- 1Department of Epidemiology, University of Alabama at Birmingham, Birmingham, AL, United States
- 2Department of Biostatistics, University of Alabama at Birmingham, Birmingham, AL, United States
- 3Department of Neurology, University of Alabama at Birmingham, Birmingham, AL, United States
- 4Division of Biomedical Informatics and Personalized Medicine, Department of Medicine, University of Colorado Anschutz Medical Campus, Aurora, CO, United States
- 5College of Public Health, University of Kentucky, Lexington, KY, United States
Background: African Americans (AAs) suffer a higher stroke burden due to hypertension. Identifying genetic contributors to stroke among AAs with hypertension is critical to understanding the genetic basis of the disease, as well as detecting at-risk individuals.
Methods: In a population comprising over 10,700 AAs treated for hypertension from the Genetics of Hypertension Associated Treatments (GenHAT) and Reasons for Geographic and Racial Differences in Stroke (REGARDS) studies, we performed an inverse variance-weighted meta-analysis of incident stroke. Additionally, we tested the predictive accuracy of a polygenic risk score (PRS) derived from a European ancestral population in both GenHAT and REGARDS AAs aiming to evaluate cross-ethnic performance.
Results: We identified 10 statistically significant (p < 5.00E-08) and 90 additional suggestive (p < 1.00E-06) variants associated with incident stroke in the meta-analysis. Six of the top 10 variants were located in an intergenic region on chromosome 18 (LINC01443-LOC644669). Additional variants of interest were located in or near the COL12A1, SNTG1, PCDH7, TMTC1, and NTM genes. Replication was conducted in the Warfarin Pharmacogenomics Cohort (WPC), and while none of the variants were directly validated, seven intronic variants of NTM proximal to our target variants, had a p-value <5.00E-04 in the WPC. The inclusion of the PRS did not improve the prediction accuracy compared to a reference model adjusting for age, sex, and genetic ancestry in either study and had lower predictive accuracy compared to models accounting for established stroke risk factors. These results demonstrate the necessity for PRS derivation in AAs, particularly for diseases that affect AAs disproportionately.
Conclusion: This study highlights biologically plausible genetic determinants for incident stroke in hypertensive AAs. Ultimately, a better understanding of genetic risk factors for stroke in AAs may give new insight into stroke burden and potential clinical tools for those among the highest at risk.
Introduction
As the second-leading global cause of death and a leading cause of disability-adjusted life-years lost (Katan and Luft, 2018), stroke is a major public health burden especially among African Americans (AAs) who have a 50% higher risk of stroke (Howard et al., 2011). The role of genetics on stroke risk has been evidenced through twin studies, where monozygotic twins are more likely to be concordant than dizygotic twins (odds ratio for concordance (OR): 1.65, 95% CI: 1.2–2.3) (Flossmann et al., 2004). Previously reported heritability estimates of stroke based on data from genome-wide association studies (GWAS) are comparable for AAs and individuals of European Ancestry (EAs); 38% for EAs (Bevan et al., 2012) and 35% for AAs (Traylor et al., 2017), yet AAs contribute far less data to a large body of literature on stroke genetic risk factors (Bentley et al., 2020).
GWAS of stroke in European populations have identified susceptibility loci located on chromosomes 4q25 and 9p21 (Gretarsdottir et al., 2008), 7p21.1 (International Stroke Genetics, 2012), 6p21 (Holliday et al., 2012), 12p13 (Ikram et al., 2009), 12q24 (Kilarski et al., 2014), and 16q22 (Traylor et al., 2012). Overall, there has been a lack of similar primary GWAS discovery efforts in AAs, and results from EAs have not generally replicated in AAs (Carty et al., 2015; Peprah et al., 2015). For example, of 520,000 participants in the large trans-ethnic GWAS meta-analysis from the MEGASTROKE consortium, only ∼4% of participants were AAs (Malik et al., 2018). The only other study with a large number of AAs, the Consortium of Minority Population Genome-Wide Association Studies of Stroke (COMPASS), confirmed the need for race-specific stroke discovery, finding weak or no validation across ethnic groups (Carty et al., 2015; Keene et al., 2020). Given the relative lack of data on this race group in the published literature, additional stroke variant discovery in this population remains needed.
GWAS data capitalizes on the polygenic nature of common diseases and has been collected on a large scale to provide useful health information over traditional clinical risk factors. So far GWAS data at the single variant level, even from large consortia studies such as those described above, has been difficult to translate to the clinic. More recently, cumulative single variant effects from GWAS, at thousands to millions of variants, are being used to estimate polygenic risk scores (PRS), which may prove useful for stroke risk prediction and prevention. Unfortunately, few stroke PRS have been developed in populations including AAs and, to the best of our knowledge, only one PRS was developed exclusively in individuals of African descent, consisting of only 29 variants (Traylor et al., 2017).
Abraham et al. (2019) comprised a composite meta-risk PRS (metaPRS) for ischemic stroke leveraging publicly available GWAS data for stroke and 19 stroke-related phenotypes generated from the British European dataset of the United Kingdom Biobank (UKB, n = 407,388). Under a split sample design, participants with ‘any stroke’ event were oversampled for the derivation set (n = 11,995 total; n = 2,065 with “any stroke” events) followed by validation in the remaining ∼395,000 participants. The authors did not report on score validation in the small sample of available individuals of African descent from the UKB (N∼8,000). Given difficulties in cross-ethnic validation of stroke variants in GWAS, we suspected that the publicly available Abraham composite metaPRS will not perform adequately in AAs.
The goals of the current study, which we set among AAs at elevated risk for stroke due to hypertension (HTN), were two-pronged. First, we aimed to increase stroke GWAS data available in this race group using data from the Genetics of Hypertension Associated Treatments (GenHAT) study and the Reasons for Geographic and Racial Differences in Stroke (REGARDS) studies. Second, we aimed to assess the predictive ability of the UKB derived stroke PRS (Abraham et al., 2019) in these two populations given the lack of previous cross-ethnic validation.
Materials and Methods
Study Participants
GenHAT was an ancillary pharmacogenetic study to the Antihypertensive and Lipid-Lowering Treatment To Prevent Heart Attack Trial (ALLHAT) and REGARDS is an ongoing cohort study of stroke risk in the continental US. The GenHAT and REGARDS studies contributed genetic and phenotypic data on 10,717 AAs with HTN at baseline (GenHAT n = 6,908; REGARDS n = 3,809), further detailed in the Supplementary Material. Participants in GenHAT were randomized to either chlorthalidone, a thiazide diuretic (TD), or lisinopril, an angiotensin-converting enzyme (ACE) inhibitor, while REGARDS participants were taking a TD, ACE inhibitor, or a combination of both for inclusion in the analysis (Supplementary Table S1). All studies were approved by local institutional review boards and/or ethics committees. All participants provided written informed consent.
Definition of Incident Stroke
In GenHAT, incident stroke was defined as the rapid onset of persistent neurologic deficit attributable to an obstruction or rupture of the arterial system, including stroke occurring during surgery, not known to be secondary to brain trauma, tumor, infection, or other non-ischemic cause and must last more than 24 h unless death supervenes or there is a demonstrable lesion compatible with acute stroke on CT or MRI scan (ALLHAT Protocol, 2000). In REGARDS, any suspected stroke events were identified every 6 months via telephone interview. The medical records associated with these events were retrieved and adjudicated by a physician, using the World Health Organization definition of stroke as focal neurologic symptoms lasting more than 24 h or those with neuroimaging data consistent with stroke (WHO Task Force on Stroke and other Cerebrovascular Disorders, 1989; Howard et al., 2011). For REGARDS, all stroke events occurring before or on September 30, 2019 were included in this analysis. REGARDS participants with a history of previous stroke were excluded.
