- 1State Key Laboratory of Quality Research in Chinese Medicines, Faculty of Chinese Medicine, Macau University of Science and Technology, Macau, China
- 2School of Pharmacy, Hangzhou Normal University, Hangzhou, Zhejiang, China
- 3Guangdong-Hong Kong-Macao Joint Laboratory for Contaminants Exposure and Health, Guangdong University of Technology, Guangzhou, Guangdong, China
- 4Key Laboratory of Elemene Class Anti-Cancer Chinese Medicines, Engineering Laboratory of Development and Application of Traditional Chinese Medicines, Collaborative Innovation Center of Traditional Chinese Medicines of Zhejiang Province, Hangzhou Normal University, Hangzhou, Zhejiang, China
- 5Zhuhai MUST Science and Technology Research Institute, Zhuhai, Guangdong, China
- 6Department of Gastrointestinal-Pancreatic Surgery, Zhejiang Provincial People’s Hospital, People’s Hospital of Hangzhou Medical College, Hangzhou, Zhejiang, China
- 7Key Laboratory of Gastroenterology of Zhejiang Province, Hangzhou, Zhejiang, China
Background: Recent studies demonstrate that N6-methyladenosine (m6A) methylation plays a crucial role in colorectal cancer (CRC). Therefore, we conducted a comprehensive analysis to assess the m6A modification patterns and identify m6A-modified genes in patients with CRC recurrence.
Methods: The m6A modification patterns were comprehensively evaluated by the NMF algorithm based on the levels of 27 m6A regulators, and tumor microenvironment (TME) cell-infiltrating characteristics of these modification patterns were systematically assessed by ssGSEA and CIBERSORT algorithms. The principal component analysis algorithm based on the m6A scoring scheme was used to explore the m6A modification patterns of individual tumors with immune responses. The weighted correlation network analysis and univariable and multivariable Cox regression analyses were applied to identify m6A-modified gene signatures. The single-cell expression dataset of CRC samples was used to explore the tumor microenvironment affected by these signatures.
Results: Three distinct m6A modification patterns with significant recurrence-free survival (RFS) were identified in 804 CRC patients. The TME characterization revealed that the m6A modification pattern with longer RFS exhibited robust immune responses. CRC patients were divided into high- and low-score subgroups according to the m6A score individually, which was obtained from the m6A-related signature genes. The patients with low m6A scores had both longer RFS and overall survival (OS) with altered immune cell infiltration. Notably, m6A-modified genes showed significant differences related to the prognosis of CRC patients in the meta-GEO cohort and TCGA cohort. Single-cell expression indicated that ALVRL1 was centrally distributed in endothelial tip cells and stromal cells.
Conclusion: The m6A modification plays an indispensable role in the formation of TME diversity and complexity. Importantly, the signatures (TOP2A, LRRC58, HAUS6, SMC4, ACVRL1, and KPNB1) were identified as m6A-modified genes associated with CRC recurrence, thereby serving as a promising predictive biomarker or therapeutic target for patients with CRC recurrence.
Introduction
Colorectal cancer (CRC) is the most common gastrointestinal malignancy and remains the main cause of cancer-related death worldwide (Siegel et al., 2021). Currently, the 5-year survival rate of CRC patients has been improved along with the development of new chemotherapeutics and advanced techniques. However, high recurrence and unsatisfactory prognosis are still major problems of CRC, due to delayed diagnosis and adverse drug effects (Sanoff et al., 2008; Hashiguchi et al., 2020). Currently, it has been recognized that CRC with recurrence is associated with genetic, genomic, and epigenetic changes (Lao and Grady, 2011). Therefore, identifying the crucial tumor biomarkers to predict the prognosis of CRC is urgently required.
N6-methyladenosine (m6A), as a reversible epigenetic reprogramming, is extensively modified in a variety of RNAs, comprising mRNAs, tRNAs, and snRNAs, as well as long-chain non-coding RNAs (Dominissini et al., 2012; Liang et al., 2020). m6A modification on RNA is abundant near the stop codon and 3-untranslated region (3-UTR) (Meyer et al., 2012; Ke et al., 2015) and translated near the 5-UTR in a cap-independent manner (Meyer et al., 2015), thereby regulating RNA transcription, translation, and metabolism. m6A modifications occur via signal transduction enzyme, methyltransferase, and demethylase, which are regarded as the “reader,” “writer,” and “eraser,” respectively. Specifically, “writers” can install the methyl to target RNAs, which include METTL3 (Schumann et al., 2016), METTL5 (Huang et al., 2022), METTL14 (Liu et al., 2014), WTAP (Ping et al., 2014), and RBM15/15B (Meyer and Jaffrey, 2017), and “erasers” mainly include FTO (Jia et al., 2011) and ALKBH5 (Zheng et al., 2013), which both selectively remove the methyl from certain RNAs. “Readers” such as YTHDC1, YTHDC2 (Haussmann et al., 2016), YTHDF1, YTHDF2, YTHDF3 (Huang et al., 2022), EIF3A (Meyer and Jaffrey, 2017), IGF2BP1, IGF2BP2, IGF2BP3 (Huang et al., 2018), HNRNPC (Huang et al., 2021), HNRNPA2B1 (Jiang et al., 2021), G3BP1, G3BP2 (Arguello et al., 2017), ELAVL1 (Pan et al., 2021), PRRC2A (Wu et al., 2019), and FMR1 (Worpenberg et al., 2021) can decipher the m6A methylation codes.
An increasing number of studies have revealed the relationship among the m6A modification, tumor microenvironment (TME) (Li et al., 2021), and chemotherapy resistance (Gu et al., 2021). Depletion of YTHDF1 in dendritic cells significantly enhances antigen presentation, resulting in CD8+T cell activation (Han et al., 2019). Macrophage-specific METTL14 knockout drives CD8+T cell differentiation in a dysfunctional direction, impairing the ability of CD8+T cells to eliminate tumors (Dong et al., 2021). METTL3 mediated gemcitabine, 5-fluorouracil, and cisplatin resistance in non-small-cell lung cancer and pancreatic cancer (Taketo et al., 2018; Jin et al., 2019). In addition, the m6A regulator-based methylation modification pattern led to different TME immune profiles in colorectal cancer (Chong et al., 2021). In addition, the relationship between m6A modification and TME characteristics and clinical prognosis in primary glioblastomas was elucidated completely by Cai et al. (2021). Therefore, m6A mRNA regulators and distinct modification patterns play an important role in the development or function of immune cells, which also promote resistance to chemotherapy and recurrence of the tumor. However, the relationship between m6A regulation and the recurrence status of CRC remains largely unknown.
In this study, we performed a comprehensive analysis of the expression of 27 m6A RNA methylation regulators using integrated data from the GEO database of patients with CRC. Afterward, consensus clustering analysis based on the gene expression of 27 m6A RNA methylation regulators was utilized to distinguish three different m6A modification subgroups. Then, the relationship between m6A modification patterns and immune infiltration related to CRC recurrence was systematically assessed. Furthermore, m6A regulators related to targeted mRNAs were screened by WGCNA analysis and regression analysis, and we identified a promising m6A-modified prognostic signature that can effectively predict the clinical outcomes of patients with CRC. Hence, our study suggests that the m6A methylation modification pattern contributes to the tumor immune microenvironment and has clinical prognostic value for colorectal cancer patients, providing novel insights into the diagnosis and treatment of CRC.
Materials and methods
Collection of publicly attainable expression datasets
The gene expression matrix and clinical traits of colorectal cancer patients were downloaded from the Gene Expression Omnibus database (https://www.ncbi.nlm.nih.gov/geo/), and a total of 804 patients were enrolled for analysis, including those from the GSE39582 (N = 558) (Marisa et al., 2013), GSE72970 (N = 95) (Del Rio et al., 2017), and GSE103479 (N = 151) (Allen et al., 2018). The ComBat method from the “SVA” R package was widely used to remove the batch effects between the different GEO datasets (Dai et al., 2018) to form meta-GEO datasets. Copy number variations of the TCGA-COAD were obtained from the UCSC Xena database (https://gdc-hub.s3.us-east-1.amazonaws.com/download/TCGA-COAD.cnv.tsv.gz). The package “Rcircos” was employed in R studio to plot the copy number variation landscape of 27 m6A regulators in human chromosomes. The clinical information and m6A regulator expression of the meta-GEO and the TCGA data are listed in Supplementary Tables S1, S2.
