- 1Department of Periodontology, Nanjing Stomatological Hospital, Medical School of Nanjing University, Nanjing, China
- 2Central Laboratory of Stomatology, Nanjing Stomatological Hospital, Medical School of Nanjing University, Nanjing, China
- 3Department of Stomatology, Nanjing Hospital of Chinese Medicine, Nanjing University of Traditional Chinese Medicine, Nanjing, China
Periodontal Ehlers–Danlos syndrome (pEDS) is a rare hereditary disorder characterized by severe early-onset periodontitis with premature tooth loss, pretibial hyperpigmentation, and skin fragility. It is caused by mutant variants in the C1R and C1S genes that result in C4 cleavage and local complement cascade activation, as well as other possible consequences. However, the exact functional consequences of this activation remain unclear. To shed light on molecular mechanisms underlying pEDS and to identify novel molecular targets that may expand treatment strategies, we performed transcriptome profiling by RNA sequencing of monocytes and gingival fibroblasts from two patients with pEDS. Compared to normal controls, differential expression of genes was found only in monocytes but not gingival fibroblasts. Most of the significant genes were enriched in biological processes such as neutrophil-mediated immunity, response to bacterium, TNF-α and IL-17 pathway which are related to inflammation response and immune response. In disease ontology enrichment analysis, genes related to periodontal host defense, inflammatory response, skin disease, and vascular development, including MMP9, VEGFA, IL10, IL1A, IL1B, IL2RA, and IL6, were significantly enriched and also validated by qPCR and ELISA. Overall, the present study provides the transcriptomic data of pEDS for the first time and the distinct molecular features in monocytes of pEDS might serve as a tool to better understand the disease.
Introduction
The Ehlers–Danlos syndromes (EDS) are a clinically and genetically heterogeneous group of heritable connective tissue disorders characterized by joint hypermobility, skin hyperextensibility, and tissue fragility (Malfait et al., 2017). In 2017, The International EDS Consortium proposed a revised EDS classification (Malfait et al., 2017), and currently, 14 subtypes of EDS are recognized (Blackburn et al., 2018). Among all subtypes of EDS, periodontal Ehlers–Danlos syndrome (pEDS) (also known as EDS type VIII, OMIM#130080) is a specific EDS subtype caused by autosomal dominant pathogenic variants in complement 1 subunit genes C1R and C1S, with early severe periodontitis as the predominant clinical feature (Kapferer-Seebacher et al., 2016). The other clinical manifestations of pEDS include lack of attached gingiva, pretibial hyperpigmentation, skin fragility with abnormal scars, and easy bruising (Malfait et al., 2017). Treatment of pEDS remains a huge challenge. Most of the current studies published have focused on describing the clinical features of the pEDS and/or identifying genetic variants (Wu et al., 2018; Kapferer-Seebacher et al., 2021). The pathogenesis of pEDS is only partly understood. From previous research (Kapferer-Seebacher et al., 2016), it is confirmed that pEDS is caused by pathogenic variants C1R (type 1, MIM 613785) and C1S (type 2, MIM 120580) genes, which encode the C1r and C1s subunits of the first step of the classical complement cascade, a major antimicrobial pathway of the innate immune system (G’al et al., 2009). Experimental evidence suggests that the C1R and/or C1S variants may cause extracellular presence of activated C1s without microbial triggers (Bally et al., 2019; Gröbner et al., 2019), which would lead to gingival hyperinflammation in response to mild biofilm accumulation, and subsequently rapidly progressing periodontal destruction. However, there are other pEDS clinical features unrelated to biofilm pathogens and apparently could not be explained by the above hypothesis and the detailed mechanisms remain largely unknown. To gain insights into altered gene expression patterns and dysregulated biological processes underlying molecular pathology of pEDS, we carried out transcriptome profiling by RNA sequencing of monocytes and gingival fibroblasts from two patients with pEDS compared with normal controls.
Methods
Study Approval
This study was conducted according to the Declaration of Helsinki for Human Rights and all procedures were reviewed and approved by the Ethics Committee of Nanjing Stomatological Hospital, Medical School of Nanjing University (2018NL-037). All participants provided written informed consent before their enrollment in the present study. Samples were de-identified before analysis.
Participant Recruitment
Monocytes were obtained from two patients with pEDS from our previous study (Wu et al., 2018). Patients’ information was as follows: the proband IV-1 (male, 25 years old, referred as pEDS1 in this study) was found with both a missense mutation in C1R (c.265T > C) and a frameshift mutation in COL3A1 (c.1322delG); the proband’s mother III-2 (female, 48 years old), referred as pEDS2 in the present study, only had the same mutation in C1R. Normal controls were periodontal healthy adults who showed no BOP, PD ≤ 3 mm, and no CAL, who underwent crown lengthening surgery for the restorative purpose in the Department of Periodontology, Nanjing Stomatological Hospital, Medical School of Nanjing University and exclusions included acute illness, pregnancy, and other systemic diseases.
Gingival tissues were obtained from two pEDS patients during their tooth extraction surgery, while control gingival tissues were obtained from three periodontal healthy adults during the crown lengthening surgery. All participants in the present study underwent clinical examination, and the detailed clinical data of all individuals involved in this study were recorded.
Isolation and Culture of Monocytes Extracted from Human Blood Samples
Blood samples collected from two pEDS patients and three normal controls were used to extract peripheral blood mononuclear cells (PBMCs) using density gradient centrifugation. PBMCs of each group were added with RPMI1640 medium (Gibco) containing 2%FBS (Gibco) and incubated at 37°C with 5% CO2 for 2 h. Then the supernatant was harvested and stored for the later experiment of enzyme-linked immunosorbent assay (ELISA). The remained cells were isolated monocytes and washed twice by sterile PBS (Servicebio) gently. RPMI1640 medium supplemented with 10% FBS, 100 U/ml penicillin, and 100 μg/ml streptomycin (HyClone) for further culture for 12 h.
Isolation and Culture of Human Gingival Fibroblasts
Gingival connective tissues were obtained from 2 pEDS patients during tooth extraction surgery and three normal controls during crown lengthening surgery. The collected gingival tissue was immersed in DMEM (Gibco) supplemented with 100 U/ml penicillin, and 100 μg/ml streptomycin (HyClone); and the tissue was cut into pieces, approximately 1 × 1 mm in size and placed in DMEM supplemented with 10% FBS, 100 U/ml penicillin, and 100 μg/ml streptomycin; tissue was then incubated at 37°C with 5% CO2, HGF at the
Gene Expression Profiling
Total RNA was isolated from monocytes and gingival fibroblasts by TRIzol reagent (Invitrogen) according to the manufacturer’s instructions. The RNA samples were quality assessed and the mRNA is enriched using magnetic beads with Oligo (dT). The mRNA was then broken into short fragments by adding fragmentation buffer, and one-stranded cDNA was synthesized using random hexamers as templates, followed by the addition of buffer, dNTPs, and DNA polymerase I and RNase H. The double-stranded cDNA was purified with AMPure XP beads and was end-repaired, added with polyA tails and adapters sequences. After size selection, PCR amplification, and purification, the library was finally obtained. After the quality assessment of the library, RNA sequencing was performed on an Illumina HiSeq X Ten instrument. The raw reads were cleaned by removing adapter sequences, trimming low-quality ends, and filtering low-quality reads (Phred quality <20) using TrimGalore (version 0.6.5). Transcriptome quantification of transcript expression was carried out by using the mapping-based mode of Salmon (version 1.5.2) with the pre-built version of the full-decoy salmon index provided by Salmon.
RNA-Seq Data Analysis
Normalisation and differential expression between patients and normal controls were evaluated using DESeq2 (version 1.32.0) (Love et al., 2014), implemented in R (version 4.1.2). DESeq2 uses a count-based negative binomial model to detect differentially expressed genes (DEGs). DEGs were defined as genes with the adjusted p.value < 0.05 and the absolute value of fold change >1.5
Principal component analysis (PCA), dendrogram and hierarchical clustering heatmap were performed with the variance stabilization transformation values obtained by DESeq2 R package from the gene expression values: PCA was calculated for the whole dataset by using princomp function of R (Anders and Huber, 2010); The dendrogram was created by hclust function and ggplot2 package (version 3.3.5) (Wickham, 2016, p. 2); The hierarchical clustering heatmap was generated by ComplexHeatmap (version 2.10.0) package (Gu et al., 2016) using the top 100 DEGs.
