- 1Division of Infection and Global Health, School of Medicine, University of St Andrews, Scotland, United Kingdom
- 2Faculty of Infectious and Tropical Diseases, London School of Hygiene & Tropical Medicine, London, United Kingdom
- 3Faculty of Epidemiology and Population Health, London School of Hygiene & Tropical Medicine, London, United Kingdom
Plasmodium knowlesi, a malaria parasite of Old World macaque monkeys, is used extensively to model Plasmodium biology. Recently, P. knowlesi was found in the human population of Southeast Asia, particularly Malaysia. P. knowlesi causes uncomplicated to severe and fatal malaria in the human host with features in common with the more prevalent and virulent malaria caused by Plasmodium falciparum. As such, P. knowlesi presents a unique opportunity to develop experimental translational model systems for malaria pathophysiology informed by clinical data from same-species human infections. Experimental lines of P. knowlesi represent well-characterized genetically stable parasites, and to maximize their utility as a backdrop for understanding malaria pathophysiology, genetically diverse contemporary clinical isolates, essentially wild-type, require comparable characterization. The Oxford Nanopore PCR-free long-read sequencing platform was used to sequence and de novo assemble P. knowlesi genomes from frozen clinical samples. The sequencing platform and assembly pipelines were designed to facilitate capturing data and describing, for the first time, P. knowlesi schizont-infected cell agglutination (SICA) var and Knowlesi-Interspersed Repeats (kir) multiple gene families in parasites acquired from nature. The SICAvar gene family members code for antigenically variant proteins analogous to the virulence-associated P. falciparum erythrocyte membrane protein (PfEMP1) multiple var gene family. Evidence presented here suggests that the SICAvar family members have arisen through a process of gene duplication, selection pressure, and variation. Highly evolving genes including PfEMP1family members tend to be restricted to relatively unstable sub-telomeric regions that drive change with core genes protected in genetically stable intrachromosomal locations. The comparable SICAvar and kir gene family members are counter-intuitively located across chromosomes. Here, we demonstrate that, in contrast to conserved core genes, SICAvar and kir genes occupy otherwise gene-sparse chromosomal locations that accommodate rapid evolution and change. The novel methods presented here offer the malaria research community not only new tools to generate comprehensive genome sequence data from small clinical samples but also new insight into the complexity of clinically important real-world parasites.
Introduction
Plasmodium knowlesi is a malaria parasite first described in a natural host, the long-tailed macaque monkey (Macaca fascicularis), in the early part of the 20th century (Knowles and Gupta, 1932). Although an incidental find, P. knowlesi was soon exploited as a model parasite for malaria research as recently reviewed (Butcher and Mitchell, 2018; Galinski et al., 2018; Pasini et al., 2018). Experimental P. knowlesi was well characterized over time with several lines adapted from natural macaque hosts and one human infection originating in geographically distinct regions (Chin et al., 1965; Chin et al., 1968; Butcher and Mitchell, 2018; Galinski et al., 2018; Pasini et al., 2018). Taken together, experimental lines of P. knowlesi remain important members of the malaria research arsenal.
What sets P. knowlesi apart is that it occupies several important niche areas—as an experimental model, a natural parasite of Southeast Asian macaque monkeys, and the causative agent of zoonotic malaria in the human host (Singh et al., 2004). In nature, transmission is established in the jungles of Southeast Asia, areas that support the sylvan mosquito vectors, the parasite, and the natural macaque hosts. People who enter transmission zones are susceptible to infected mosquito bites and infection. P. knowlesi has effectively crossed the vertebrate host species divide and is responsible for malaria in contemporary human hosts (World-Health-Organization, 2021).
Zoonotic malaria caused by P. knowlesi is currently the most common type of malaria in Malaysia, with most of the cases reported in Malaysian Borneo (Chin et al., 2020). Indeed, naturally acquired P. knowlesi malaria causes a spectrum of disease from uncomplicated to severe and fatal infections with tantalizing similarity to severe adult malaria caused by P. falciparum (Cox-Singh et al., 2008; Daneshvar et al., 2009; Cox-Singh et al., 2010; Daneshvar et al., 2018).
The clinical similarities observed in patients with severe P. knowlesi and P. falciparum infections suggest that P. knowlesi has the potential to serve as a translational animal model system for severe malaria pathophysiology that has hitherto eluded medical science (Ozwara et al., 2003; Cox-Singh et al., 2010; Cox-Singh and Culleton, 2015; Onditi et al., 2015; Cox-Singh, 2018).
To take this idea forward, it seemed prudent to compare genome sequences derived from contemporary clinical isolates of P. knowlesi with P. knowlesi genomes generated from P. knowlesi-infected red blood cells propagated in rhesus monkeys (Pain et al., 2008; Lapp et al., 2018) or in vitro cultures (Benavente et al., 2018). Our data from clinical isolates were primarily compared to the first reference genome (Pain et al., 2008) and a cultured parasite (PkA1-H.1) (Benavente et al., 2018) control reference sequence and assembly generated using the same procedures.
Previously, we developed methods to produce high-quality Illumina short-read P. knowlesi genome sequence data from frozen clinical blood samples (Pinheiro et al., 2015). The outputs of that work identified genome-wide diversity, including a genomic dimorphism in P. knowlesi isolated from patients, but the Illumina platform was not suitable to resolve complex multiple gene family members.
Plasmodium species have a number of multiple gene families that code for infected host red blood cell surface proteins. The proteins are antigenic and highly variable to avoid host immune recognition and parasite destruction (Wahlgren et al., 2017; Harrison et al., 2020). Among these are the P. falciparum erythrocyte membrane protein (PfEMP1) gene family members with an estimated 67 copies in the P. falciparum 3D7 reference genome and variable copy numbers in clinical isolates (n = 47–90) (Gardner et al., 2002; Otto et al., 2018). PfEMP1 genes are expressed in a mutually exclusive manner with only one predominantly expressed at any one time (Hviid and Jensen, 2015; Abdi et al., 2017; Andrade et al., 2020). Importantly, PfEMP1 gene expression is implicated in P. falciparum virulence and progression to severe disease (Lavstsen et al., 2012; Abdi et al., 2017; Shabani et al., 2017; Wahlgren et al., 2017; Milner, 2018; Tessema et al., 2019; Jensen et al., 2020). While other multiple gene families are described in all Plasmodium species studied to date, PfEMP1 gene-like families are rare, and among the parasites that cause human disease, they are found only in P. falciparum and P. knowlesi (Gardner et al., 2002; Pain et al., 2008). The comparable P. knowlesi schizont-infected cell agglutination variant antigen (SICAvar) gene family has been reported in detail in experimental parasites that had been passaged in rhesus monkeys (al-Khedery et al., 1999; Pain et al., 2008; Lapp et al., 2015; Galinski et al., 2018; Lapp et al., 2018) but to our knowledge not in wild-type parasites, including P. knowlesi isolated from patients. Given the PfEMP1 gene association with severe disease in P. falciparum, we are particularly interested in describing P. knowlesi SICAvar multiple gene family member organization, location, and copy number in clinical isolates using amplification-free genome sequencing. With the methodology presented here and in the study by Oresegun et al. (2021), we can move forward in subsequent studies to achieve this goal.
Multiple gene family members are similar with long stretches of regions of low complexity that require long-read sequencing technologies to resolve (Pinheiro et al., 2015; Heather and Chain, 2016). Recently, the PacBio long-read sequencing platform was used to describe, for the first time, the core P. falciparum genome in clinical isolates and demark sub-telomeric regions to compare genome organization and diversity between clinical isolates from different geographical regions and the commonly used P. falciparum clone 3D7 (Otto et al., 2018).
The PacBio platform is outside of our reach because we have small-volume frozen whole blood samples that yield parasite DNA well below the quantity required for amplification-free PacBio sequencing (Benavente et al., 2018; Lapp et al., 2018; Otto et al., 2018). Here, we use the accessible, portable, and affordable Oxford Nanopore Technologies MinION long-read sequencing platform, suitable for small-quantity input DNA, to sequence and de novo assemble two new P. knowlesi reference genome sequences representing each genetically dimorphic form of P. knowlesi found in our patient cohort (Pinheiro et al., 2015; Ahmed et al., 2014).
The new reference genomes will, for the first time, provide insight into clinically relevant contemporary P. knowlesi parasites. These diverse parasites are essentially wild-type and the product of ongoing mosquito transmission and recombination in nature (Assefa et al., 2015; Pinheiro et al., 2015; Divis et al., 2018; Ahmed and Quan, 2019; Fong et al., 2019). The genomes will offer a valuable resource not only for our studies on members of the SICAvar gene family and virulence but also to the wider malaria research community working on comparative biology of malaria parasites, drug discovery, and vaccine development.
Materials and Methods
Sample Selection
P. knowlesi DNA extracted from archived clinical samples collected with informed consent as part of a non-interventional study were used (Ahmed et al., 2014). The isolates were selected to represent each of the two genetically distinct clusters, KH273 (sks047) and KH195 (sks048), of P. knowlesi–infected patients in the study cohort (Ahmed et al., 2014; Pinheiro et al., 2015). Control P. knowlesi DNA was extracted from the experimental line P. knowlesi A1-H.1 adapted to in vitro culture in human erythrocytes, the culture kindly donated by Robert Moon (Moon et al., 2013). In order to distinguish the genome data generated here for P. knowlesi A1-H.1 from those already existing, we use the unique abbreviation StAPkA1H1 (Diez Benavente et al., 2017; Benavente et al., 2018).
Plasmodium DNA Extraction
Human DNA was depleted from 200 to 400 µl thawed clinical samples using a previously described method (Oresegun et al., 2021). Briefly, surviving human leucocytes in thawed samples were removed using anti-human CD45 DynaBeads (ThermoFisher Scientific). The resulting parasite pellet was washed to remove soluble human DNA (hDNA), and parasite-enriched DNA (pDNA) was extracted using the QIAamp Blood Mini Kit (QIAGEN) with final elution into 150 µl AE buffer. DNA concentrations were quantified using the Qubit 2.0 fluorometer (Qubit™, Invitrogen) and real-time qPCR on RotorGene (QIAGEN). Recovered DNA was concentrated, and short fragments were removed by mixing at a ratio of 1:1 by volume with AMPureXP magnetic beads (Beckman Coulter) following the manufacturer’s instructions. Briefly, the AMPureXP bead mixture was placed in a magnetic field, and DNA bound to the beads was rinsed twice with 70% ethanol before air-drying to allow residual ethanol to evaporate. Parasite-enriched DNA was eluted in 10 µl nuclease-free H2O (Ambion). One microliter of recovered DNA concentrate was used for DNA quantification using a Qubit fluorimeter (ThermoFisher Scientific), and 7.5 µl was taken forward for sequencing library preparation.
Library Preparation and Sequencing
Parasite-enriched DNA was sequenced using the Oxford Nanopore Technologies (ONT) MinION long-read sequencing platform. Library preparations were selected to suit PCR-free sequencing for the small pDNA quantities available to study (∼400 ng). Sequencing libraries were prepared following the manufacturer’s instructions for the SQK-RBK004 ONT sequencing kit. Sequencing was performed using R9.4.1 flowcells or R10 flowcells (Oresegun et al., 2021). Previously sequenced Illumina reads for the patient isolates (sks047 and sks048) were retrieved from the European Nucleotide Archive, with accession codes ERR366425 and ERR274221, respectively (Pinheiro et al., 2015). Further short-read sequencing was carried out on PCR-enriched DNA using the Illumina MiSeq platform at the London School of Hygiene and Tropical Medicine and methods established by Diez Benavente et al. (2019).
