive, true negative, false positive, and false negative, respectively. The classification analyses were performed using PARTEK Genomics Suite (Partek Inc., St. Louis, MO, United States).
Statistical Analysis
Statistical analyses were conducted using MATLAB software packages (Mathworks, Natick, MA, United States) and PARTEK Genomics Suite (Partek Inc., St. Lois, MO, United States). All statistical tests were two-sided and p-value < 0.05 was considered statistically significant.
Results
Identification of a Blood-Based Gene Signature for Early HCC (eHCC)
Genome-wide gene expression profiling provides valuable insight into the transcriptional changes that appear during the carcinogenic process beyond what may be obvious from studies evaluating only clinicopathologic characteristics. The investigation of human diseases using a combination of the human genome-wide molecular data and interactome may further provide an important viewpoint for understanding the molecular features of diseases (Al-Harazi et al., 2016; Al-Harazi et al., 2019). Here, we used several genome-wide gene expression profiling datasets from human eHCCs and a rat model of early HCC to conduct an integrative analysis. We identified 307 differentially expressed genes (DEGs) using our previous rat model of early HCC (Colak et al., 2010). We then analyzed human HCC data, focusing only on patients with early HCC, from Chiang et al. (Chiang et al., 2008) and Wurmbach et al. (Wurmbach et al., 2007), that revealed 4,289 and 5,342 DEGs that were significantly dysregulated in patients compared to normal controls (adjusted p-value < 0.05 and absolute fold-change > 1.5). The Venn diagram approach indicated 2,796 DEGs were shared by both human datasets and 54 of which were conserved across both species (Figure 1). Cross-species conserved genes in eHCC that are also expressed in blood revealed 41 genes that we define as “41-gene signature” (Figure 2A and Table 1). We then performed functional and network analyses as well as extensive validations for the diagnostic and prognostic potential of the identified geneset (Figure 1).
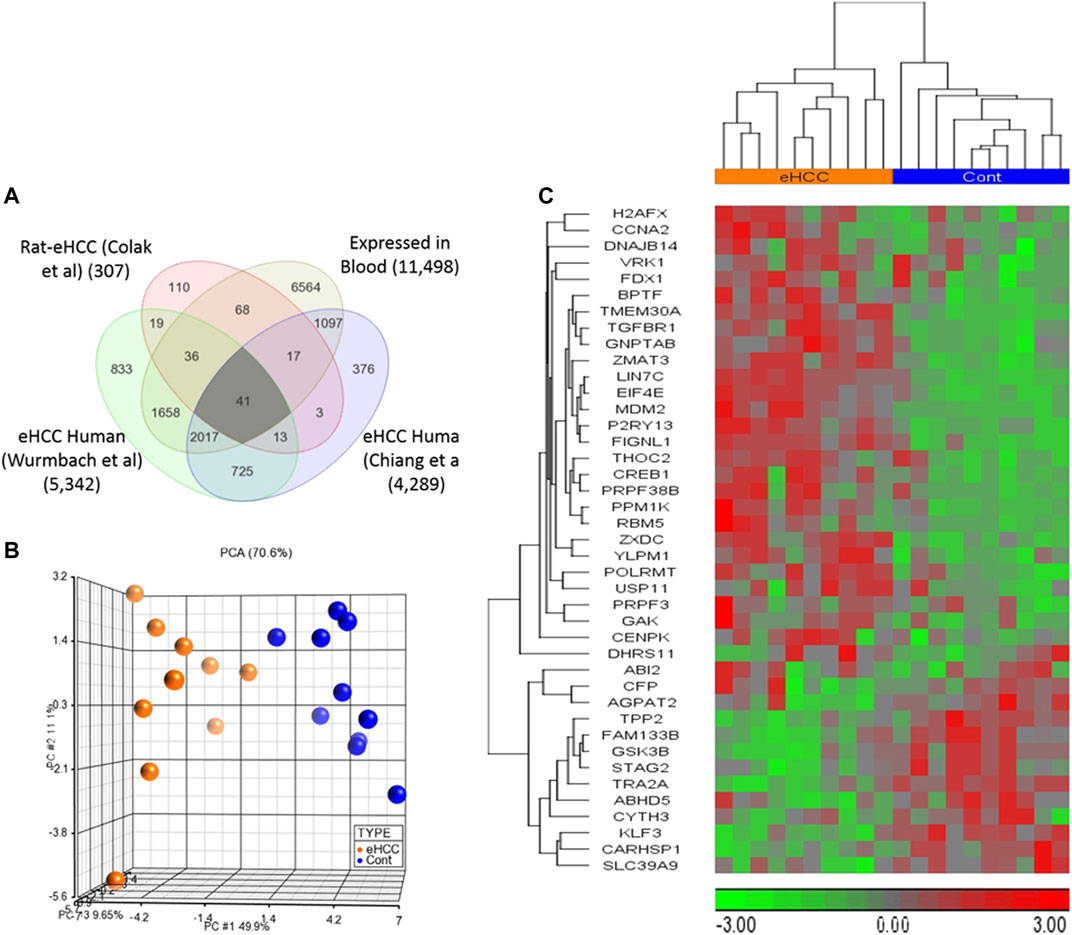
FIGURE 2. (A) Venn diagram demonstrates that there are 41 up- or down-regulated DEGs common among three different datasets and expressed in blood. (B,C) Unsupervised principal component analysis (PCA) and two-dimensional hierarchical clustering using our 41-geneset in microarray dataset derived from blood samples from patients with early HCC and controls (Shi et al., 2014). The PCA clearly separated eHCC patients from normal controls. The hierarchical clustering revealed two main sample-clusters: one with eHCC patients and the other with healthy controls. Samples are denoted in columns and genes are in rows. The orange color refers to eHCC samples, and blue for normal controls.
Diagnostic and Prognostic Significance of the 41-Gene Signature
We employed two independent datasets for early human HCCs to confirm the diagnostic significance of the 41-geneset. The first dataset is a microarray data extracted from PBMC RNA samples of patients with HCC (Shi et al., 2014). The unsupervised principal components analyses (PCA) and two-dimensional hierarchical clustering using our 41-geneset clearly distinguished patients as either eHCC or normal controls in both datasets (Figures 2B,C, respectively). Moreover, we also tested the 41-gene using mRNAseq data from TCGA (n = 421). We selected only the patients with eHCC and controls (n = 171 patients, 50 controls). The unsupervised two-dimensional hierarchical clustering using the 41-geneset separated samples into two main clusters with differing gene expression patterns, one cluster with eHCC samples and the other one mainly composed of controls (Figure 3A).
