- 1Department of Pharmacy, Peking University First Hospital, Beijing, China
- 2Department of Pharmacy, The Third Hospital of Changsha, Changsha, China
- 3School of Pharmaceutical Sciences, Peking University Health Science Center, Beijing, China
- 4Department of Cardiology, Peking University First Hospital, Beijing, China
- 5Institute of Clinical Pharmacology, Peking University, Beijing, China
Background: The purpose of this study was to identify genetic variations associated with the metabolism of dabigatran in healthy Chinese subjects, with particular focus given to pharmacokinetics (PK) and pharmacodynamics (PD).
Methods: Healthy Chinese adults aged 18–65 years with unknown genotypes from a bioequivalence trial were included according to the protocol registered at ClinicalTrial.org (NCT03161496). All subjects received a single dose (150 mg) of dabigatran etexilate. PK (main outcomes: area under the concentration-time, AUC0-t, of total and free dabigatran) and PD (main outcomes: anti-FIIa activity, APTT, and PT) parameters were evaluated. Whole-exome sequencing and genome-wide association analyses were performed. Additionally, candidate gene association analyses related to dabigatran were conducted.
Results: A total of 118 healthy Chinese subjects were enrolled in this study. According to the p-value suggestive threshold (1.0 × 10−4), the following three SNPs were found to be associated with the AUC0–t of total dabigatran: SLC4A4 SNP rs138389345 (p = 5.99 × 10−5), FRAS1 SNP rs6835769 (p = 6.88 × 10−5), and SULT1A1 SNP rs9282862 (p = 7.44 × 10−5). Furthermore, these SNPs were also found to have significant influences on the AUC0–t of free dabigatran, maximum plasma concentration, and anti-FIIa activity (p < 0.05). Moreover, we identified 30 new potential SNPs of 13 reported candidate genes (ABCB1, ABCC2, ABCG2, CYP2B6, CYP1A2, CYP2C19, CYP3A5, CES1, SLCO1B1, SLC22A1, UGT1A1, UGT1A9, and UGT2B7) that were associated with drug metabolism.
Conclusion: Genetic variations were indeed found to impact dabigatran metabolism in a population of healthy Chinese subjects. Further research is needed to explore the more detailed functions of these SNPs. Additionally, our results should be verified in studies that use larger sample sizes and investigate other ethnicities.
1 Introduction
Oral direct thrombin inhibitor (DTI), a new type of direct oral anticoagulants (DOACs), has been increasingly used in recent years for the prevention of stroke and systemic embolism in cases of non-valvular atrial fibrillation (NVAF) and are currently recommended as the first line of medication-based treatment for this condition, according to American CHEST Guidelines (Lip et al., 2018). Dabigatran, which is converted by liver esterase carboxylesterase 1 (CES1) from the oral prodrug dabigatran etexilate, is the sole reversible and active DTI (Stangier and Clemens, 2009). It was first approved by the United States (US) Food and Drug Administration (FDA) in 2010 for stroke prevention in patients with NVAF, and subsequently launched in China in 2013 (Li et al., 2020). With the advantages of rapid absorption, high bioavailability, fixed-dose regimens, requiring no coagulation monitoring, and having no food and few drug interactions, dabigatran is becoming more widely used in clinical application (Kanuri and Kreutz, 2019; Wieland and Shipkova, 2019).
Although the above characteristics of dabigatran are either equivalent or superior to Vitamin k Antagonists (Connolly et al., 2009), more attention should be given to its relative high risk for bleeding. In the US, dabigatran was the 10th most implicated drug for adverse drug events in emergency department visits among older adults in 2013–2014 (Shehab et al., 2016). However, the risk that dabigatran poses for bleeding may depend on inter-individual (as opposed to intra-individual) and ethnic variations. Extensive clinical experiences and studies have already demonstrated that inter-individual variations regarding pharmacokinetics (PK) and pharmacodynamics (PD) do exist. For example, Stangier et al. (2007) reported that the inter-individual coefficient of variation (CV) in PK parameters of dabigatran was approximately 30%, and that the CV in PD parameters was <10%. Besides, Chan et al. (2015) demonstrated that inter-patient variability in dabigatran level (geometric CV: 51–64%) was greater than intra-patient variability (geometric CV: 32–40%). Many factors, including renal function, age, weight, sex, and food consumption, may influence the PK parameters of dabigatran (Reilly et al., 2014). Yet these factors alone do not fully explain inter-individual variation, whether in the case of healthy volunteers or enrolled patients (Fawzy and Lip, 2019; Wieland and Shipkova, 2019).
The Framingham heart study (O'Donnell et al., 2001) would suggest that genetic factors play a major role in determining coagulation function. Dabigatran etexilate is a substrate of p-glycoprotein (P-gp), which is encoded by the ABCB1 gene and generally not metabolized by cytochrome oxidase P450 (CYP450), except at supra-therapeutic concentrations (Raymond et al., 2021). Besides activation by the CES1 enzyme as previously mentioned, dabigatran is also metabolized to four active metabolites by UDP-glucuronyltransferase (UGT) isoforms 1A9, 2B7, and 2B15 (<10%) (Raymond et al., 2021). So far, there have indeed been several previous pharmacogenomics studies of dabigatran that explore the effects of genetic variation on its PK/PD parameters and clinical outcomes (Supplementary 1, Supplementary Table S1). However, these studies were mainly focused on genetic polymorphisms of ABCB1 (rs1045642, rs1128503, rs2032582, and rs4148738) and CES1 (rs2244613, rs8192935, and rs7164787). Only two studies (Lähteenmäki et al., 2021; Liu et al., 2021) have explored the influences of other genes, such as CYP2D6, CYP3A5, SLC22A1, and so on. Furthermore, the results of previous studies have not been totally consistent (Ašić et al., 2018; Cullell et al., 2018; Xie et al., 2018; Kanuri and Kreutz, 2019; Raymond et al., 2021; Shnayder et al., 2021). Most analytical methods involved a candidate gene approach, and only one study applied genome-wide association (GWA) analysis (Paré et al., 2013). As stated in the GWA study (GWAS), the frequency of the C allele in CES1 rs2244613 varied from 15% in Europeans to 60% in Asians according to data from the 1000 Genomes Project (accessed July 2012), which has been verified in a recent Asian study (Ji et al., 2021). Finally, of all previous studies, only three have investigated Asian populations (Chinese and Japanese) (Tomita et al., 2016; Ji et al., 2021; Liu et al., 2021), where only one analyzed PD parameters (Ji et al., 2021) and none performed GWA.