Genotyping, Quality Control, and Imputation
Genome-wide genotyping was performed within each study independently using Illumina Infinium Multi-Ethnic AMR/AFR Extended BeadChip arrays (MEGA chip; Illumina, Inc., San Diego, CA). Supplementary Table S2 includes detailed information on the variant and sample quality control (QC). Filtered genotype calls were imputed using the NHLBI Trans-omics for Precision Medicine (TOPMed) release 2 (Freeze 8) reference panel, which leverages data on ∼186,000 samples (∼30% AA) (Fuchsberger et al., 2015; Das et al., 2016; Taliun et al., 2021). Post-imputation QC excluded variants with imputation quality scores (Rsq) < 0.3 and a Minor Allele Count (MAC) < 20 in each cohort, as previously described using TOPMed data (Sarnowski et al., 2021). The variants not represented in both GenHAT and REGARDS were excluded from subsequent analyses.
Statistical Analysis
Baseline descriptive statistics for cases and controls are presented as counts (percentages) for categorical variables or mean ± standard deviation (SD) for continuous variables, and were compared using χ2 tests and t-tests, respectively (Table 1).
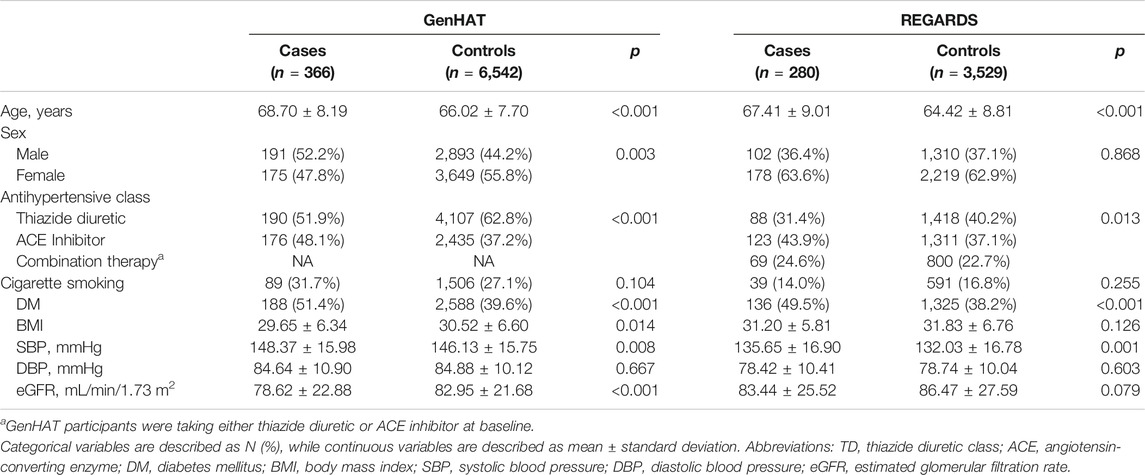
TABLE 1. Demographic characteristics of GenHAT and REGARDS participants stratified by incident stroke case-control status.
Firth logistic regression models implemented in PLINK2 (Ma et al., 2013; Chang et al., 2015) were used for genome-wide association analysis of incident stroke status. Models of the imputed effect allele dosage were adjusted for age, sex, and the top 10 principal components (PCs) for ancestry derived in EIGENSTRATv6.1.4 (Price et al., 2006). An inverse variance-weighted, fixed effects meta-analysis was performed on the summary statistics from GenHAT and REGARDS, using METAL software (Willer et al., 2010). Statistical heterogeneity was evaluated using Cochran’s chi-square test in METAL. Regional plots were created using LocusZoom v0.12 (Pruim et al., 2010; Boughton et al., 2021). Gene annotation was completed using ANNOVAR (Wang et al., 2010). Genome-wide significance was set at p < 5.00E-08 after a Bonferroni correction for multiple testing. A randomization drug-adjusted sensitivity model was performed in the GenHAT data to account for any effects of the antihypertensive agent, and the results were similar to the discovery model (data not shown). To address potential issues associated with case-control imbalance, we ran sensitivity analyses in the GenHAT and REGARDS data using the saddle point approximation (SPA) in the Scalable and Accurate Implementation of Generalized (SAIGE) program and meta-analyzed the results (Zhou et al., 2018). This approach provides good control of type 1 error for binary traits, however the SPA approach in SAIGE is very conservative and has been described to result in inflated effect-size estimates (Mbatchou et al., 2021). To determine if there are any shared genetic risk factors for stroke with EAs, we conducted a fixed effects, inverse-variance weighted meta-analysis incorporating top (p < 1.00E-07) variants (n = 356) from the publicly available MEGASTROKE European analysis summary statistics (Malik et al., 2018).
To analyze the putative biological mechanisms of a subset of significant and suggestive variants identified in the meta-analysis with p < 1.00E-06, we utilized the Functional Mapping and Annotation of Genome-Wide Association Studies (FUMA) version 1.3.6a online platform and the GENE2FUNC process (Watanabe et al., 2017). Within FUMA, over 22,000 genes underwent zero-mean normalization and log2 transformation of the expression values [zero mean of log2 (reads per kilobase per million +1)]. Differentially expressed gene (DEG) sets for each of the 53 specific tissue types from the Genotype-Tissue Expression (GTEx) project version eight RNA sequencing data (GTEx Consortium, 2017), were determined from two-sided Student’s t-test performed per gene per tissue type against all other tissue types. Genes with p ≤ 0.05 after Bonferroni correction and absolute log fold change of ≥0.58, were included in the DEG set for a given tissue (Watanabe et al., 2017). Furthermore, FUMA distinguished between genes that were upregulated and downregulated in a specific tissue type compared to other tissues via the sign of the t-statistic (Watanabe et al., 2017). Genes identified from the meta-analysis were uploaded into FUMA and mapped to Ensembl identifiers. Our prioritized genes were tested against biologically relevant tissue (brain n = 12, artery n = 3, heart n = 2, and kidney n = 2) DEG sets using hypergeometric tests to evaluate if the targeted genes were overrepresented in FUMA DEG sets in specific tissue types. Multiple testing correction was performed using a Benjamini-Hochberg adjustment. Statistical significance was calculated using a p-threshold of p < 0.05.
We utilized the publicly available risk score weights for the Abraham metaPRS to assess the predictive accuracy of this score in both GenHAT and REGARDS AAs (Abraham et al., 2019). Specifically, the PRS were generated separately for each study population using the allelic scoring option in PLINK2, resulting in the inclusion of 466,657 and 466,614 variants in GenHAT and REGARDS, respectively. We then employed a series of nine logistic regression models. Model 1 (reference model) regressed the ‘any stroke’ outcome on age, sex, and PCs 1–10. The metaPRS and clinical risk factors (SBP, DBP, DM, baseline cigarette smoking, or BMI) were individually added to Model 1. A clinical model added each clinical risk factor (SBP + DBP + DM + baseline cigarette smoking + BMI) to model 1. Lastly, we generated a composite model that consisted of Model one plus clinical factors and the metaPRS (SBP + DBP + DM + baseline cigarette smoking + BMI + metaPRS). Using the predicted values for stroke, the performance of each model was determined by the area under the receiver operator characteristic (ROC) curve (AUC). Delong’s test for two correlated ROC curves compared the pairwise performance of the nested models (i.e., model one to each of the subsequent models). The fit of each model was determined by Nagelkerke’s pseudo-R2. All predictive regression modeling and model fit calculations were performed in R version 3.6.2.