Non-negative matrix factorization clustering
Next, to assess the differences of m6A regulation between the different CRC clusters, the meta-GEO cohort was used for non-negative matrix factorization clustering, a method that can classify samples better than consensus clustering. Also then, the NMF rank survey and consensus heatmap were used to evaluate the optimal k value, and the meta-GEO cohort was divided into three clusters. Kaplan–Meier survival analysis was used to evaluate recurrence-free and overall survival between the different clusters.
Gene set variation analysis and GO analysis
We utilized GSVA analysis to identify underlying signaling pathways that are differentially stimulated behind the different m6A modification patterns in the meta-GEO cohort (Hänzelmann et al., 2013). The well-defined molecular biological signatures (h.all.v2022.1. Hs.symbols.gmt) were derived from the Hallmarker gene set (http://www.gsea/msigdb.org/gsea/msigdb) (Subramanian et al., 2005). In addition, Gene Ontology, a functional enrichment analysis, including three components: cellular components, biological processes, and molecular functions, was performed for understanding the underlying biological meaning of key genes extracted by WGCNA.
Estimation of immune cell infiltration by the ssGSEA and deconvolution algorithm
Initially, we used single-sample gene set enrichment analysis (ssGSEA) to quantify the relative abundance of 23 immune cell types behind the tumor microenvironment among the different m6A-modified clusters. Special feature gene panels for marking each immune cell type were curated from a recent study (Charoentong et al., 2017; Jia et al., 2018). The relative abundance of each immune cell type is represented by an enrichment fraction in the ssGSEA analysis and normalized to a uniform distribution. Afterward, CIBERSORT (Newman et al., 2015) (http://cibersort.stanford.edu/), a deconvolution approach, was used to estimate the abundances of 22 distinct leukocyte fractions with the gene expression profile of colorectal cancer patients individually, and participants with p value <0.05 were considered for differential analysis of leukocyte fraction between the m6A low-risk group and the m6A high-risk group.
Extraction of mRNAs from transcription profiles
Three microarray datasets from different platforms were matched with each GPL annotation file to explore m6A-targeted mRNAs. The probes for GSE39582 and GSE72970 are extracted from the Affymetrix HG-U133_Plus 2.0 microarray, and for GSE103479, the probes are extracted from the Almac Diagnostics Custom Xcel array. Subsequently, we extracted probe sets annotated with “protein coding” in the GENCODE project by matching the GENCODE (release 39). Finally, a total of 16,346 mRNAs in the meta-GEO cohorts were obtained for subsequent analysis.
Weighted correlation network analysis
First, Pearson’s correlation analysis between 27 m6A regulators and m6A-targeted mRNAs was performed, and 6,771 m6A-targeted mRNAs were identified (cor > 0.3, p < 0.05). Then, weighted correlation network analysis (WGCNA) was performed to acquire the required gene modules based on the gene expressions and patient traits by using the R “WGCNA” package (Langfelder and Horvath, 2008). First, a soft thresholding power value was calculated to produce a scale-free network topology. Hereafter, one-step network construction and detection of consensus modules were executed. Also, the similarity modules were assigned. Finally, correlations between clinical traits (age, sex, recurrence events, recurrence-free survival, status, and OS) and each module were calculated.
Identification of the m6A-modified gene with prognostic value
Univariable and multivariable Cox regression analyses were utilized to narrow the gene range and maximize the accuracy (Huang et al., 2018), Subsequently, we selected the meaning genes from the multivariate Cox regression analysis and analyzed the overall survival and recurrence-free survival probability of these genes in the meta-GEO and the TCGA cohort.
Single-cell RNA-seq analysis
To explore the tumor microenvironment affected by m6A-modified signatures, we downloaded the cell plots of single-cell sequencing of colorectal tumor samples and adjacent non-tumor samples from Single-Cell Expression Altas ((https://www.ebi.ac.uk/gxa/sc/home), and the parameters of drawing path are as follows: plot type: UMAP, ploy options: n_neibors:100, color plot by: ontology labels, gene names: by ALVRL1 and HAUS6.
Statistical analyses
Statistical analyses in this study were performed using R-4.1.2. Student's t-test or Wilcoxon rank-sum test was used to estimate the quantitative data for normally distributed or non-normally distributed data, respectively. The Kruskal–Wallis test and one-way analysis of variance were used for the comparison of the three distinct groups for the non-parametric and parametric data, respectively. The association between the m6A cluster and prognosis, risk group, and prognosis was analyzed by Kaplan–Meier survival analysis and the Cox proportional hazard model with the R package “Survminer” (0.4.9). The survival-cutoff function from the “survival” package in R studio was applied to stratify CRC patients into low-risk and high-risk subgroups. The alpha level for all comparisons was 0.05, and the Benjamini–Hochberg method was applied to control for the false discovery rate for multiple hypothesis testing procedures (Hazra and Gogtay, 2016).
Results
The landscape of expression variation of m6A regulators in colorectal cancer recurrence patients
In this study, we investigated the differential expression of 27 m6A RNA methylation regulatory genes, including “writes,” “readers,” and “erasers” (Figure 1A), between the recurrence group and no recurrence group, low-stage group (stage I/II), and high-stage group (stage III/IV) of colorectal cancer tissue by using a dataset from the meta-GEO cohort. We found that some m6A RNA methylation regulators were significantly linked to the recurrence and stage status in patients with CRC (Figures 1B,C). Then, in the TCGA COAD cohort, we performed the copy number variation (CNV) analysis alterations of m6A regulators in CRC patients with recurrence. For CNV events, approximately 59% (16/27) of m6A regulators lost DNA copy number, with YTHDC1 having the highest degree of copy number loss. Eleven m6A regulators gained copy number, among which YTHDF1 had the highest percentage increase (Figure 1D). The m6A regulator CNV alterations and locations on chromosomes are shown in Figure 1E.