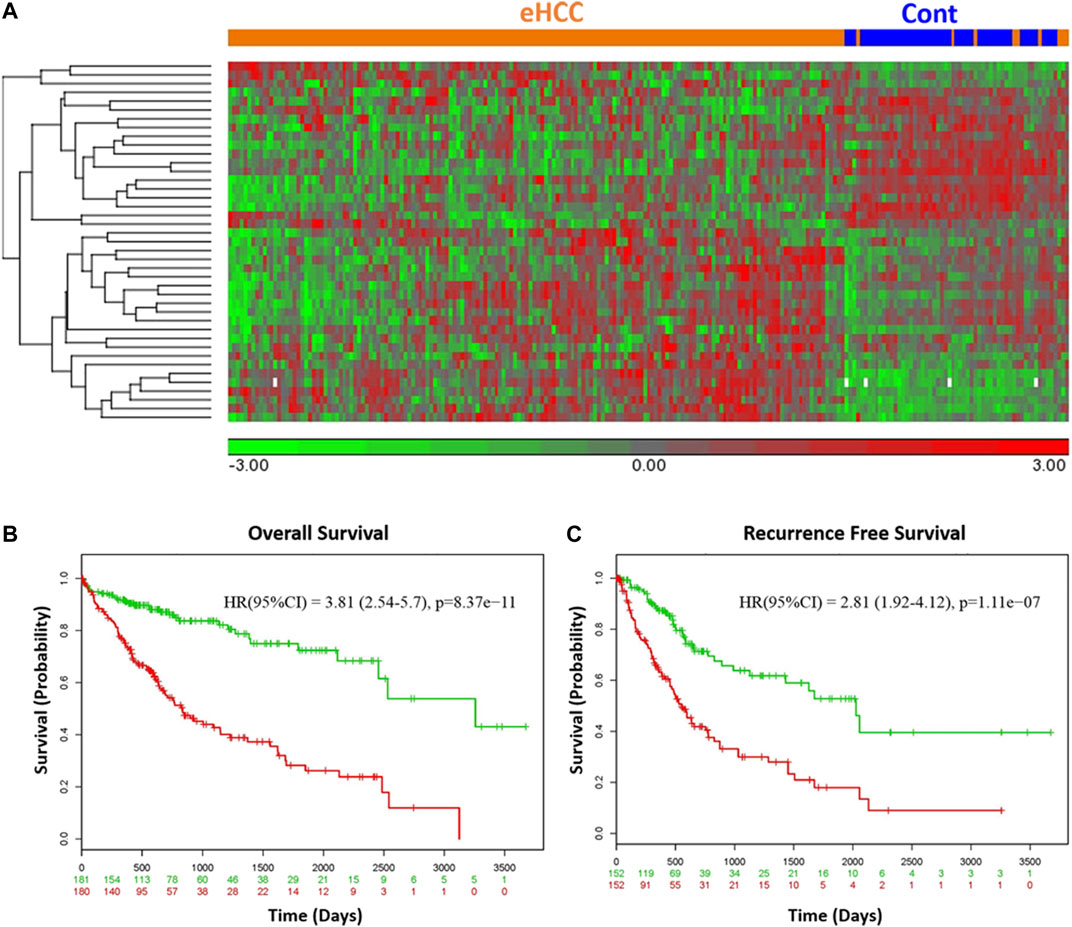
FIGURE 3. (A) Unsupervised two-dimensional hierarchical clustering of TCGA using our 41-gene signature. The hierarchical clustering distinguished samples as either eHCC or normal controls. Samples are indicated in columns, while genes are in rows. Orange indicates eHCC and blue control samples. The expression level of each gene across the samples is normalized to (−3, 3). Overall (B) and recurrence free survival (C) of 41-gene signature using TCGA dataset. Kaplan-Meier curves for risk groups, “+” mark indicates censoring samples. Horizontal axis denotes time to event. Red/Green curves indicate High/Low-risk groups respectively. The red and green numbers below horizontal axis represent the number of individuals not presenting the event of the corresponding risk group along time.
We confirmed the prognostic significance of our gene signature using TCGA HCC cohort for overall as well as recurrence-free survival. A prognostic risk score is calculated using our 41-gene as described in the methods section and patients are classified as high or low risk using the median risk score as cutoff. The survival analyses demonstrated that high risk score cohort were significantly associated with poor disease outcome (Figures 3B,C). Indeed, patients in the high-risk group (n = 180) had a significantly poorer prognosis with hazard ratio (HR) = 3.81 (p = 8.37 × 10−11, 95% confidence interval [CI]: 2.54–5.7) for overall survival (Figure 3B) and HR = 2.81 (95% CI = 1.92–4.12; p = 1.1 × 10-7) for the recurrence-free survival (Figure 3C).
The 41-Gene Signature Is an Independent Prognostic Factor
We used Cox’s regression models to determine whether 41-gene signature could prognosticate disease outcome independent of other clinical factors. Univariate regression analysis of the TCGA dataset showed that the 41-gene risk score (HR = 3.81, 95% CI = 2.54–5.70; P = 8.37 × 10-11), stage (HR = 2.56, 95% CI = 1.75–3.7; P = 1.38 × 10-6), and macrovascular invasion (HR = 2.20, 95% CI = 1.00–4.84; P = 0.05) were significantly associated with patient prognosis, while age, gender, grade and AFP showed no significant association with overall survival. However, the multivariate regression analysis revealed that only the 41-gene signature predicted the outcome of HCC independent of other clinical variables (HR = 3.24, 95% CI = 1.95–5.38; P = 5.58 × 10-6) (Table 2).
Association of the 41-Gene Signature With the HCC Dynamic Progression
We also investigated if genes in 41-geneset are involved in dynamic progression of the disease using a transcriptomic dataset that included information on HCC patients at presentation according to fast- or slow-growth speed (Villa et al., 2016).The tumor doubling times ranged from 30 to 621 days were divided into quartiles and classified as fast-growing (≤53 days; n = 19) and slow-growing (>54 days; n = 59) (Villa et al., 2016). Testing the mRNA expression of genes in 41-geneset revealed that CCNA2, CENPK, CYTH3, FIGNL1, and H2AFX have significantly higher level of expression in fast-growing tumors compared to slow-growing tumors and AGPAT2, CFP, and FDX1 have significantly lower level of expression in fast-growing tumors compared to slow-growing tumors (Figure 4).