Since there are only two pEDS samples and one of them was a rare case with both pEDS and vEDS, three-dimensional volcano plots were created with scatterplot3d (version 0.3–41) (Ligges & Mächler, 2003): the logarithm of fold change between pEDS1 and normal controls (
For Over-Representation Analysis (ORA) and Gene Set Enrichment Analysis (GSEA), GO and KEGG enrichment analysis using detected DEGs and gene set enrichment analysis (GSEA) using ranked gene lists were performed with the software package clusterProfiler (Yu et al., 2012; Wu et al., 2021) (R-version 4.1.2, clusterProfiler_4.0.5) to identify enriched biological processes and molecular functions; The filtering standard for ORA and GSEA geneList is |
All the R packages we used in the analysis are listed in Supplementary Table S1.
STRING Network Analysis
STRING networks can provide information on the molecular mechanism underlying clinical features. A STRING network of DEGs involved in periodontitis and genes involved in the classical complement activation pathway was constructed using the STRING protein query (Szklarczyk et al., 2021) (STRING Version 11.5) and Cytoscape software (Shannon et al., 2003; Doncheva et al., 2019) (Cytoscape Version 3.8.2). The lines represent interaction associations between nodes and line thickness indicates the strength of data support. Selected DEGs were mapped to STRING to identify the interactive relationships among those genes. A confidence score of 0.4 was set as the cut-off criterion, and the node size in periodontitis-related genes is mapped to the logFC values of gene expression value in monocytes. Additionally, an extended STRING network showing the interaction between classical complement pathway and all DEGs in this study was also created, which could be found in Supplementary Figure S2A. We also identified the top 10 hub genes and sub-networks by using a Cytoscape plugin cytoHubba (Supplementary Figure S2B) (Chin et al., 2014).
Quantitative Reverse Transcription PCR for Validation
Relative expression levels of a series of selected DEGs (MMP9, VEGFA, IL10, IL1A, IL1B, IL2RA, and IL6) identified by RNA sequencing were confirmed by RT-qPCR using different RNA extractions obtained from monocytes cultures of corresponding pEDS and normal controls. 3 µg of total RNA were reverse-transcribed with random primers by standard procedure. RT-qPCR was performed with SYBR Green qPCR Master Mix (Life Technologies), 10 ng of Cdna, and with 10
Enzyme-Linked Immunosorbent Assay
Enzyme-linked Immunosorbent Assay (ELISA) based quantification of IL-1β (Neobioscience Technology) concentration was measured using concentrated supernatants that we collected in monocytes isolation steps, following the supplier’s instructions.
Statistics
For RT-qPCR, relative mRNA expression levels were normalized to the geometric mean of these reference genes and analyzed using the
Results
Participant Enrollment
Two pEDS patients and three periodontal healthy adults (two males at 25 and 28 years old as normal1 and normal2 respectively; one female at 25 years old as normal3) were recruited in this study. The detailed clinical data of all individuals involved in this study were summarized in Table 1, oral photographs of all individuals were available in Supplementary Figure S1. As described in our previous work (Wu et al., 2018), it is worth noting that pEDS1 also carried a frameshift variant in COL3A1 and thus might be affected by vascular EDS (vEDS).
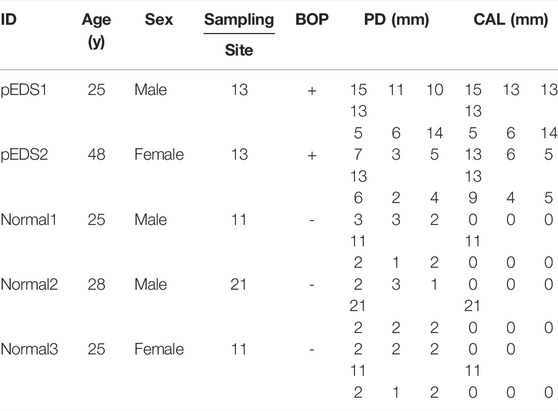
TABLE 1. The clinical findings of all individuals including age, gender, gingiva specimen sampling position, and periodontal examination results.
Quality Assessment of Transcriptome Profiling
The correlation between pEDS and control group was evaluated by quality assessment methods including principal component analysis (PCA) and dendrogram. For the monocytes, PCA shows two principal components that could account for 73.8% of the variability between the samples (Figure 1A). The dendrogram of hierarchical clustering (Figure 1B) show a clear clustering of the controls and the pEDS patients based on gene expression values. However, for the HGF, no significant pattern can be found in the clustering of gene expression values between different samples (Figures 1C,D). The normalized count matrix files of monocyte and fibroblast can be found in Supplementary Tables S3, S4.
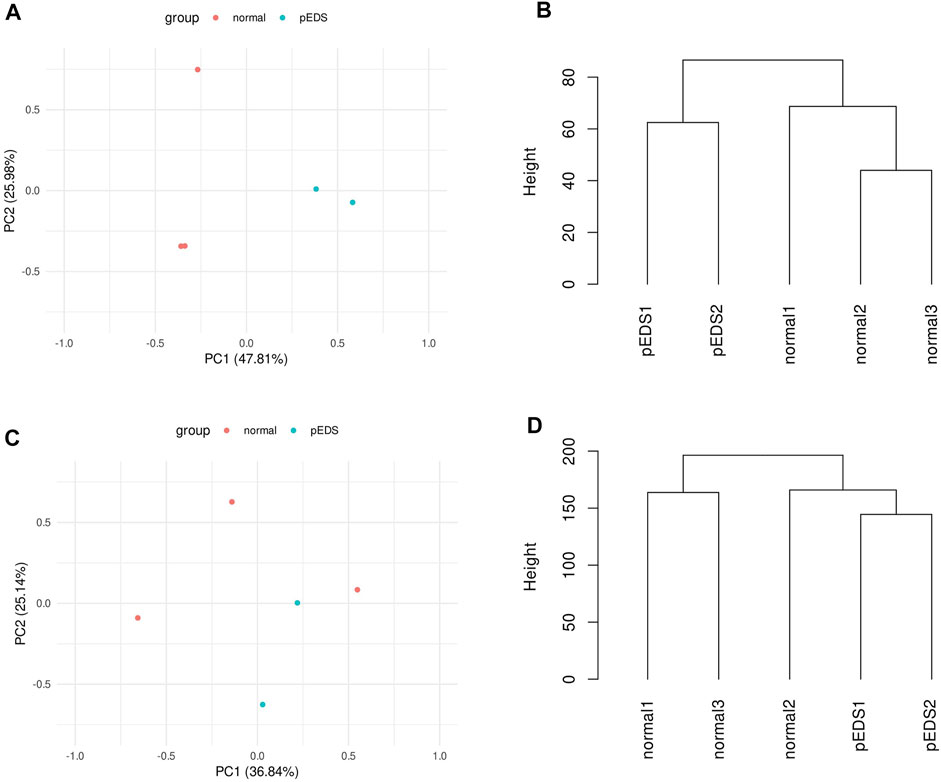
FIGURE 1. The correlation between pEDS and control group was evaluated by quality assessment methods including principal component analysis (PCA) and dendrogram. (A) PCA shows 73.8% of the variability within the monocyte samples is accounted by two principal components. Note the clustering of pEDS (green) vs. normal controls (red); (B) For monocytes, dendrogram shows a clear clustering of the controls and the pEDS subjects based on gene expression values; (C,D) As for HGF samples, the first two principal components can not separate pEDS (green) from normal controls (red); and samples from same group do not cluster. Dendrogram, consistent with PCA result, does not show a significant clustering pattern.
Differential Expression Analysis
Differentially expressed genes (DEGs) were identified by DESeq2 R package according to the filtering criteria. Approximately 3% of the detected transcriptome showed differential expression in monocytes and there were 338 DEGs in pEDS patient-derived monocytes, of which 246 genes were up-regulated and 92 genes were down-regulated. The complete list of DEGs could be found in Supplementary Table S5. Hierarchical heatmaps of the top 100 differential expressed genes within the monocyte and gingival fibroblast samples were shown in Figures 2A,B, which implied that a significant pattern can only be observed in monocytes but not in gingival fibroblasts. In addition, it should be noted that C1R and C1S were not differentially expressed in both monocytes and gingival fibroblasts from pEDS group.