With this information in mind, the current fixed doses of dabigatran may not be applicable to all real-world subjects. Additionally, data on genetic polymorphisms in Chinese populations is insufficient, and their potential roles in influencing PK/PD and clinical outcomes of dabigatran application remain unclear. Our study carried out whole-exome sequencing and genome-wide association analyses in healthy Chinese subjects, thus aiming to explore the potential metabolic pathways of dabigatran. Through studying the associations between various biomarkers and PK/PD outcomes in a separate cohort of healthy volunteers, we attempted to discover novel genetic marks and their respective values of influence on individual anticoagulation therapy with dabigatran.
Materials and Methods
Study Design
This genome-wide association study was based on a bioequivalence (BE) trial conducted in China, which was mainly performed to assess the BE of domestic generic dabigatran etexilate capsules, with reference to the original product (brand name: Pradaxa®), in healthy subjects under fasting and fed conditions (Li et al., 2020). Enrolled subjects with unknown genotypes were required to be 18–65 years old, have a BMI ranging from 19 to 26 kg/m2, and meet all other inclusion and exclusion criteria following screening visits. This BE trial followed an open-label, single-center, randomized, single-dose, four-period crossover design. Eligible healthy participants were randomly divided into either a test-reference-test-reference sequence group or a reference-test-reference-test sequence group. Subjects received a single dose of dabigatran etexilate capsule (150 mg) with 240 ml of warm water after either being fed a standard high-fat meal (Li et al., 2020) within 30 min or fasting overnight for at least 10 h. Then, they were discharged 48 h after drug administration. Based on the BE trial, we added genotype and PD parameter tests that were conducted during any one of the two reference periods. Additionally, PK parameters used for subsequent matching analysis were also obtained during this time.
This protocol was approved by an independent ethics committee and the Institutional Review Board of Peking University First Hospital (Protocol Code: 2016[1236]; date of approval: 11 October 2017) and the Third Hospital of Changsha (Protocol Code: 2018-KL-012; date of approval: 5 July 2018). The ClinicalTrial.org registration number was NCT03161496. This study was conducted in accordance with both the Good Clinical Practice guidelines and the Declaration of Helsinki. Prior to the beginning of the study, all involved subjects were briefed on the purpose, duration, and potential risks of the study and provided written informed consent.
Sample Collection
Serial blood samples used for PK parameter analysis were collected from the BE trial. Venous blood samples (4 ml) were collected before dosing and then at 17–18 time points after drug administration (Li et al., 2020). We also collected 2 ml blood samples for genotyping before dosing, and 2.7 ml blood samples for PD parameter tests at 0 and 2 h after administration.
For PK analysis, blood samples were collected in EDTA-K2 tubes and centrifuged for 10 min at 4°C and 1700 g within 1 hour of sampling. Plasma samples were transferred to cryovials and stored at −80°C until PK analysis. For PD analysis, blood samples were collected in sodium citrate (3.2% v/v) tubes and centrifuged for 15 min at room temperature and 2500 g within 1 hour of sampling. Plasma samples were transferred to cryovials that were stored at −80°C, and were subjected to PD analysis within 6 months of sampling. For genetic polymorphism testing, blood samples were collected in EDTA-K2 tubes, transferred to cryovials, and stored at below −60°C until genotype testing.
Pharmacokinetic Evaluations
Plasma concentrations of total and free (nonconjugated) dabigatran from BE trial center samples were determined by a home-validated liquid chromatography-tandem mass spectrometry (LC-MS/MS) method, which has been previously published (Li et al., 2020). PK parameters for total and free dabigatran were determined by standard noncompartmental methods using a WinNonlin 7.0 (Pharsight Corporation, California, United States). Maximum concentration (Cmax) and the time to Cmax (Tmax) were recorded from the observed data. Values for the terminal half-life (t1/2) and area under the curve (AUC) for plasma concentration at time zero versus the last measurable time point (AUC0-t) were calculated. AUC0-t(total), Cmax(total), Tmax(total), and t1/2(total) reflected the PK parameters of total dabigatran, while AUC0-t(free), Cmax(free), Tmax(free), and t1/2(free) represented that of free dabigatran. No significant matrix effect was observed. All samples were analyzed within their established storage stability periods.
Pharmacodynamic Evaluations
The prothrombin time (PT), activated partial thromboplastin time (APTT), and levels of anti-FIIa activity were assessed using the Sysmex® CS-2100i fully automated multiparameter hemostasis analyzer (Sysmex, Kobe, Japan) at Peking University First Hospital. PT and APTT were measured by validated Coagulation Method Assay Kits (Thromborel-S® and Actin®, Siemens Healthcare Diagnostics Products GmbH, Marburg, Germany). During the 2018 International Council for Standardization in Hematology (ICSH) (Gosselin et al., 2018), anti-FIIa activity was recommended as a marker for the qualitative assessment of dabigatran–furthermore, the correlation between anti-FIIA activity and drug concentration (p < 0.001) was demonstrated in our previous study of Chinese patients (Liu et al., 2020). Anti-FIIa activity was measured using a validated Chromogenic Method Anti-FIIa Kit (HEMOCLOT Thrombin Inhibitors®, HYPHEN BioMed, Neuville sur Oise, France). Specific calibrators and controls were used for dabigatran (BIOPHEN dabigatran® Calibrator and Control, HYPHEN BioMed, Neuville sur Oise, France). The results of the anti-FIIa assay were reported in dabigatran units (with limits of detection for dabigatran of 0–550 ng/ml). The two QC sample concentrations used were 24 and 399 ng/ml. The inter- and intra-day precision (RSD%) were measured as < 6.76% and 7.53%, whereas inter- and intra-day accuracy were determined to be 97.71% and 86.69%. Relative changes in PT and APTT were also used for PD assessments, which involved taking the ratio after administration to before administration (i.e., ∆PT2h = PT2h/PT0h).
Whole-Exome Sequencing
For DNA preparation, the quality of isolated genomic DNA was assessed by using the following three methods in combination: 1) DNA degradation and contamination were monitored on 0.8% agarose gels; 2) DNA purity was checked using the NanoPhotometer® Spectrophotometer (IMPLEN, CA, United States); 3) DNA concentration was measured using a Qubit® DNA Assay Kit in a Qubit® 3.0 Flurometer (Invitrogen, United States).