Reference Genomes
For chromosome scaffolding and quality assessment comparison, the P. knowlesi PKNH reference genome (Pain et al., 2008) (version 2) was downloaded from Sanger (ftp://ftp.sanger.ac.uk/pub/genedb/releases/latest/Pknowlesi/#). In addition, further comparisons were carried out using the P. knowlesi PkA1H1 reference genome (Benavente et al., 2018) from NCBI [accession code: GCA_900162085].
De Novo Genome Assembly
MinION FAST5 file outputs were locally base called using the high accuracy model of the guppy basecaller (v4.0.15; Ubuntu 19.10; GTX1060) with the following parameters: “-r -v -q 0 --qscore-filtering -x auto.” Demultiplexing was carried out using qcat software (v1.1.0) with the “--detect-middle --trim -k --guppy” parameters, and then adapter removal was carried out using porechop (v0.2.4) with default parameters and the most recent versions released from ONT technologies. Human DNA (hDNA) contamination was removed from the adapter-free reads by alignment against the human GRCh38.p13 reference genome (retrieved from NCBI accession code: GCF_000001405.39) (Lander et al., 2001) using minimap2 (v2.17) (Li, 2018) with “-ax map-ont” default parameters. Unmapped reads were separated from the binary sequence alignment (BAM) file using samtools (v1.10) (Li et al., 2009; Li, 2011) and converted back to FASTQ using bedtools (v2.29.2) (Quinlan and Hall, 2010) for de novo genome assembly using Flye (v2.8.1) (Kolmogorov et al., 2019) with an expected genome size of 25 Mb and “--nano-raw” default parameters. Successful assemblies were assessed for contamination using BlobTools (v1.0.1) (Laetsch and Blaxter, 2017). Contigs not taxonomically assigned as Apicomplexan were discarded.
Assembly Polishing and Correction
Draft assemblies were polished using four iterations of racon (v1.4.13) (Vaser et al., 2017); in the default setting, raw long-read isolate sequence reads which did not align to the human GRCh38.p13 (henceforth parasite-reads) were retained. As part of the polishing step, alignments of parasite-reads against the draft assembly were performed using minimap2 (v2.17) (Li, 2018). A consensus sequence was subsequently generated from the racon output using medaka (v1.0.3; default settings) (Oxford Nanopore Technologies, 2019). Further polishing and correction were carried out using Illumina paired-end reads where available, using three iterations of pilon (v1.23) with default parameters “-Xmx120G, --tracks, --fix all, circles” (Walker et al., 2014).
Masking Repetitive Elements
The P. knowlesi PKNH reference mitochondrial (MIT) and apicoplast (API) sequences were extracted and individually aligned against draft P. knowlesi assemblies using MegaBLAST (v.2.9; default parameters) (Morgulis et al., 2008; Pain et al., 2008). Contigs which aligned to the reference PKNH MIT and API genomes were subsequently removed and circularized on Circlator (v1.5.5) (Hunt et al., 2015) with the command “circlator all --data_type nanopore-raw --bwa_opts ‘-x ont2d’ --merge_min_id 85 --merge_breaklen 1000.” API/MIT-free draft nuclear assemblies (henceforth, draft assemblies) were taken forward through RepeatModeler (v1.0.10) (Flynn et al., 2020), and the outputs were utilized as input for Censor (Kohany et al., 2006) where the options “Eukaryota” and “Report simple repeats” were selected. Identified transposable elements and repeats in the censor outputs were classified based on the class of repeats to make a repeat library for each assembly. Repeat libraries of each draft assembly were combined and misplaced, redundant sequences were removed using CD-HIT (v4.8.1; “-c 1.0 -n 10 -d 0 -g 1 -M 60000” parameters) (Li and Godzik, 2006; Fu et al., 2012). This generated a singular “master” repeat library encompassing the non-redundant list of identified elements across the three draft assemblies.
With the master repeat library, RepeatMasker (v4.0.7) was run on each draft assembly producing a tab-separated value (TSV) output of the identified repeats in the assembly. Then, using ‘One Code to Find Them All’ (OCFTA) (Bailly-Bechet et al., 2014), each TSV file was parsed to clarify further repeat positions found using RepeatMasker. Next, the LTRHarvest (Ellinghaus et al., 2008) module of GenomeTools (v1.6.1) (Gremme et al., 2013) was used to find secondary structures of long terminal repeats (LTRs) and other alternatives in the DRAFT assemblies. Here, the “suffixerator” function was implemented with “-tis -suf -lcp -des -ssp -sds -dna” parameters while the “ltrharvest” function was run with “-mintsd 5 -maxtsd 100”’ parameters. Concurrently, TransposonPSI was also used on the DRAFT assemblies with default parameters to find repeat elements based on their coding sequences.
Redundant repeat element sequences were removed from the outputs of RepeatMasker, OCTFA, LTRHarvest, and TransposonPSI using a custom script, to generate a genome feature file (GFF3) where each transposable and repetitive element of each DRAFT assembly is represented once. Then, within each draft assembly, repeat elements were masked using the coordinates present in the non-redundant GFF3 file and the “maskfasta” function of bedtools (v2.27; default settings and “-soft”).
Prediction and Annotation
The masked draft assemblies were checked for chimeric contigs using Ragtag (v1.0.1) (Alonge et al., 2019) where both the “correct” and “scaffold” functions were run with the “--debug --aligner nucmer --nucmer-params = ‘-maxmatch -l 100 -c 500’” parameters (Li et al., 2009; Li, 2011).
With the chimeric contigs broken, masked draft assemblies were uploaded on the Companion webserver (Steinbiss et al., 2016) for gene prediction and annotation using the sequence prefix of “PKA1H1_STAND” for the cultured experimental line (StAPKA1H1) and “PKCLINC” for patient isolates (sks047 and sks048). Companion software was run with no transcript evidence, 500 bp minimum match length, and 80% match similarity for contig placement, 0.8 AUGUSTUS (Stanke et al., 2006) score threshold, and taxid 5851. Additionally, pseudochromosomes were contiguated, reference proteins were aligned to the target sequence, pseudogene detection was carried out, and RATT was used for reference gene models.
Comparative Genomics, Quality Assessment and Analyses
As the pipeline progressed, assembly metrics were checked using assembly-stats (v1.0.1) and pomoxis (v0.3.4). Additionally, draft genomes were further assessed for completeness and accuracy using Benchmarking Universal Single-Copy Orthologues (BUSCO) v5.0 with “-l plasmodium_odb10 -f -m geno --long” parameters (Simão et al., 2015). GFF3 files generated on Companion were parsed for genes of interest, including multigene families known to span the core genome and telomeric regions. Chromosomes of the annotated draft genomes were individually aligned against the corresponding P. knowlesi PKNH reference chromosome (Pain et al., 2008) with minimap2 parameters “-ax asm5. ” The resulting alignment files were analyzed on Qualimap (v.2.2.2) (Okonechnikov et al., 2016) with parameters “−nw 800−hm 7. ” Gene density, chromosome structure, and multigene family plots were generated using the karyoploteR visualization package (Gel and Serra, 2017). Dotplots to identify repetitions, breaks, and inversions were generated from minimap2 whole genome alignments using D-GENIES default settings (Cabanettes and Klopp, 2018).
Structural Variant Analyses
The StAPkA1H1 draft genome, assembled here, was used as the reference for structural variant calling and subsequent variant annotation to ensure parity across sequencing technologies. Read alignment-based structural variant calling (henceforth reads-based) was achieved using the Oxford Nanopore structural variation pipeline (ONTSVP) (https://github.com/nanoporetech/pipeline-structural-variation), while the assembly-based approach was completed with Assemblytics (Nattestad and Schatz, 2016). Using a modified Snakefile, FASTQ isolates parasite-reads and the StAPkA1H1 draft genome; the ONTSVP first parses the input reads using catfishq (https://github.com/philres/catfishq) and seqtk (https://github.com/lh3/seqtk) before carrying out alignment using lra with parameters “-ONT -p s” (Ren and Chaisson, 2020). The resulting alignment file was sorted and indexed using samtools, and read coverage was then calculated using mosdepth (“−x−n−b 1000000”) (Pedersen and Quinlan, 2018). Structural variants (SVs) were called using cuteSV (Jiang et al., 2020) with parameters “--min-size 30 --max-size 100,000 --retain_work_dir --report_readid --min_support 2.” Variants were subsequently filtered for length (30 bp), depth (8 reads), quality (Q30), and structural variant type (SVTYPE) such as insertions (INS) by default, before filtered variants were sorted and indexed. Failed SV types were manually filtered based on length (30 bp) and quality (Q30) alone to determine the presence of high-quality, low-occurrence variants.
For the assembly-based structural variant calling for the clinical isolates sks047 and sks048 and StAPkA1H1, draft genomes were aligned against the PKNH reference genome (Pain et al., 2008) using nucmer with “--maxmatch -l 100 -c 500” parameters and outputs uploaded onto Assemblytics (http://assemblytics.com) (Nattestad and Schatz, 2016) with default parameters and a minimum SV length of 30 bp. BEDfile outputs of Assemblytics were converted to variant call format (VCF) files using SURVIVOR (v1.0.7) (Jeffares et al., 2017). VCF files for successful reads-based and assembly-based SV calling as well as the failed SV-type VCF files were further filtered to remove any variants less than 50 bp in length and less than Q5 in quality using a bcftools one-liner (https://github.com/samtools/BCFtools). A quality filter was not applicable for the assembly-based approach due to the lack of quality information in the original BEDfile output of Assemblytics. Variants exceeding these thresholds were annotated using vcfanno (v0.3.2) (Nattestad and Schatz, 2016) and subsequently sorted and indexed. Annotated variants, relevant BAM alignment files, and GFF files were visualized on IGV (Thorvaldsdóttir et al., 2013). Using IGV, a gene locus previously identified to be associated with dimorphism—PknbpXa (Pinheiro et al., 2015)—was analyzed to determine the presence of structural variants. Summary statistics were calculated using the ‘stats’ function of SURVIVOR with parameters “−1−1−1.” VCF files were compared using the “isec” function of bcftools with default settings, including analyses of the variants present within genes.
Duplication, Clustering, Genomic Organization and dN/dS Analyses
Scripts used can be found here: https://github.com/peterthorpe5/plasmidium_genomes. Gene duplication analyses were performed using the similarity searches from DIAMOND-BlastP (1e-5) with the MCSanX toolkit (Wang et al., 2012). Orthologues clustering and dN/dS were performed as described in the study by Thorpe et al. (2018). Briefly, OrthoFinder (v2.2.7) (Emms and Kelly, 2019) was used to cluster all the amino acids sequences for the genomes used in this study. The resulting sequences from the clusters of interest were aligned using MUSCLE (v3.8.1551) (Edgar, 2004) and refined using MUSCLE. The resulting amino acid alignment was used as a template to back-translate the nucleotide coding sequence using Biopython for subsequent nucleotide alignment (Cock et al., 2009). The nucleotide alignment was filtered to remove any insertions and deletions and return an alignment with no gaps using trimAL (v1.4.1) (Capella-Gutierrez et al., 2009). The resulting alignment was subjected to dN/dS analysis using Codonphyml (v1.00 201407.24) (-m GY --fmodel F3X4 -t e -f empirical -w g -a e) (Gil et al., 2013). Genomic organization of classes of genes of interest was performed as described in the studies by Eves-van den Akker et al. (2016) and Thorpe et al. (2018, 2020). For UpSet visualization the scripts can be found in the github link above.