Replication for Meta-analysis
We sought replication in AAs from the University of Alabama at Birmingham Warfarin Pharmacogenomics Cohort (WPC) (Shendre et al., 2016; Yanik et al., 2017). The WPC replication population included 790 AAs, of which 145 had an incident stroke. In the WPC, incident stroke case-control status was regressed onto genotypes imputed to the TOPMed release 2 (Freeze 8) reference panel, adjusting for age, sex, the top 4 PCs, and genotyping platform using PLINK2. Further details are described in the Supplementary Material. We also expanded our replication lookups to the region around our index variants from the meta-analysis. Based on prior research, we considered a window within 100 KB of the target variant (Genomes Project et al., 2015).
Further replication was obtained using the publicly available summary statistics from the recent COMPASS meta-analysis study (Keene et al., 2020). In the COMPASS meta-analysis, 22,051 AAs were included, of which 3,734 had a physician-adjudicated stroke. Due to the imputation reference panel differences (TOPMed vs. 1,000 Genomes), we did not have sufficient overlap to replicate on the variant level. Our replication efforts examined all variants located in the gene region [5′ untranslated region (UTR) through the 3′ UTR] of the target variant, or in the case of intergenic variants, the entire region between the two flanking genes. Of note, there is an overlap of 864 participants (66 cases) from our discovery REGARDS population and those included in the published COMPASS meta-analysis of IS, which could inflate the results on a single-variant level.
Results
The baseline characteristics for GenHAT and REGARDS participants are presented in Table 1. In the 6,908 GenHAT participants, approximately 5% (n = 366) had an incident stroke. Males comprised approximately 52% of stroke cases and 44% of controls. The average age of cases was nearly 69 years of age, while the controls were younger at 66 years (p < 0.001). In GenHAT, stroke cases were more likely current cigarette smokers (32% v. 27%; p = 0.104) and diabetic (51% v. 40%; p < 0.001) compared to controls. Likewise, stroke cases had worse kidney function as estimated by the mean glomerular filtration rate (eGFR) (78.62 v. 82.95 ml/min/1.73 m2; p < 0.001), higher mean SBP (148.37 v. 146.13 mmHg; p = 0.008) and a negligible difference in mean DBP (84.64 v. 84.88 mmHg; p = 0.667) compared to controls.
In the 3,809 REGARDS participants, approximately 7% (n = 280) had an incident stroke. Males comprised approximately 36% of incident stroke cases versus 37% of controls. The average age of stroke cases was 67 vs. 64 years for controls (p < 0.001). Of stroke cases, 14% were current cigarette smokers compared to 17% of controls (p = 0.255), and almost 50% had DM versus 38% of controls (p < 0.001). The stroke cases had slightly higher baseline SBP (135.65 mmHg v. 132.03 mmHg; p = 0.001), lower baseline DBP (78.42 mmHg v. 78.74 mmHg; p = 0.603), and worse kidney function (83.44 ml/min/1.73 m2 v. 86.47 ml/min/1.73 m2; p = 0.079) compared to controls (Table 1).
In Table 2, we present 21 top variants (p < 1.00E-07) across nine unique genes, of which 10 variants were statistically significant (p < 5.00E-08) from the inverse variance-weighted meta-analysis (Figure 1). An additional 79 variants were marginally significant (p < 1.00E-06) and are described in Supplementary Table S3. In the sensitivity analysis using SAIGE, we observed consistent results in our top finding, although we saw marginal reduction in the significance (Supplementary Table S4). The most significant variant, rs117880209 (p = 6.45E-09), was located in the intergenic region between LINC01443 and LOC644669 on chromosome 18. At this variant the odds ratio for incident stroke was 2.98 (95% CI 2.06-4.31) for the C (v. T) allele and the direction of the effect was consistent across both GenHAT and REGARDS. An additional 10 variants in this intergenic region met or exceeded p < 1.00E-06.
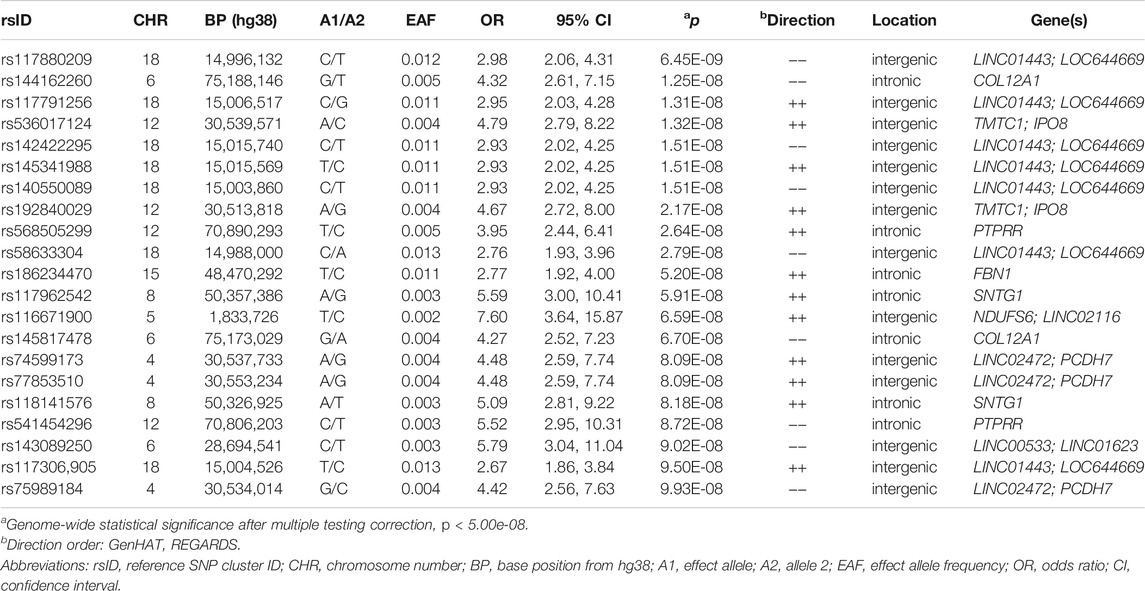
TABLE 2. Top variants (p < 1.00E-07) associated with incident stroke from inverse variance-weighted meta-analysis.
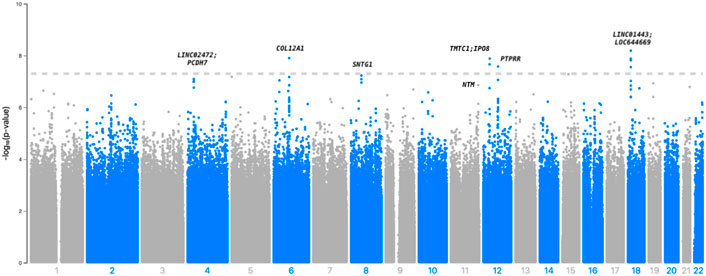
FIGURE 1. Manhattan plot depicting the top associations with incident stroke in GenHAT-REGARDS meta-analysis. The dotted line is representative of genome-wide significance (p < 5.00E-08).