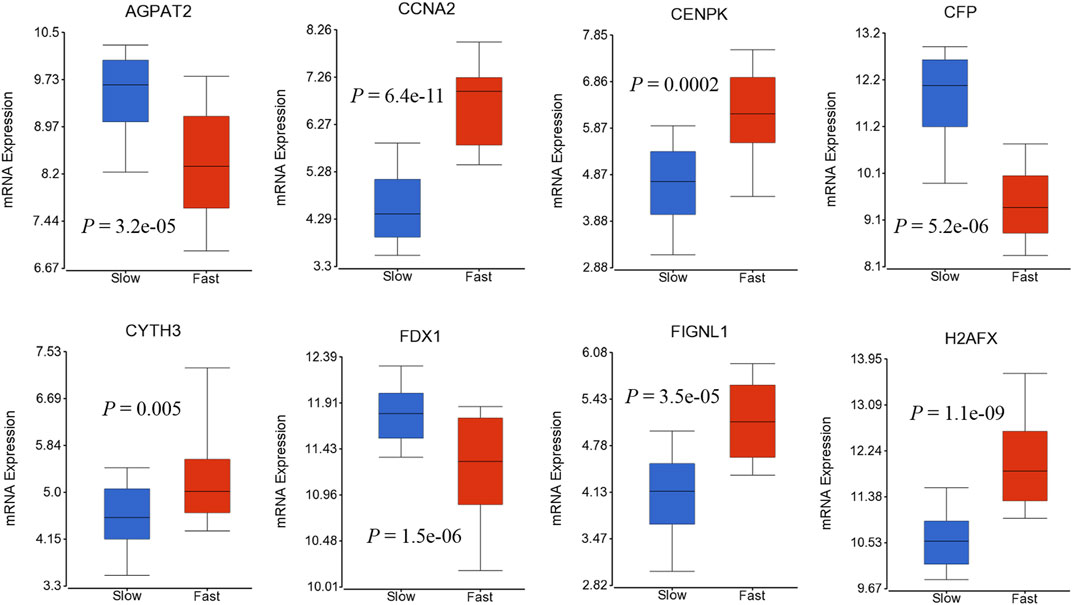
FIGURE 4. Boxplots displaying the gene expression levels of selected genes in fast-growing (≤53 days; n = 19) and slow-growing (>54 days; n = 59) using dataset by (Villa et al., 2016).
Gene Ontology Enrichment, Pathway and Network Analyses
Gene ontology enrichment and functional analyses of the blood-based gene signature revealed that 41-gene signature is enriched for functional categories related to DNA replication, recombination, and repair, RNA metabolic process, cell cycle, tissue development, cell death, and survival (Figure 5A; Table 3). The significantly altered canonical pathways include a number of cancer pathways, including Cell Cycle: G1/S Checkpoint Regulation, ATM Signaling, Antiproliferative Role of TOB in T Cell Signaling, PI3K/AKT Signaling, Wnt/β-catenin and Wnt/Ca2+ pathways. The gene interaction network of 41-gene is displayed in Figure 5B. Furthermore, network analysis indicated hub genes that may be potentially important in eHCC transformation, including EIF4E, GSK3β, CCNA2, H2AFX, TGFBR1 and CREB1 (Figure 5C).
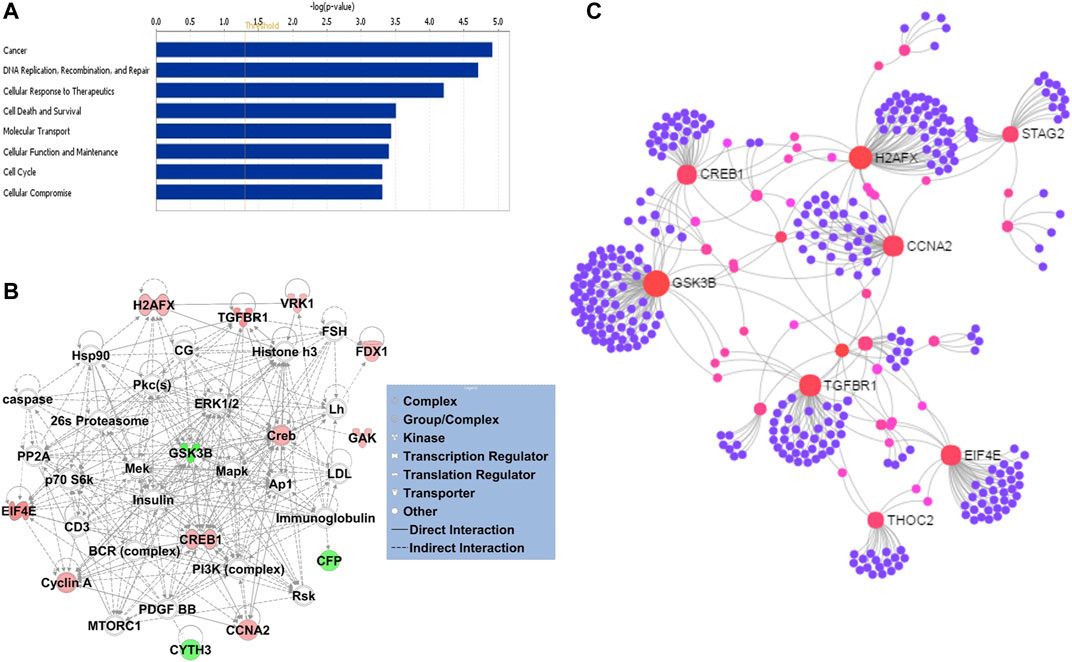
FIGURE 5. (A) The GO and functional, and (B) gene interaction network analyses of the gene signature. Red/green indicates higher/lower expression in eHCCs compared to controls. Straight lines are for direct interactions and dashed lines for indirect ones. (C) A subnetwork of the 41-gene signature with the hub genes labelled. The size of nodes is proportional to their betweenness centrality values.
Classification Model for HCC and Validation Analysis
We used several classification algorithms, including KNN, SVM, LDA, and NB, to design an optimal classifier for HCC with the 41-gene signature. The KNN (k = 1 and Pearson’s Dissimilarity distance measure) has outperformed the others and displayed the best classification power. We developed multiple KNN classifiers using different parameters and then tested their performance on independent validation datasets (Table 4). First, we used the Chiang et al. dataset as training and the TCGA dataset (consisting of only early stage HCC cases and controls; the TCGA-eHCC dataset) as testing. The clasisifcation model achieved a high prediction accuracy of 95% and sensitivity, specificity and AUC of 95, 96 and 95%, respectively. We then used the TCGA dataset that included all HCC samples with all disease stages (n = 421) as well as using only early stage HCC samples (n = 221) as a training dataset to develop the KNN classifier and performed 10-fold cross-validations. The 41-gene classifier displayed high prediction accuracy of 95 and 93% using all HCC samples and early HCC samples, respectively, and both displayed much superior performance compared to using serum biomarker AFP (Table 4). Finally, we trained the classifier on early stage HCC samples from TCGA (n = 221) and tested the classifier performance on an independent validation dataset (GSE25097) that included 511 samples (268 HBV-infected HCC tumors and 243 normals). Again, the designed 41-gene model has achieved a superior classification performance (accuracy of 90%) compared to using AFP (accuracy = 53%) alone (Table 4).