Results
Evaluating Draft de novo Genomes
The genome pipeline, beginning with Oxford Nanopore Technologies (ONT) MinION sequencing through to de novo assembly and genome annotation with downstream analyses, is shown (Figure 1). The pipeline was used to produce P. knowlesi genomes using DNA extracted from two clinical isolates, sks047 and sks048, and, for comparison, DNA extracted from the well-characterized cultured line, P. knowlesi A1-H.1. For the purpose of clarity, the P. knowlesi A1-H.1 de novo draft genome assembled here is referred to as StAPkA1H1 (please see the Methods section). Read coverages of 225x, 71x, and 65x were obtained for StAPkA1H1, sks047, and sks048, respectively (Table 1). The draft assemblies resolved into 100 or fewer contigs before further reduction to <72 contigs after scaffolding (Table 1). The quality of the draft assemblies was improved with Medaka’s polishing resulting in BUSCO scores that increased from 68.6 to 89.7 (a 30.8% increase), 67.2 to 85.5 (a 27.2% increase), and 68.8 to 85.9 (a 24.8% increase) for StAPkA1H1, sks047, and sks048, respectively, with BUSCO completeness scores for the clinical isolates reaching 95% (Table 1). The observed increase in the number of contigs from 23.57 to 23.63 Mb (0.22% increase) for sks047 and 24.49 to 24.56 Mb (0.32% increase) for sks048 was likely due to the addition of relatively shorter reads (Table 1).
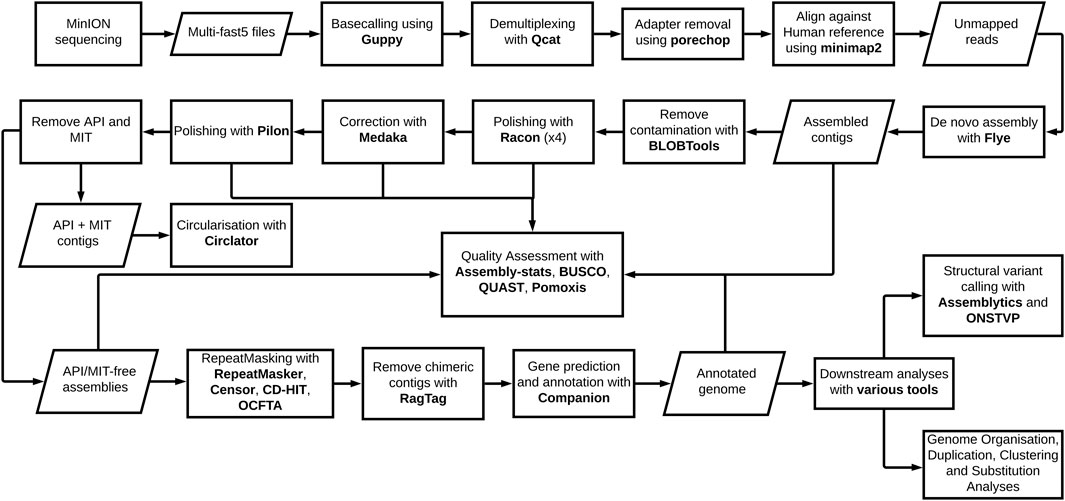
FIGURE 1. Plasmodium knowlesi de novo genome pipeline. The pipeline represents major forms of manipulation taken and tools utilized to generate, annotate, and analyze the two reference genomes derived from clinical isolates and the experimental line.
The combination of previously sequenced Illumina reads data with 34x and 166x short read coverage for sks047 and sks048, respectively, offered the opportunity for Pilon polishing the newly generated ONT sequence data for the clinical isolates. Pilon polishing resulted in improved BUSCO scores with sks047 seeing an 11.9% improvement (85.5–95.7) and sks048 showing an 11.4% improvement (85.9–95.7) (Table 1). Although Pilon did not change the number of contigs, both sks047 and sks048 saw a total length increase of 0.05% and increases in BUSCO scores. Additional Illumina sequencing was not available for StAPkA1H1, and Pilon polishing was not possible.
Scaffolding, chromosome structuring, and subsequent annotation initially proved difficult due to large sections of chromosomes 2 and 3 consistently being incorrectly placed in chromosomes 14 and 13, respectively. These large-scale inconsistencies were the result of contig chimers and were minimized or entirely corrected by de-chimerization using RagTag. Chromosomes corrected by RagTag retained regions of variability for the draft assemblies, although RagTag did not provide a complete solution in resolving all variable sequences (Supplementary Figures S1, S2). In addition, it is possible that RagTag did not entirely retain highly variable regions such as telomeric regions that may have resulted in loss of coverage of genes positioned at extreme chromosomal boundaries (Supplementary Figure S1).
Genome Annotation and Gene Content
Companion software resolved all three nuclear genomes, StAPkA1H1, sks047, and sks048, into 15 chromosomes–14 Pk chromosomes and 1 “bin” or “00” chromosome (chr 00) holding sequence fragments which could not be confidently placed by the Companion pipeline (Table 2). Each draft genome was assigned a similar or greater number of coding genes than the P. knowlesi PKNH reference genome (5327 genes) when full protein-coding genes and pseudogenes annotated with predicted function (implying missing “start” and/or “stop” codons) were combined. The StAPkA1H1 draft assembly had 5358 genes (4385 coding +973 pseudogenes), while the patient isolate draft genomes sks047 and sks048 had 5327 genes (4886 coding +441 pseudogenes) and 5398 genes (4904 coding +494 pseudogenes), respectively (Table 2). Non-coding genes were also found in all three draft genomes, including multiple small nuclear RNA (snRNA) (Supplementary File S1). P. knowlesi schizont-infected cell agglutination variant antigen (SICAvar) and the Knowlesi-Interspersed Repeats (kir) multiple gene families were annotated in each draft genome (Table 2). There were consistently fewer kir gene family members in the draft genomes derived from the clinical isolates sks047 and sks048 with 26 and 25 kir genes, respectively, compared with 51 kir genes in the experimental cultured line StAPkA1H1 and 56 in the published PKNH reference genome (Table 2). It is unlikely that this is a result of assembly error given that StAPkA1H1 and the clinical isolates sks047 and sks048 were sequenced and de novo assembled in parallel using the same methodologies with the exception of Pilon polishing for StAPkA1H1.
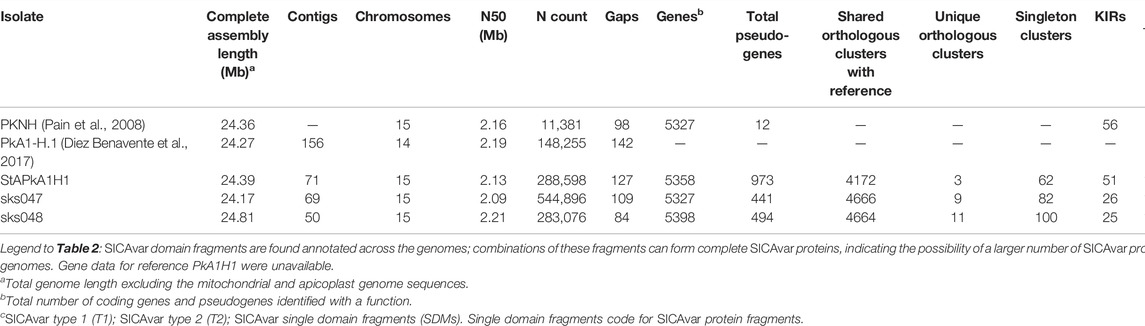
TABLE 2. Summary of the complete de novo draft genomes compared to the published P. knowlesi PKNH and PkA1H1 reference genomes.
All three draft genomes had more SICAvar type 1 genes annotated than the reference PKNH genome. StAPkA1H1 had 191 SICAvar type 1 genes, sks047 had 115 SICAvar type 1 genes, and sks048 had 153 SICAvar type 1 genes. The reference PKNH genome is reported with 89 SICAvar type 1 genes (Table 2). SICAvar gene fragments in each of the clinical isolate draft genomes, sks047 and sks048, outnumbered annotated SICAvar type 1 genes (Table 2). Conversely, the StAPkA1H1 draft genome had approximately half the number of SICAvar gene fragments compared with the clinical isolates and compared with StAPkA1H1 SICAvar type 1 genes (Table 2).
In regions of the draft genomes where gaps could not be resolved, contigs with evidence that they belonged together, either by long reads spanning them or by similarity to the reference, were scaffolded with N bases proportional to the gap size (Table 2). Higher N counts were observed in the three draft genomes generated here compared with the published reference genome (PKNH). In addition, sequences placed in the draft genome chr 00 may reflect the higher N counts in chromosomes 1–14. The chr 00 of StAPkA1H1 clustered with the PKNH reference chr 00 (Supplementary Figures S1,i) suggesting the StAPkA1H1 draft genome had a similar structure to the PKNH reference genome, including “unplaced” genes. In contrast, sks047 and sks048 chr 00 sequences were distributed across the reference genome, suggesting no single chromosome was more challenging to scaffold after de-chimerization (Supplementary Figure S2ii,iii). The number of gaps in the three draft genomes was variable but within the range of the PKNH reference genome (Table 2).
Orthologous genes were determined using a similarity approach by OrthoMCL in Companion and showed that all three draft genomes shared >4000 orthologs with the PKNH reference genome (Table 2). These orthologous genes can be considered as the core P. knowlesi gene set and are indicative of reliable and accurate assemblies (Table 2). In particular, draft genomes from the contemporary patient isolates sks047 and sks048 had >4600 shared orthologues with the PKNH reference genome (Table 2).
Chromosome Structure
Dotplots of alignment of the three draft genomes show that they are syntenic with the PKNH reference regardless of gaps present in the genomes generated from patient isolates (Supplementary Figure S3). The unplaced sequences in chr00 account for at least 40% of gaps in the three draft genomes (Table 2). Indeed, each draft genome’s chromosome structure conforms to that of the PKNH reference genome with uniform coverage across the chromosomes in regions with no gaps (Supplementary Figure S4). This is also apparent in fragmented chromosomes, which retained the same chromosomal structure as PKNH (Supplementary Figure S5). While coverage remained largely uniform, structural variations (>10 kb), for example, duplications and inversions, were present in the draft assemblies as seen in duplications present in multiple chromosomes in sks047 and sks048 (Supplementary Figure S4b).
Additionally, inversions were present in almost every chromosome, often as inverted duplicate sequences, with the most striking instance observed in chromosome 5 of sks048 (Supplementary Figure S4a,iii), where multiple duplicated inversions were observed. Frameshifts were present across chromosomes in all of the draft genomes (Supplementary Figure S4b). Given the robust clinical isolate draft genome assembly, the frameshifts observed deserve further investigation. Associated gaps do not appear to have impacted the distribution of genes within the draft genomes (Figure 2). The mean annotated gene density shows the PKNH reference genome to have 22.05 genes per 100 kbp, StAPkA1H1 to have 18.15, sks047 to have 20.25, and sks048 to have 19.80 (Figure 2). Increased gene density may be achieved with manual pseudogene curation since mean gene density is inversely correlated with the number of pseudogenes, p = 0.003186 (Table 2).