Furthermore, we observed 13 intronic variants in COL12A1 on chromosome 6 with p < 1.00E-6. One intronic variant in this gene, rs144162260, reached statistical significance (p = 1.25E-08; OR = 4.32; 95% CI = 2.61–7.15). Three additional variants exceeded genome-wide significance, including two intergenic variants between TMTC1 and IP O 8 (top variant: rs536017124; p = 1.32E-08; OR = 4.79; 95% CI = 2.79–8.22), and one intronic variant of PTPRR (rs568505299; p = 2.64E-08, OR = 3.95, 95% CI = 2.44–6.41) (Table 2).
Other variants of biological interest include 11 intergenic variants between LINC02472 and PCDH7 (top variant: rs74599173, p = 8.09E-08), three intronic variants of SNTG1 (top variant: rs117962542, p = 5.91E-08), and two intronic variants of NTM (top variant: rs185159493, p = 1.37E-07) (Table 2; Supplementary Table S3). The genomic inflation factor (λ) from the individual cohorts (GenHAT λ = 0.952; REGARDS λ = 1.002) and the meta-analysis (λ = 0.992) showed no evidence of systematic inflation (Supplementary Table S5; Supplementary Figure S1). LocusZoom plots of the top variants in COL12A1, PTPRR, NTM, and the intergenic regions of LINC01443- LOC644669, TMTC1- IP O 8, and LINC02472- PCDH7, show that the suggestive variants within these regions are in moderate-to-strong linkage disequilibrium (LD) with the sentinel variant (Supplementary Figures S2–S7). Variant-specific LD estimates generated from the GenHAT/REGARDS data are shown in Supplementary Table S6.
None of our single variant results were replicated in individuals from the WPC at a threshold of p < 5.00E-04 (p = 0.05/100 suggestive variants from Table 2 and Supplementary Table S3). Of the 10 statistically significant variants, rs144162260 in COL12A1, rs536017124 and rs192840029 in the TMTC1-IPO8 intergenic region, and rs58633304 in the LINC01443-LOC644669 intergenic region had the same direction of effect but non-significant p-values (Supplementary Table S3). Our extended look-up of variants ±100 kb of the target variant, identified seven intronic variants within the NTM gene that had p-values <5E-04. These variants were all located within 60 kb from either rs185159493 or rs184866696 (Supplementary Table S7).
Our lookup efforts in the COMPASS summary statistics provided marginal support for replication at the gene level, as none of the variants from our discovery were identified in COMPASS, most likely due to differences in imputation reference panels. Genes of interest based on the meta-analysis results included COL12A1, NTM, PTPRR, and SNTG1, as well as the LINC01443-LOC64466, LINC02472-PCDH7, and TMTC1-IPO8 intergenic regions. While we could not replicate our meta-analysis findings on the single-variant level, rs192315401, an upstream variant of the LINC02472-PCDH7 reached nominal significance in COMPASS (Supplementary Table S8, p = 3.20E-04). Results from the meta-analysis of MEGASTROKE top findings (N = 356 variants with p < 1.00E-07) with our AA data are presented in Supplementary Table S9. The top findings from MEGASTROKE were not significant in our data and the race-combined meta-analysis did not notably change any MEGASTROKE findings. We specifically focused on variants located within two well-characterized stroke loci: PITX2 (n = 118) and HDAC9 (n = 7). For the 118 PITX2 variants, 102 had a consistent direction of effect across all three cohorts, while the remaining 16 were consistent across MEGASTROKE and REGARDS. In the HDAC9 gene locus, all seven variants had the same direction of effect across all three cohorts (Supplementary Table S9).
We utilized FUMA to identify any tissue specificity of genes represented in our top findings. Of 64 unique gene names identified among variants with p < 1.00E-06, 57 were mapped to Ensembl identifiers by FUMA. Tissue analysis on an a priori selected 19 specific tissue types from GTEx revealed statistically significant, differential upregulation in brain tissues, specifically the hippocampus (padj = 9.67E-05), hypothalamus (padj = 2.05E-03), amygdala (padj = 5.28E-03), frontal cortex BA9 (padj = 6.36E-03), putamen basal ganglia (padj = 1.56E-02), anterior cingulate cortex BA24 (padj = 1.62E-02), and the caudate basal ganglia (padj = 3.17E-02) (Supplementary Table S10).
Accuracy in predicting incident stroke was assessed in GenHAT and REGARDS separately, using variant weights for over 400,000 overlapping variants in the UKB metaPRS (Abraham et al., 2019) (Supplementary Figure S8). In GenHAT, model 1 (AUC 62.68%; 95% CI 59.89–65.48%) was not statistically different than the model adding the metaPRS (Model 1 + metaPRS; AUC 62.68%; 95% CI 59.89–65.48%; p = 0.981). However, the models adding DM (Model 1 + DM; AUC 64.64%; 95% CI 61.68–67.43%; p = 0.025), all the clinical factors (Model 1 + All Clinical Factors; AUC 66.44%; 95% CI 63.29–69.60%; p = 0.003), and all the clinical factors plus the metaPRS (Model 1 + All Clinical Factors + metaPRS; AUC 66.46%; 95% CI 63.31–69.62; p = 0.003) were significantly different from the reference (i.e., Model one; Supplementary Table S11). Less than 0.01% of the variance was attributed to the metaPRS in GenHAT based on the difference of Nagelkerke pseudo-R2 values between the reference and metaPRS models (Figure 2; Supplementary Table S11). Similar results were observed in the REGARDS population. The reference model 1 (AUC 60.88%; 95% CI 57.45–64.30%) was not statistically different to the metaPRS model (AUC 60.87%; 95% CI 57.45–64.30%; p = 0.856). The models adding DM (AUC 62.94%; 95% CI 59.57–66.32%; p = 0.035), all the clinical factors (AUC 64.15%; 95% CI 60.76–67.54; p = 0.006), and all the clinical factors plus the metaPRS (AUC 64.16%; 95% CI 60.77–67.54; p = 0.006) were statistically significant, while the model adding SBP (AUC 62.37%; 95% CI 58.94–65.80%; p = 0.071) was marginally significant. Likewise, less than 0.001% of the variance was explained by adding the risk score for REGARDS based on the pseudo-R2 values between the reference and metaPRS model (Figure 2; SupplementaryTable S12).
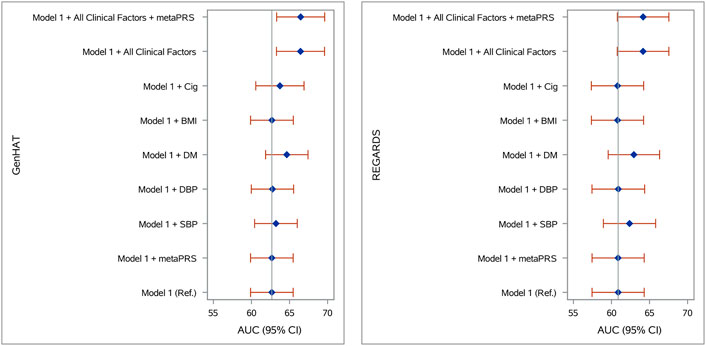
FIGURE 2. Stroke prediction model comparison in (A) GenHAT and (B) REGARDS populations. Shown are the area under the receiver operator characteristic curve (AUC) for eight logistic regression models (with and without the Abraham et al. metaPRS and clinical risk factors) used to predict stroke risk in comparison to a basic model 1 (age + sex +10 ancestry PCs). Error bars represent the 95% confidence intervals of the AUC. Abbreviations: PRS, polygenic risk score; Ref, reference model; SBP, systolic blood pressure; DBP, diastolic blood pressure; DM, diabetes mellitus; BMI, body mass index, kg/m2; Cig, cigarette smoking at baseline.