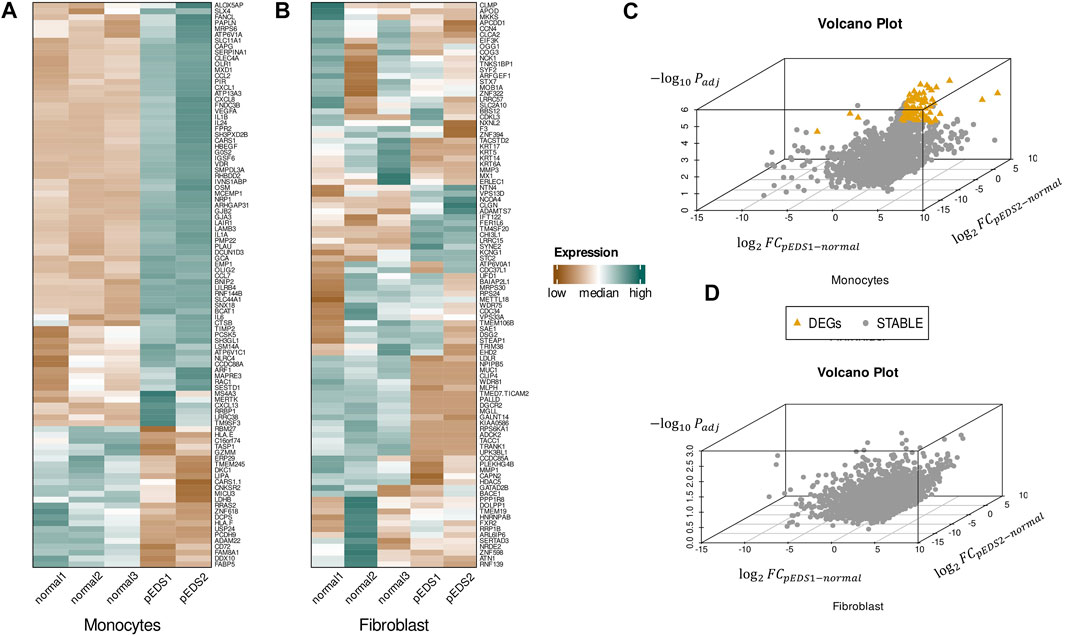
FIGURE 2. Three dimensional volcano plot and hierarchical heatmap of the DEGs found within the monocyte and gingival fibroblast samples. (A,B) Hierarchical heatmap of the top 100 differential expressed genes within the monocyte and gingival fibroblast samples. Red denotes increased expression, and green denotes decreased expression. A significant pattern can only be observed in monocytes but not in gingival fibroblasts. (C,D) Three-dimensional volcano plots for monocytes and fibroblasts:
Since one of two pEDS patients was a rare case with both pEDS and vEDS, three-dimensional volcano plots were created to present the DEGs (Figures 2C,D): A total of 92 DEGs were identified in monocytes but no DEGs could be found in HGF. It should also be noted that 45 DEGs could be identified between sample pEDS1 and pEDS2 but no significant result was identified in the subsequent ORA and GSEA analysis (data not shown).
Over-Representation Analysis and Gene Set Enrichment Analysis of Differentially Expressed Genes in Monocytes.
With the identified 338 DEGs, GO and KEGG enrichment analysis, as well as GSEA, were performed using the software package clusterProfiler (Version 4.0.5) (Yu et al., 2012; Wu et al., 2021). Most of the significant results are enriched in up-regulated genes (Figure 3B), especially in the pathway of biological processes, which are mainly related to inflammation response and immune response (Figure 3A). This finding is consistent with GSEA results (shown in Figure 3C), which are also highly enriched in pathways with positive enrichment scores (NES). Combined with differential expression analysis, it could be identified that some DEGs, i. e, RETN, DEFA1, ANXA3, LTF, and LCN2, are specifically involved in neutrophil, myeloid cell activation, and mediated immunity events. The complete list of GO-GSEA results were summarized in Supplementary Table S6. For the KEGG enrichment analysis, the most significant results were focused on the IL-17 signaling pathway and TNF signaling pathway. The complete enriched results of GO and KEGG analysis are summarized in Supplementary Table S7.
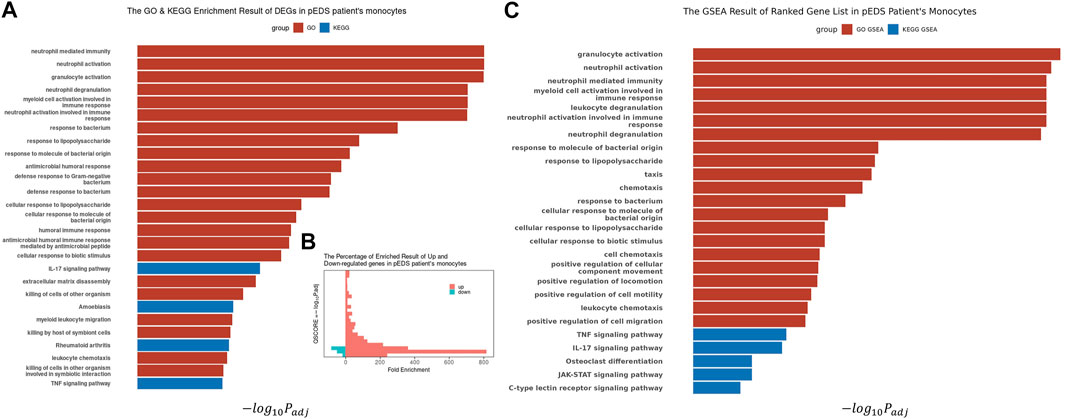
FIGURE 3. The Biological Processes GO Enrichment Result and GSEA result of DEGs in pEDS patients’ monocytes. (A) Biological processes related to inflammation response and immune response are significantly enriched; (B) Most of the significant results are contributed by upregulated genes, which also have higher Fold Enrichment (defined as GeneRatio/BgRatio in clusterProfiler) compared to downregulated genes; (C) The GSEA analysis result also showed similar enriched result in pathways related to immunity, inflammation, IL-17 pathway and TNF-α pathway.
The Disease Ontology Enrichment Analysis of DEGs in Monocytes and Validation by Reverse Transcription-qPCR and Enzyme-Linked Immunosorbent Assay
The detailed mechanism behind complement pathway disruption and related pEDS clinical manifestations is not yet explained. Disease Ontology (DO) was developed to create a consistent description of gene products with disease perspectives, and accurate disease descriptions can discover new relationships between genes and disease (Schriml et al., 2012). Thus, we performed DO analysis with upregulated DEGs to help us to better understand the relationship between the mutant variants and pEDS. As shown in Figure 4A, the DO enriched results can be divided into several categories, which is separately related to different aspects of clinical features of pEDS: periodontal destruction, skin fragility, vascular complication, and joint hypermobility [Colors represent different clinical features of pEDS; Horizontal axis represent Fold Enrichment of each DO category, which is defined as GeneRatio/BgRatio in clusterProfiler (Yu et al., 2015)]. Besides, to identify genes related to the pathogenesis of pEDS, the overlapping between DO categories of periodontal destruction, vascular complications, and skin disease were selected (Figure 4B). The complete list of DO terms of upregulated DEGs is shown in Supplementary Table S8. The selected DEGs including MMP9, VEGFA, IL10, IL1A, IL1B, IL2RA, and IL6 were identified and validated by RT-qPCR. All 7 genes are significantly expressed in the pEDS group compared to controls (Figure 5A). MMP9, IL10, IL1A, IL1B, IL6 were also measured as the top 10 hub genes (Supplementary Figure S2B) by cytoHubba (Chin et al., 2014), confirming their important roles in pathomechanism of pEDS. ELISA was also performed to measure the IL-1
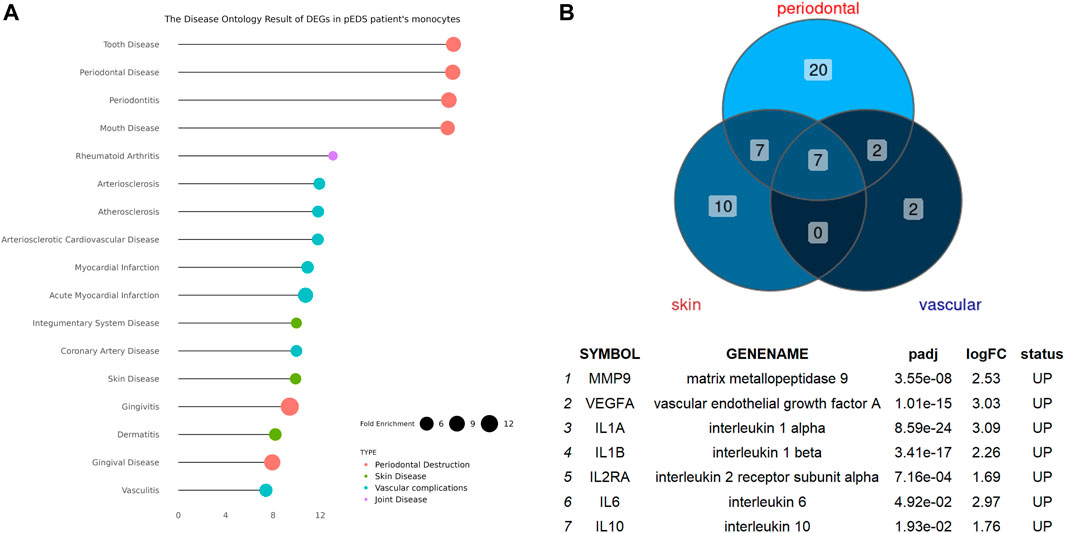
FIGURE 4. The Disease Ontology Enrichment Analysis Result of DEGs in pEDS patients’ monocytes and the overlapped enriched genes between DO categories of periodontal destruction, vascular complication, and skin disease in pEDS patients’ monocytes. (A) The DO results can be divided into four categories which are separately related to different clinical features of pEDS: periodontal destruction, skin fragility, vascular complication, and joint hypermobility (Colors represent different clinical features of pEDS; Horizontal axis represent Fold Enrichment of each DO category, which is defined as GeneRatio/BgRatio in clusterProfiler); (B) seven genes including MMP9, VEGFA, IL10, IL1A, IL1B, IL2RA, and IL6 have been found as the overlapped genes in three different DO categories and all of them were significantly upregulated in monocytes of pEDS.