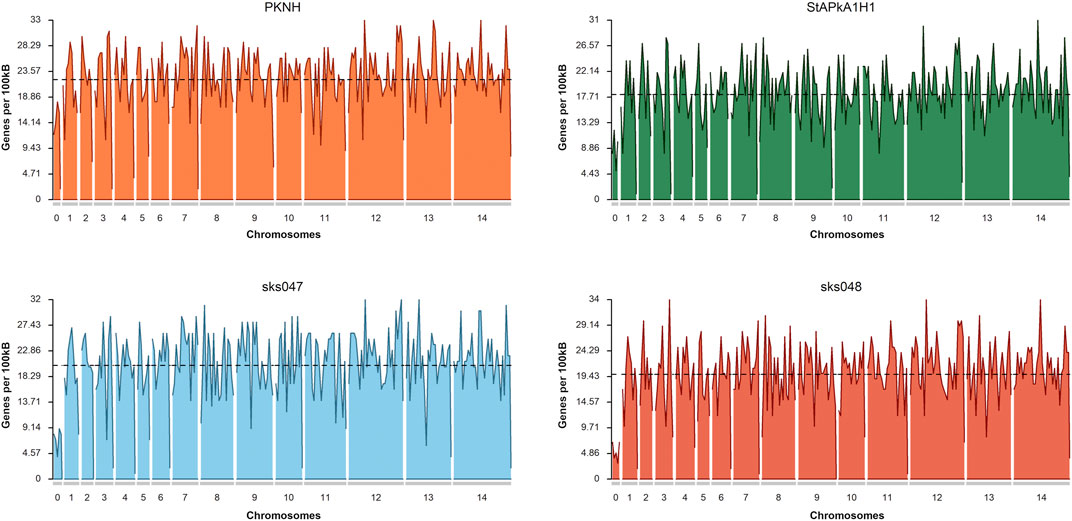
FIGURE 2. Gene density plots for the P. knowlesi PKNH reference genome, StPkA1H1, sks047, and sks048 draft genomes. Gene density is calculated based on the number of identified genes within a sliding window of 100 kb. Mean density shows the PKNH reference genome to have 22.05 genes per 100 kb, StAPkA1H1 to have 18.15, sks047 to have 20.25, and sks048 to have 19.8. Plots were generated using karyoploteR (Gel and Serra, 2017).
With the exception of SICAvar and the Interspersed Repeat (IR) genes, analysis of the other multigene families reveals similar retention copy numbers in the three draft genomes and the PKNH reference (Table 3). Given the high similarity between the experimental lines StAPkA1H1 and PKNH in dotplots and other analyses, the total number of IR genes in the two different laboratory passaged lines, PKNH with 70 and StAPkA1H1 with 67, compared with clinical isolates, sks047 with 53 and sks047 with 52, may reflect gene retention through passive artificial passage. The clinical samples had fewer annotated kir genes than the experimental lines and in contrast have interspersed genes annotated as P. vivax vir that are absent in experimental lines (Table 3). Clinical isolates are effectively wild-type P. knowlesi, and the lower kir gene copy number and the presence of vir-like genes possibly reflect continual recombination and selection pressure during mosquito transmission in nature.
Chromosome positional analyses of the kir genes show varied distribution across chromosomes with only three kir genes represented in chr 00 in the clinical isolate draft genomes, perhaps supporting constrained kir gene copy number in nature (Supplementary Figure S6). SICAvar genes appear to be distributed across the genome, on all chromosomes, including the chromosomal extremities with more members annotated than previously reported by Pain et al. (2008), particularly on chromosomes 10, 11, and 12 (Supplementary Figure S7).
Structural Variation
Following filtering for length, quality, and depth, reads-based structural variants (SVs) were called using the ONT SV pipeline and assembly-based SVs were called using Assemblytics (Nattestad and Schatz, 2016). The reads-based approach returned 1,316 and 1,398 SVs for sks047 and sks048, respectively (Table 4). The assembly-based approach returned 856 and 839 SVs for sks047 and sks048, respectively (Table 4). The reads-based approach is expected to return more variants due to a higher error rate in the raw reads used than in the collapsed assembly-based methodology.
SVs that exceeded the quality, length, and read depth threshold are distributed across the genome on all chromosomes within coding and non-coding regions. Within the 101 shared SVs, 68 were within annotated genes, including within the SICAvar and kir multigene family members (Supplementary Table S1). There were different variation signatures between the experimental cultured line StAPkA1H1 compared with the two clinical isolates, sks047 and sks048 (Figure 3). StAPkA1H1 had more tandem variants than the clinical isolates, sks047 and sks048. In comparison, the clinical isolates show more variation in their repeat sequences with similar insertion and deletion (red and blue) and repeat expansion and contraction (green and purple) signatures than StAPkA1H1 (Figure 3).
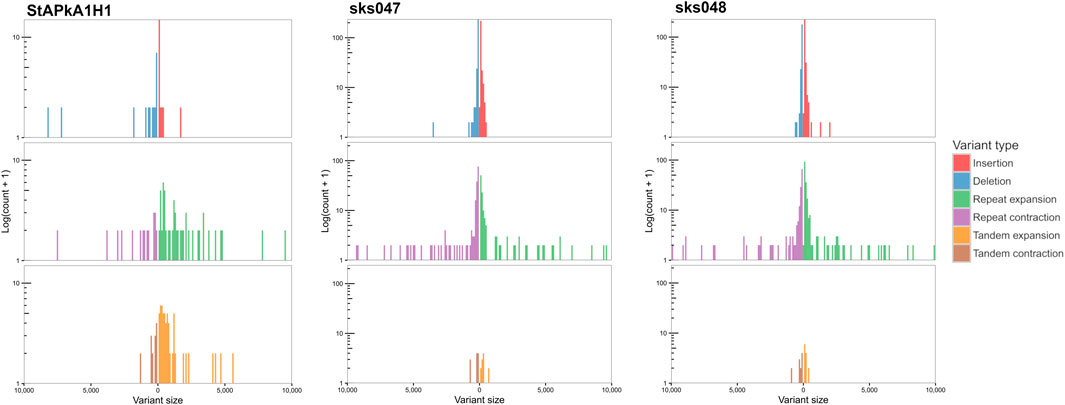
FIGURE 3. Assembly-based structural variation, size 50–10,000 bp, of StAPkA1H1, sks047, and sks048 draft genomes against the PKNH reference genome (Pain et al., 2008). Nucmer alignment was generated using parameters “—maxmatch−l 100−c 500” with default and Assemblytics parameters (Nattestad and Schatz, 2016). Expansions (green and orange) refer to insertions that occur within repeat or tandem variants, while contractions (purple and brown) refer to deletions in these regions. More variation is present in the tandem variants (brown and orange) of StAPkA1H1 than those of the draft clinical isolate genomes, sks047 and sks048. In comparison the clinical isolates show more variation in their repeat sequences with similar insertion and deletions (red and blue) and repeat expansion and contraction (green and purple) signatures.
Gene Duplication
Gene duplication was quantified and classified using MCScanX (Wang et al., 2012). All genes within the draft genomes for the StAPkA1H1 cultured line and sks047 and sks048 clinical isolates were classified as either singleton (no identified duplication, proximal (two identified duplicated genes with <20 genes between them), dispersed (>20 genes between the 2 candidate genes), tandem (duplication events next to each other), and segmental/whole genome duplication (WGD) (>4 co-linear genes with <25 genes between them). To gain an insight into differences in gene duplication, duplication types were classified for the BUSCO eucaryotic core control gene population and for the PkSICAvar type 1, PkSICAvar type 2, and the kir multiple gene families in the three draft genomes, StAPkA1H1, sks047, and sks048 (Figure 4). The duplication profile of the control population BUSCO genes was well matched between each of the draft genomes and also to the BUSCO duplication profile for the PKNH reference genome (Mann–Whitney U test StAPkA1H1, p = 0.92; sks047, p = 0.67; sks048, p = 0.66; PKNH p = 0.40). Therefore, there were no observed excess duplication types for BUSCO genes (Figure 4). However, duplication profiles for the genes annotated SICAvar type 1, SICAvar type 2, and kir in the draft genomes, StAPkA1H1, sks047, and sks048, were markedly different from the BUSCO gene profiles with no evidence for singleton genes (Figure 4). When compared to 100 randomly obtained genes as a population, this result profile was statistically significant (Mann–Whitney U test, p < 1.0e−9).
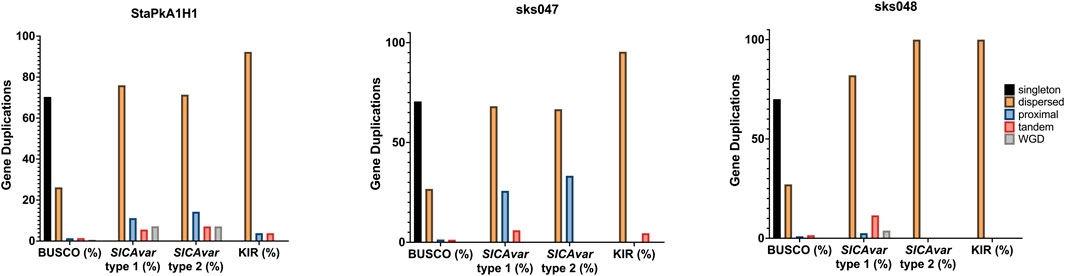
FIGURE 4. Gene duplication classes for the draft genome assemblies for StAPkA1H1 (experimental cultured line) and the clinical isolates sks047 and sks048. Gene duplication was quantified and classified using MCScanX (Wang et al., 2012) for all genes in each genome and identified as black bars, singleton (no identified duplication); orange bars, dispersed (>20 genes between the 2 candidate genes); blue bars, proximal (two identified duplicated genes with <20 genes between them); pink bars, tandem (duplication events next to each other); and green bars, segmental/whole genome duplication (WGD) (>4 co-linear genes with <25 genes between them). The gene pools for each genome were divided into BUSCO (core genome genes) for comparison with the genes making up the SICAvar type 1 or SICAvar type 2 or kir multiple gene families. The draft genomes, StAPkA1H1, sks047, and sks048, had roughly similar profiles for BUSCO genes. Singletons (dark blue bars) were absent from the multiple gene families for all of the draft genomes.
Positive Selection: Nonsynonymous (dN)/Synonymous (dS) Substitutions
In order to determine if the SICAvar type 1, SICAvar type 2, and kir genes are under selection pressure, the associated predicted proteins from each genome, StAPkA1H1, PKNH, sks047, and sks048, were translated into amino acid sequences and grouped into putative orthologous gene clusters containing SICAvar type 1 or SICAvar type 2 or kir or BUSCO (control group) using OrthoFinder. The amino acid sequences were aligned, and the alignments used to “backtranslate” into nucleotide coding sequences. The mean dN/dS values for SICAvar type 1, SICAvar type 2, kir, and BUSCO gene clusters were 2.40, 2.74, 2.35, and 0.35, respectively, and the differences were statistically significant (Wilcoxon rank sum test p-value adjustment method Bonferroni: SICAvar type 1, = 4.1e-08; SICAvar type 2 = 0.0063 and kir, p = 6.7e-13, Table 5 and Supplementary Figure S8).
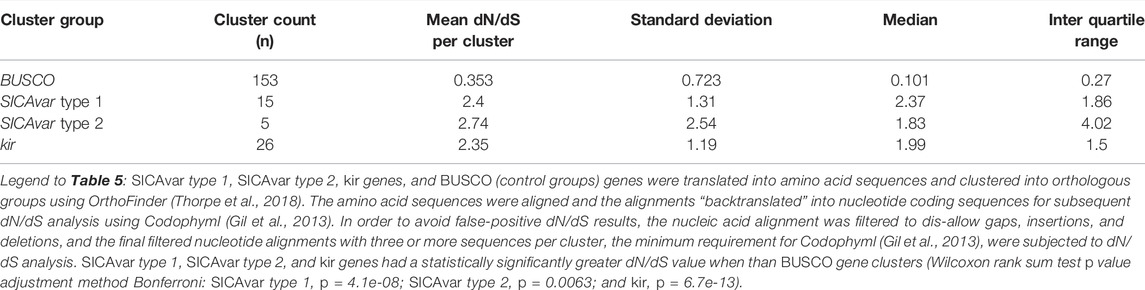
TABLE 5. Non-synonymous versus synonymous (dN/dS) analysis of SICAvar type 1, SICAvar type 2, kir, and BUSCO gene clusters represented collectively in the StAPkA1H1, sks047, and sks048 draft genomes and the PKNH reference genome.