Discussion
While numerous stroke outcome GWAS have been published in the past several years, few studies have been performed exclusively in AAs. Using data from over 10,700 individuals from the GenHAT and REGARDS studies, we identified 10 statistically significant and an additional 90 suggestive genetic variants associated with incident stroke in individuals with HTN. While none of our findings were directly replicated, their gene level associations with stroke warrant future replication efforts, particularly variants located in or near the NTM or PCDH7 genes.
In our meta-analysis, we identified 10 statistically significant variants, including six intergenic variants between a long-intergenic non-protein coding RNA (LINC01443) and a pseudogene (LOC644669). Four of these six variants were identified in a 2020 intracranial aneurysm (IA) GWAS in an East Asian population (Bakker et al., 2020). Additional significant findings include those variants located in COL12A1. This gene encodes the collagen alpha chain of type XII collagen, which interacts with type 1 collagen-containing fibrils (UniProt, 2021), and is a predicted target of the human microRNA-21, which is induced by Angiotensin II (Wang et al., 2017). Angiotensin II is the principal effector hormone of the renin-angiotensin system and increases blood pressure through vasoconstriction and increased sodium and water retention (de Leeuw, 1999), which is clinically relevant to our hypertensive study base.
Of additional interest were the variants downstream of the TMTC1 (transmembrane O-Mannosyltransferase Targeting Cadherins 1) gene. TMTC1 encodes a protein that transfers mannosyl residues to the hydroxyl group of serine or threonine residues and is primarily dedicated to the cadherin superfamily (UniProt, 2021). In a prior meta-analysis, variants in TMTC1 were associated with heart failure (HF) in African ancestry populations (Smith et al., 2010). Other TMTC1 variants have been associated with lipid metabolism (Talmud et al., 2009; Della-Morte et al., 2011). A 2011 study using data from the Northern Manhattan Stroke Study identified an interaction of TMTC1 with abdominal obesity contributing to phenotypic variation in left ventricular mass (LVM). Increased LVM is a known risk factor for HF, stroke, and CVD (Della-Morte et al., 2011).
The only variants with gene-based replication were located in the first intron of NTM and upstream of PCDH7. NTM encodes a member of the IgLON glycosylphosphatidylinositol-anchored cell adhesion molecular family (Gene, 2004) and is primarily expressed in the heart and lungs (GTEx Consortium, 2015). A 2012 study found a balanced translocation break in NTM in a family with intracranial and thoracic aortic aneurysms (Luukkonen et al., 2012). Furthermore, intronic NTM variants have been previously implicated in intracerebral hemorrhage and small vessel ischemic stroke in Europeans (Chung et al., 2019). Additional studies have reported associations between NTM and CVD risk factors such as triglyceride levels (Li et al., 2015) and elevated protein levels in plasma serum (Cao et al., 2015). A recent study from the International Consortium for Antihypertensive Pharmacogenomics Studies concluded that variation in the NTM gene is associated with an increased risk of adverse cardiovascular outcomes in patients treated with beta-blockers, as well as an increase in blood pressure after beta-blocker treatment (McDonough et al., 2021). Although not statistically significant, we identified 11 variants located upstream of the PCDH7 (protocadherin 7) gene. In previous studies, PCDH7 was differentially expressed in aneurysm wall tissue compared to superficial temporal artery tissue (Shi et al., 2009), as well as being associated with white matter hyperintensities in EAs with ischemic stroke (Traylor et al., 2016).
Of the three marginally associated intronic variants within the SNTG1 (syntrophin gamma-1) gene, rs117962542 has been previously implicated with stroke risk in a sample of ∼70,000 individuals of European descent from MEGASTROKE (Malik et al., 2018). SNTG1 encodes a protein that mediates gamma-enolase trafficking to the plasma membrane and enhances its neurotrophic activity (UniProt, 2021). An epistasis analysis performed in 2,800 EAs found an association between an SNTG1 variant and a history of arterial HTN (Zhou et al., 2020). Tissue specificity of our top identified genes, specifically SNTG1 and NTM, showed differential expression in specific brain tissues, compared to other available tissues from GTEx RNA sequence data.
Complex diseases, such as stroke, have shown additive genetic architecture in previous association studies, making PRS a widely lucrative approach. PRS have been utilized to estimate an individual’s lifetime genetic risk of disease. While the current discriminative ability is low in the overall population, PRS may be useful in populations where there is a higher probability of disease to assist in prevention or diagnosis, or to inform treatment choices (Lewis and Vassos, 2020). Currently, there are several pitfalls of PRS implementation (Arnold and Koenig, 2021). One of the most impactful is the shortage of data describing PRS performance in African populations, especially since differences in allele frequencies and/or LD may limit cross-ethnic utility (Martin et al., 2019). Our data show that the application of the genome-wide Abraham metaPRS (derived and validated in >400K EAs) to >10,000 AAs does not aid in stroke prediction beyond age, sex, and genetic ancestry (reference model). We chose to test the Abraham metaPRS compared to other previously published PRS due to the metaPRS being similarly predictive to several stroke risk factors, including family history, BP, BMI, and smoking, as well as reports that the metaPRS (HR 1.26; 95% CI 1.22–1.31 per SD) doubled the predictive accuracy of ischemic stroke compared to the 90-variant score (HR 1.13, 95% CI 1.10–1.17) (Rutten-Jacobs et al., 2018; Abraham et al., 2019) in EAs. In both GenHAT and REGARDS, the models accounting for clinical risk factors (SBP, DBP, DM, BMI, and cigarette smoking) had the highest predictive accuracy and a negligible improvement was observed when adding the metaPRS. This suggests that Abraham score utility is not transferable to AA adults. Therefore, there remains a strong need for the generation and validation of stroke scores in minority populations, specifically AAs.
This study has several strengths. Both GenHAT and REGARDS collected adjudicated stroke data on a large sample of AAs with HTN who are disproportionally at-risk for stroke. We also utilized contemporary genotyping and imputation methods designed to be more inclusive for research in minority populations, allowing for more accurate genetic interrogation of our population (e.g., linkage patterns). We also must note some weaknesses. We were unable to focus on stroke type or sub-type due to a lack of that data in ALLHAT/GenHAT. Additionally, because of our inclusion/exclusion criteria focused on HTN, our findings may not be generalizable to younger, healthier populations, or individuals taking other antihypertensives (i.e., not a thiazide diuretic or ACE inhibitor). With our study base we may not find HTN genes related to stroke. However, this is an issue in older AA population studies of stroke in general as the prevalence of HTN is high (e.g., ∼70% in the parent REGARDS study). This could also be reflected in the FUMA results, where there was limited differential expression in vascular, non-brain tissues (e.g., artery, heart). Additionally, the GTEx data used in the FUMA analysis is comprises only 12.9% AA samples. Also, the lack of single-variant replication in the WPC was limited by the lower allele frequency variants from the discovery being underrepresented in the smaller sample size of the WPC. Finally, while the relatively recent and more racially inclusive TOPMed imputation panel allowed for interrogation of millions of novel variants, many of which were of lower allele frequency, it limited our replication efforts in published data that was imputed into earlier reference datasets, which highlights the necessity for additional stroke datasets in AAs.