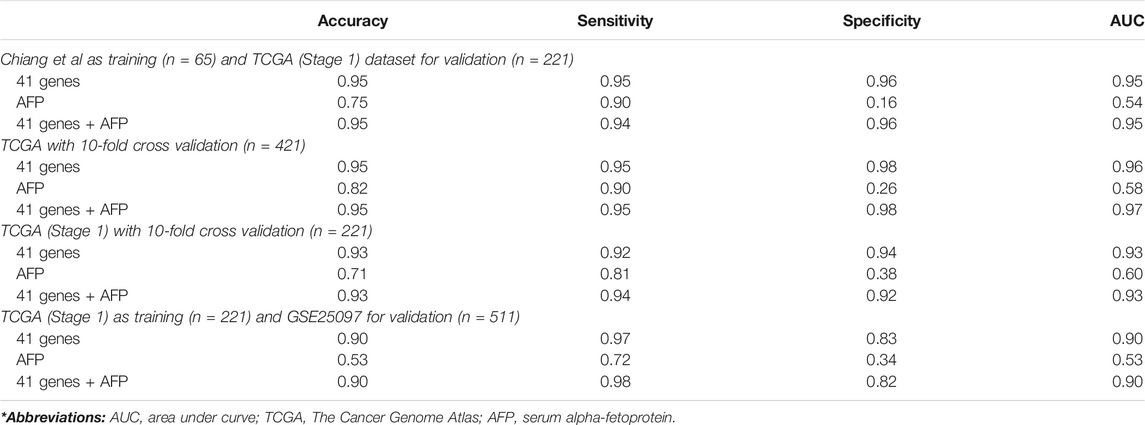
TABLE 4. Classification analyses. Prediction performance of KNN classification on different validation datasets.
Discussion
Hepatocellular carcinoma (HCC) is the fourth leading global cause of cancer-related death in the world (Bray et al., 2018). It is a malevolent disease that develops furtively and is frequently diagnosed at advanced stages, resulting in a poor prognosis. Robust early detection biomarkers are needed to detect the disease at its onset as well as to enable prognostic estimation to improve the outcomes of the patients with HCC (Colak et al., 2010; Shi et al., 2014; Allain et al., 2016; Wang et al., 2017; Guan et al., 2020; Li et al., 2020). Currently there are a number of biomarkers that have been used as HCC diagnostic markers, such as AFP that has been commonly used for its feasibility and low cost. Other biomarkers include AFP-L3, des-gamma-carboxyprothrombin (DCP), glypican-3, insulin-like growth factor (IGF)-1, and hepatocyte growth factor (HGF) (Schütte et al., 2014). However, alpha-Fetoprotein bears low specificity owing to its presence in other cancer types (Schütte et al., 2014). Recent genomic works focus on using RNA-related approaches such as mRNA (Wang et al., 2017; Guan et al., 2020; Zhou et al., 2020) and miRNA signatures (Liu et al., 2017; Bai et al., 2018a), long non-coding RNAs (Arun et al., 2018; Ma et al., 2020) targeted mRNAs for critical genes (Lin et al., 2016), and DNA based approaches such as driver mutations or circulating tumor DNAs (Yang et al., 2019b; Cai et al., 2019). However, some of the highly predictive biomarkers suffer from requiring invasive procedures or not able to detect the early HCC development. Therefore, there is clear and unmet need for biomarkers that are more informative and sensitive with high accuracy for the early detection and prognostication of HCC. In this study, we performed an integrated transcriptomic and network-based analyses of eHCC using several whole-genome gene expression profiling datasets from human eHCCs and rat model of eHCC that we developed previously (Colak et al., 2010). We aimed to identify a gene signature that is conserved across species that detect the disease in early stages in patients’ biological fluids instead of using invasive techniques and also have prognostic significance for differentiating the high-risk patient group from the low-risk group.
We identified 41-gene signature and validated its diagnostic and prognostic potential using independent datasets from large cohort of HCC patients (over 600 cases), including gene expression profiling of PBMC from patients with eHCC as well as the eHCC cohort in TCGA. As we used comparative genomic analysis of rat and human eHCCs, the resulting gene signature had conserved genomic profile of the tumor. The previous studies have demonstrated that cross-species comparative genomic method is a robust methodology for identifying essential genes that are involved in tumorigenesis, invasion and progression, and hence has therapeutic promise (Sweet-Cordero et al., 2005; Paoloni et al., 2009; Colak et al., 2010; Colak et al., 2013). Several driver mutations and critical pathways in disease progression have been identified using this approach (Sweet-Cordero et al., 2005; Jin et al., 2019).
Our results reveal alterations in several cancer-related pathways as well as key hub genes, including EIF4E, GSK3β, CCNA2, H2AFX, TGFBR1, CREB1, TGFBR1, THOC2, ZMAT3, and STAG2, potentially critical in early HCC transformation and progression. The EIF4E aids in translation initiation by uniting the 4 F complex. EIF4E behaves as a proto-oncogene involved in transformation and tumorigenesis. EIF4E have previously been linked to human cancer. It has been reported that EIF4E protein contributes to malignant transformation and progression by enhancing translation of cancer-related mRNAs in eukaryotic cells (Jiang et al., 2016). In addition, there are several studies investigated the association of EIF4E and human tumors such as lung cancer (Seki et al., 2010), colorectal cancer (Niu et al., 2014; Diab-Assaf et al., 2015; Slattery et al., 2017), breast cancer (Heikkinen et al., 2013; Hu et al., 2014), and head and neck carcinoma (Nathan et al., 1997; Culjkovic and Borden, 2009). In patients with HCC, the high expression of EIF4E is associated to tumorigenesis (Jiang et al., 2016). Moreover, patients with increased EIF4E expression had a poorer prognosis compared to patients with decreased EIF4E expression (Jiang et al., 2016). Moreover, it is also found in another study that patients with high expression levels of EIF4E had more recurrent liver metastasis (Li et al., 2016). However, the molecular role of EIF4E in eHCC blood samples has not been well-defined. Herein, we found that EIF4E is one of the significant genes that show high expression in blood samples from eHCC compared to normal samples (Figure 2C and Supp Figure 1). The functional analysis indicates that EIF4E is associated with several GO biological functions including the cell cycle, regulation of cell development, RNA metabolic processes, viral processes, and gene expression (Table 2).
Our findings indicated that the expression of GSK3β is significantly lower in eHCC tumor samples in comparison to normal samples in blood (Figure 2C and Supp Figure 1). Previous studies indicated that the GSK-3 gene family plays a significant role in various human cancers, including hepatocarcinoma (McCubrey et al., 2014; Mancinelli et al., 2017). The authors have also reported that GSK-3 has an effect on tumor progression by stabilizing the beta-catenin complex components (McCubrey et al., 2014; Mancinelli et al., 2017). Furthermore, it has been reported that the dysregulation of GSK3β phosphorylation and inhibition of GSK3β activity contributes to hepatocarcinogenesis (Desbois-Mouthon et al., 2002; McCubrey et al., 2014). Previous studies indicate that GSK3β is possibly a suppressor gene in HCC tumors, due to the loss of GSK3β expression and/or activity participating in HCC progression (McCubrey et al., 2014; Cervello et al., 2017; Fang et al., 2019).