Genomic Organization of SICAvar Type 1, SICAvar Type 2 and kir Gene Family Members
P. knowlesi SICAvar type 1, SICAvar type 2, and the kir gene family members appear to be variable, rapidly evolving genes, yet they are distributed across chromosomes, potentially destabilizing the core genome. To investigate this further, the distance from one gene to its neighbor was quantified in both a 3 prime (3’) and 5 prime (5’) direction, excluding genes at the start or end of a scaffold. The values were subjected to further analysis using the BUSCO core genes for comparison (Figure 5A). With the exception of SICAvar type 2 in the 3’ direction, all of the SICAvar type 1, SICAvar type 2, and kir genes had a statistically significantly greater distance to their neighboring genes in both the 3’ and 5’ directions than BUSCO genes. In the 3’ direction, Kruskal–Wallis chi-squared = 272.15, df = 4, p-value < 2.2e-16. The Wilcoxon signed-rank test with Bonferroni p-value adjustment was SICAvar type 1 p = 2e-16, SICAvar type 2 p = 0.457, and kir p = 1.1e-10. In the 5’ direction, all distances for SICAvar and kir genes were significantly different to the BUSCO control population. Kruskal–Wallis chi-squared = 269.33, df = 4, p-value < 2.2e-16 with Wilcoxon signed-rank test, Bonferroni p-value adjustment in comparison to BUSCO: SICAvar type 1 p = 2e-16, SICAvar type 2 p = 0.00123, and kir p = 3.6e-09. The distribution of potentially destabilizing highly evolving SICAvar and kir genes across chromosomes in gene-sparse regions of the P. knowlesi genome would offer protection to core genes.
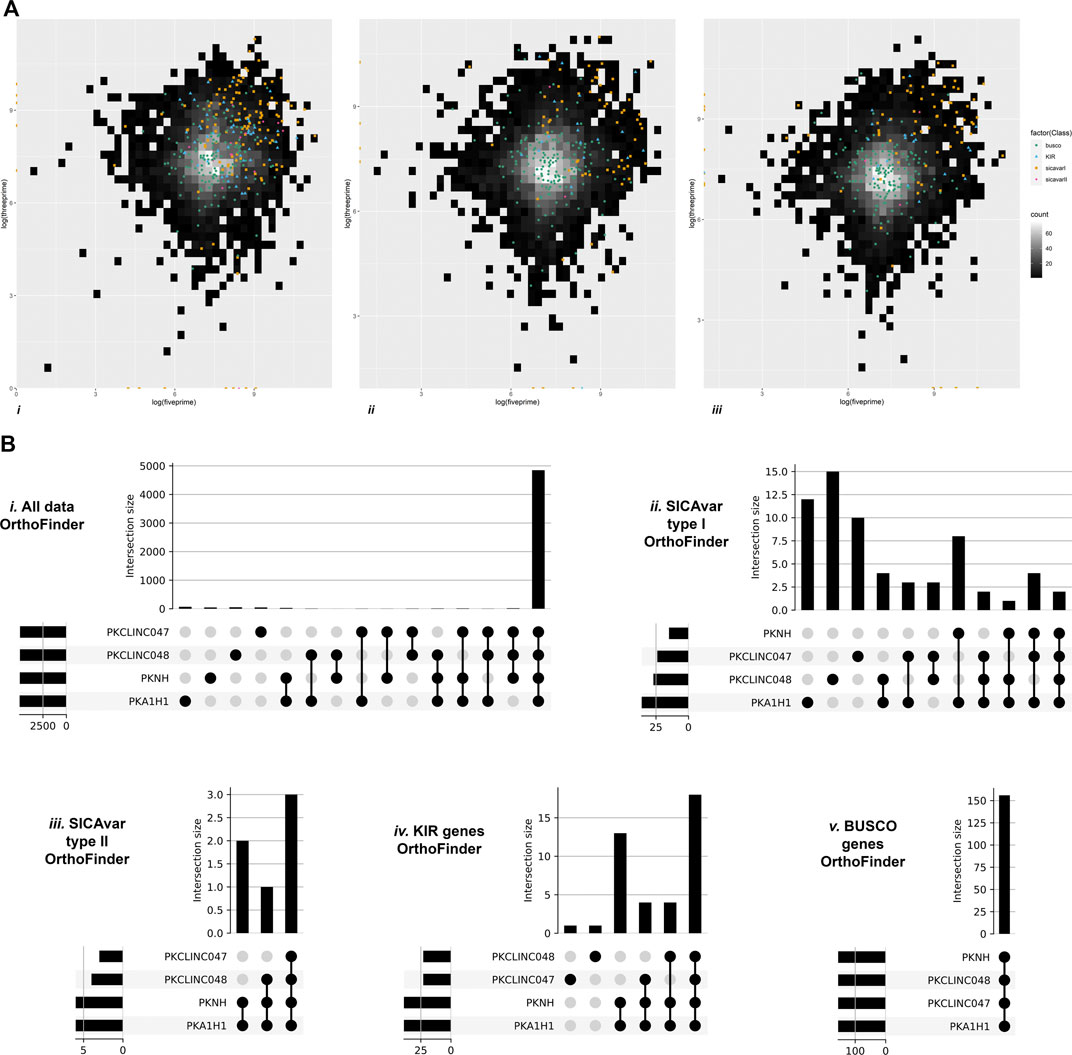
FIGURE 5. Genomic organization of multiple gene family members. Figure 5A Heatmap gene density plots showing 5’ against 3’ intergenic distances (log10) for the draft genomes. (i) StAPkA1H1 experimental cultured line, (ii) clinical isolate sk047, and (iii) clinical isolate sk048. Gene density for intergenic distances is represented by color scale ranging from black (low) to white (high, maximum of 60 genes per bin). Genes classed as BUSCO (green dots), SICAvar type 1 (orange squares), SICAvar type 2 (pink diamond), and kir (blue triangles) are shown. SICAvar type 1 and kir genes had a significantly greater distance to their neighboring genes than the BUSCO genes (Wilcoxon signed-rank test, Bonferroni p-value adjustment, p = < 1e-09), suggesting that these gene family members are in gene-sparse regions. Genes situated at the start or end of scaffolds were excluded from the analysis. Figure 5B OrthoFinder gene cluster outputs were visualized using “UpSets” to determine the membership of genes between clusters in the PKNH reference genome (Pain et al., 2008) and the draft genomes; StAPkA1H1 experimental cultured line, clinical isolate sk047, and clinical isolate sk048. All gene clusters (i), SICAvar type 1 gene clusters (ii), SICAvar type 2 gene clusters (iii), kir gene clusters (iv), and BUSCO gene clusters (v) are shown. The majority of all gene clusters were present in all isolates with the exception of SICAvar type 1 gene clusters with 10–15 SICAvar type 1 clusters being unique per isolate. For kir genes, the majority of clusters were shared between all isolates with the exception of a single unique kir gene cluster in each of sk047 and sk048. The majority of SICAvar type 2 genes were orthologues between all isolates with some not identified in sk047 and sk048.
OrthoFinder gene cluster outputs were further visualized using “UpSets” to determine the membership of genes within each cluster. The majority of all gene clusters were present in all isolates with the exception of SICAvar type 1 clusters with between 10–15 unique SICAvar type 1 clusters per isolate (Figure 5B). For kir genes, the majority of clusters were shared between all isolates with the exception of a single unique kir gene cluster in each of sk047 and sk048. The majority of SICAvar type 2 genes were orthologues between all isolates with some not identified in sk047 and sk048 (Figure 5B).
Discussion
Here, we demonstrate the utility of accessible, portable, and affordable PCR-free long-read ONT MinION sequencing to de novo assemble P. knowlesi genomes from small clinical samples, essentially wild-type parasites. The new genome sequences are robust and add context to our understanding of P. knowlesi genome structure, organization, and variability.
Three Plasmodium knowlesi draft genomes were assembled from two P. knowlesi clinical isolates (sks047 and sks048), and the other was a control genome from the P. knowlesi A1-H.1 (StAPkA1H1) experimental cultured line (Moon et al., 2013). Comparison of the de novo StAPkA1H1 genome assembled here with the P. knowlesi A.1-H1 genome generated using Illumina and PacBio platforms (Benavente et al., 2018) and the P. knowlesi reference genome PKNH (Pain et al., 2008) demonstrated that our sequencing platform and subsequent assembly pipeline produced robust and reliable de novo P. knowlesi genome sequences.
The two clinical isolates (sks047 and sks048) and the control (StAPkA1H1) resolved into 14 chromosomes as expected for Plasmodium spp. and one “bin” chr00. The PKNH reference genome also resolves into 14 chromosomes and one chr00 where 1.73% of the total sequence comprising 62 genes was assigned (Pain et al., 2008). Chr00 of StAPkA1H1, sks047, and sks048 contain 1.59%, 2.09%, and 1.94% total sequence length with 18, 35, and 25 genes, respectively. Failure of sequences to pass quality thresholds would be expected to be randomly distributed genome-wide as observed in sks047 and sks048 chr00 sequences. The observed clustering of StAPkA1H1 chr00 with PKNH chr00 is difficult to explain unless de novo chromosome structuring was being overridden, forcing StAPkA1H1 contigs into a chr00 to fit the pattern set by the PKNH reference genome (Assefa et al., 2015; Steinbiss et al., 2016).
During chromosome structuring, we found that the minimap2 alignment function of RagTag was unable to resolve chimeric contigs for sks047, sks048, and StAPkA1H1, perhaps as a function of the algorithm heuristics in minimap2 or localized flaws in our pipeline. Consequently, sections of sks047 chromosomes 02 and 03, which were incorrectly placed in chromosomes 14 and 13 due to chimeric contigs, were successfully corrected using the nucmer aligner function of RagTag.
In general, RagTag struggled to resolve regions of low complexity and high variability, such as telomeric regions, although we report predicted genes within these telomeric regions, including some members of the SICAvar gene family and those described by Lapp et al. (2018). More strikingly, the Duffy-binding protein and TrpRA genes are almost exclusively located at the extreme ends of the de novo assembled genomes presented here. Indeed, Otto et al., 2018 reported that Companion, as used here, can construct Plasmodium chromosomes in their entirety (Otto et al., 2018).
The published PKNH P. knowlesi genome (Pain et al., 2008) and de novo assembled StPkA1H1 genome have a similar compliment of Interspersed Repeat (IR) genes while the clinical samples are similar to each other but quite different to the experimental parasite lines. The clinical isolates have approximately half the number of kir genes compared with PKNH and StPkA1H1. Unexpectedly, the clinical isolates have IR genes annotated as P. vivax (vir) that are absent in PKNH and StPkA1H1. The most parsimonious explanation for this difference is that the data from P. vivax vir genes are derived from clinical samples, and there are no well-established experimental lines for P. vivax. Published virs may more closely reflect IR diversity accrued in contemporary parasites from the Old World monkey parasite clade that includes P. knowlesi and P. vivax (Singh et al., 2004). Nonetheless, it is an interesting observation that deserves further investigation.