For library preparation and sequencing, the whole exome library was prepared using the Agilent SureSelectXT Human All Exon V6 Kit (Agilent Technologies Inc., United States) according to the manufacturer’s standard protocol. Briefly, qualified genomic DNA was fragmented to an average size of 200bp. The ends of the DNA fragments were subjected to end repair processes, followed by A-tailing and adapter ligation. DNA fragments with adapters ligated on both ends were selectively enriched by polymerase chain reaction (PCR). After PCR, library hybridization and exome capture were performed using biotin labeled probes and magnetic bead selection. Captured libraries were enriched and tagged by PCR to prepare for sequencing. The final libraries were quantified using a KAPA Library Quantification Kit (KAPA Biosystems, South Africa) and an Agilent 2100 Bioanalyzer. Paired-end sequencing (2 × 150 bp) was performed on an Illumina NovaSeq 6000 sequencer (Illumina, United States).
Variant filtering and prediction were performed on 347,010 single-nucleotide polymorphisms (SNPs) detected in exons, with 200 bp extensions on either end. SNPs were retained for association analysis when the missing rate was <10%, minor allele frequency was >5%, and Hardy-Weinberg equilibrium p-value was >10–6. Based on the high-quality data obtained after quality control, PCA conducted in PLINK 1.09 was used for stratified population assessment to remove outlier samples. Finally, 75,348 SNPs were tested for association with PK and PD parameters of dabigatran.
Statistical Analysis
Genome-wide analyses of PK and PD parameters pertaining to dabigatran were performed by linear regression, assuming an additive genetic model, in PLINK 1.09. To account for possible population stratification, all analyses were adjusted for the first four genetic principal components. Covariates of PK and PD indexes were also further adjusted for age, sex, food, and high-density lipoprotein (HDL) to correct for residual population stratification (the effect of sex and food on metabolism is shown in Supplementary 1, Supplementary Table S2). These variables were chosen because they were associated with PK and PD parameters of dabigatran in univariate analyses. Analyses of non-imputed data were performed using the Gen ABEL package in R, whereas imputed data were analyzed using the Prob ABEL package. According to our original plan, the genome-wide p-value significance threshold was set at the Bonferroni limit of 6.6 × 10–7 in the discovery phase–SNPs reaching this threshold were taken forward for replication. The significance level for successful replication was set at p = 0.05/75,348 of SNPs for which replication was attempted. Following the genome-wide analyses of the merged and imputed discovery and replication phase data, follow-up analyses were performed by adding each top hit SNP as an additional covariate in GWA. This procedure was performed in a sequential manner until no significant genome-wide signals remained. Regional plots were generated with LocusZoom (Pruim et al., 2010) and all other figures were created in R. Continuous variables are presented as mean ± standard deviation (SD), and two-sided p-values < 0.05 were considered statistically significant.
Candidate Gene Association Analysis
To extensively investigate the influences of previously reported candidate genes on PK and PD parameters of dabigatran in our study population, we also identified related genes and made detailed analyses of these genes. According to previous pharmacogenomic studies (Supplementary 1, Supplementary Table S1) and reviews (Raymond et al., 2021; Shnayder et al., 2021), the following 22 genes were selected: ABCB1, ABCC2, ABCG2, CES1, CES1P2, CYP1A2, CYP2A6, CYP2B6, CYP2C8, CYP2C9, CYP2C19, CYP2J2, CYP2D6, CYP3A4, CYP3A5, CYP4F2, SLCO1B1, SLC22A1, UGT1A1, UGT1A9, UGT2B7, and UGT2B15. According to the number of independent variables and whether the data were normally distributed, we used the Student’s t-test or one-way analysis of variance (ANOVA) and the Mann-Whitney or nonparametric Kruskal–Wallis H test to analyze the data. Age, sex, food, and HDL were adjusted for by multivariate linear regression. Two-sided p-values < 0.05 were considered statistically significant.
Results
Basic Characteristics and PK/PD Parameters
A total of 118 healthy Chinese subjects were enrolled, of which mean age and BMI were 24 years (range: 18–41 years) and 22.2 kg/m2 (range: 19.1–25.9 kg/m2), respectively. Basic participant characteristics are presented in Table 1. Among the subjects, 59 volunteers completed the study under the fasting condition and the remaining 59 under the fed condition. After a single dose (150 mg) of dabigatran, the PK and PD outcomes for all subjects were as follows: for total dabigatran, AUC0–t: 1247.43 ± 442.08 ng h/mL, Cmax: 139.99 ± 55.61 ng/ml, Tmax: 3.61 ± 2.09 h, and t1/2: 8.78 ± 1.33 h; for free dabigatran, AUC0–t: 1110.42 ± 404.57 ng h/mL, Cmax: 125.22 ± 48.26 ng/ml, Tmax: 3.65 ± 2.10 h, and t1/2: 8.57 ± 1.57 h.
Effect of Suggestive Genetic Variations on the Metabolism of Dabigatran
According to the genome-wide significance threshold (p = 6.6 × 10–7), no SNP was identified as significant. Considering the sample size of this exploratory experiment was small (n = 118), the p-value was adjusted to 1.0 × 10−4 for screening suggestive genetic variations on the main metabolic parameter of AUC0–t(total). Three SNPs, SLC4A4 SNP rs138389345 (p = 5.99 × 10−5), FRAS1 SNP rs6835769 (p = 6.88 × 10−5), and SULT1A1 SNP rs9282862 (p = 7.44 × 10−5), exceeded our suggestive threshold for association with AUC0–t(total) (Manhattan plots and quantile-quantile plots presented in Supplementary 2, Supplementary Figures S1, S2). The related information of the three SNPs and their effects on outcomes are summarized in Table 2. Regional association plots within 500 kilobases of each SNP are shown in Figure 1. The minor alleles of SLC4A4 SNP rs138389345 and SULT1A1 SNP rs9282862 were both significantly associated with a lower AUC0–t(total), while the minor allele of FRAS1 SNP rs6835769 was associated with a higher AUC0–t(total). The trends of influence on AUC0–t(free) for these suggestive SNPs could be also shown significantly (p < 1.0 × 10−3), with regional association plots presented in Figure 2. The effects of the three SNPs on Cmax(total), Cmax(free), and IIa2h were also similar (p < 0.05). However, these SNPs had no significant effect on the Tmax or t1/2 of total or free dabigatran. As for the other PD parameters, the minor allele of SLC4A4 SNP rs138389345 corresponded to a significantly lower APTT2h, and the minor allele of SULT1A1 SNP rs9282862 had a significantly lower ∆PT2h (p < 0.05). However, FRAS1 SNP rs6835769 was not associated with any index of APTT or PT.