In conclusion, we identified 10 statistically significant variants associated with incident stroke in AAs with HTN. The individual variants were not independently validated in the WPC or previously reported. However, many gene regions were biologically plausible and we found gene-based validation in previously published data. When accounting for case-control imbalance using SAIGE, our top findings remained significant and the same direction of the effect size was observed. This highlights the need for additional validation in large, stroke studies with AA populations and contemporary methods (e.g., use of whole genome sequence data and/or TOPMed imputed data) to capture genetic variation in non-European populations. The majority of the results identified genes (NTM, PCDH7, COL12A1) related to stroke or other CVD related diseases in Asian and European ancestral populations, with the exception of one region near TMTC1. This suggests both ancestry-common and ancestry-specific stroke risk genes are present in AAs. As hypothesized, this will necessitate discovery efforts to be more inclusive as genetic diagnostics trained on GWAS data are considered for use in the clinic. To that point, the published Abraham composite metaPRS trained in a large sample of EAs was not validated in the REGARDS or GenHAT study AAs. This research highlights the need to collect additional data through large biobanks and consortia efforts that can alleviate the potential for genetic discovery disparities.
Data Availability Statement
The raw GenHAT genotypic and phenotypic data used in this study are deposited in the National Center for Biotechnology Information (NCBI) Database for Genotypes and Phenotypes (dbGaP), accession number phs002716.v1.p1. The raw REGARDS genotype and phenotype data used in this study can be found in dbGaP, accession number phs002719.v1.p1.
Ethics Statement
The studies involving human participants were reviewed and approved by the University of Alabama at Birmingham Institutional Review Board. The patients/participants provided their written informed consent to participate in this study.
Author Contributions
NA, BH, HT, DA, and MI contributed to the conception and design of the study. NA, RT, BH, HT, NL, EL, LL, DA, and MI contributed to the interpretation of results. MI, LL, and NL provided funding for the study. NA, VS, and AP performed the statistical analysis. NA wrote the first draft of the manuscript. All authors contributed to the manuscript revisions, read, and approved the final, submitted version.
Funding
The study was supported by the National Institutes of Health (NIH) National Heart, Lung, and Blood Institute (NHLBI) grants R01HL123782 (MI) and R01HL136666 (MI, LL). NA was supported by an NIH NHLBI T32 Fellowship (T32HL007457). The Warfarin Pharmacogenomics Cohort was supported by NHLBI grant R01HL092173 (NL). The REGARDS study is supported by a cooperative agreement U01 NS041588 from the National Institute of Neurological Disorders and Stroke, National Institutes of Health, U.S. Department of Health and Human Services. The content is solely the responsibility of the authors and does not necessarily represent the official views of the National Institute of Neurological Disorders and Stroke or the National Institutes of Health. Representatives of the funding agency have been involved in the review of the manuscript but not directly involved in the collection, management, analysis, or interpretation of the data.
Conflict of Interest
The authors declare that the research was conducted in the absence of any commercial or financial relationships that could be construed as a potential conflict of interest.
Publisher’s Note
All claims expressed in this article are solely those of the authors and do not necessarily represent those of their affiliated organizations, or those of the publisher, the editors and the reviewers. Any product that may be evaluated in this article, or claim that may be made by its manufacturer, is not guaranteed or endorsed by the publisher.
Acknowledgments
The authors thank the other investigators, the staff, and the participants of the REGARDS study for their valuable contributions. A full list of participating REGARDS investigators and institutions can be found at http://www.regardsstudy.org. The MEGASTROKE project received funding from sources specified at http://www.megastroke.org/acknowledgments.html.
Supplementary Material
The Supplementary Material for this article can be found online at: https://www.frontiersin.org/articles/10.3389/fgene.2021.781451/full#supplementary-material
References
Abraham, G., Malik, R., Yonova-Doing, E., Salim, A., Wang, T., and Danesh, J. (2019). Genomic Risk Score Offers Predictive Performance Comparable to Clinical Risk Factors for Ischaemic Stroke. Nat. Commun. 10(1), 5819. doi:10.1038/s41467-019-13848-1
ALLHAT Protocol (2000). Antihypertensive and Lipid Lowering Treatment to Prevent Heart Attack Trial. (ALLHAT) Protocol [Online]. Available: https://ccct.sph.uth.tmc.edu/ALLHAT/Documents/Protocol.pdf.
Arnold, N., and Koenig, W. (2021). Polygenic Risk Score: Clinically Useful Tool for Prediction of Cardiovascular Disease and Benefit from Lipid-Lowering Therapy? Cardiovasc. Drugs Ther. 35 (3), 627–635. doi:10.1007/s10557-020-07105-7
Bakker, M. K., van der Spek, R. A. A., van Rheenen, W., Morel, S., Bourcier, R., Hostettler, I. C., et al. (2020). Genome-wide Association Study of Intracranial Aneurysms Identifies 17 Risk Loci and Genetic Overlap with Clinical Risk Factors. Nat. Genet. 52 (12), 1303–1313. doi:10.1038/s41588-020-00725-7
Bentley, A. R., Callier, S. L., and Rotimi, C. N. (2020). Evaluating the Promise of Inclusion of African Ancestry Populations in Genomics. NPJ Genom Med. 5, 5. doi:10.1038/s41525-019-0111-x
Bevan, S., Traylor, M., Adib-Samii, P., Malik, R., Paul, N. L., Jackson, C., et al. (2012). Genetic Heritability of Ischemic Stroke and the Contribution of Previously Reported Candidate Gene and Genomewide Associations. Stroke 43 (12), 3161–3167. doi:10.1161/STROKEAHA.112.665760
Boughton, A. P., Welch, R. P., Flickinger, M., VandeHaar, P., Taliun, D., Abecasis, G. R., et al. (2021). LocusZoom.js: Interactive and Embeddable Visualization of Genetic Association Study Results. Bioinformatics. doi:10.1093/bioinformatics/btab186
Cao, T. H., Quinn, P. A., Sandhu, J. K., Voors, A. A., Lang, C. C., Parry, H. M., et al. (2015). Identification of Novel Biomarkers in Plasma for Prediction of Treatment Response in Patients with Heart Failure. Lancet 385 (Suppl. 1), S26. doi:10.1016/S0140-6736(15)60341-5
Carty, C. L., Keene, K. L., Cheng, Y. C., Meschia, J. F., Chen, W. M., Nalls, M., et al. (2015). Meta-Analysis of Genome-wide Association Studies Identifies Genetic Risk Factors for Stroke in African Americans. Stroke 46 (8), 2063–2068. doi:10.1161/STROKEAHA.115.009044
Chang, C. C., Chow, C. C., Tellier, L. C., Vattikuti, S., Purcell, S. M., and Lee, J. J. (2015). Second-generation PLINK: Rising to the challenge of Larger and Richer Datasets. Gigascience 4, 7. doi:10.1186/s13742-015-0047-8
Chung, J., Marini, S., Pera, J., Norrving, B., Jimenez-Conde, J., Roquer, J., et al. (2019). Genome-wide Association Study of Cerebral Small Vessel Disease Reveals Established and Novel Loci. Brain 142 (10), 3176–3189. doi:10.1093/brain/awz233
Das, S., Forer, L., Schonherr, S., Sidore, C., Locke, A. E., Kwong, A., et al. (2016). Next-generation Genotype Imputation Service and Methods. Nat. Genet. 48 (10), 1284–1287. doi:10.1038/ng.3656
de Leeuw, P. W. (1999). How Do Angiotensin II Receptor Antagonists Affect Blood Pressure? Am. J. Cardiol. 84 (2A), 5K–6K. doi:10.1016/s0002-9149(99)00399-9
Della-Morte, D., Beecham, A., Rundek, T., Wang, L., McClendon, M. S., Slifer, S., et al. (2011). A Follow-Up Study for Left Ventricular Mass on Chromosome 12p11 Identifies Potential Candidate Genes. BMC Med. Genet. 12, 100. doi:10.1186/1471-2350-12-100
Flossmann, E., Schulz, U. G., and Rothwell, P. M. (2004). Systematic Review of Methods and Results of Studies of the Genetic Epidemiology of Ischemic Stroke. StrokeAA 35 (1), 212–227. doi:10.1161/01.STR.0000107187.8439010.1161/01.STR.0000107187.84390.AA
Fuchsberger, C., Abecasis, G. R., and Hinds, D. A. (2015). minimac2: Faster Genotype Imputation. Bioinformatics 31 (5), 782–784. doi:10.1093/bioinformatics/btu704
Gene (2004). National Library of Medicine, National Center for Biotechnology Information. Available at: https://www.ncbi.nlm.nih.gov/gene/.