BUSCO genes, with a similar duplication composition in the sks047, sks048, and StAPkA1H1 draft genomes, were used to compare duplication profiles for SICAvar type 1, SICAvar type 2, and kir gene family members that code for antigenically variable parasite proteins expressed on the surface of infected host red blood cells. The SICAvar type 1, SICAvar type 2, and kir gene population in all three draft genomes had significantly different duplication profiles when compared with 100 randomly selected genes (Mann–Whitney U test: p << 0.001). This suggests that the parasite genome tolerates high levels of duplication at these loci. Non-synonymous substitution over synonymous substitution (dN/dS) values greater than 1.0 are associated with positive selection pressure. BUSCO core eukaryotic genes are not thought to be under undue selection pressure and were used as a control gene set in dN/dS analysis to investigate selection pressure on clusters containing SICAvar type 1, SICAvar type 2, and kir genes. The mean dN/dS was 2.4 for SICAvar type 1 gene clusters, 2.74 for SICAvar type 2 clusters, and 2.35 for kir gene clusters while dN/dS scores for BUSCO gene clusters was 0.35, suggesting that the SICAvar type 1, SICAvar type 2, and kir gene populations are under strong positive selection pressure. Given that the protein products of these multiple gene family members are expressed at the forefront of parasite–host interactions, positive selection in addition to the gene duplication profiles observed would be expected to accommodate antigenic variability and increase the chance of parasite survival in a hostile host environment.
On the backdrop of signatures of change and variability observed and the potential for genome destabilization at these rapidly evolving loci, the distribution of the SICAvar and kir genes within chromosomes seemed counterintuitive. Indeed, the ability of P. falciparum to tolerate the highly evolving PfEMP 1 gene family members is explained by their positioning in the extreme sub-telomeric regions of chromosomes that support higher rates of recombination in comparison to relatively more conserved centromeric regions (Otto et al., 2018). In the case of P. knowlesi, we found the rapidly evolving SICAvar and kir genes positioned in otherwise gene-sparse regions of chromosomes. With the exception of SICAvar type 2 genes in the 3’ direction, SICAvar and kir genes had significantly greater distances to neighboring genes in the 3’ and 5’ directions than BUSCO genes. Gene-sparse regions tolerate transposon and repetitive rich regions necessary to generate antigenic variability at these important loci while reducing the probability of impacting essential core gene function. Similar protective positioning of highly evolving genes is found in plant pathogens, for example, nematodes (Eves-van den Akker et al., 2016), aphids (Thorpe et al., 2018), phytophthora (Haas et al., 2009; Thorpe et al., 2021), and fungi (Dong et al., 2015). The capacity of some genomic regions to generate more variation than others is poorly understood, but in the field of plant pathogens, it is termed “the two speed genome” (Dong et al., 2015). The “two speed genome” concept may well describe accumulation of multiple gene family members in Plasmodium species, particularly the var genes, and consequently provide a biological model with which to explain antigenic variation in P. knowlesi.
To further demonstrate SICAvar type 1 genetic divergence, UpSet visualization of each of the draft genomes assembled here had between 10 and 15 unique SICAvar type 1 gene clusters, more than any other orthologous gene cluster. Indeed, only two SICAvar type 1 geneclusters were shared among the draft genomes. In contrast, the kir genes were less divergent with only one unique gene-cluster in sk048 and in sk047 with most kir gene clusters common between clinical isolates and experimental lines.
The ability to generate variation and maintain fitness is fundamental to pathogen–host interactions. The pathogen needs a lifespan long enough to replicate, disseminate, and maintain germlines. The ability to generate diversity on genes that code for “exposed” proteins while protecting core gene function increases the chance of pathogen survival. The strong signatures of positive selection pressure and gene duplication on the P. knowlesi SICAvar type 1, SICAvar type 2, and kir genes irrefutably demonstrate their importance in the fitness and evolution of this particular pathogen. The methods developed here will be used to generate P. knowlesi genomes from patient isolates with matched metadata for parasite genome-wide disease association analyses. Experimental Plasmodium knowlesi is particularly receptive to genome editing, facilitating allele-specific phenotyping (Mohring et al., 2020). Parasites edited with clinically relevant disease-associated alleles can be taken forward and characterized in vitro and in vivo for cause and effect. In essence, P. knowlesi as the agent of zoonotic malaria and as an experimental parasite has the potential to closely model severe malaria pathophysiology.
On a broader landscape, an opportunity is presented to the global research community to generate genome-wide data from clinical infections to add “real world” context to malaria research.
Data Availability Statement
The datasets presented in this study can be found in online repositories. The names of the repository/repositories and accession number(s) can be found below: Sequencing data can be found at NCBI SRA BioProject, accession no: PRJNA799698; Scripts used to generate the data in this project are available in github: https://github.com/damioresegun/Pknowlesi_denovo_genome_assembly and https://github.com/peterthorpe5/plasmidium_genomes.
Ethics Statement
The studies involving human participants were reviewed and approved by the University of St. Andrews Teaching and Research Ethics Committee. The patients/participants provided their written informed consent to participate in this study.
Author Contributions
DRO: data curation, formal analyses, investigation, methodology, and visualization. PT: formal analyses, software, investigation, and supervision. EDB: supervision. SC: resources. FM: resources. RWM: resources and draft editing. TGC: supervision, writing, and editing. JC-S: conceptualization, funding acquisition, methodology, project administration, resources, supervision, and writing the manuscript.
Funding
DRO is supported by the Wellcome Trust ISSF award 204821/Z/16/Z. Bioinformatics and computational biology analyses were supported by the University of St. Andrews Bioinformatics Unit (AMD3BIOINF), funded by Wellcome Trust ISSF awards 105621/Z/14/Z and 204821/Z/16/Z. The sample BioBank was compiled with informed consent (Medial Research Council, www.mrc.ac.uk, grant G0801971). Genome sequencing was supported by Tenovus Scotland (T16/03). TGC is funded by the Medical Research Council United Kingdom (grant nos. MR/M01360X/1, MR/N010469/1, MR/R025576/1, and MR/R020973/1) and BBSRC (grant no. BB/R013063/1). SC is funded by Medical Research Council United Kingdom grants (Refs. MR/M01360X/1, MR/R025576/1, and MR/R020973/1). RWM was supported by the UK Medical Research Council Career Award MR/M021157/1.
Conflict of Interest
The authors declare that the research was conducted in the absence of any commercial or financial relationships that could be construed as a potential conflict of interest.
Publisher’s Note
All claims expressed in this article are solely those of the authors and do not necessarily represent those of their affiliated organizations, or those of the publisher, the editors, and the reviewers. Any product that may be evaluated in this article, or claim that may be made by its manufacturer, is not guaranteed or endorsed by the publisher.
Acknowledgments
We would like to thank Joseph Ward for help with software and resources, Fiona Cook for providing resources for optimizing methodologies, and Cyrus J. Daneshvar for critically reading the manuscript.
Supplementary Material
The Supplementary Material for this article can be found online at: https://www.frontiersin.org/articles/10.3389/fgene.2022.855052/full#supplementary-material
References
Abdi, A. I., Hodgson, S. H., Muthui, M. K., Kivisi, C. A., Kamuyu, G., and Kimani, D. (2017). Plasmodium Falciparum Malaria Parasite Var Gene Expression Is Modified by Host Antibodies: Longitudinal Evidence from Controlled Infections of Kenyan Adults with Varying Natural Exposure. BMC Infect. Dis. 17 (1), 585. doi:10.1186/s12879-017-2686-0
Ahmed, A. M., Pinheiro, M. M., Divis, P. C., Siner, A., Zainudin, R., Wong, I. T., et al. (2014). Disease Progression in Plasmodium Knowlesi Malaria Is Linked to Variation in Invasion Gene Family Members. PLoS Negl. Trop. Dis. 8 (8), e3086. doi:10.1371/journal.pntd.0003086
Ahmed, M. A., and Quan, F. S. (2019). Plasmodium Knowlesi Clinical Isolates from Malaysia Show Extensive Diversity and Strong Differential Selection Pressure at the Merozoite Surface Protein 7D (MSP7D). Malar. J. 18 (1), 150. doi:10.1186/s12936-019-2782-2
Al-Khedery, B., Barnwell, J. W., and Galinski, M. R. (1999). Antigenic Variation in Malaria: a 3' Genomic Alteration Associated with the Expression of a P. Knowlesi Variant Antigen. Mol. Cell 3 (2), 131–141. doi:10.1016/s1097-2765(00)80304-4
Alonge, M., Soyk, S., Ramakrishnan, S., Wang, X., Goodwin, S., Sedlazeck, F. J., et al. (2019). RaGOO: Fast and Accurate Reference-Guided Scaffolding of Draft Genomes. Genome Biol. 20 (1), 224. doi:10.1186/s13059-019-1829-6