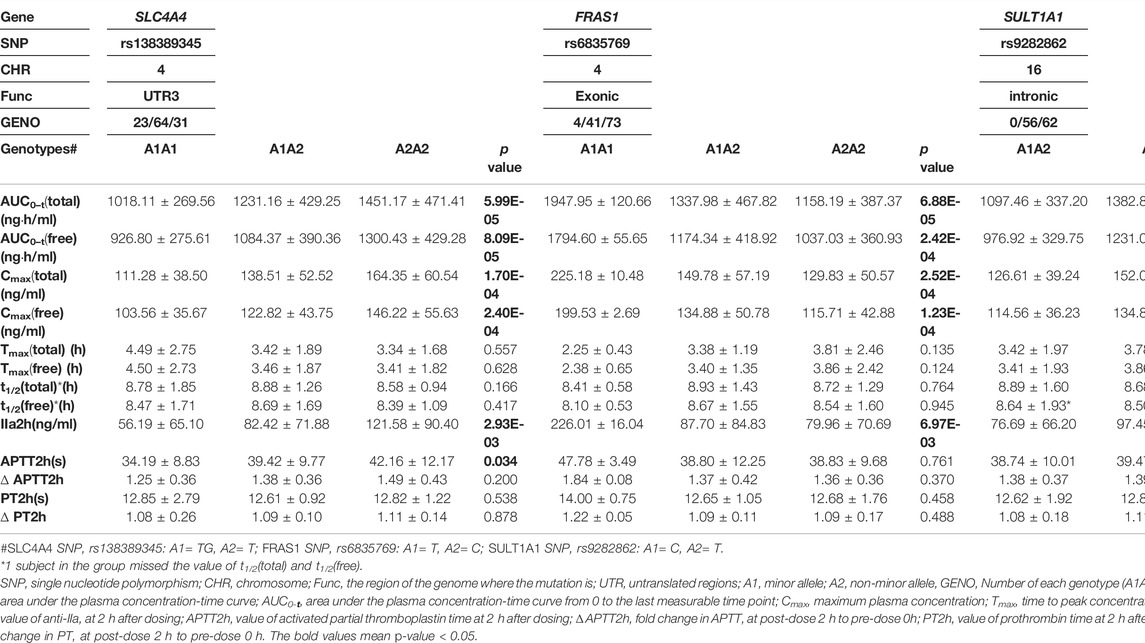
TABLE 2. Effect of suggestive genetic variations on pharmacokinetic and pharmacodynamic parameters of dabigatran.
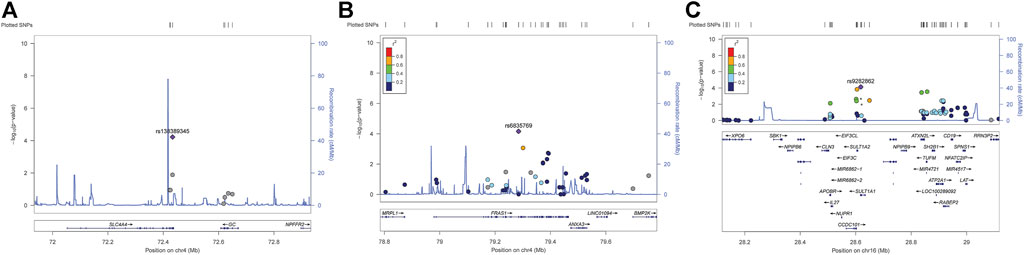
FIGURE 1. Regional association plots of suggestive SNPs on AUC0–t of total dabigatran. (A) SLC4A4 SNP rs138389345 (B) FRAS1 SNP rs6835769 (C) SULT1A SNP rs9282862. Annotation: SNPs are shown according to their physical location and–log10 p-values for association. Also shown is the recombination rate in centimorgans per megabase (blue line) and the linkage disequilibrium (r2) of each SNP with the SNP having the lowest p-value.
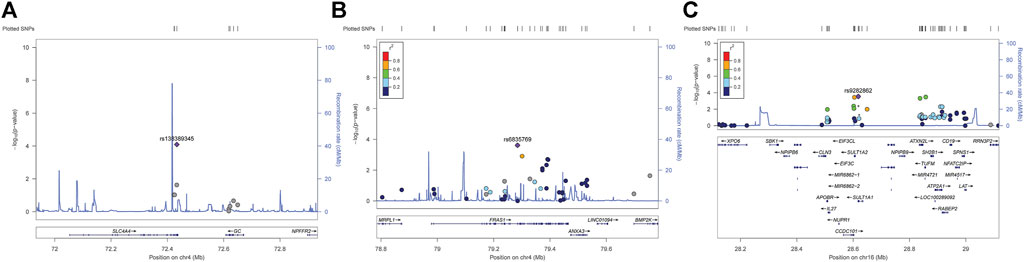
FIGURE 2. Regional association plots of suggestive SNPs on AUC0–t of free dabigatran. (A) SLC4A4 SNP rs138389345 (B) FRAS1 SNP rs6835769 (C) SULT1A SNP rs9282862. Annotation: SNPs are shown according to their physical location and–log10 p-values for association. Also shown is the recombination rate in centimorgans per megabase (blue line) and the linkage disequilibrium (r2) of each SNP with the SNP having the lowest p-value.