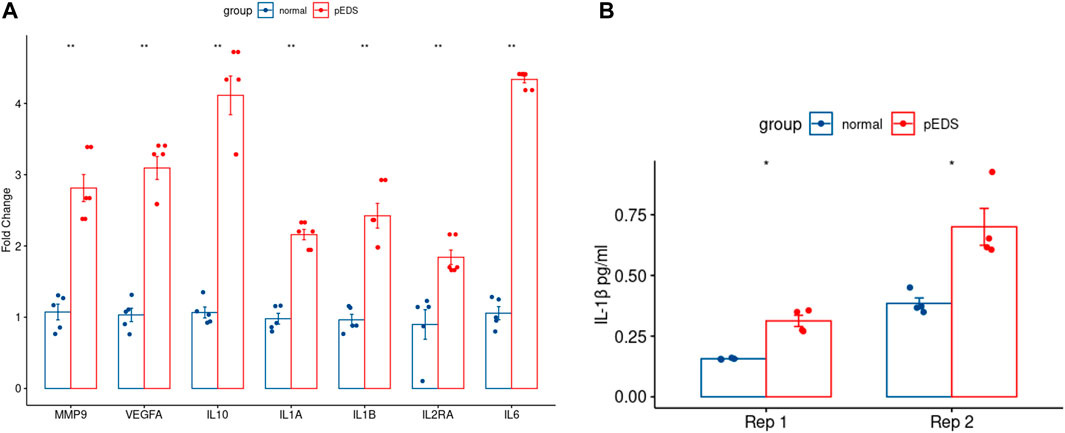
FIGURE 5. RT-qPCR of the overlapped genes and the ELISA result of IL-1β expression in pEDS patients’ monocytes were performed to validate the RNA sequencing results of pEDS patients. (A) In RT-qPCR analysis, gene expression levels were measured in four independent replicates per subject, and t-tests were performed (ns = not significant, *p < 0.05, **p < 0.01, ***p < 0.001, ****p < 0.0001). All seven genes including MMP9, VEGFA, IL10, IL1A, IL1B, IL2RA, and IL6 are significantly expressed in pEDS group (p < 0.01); (B) For ELISA experiment, data are expressed as mean (SD). (ns = not significant, *p < 0.05, **p < 0.01, ***p < 0.001, ****p < 0.0001). IL-1β concentration was also significantly higher in the monocytes of pEDS patients (p < 0.05).
STRING Network
As shown in Figure 6, the STRING network shows the relationship between genes involved in the classical complement activation pathway and the DEGs related to periodontitis. The overlapped genes from DO analysis including MMP9, VEGFA, IL10, IL1A, IL1B, IL2RA, IL6 are also in the STRING networks and showed closer interaction among the nodes. The pathogenic mutant variant, C1R/C1S were located the upstream of STRING network and not directly connected to the periodontitis-related genes. Additionally, in the extended STRING network consisted of classical complement pathway and DEGs in this study (Supplementary Figure S2A), the nodes of C1R/C1S only connected to other complement components but not any DEG, which implied that the extracellularly active C1s protein might have other potential targets that has yet been found (Bally et al., 2019).
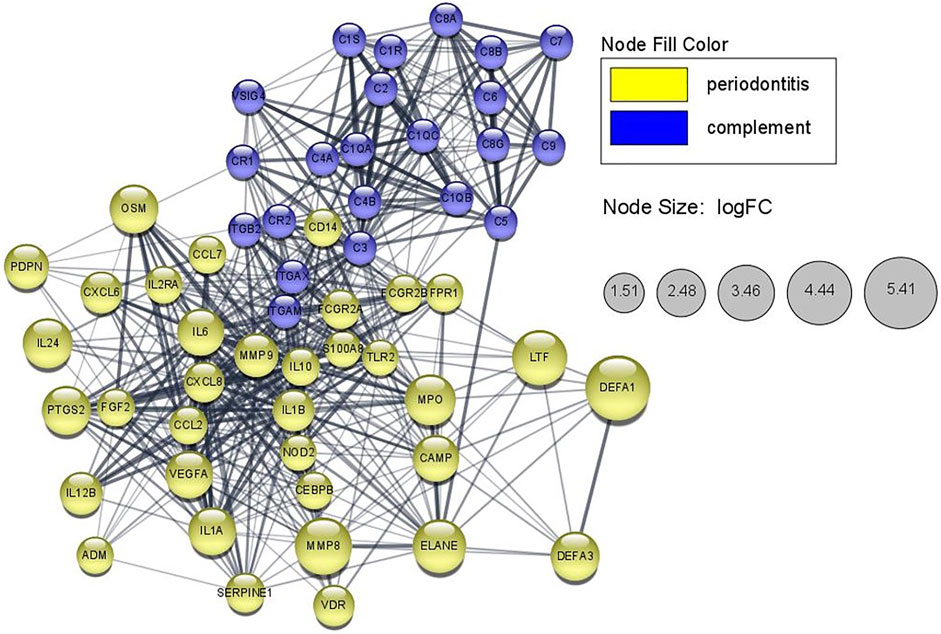
FIGURE 6. STRING network showing periodontitis-related proteins that possibly interacted with the classical complement pathway in monocytes of pEDS patients. The lines represent interaction associations between nodes and line thickness indicates the strength of data support. The node size in periodontitis-related genes is mapped to the logFC values of gene expression value in monocytes of pEDS patients.
Discussion
Transcriptomics have been utilized to disclose the key alterations of biological processes triggering human diseases, thus offering novel instruments useful not only for the comprehension of their underlying mechanisms but also for their molecular diagnosis and clinical therapy (Casamassimi et al., 2017). Previous studies (Chiarelli et al., 2016, 2018, 2019; Lim et al., 2019) performed transcriptome analysis on other EDS subtypes, which succeeded in revealing some of the mechanisms underlying these rare diseases. Up until now, the pathophysiology of pEDS is not well understood, and the current study described the first molecular evidence of significant gene expression changes in pEDS cells (monocytes and gingival fibroblasts) that could provide insights into the pathogenesis of the disease. Although the sample size is small and the findings will therefore need to be confirmed in other patients, our results provide a step forward towards understanding of the complex pathogenetic basis of pEDS. Multiple DEGs were identified only in monocytes but not in gingival fibroblasts. Approximately 3% of the detected transcriptome showing differential expression in monocytes and a total of 338 DEGs were identified in monocytes compared to normal control. ORA and GSEA analysis observed changes in neutrophil-mediated immunity, response to bacterium, humoral immune response, IL-17 pathway, and TNF-α pathway, of which are related to immune response and inflammatory response; DO analysis also identified potential target genes including MMP9, VEGFA, IL10, IL1A, IL1B, IL2RA, and IL6, which may be related to periodontal destruction, vascular complication, and skin disease of pEDS; In addition, STRING network analysis showed the relationship between genes involved in the classical complement activation pathway and the DEGs related to periodontitis. The overlapped genes from DO analysis are also in the STRING networks and some of them are considered as hub genes which highly interacted with other DEGs. This finding implied that these genes might play important roles in the pathophysiology of pEDS.