Our results also revealed that CCNA2 and H2AFX have significantly higher level of mRNA expression in both tissue and blood samples from eHCC (Figure 2C, 3A, and Supp Figure 1). CCNA2 is considered a biomarker for ER-positive breast cancer prognosis and it can help monitor tamoxifen efficacy (Gao et al., 2014). In some recent studies, CCNA2 was suggested to be a prognostic biomarker for liver carcinoma, as it may help in developing an effective therapeutic and/or preventative approach for HCC (Bai et al., 2018b; Zhang et al., 2018; Wu et al., 2019). The H2AFX and its phosphorylated C-terminal (γ-H2AX) are potential regulators of DNA repair and are essential in DNA damage response (Celeste et al., 2003; Bassing and Alt, 2004; Fernandez-Capetillo et al., 2004). Matsuda et al. reported that histological grades of HCC are associated with the level of labeling index (LI) of γ-H2AX, which indicates that γ-H2AX may play a significant role in the development of HCC, particularly throughout the early stages of carcinogenesis (Matsuda et al., 2013). Interestingly, we also found that CCNA2 and H2AFX have significantly higher level of expression in fast-growing tumors compared to slow-growing tumors (Figure 4), indicating their association with rapid tumor growth.
Gene ontology, gene network and pathway analyses of the 41-gene signature revealed enrichment of biological functions including DNA replication, recombination and repair, cellular response to therapeutics, cell cycle, cell death and survival, and regulation of cell development (Figure 5A). DNA repair genes are overexpressed in cancer tissues, and hence develop larger DNA repair capacity compared to normal tissues (Kirkali et al., 2011; Lin et al., 2016). Consequently, numerous DNA damage signals and DNA repair pathways may have a major influence on prognosis and response to therapy for different types of cancers (Dizdaroglu, 2015; Lin et al., 2016). The cell death process appears in almost all types of human liver diseases including HCC, and it is considered a sensitive parameter for the detection of disease (Luedde et al., 2014). Our results revealed significantly dysregulated genes that are associated with cell death and survival, including TGFBR1, RBM5, THOC2, USP11, MDM2, TPP2, EIF4E, VRK1, CCNA2, ZMAT3, AGPAT2, H2AFX, CREB1, FIGNL1, and GSK3B. Identifying the characteristics of these genes and their network of interaction is important to understand the pathophysiology of HCC and discover new therapeutic targets for the disease.
Conclusion
In summary, our study reveals several genes and pathways that are essential for eHCC transformation and validate our gene signature’s potential to detect the disease in patients’ biological fluids instead of utilizing invasive techniques and predict the disease prognosis. Having genomic biomarkers with diagnostic and prognostic potential is invaluable for HCC patients for early detection of the disease at its earliest stage as well as differentiating the high risk patient group with poor disease outcome from the low risk ones. Our results suggest that the integrated cross-species transcriptomic analysis with the gene networks may provide a robust methodology for understanding the key biological programs in eHCC and may lead to better diagnosis, prognosis and therapeutic choices.
Data Availability Statement
Publicly available datasets were analyzed in this study. These data can be found here: The Cancer Genome Atlas (TCGA) and the NCBI Gene Expression Omnibus.
Author Contributions
DC conception, design, and supervision. DC, OA, and IHK collected, analyzed, interpreted the data, and drafted the manuscript. NK, MA, LA, MA, and AA participated in revising the manuscript. All authors read and approved the manuscript.
Funding
This study is funded by the Research Grant (RAC#2110006 and 2180030 to DC). We would like to extend our thanks and appreciation to our individual sponsor who generously donated to this research. The funder had no role in the study design and collection, analysis, and interpretation of the results.
Conflict of Interest
The authors declare that the research was conducted in the absence of any commercial or financial relationships that could be construed as a potential conflict of interest.
Publisher’s Note
All claims expressed in this article are solely those of the authors and do not necessarily represent those of their affiliated organizations, or those of the publisher, the editors and the reviewers. Any product that may be evaluated in this article, or claim that may be made by its manufacturer, is not guaranteed or endorsed by the publisher.
Acknowledgments
We would like to thank King Faisal Specialist Hospital and Research Centre (KFSH&RC) and our individual sponsor who generously donated to this research (RAC#2110006 and 2180030 to DC). We also would like to thank Sukina Qanbar for administrative assistance. This work was under an institutionally approved King Faisal Specialist Hospital and Research Centre project (RAC# 2110006 and 2180030).
Supplementary Material
The Supplementary Material for this article can be found online at: https://www.frontiersin.org/articles/10.3389/fgene.2021.710049/full#supplementary-material
Supplementary Figure 1 | Boxplots display the gene expression levels of selected genes in blood of early HCC and controls using dataset from (Shi et al., 2014).
Abbreviations
AFP, alpha-fetoprotein; DCP, des-gamma-carboxyprothrombin; DEG, differentially expressed gene; eHCC, early HCC; FDR, false discovery rate; GO, gene ontology; GTEx, Genotype-Tissue Expression; HCC, hepatocellular carcinoma; HGF, hepatocyte growth factor; IGF, insulin-like growth factor; OS, Overall Survival; PBMC, peripheral blood mononuclear cell; PCA, principal component analysis; PPI, protein-protein interaction; TCGA, The Cancer Genome Atlas.