Genomes Project, C., Auton, A., Brooks, L. D., Durbin, R. M., Garrison, E. P., Kang, H. M., et al. (2015). A Global Reference for Human Genetic Variation. Nature 526 (7571), 68–74. doi:10.1038/nature15393
Gretarsdottir, S., Thorleifsson, G., Manolescu, A., Styrkarsdottir, U., Helgadottir, A., Gschwendtner, A., et al. (2008). Risk Variants for Atrial Fibrillation on Chromosome 4q25 Associate with Ischemic Stroke. Ann. Neurol. 64 (4), 402–409. doi:10.1002/ana.21480
GTEx Consortium (2017). Genetic Effects on Gene Expression across Human Tissues, Laboratory, D.A., Coordinating Center -Analysis Working, G., Statistical Methods groups-Analysis Working, Enhancing, G.g., Fund, N.I.H.C. Nature 550 (7675), 204–213. doi:10.1038/nature24277
GTEx Consortium (2015). Human Genomics. The Genotype-Tissue Expression (GTEx) Pilot Analysis: Multitissue Gene Regulation in Humans. Science 348 (6235), 648–660. doi:10.1126/science.1262110
Holliday, E. G., Maguire, J. M., Evans, T. J., Koblar, S. A., Jannes, J., Sturm, J. W., et al. (2012). Common Variants at 6p21.1 Are Associated with Large Artery Atherosclerotic Stroke. Nat. Genet. 44 (10), 1147–1151. doi:10.1038/ng.2397
Howard, V. J., Kleindorfer, D. O., Judd, S. E., McClure, L. A., Safford, M. M., Rhodes, J. D., et al. (2011). Disparities in Stroke Incidence Contributing to Disparities in Stroke Mortality. Ann. Neurol. 69 (4), 619–627. doi:10.1002/ana.22385
Ikram, M. A., Seshadri, S., Bis, J. C., Fornage, M., DeStefano, A. L., Aulchenko, Y. S., et al. (2009). Genomewide Association Studies of Stroke. N. Engl. J. Med. 360 (17), 1718–1728. doi:10.1056/NEJMoa0900094
International Stroke Genetics (2012). Genome-wide Association Study Identifies a Variant in HDAC9 Associated with Large Vessel Ischemic Stroke, Wellcome Trust Case Control, Bellenguez, C., Bevan, S., Gschwendtner, A., Spencer, C.C. Nat. Genet. 44 (3), 328–333. doi:10.1038/ng.1081
Katan, M., and Luft, A. (2018). Global Burden of Stroke. Semin. Neurol. 38 (2), 208–211. doi:10.1055/s-0038-1649503
Keene, K. L., Hyacinth, H. I., Bis, J. C., Kittner, S. J., Mitchell, B. D., Cheng, Y. C., et al. (2020). Genome-Wide Association Study Meta-Analysis of Stroke in 22 000 Individuals of African Descent Identifies Novel Associations with Stroke. Stroke 51 (8), 2454–2463. doi:10.1161/STROKEAHA.120.029123
Kilarski, L. L., Achterberg, S., Devan, W. J., Traylor, M., Malik, R., Lindgren, A., et al. (2014). Meta-analysis in More Than 17,900 Cases of Ischemic Stroke Reveals a Novel Association at 12q24.12. Neurology 83 (8), 678–685. doi:10.1212/WNL.0000000000000707
Lewis, C. M., and Vassos, E. (2020). Polygenic Risk Scores: from Research Tools to Clinical Instruments. Genome Med. 12 (1), 44. doi:10.1186/s13073-020-00742-5
Li, C., Bazzano, L. A., Rao, D. C., Hixson, J. E., He, J., Gu, D., et al. (2015). Genome-wide Linkage and Positional Association Analyses Identify Associations of Novel AFF3 and NTM Genes with Triglycerides: the GenSalt Study. J. Genet. Genomics 42 (3), 107–117. doi:10.1016/j.jgg.2015.02.003
Luukkonen, T. M., Poyhonen, M., Palotie, A., Ellonen, P., Lagstrom, S., Lee, J. H., et al. (2012). A Balanced Translocation Truncates Neurotrimin in a Family with Intracranial and Thoracic Aortic Aneurysm. J. Med. Genet. 49 (10), 621–629. doi:10.1136/jmedgenet-2012-100977
Ma, C., Blackwell, T., Boehnke, M., Scott, L. J., and Go, T. D. i. (2013). Recommended Joint and Meta-Analysis Strategies for Case-Control Association Testing of Single Low-Count Variants. Genet. Epidemiol. 37 (6), 539–550. doi:10.1002/gepi.21742
Malik, R., Chauhan, G., Traylor, M., Sargurupremraj, M., Okada, Y., Mishra, A., et al. (2018). Multiancestry Genome-wide Association Study of 520,000 Subjects Identifies 32 Loci Associated with Stroke and Stroke Subtypes. Nat. Genet. 50 (4), 524–537. doi:10.1038/s41588-018-0058-3
Mbatchou, J., Barnard, L., Backman, J., Marcketta, A., Kosmicki, J. A., Ziyatdinov, A., et al. (2021). Computationally Efficient Whole-Genome Regression for Quantitative and Binary Traits. Nat. Genet. 53 (7), 1097–1103. doi:10.1038/s41588-021-00870-7
McDonough, C. W. W., Jack, J. R., Motsinger-Reif, A. A., Armstrong, N. D., Bis, J. C., House, J. S., et al. (2021). Adverse Cardiovascular Outcomes and Antihypertensive Treatment: A Genome-wide Interaction Meta-Analysis in the International Consortium for Antihypertensive Pharmacogenomics Studies (ICAPS). Clin. Pharmacol. Ther. 110 (3), 723–732. doi:10.1002/cpt.2355
Peprah, E., Xu, H., Tekola-Ayele, F., and Royal, C. D. (2015). Genome-wide Association Studies in Africans and African Americans: Expanding the Framework of the Genomics of Human Traits and Disease. Public Health Genomics 18 (1), 40–51. doi:10.1159/000367962
Price, A. L., Patterson, N. J., Plenge, R. M., Weinblatt, M. E., Shadick, N. A., and Reich, D. (2006). Principal Components Analysis Corrects for Stratification in Genome-wide Association Studies. Nat. Genet. 38 (8), 904–909. doi:10.1038/ng1847
Pruim, R. J., Welch, R. P., Sanna, S., Teslovich, T. M., Chines, P. S., Gliedt, T. P., et al. (2010). LocusZoom: Regional Visualization of Genome-wide Association Scan Results. Bioinformatics 26 (18), 2336–2337. doi:10.1093/bioinformatics/btq419
Rutten-Jacobs, L. C., Larsson, S. C., Malik, R., and Rannikmae, K.MEGASTROKE consortium, International Stroke Genetics Consortium (2018). International Stroke GeneticsGenetic Risk, Incident Stroke, and the Benefits of Adhering to a Healthy Lifestyle: Cohort Study of 306 473 UK Biobank Participants. BMJ 363, k4168. doi:10.1136/bmj.k4168