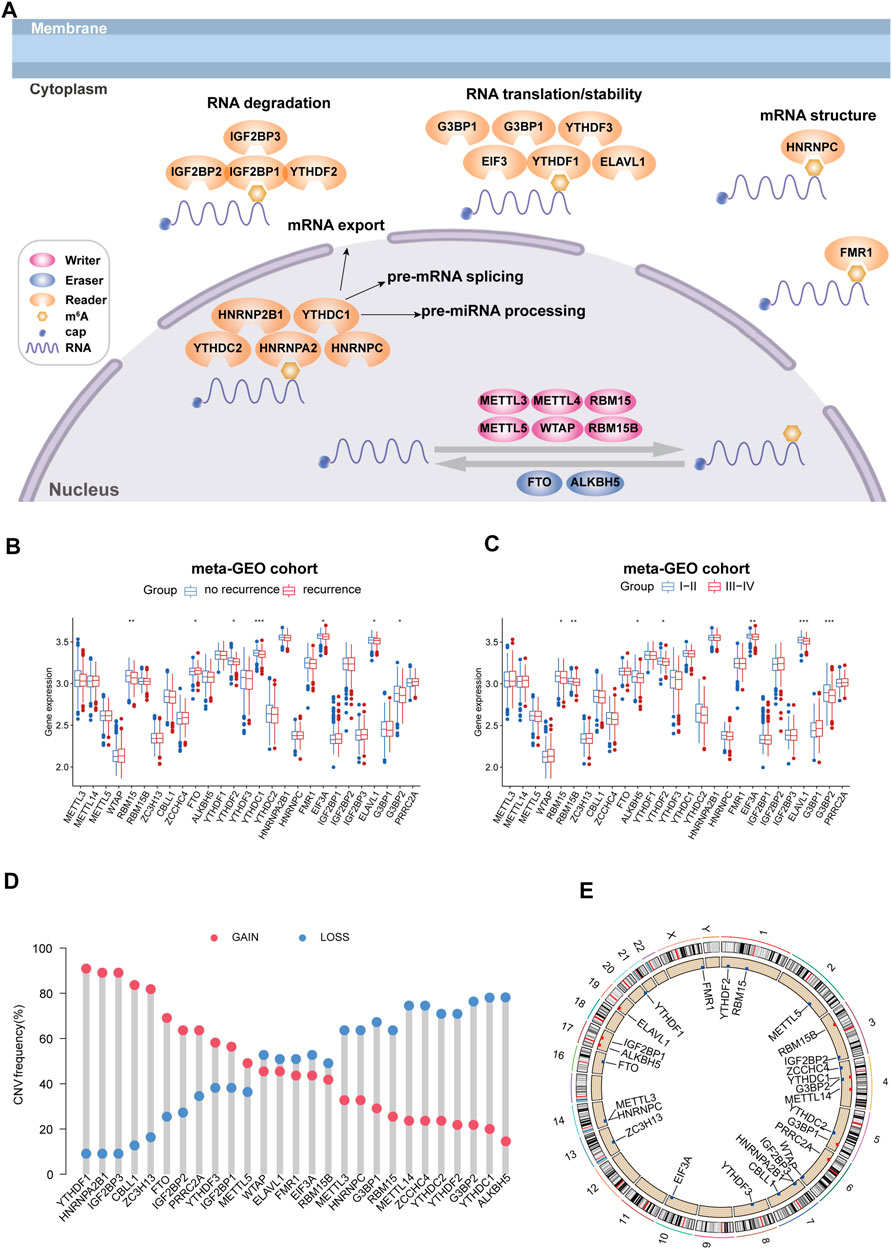
FIGURE 1. Landscape of genetic and expression variation of m6A regulators in colorectal cancer recurrence population. (A) Regulation of m6A regulation and its biological functions in RNA metabolism. (B) Expression change of m6A regulators in colorectal cancer with recurrence compared with no recurrence. (C) Expression changes of m6A regulators in colorectal cancer with high stage compared with low stage. *p < 0.05, **p < 0.01, and ***p < 0.001. (D) CNV variation frequency of m6A regulators in the TCGA cohort. The height of the column represented the alteration frequency. The deletion frequency is represented by a blue dot; The amplification frequency is represented by a red dot. (E) Location of CNV alteration of m6A regulators on 23 chromosomes using the TCGA cohort.
Determining the relationship between m6A-modified patterns and prognosis of CRC patients
Three GEO datasets (GSE39582, GSE72970, and GSE103479) with gene expression and available relapse-free survival time, overall survival time, and clinical traits were enrolled in our meta-GEO cohort. The comprehensive network of interactions of the 27 m6A regulators and the recurrence status of CRC patients is shown in Figure 2A. The results firmly indicate that these regulators played a critical role in the recurrence of CRC. Then, the NMF algorithm was used to divide 804 patients into different m6A clusters, according to the expression of 27 m6A regulators. Then, we adopted three clusters as an acceptable criterion according to the results of the NMF rank survey (Figures 2B,C), and then, the meta-GEO cohort was divided into three distinct clusters according to the expression of 27 m6A regulators by using the NMF algorithm, including 105 cases in “cluster 1,” 313 cases in “cluster 2,” and 386 cases in “cluster 3” (Supplementary Table S2). Importantly, the Kaplan–Meier survival analysis revealed that cluster 1 had better recurrence-free probability (p = 0.019) than cluster 2 and cluster 3 (Figure 2D). However, cluster 1 also showed longer overall survival than the other two clusters, but there was no significant statistical difference (p = 0.081) (Figure 2E).
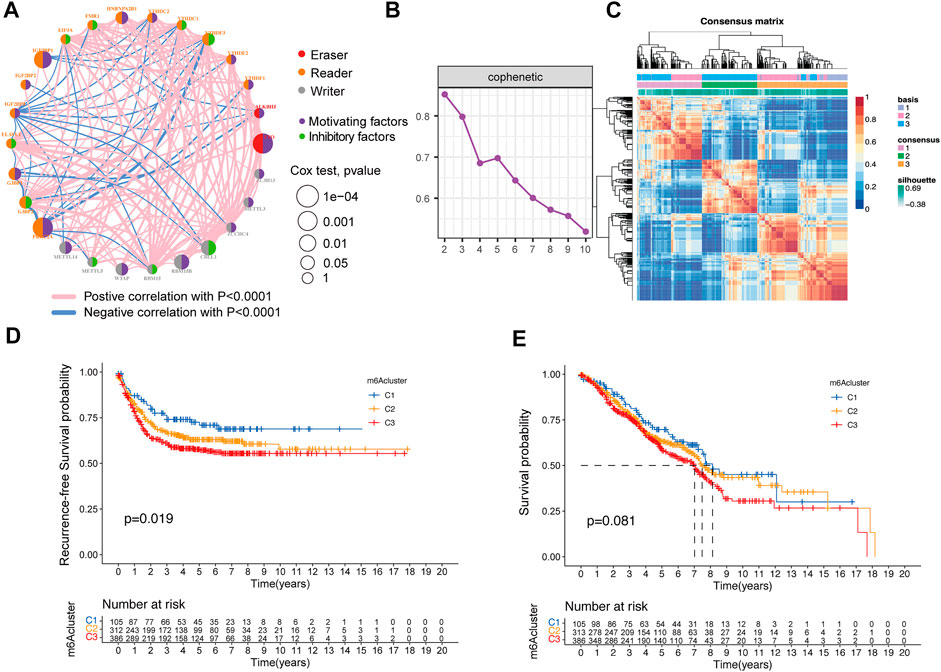
FIGURE 2. Relationship between the m6A methylation modification pattern and prognostic characteristics. (A) Interaction of expression on 27 m6A regulators in colorectal cancer. The m6A regulators in three RNA modification clusters were depicted by circles in different colors. Readers, orange; writers, gray; erasers, red. The lines connecting m6A regulators represented their interaction with each other. The size of each circle represented the recurrence effect of each regulator and was scaled by the p-value. Inhibitory factors for patients’ recurrence were indicated by a green right semicircle and motivating factors indicated by a purple right semicircle. (B) NMF rank survey result. (C) NMF analysis identification of the three m6A modification clusters. (D,E) Kaplan–Meier curves of recurrence-free survival (D) and overall survival (E) for 804 CRC patients in the meta-GEO cohort with different m6A cluster patterns. The numbers of patients in m6A-cluster 1, m6A-cluster 2, and m6A-cluster 3 three phenotypes are 105, 313, and 386, respectively.
Immune profiles among the distinct m6A methylation-modified patterns
The aforementioned findings confirmed that the different clusters were significantly associated with the outcomes of CRC patients. Then, GSVA enrichment analysis was performed to explore the underlying molecular mechanisms behind three different clusters. Intriguingly, we found that cluster 2, compared with cluster 1, was highly enriched in beta-catenin signaling, DNA repair, MYC target, and E2F targets pathway, whereas it was downregulated in androgen response and TGF beta signaling transduction. In addition, cluster 3 was significantly upregulated in beta-catenin signaling and the myogenesis-related pathway. Remarkably, cluster 3 showed that the IL6 JAK STAT3 signaling pathway was activated when compared to cluster 1 and downregulated in interferon-gamma response when compared to cluster 2 (Figures 3A–C). Furthermore, to better-understand the association between immune profiles and m6A modification, we compared and visualized the relative abundances of 23 immune infiltrating cell subpopulations among three m6A modification patterns by using the ssGSEA algorithm. By contrast, cluster 1 was markedly enriched in innate and adaptive immune cell infiltration, including activated CD4 T cell, activated CD8 T cell, activated dendritic cell, CD56 dim natural killer cell, type 17 helper cell, and gamma delta T cell (Figure 3D).