References
Aguirre-Gamboa, R., Gomez-Rueda, H., Martínez-Ledesma, E., Martínez-Torteya, A., Chacolla-Huaringa, R., Rodriguez-Barrientos, A., et al. (2013). SurvExpress: an Online Biomarker Validation Tool and Database for Cancer Gene Expression Data Using Survival Analysis. PLoS One 8 (9), e74250. doi:10.1371/journal.pone.0074250
Al-Harazi, O., Al Insaif, S., Al-Ajlan, M. A., Kaya, N., Dzimiri, N., and Colak, D. (2016). Integrated Genomic and Network-Based Analyses of Complex Diseases and Human Disease Network. J. Genet. Genomics 43 (6), 349–367. doi:10.1016/j.jgg.2015.11.002
Al-Harazi, O., El Allali, A., and Colak, D. (2019). Biomolecular Databases and Subnetwork Identification Approaches of Interest to Big Data Community: An Expert Review. OMICS: A J. Integr. Biol. 23 (3), 138–151. doi:10.1089/omi.2018.0205
Allain, C., Angenard, G., Clément, B., and Coulouarn, C. (2016). Integrative Genomic Analysis Identifies the Core Transcriptional Hallmarks of Human Hepatocellular Carcinoma. Cancer Res. 76 (21), 6374–6381. doi:10.1158/0008-5472.CAN-16-1559
Arun, G., Diermeier, S. D., and Spector, D. L. (2018). Therapeutic Targeting of Long Non-coding RNAs in Cancer. Trends Mol. Med. 24 (3), 257–277. doi:10.1016/j.molmed.2018.01.001
Bai, F., Zhou, H., Ma, M., Guan, C., Lyu, J., and Meng, Q. H. (2018). A Novel RNA Sequencing-Based Mi RNA Signature Predicts with Recurrence and Outcome of Hepatocellular Carcinoma. Mol. Oncol. 12 (7), 1125–1137. doi:10.1002/1878-0261.12315
Bai, G., Zheng, W., and Ma, W. (2018). Identification and Functional Analysis of a Core Gene Module Associated with Hepatitis C Virus-Induced Human Hepatocellular Carcinoma Progression. Oncol. Lett. 15 (5), 6815–6824. doi:10.3892/ol.2018.8221
Bassing, C. H., and Alt, F. W. (2004). H2AX May Function as an Anchor to Hold Broken Chromosomal DNA Ends in Close Proximity. Cell Cycle 3 (2), 149–153. doi:10.4161/cc.3.2.689
Benjamini, Y., and Hochberg, Y. (1995). Controlling the False Discovery Rate: a Practical and Powerful Approach to Multiple Testing. J. R. Stat. Soc. Ser. B (Methodological) 57, 289–300. doi:10.1111/j.2517-6161.1995.tb02031.x
Bray, F., Ferlay, J., Soerjomataram, I., Siegel, R. L., Torre, L. A., and Jemal, A. (2018). Global Cancer Statistics 2018: GLOBOCAN Estimates of Incidence and Mortality Worldwide for 36 Cancers in 185 Countries. CA: A Cancer J. Clinicians 68 (6), 394–424. doi:10.3322/caac.21492
Budhu, A., Roessler, S., Zhao, X., Yu, Z., Forgues, M., Ji, J., et al. (2013). Integrated Metabolite and Gene Expression Profiles Identify Lipid Biomarkers Associated with Progression of Hepatocellular Carcinoma and Patient Outcomes. Gastroenterology 144 (5), 1066–1075 e1. doi:10.1053/j.gastro.2013.01.054
Cai, Z., Chen, G., Zeng, Y., Dong, X., Li, Z., Huang, Y., et al. (2019). Comprehensive Liquid Profiling of Circulating Tumor DNA and Protein Biomarkers in Long-Term Follow-Up Patients with Hepatocellular Carcinoma. Clin. Cancer Res. 25 (17), 5284–5294. doi:10.1158/1078-0432.CCR-18-3477
Celeste, A., Fernandez-Capetillo, O., Kruhlak, M. J., Pilch, D. R., Staudt, D. W., Lee, A., et al. (2003). Histone H2AX Phosphorylation Is Dispensable for the Initial Recognition of DNA Breaks. Nat. Cel Biol 5 (7), 675–679. doi:10.1038/ncb1004
Cervello, M., Augello, G., Cusimano, A., Emma, M. R., Balasus, D., Azzolina, A., et al. (2017). Pivotal Roles of Glycogen Synthase-3 in Hepatocellular Carcinoma. Adv. Biol. Regul. 65, 59–76. doi:10.1016/j.jbior.2017.06.002
Chen, E. Y., Dobrinski, K. P., Brown, K. H., Clagg, R., Edelman, E., Ignatius, M. S., et al. (2013). Cross-species Array Comparative Genomic Hybridization Identifies Novel Oncogenic Events in Zebrafish and Human Embryonal Rhabdomyosarcoma. Plos Genet. 9 (8), e1003727. doi:10.1371/journal.pgen.1003727
Chiang, D. Y., Villanueva, A., Hoshida, Y., Peix, J., Newell, P., Minguez, B., et al. (2008). Focal Gains of VEGFA and Molecular Classification of Hepatocellular Carcinoma. Cancer Res. 68 (16), 6779–6788. doi:10.1158/0008-5472.CAN-08-0742
Colak, D., Al-Harazi, O., Mustafa, O. M., Meng, F., Assiri, A. M., Dhar, D. K., et al. (2020). RNA-seq Transcriptome Profiling in Three Liver Regeneration Models in Rats: Comparative Analysis of Partial Hepatectomy, ALLPS, and PVL. Sci. Rep. 10 (1), 5213. doi:10.1038/s41598-020-61826-1
Colak, D., Chishti, M. A., Al-Bakheet, A.-B., Al-Qahtani, A., Shoukri, M. M., Goyns, M. H., et al. (2010). Integrative and Comparative Genomics Analysis of Early Hepatocellular Carcinoma Differentiated from Liver Regeneration in Young and Old. Mol. Cancer 9, 146. doi:10.1186/1476-4598-9-146
Colak, D., Nofal, A., AlBakheet, A., Nirmal, M., Jeprel, H., Eldali, A., et al. (2013). Age-Specific Gene Expression Signatures for Breast Tumors and Cross-Species Conserved Potential Cancer Progression Markers in Young Women. PLOS ONE 8 (5), e63204. doi:10.1371/journal.pone.0063204
Culjkovic, B., and Borden, K. L. (2009). Understanding and Targeting the Eukaryotic Translation Initiation Factor eIF4E in Head and Neck Cancer. J. Oncol. 2009, 1–12. doi:10.1155/2009/981679
Dennis, G., Sherman, B. T., Hosack, D. A., Yang, J., Gao, W., Lane, H. C., et al. (2003). DAVID: Database for Annotation, Visualization, and Integrated Discovery. Genome Biol. 4 (5), P3. doi:10.1186/gb-2003-4-5-p3
Desbois-Mouthon, C., Blivet-Van Eggelpoë, M.-J., Beurel, E., Boissan, M., Delélo, R., Cadoret, A., et al. (2002). Dysregulation of Glycogen Synthase Kinase-3β Signaling in Hepatocellular Carcinoma Cells. Hepatology 36 (6), 1528–1536. doi:10.1053/jhep.2002.37192
Diab-Assaf, M., Abou-Khouzam, R., Saadallah-Zeidan, N., Habib, K., Bitar, N., Karam, W., et al. (2015). Expression of Eukaryotic Initiation Factor 4E and 4E Binding Protein 1 in Colorectal Carcinogenesis. Int. J. Clin. Exp. Pathol. 8 (1), 404–413.