IL-17 Pathway and Periodontal Destruction
In ORA and GSEA, genes related to the IL-17 pathway were enriched in pEDS monocytes. Previous studies has reported that, IL-17, IL-17–producing lymphocytes and innate immune cells are potentially important players in the pathogenesis of periodontitis (Hajishengallis, 2014). Besides, elevated expression level of IL-17 has been closely related to periodontitis (Ohyama et al., 2009; Darveau, 2010). Overall, IL-17 is a double-edged sword when it comes to inflammatory illnesses like periodontitis (Zenobia & Hajishengallis, 2015).
On the one hand, IL-17 has been demonstrated to protect against extracellular pathogens (Khader et al., 2009; Hernandez-Santos and Gaffen, 2012) and can trigger the generation of antimicrobial peptides (Liang et al., 2010), which are assumed to be protective in periodontitis (Diamond et al., 2008; Gorr, 2009). On the other hand, through upregulating matrix metalloproteases and RANKL, IL-17 can also facilitate connective tissue degradation and bone resorption (Lubberts, 2008). IL-17 could also facilitate neutrophil recruitment and increase inflammation by downregulating the endogenous anti-inflammatory molecule Del-1 (Eskan et al., 2012).
IL17 can also be involved in periodontitis development through the complement pathway. Complement and IL-17 are both involved in the regulation of neutrophil recruitment, which is an important mechanism in maintaining periodontal homeostasis (Eskan et al., 2012; Hajishengallis and Hajishengallis, 2014). It is also reported that single nucleotide polymorphisms in the components C5 and IL-17 have been linked to a higher risk of periodontal disease (Chai et al., 2010a; Corrêa et al., 2012; Kadkhodazadeh et al., 2013), implying that both molecules may play a role in the illness’s development. Although complement has a complex effect on IL-17 expression, it has been observed that complement, in collaboration with Toll-like receptors, can increase IL-17 synthesis in murine periodontal tissue (Abe et al., 2012). Mice lacking either C5Ar or Toll-like receptor-2, on the other hand, are protected from experimental periodontitis (Hajishengallis et al., 2011; Liang et al., 2011). In pEDS, the extracellularly activated C1s can activate the classical complement cascade and cleave more C4 and C2, which are components of the C3 convertase complex in the classical pathway (Bally et al., 2019). This disruption might ultimately induce the production of C5a (Ricklin and Lambris, 2013). With the support of Toll-like receptor-2 (TLR2 is also one of the upregulated DEGs and top 10 hub genes found in monocytes), abundant C5a may activate C5aR and upregulate IL-17, IL-1, IL-6, and TNF (Abe et al., 2012). Ultimately, IL-17 could facilitate neutrophil recruitment and result in periodontal destruction in synergy of other cytokines (Abe et al., 2012).
Selected DEGs and Periodontal Destruction
Apart from IL-17, other upregulated genes we identified in DO analysis also can be potential players in the periodontal destruction of pEDS. For upregulated MMP9, it has been suggested that matrix metalloproteinases (MMPs) are key proteases involved in destructive periodontal diseases (Buduneli and Kinane, 2011; Sorsa et al., 2011). The most prevalent MMPs in periodontal tissues are MMP-8 and MMP-9, which reflect the severity, development, and treatment response of periodontal disease (Mäntylä et al., 2006). MMP-9 has been linked to periodontal soft tissue degradation and has been found to work with MMP-13 in alveolar bone resorption and periodontal tissue distruction (Hernández et al., 2006; Hernández et al., 2011).
IL-1α, IL-1β and IL-6 are proinflammatory cytokines that are thought to play a role in periodontitis development. Nonsurgical periodontal therapy has been shown to result in a statistically significant reduction in overall levels of these cytokines in gingival crevicular fluid (GCF) (Reis et al., 2014). Nonetheless, IL-6 and IL-10 polymorphisms are found to be potential risk factors for periodontitis (Scapoli et al., 2012). In addition, Afacan et al. reported that the concentrations of VEGF and TNF-α in GCF were significantly higher in the periodontitis group than in the gingivitis and healthy groups (Afacan et al., 2018). Total amounts of VEGF and TNF-α in GCF were positively correlated with the site-specific clinical periodontal parameters and with each other. Increased GCF VEGF and TNF-α levels in both chronic and aggressive forms of periodontitis might suggest the role of the TNF-α/VEGF pathway in the pathogenesis of periodontal diseases (Afacan et al., 2018). In conclusion, the up-regulation of these selected DEGs is strongly connected to the pathogenesis of periodontitis and has the potential to be the possible pharmacological target.
Classical Complement Pathway and Periodontitis
The gain-of-function C1R and/or C1S variants are now recognized to be the essential element in the pathogenesis of pEDS. The extracellular presence of activated C1s can activate the conventional complement cascade without the presence of other signals (Gröbner et al., 2019). In vitro studies suggests that the production or release of active C1r serine protease may promote gingival hyperinflammation in response to mild biofilm, leading to severe periodontal damage (Bally et al., 2019; Gröbner et al., 2019).
The potential role of complement in human periodontitis was first recognized in the 1970s and 1980s when researchers looked into the GCF under different periodontal statuses (Attstroum et al., 1975; Courts et al., 1977; Schenkein and Genco, 1977). GCF samples from periodontitis patients were found to have complement-dependent hemolytic activity, indicating that GCF contains a functional complement system (Courts et al., 1977; Boackle, 1991). Recent studies also suggest an association between complement and periodontitis. A rare case of aggressive periodontitis with gingival angioedema was linked to deficiency of the C1INH (Roberts et al., 2003). People with periodontitis had a significantly higher occurrence of a single nucleotide polymorphism affecting C5 (rs17611), compared to healthy controls (Chai et al., 2010b). Another study identified C3 as one of the top 21 most promising candidate genes involved in periodontal disease using microarray experiments (Zhan et al., 2014). As for C5, it was reported that C5a can induce the activation of C5Ar in a murine periodontal disease and cause significant bone loss with the help of cytokines like IL-17, IL-1β, IL-6, and TNF (Abe et al., 2012). Conversely, C4 might have a protective effect against periodontitis since partial C4 gene deficiencies are significantly more common in periodontitis patients than in healthy individuals (Seppänen et al., 2007).
In this study, STRING network analysis showed a close relationship between genes involved in the classical complement pathway and DEGs in this study. C3, as previously reported, was located as the central element in the complement pathway (Ricklin and Lambris, 2013) and also showed closer links to other nodes in the STRING network. Meanwhile, C1R and C1S were upstream of the classical complement pathway and not directly connected to DEGs according to the evidences provided by STRING. Several DEGs such as MMP9, VEGFA, IL10, IL1A, IL1B, and IL6 that validated by RT-qPCR were also included in the STRING network and showed close connections to the complement pathway, which implied that they may play as important regulators between C1R/C1S mutation and downstream periodontal phenotypes. However, further research is necessary to confirm the relationship between genes involved in the classical complement pathway and our selected DEGs.
Limitations
Firstly, we acknowledged that our study has a small sample size (due to patient cohort size), which may affect the power of some observations. In addition, only two pEDS patients were enrolled and one of them was affcted by both pEDS and vEDS, which means our result need to be confirmed in other patients in further research. Besides, compared to previous transcriptomic profiling of other EDS subtypes (Chiarelli et al., 2016, 2018, 2019), we used gingival fibroblasts rather than skin fibroblasts. It is not certain that other ECM producing cells, i.e., vascular smooth muscle cells, and skin fibroblasts, would show the same results in terms of gene expression profile. Finally, in the present study, we only validated the RNA-sequencing result by RT-qPCR and ELISA. Further research must be designed and performed to validate the results we found in this study.