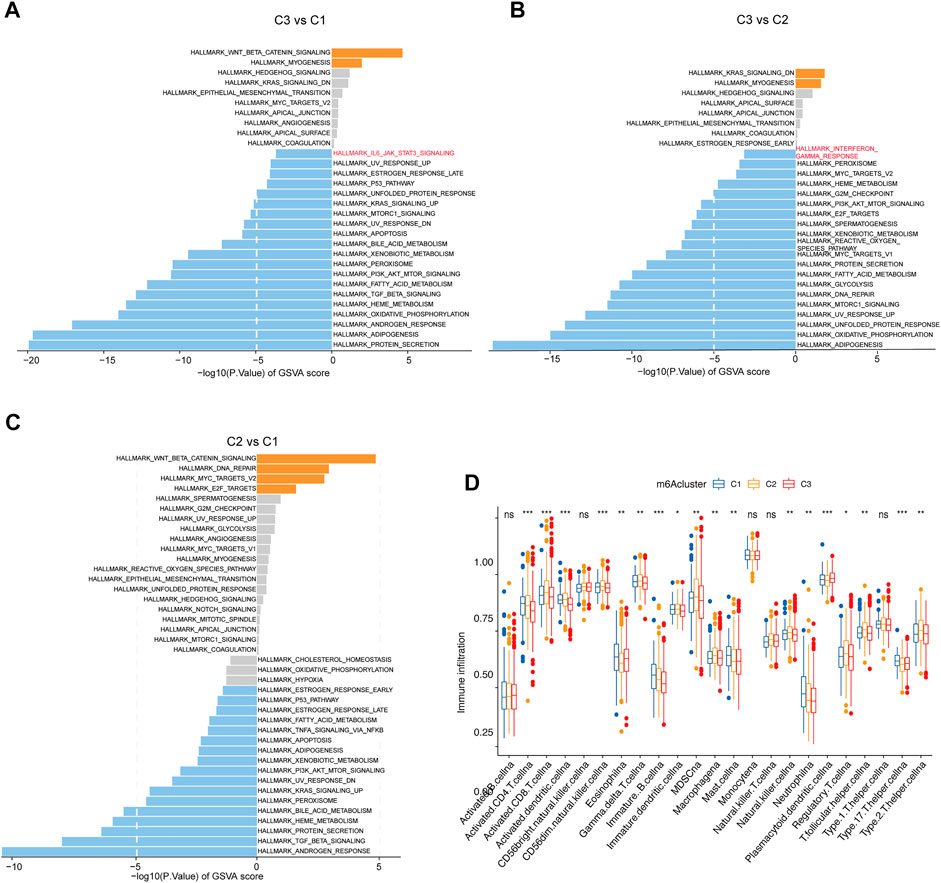
FIGURE 3. Immune profiles among the different m6A methylation modification patterns. (A–C) GSVA enrichment analysis shows the activation states of biological pathways in the three clusters. The biological processes are visualized with the bar plot: orange represents activated pathways; blue represents inhibited pathways. (D) Fraction of tumor-infiltrating lymphocyte cells in three m6A clusters using the ssGSEA algorithm. Within each group, the scattered dots represented TME cell expression values. The thick line represented the median value. The bottom and top of the boxes were the 25th and 75th percentiles, respectively (interquartile range). The statistical difference between the three gene clusters was compared through the Kruskal–Wallis H test. *p < 0.05; **p < 0.01; ***p < 0.001.
Construction of the m6A score and exploration of its clinical relevance
For the assessed m6A score of each CRC patient, a total of 195 differential expression genes were extracted among the distinct m6A clusters, and then univariable Cox regression analysis was performed to screen key genes, which were related to the recurrence-free survival (Supplementary Table S3). Subsequently, the m6A score of each patient was calculated according to the PCA algorithm (Supplementary Table S2). The KM survival plot was performed to evaluate the relationship between the low-/high-m6A score group and prognosis. Importantly, patients in the low-m6A score group exhibited significantly longer recurrence-free time and survival time than those in the high-m6A score group (Figures 4A,B).
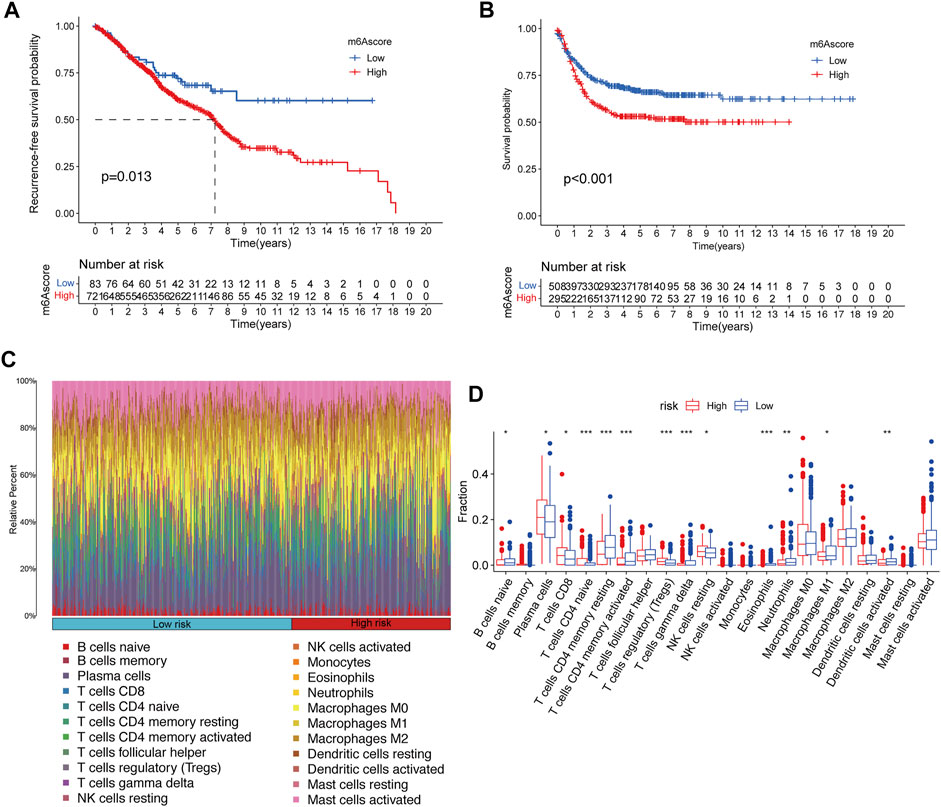
FIGURE 4. Construction of m6A signatures and TME cell infiltration analysis. (A,B) Kaplan–Meier curves of recurrence-free survival (A) and overall survival (B) for 804 CRC patients in the meta-GEO cohort with different m6A scores. (C) Bar plot visualizes the relative percent of 22 immune cells in each sample. (D) Boxplot of all 22 immune cells differentially infiltrated fraction. *p < 0.05; **p < 0.01; ***p < 0.001.
TME immune cell infiltration characteristics in distinct m6A risk groups
Furthermore, to better-understand the underlying immune regulatory mechanisms of the different m6A score groups, hierarchical clustering was performed to explore the distinct patterns of tumor immune cell infiltration based on the immune cell fractions of CRC samples with CIBERSORT p < 0.05 between the high-m6A risk group and the low-m6A score group, and the tumor immune cell proportions by two clusters are shown in Figure 4C. We compared the fractions of 22 immunocytes between the high-m6A score group and low-m6A score group, and 13 immunocytes were altered in the low-m6A score group, including eight increasing immunocyte fractions (B cell naïve, T cells CD4 memory resting, T cells CD4 memory activated, T cells gamma–delta, eosinophils, neutrophils, M1 macrophage, and dendritic cells activated) and five decreasing immunocyte fractions (plasma cell, T cell CD8, T cell CD4 naïve, T cells regulatory, and NK cell resting) (Figure 4D).