Andrade, C. M., Fleckenstein, H., Thomson-Luque, R., Doumbo, S., Lima, N. F., Anderson, C., et al. (2020). Increased Circulation Time of Plasmodium Falciparum Underlies Persistent Asymptomatic Infection in the Dry Season. Nat. Med. 26 (12), 1929–1940. doi:10.1038/s41591-020-1084-0
Assefa, S., Lim, C., Preston, M. D., Duffy, C. W., Nair, M. B., Adroub, S. A., et al. (2015). Population Genomic Structure and Adaptation in the Zoonotic Malaria Parasite Plasmodium Knowlesi. Proc. Natl. Acad. Sci. U. S. A. 112 (42), 13027–13032. doi:10.1073/pnas.1509534112
Bailly-Bechet, M., Haudry, A., and Lerat, E. (2014). “One Code to Find Them All”: a Perl Tool to Conveniently Parse RepeatMasker Output Files. Mob. DNA 5 (1), 13. doi:10.1186/1759-8753-5-13
Benavente, E. D., de Sessions, P. F., Moon, R. W., Grainger, M., Holder, A. A., Blackman, M. J., et al. (2018). A Reference Genome and Methylome for the Plasmodium Knowlesi A1-H.1 Line. Int. J. Parasitol. 48 (3-4), 191–196. doi:10.1016/j.ijpara.2017.09.008
Benavente, E. D., Gomes, A. R., De Silva, J. R., Grigg, M., Walker, H., Barber, B. E., et al. (2019). Whole Genome Sequencing of Amplified Plasmodium Knowlesi DNA from Unprocessed Blood Reveals Genetic Exchange Events between Malaysian Peninsular and Borneo Subpopulations. Sci. Rep. 9 (1), 9873. doi:10.1038/s41598-019-46398-z
Butcher, G. A., and Mitchell, G. H. (2018). The Role of Plasmodium Knowlesi in the History of Malaria Research. Parasitology 145 (1), 6–17. doi:10.1017/S0031182016001888
Cabanettes, F., and Klopp, C. (2018). D-GENIES: Dot Plot Large Genomes in an Interactive, Efficient and Simple Way. PeerJ 6, e4958. doi:10.7717/peerj.4958
Capella-Gutierrez, S., Silla-Martinez, J. M., and Gabaldon, T. (2009). trimAl: a Tool for Automated Alignment Trimming in Large-Scale Phylogenetic Analyses. Bioinformatics 25 (15), 1972–1973. doi:10.1093/bioinformatics/btp348
Chin, A. Z., Maluda, M. C. M., Jelip, J., Jeffree, M. S. B., Culleton, R., and Ahmed, K. (2020). Malaria Elimination in Malaysia and the Rising Threat of Plasmodium Knowlesi. J. Physiol. Anthropol. 39 (1), 36. doi:10.1186/s40101-020-00247-5
Chin, W., Contacos, P. G., Coatney, G. R., and Kimball, H. R. (1965). A Naturally Acquited Quotidian-type Malaria in Man Transferable to Monkeys. Science 149 (3686), 865. doi:10.1126/science.149.3686.86510.1126/science.149.3686.865.a
Chin, W., Contacos, P. G., Collins, W. E., Jeter, M. H., and Alpert, E. (1968). Experimental Mosquito-Transmission of Plasmodium Knowlesi to Man and Monkey. Am. J. Trop. Med. Hyg. 17 (3), 355–358. doi:10.4269/ajtmh.1968.17.355
Cock, P. J., Antao, T., Chang, J. T., Chapman, B. A., Cox, C. J., Dalke, A., et al. (2009). Biopython: Freely Available Python Tools for Computational Molecular Biology and Bioinformatics. Bioinformatics 25 (11), 1422–1423. doi:10.1093/bioinformatics/btp163
Cox-Singh, J. (2018). Plasmodium Knowlesi: Experimental Model, Zoonotic Pathogen and Golden Opportunity? Parasitology 145 (1), 1–5. doi:10.1017/S0031182017001858
Cox-Singh, J., and Culleton, R. (2015). Plasmodium Knowlesi: from Severe Zoonosis to Animal Model. Trends Parasitol. 31 (6), 232–238. doi:10.1016/j.pt.2015.03.003
Cox-Singh, J., Davis, T. M., Lee, K. S., Shamsul, S. S., Matusop, A., Ratnam, S., et al. (2008). Plasmodium Knowlesi Malaria in Humans Is Widely Distributed and Potentially Life Threatening. Clin. Infect. Dis. 46 (2), 165–171. doi:10.1086/524888
Cox-Singh, J., Hiu, J., Lucas, S. B., Divis, P. C., Zulkarnaen, M., Chandran, P., et al. (2010). Severe Malaria - a Case of Fatal Plasmodium Knowlesi Infection with Post-mortem Findings: a Case Report. Malar. J. 9, 10. doi:10.1186/1475-2875-9-10
Daneshvar, C., Davis, T. M., Cox-Singh, J., Rafa'ee, M. Z., Zakaria, S. K., Divis, P. C., et al. (2009). Clinical and Laboratory Features of Human Plasmodium Knowlesi Infection. Clin. Infect. Dis. 49 (6), 852–860. doi:10.1086/605439
Daneshvar, C., William, T., and Davis, T. M. E. (2018). Clinical Features and Management of Plasmodium Knowlesi Infections in Humans. Parasitology 145 (1), 18–31. doi:10.1017/S0031182016002638
Diez Benavente, E., Florez de Sessions, P., Moon, R. W., Holder, A. A., Blackman, M. J., Roper, C., et al. (2017). Analysis of Nuclear and Organellar Genomes of Plasmodium Knowlesi in Humans Reveals Ancient Population Structure and Recent Recombination Among Host-specific Subpopulations. PLOS Genet. 13 (9), e1007008. doi:10.1371/journal.pgen.1007008
Divis, P. C. S., Duffy, C. W., Kadir, K. A., Singh, B., and Conway, D. J. (2018). Genome-wide Mosaicism in Divergence between Zoonotic Malaria Parasite Subpopulations with Separate Sympatric Transmission Cycles. Mol. Ecol. 27 (4), 860–870. doi:10.1111/mec.14477
Dong, S., Raffaele, S., and Kamoun, S. (2015). The Two-Speed Genomes of Filamentous Pathogens: Waltz with Plants. Curr. Opin. Genet. Dev. 35, 57–65. doi:10.1016/j.gde.2015.09.001
Edgar, R. C. (2004). MUSCLE: Multiple Sequence Alignment with High Accuracy and High Throughput. Nucleic Acids Res. 32 (5), 1792–1797. doi:10.1093/nar/gkh340
Ellinghaus, D., Kurtz, S., and Willhoeft, U. (2008). LTRharvest, an Efficient and Flexible Software for De Novo Detection of LTR Retrotransposons. BMC Bioinforma. 9, 18. doi:10.1186/1471-2105-9-18
Emms, D. M., and Kelly, S. (2019). OrthoFinder: Phylogenetic Orthology Inference for Comparative Genomics. Genome Biol. 20 (1), 238. doi:10.1186/s13059-019-1832-y
Eves-van den Akker, S., Laetsch, D. R., Thorpe, P., Lilley, C. J., Danchin, E. G., Da Rocha, M., et al. (2016). The Genome of the Yellow Potato Cyst Nematode, Globodera Rostochiensis, Reveals Insights into the Basis of Parasitism and Virulence. Genome Biol. 17 (1), 124. doi:10.1186/s13059-016-0985-1
Flynn, J. M., Hubley, R., Goubert, C., Rosen, J., Clark, A. G., Feschotte, C., et al. (2020). RepeatModeler2 for Automated Genomic Discovery of Transposable Element Families. Proc. Natl. Acad. Sci. U. S. A. 117 (17), 9451–9457. doi:10.1073/pnas.1921046117
Fong, M. Y., Lau, Y. L., Jelip, J., Ooi, C. H., and Cheong, F. W. (2019). Genetic Characterisation of the Erythrocyte-Binding Protein (PkbetaII) of Plasmodium Knowlesi Isolates from Malaysia. J. Genet. 98. doi:10.1007/s12041-019-1109-y
Fu, L., Niu, B., Zhu, Z., Wu, S., and Li, W. (2012). CD-HIT: Accelerated for Clustering the Next-Generation Sequencing Data. Bioinforma. Oxf. Engl. 28 (23), 3150–3152. doi:10.1093/bioinformatics/bts565
Galinski, M. R., Lapp, S. A., Peterson, M. S., Ay, F., Joyner, C. J., Kg, L. E. R., et al. (2018). Plasmodium Knowlesi: a Superb In Vivo Nonhuman Primate Model of Antigenic Variation in Malaria. Parasitology 145 (1), 85–100. doi:10.1017/S0031182017001135
Gardner, M. J., Hall, N., Fung, E., White, O., Berriman, M., Hyman, R. W., et al. (2002). Genome Sequence of the Human Malaria Parasite Plasmodium Falciparum. Nature 419 (6906), 498–511. doi:10.1038/nature01097
Gel, B., and Serra, E. (2017). karyoploteR: an R/Bioconductor Package to Plot Customizable Genomes Displaying Arbitrary Data. Bioinformatics 33 (19), 3088–3090. doi:10.1093/bioinformatics/btx346
Gil, M., Zanetti, M. S., Zoller, S., and Anisimova, M. (2013). CodonPhyML: Fast Maximum Likelihood Phylogeny Estimation under Codon Substitution Models. Mol. Biol. Evol. 30 (6), 1270–1280. doi:10.1093/molbev/mst034
Gremme, G., Steinbiss, S., and Kurtz, S. (2013). GenomeTools: a Comprehensive Software Library for Efficient Processing of Structured Genome Annotations. IEEE/ACM Trans. Comput. Biol. Bioinform 10 (3), 645–656. doi:10.1109/tcbb.2013.68
Haas, B. J., Kamoun, S., Zody, M. C., Jiang, R. H., Handsaker, R. E., Cano, L. M., et al. (2009). Genome Sequence and Analysis of the Irish Potato Famine Pathogen Phytophthora Infestans. Nature 461 (7262), 393–398. doi:10.1038/nature08358
Harrison, T. E., Reid, A. J., Cunningham, D., Langhorne, J., and Higgins, M. K. (2020). Structure of the Plasmodium-Interspersed Repeat Proteins of the Malaria Parasite. Proc. Natl. Acad. Sci. U. S. A. 117 (50), 32098–32104. doi:10.1073/pnas.2016775117
Heather, J. M., and Chain, B. (2016). The Sequence of Sequencers: The History of Sequencing DNA. Genomics 107 (1), 1–8. doi:10.1016/j.ygeno.2015.11.003
Hunt, M., Silva, N. D., Otto, T. D., Parkhill, J., Keane, J. A., and Harris, S. R. (2015). Circlator: Automated Circularization of Genome Assemblies Using Long Sequencing Reads. Genome Biol. 16, 294. doi:10.1186/s13059-015-0849-0
Hviid, L., and Jensen, A. T. (2015). PfEMP1-A Parasite Protein Family of Key Importance in Plasmodium Falciparum Malaria Immunity and Pathogenesis. Adv. Parasitol. 88, 51–84. doi:10.1016/bs.apar.2015.02.004
Jeffares, D. C., Jolly, C., Hoti, M., Speed, D., Shaw, L., Rallis, C., et al. (2017). Transient Structural Variations Have Strong Effects on Quantitative Traits and Reproductive Isolation in Fission Yeast. Nat. Commun. 8 (1), 14061. doi:10.1038/ncomms14061
Jensen, A. R., Adams, Y., and Hviid, L. (2020). Cerebral Plasmodium Falciparum Malaria: The Role of PfEMP1 in its Pathogenesis and Immunity, and PfEMP1-Based Vaccines to Prevent it. Immunol. Rev. 293 (1), 230–252. doi:10.1111/imr.12807
Jiang, T., Liu, Y., Jiang, Y., Li, J., Gao, Y., Cui, Z., et al. (2020). Long-read-based Human Genomic Structural Variation Detection with cuteSV. Genome Biol. 21 (1), 189. doi:10.1186/s13059-020-02107-y
Knowles, R., and Gupta, B. M. D. (1932). A Study of Monkey-Malaria, and its Experimental Transmission to Man. Ind. Med. Gaz. 67 (6), 301–320.