Conclusion
In conclusion, our approach illustrates global Mrna profiling changes of several genes and related biological processes that could offer novel insights into the pEDS pathophysiology. Approximately 3% of the detected transcriptome showed differential expression and were identified only in monocytes but not in gingival fibroblasts, multiple DEGs were enriched in neutrophil-mediated immunity, response to bacterium, humoral immune response, IL-17 pathway, and TNF-
Data Availability Statement
The datasets presented in this study can be found in online repositories. The names of the repository/repositories and accession number(s) can be found below: https://www.ncbi.nlm.nih.gov/geo/query/acc.cgi?acc=GSE190786.
Ethics Statement
The studies involving human participants were reviewed and approved by the Ethics Committee of Nanjing Stomatological Hospital, Medical School of Nanjing University. The patients/participants provided their written informed consent to participate in this study. Written informed consent was obtained from the individual(s) for the publication of any potentially identifiable images or data included in this article.
Author Contributions
Contribution: TZ and NW performed RNA sequencing experiments; ZL analyzed results; JC made the figures; TZ recruited patients, performed consent, and recorded clinical data; ZL, WS, and JW designed the research and wrote the paper.
Funding
This work was supported by grants from the Natural Science Foundation of Jiangsu Province (BK20200149), Medical Science and technology development Foundation, Nanjing Department of Health (YKK20152), and National Natural Science Foundation of China (82001111). The sponsors of this study are public or nonprofit organizations that support science in general.
Conflict of Interest
The authors declare that the research was conducted in the absence of any commercial or financial relationships that could be construed as a potential conflict of interest.
Publisher’s Note
All claims expressed in this article are solely those of the authors and do not necessarily represent those of their affiliated organizations, or those of the publisher, the editors and the reviewers. Any product that may be evaluated in this article, or claim that may be made by its manufacturer, is not guaranteed or endorsed by the publisher.
Acknowledgments
The authors thank the patients for their kind availability for this study.
Supplementary Material
The Supplementary Material for this article can be found online at: https://www.frontiersin.org/articles/10.3389/fgene.2022.834928/full#supplementary-material
Supplementary Figure S1 | Oral photographs of all individuals enrolled in this study.
Supplementary Figure S2 | The extended STRING network as well as the top 10 hub genes calculated by cytoHubba. A: The STRING network constructed by the classical complement pathway and all DEGs. C1R/C1S nodes only showed direct connections to other complement component. B: Top 10 hub genes included CCL2, CXCL1 ,CXCL2, CXCL8, IL1A, IL1B, IL6, IL10, MMP9 and TLR2. the color from yellow to red denotes the degree of hub genes interacted with other DEGs.
Supplementary Table S1 | All the R packages and software used for RNA sequencing data analysis in this study.
Supplementary Table S2 | Specific gene primers used for RT-qPCR in the study.
Supplementary Table S3 | The normalized read counts of monocytes.
Supplementary Table S4 | The normalized read counts of fibroblasts.
Supplementary Table S5 | List of DEGs that are up-regulated and down-regulated in pEDS patients’ monocytes over controls.
Supplementary Table S6 | The complete list of GO-GSEA results in pEDS patients’ monocytes over controls.
Supplementary Table S7 | The complete list of enriched GO and KEGG terms of DEGs in pEDS patients’ monocytes over controls.
Supplementary Table S8 | The complete list of enriched DO terms of upregulated DEGs in pEDS patients’ monocytes over controls.
References
Abe, T., Hosur, K. B., Hajishengallis, E., Reis, E. S., Ricklin, D., Lambris, J. D., et al. (2012). Local Complement-Targeted Intervention in Periodontitis: Proof-Of-Concept Using a C5a Receptor (CD88) Antagonist. J.I. 189, 5442–5448. doi:10.4049/jimmunol.1202339
Afacan, B., Öztürk, V. Ö., Paşalı, Ç., Bozkurt, E., Köse, T., and Emingil, G. (2018). Gingival Crevicular Fluid and Salivary HIF‐1α, VEGF, and TNF‐α Levels in Periodontal Health and Disease. J. Periodontol. 18, 0412. doi:10.1002/jper.18-0412
Anders, S., and Huber, W. (2010). Differential Expression Analysis for Sequence Count Data. Genome Biol. 11 (10), R106. doi:10.1186/gb-2010-11-10-r106
Attstroum, R., Laurel, A.-B., Lahsson, U., and Sjouholm, A. (1975). Complement Factors in Gingival Crevice Material from Healthy and Inflamed Gingiva in Humans. J. Periodontal Res. 10, 19–27. doi:10.1111/j.1600-0765.1975.tb00003.x
Bally, I., Dalonneau, F., Chouquet, A., Gröbner, R., Amberger, A., Kapferer-Seebacher, I., et al. (2019). Two Different Missense C1S Mutations, Associated to Periodontal Ehlers-Danlos Syndrome, Lead to Identical Molecular Outcomes. Front. Immunol. 10, 2962. doi:10.3389/fimmu.2019.02962
Bally, I., Dalonneau, F., Chouquet, A., Gröbner, R., Amberger, A., Kapferer-Seebacher, I., et al. (2019). Two Different Missense C1S Mutations, Associated to Periodontal Ehlers-Danlos Syndrome, lead to Identical Molecular Outcomes. Front. Immunol. 10, 2962. doi:10.3389/fimmu.2019.02962
Beikler, T., Peters, U., Prior, K., Eisenacher, M., and Flemmig, T. F. (2008). Gene Expression in Periodontal Tissues Following Treatment. BMC Med. Genomics 1, 30. doi:10.1186/1755-8794-1-30
Blackburn, P. R., Xu, Z., Tumelty, K. E., Zhao, R. W., Monis, W. J., Harris, K. G., et al. (2018). Bi-allelic Alterations in AEBP1 Lead to Defective Collagen Assembly and Connective Tissue Structure Resulting in a Variant of Ehlers-Danlos Syndrome. Am. J. Hum. Genet. 102, 696–705. doi:10.1016/j.ajhg.2018.02.018
Blighe, K., Rana, S., and Lewis, M. (2021). EnhancedVolcano: Publication-Ready Volcano Plots with Enhanced Colouring and Labeling. .