Functional enrichment and WGCNA analysis in m6A-related genes
A total of 6,770 m6A modified genes were obtained by Pearson’s correlation analysis (cor > 0.3, p < 0.05) (Supplementary Table S4). Then, these genes were used for weighted gene co-expression network analysis, which is commonly used to analyze the relationship between co-expression modules and external sample traits (Hazra and Gogtay, 2016). In our research, value “8” was selected as a soft thresholding power value because it produced a high similarity with a scale-free network and contributed to gene clustering (Figures 5A,B). Then, a one-step network to estimate the relationship between modules and clinical characteristics (sex, age, RFS events, RFS, status, and OS) was constructed. Here, a clustering dendrogram of m6A-related genes (Figures 5C,D) and 18 modules were obtained. Remarkably, module red, cyan, gray60, dark turquoise, and dark gray were significantly correlated to the recurrence-free survival and positively linked to the recurrence event in colorectal cancer, and these modules were adopted for further regression analysis (Figure 5E). These significant modules were shown upregulated in chromosome segregation, RNA localization, and mRNA 5′-UTR binding by using Gene Ontology analysis (Figure 5F).
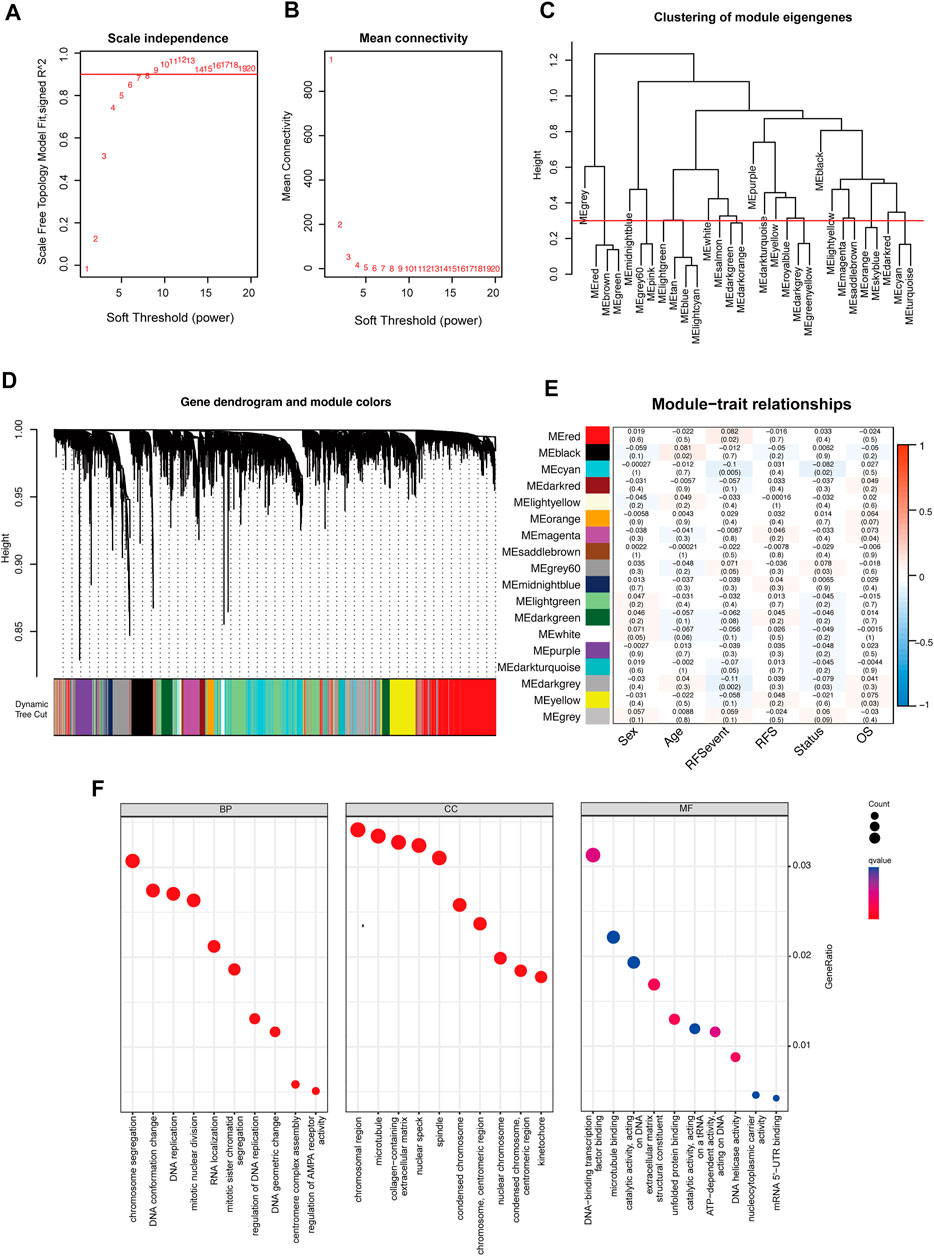
FIGURE 5. Weighted gene correlation network analysis of m6A methylation regulators. (A,B) Analysis of network topology to determine soft-thresholding power. (C) Eigengene dendrogram identified groups of correlated modules. (D) Gene dendrogram was obtained by clustering the dissimilarity based on consensus topological overlap with the corresponding module colors indicated by the color row. Each colored row represents a color-coded module that contains a group of highly connected genes. (E) Heatmap of the correlation between the module eigengenes and clinical traits of colorectal cancer. We selected the module red, cyan, gray60, dark turquoise, and dark gray blocks for subsequent analysis. (F) Gene Ontology analysis of genes in module red, cyan, gray60, dark turquoise, and dark gray.
Identification of m6A- and recurrence-related genes
Initially, m6A-modified and recurrence-related genes were screened by WGCNA, then the univariable Cox analysis was carried out to attain the genes that were significantly correlated to recurrence and overall survival, and we acquired 225 genes (Supplementary Table S4). Subsequently, the multivariable Cox regression was adopted to determine the final prognostic factors by using 37 significant genes from the univariable Cox regression (p < 0.01) (Figure 6A), and six genes were recognized linked to CRC patient prognosis, namely, TOP2A, LRRC58, HAUS6, SMC4, ACVRL1, and KPNB1. We also found that ALVRL1 and HAUS6 were significantly related to the prognosis of CRC patients, including recurrence-free survival and overall survival (Figure 6B). Next, to evaluate the accuracy of the signatures obtained by the multivariable Cox regression analysis from the meta-GEO cohort, we downloaded the overall survival of these genes of CRC patients in the TCGA cohort from the GEPIA database. Importantly, these signatures were also significantly associated with the survival of the CRC patients (p = 0.034) (Figure 6C), and we also found that ALVRL1 and HAUS6 were differentially expressed in COAD and READ patients when compared to those in the normal sample in the TCGA cohort (Figures 6D,E). Finally, to confirm the underlying tumor microenvironment affected by ALVRL1 and HAUS6, the transcriptomes of single cells from CRC samples were downloaded from Single-Cell Expression Altas (https://www.ebi.ac.uk/gxa/sc/experiments/E-MTAB-8410/results/tsne), and the results indicated that ALVRL1 was centrally distributed in endothelial tip cells and stromal cells, whereas HAUS6 did not show central distribution (Figures 6F,G,H).