Candidate Gene Association Analysis
For correlation analyses of candidate genes, the following 18 previously reported genes were finally included in this study: ABCB1, ABCC2, ABCG2, CES1, CYP1A2, CYP2A6, CYP2B6, CYP2C8, CYP2C19, CYP2J2, CYP2D6, CYP3A5, CYP4F2, SLCO1B1, SLC22A1, UGT1A1, UGT1A9, and UGT2B7. Among the 95 detected SNPs in this study, 10 SNPs of seven genes were reported in previous pharmacogenomic studies, including ABCB1 SNP rs1045642, ABCC2 SNPs rs2273697 & rs717620, ABCG2 SNP rs2231142, CES1 SNP rs2244613, CYP2C19 SNP rs4244285, CYP2D6 SNPs rs1065852 & rs28371725, and SLCO1B1 SNPs rs2306283 & rs4149056. The effects of candidate genes on AUC0–t and other PK/PD parameters are presented in Table 3 and Supplementary 1, Supplementary Tables S3, S4. Most of the results for these SNPs were consistent with previous studies, except those for three SNPs. ABCC2 SNP rs2273697 and SLCO1B1 SNP rs4149056 were significantly associated with AUC0–t and Cmax, while ABCB1 SNP rs1045642 had no influence on any of the PK or PD indexes in our study. Furthermore, 30 new potential SNPs of 13 genes were determined to be associated with PK/PD parameters. For the PK parameters, ABCC2 SNP rs4148395, SLCO1B1 SNPs rs2291075 & rs11045748, CYP2B6 SNP rs56156262, UGT1A1 SNP rs4148323, and UGT1A9 SNP rs7586110 may have influences on AUC0–t and Cmax; additionally, the last mentioned SNP may even be associated with Tmax. Furthermore, three SNPs (ABCB1 rs2235015, CYP2B6 rs434606, and UGT1A1 rs4148327) were associated with Cmax, six (ABCB1 rs2235047, ABCG2 rs2231156, UGT2B7 chr4:69879878 & rs115791839, and UGT1A9 rs7563561 & rs7608175) with Tmax, and two (CES1 rs56278207 and CYP1A2 rs4646427) with t1/2. For the PD parameters, only two SNPs (CYP2B6 rs2279342 and rs34433978) might influence IIa2h, where the latter has also been associated with APTT2h, PT2h, and ∆PT2h. SLCO1B1 SNP rs11045748 might influence APTT2h, ∆APTT2h, and ∆PT2h. CYP2C19 SNPs rs12769205, rs3758580, and rs4244285 might affect both APTT2h and PT2h. The remaining two (ABCG2 rs2231138 and SLC22A1 rs622591) and one (CYP1A2 rs4646427) SNPs were associated with APTT2h and ∆APTT2h, respectively. At last, CYP3A5 SNPs rs15524 and rs4646453 had effects on ∆PT2h.
Discussion
To the best of our knowledge, this was the first genome-wide association study to investigate genetic variations associated with pharmacokinetic and pharmacodynamic characteristics of dabigatran metabolism among healthy Chinese individuals. We performed whole-exome sequencing and candidate gene analysis to extensively explore and verify both novel and reported SNPs that may impact PK/PD parameters. First, we identified three suggestive SNPs (SLC4A4 rs138389345, FRAS1 rs6835769, and SULT1A1 rs9282862) that were associated with the metabolism of dabigatran. AUC0–t(total), AUC0–t(free), Cmax(total), Cmax(free), and IIa2h values in the minor alleles of SLC4A4 rs138389345 and SULT1A1 rs9282862 were significantly lower compared to in their wild type counterparts. Conversely, these values were significantly higher in FRAS1 SNP rs6835769. Additionally, SLC4A4 rs138389345 and SULT1A1 rs9282862 were found to have significant influences on APTT and PT, respectively. Moreover, through correlation analyses of reported candidate genes, we found inconsistencies in the results for three previously reported SNPs (ABCC2 rs2273697, SLCO1B1 rs4149056, and ABCB1 rs1045642) regarding their associations with AUC0–t, Cmax, and PD indexes. Furthermore, we identified 30 new potential SNPs of 13 reported candidate genes in our study. ABCB1, ABCC2, ABCG2, CYP2B6, CYP1A2, CES1, SLCO1B1, UGT1A1, UGT1A9, and UGT2B7 might influence PK parameters, while ABCG2, CYP1A2, CYP2B6, CYP2C19, CYP3A5, SLCO1B1, and SLC22A1 might influence PD characteristics. Since our study was based on an original BE trial in healthy subjects, the accuracy of the PK/PD results was high and no known diseases or other factors affected drug metabolism. These same advantages of the previous study, which followed a similar experimental design (Liu et al., 2021), increased the quality and reliability of our results for determining the impact of various genetic polymorphisms on PK and PD parameters of dabigatran metabolism.
The major pharmacokinetic features of dabigatran etexilate include having a rapid absorption, low bioavailability (3–7%), variable peak concentration, steady state concentration attainment within two to 3 days, bi-exponential distribution phase, volume of distribution (50–70 L), 35% bind rate to plasma proteins, hepatic conjugation with glucuronide, and ability for renal elimination (80%) (Kanuri and Kreutz, 2019). As a BCS Class IIb drug, dabigatran etexilate exhibits high solubility at acidic pHs, but tends to precipitate after emptying from the stomach into the small intestine, leading to low bioavailability (Tsume et al., 2014). As previous reported, dabigatran is mainly eliminated in a non-metabolized form by urine (80%), and approximately 20% is conjugated to form dabigatran acylglucuronide eliminated in stools (Blech et al., 2008). While recent research by Park et al. (2021) with a new direct measurement method revealed that the portion of dabigatran conjugated to form dabigatran acylglucuronide would be much higher than previous results by the old indirect method. As renal excretion is the predominant elimination pathway for dabigatran, the medication is avoided in patients with impaired kidney function–especially those with end-stage renal disease (Ašić et al., 2018). Compared with the negligible role of phase I metabolic reactions, the formation of 1-O-acylglucuronide in phase II is the predominant route of dabigatran metabolism in humans (Blech et al., 2008). After conjugation with activated glucuronic acid, dabigatran acylglucuronide as the main metabolite possess the similar or even weaker anticoagulant effects to prolong APTT, PT, and TT compared with dabigatran (Blech et al., 2008; Kim et al., 2022).