Boackle, R. J. (1991). The Interaction of Salivary Secretions with the Human Complement System - A Model for the Study of Host Defense Systems on Inflamed Mucosal Surfaces. Crit. Rev. Oral Biol. Med. 2, 355–367. doi:10.1177/10454411910020030401
Buduneli, N., and Kinane, D. F. (2011). Host-derived Diagnostic Markers Related to Soft Tissue Destruction and Bone Degradation in Periodontitis. J. Clin. Periodontol. 38, 85–105. doi:10.1111/j.1600-051x.2010.01670.x
Casamassimi, A., Federico, A., Rienzo, M., Esposito, S., and Ciccodicola, A. (2017). Transcriptome Profiling in Human Diseases: New Advances and Perspectives. Ijms 18 (8), 1652. doi:10.3390/ijms18081652
Chai, L., Song, Y.-Q., Zee, K.-Y., and Leung, W. K. (2010a). Single Nucleotide Polymorphisms of Complement Component 5 and Periodontitis. J. Periodontal Res. 45, 301–308. doi:10.1111/j.1600-0765.2009.01234.x
Chai, L., Song, Y.-Q., Zee, K.-Y., and Leung, W. K. (2010b). Single Nucleotide Polymorphisms of Complement Component 5 and Periodontitis. J. Periodontal Res. 45, 301–308. doi:10.1111/j.1600-0765.2009.01234.x
Chiarelli, N., Carini, G., Zoppi, N., Dordoni, C., Ritelli, M., Venturini, M., et al. (2016). Transcriptome-Wide Expression Profiling in Skin Fibroblasts of Patients with Joint Hypermobility Syndrome/Ehlers-Danlos Syndrome Hypermobility Type. PLoS ONE 11, e0161347. doi:10.1371/journal.pone.0161347
Chiarelli, N., Carini, G., Zoppi, N., Dordoni, C., Ritelli, M., Venturini, M., et al. (2016). Transcriptome-wide Expression Profiling in Skin Fibroblasts of Patients with Joint Hypermobility Syndrome/Ehlers-Danlos Syndrome Hypermobility Type. PLoS ONE 11 (8), e0161347. doi:10.1371/journal.pone.0161347
Chiarelli, N., Carini, G., Zoppi, N., Ritelli, M., and Colombi, M. (2019). Molecular Insights in the Pathogenesis of Classical Ehlers-Danlos Syndrome from Transcriptome-wide Expression Profiling of Patients' Skin Fibroblasts. PLoS ONE 14, e0211647. doi:10.1371/journal.pone.0211647
Chiarelli, N., Carini, G., Zoppi, N., Ritelli, M., and Colombi, M. (2018). Transcriptome Analysis of Skin Fibroblasts with Dominant Negative COL3A1 Mutations Provides Molecular Insights into the Etiopathology of Vascular Ehlers-Danlos Syndrome. PLoS ONE 13, e0191220. doi:10.1371/journal.pone.0191220
Chiarelli, N., Carini, G., Zoppi, N., Ritelli, M., and Colombi, M. (2018). Transcriptome Analysis of Skin Fibroblasts with Dominant Negative COL3A1 Mutations Provides Molecular Insights into the Etiopathology of Vascular Ehlers-Danlos Syndrome. PLoS ONE 13 (1), e0191220. doi:10.1371/journal.pone.0191220
Chiarelli, N., Ritelli, M., Zoppi, N., and Colombi, M. (2019). Cellular and Molecular Mechanisms in the Pathogenesis of Classical, Vascular, and Hypermobile Ehlers‒Danlos Syndromes. Genes 10, 609. doi:10.3390/genes10080609
Chin, C.-H., Chen, S.-H., Wu, H.-H., Ho, C.-W., Ko, M.-T., and Lin, C.-Y. (2014). cytoHubba: Identifying Hub Objects and Sub-networks from Complex Interactome. BMC Syst. Biol. 8. doi:10.1186/1752-0509-8-S4-S11
Corrêa, J. D., Madeira, M. F., Resende, R. G., Correia-Silva, Jde. F., Gomez, R. S., de Souza, Dda. G., et al. (20122012). Association between Polymorphisms in interleukin-17A and -17F Genes and Chronic Periodontal Disease. Mediators Inflamm. 2012, 846052. doi:10.1155/2012/846052
Courts, F. J., Boackle, R. J., Fudenberg, H. H., and Silverman, M. S. (1977). Detection of Functional Complement Components in Gingival Crevicular Fluid from Humans with Periodontal Disease. J. Dent Res. 56, 327–331. doi:10.1177/00220345770560032001
Darveau, R. P. (2010). Periodontitis: A Polymicrobial Disruption of Host Homeostasis. Nat. Rev. Microbiol. 8, 481–490. doi:10.1038/nrmicro2337
Diamond, G., Beckloff, N., and Ryan, L. K. (2008). Host Defense Peptides in the Oral Cavity and the Lung: Similarities and Differences. J. Dent Res. 87, 915–927. doi:10.1177/154405910808701011
Doncheva, N. T., Morris, J. H., Gorodkin, J., and Jensen, L. J. (2019). Cytoscape StringApp: Network Analysis and Visualization of Proteomics Data. J. Proteome Res. 18, 623–632. doi:10.1021/acs.jproteome.8b00702
Dutzan, N., Konkel, J. E., Greenwell-Wild, T., and Moutsopoulos, N. M. (2016). Characterization of the Human Immune Cell Network at the Gingival Barrier. Mucosal Immunol. 9, 1163–1172. doi:10.1038/mi.2015.136
Eskan, M. A., Jotwani, R., Abe, T., Chmelar, J., Lim, J.-H., Liang, S., et al. (2012). The Leukocyte Integrin Antagonist Del-1 Inhibits IL-17-mediated Inflammatory Bone Loss. Nat. Immunol. 13, 465–473. doi:10.1038/ni.2260
G’al, P., Dob’o, J., Z’avodszky, P., and Sim, R. B. M. (2009). Early Complement Proteases: C1r, C1s and MASPs. A Structural Insight into Activation and Functions. Mol. Immunol. 46, 2745–2752.
Gorr, S.-U. (20092000). Antimicrobial Peptides of the Oral Cavity. Periodontol 51, 152–180. doi:10.1111/j.1600-0757.2009.00310.x
Gröbner, R., Kapferer-Seebacher, I., Amberger, A., Redolfi, R., Dalonneau, F., Björck, E., et al. (2019). C1R Mutations Trigger Constitutive Complement 1 Activation in Periodontal Ehlers-Danlos Syndrome. Front. Immunol. 10, 2537. doi:10.3389/fimmu.2019.02537
Gu, Z., Eils, R., and Schlesner, M. (2016). Complex Heatmaps Reveal Patterns and Correlations in Multidimensional Genomic Data. Bioinformatics 32 (18), 2847–2849. doi:10.1093/bioinformatics/btw313
Hajishengallis, E., and Hajishengallis, G. (2014). Neutrophil Homeostasis and Periodontal Health in Children and Adults. J. Dent Res. 93, 231–237. doi:10.1177/0022034513507956
Hajishengallis, G. (2014). Immunomicrobial Pathogenesis of Periodontitis: Keystones, Pathobionts, and Host Response. Trends Immunol. 35, 3–11. doi:10.1016/j.it.2013.09.001
Hajishengallis, G., Liang, S., Payne, M. A., Hashim, A., Jotwani, R., Eskan, M. A., et al. (2011). Low-abundance Biofilm Species Orchestrates Inflammatory Periodontal Disease through the Commensal Microbiota and Complement. Cell Host & Microbe 10, 497–506. doi:10.1016/j.chom.2011.10.006
Hernández, M., Dutzan, N., García-Sesnich, J., Abusleme, L., Dezerega, A., Silva, N., et al. (2011). Host-Pathogen Interactions in Progressive Chronic Periodontitis. J. Dent Res. 90, 1164–1170. doi:10.1177/0022034511401405
Hernandez, M., Valenzuela, M. A., Lopez-Otin, C., Alvarez, J., Lopez, J. M., Vernal, R., et al. (2006). Matrix Metalloproteinase-13 Is Highly Expressed in Destructive Periodontal Disease Activity. J. Periodontol. 77, 1863–1870. doi:10.1902/jop.2006.050461
Hern’andez-Santos, N., and Gaffen, S. L. (2012). Th17 Cells in Immunity to Candida Albicans. Cell Host Microbe 11, 425–435.
Kadkhodazadeh, M., Baghani, Z., Ebadian, A. R., Youssefi, N., Mehdizadeh, A. R., and Azimi, N. (2013). IL-17 Gene Polymorphism Is Associated with Chronic Periodontitis and Peri-Implantitis in Iranian Patients: A Cross-Sectional Study. Immunological Invest. 42, 156–163. doi:10.3109/08820139.2012.746697
Kapferer-Seebacher, I., Oakley-Hannibal, E., Lepperdinger, U., Johnson, D., Ghali, N., Brady, A. F., et al. (2021). Prospective Clinical Investigations of Children with Periodontal Ehlers-Danlos Syndrome Identify Generalized Lack of Attached Gingiva as a Pathognomonic Feature. Genet. Med. 23, 316–322. doi:10.1038/s41436-020-00985-y
Kapferer-Seebacher, I., Pepin, M., Werner, R., Aitman, T. J., Nordgren, A., Stoiber, H., et al. (2016). Periodontal Ehlers-Danlos Syndrome Is Caused by Mutations in C1R and C1S , Which Encode Subcomponents C1r and C1s of Complement. Am. J. Hum. Genet. 99, 1005–1014. doi:10.1016/j.ajhg.2016.08.019
Kassambara, A. (2020). Ggpubr: ’ggplot2’ Based Publication Ready Plots. Available at: https://CRAN.R-project.org/package=ggpubr.