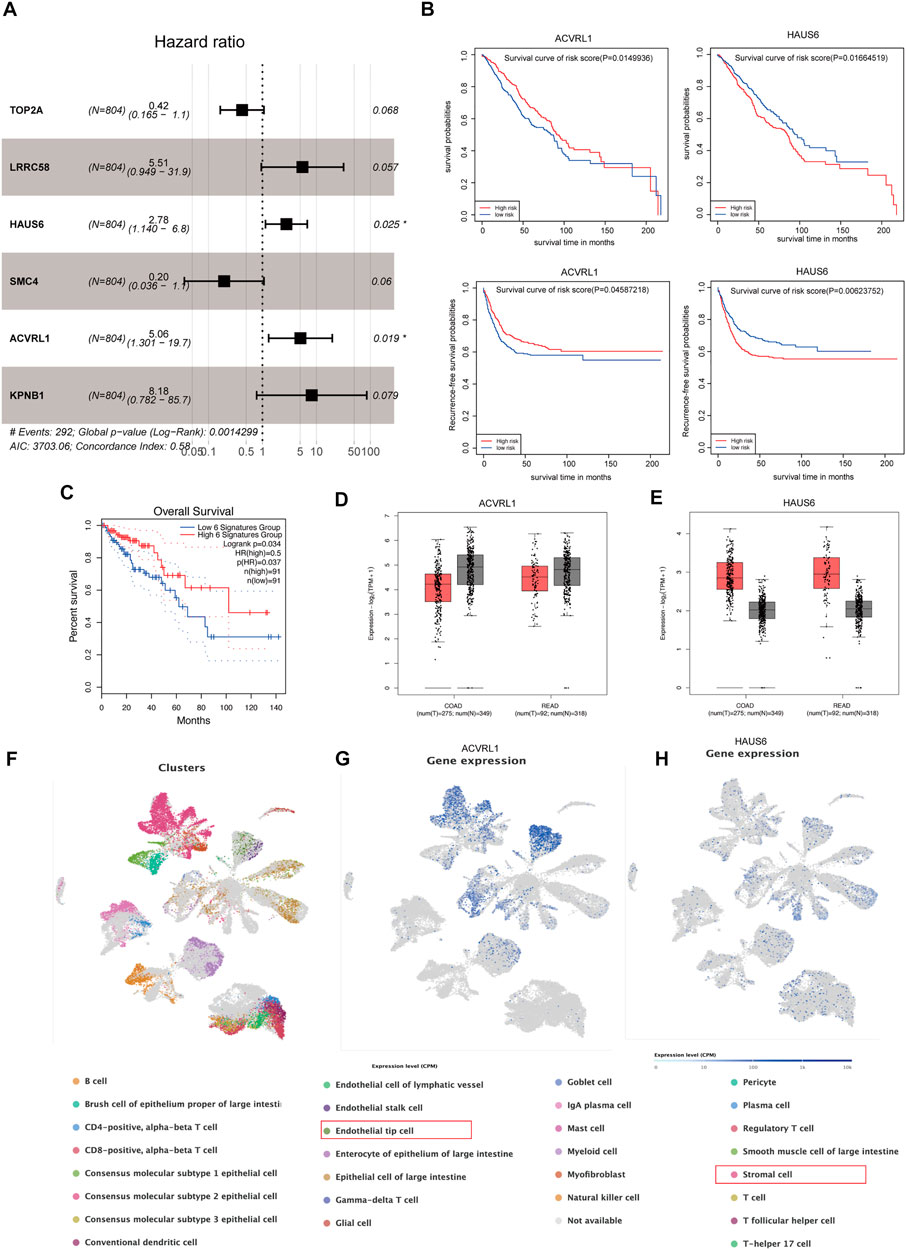
FIGURE 6. Identification of key genes modified by m6A. (A) Multivariable Cox regression analyses in the meta-GEO cohort by using the RFS model. (B) Survival plot of the significant genes obtained by multivariable Cox regression, including ACVRL1 and HAUS6. (C) Overall survival of the signature was obtained by multivariable Cox regression from the meta-GEO cohort in the TCGA cohort. (D,E) Gene expression of ACVRL1 and HAUS6 in the TCGA cohort. (F) Thirty Q21 clusters of the single-cell RNA-seq analysis. (G,H) Distribution of ACVRL1 and HAUS6 in colorectal cancer patients.
Discussion
Based on accumulating evidence, dysregulation of m6A RNA methylation regulators, especially the m6A modification pattern, assumed an indispensable role in the occurrence and recurrence of the tumor. As most studies focus on a single regulator, the integrated patterns to decipher the characteristics of m6A regulators and underlying TME infiltration are not fully recognized in CRC patients with recurrence. Therefore, we explored the relationship between the distinct m6A modification patterns and CRC recurrence and built an m6A-based risk model to predict the prognosis of CRC.
In the current study, we analyzed and compared the expression of 27 key m6A RNA methylation regulators in CRC tissues with recurrence and no recurrence, and we observed differential expression levels of m6A regulators (RBM15, FTO, YTHDF2, YTHDC1, EIF3A, ELAVL1, and G3BP2) both in the recurrence tissues and in the high-stage tissues, indicating their potential functions as tumor motivators in CRC tumorigenesis and recurrence. Next, considering the universality and importance of the m6A modification pattern, we performed consensus clustering of 27 m6A RNA regulators and identified three subgroups: m6A cluster 1, m6A cluster 2, and m6A cluster 3. m6A cluster subgroups were verified to influence recurrence-free survival and overall survival. The immune profile analysis underlying distinct m6A clusters revealed that immune responses, including innate immunity and adaptive immunity, were enhanced in m6A cluster 1 with longer recurrence-free survival, and tumor immune cells were also enriched in m6A cluster 1.
To quantitatively illustrate the m6A signature, we calculated the m6A score of CRC patients individually by PCA based on 32 significantly prognostic m6A phenotype-related DEGs between the three m6A cluster subgroups. Also, the lower m6A score was significantly associated with better prognosis both in RFS and OS. Furthermore, the m6A score was negatively correlated with five of 23 immune-associated cells. CIBERSORT results also showed that B cell naïve, T cells CD4 memory resting, T cells CD4 memory activated, T cells gamma–delta, eosinophils, neutrophils, and dendritic cells activated elevated significantly in the low-risk group (lower m6A score group), which indicates a potential mechanism by which the m6A signature protects against CRC progression is by positively regulating immune cell infiltration.
Subsequently, WGCNA analysis, indeed, verified the connection between CRC recurrence and m6A regulator-related genes. Hence, we identified the prognostic value of m6A-modified gene signatures (TOP2A, LRRC58, HAUS6, SMC4, ACVRL1, and KPNB1) which were selected by the univariable and multivariable Cox regression analyses. Notably, these signatures also showed a significant relation to the survival in the TCGA cohort. In addition, the significant genes (ACVRL1 and HAUS6) obtained from multivariable Cox regression could serve as biomarkers for CRC patient survival. The single-cell expression dataset (Lee et al., 2020) of colorectal cancer samples demonstrated that ALVRL1 was centrally distributed in endothelial tip cells and stromal cells, whereas HAUS6 did not show central distribution, which also indicates that m6A target mRNAs affected the tumor microenvironment, thereby influencing the prognosis of CRC.
Conclusion
We evaluated the m6A modification patterns of 804 primary CRC patients based on 27 m6A regulators and revealed the biological mechanism behind the distinct m6A modification patterns, which will help improve our understanding of the characteristics of TME cell infiltration and predict clinical prognosis. The m6A modification plays an indispensable role in the formation of TME diversity and complexity. Notably, TOP2A, LRRC58, HAUS6, SMC4, ACVRL1, and KPNB1 were identified as m6A-modified genes associated with CRC recurrence, thereby serving as a promising predictive biomarker panel or therapeutic target for patients with CRC recurrence.
Data availability statement
The original contributions presented in the study are included in the article/Supplementary Material; further inquiries can be directed to the corresponding authors.
Ethics statement
The studies involving human participants were reviewed and approved by TCGA and GEO belonging to public databases. The patients involved in the database have obtained ethical approval. Users can download relevant data for free for research and publish relevant articles. Our study is based on open-source data, so there are no ethical issues and other conflicts of interest. The patients/participants provided their written informed consent to participate in this study.
Author contributions
QZ: data collection and analysis, investigation, visualization, and writing—original draft. XH, SY, LS, RZ, HX, ZL, MZ, and YX: review and technical support. XS, TD, JF, and WJ: review, technical support, and funding acquisition. LY, WJ, and QW: conceptualization, writing—review and editing, supervision, and funding acquisition. All authors have read and agreed to the published version of the manuscript.