Drug transporters, including members of the ATP-binding cassette (ABC) and solute carrier (SLC) superfamilies, are recognized as important determinants for governing the therapeutic response of many drugs (César-Razquin et al., 2015). SLC4A4, located in chromosome 4q13.3, encodes an anion exchange protein named electrogenic sodium bicarbonate cotransporter 1 (NBCe1) (Demirci et al., 2006). NBCe1 is involved in the regulation of bicarbonate secretion and absorption, as well as intracellular pH. Mutations in this gene are associated with proximal renal tubular acidosis, an extremely rare autosomal recessive syndrome involving ocular abnormalities and developmental delay (Dinour et al., 2004). SLC4A4 SNP rs138389345 is a variant of SLC4A4 in which a single G base is deleted (G > -). From the HPA RNA-seq normal tissue data, the SLC4A4 gene is most expressed in the kidney. NBCe1 plays a major role in renal bicarbonate absorption via the proximal tubules and maintaining normal blood pH (Demirci et al., 2006). Abnormal function of NBCe1 can cause severe HCO3- reabsorption and decrease blood HCO3- concentration, resulting in acidic pH. Due to the strong pH-dependent solubility of dabigatran etexilate, with decreased solubility at basic pH values (Chai et al., 2016), it is reasonable to speculate the influence of SLC4A4 genetic variance on dabigatran metabolism. In our study, SLC4A4 SNP rs138389345 showed a significantly higher AUC0-t(total) (TGTG 1018.11 ± 269.56, TGT 1231.16 ± 429.25, TT 1451.17 ± 471.41, ngh/ml). Additionally, this SNP resulted in increased values for AUC0-t(free), Cmax(total), Cmax(free), IIa2h, and APTT2h.
The FRAS1 gene, also known as KIAA1500, is located in chromosome 4q21.21, encodes the extracellular matrix (ECM) proteins, and regulates epidermal-basement membrane adhesion and organogenesis during development (Hoefele et al., 2013). FRAS1 SNP rs6835769 involves the mutation of a C base to a T base (C > T) within the gene, which can lead to the A817V mutation at the protein level. FRAS1 mutations have been confirmed to cause an extremely rare autosomal recessive genetic disorder named Fraser Syndrome (FRASRS), and seem to be responsible for non-syndromic unilateral renal agenesis (Hoefele et al., 2013). Furthermore, FRAS1 mutations are associated with bone metabolism, where FRAS1 may be involved in the metabolism of calcium and phosphorous (Reyer et al., 2019). While most studies of FRAS1 mutation have focused on its role in the pathogenesis and prognosis of diseases (Zhan et al., 2014), there has only been one GWAS (Fridley et al., 2016) that investigated drug related variations. From a study of 74 invasive epithelial ovarian cancer patients observed at the Mayo Clinic, it was found that some genes including FRAS1 may explain inter-patient variation in clinical response to platinum-taxane therapies. In our study, FRAS1 SNP rs6835769 was associated with the PK parameters of AUC0-t and Cmax, and followed the same trend of SLC4A4 mutations. Perhaps the reasoning behind this is as follows: the FRAS1 gene is secondarily expressed in the kidney, including in the glomerular podocytes and podocyte precursors (Pitera et al., 2008), while dabigatran is primarily excreted via the kidney. While this may provide preliminary insight into how FRAS1 impacts dabigatran metabolism, the detailed mechanism must be further explored and investigated through in vivo and in vitro studies.
Drugs usually undergo two phases of metabolism in the body. The main purpose of phase II metabolic reactions is to combine reactions, increase drug polarity, and facilitate drug exclusion from the body. The most common phase II biotransformation enzymes include sulfotransferases (SULTs), N-acetyltransferases (NATs), and methyltransferases (MTs). SULTs utilizes 3′-phospho-5′-adenylyl sulfate (PAPS) as a sulfonate donor to catalyze the sulfate conjugation of a wide variety of acceptor molecules that bear a hydroxyl or an amine group (Daniels and Kadlubar, 2014). Sulfonation increases the water solubility of most compounds, and therefore their potential for renal excretion, but it can also result in bioactivation to form active metabolites. The SULT1A1 gene locates in chromosome 16q11.2, and rs9282862 is a mutation of a T base to C base within a SULT1A1 intron. Proteins encoded by SULT1A1 are present in many human tissues, including the liver, kidney, and platelets (Raftogianis et al., 1997). The two most common SULT1A1 variant alleles are 638G > A (formerly named rs9282861, now named rs1042028, SULT1A1*2) and 667A > G (rs1801030, SULT1A1*3). Previous reports have confirmed that these alleles have significantly different frequencies depending on race (Carlini et al., 2001). SULT1A1 mutations could explain the wide range of individual differences in tamoxifen efficacy (Gjerde et al., 2008), and have also been shown to be involved in the heparan sulfate and heparin metabolism pathway (Jiang et al., 2011). Although no study (Shnayder et al., 2021) has investigated the impact of SULT1A1 gene variants on the efficacy or toxicity of apixaban, another DOAC, SULT1A1 is indeed involved in the conversion of approximately one fourth of the absorbed amount of Apixaban to inactive metabolites (Raymond et al., 2021). For the metabolism of Apixaban (leading to O-desmethyl-apixaban sulfate), SULT1A1 and SULT1A2 account for a quarter of its conversion to inactive metabolites (Kanuri and Kreutz, 2019). Several SNPs in linkage disequilibrium with each other and with SULT1A1*1 have also been identified in both the distal and proximal promoter region of SULT1A1, and are associated with platelet enzymatic activity (Ning et al., 2005; Lin et al., 2012). SNP rs9282862 is also in strong linkage disequilibrium with SULT1A1*1 (r2 = 1.00, D´= 1.00), as seen in data from the Gambian Genome Variation Project (GGVP). SLC4A4 SNP rs138389345 showed a significantly decreased AUC0-t(total) (TT 1382.89 ± 476.91, TC 1097.46 ± 337.20, ng h/ml), with similar trends observed in AUC0-t(free), Cmax(total), Cmax(free), IIa2h, PT2h, and ∆PT2h. Although this linkage disequilibrium needs to be verified in Asian populations, and UGTs were the main phase II enzymes involved, SULT1A1 variation may have the potential to be a new biomarker for antithrombotic therapy–it would certainly be worthy to conduct more detailed studies to investigate this possibility.