Khader, S. A., Gaffen, S. L., and Kolls, J. K. (2009). Th17 Cells at the Crossroads of Innate and Adaptive Immunity against Infectious Diseases at the Mucosa. Mucosal Immunol. 2, 403–411. doi:10.1038/mi.2009.100
Liang, S. C., Nickerson-Nutter, C., Pittman, D. D., Carrier, Y., Goodwin, D. G., Shields, K. M., et al. (2010). IL-22 Induces an Acute-phase Response. J.I. 185, 5531–5538. doi:10.4049/jimmunol.0904091
Liang, S., Krauss, J. L., Domon, H., McIntosh, M. L., Hosur, K. B., Qu, H., et al. (2011). The C5a Receptor Impairs IL-12-Dependent Clearance ofPorphyromonas Gingivalisand Is Required for Induction of Periodontal Bone Loss. J.I. 186, 869–877. doi:10.4049/jimmunol.1003252
Ligges, U., and Mächler, M. (2003). Scatterplot3d—An R Package for Visualizing Multivariate Data. J. Stat. Softw. 8 (11), 1–20. doi:10.18637/jss.v008.i11
Lim, L., Opitz, L., Opitz, H., and Rohrbach, G. (2019). Transcriptome Profiling of Primary Skin Fibroblasts Reveal Distinct Molecular Features between PLOD1- and FKBP14-Kyphoscoliotic Ehlers-Danlos Syndrome. Genes 10 (7), 517. doi:10.3390/genes10070517
Love, M. I., Huber, W., and Anders, S. (2014). Moderated Estimation of Fold Change and Dispersion for RNA-Seq Data with DESeq2. Genome Biol. 15, 550. doi:10.1186/s13059-014-0550-8
Lubberts, E. (2008). IL-17/Th17 Targeting: On the Road to Prevent Chronic Destructive Arthritis? Cytokine 41, 84–91. doi:10.1016/j.cyto.2007.09.014
Malfait, F., Francomano, C., Byers, P., Belmont, J., Berglund, B., Black, J., et al. (2017). The 2017 International Classification of the Ehlers-Danlos Syndromes. Am. J. Med. Genet. C Semin. Med. Genet. 175, 8–26. doi:10.1002/ajmg.c.31552
Mäntylä, P., Stenman, M., Kinane, D., Salo, T., Suomalainen, K., Tikanoja, S., et al. (2006). Monitoring Periodontal Disease Status in Smokers and Nonsmokers Using a Gingival Crevicular Fluid Matrix Metalloproteinase-8-specific Chair-Side Test. J. Periodontal Res. 41, 503–512. doi:10.1111/j.1600-0765.2006.00897.x
Ohyama, H., Kato-Kogoe, N., Kuhara, A., Nishimura, F., Nakasho, K., Yamanegi, K., et al. (2009). The Involvement of IL-23 and the Th17 Pathway in Periodontitis. J. Dent Res. 88, 633–638. doi:10.1177/0022034509339889
Reis, C., Da Costa, A. V., Guimarães, J. T., Tuna, D., Braga, A. C., Pacheco, J. J., et al. (2014). Clinical Improvement Following Therapy for Periodontitis: Association with a Decrease in IL-1 and IL-6. Exp. Ther. Med. 8, 323–327. doi:10.3892/etm.2014.1724
Ricklin, D., and Lambris, J. D. (2013). Complement in Immune and Inflammatory Disorders: Pathophysiological Mechanisms. J.I. 190, 3831–3838. doi:10.4049/jimmunol.1203487
Ritchie, M. E., Phipson, B., Wu, D., Hu, Y., Law, C. W., Shi, W., et al. (2015). Limma powers Differential Expression Analyses for RNA-Sequencing and Microarray Studies. Nucleic Acids Res. 43 (7), e47. doi:10.1093/nar/gkv007
Roberts, A., Shah, M., and Chapple, I. L. C. (2003). C-1 Esterase Inhibitor Dysfunction Localised to the Periodontal Tissues: Clues to the Role of Stress in the Pathogenesis of Chronic Periodontitis? J. Clin. Periodontol. 30, 271–277. doi:10.1034/j.1600-051x.2003.01266.x
Robinson, M. D., McCarthy, D. J., and Smyth, G. K. (2010). EdgeR: a Bioconductor Package for Differential Expression Analysis of Digital Gene Expression Data. Bioinformatics 26 (1), 139–140. doi:10.1093/bioinformatics/btp616
Scapoli, L., Girardi, A., Palmieri, A., Carinci, F., Testori, T., Zuffetti, F., et al. (2012). IL6 and IL10 Are Genetic Susceptibility Factors of Periodontal Disease. Dent Res. J. (Isfahan) 9, S197–S201. doi:10.4103/1735-3327.109754
Schenkein, H. A., and Genco, R. J. (1977). Gingival Fluid and Serum in Periodontal Diseases: II. Evidence for Cleavage of Complement Components C3, C3 Proactivator (Factor B) and C4 in Gingival Fluid. J. Periodontol. 48, 778–784. doi:10.1902/jop.1977.48.12.778
Schriml, L. M., Arze, C., Nadendla, S., Chang, Y.-W. W., Mazaitis, M., Felix, V., et al. (2012). Disease Ontology: A Backbone for Disease Semantic Integration. Nucleic Acids Res. 40, D940–D946. doi:10.1093/nar/gkr972
Seppänen, M., Lokki, M.-L., Notkola, I.-L., Mattila, K., Valtonen, V., Nieminen, A., et al. (2007). Complement and C4 Null Alleles in Severe Chronic Adult Periodontitis. Scand. J. Immunol. 65, 176–181. doi:10.1111/j.1365-3083.2006.01886.x
Shannon, P., Markiel, A., Ozier, O., Baliga, N. S., Wang, J. T., Ramage, D., et al. (2003). Cytoscape: A Software Environment for Integrated Models of Biomolecular Interaction Networks. Genome Res. 13, 2498–2504. doi:10.1101/gr.1239303
Sorsa, T., Mäntylä, P., Tervahartiala, T., Pussinen, P. J., Gamonal, J., and Hernandez, M. (2011). MMP Activation in Diagnostics of Periodontitis and Systemic Inflammation. J. Clin. Periodontol. 38, 817–819. doi:10.1111/j.1600-051x.2011.01753.x
Szklarczyk, D., Gable, A. L., Nastou, K. C., Lyon, D., Kirsch, R., Pyysalo, S., et al. (2021). The STRING Database in 2021: Customizable Protein-Protein Networks, and Functional Characterization of User-Uploaded Gene/measurement Sets. Nucleic Acids Res. 49, D605–D612. doi:10.1093/nar/gkaa1074
Wickham, H. (2016). ggplot2: Elegant Graphics for Data Analysis. Springer-Verlag New York. Available at: https://ggplot2.tidyverse.org.
Wu, J., Yang, J., Zhao, J., Wu, J., Zhang, X., Leung, W. K., et al. (2018). A Chinese Family with Periodontal Ehlers-Danlos Syndrome Associated with Missense Mutation in the C1R Gene. J. Clin. Periodontol. 45, 1311–1318. doi:10.1111/jcpe.12988
Wu, T., Hu, E., Xu, S., Chen, M., Guo, P., Dai, Z., et al. (2021). clusterProfiler 4.0: A Universal Enrichment Tool for Interpreting Omics Data. The Innovation 2, 100141. doi:10.1016/j.xinn.2021.100141
Yu, G., Wang, L.-G., Han, Y., and He, Q.-Y. (2012). clusterProfiler: An R Package for Comparing Biological Themes Among Gene Clusters. OMICS: A J. Integr. Biol. 16, 284–287. doi:10.1089/omi.2011.0118
Yu, G., Wang, L.-G., Yan, G.-R., and He, Q.-Y. (2015). DOSE: An R/Bioconductor Package for Disease Ontology Semantic and Enrichment Analysis. Bioinformatics 31, 608–609. doi:10.1093/bioinformatics/btu684
Zenobia, C., and Hajishengallis, G. (20152000). Basic Biology and Role of Interleukin-17 in Immunity and Inflammation. Periodontol. 2000 69 (1), 142–159. doi:10.1111/prd.12083
Keywords: Ehlers-Danlos syndrome, periodontitis, transcriptomics, monocytes, RNA-sequencing
Citation: Liao Z, Zhao T, Wang N, Chen J, Sun W and Wu J (2022) Transcriptome Analysis of Monocytes and Fibroblasts Provides Insights Into the Molecular Features of Periodontal Ehlers-Danlos Syndrome. Front. Genet. 13:834928. doi: 10.3389/fgene.2022.834928
Received: 14 December 2021; Accepted: 30 March 2022;
Published: 28 April 2022.
Edited by:
Loredana Bury, University of Perugia, ItalyReviewed by:
Ines Kapferer-Seebacher, Innsbruck Medical University, AustriaChengsong Zhu, University of Texas Southwestern Medical Center, United States
Copyright © 2022 Liao, Zhao, Wang, Chen, Sun and Wu. This is an open-access article distributed under the terms of the Creative Commons Attribution License (CC BY). The use, distribution or reproduction in other forums is permitted, provided the original author(s) and the copyright owner(s) are credited and that the original publication in this journal is cited, in accordance with accepted academic practice. No use, distribution or reproduction is permitted which does not comply with these terms.
*Correspondence: Weibin Sun, d2JzdW5Abmp1LmVkdS5jbg==; Juan Wu, anVhbnd1QHNtYWlsLm5qdS5lZHUuY24=
†These authors have contributed equally to this work