Funding
This work was supported by grants from the National Natural Science Foundation of China (No. 82104207, to XS), the Science and Technology Development Fund, Macau SAR (Nos. 130/2017/A3, 0099/2018/A3, and 0098/2021/A2, to QW), the Science and Technology Planning Project of Guangdong Province (2020B1212030008, to QW), Zhejiang Provincial Natural Science Foundation of China (Nos. LQ20H160013, to TD; LQ21H160038, to JF; LQ22H280001, to XS), Zhejiang Province Science and Technology Project of TCM (2021ZQ058, to RZ), and Zhejiang Province Medicine and Health Project (2021KY248, to QZ).
Acknowledgments
The authors thank GEO, TCGA, and GEPIA databases for sharing the data about colorectal cancer.
Conflict of interest
The authors declare that the research was conducted in the absence of any commercial or financial relationships that could be construed as a potential conflict of interest.
Publisher’s note
All claims expressed in this article are solely those of the authors and do not necessarily represent those of their affiliated organizations, or those of the publisher, the editors, and the reviewers. Any product that may be evaluated in this article, or claim that may be made by its manufacturer, is not guaranteed or endorsed by the publisher.
Supplementary material
The Supplementary Material for this article can be found online at: https://www.frontiersin.org/articles/10.3389/fgene.2022.1043297/full#supplementary-material
References
Allen, W. L., Dunne, P. D., McDade, S., Scanlon, E., Loughrey, M., Coleman, H., et al. (2018). Transcriptional subtyping and CD8 immunohistochemistry identifies poor prognosis stage II/III colorectal cancer patients who benefit from adjuvant chemotherapy. JCO Precis. Oncol. 17, 00241. doi:10.1200/PO.17.00241
Arguello, A. E., DeLiberto, A. N., and Kleiner, R. E. (2017). RNA chemical proteomics reveals the N(6)-methyladenosine (m6A)-Regulated protein-RNA interactome. J. Am. Chem. Soc. 139 (48), 17249–17252. doi:10.1021/jacs.7b09213
Cai, Z., Zhang, J., Liu, Z., Su, J., Xu, J., Li, Z., et al. (2021). Identification of an N6-methyladenosine (m6A)-related signature associated with clinical prognosis, immune response, and chemotherapy in primary glioblastomas. Ann. Transl. Med. 9 (15), 1241. doi:10.21037/atm-21-3139
Charoentong, P., Finotello, F., Angelova, M., Mayer, C., Efremova, M., Rieder, D., et al. (2017). Pan-cancer immunogenomic analyses reveal genotype-immunophenotype relationships and predictors of response to checkpoint blockade. Cell Rep. 18 (1), 248–262. doi:10.1016/j.celrep.2016.12.019
Chong, W., Shang, L., Liu, J., Fang, Z., Du, F., Wu, H., et al. (2021). m6A regulator-based methylation modification patterns characterized by distinct tumor microenvironment immune profiles in colon cancer. Theranostics 11 (5), 2201–2217. doi:10.7150/thno.52717
Dai, W., Li, Y., Mo, S., Feng, Y., Zhang, L., Xu, Y., et al. (2018). A robust gene signature for the prediction of early relapse in stage I-III colon cancer. Mol. Oncol. 12 (4), 463–475. doi:10.1002/1878-0261.12175
Del Rio, M., Mollevi, C., Bibeau, F., Vie, N., Selves, J., Emile, J. F., et al. (2017). Molecular subtypes of metastatic colorectal cancer are associated with patient response to irinotecan-based therapies. Eur. J. Cancer 76, 68–75. doi:10.1016/j.ejca.2017.02.003
Dominissini, D., Moshitch-Moshkovitz, S., Schwartz, S., Salmon-Divon, M., Ungar, L., Osenberg, S., et al. (2012). Topology of the human and mouse m6A RNA methylomes revealed by m6A-seq. Nature 485 (7397), 201–206. doi:10.1038/nature11112
Dong, L., Chen, C., Zhang, Y., Guo, P., Wang, Z., Li, J., et al. (2021). The loss of RNA N6-adenosine methyltransferase Mettl14 in tumor-associated macrophages promotes CD8+ T cell dysfunction and tumor growth. Cancer Cell 39 (7), 945–957. doi:10.1016/j.ccell.2021.04.016
Gu, J., Zhan, Y., Zhuo, L., Zhang, Q., Li, G., Li, Q., et al. (2021). Biological functions of m(6)A methyltransferases. Cell Biosci. 11 (1), 15. doi:10.1186/s13578-020-00513-0
Han, D., Liu, J., Chen, C., Dong, L., Liu, Y., Chang, R., et al. (2019). Anti-tumour immunity controlled through mRNA m(6)A methylation and YTHDF1 in dendritic cells. Nature 566 (7743), 270–274. doi:10.1038/s41586-019-0916-x
Hänzelmann, S., Castelo, R., and Guinney, J. (2013). Gsva: Gene set variation analysis for microarray and RNA-seq data. BMC Bioinforma. 14, 7. doi:10.1186/1471-2105-14-7
Hashiguchi, Y., Muro, K., Saito, Y., Ito, Y., Ajioka, Y., and Hamaguchi, T. (2020). Japanese Society for Cancer of the Colon and Rectum (JSCCR) guidelines 2019 for the treatment of colorectal cancer. Int. J. Clin. Oncol. 25 (1), 1–42. doi:10.1007/s10147-019-01485-z
Haussmann, I. U., Bodi, Z., Sanchez-Moran, E., Mongan, N. P., Archer, N., Fray, R. G., et al. (2016). m6A potentiates Sxl alternative pre-mRNA splicing for robust Drosophila sex determination. Nature 540 (7632), 301–304. doi:10.1038/nature20577
Hazra, A., and Gogtay, N. (2016). Biostatistics series module 3: Comparing groups: Numerical variables. Indian J. Dermatol 61 (3), 251–260. doi:10.4103/0019-5154.182416
Huang, H., Li, H., Pan, R., Wang, S., Khan, A. A., Zhao, Y., et al. (2022). Ribosome 18S m6A methyltransferase METTL5 promotes pancreatic cancer progression by modulating c-Myc translation. Int. J. Oncol. 60(1), 9. doi:10.3892/ijo.2021.5299
Huang, H., Weng, H., Sun, W., Qin, X., Shi, H., Wu, H., et al. (2018). Recognition of RNA N(6)-methyladenosine by IGF2BP proteins enhances mRNA stability and translation. Nat. Cell Biol. 20 (3), 285–295. doi:10.1038/s41556-018-0045-z
Huang, X. T., Li, J. H., Zhu, X. X., Huang, C. S., Gao, Z. X., Xu, Q. C., et al. (2021). HNRNPC impedes m6A-dependent anti-metastatic alternative splicing events in pancreatic ductal adenocarcinoma. Cancer Lett. 518, 196–206. doi:10.1016/j.canlet.2021.07.016
Jia, G., Fu, Y., Zhao, X., Dai, Q., Zheng, G., Yang, Y., et al. (2011). N6-methyladenosine in nuclear RNA is a major substrate of the obesity-associated FTO. Nat. Chem. Biol. 7 (12), 885–887. doi:10.1038/nchembio.687
Jia, Q., Wu, W., Wang, Y., Alexander, P. B., Sun, C., Gong, Z., et al. (2018). Local mutational diversity drives intratumoral immune heterogeneity in non-small cell lung cancer. Nat. Commun. 9 (1), 5361. doi:10.1038/s41467-018-07767-w
Jiang, F., Tang, X., Tang, C., Hua, Z., Ke, M., Wang, C., et al. (2021). HNRNPA2B1 promotes multiple myeloma progression by increasing AKT3 expression via m6A-dependent stabilization of ILF3 mRNA. J. Hematol. Oncol. 14 (1), 54. doi:10.1186/s13045-021-01066-6