For the reported candidate genes, 13 genes (ABCB1, ABCC2, ABCG2, CYP2B6, CYP1A2, CYP2C19, CYP3A5, CES1, SLCO1B1, SLC22A1, UGT1A1, UGT1A9, and UGT2B7) were found to have significant effects on drug metabolism. The most commonly reported SNPs, including ABCB1 rs1045642 and CES1 rs2244613, had no influence on any PK or PD parameters in our study, which was a finding consistent with Ji Q’s research (Ji et al., 2021) but not totally with Liu Y’s study of Chinese participants (Liu et al., 2021). As we know, UGTs are involved in the production of active metabolites in a small proportion of dabigatran (Ishiguro et al., 2014). Besides, polymorphisms of CYP450 for isoenzymes 1A2, 2C8, 2C9, 2C19, and 2J2 have been shown to affect the pharmacokinetics of dabigatran, and especially the co-administration of DOACs with other drugs (Shnayder et al., 2021). Outside of the ABCB1 and CES1 genes, almost no study to date has focused on the associations between carriers of the UGT (including UGT1A9, 2B7, and 2B15), CYP, and SLC gene families and dabigatran metabolism in humans (Raymond et al., 2021; Shnayder et al., 2021). Zubiaur et al. (2020) investigated the influence of genetic polymorphisms in UGT and CYP, which encode for enzymes, and SLC, which encodes for transporters, on the pharmacokinetics and safety of dabigatran. In 107 healthy Caucasian and Latin-American volunteers, UGT1A1 rs887829, SLCO1B1, and most CYP polymorphisms were found to have no influence in this regard. On the other hand, while CYP2D6 poor metabolizers, SLC22A1 haplotype, and CYP3A5-expressing subjects (*1/*1) had positive change. Lähteenmäki et al. (2021) investigated the effects of ABCG2 and CYP3A5 variants on bleeding and thromboembolic events in Finnish patients who were given dabigatran, where the results were all negative. In our study, the positive SNPs which had the most effects on PK or PD parameters included SLCO1B1 rs11045748 (AUC0-t, Cmax, APTT2h, PT2h, and ∆PT2h), SLCO1B1 rs2291075 & rs4149056 (AUC0-t and Cmax), UGT1A9 rs7586110 (AUC0-t, Cmax, and Tmax), UGT1A1 rs4148323 & rs4148327, ABCC2 rs2273697 & rs4148395 (AUC0-t and Cmax), CYP2B6 rs56156262 (AUC0-t and Cmax), and CYP2B6 rs34433978 (IIa2h, APTT2h, PT2h, and ∆PT2h). The reported positive SNPs of CYP2D6, SLC22A1, CYP3A5, and ABCG2 were not consistent with previous studies (Zubiaur et al., 2020; Lähteenmäki et al., 2021). Accordingly, the impacts of all aforementioned SNPs should be verified in studies with larger sample sizes and that investigate other ethnicities.
There were a few key limitations to our study worth discussing. First, as the sample size was limited, no SNP was found to meet the genome-wide significance threshold. Therefore, future multi-center studies with larger sample sizes should be conducted. Second, not all potential SNPs could be detected by our genotyping method, such as the reported SNPs of ABCB1, CES1, SULT1A1 rs9282861, and UGT2B15 (Shnayder et al., 2021). Supplementary genome-wide detection or other forms of exploration should be performed to account for this. Third, as our study was only performed in healthy Chinese subjects, it should be replicated in another population–especially among patients and other ethnicities. As mentioned in the background, many factors may influence the metabolism of dabigatran and these indexes have different characteristics of distribution between healthy subjects and patients. Recent pharmacogenetic studies also showed different results between these two groups (Supplementary 1, Supplementary Table S1). Our findings Whether our findings can be replicated in patients or other ethnicities is worth exploring further. Finally, explorations into the functions of our newfound suggestive genes were mostly insufficient. More identification studies (both in vivo and in vitro) should be performed to document their detailed pathways in drug metabolism.
Conclusion
Our study found that genetic variations do impact the metabolism of dabigatran in healthy Chinese populations. The suggestive genes of SLC4A4, FRAS1, and SULT1A1 may be novel biomarkers and potential targets for anticoagulation therapy involving dabigatran. Furthermore, newly identified SNPs of 13 reported candidate genes (ABCB1, ABCC2, ABCG2, CYP2B6, CYP1A2, CYP2C19, CYP3A5, CES1, SLCO1B1, SLC22A1, UGT1A1, UGT1A9, and UGT2B7) may also affect dabigatran drug metabolism. Further studies are required to explore the more detailed functions of these SNPs. Additionally, the results of our study should be verified in a larger study among patients and to investigate their relationships with the clinical outcomes of antithrombotic therapy.
Data Availability Statement
The data presented in the study are deposited in the National Population Health Data Center (NPHDC) repository, accession number 10.12213/11.A0028.202009.338.V1.0 (https://www.ncmi.cn/phda/dataDetails.html?type=project_data&id=CSTR:A0006.11.A0028.202009.338.V1.0-V1.0). As the data of our paper was included in the whole data of the National Key R&D Program of China (No.2016YFC0904900), the name of deposited dataset was Chinese pharmacogenomics and precision medication gene sequencing dataset. The detailed information and the number of this program were also shown in NPHDC (link: https://www.ncmi.cn/phda/projectDataDetail.html?id=61d77ecc-5176-398e-a33a-c8458ad73b52).
Ethics Statement
The studies involving human participants were reviewed and approved by an independent ethics committee and the Institutional Review Board of Peking University First Hospital and the Third Hospital of Changsha. The patients/participants provided their written informed consent to participate in this study.
Author Contributions
YC, QiaX, XL, and QiuX designed the research; QiuX, YL, ZL, and HZ collected the data; QiuX, ZL, GM, and ZhW analyzed the data; QfX wrote the manuscript; ZiW, SZ, JJ, XL, QiaX, and YC involved in the discussion of results. All the authors have read and approved the final manuscript.
Funding
This study was supported by grants from the National Key R&D Program of China (2016YFC0904900), National Science and Technology Major Projects for “Major New Drugs Innovation and Development” (2017ZX09101001), National Natural Science Foundation of China (82073935, 81973395, and 81872940), and Beijing Municipal Commission of Science and Technology of China Pharmaceutical Innovation Cultivation and Industry Support Platform Capacity Construction Project (Z191100007619038).
Conflict of Interest
The authors declare that the research was conducted in the absence of any commercial or financial relationships that could be construed as a potential conflict of interest.
Publisher’s Note
All claims expressed in this article are solely those of the authors and do not necessarily represent those of their affiliated organizations, or those of the publisher, the editors and the reviewers. Any product that may be evaluated in this article, or claim that may be made by its manufacturer, is not guaranteed or endorsed by the publisher.