Dizdaroglu, M. (2015). Oxidatively Induced DNA Damage and its Repair in Cancer. Mutat. Research/Reviews Mutat. Res. 763, 212–245. doi:10.1016/j.mrrev.2014.11.002
Fang, G., Zhang, P., Liu, J., Zhang, X., Zhu, X., Li, R., et al. (2019). Inhibition of GSK-3β Activity Suppresses HCC Malignant Phenotype by Inhibiting Glycolysis via Activating AMPK/mTOR Signaling. Cancer Lett. 463, 11–26. doi:10.1016/j.canlet.2019.08.003
Fernandez-Capetillo, O., Lee, A., Nussenzweig, M., and Nussenzweig, A. (2004). H2AX: the Histone Guardian of the Genome. DNA Repair 3 (8-9), 959–967. doi:10.1016/j.dnarep.2004.03.024
Gao, T., Han, Y., Yu, L., Ao, S., Li, Z., and Ji, J. (2014). CCNA2 Is a Prognostic Biomarker for ER+ Breast Cancer and Tamoxifen Resistance. PLoS One 9 (3), e91771. doi:10.1371/journal.pone.0091771
Guan, W.-j., Ni, Z.-y., Hu, Y., Liang, W.-h., Ou, C.-q., He, J.-x., et al. (2020). Clinical Characteristics of Coronavirus Disease 2019 in China. N. Engl. J. Med. 382, 1708–1720. doi:10.1056/NEJMoa2002032
Heikkinen, T., Korpela, T., Fagerholm, R., Khan, S., Aittomäki, K., Heikkilä, P., et al. (2013). Eukaryotic Translation Initiation Factor 4E (eIF4E) Expression Is Associated with Breast Cancer Tumor Phenotype and Predicts Survival after Anthracycline Chemotherapy Treatment. Breast Cancer Res. Treat. 141 (1), 79–88. doi:10.1007/s10549-013-2671-2
Hu, A., Sun, M., Yan, D., and Chen, K. (2014). Clinical Significance of mTOR and eIF4E Expression in Invasive Ductal Carcinoma. Tumori J. 100 (5), 541–546. doi:10.1700/1660.1817610.1177/1660.18176
Ivanovska, I., Zhang, C., Liu, A. M., Wong, K. F., Lee, N. P., Lewis, P., et al. (2011). Gene Signatures Derived from a C-MET-Driven Liver Cancer Mouse Model Predict Survival of Patients with Hepatocellular Carcinoma. PLOS ONE 6 (9), e24582. doi:10.1371/journal.pone.0024582
Jiang, X.-M., Yu, X.-N., Huang, R.-Z., Zhu, H.-R., Chen, X.-P., Xiong, J., et al. (2016). Prognostic Significance of Eukaryotic Initiation Factor 4E in Hepatocellular Carcinoma. J. Cancer Res. Clin. Oncol. 142 (11), 2309–2317. doi:10.1007/s00432-016-2232-2
Jin, Z., Liu, S., Zhu, P., Tang, M., Wang, Y., Tian, Y., et al. (2019). Cross-Species Gene Expression Analysis Reveals Gene Modules Implicated in Human Osteosarcoma. Front. Genet. 10, 697. doi:10.3389/fgene.2019.00697
Kirkali, G., Keles, D., Canda, A. E., Terzi, C., Reddy, P. T., Jaruga, P., et al. (2011). Evidence for Upregulated Repair of Oxidatively Induced DNA Damage in Human Colorectal Cancer. DNA Repair 10 (11), 1114–1120. doi:10.1016/j.dnarep.2011.08.008
Lamb, J. R., Zhang, C., Xie, T., Wang, K., Zhang, B., Hao, K., et al. (2011). Predictive Genes in Adjacent Normal Tissue Are Preferentially Altered by sCNV during Tumorigenesis in Liver Cancer and May Rate Limiting. PLOS ONE 6 (7), e20090. doi:10.1371/journal.pone.0020090
Li, L., Xu, T., Zong, Y., Peng, L., Kong, S., Zhou, M., et al. (2016). Overexpression of eIF4E in Colorectal Cancer Patients Is Associated with Liver Metastasis. Ott 9, 815–822. doi:10.2147/OTT.S98330
Li, R., Shui, L., Jia, J., and Wu, C. (2020). Construction and Validation of Novel Diagnostic and Prognostic DNA Methylation Signatures for Hepatocellular Carcinoma. Front. Genet. 11, 906. doi:10.3389/fgene.2020.00906
Lin, Z., Xu, S.-H., Wang, H.-Q., Cai, Y.-J., Ying, L., Song, M., et al. (2016). Prognostic Value of DNA Repair Based Stratification of Hepatocellular Carcinoma. Sci. Rep. 6, 25999. doi:10.1038/srep25999
Liu, G., Wang, H., Fu, J.-d., Liu, J.-y., Yan, A.-g., and Guan, Y.-y. (2017). A Five-miRNA Expression Signature Predicts Survival in Hepatocellular Carcinoma. APMIS 125 (7), 614–622. doi:10.1111/apm.12697
Llovet, J. M., Zucman-Rossi, J., Pikarsky, E., Sangro, B., Schwartz, M., Sherman, M., et al. (2016). Hepatocellular Carcinoma. Nat. Rev. Dis. Primers 2, 16018. doi:10.1038/nrdp.2016.18
Luedde, T., Kaplowitz, N., and Schwabe, R. F. (2014). Cell Death and Cell Death Responses in Liver Disease: Mechanisms and Clinical Relevance. Gastroenterology 147 (4), 765–783 e4. doi:10.1053/j.gastro.2014.07.018 (
Ma, T., Zhou, X., Wei, H., Yan, S., Hui, Y., Liu, Y., et al. (2020). Long Non-coding RNA SNHG17 Upregulates RFX1 by Sponging miR-3180-3p and Promotes Cellular Function in Hepatocellular Carcinoma. Front. Genet. 11, 607636. doi:10.3389/fgene.2020.607636
Mancinelli, R., Carpino, G., Petrungaro, S., Mammola, C. L., Tomaipitinca, L., Filippini, A., et al. (2017). Multifaceted Roles of GSK-3 in Cancer and Autophagy-Related Diseases. Oxidative Med. Cell Longevity 2017, 1–14. doi:10.1155/2017/4629495
Matsuda, Y., Wakai, T., Kubota, M., Osawa, M., Takamura, M., Yamagiwa, S., et al. (2013). DNA Damage Sensorγ-H2ax Is Increased in Preneoplastic Lesions of Hepatocellular Carcinoma. Scientific World J. 2013, 1–7. doi:10.1155/2013/597095
McCubrey, J. A., Steelman, L. S., Bertrand, F. E., Davis, N. M., Sokolosky, M., Abrams, S. L., et al. (2014). GSK-3 as Potential Target for Therapeutic Intervention in Cancer. Oncotarget 5 (10), 2881–2911. doi:10.18632/oncotarget.2037
Mi, H., Huang, X., Muruganujan, A., Tang, H., Mills, C., Kang, D., et al. (2017). PANTHER Version 11: Expanded Annotation Data from Gene Ontology and Reactome Pathways, and Data Analysis Tool Enhancements. Nucleic Acids Res. 45 (D1), D183–D189. doi:10.1093/nar/gkw1138
Nathan, C.-A. O., Liu, L., Li, B. D., Abreo, F. W., Nandy, I., and De Benedetti, A. (1997). Detection of the Proto-Oncogene eIF4E in Surgical Margins May Predict Recurrence in Head and Neck Cancer. Oncogene 15 (5), 579–584. doi:10.1038/sj.onc.1201216
Niu, Z., Wang, J., Muhammad, S., Niu, W., Liu, E., Peng, C., et al. (2014). Protein Expression of eIF4E and Integrin αvβ6 in colon Cancer Can Predict Clinical Significance, Reveal Their Correlation and Imply Possible Mechanism of Interaction. Cell Biosci 4, 23. doi:10.1186/2045-3701-4-23