Kohany, O., Gentles, A. J., Hankus, L., and Jurka, J. (2006). Annotation, Submission and Screening of Repetitive Elements in Repbase: RepbaseSubmitter and Censor. BMC Bioinforma. 7 (1), 474. doi:10.1186/1471-2105-7-474
Kolmogorov, M., Yuan, J., Lin, Y., and Pevzner, P. A.. (2019). Assembly of Long, Error-Prone Reads Using Repeat Graphs. Nat. Biotechnol. 37 (5), 540–546. doi:10.1038/s41587-019-0072-8
Laetsch, D. R., and Blaxter, M. L. (2017). BlobTools: Interrogation of Genome Assemblies. F1000Research 6, 1287. doi:10.12688/f1000research.12232.1
Lander, E. S., Linton, L. M., Birren, B., Nusbaum, C., Zody, M. C., Baldwin, J., et al. International Human Genome Sequencing Consortium (2001). Initial Sequencing and Analysis of the Human Genome. Nature 409 (6822), 860–921. doi:10.1038/35057062
Lapp, S. A., Geraldo, J. A., Chien, J. T., Ay, F., Pakala, S. B., Batugedara, G., et al. (2018). PacBio Assembly of a Plasmodium Knowlesi Genome Sequence with Hi-C Correction and Manual Annotation of the SICAvar Gene Family. Parasitology 145 (1), 71–84. doi:10.1017/S0031182017001329
Lapp, S. A., Mok, S., Zhu, L., Wu, H., Preiser, P. R., Bozdech, Z., et al. (2015). Plasmodium Knowlesi Gene Expression Differs in Ex Vivo Compared to In Vitro Blood-Stage Cultures. Malar. J. 14, 110. doi:10.1186/s12936-015-0612-8
Lavstsen, T., Turner, L., Saguti, F., Magistrado, P., Rask, T. S., Jespersen, J. S., et al. (2012). Plasmodium Falciparum Erythrocyte Membrane Protein 1 Domain Cassettes 8 and 13 Are Associated with Severe Malaria in Children. Proc. Natl. Acad. Sci. U. S. A. 109 (26), E1791–E1800. doi:10.1073/pnas.1120455109
Li, H. (2011). A Statistical Framework for SNP Calling, Mutation Discovery, Association Mapping and Population Genetical Parameter Estimation from Sequencing Data. Bioinforma. Oxf. Engl. 27 (21), 2987–2993. doi:10.1093/bioinformatics/btr509
Li, H. (2018). Minimap2: Pairwise Alignment for Nucleotide Sequences. Bioinformatics 34 (18), 3094–3100. doi:10.1093/bioinformatics/bty191
Li, H., Handsaker, B., Wysoker, A., Fennell, T., Ruan, J., Homer, N., et al. (2009). The Sequence Alignment/Map Format and SAMtools. Bioinformatics 25 (16), 2078–2079. doi:10.1093/bioinformatics/btp352
Li, W., and Godzik, A. (2006). Cd-hit: a Fast Program for Clustering and Comparing Large Sets of Protein or Nucleotide Sequences. Bioinforma. Oxf. Engl. 22 (13), 1658–1659. doi:10.1093/bioinformatics/btl158
Milner, D. A. (2018). Malaria Pathogenesis. Cold Spring Harb. Perspect. Med. 8 (1), a025569. doi:10.1101/cshperspect.a025569
Mohring, F., Hart, M. N., Patel, A., Baker, D. A., and Moon, R. W. (2020). CRISPR-Cas9 Genome Editing of Plasmodium Knowlesi. Bio Protoc. 10 (4), e3522. doi:10.21769/BioProtoc.3522
Moon, R. W., Hall, J., Rangkuti, F., Ho, Y. S., Almond, N., Mitchell, G. H., et al. (2013). Adaptation of the Genetically Tractable Malaria Pathogen Plasmodium Knowlesi to Continuous Culture in Human Erythrocytes. Proc. Natl. Acad. Sci. U. S. A. 110 (2), 531–536. doi:10.1073/pnas.1216457110
Morgulis, A., Coulouris, G., Raytselis, Y., Madden, T. L., Agarwala, R., and Schäffer, A. A. (2008). Database Indexing for Production MegaBLAST Searches. Bioinformatics 24 (16), 1757–1764. doi:10.1093/bioinformatics/btn322
Nattestad, M., and Schatz, M. C. (2016). Assemblytics: a Web Analytics Tool for the Detection of Variants from an Assembly. Bioinformatics 32 (19), 3021–3023. doi:10.1093/bioinformatics/btw369
Okonechnikov, K., Conesa, A., and García-Alcalde, F. (2016). Qualimap 2: Advanced Multi-Sample Quality Control for High-Throughput Sequencing Data. Bioinformatics 32 (2), 292–294. doi:10.1093/bioinformatics/btv566
Onditi, F. I., Nyamongo, O. W., Omwandho, C. O., Maina, N. W., Maloba, F., Farah, I. O., et al. (2015). Parasite Accumulation in Placenta of Non-immune Baboons during Plasmodium Knowlesi Infection. Malar. J. 14, 118. doi:10.1186/s12936-015-0631-5
Oresegun, D. R., Daneshvar, C., and Cox-Singh, J. (2021). Plasmodium Knowlesi – Clinical Isolate Genome Sequencing to Inform Translational Same-Species Model System for Severe Malaria. Front. Cell. Infect. Microbiol. 11 (90), 607686. doi:10.3389/fcimb.2021.607686
Otto, T. D., Bohme, U., Sanders, M., Reid, A., Bruske, E. I., Duffy, C. W., et al. (2018). Long Read Assemblies of Geographically Dispersed Plasmodium Falciparum Isolates Reveal Highly Structured Subtelomeres. Wellcome Open Res. 3, 52. doi:10.12688/wellcomeopenres.14571.1
Oxford Nanopore Technologies (2019). Medaka: Consensus Sequence Tool for Nanopore Sequences (version v0.6.5). Linux, Python. 2017. Oxford: Oxford Nanopore Technologies. Available at: https://github.com/nanoporetech/medaka.
Ozwara, H., Langermans, J. A., Maamun, J., Farah, I. O., Yole, D. S., Mwenda, J. M., et al. (2003). Experimental Infection of the Olive Baboon (Paplio Anubis) with Plasmodium Knowlesi: Severe Disease Accompanied by Cerebral Involvement. Am. J. Trop. Med. Hyg. 69 (2), 188–194. doi:10.4269/ajtmh.2003.69.188
Pain, A., Bohme, U., Berry, A. E., Mungall, K., Finn, R. D., Jackson, A. P., et al. (2008). The Genome of the Simian and Human Malaria Parasite Plasmodium Knowlesi. Nature 455 (7214), 799–803. doi:10.1038/nature07306
Pasini, E. M., Zeeman, A. M., Voorberg-Van Der Wel, A., and Kocken, C. H. M. (2018). Plasmodium Knowlesi: a Relevant, Versatile Experimental Malaria Model. Parasitology 145 (1), 56–70. doi:10.1017/S0031182016002286
Pedersen, B. S., and Quinlan, A. R. (2018). Mosdepth: Quick Coverage Calculation for Genomes and Exomes. Bioinformatics 34 (5), 867–868. doi:10.1093/bioinformatics/btx699
Pinheiro, M. M., Ahmed, M. A., Millar, S. B., Sanderson, T., Otto, T. D., Lu, W. C., et al. (2015). Plasmodium Knowlesi Genome Sequences from Clinical Isolates Reveal Extensive Genomic Dimorphism. PLoS One 10 (4), e0121303. doi:10.1371/journal.pone.0121303
Quinlan, A. R., and Hall, I. M. (2010). BEDTools: a Flexible Suite of Utilities for Comparing Genomic Features. Bioinformatics 26 (6), 841–842. doi:10.1093/bioinformatics/btq033
Ren, J., and Chaisson, M. J. P. (2020). Lra: the Long Read Aligner for Sequences and Contigs. PLoS Comput. Biol. 17 (6), e1009078. doi:10.1371/journal.pcbi.1009078
Shabani, E., Hanisch, B., Opoka, R. O., Lavstsen, T., and John, C. C. (2017). Plasmodium Falciparum EPCR-Binding PfEMP1 Expression Increases with Malaria Disease Severity and Is Elevated in Retinopathy Negative Cerebral Malaria. BMC Med. 15 (1), 183. doi:10.1186/s12916-017-0945-y
Simão, F. A., Waterhouse, R. M., Ioannidis, P., Kriventseva, E. V., and Zdobnov, E. M. (2015). BUSCO: Assessing Genome Assembly and Annotation Completeness with Single-Copy Orthologs. Bioinformatics 31 (19), 3210–3212. doi:10.1093/bioinformatics/btv351
Singh, B., Kim Sung, L., Matusop, A., Radhakrishnan, A., Shamsul, S. S., Cox-Singh, J., et al. (2004). A Large Focus of Naturally Acquired Plasmodium Knowlesi Infections in Human Beings. Lancet 363 (9414), 1017–1024. doi:10.1016/S0140-6736(04)15836-4
Stanke, M., Keller, O., Gunduz, I., Hayes, A., Waack, S., and Morgenstern, B. (2006). AUGUSTUS: Ab Initio Prediction of Alternative Transcripts. Nucleic Acids Res. 34 (Web Server issue), W435–W439. doi:10.1093/nar/gkl200
Steinbiss, S., Silva-Franco, F., Brunk, B., Foth, B., Hertz-Fowler, C., Berriman, M., et al. (2016). Companion: a Web Server for Annotation and Analysis of Parasite Genomes. Nucleic Acids Res. 44 (Web Server issue), W29–W34. doi:10.1093/nar/gkw292
Tessema, S. K., Nakajima, R., Jasinskas, A., Monk, S. L., Lekieffre, L., Lin, E., et al. (2019). Protective Immunity against Severe Malaria in Children Is Associated with a Limited Repertoire of Antibodies to Conserved PfEMP1 Variants. Cell Host Microbe 26 (5), 579–590 e575. doi:10.1016/j.chom.2019.10.012
Thorpe, P., Escudero-Martinez, C. M., Cock, P. J. A., Eves-van den Akker, S., and Bos, J. I. B. (2018). Shared Transcriptional Control and Disparate Gain and Loss of Aphid Parasitism Genes. Genome Biol. Evol. 10 (10), 2716–2733. doi:10.1093/gbe/evy183
Thorpe, P., Escudero-Martinez, C. M., Eves-van den Akker, S., and Bos, J. I. B. (2020). Transcriptional Changes in the Aphid Species Myzus Cerasi under Different Host and Environmental Conditions. Insect Mol. Biol. 29 (3), 271–282. doi:10.1111/imb.12631
Thorpe, P., Vetukuri, R. R., Hedley, P. E., Morris, J., Whisson, M. A., Welsh, L. R. J., et al. (2021). Draft Genome Assemblies for Tree Pathogens Phytophthora Pseudosyringae and Phytophthora Boehmeriae. G3 (Bethesda) 11 (11), jkab282. doi:10.1093/g3journal/jkab282
Thorvaldsdóttir, H., Robinson, J. T., and Mesirov, J. P. (2013). Integrative Genomics Viewer (IGV): High-Performance Genomics Data Visualization and Exploration. Briefings Bioinforma. 14 (2), 178–192. doi:10.1093/bib/bbs017
Vaser, R., Sović, I., Nagarajan, N., and Šikić, M. (2017). Fast and Accurate De Novo Genome Assembly from Long Uncorrected Reads. Genome Res. 27 (5), 737–746. doi:10.1101/gr.214270.116
Wahlgren, M., Goel, S., and Akhouri, R. R. (2017). Variant Surface Antigens of Plasmodium Falciparum and Their Roles in Severe Malaria. Nat. Rev. Microbiol. 15 (8), 479–491. doi:10.1038/nrmicro.2017.47
Walker, B. J., Abeel, T., Shea, T., Priest, M., Abouelliel, A., Sakthikumar, S., et al. (2014). Pilon: An Integrated Tool for Comprehensive Microbial Variant Detection and Genome Assembly Improvement. PLoS ONE 9 (11), e112963. doi:10.1371/journal.pone.0112963
Wang, Y., Tang, H., Debarry, J. D., Tan, X., Li, J., Wang, X., et al. (2012). MCScanX: a Toolkit for Detection and Evolutionary Analysis of Gene Synteny and Collinearity. Nucleic Acids Res. 40 (7), e49. doi:10.1093/nar/gkr1293
Keywords: Plasmodium knowlesi, genomes, clinical samples, SICAvar, kir, Nanopore, de novo, malaria
Citation: Oresegun DR, Thorpe P, Benavente ED, Campino S, Muh F, Moon RW, Clark TG and Cox-Singh J (2022) De Novo Assembly of Plasmodium knowlesi Genomes From Clinical Samples Explains the Counterintuitive Intrachromosomal Organization of Variant SICAvar and kir Multiple Gene Family Members. Front. Genet. 13:855052. doi: 10.3389/fgene.2022.855052
Received: 14 January 2022; Accepted: 15 April 2022;
Published: 23 May 2022.
Edited by:
Andrew Paul Jackson, University of Liverpool, United KingdomReviewed by:
Chenqi Wang, University of South Florida, United StatesMary Rose Galinski, Emory University, United States
Copyright © 2022 Oresegun, Thorpe, Benavente, Campino, Muh, Moon, Clark and Cox-Singh. This is an open-access article distributed under the terms of the Creative Commons Attribution License (CC BY). The use, distribution or reproduction in other forums is permitted, provided the original author(s) and the copyright owner(s) are credited and that the original publication in this journal is cited, in accordance with accepted academic practice. No use, distribution or reproduction is permitted which does not comply with these terms.
*Correspondence: Janet Cox-Singh, amNzMjZAc3QtYW5kcmV3cy5hYy51aw==
†These authors have contributed equally to this work