Sarnowski, C., Chen, H., Biggs, M. L., Wassertheil-Smoller, S., Bressler, J., Irvin, M. R., et al. (2021). Identification of Novel and Rare Variants Associated with Handgrip Strength Using Whole Genome Sequence Data from the NHLBI Trans-omics in Precision Medicine (TOPMed) Program. PLoS One 16 (7), e0253611. doi:10.1371/journal.pone.0253611
Shendre, A., Brown, T. M., Liu, N., Hill, C. E., Beasley, T. M., Nickerson, D. A., et al. (2016). Race-Specific Influence of CYP4F2 on Dose and Risk of Hemorrhage Among Warfarin Users. Pharmacotherapy 36 (3), 263–272. doi:10.1002/phar.1717
Shi, C., Awad, I. A., Jafari, N., Lin, S., Du, P., Hage, Z. A., et al. (2009). Genomics of Human Intracranial Aneurysm wall. Stroke 40 (4), 1252–1261. doi:10.1161/STROKEAHA.108.532036
Smith, N. L., Felix, J. F., Morrison, A. C., Demissie, S., Glazer, N. L., Loehr, L. R., et al. (2010). Association of Genome-wide Variation with the Risk of Incident Heart Failure in Adults of European and African Ancestry: a Prospective Meta-Analysis from the Cohorts for Heart and Aging Research in Genomic Epidemiology (CHARGE) Consortium. Circ. Cardiovasc. Genet. 3 (3), 256–266. doi:10.1161/CIRCGENETICS.109.895763
Taliun, D., Harris, D. N., Kessler, M. D., Carlson, J., Szpiech, Z. A., Torres, R., et al. (2021). Sequencing of 53,831 Diverse Genomes from the NHLBI TOPMed Program. Nature 590 (7845), 290–299. doi:10.1038/s41586-021-03205-y
Talmud, P. J., Drenos, F., Shah, S., Shah, T., Palmen, J., Verzilli, C., et al. (2009). Gene-centric Association Signals for Lipids and Apolipoproteins Identified via the HumanCVD BeadChip. Am. J. Hum. Genet. 85 (5), 628–642. doi:10.1016/j.ajhg.2009.10.014
Traylor, M., Farrall, M., Holliday, E. G., Sudlow, C., Hopewell, J. C., Cheng, Y. C., et al. (2012). Genetic Risk Factors for Ischaemic Stroke and its Subtypes (The METASTROKE Collaboration): a Meta-Analysis of Genome-wide Association Studies. Lancet Neurol. 11 (11), 951–962. doi:10.1016/S1474-4422(12)70234-X
Traylor, M., Rutten-Jacobs, L., Curtis, C., Patel, H., Breen, G., Newhouse, S., et al. (2017). Genetics of Stroke in a UK African Ancestry Case-Control Study: South London Ethnicity and Stroke Study. Neurol. Genet. 3 (2), e142. doi:10.1212/NXG.0000000000000142
Traylor, M., Zhang, C. R., Adib-Samii, P., Devan, W. J., Parsons, O. E., Lanfranconi, S., et al. (2016). Genome-wide Meta-Analysis of Cerebral white Matter Hyperintensities in Patients with Stroke. Neurology 86 (2), 146–153. doi:10.1212/WNL.0000000000002263
UniProt, C. (2021). UniProt: the Universal Protein Knowledgebase in 2021. Nucleic Acids Res. 49 (D1), D480–D489. doi:10.1093/nar/gkaa1100
Wang, G., Wu, L., Chen, Z., and Sun, J. (2017). Identification of Crucial miRNAs and the Targets in Renal Cortex of Hypertensive Patients by Expression Profiles. Ren. Fail. 39 (1), 92–99. doi:10.1080/0886022X.2016.1244083
Wang, K., Li, M., and Hakonarson, H. (2010). ANNOVAR: Functional Annotation of Genetic Variants from High-Throughput Sequencing Data. Nucleic Acids Res. 38 (16), e164. doi:10.1093/nar/gkq603
Watanabe, K., Taskesen, E., van Bochoven, A., and Posthuma, D. (2017). Functional Mapping and Annotation of Genetic Associations with FUMA. Nat. Commun. 8 (1), 1826. doi:10.1038/s41467-017-01261-5
WHO Task Force on Stroke and other Cerebrovascular Disorders (1989). Stroke--1989. Recommendations on Stroke Prevention, Diagnosis, and Therapy. Report from the WHO Task Force on Stroke and Other Cerebrovascular Disorders. Stroke 20 (10), 1407–1431. doi:10.1161/01.str.20.10.1407
Willer, C. J., Li, Y., and Abecasis, G. R. (2010). METAL: Fast and Efficient Meta-Analysis of Genomewide Association Scans. Bioinformatics 26 (17), 2190–2191. doi:10.1093/bioinformatics/btq340
Yanik, M. V., Irvin, M. R., Beasley, T. M., Jacobson, P. A., Julian, B. A., and Limdi, N. A. (2017). Influence of Kidney Transplant Status on Warfarin Dose, Anticoagulation Control, and Risk of Hemorrhage. Pharmacotherapy 37 (11), 1366–1373. doi:10.1002/phar.2032
Zhou, J., Passero, K., Palmiero, N. E., Muller-Myhsok, B., Kleber, M. E., Maerz, W., et al. (2020). Investigation of Gene-Gene Interactions in Cardiac Traits and Serum Fatty Acid Levels in the LURIC Health Study. PLoS One 15 (9), e0238304. doi:10.1371/journal.pone.0238304
Keywords: incident stroke, hypertension, antihypertensives, disparities, polygenic risk score, genome wide association studies
Citation: Armstrong ND, Srinivasasainagendra V, Patki A, Tanner RM, Hidalgo BA, Tiwari HK, Limdi NA, Lange EM, Lange LA, Arnett DK and Irvin MR (2021) Genetic Contributors of Incident Stroke in 10,700 African Americans With Hypertension: A Meta-Analysis From the Genetics of Hypertension Associated Treatments and Reasons for Geographic and Racial Differences in Stroke Studies. Front. Genet. 12:781451. doi: 10.3389/fgene.2021.781451
Received: 22 September 2021; Accepted: 23 November 2021;
Published: 21 December 2021.
Edited by:
Alpo Juhani Vuorio, University of Helsinki, FinlandCopyright © 2021 Armstrong, Srinivasasainagendra, Patki, Tanner, Hidalgo, Tiwari, Limdi, Lange, Lange, Arnett and Irvin. This is an open-access article distributed under the terms of the Creative Commons Attribution License (CC BY). The use, distribution or reproduction in other forums is permitted, provided the original author(s) and the copyright owner(s) are credited and that the original publication in this journal is cited, in accordance with accepted academic practice. No use, distribution or reproduction is permitted which does not comply with these terms.
*Correspondence: Nicole D. Armstrong, bm1kYUB1YWIuZWR1