Jin, D., Guo, J., Wu, Y., Du, J., Yang, L., Wang, X., et al. (2019). m6A mRNA methylation initiated by METTL3 directly promotes YAP translation and increases YAP activity by regulating the MALAT1-miR-1914-3p-YAP axis to induce NSCLC drug resistance and metastasis. J. Hematol. Oncol. 12 (1), 135. doi:10.1186/s13045-019-0830-6
Ke, S., Alemu, E. A., Mertens, C., Gantman, E. C., Fak, J. J., Mele, A., et al. (2015). A majority of m6A residues are in the last exons, allowing the potential for 3′ UTR regulation. Genes Dev. 29 (19), 2037–2053. doi:10.1101/gad.269415.115
Langfelder, P., and Horvath, S. (2008). Wgcna: an R package for weighted correlation network analysis. BMC Bioinforma. 9 (1), 559. doi:10.1186/1471-2105-9-559
Lao, V. V., and Grady, W. M. (2011). Epigenetics and colorectal cancer. Nat. Rev. Gastroenterol. Hepatol. 8 (12), 686–700. doi:10.1038/nrgastro.2011.173
Lee, H. O., Hong, Y., Etlioglu, H. E., Cho, Y. B., and Pomella, V. (2020). Lineage-dependent gene expression programs influence the immune landscape of colorectal cancer. Nat. Genet. 52 (6), 594–603. doi:10.1038/s41588-020-0636-z
Li, M., Zha, X., and Wang, S. (2021). The role of N6-methyladenosine mRNA in the tumor microenvironment. Biochim. Biophys. Acta Rev. Cancer 1875 (2), 188522. doi:10.1016/j.bbcan.2021.188522
Liang, Z., Kidwell, R. L., Deng, H., and Xie, Q. (2020). Epigenetic N6-methyladenosine modification of RNA and DNA regulates cancer. Cancer Biol. Med. 17 (1), 9–19. doi:10.20892/j.issn.2095-3941.2019.0347
Liu, J., Yue, Y., Han, D., Wang, X., Fu, Y., Zhang, L., et al. (2014). A METTL3-METTL14 complex mediates mammalian nuclear RNA N6-adenosine methylation. Nat. Chem. Biol. 10 (2), 93–95. doi:10.1038/nchembio.1432
Marisa, L., de Reyniès, A., Duval, A., Selves, J., Gaub, M. P., Vescovo, L., et al. (2013). Gene expression classification of colon cancer into molecular subtypes: Characterization, validation, and prognostic value. PLoS Med. 10 (5), e1001453. doi:10.1371/journal.pmed.1001453
Meyer, K. D., and Jaffrey, S. R. (2017). Rethinking m6A readers, writers, and erasers. Annu. Rev. Cell Dev. Biol. 33, 319–342. doi:10.1146/annurev-cellbio-100616-060758
Meyer, K. D., Patil, D. P., Zhou, J., Zinoviev, A., Skabkin, M. A., Elemento, O., et al. (2015). 5′ UTR m6A promotes cap-independent translation. Cell 163 (4), 999–1010. doi:10.1016/j.cell.2015.10.012
Meyer, K. D., Saletore, Y., Zumbo, P., Elemento, O., Mason, C. E., and Jaffrey, S. R. (2012). Comprehensive analysis of mRNA methylation reveals enrichment in 3′ UTRs and near stop codons. Cell 149 (7), 1635–1646. doi:10.1016/j.cell.2012.05.003
Newman, A. M., Liu, C. L., Green, M. R., Gentles, A. J., Feng, W., Xu, Y., et al. (2015). Robust enumeration of cell subsets from tissue expression profiles. Nat. Methods 12 (5), 453–457. doi:10.1038/nmeth.3337
Pan, Y., Xiao, K., Li, Y., Li, Y., and Liu, Q. (2021). RNA N6-methyladenosine regulator-mediated methylation modifications pattern and immune infiltration features in glioblastoma. Front. Oncol. 11, 632934. doi:10.3389/fonc.2021.632934
Ping, X. L., Sun, B. F., Wang, L., Xiao, W., Yang, X., Wang, W. J., et al. (2014). Mammalian WTAP is a regulatory subunit of the RNA N6-methyladenosine methyltransferase. Cell Res. 24 (2), 177–189. doi:10.1038/cr.2014.3
Sanoff, H. K., Sargent, D. J., Campbell, M. E., Morton, R. F., Fuchs, C. S., Ramanathan, R. K., et al. (2008). Five-year data and prognostic factor analysis of oxaliplatin and irinotecan combinations for advanced colorectal cancer: N9741. J. Clin. Oncol. 26 (35), 5721–5727. doi:10.1200/JCO.2008.17.7147
Schumann, U., Shafik, A., and Preiss, T. (2016). METTL3 gains R/W access to the epitranscriptome. Mol. Cell 62 (3), 323–324. doi:10.1016/j.molcel.2016.04.024
Siegel, R. L., Miller, K. D., Fuchs, H. E., and Jemal, A. (2021). Cancer statistics, 2021. CA A Cancer J. Clin. 71 (4), 7–33. doi:10.3322/caac.21654
Subramanian, A., Tamayo, P., Mootha, V. K., Mukherjee, S., Ebert, B. L., Gillette, M. A., et al. (2005). Gene set enrichment analysis: A knowledge-based approach for interpreting genome-wide expression profiles. Proc. Natl. Acad. Sci. U. S. A. 102 (43), 15545–15550. doi:10.1073/pnas.0506580102
Taketo, K., Konno, M., Asai, A., Koseki, J., Toratani, M., Satoh, T., et al. (2018). The epitranscriptome m6A writer METTL3 promotes chemo- and radioresistance in pancreatic cancer cells. Int. J. Oncol. 52 (2), 621–629. doi:10.3892/ijo.2017.4219
Worpenberg, L., Paolantoni, C., Longhi, S., Mulorz, M. M., Lence, T., Wessels, H. H., et al. (2021). Ythdf is a N6-methyladenosine reader that modulates Fmr1 target mRNA selection and restricts axonal growth in Drosophila. Embo J. 40 (4), e104975. doi:10.15252/embj.2020104975
Wu, R., Li, A., Sun, B., Sun, J. G., Zhang, J., Zhang, T., et al. (2019). A novel m6A reader Prrc2a controls oligodendroglial specification and myelination. Cell Res. 29 (1), 23–41. doi:10.1038/s41422-018-0113-8
Keywords: colorectal cancer, m6A methylation modification, tumor immune microenvironment, recurrence, overall survival
Citation: Zhu Q, Huang X, Yu S, Shou L, Zhang R, Xie H, Liang Z, Sun X, Feng J, Duan T, Zhang M, Xiang Y, Sui X, Jin W, Yu L and Wu Q (2022) Identification of genes modified by N6-methyladenosine in patients with colorectal cancer recurrence. Front. Genet. 13:1043297. doi: 10.3389/fgene.2022.1043297
Received: 13 September 2022; Accepted: 29 September 2022;
Published: 17 October 2022.
Edited by:
Elaine Leung, University of Macau, ChinaReviewed by:
Jinming Zhang, Chengdu University of Traditional Chinese Medicine, ChinaYu Cai, Jinan University, China
Copyright © 2022 Zhu, Huang, Yu, Shou, Zhang, Xie, Liang, Sun, Feng, Duan, Zhang, Xiang, Sui, Jin, Yu and Wu. This is an open-access article distributed under the terms of the Creative Commons Attribution License (CC BY). The use, distribution or reproduction in other forums is permitted, provided the original author(s) and the copyright owner(s) are credited and that the original publication in this journal is cited, in accordance with accepted academic practice. No use, distribution or reproduction is permitted which does not comply with these terms.
*Correspondence: Weiwei Jin, amlud3dAemp1LmVkdS5jbg==; Lili Yu, bGx5dUBtdXN0LmVkdS5tbw==; Qibiao Wu, cWJ3dUBtdXN0LmVkdS5tbw==