Paoloni, M., Davis, S., Lana, S., Withrow, S., Sangiorgi, L., Picci, P., et al. (2009). Canine Tumor Cross-Species Genomics Uncovers Targets Linked to Osteosarcoma Progression. BMC Genomics 10 (1), 625. doi:10.1186/1471-2164-10-625
Schütte, K., Schulz, C., Link, A., and Malfertheiner, P. (2014). Current Biomarkers for Hepatocellular Carcinoma: Surveillance, Diagnosis and Prediction of Prognosis. World. J. Heptol. 7 (2), 139–149. doi:10.4254/wjh.v7.i2.139
Seki, N., Takasu, T., Sawada, S., Nakata, M., Nishimura, R., Segawa, Y., et al. (2010). Prognostic Significance of Expression of Eukaryotic Initiation Factor 4E and 4E Binding Protein 1 in Patients with Pathological Stage I Invasive Lung Adenocarcinoma. Lung Cancer 70 (3), 329–334. doi:10.1016/j.lungcan.2010.03.006
Shi, M., Chen, M.-S., Sekar, K., Tan, C.-K., Ooi, L. L., and Hui, K. M. (2014). A Blood-Based Three-Gene Signature for the Non-invasive Detection of Early Human Hepatocellular Carcinoma. Eur. J. Cancer 50 (5), 928–936. doi:10.1016/j.ejca.2013.11.026
Slattery, M. L., Trivellas, A., Pellatt, A. J., Mullany, L. E., Stevens, J. R., Wolff, R. K., et al. (2017). Genetic Variants in the TGFβ-Signaling Pathway Influence Expression of miRNAs in colon and Rectal normal Mucosa and Tumor Tissue. Oncotarget 8 (10), 16765–16783. doi:10.18632/oncotarget.14508
Sweet-Cordero, A., Mukherjee, S., Subramanian, A., You, H., Roix, J. J., Ladd-Acosta, C., et al. (2005). An Oncogenic KRAS2 Expression Signature Identified by Cross-Species Gene-Expression Analysis. Nat. Genet. 37 (1), 48–55. doi:10.1038/ng1490
Tsuchiya, N., Sawada, Y., Endo, I., Saito, K., Uemura, Y., and Nakatsura, T. (2015). Biomarkers for the Early Diagnosis of Hepatocellular Carcinoma. World. J. Heptol. 21 (37), 10573–10583. doi:10.3748/wjg.v21.i37.10573
Villa, E., Critelli, R., Lei, B., Marzocchi, G., Cammà, C., Giannelli, G., et al. (2016). Neoangiogenesis-related Genes Are Hallmarks of Fast-Growing Hepatocellular Carcinomas and Worst Survival. Results from a Prospective Study. Gut 65 (5), 861–869. doi:10.1136/gutjnl-2014-308483
Villanueva, A. (2019). Hepatocellular Carcinoma. N. Engl. J. Med. 380 (15), 1450–1462. doi:10.1056/NEJMra1713263
Waghray, A., Murali, A. R., and Menon, K. N. (2015). Hepatocellular Carcinoma: From Diagnosis to Treatment. World. J. Heptol. 7 (8), 1020–1029. doi:10.4254/wjh.v7.i8.1020
Wang, F., Wang, R., Li, Q., Qu, X., Hao, Y., Yang, J., et al. (2017). A Transcriptome Profile in Hepatocellular Carcinomas Based on Integrated Analysis of Microarray Studies. Diagn. Pathol. 12 (1), 4. doi:10.1186/s13000-016-0596-x
Wu, M., Liu, Z., Li, X., Zhang, A., Lin, D., and Li, N. (2019). Analysis of Potential Key Genes in Very Early Hepatocellular Carcinoma. World J. Surg. Onc 17 (1), 77. doi:10.1186/s12957-019-1616-6
Wurmbach, E., Chen, Y.-b., Khitrov, G., Zhang, W., Roayaie, S., Schwartz, M., et al. (2007). Genome-wide Molecular Profiles of HCV-Induced Dysplasia and Hepatocellular Carcinoma. Hepatology 45 (4), 938–947. doi:10.1002/hep.21622
Xia, J., Benner, M. J., and Hancock, R. E. W. (2014). NetworkAnalyst - Integrative Approaches for Protein-Protein Interaction Network Analysis and Visual Exploration. Nucleic Acids Res. 42 (Web Server issue), W167–W174. doi:10.1093/nar/gku443
Xia, J., Gill, E. E., and Hancock, R. E. W. (2015). NetworkAnalyst for Statistical, Visual and Network-Based Meta-Analysis of Gene Expression Data. Nat. Protoc. 10 (6), 823–844. doi:10.1038/nprot.2015.052
Yang, J. D., Hainaut, P., Gores, G. J., Amadou, A., Plymoth, A., and Roberts, L. R. (2019). A Global View of Hepatocellular Carcinoma: Trends, Risk, Prevention and Management. Nat. Rev. Gastroenterol. Hepatol. 16 (10), 589–604. doi:10.1038/s41575-019-0186-y
Yang, J. D., Liu, M. C., and Kisiel, J. B. (2019). Circulating Tumor DNA and Hepatocellular Carcinoma. Semin. Liver Dis. 39 (4), 452–462. doi:10.1055/s-0039-1688503
Zhang, L., Huang, Y., Ling, J., Zhuo, W., Yu, Z., Shao, M., et al. (2018). Screening and Function Analysis of Hub Genes and Pathways in Hepatocellular Carcinoma via Bioinformatics Approaches. Cancer. Biomark. 22 (3), 511–521. doi:10.3233/CBM-171160
Keywords: hepatocellular carcinoma, diagnosis, prognosis, gene signature, blood, transcriptome, early HCC, gene network
Citation: Al-Harazi O, Kaya IH, Al-Eid M, Alfantoukh L, Al Zahrani AS, Al Sebayel M, Kaya N and Colak D (2021) Identification of Gene Signature as Diagnostic and Prognostic Blood Biomarker for Early Hepatocellular Carcinoma Using Integrated Cross-Species Transcriptomic and Network Analyses. Front. Genet. 12:710049. doi: 10.3389/fgene.2021.710049
Received: 11 June 2021; Accepted: 09 September 2021;
Published: 29 September 2021.
Edited by:
PriyankaBaloni, Institute for Systems Biology (ISB), United StatesReviewed by:
Jyothipadiadpu, Oregon State University, United StatesBhanwarLalPuniya, University of Nebraska-Lincoln, United States
Copyright © 2021 Al-Harazi, Kaya, Al-Eid, Alfantoukh, Al Zahrani, Al Sebayel, Kaya and Colak. This is an open-access article distributed under the terms of the Creative Commons Attribution License (CC BY). The use, distribution or reproduction in other forums is permitted, provided the original author(s) and the copyright owner(s) are credited and that the original publication in this journal is cited, in accordance with accepted academic practice. No use, distribution or reproduction is permitted which does not comply with these terms.
*Correspondence: Dilek Colak, ZGNvbGFra2F5YUBrZnNocmMuZWR1LnNh
†These authors have contributed equally to this work and share first authorship