Supplementary Material
The Supplementary Material for this article can be found online at: https://www.frontiersin.org/articles/10.3389/fgene.2022.873031/full#supplementary-material
References
Ašić, A., Marjanović, D., Mirat, J., and Primorac, D. (2018). Pharmacogenetics of Novel Oral Anticoagulants: A Review of Identified Gene Variants & Future Perspectives. Per. Med. 15 (3), 209–221. doi:10.2217/pme-2017-0092
Blech, S., Ebner, T., Ludwig-Schwellinger, E., Stangier, J., and Roth, W. (2008). The Metabolism and Disposition of the Oral Direct Thrombin Inhibitor, Dabigatran, in Humans. Drug Metab. Dispos. 36 (2), 386–399. doi:10.1124/dmd.107.019083
Carlini, E. J., Raftogianis, R. B., Wood, T. C., Jin, F., Zheng, W., Rebbeck, T. R., et al. (2001). Sulfation pharmacogenetics:SULT1A1 and SULT1A2 Allele Frequencies in Caucasian, Chinese and African-American Subjects. Pharmacogenetics 11 (1), 57–68. doi:10.1097/00008571-200102000-00007
César-Razquin, A., Snijder, B., Frappier-Brinton, T., Isserlin, R., Gyimesi, G., Bai, X., et al. (2015). A Call for Systematic Research on Solute Carriers. Cell 162 (3), 478–487. doi:10.1016/j.cell.2015.07.022
Chai, F., Sun, L., Ding, Y., Liu, X., Zhang, Y., Webster, T. J., et al. (2016). A Solid Self-Nanoemulsifying System of the BCS Class IIb Drug Dabigatran Etexilate to Improve Oral Bioavailability. Nanomedicine 11 (14), 1801–1816. doi:10.2217/nnm-2016-0138
Chan, N. C., Coppens, M., Hirsh, J., Ginsberg, J. S., Weitz, J. I., Vanassche, T., et al. (2015). Real-world Variability in Dabigatran Levels in Patients with Atrial Fibrillation. J. Thromb. Haemost. 13 (3), 353–359. doi:10.1111/jth.12823
Connolly, S. J., Ezekowitz, M. D., Yusuf, S., Eikelboom, J., Oldgren, J., Parekh, A., et al. (2009). Dabigatran versus Warfarin in Patients with Atrial Fibrillation. N. Engl. J. Med. 361 (12), 1139–1151. doi:10.1056/nejmoa0905561
Cullell, N., Carrera, C., Muiño, E., Torres, N., Krupinski, J., and Fernandez-Cadenas, I. (2018). Pharmacogenetic Studies with Oral Anticoagulants. Genome-wide Association Studies in Vitamin K Antagonist and Direct Oral Anticoagulants. Oncotarget 9 (49), 29238–29258. doi:10.18632/oncotarget.25579
Daniels, J., and Kadlubar, S. (2014). Pharmacogenetics of SULT1A1. Pharmacogenomics 15 (14), 1823–1838. doi:10.2217/pgs.14.134
Demirci, F. Y., Chang, M. H., Mah, T. S., Romero, M. F., and Gorin, M. B. (2006). Proximal Renal Tubular Acidosis and Ocular Pathology: a Novel Missense Mutation in the Gene (SLC4A4) for Sodium Bicarbonate Cotransporter Protein (NBCe1). Mol. Vis. 12, 324–330.
Dinour, D., Chang, M.-H., Satoh, J.-i., Smith, B. L., Angle, N., Knecht, A., et al. (2004). A Novel Missense Mutation in the Sodium Bicarbonate Cotransporter (NBCe1/SLC4A4) Causes Proximal Tubular Acidosis and Glaucoma through Ion Transport Defects. J. Biol. Chem. 279 (50), 52238–52246. doi:10.1074/jbc.m406591200
Fawzy, A. M., and Lip, G. Y. H. (2019). Pharmacokinetics and Pharmacodynamics of Oral Anticoagulants Used in Atrial Fibrillation. Expert Opin. Drug Metab. Toxicol. 15 (5), 381–398. doi:10.1080/17425255.2019.1604686
Fridley, B. L., Ghosh, T. M., Wang, A., Raghavan, R., Dai, J., Goode, E. L., et al. (2016). Genome-Wide Study of Response to Platinum, Taxane, and Combination Therapy in Ovarian Cancer: In Vitro Phenotypes, Inherited Variation, and Disease Recurrence. Front. Genet. 7, 37. doi:10.3389/fgene.2016.00037
Gjerde, J., Hauglid, M., Breilid, H., Lundgren, S., Varhaug, J. E., Kisanga, E. R., et al. (2008). Effects of CYP2D6 and SULT1A1 Genotypes Including SULT1A1 Gene Copy Number on Tamoxifen Metabolism. Ann. Oncol. 19 (1), 56–61. doi:10.1093/annonc/mdm434
Gosselin, R. C., Adcock, D. M., Bates, S. M., Douxfils, J., Favaloro, E. J., Gouin-Thibault, I., et al. (2018). International Council for Standardization in Haematology (ICSH) Recommendations for Laboratory Measurement of Direct Oral Anticoagulants. Thromb. Haemost. 118 (3), 437–450. doi:10.1055/s-0038-1627480
Hoefele, J., Wilhelm, C., Schiesser, M., Mack, R., Heinrich, U., Weber, L. T., et al. (2013). Expanding the Mutation Spectrum for Fraser Syndrome: Identification of a Novel Heterozygous Deletion in FRAS1. Gene 520 (2), 194–197. doi:10.1016/j.gene.2013.02.031
Ishiguro, N., Kishimoto, W., Volz, A., Ludwig-Schwellinger, E., Ebner, T., and Schaefer, O. (2014). Impact of Endogenous Esterase Activity on In Vitro P-Glycoprotein Profiling of Dabigatran Etexilate in Caco-2 Monolayers. Drug Metab. Dispos. 42 (2), 250–256. doi:10.1124/dmd.113.053561
Ji, Q., Zhang, C., Xu, Q., Wang, Z., Li, X., and Lv, Q. (2021). The Impact of ABCB1 and CES1 Polymorphisms on Dabigatran Pharmacokinetics and Pharmacodynamics in Patients with Atrial Fibrillation. Br. Jnl Clin. Pharma 87 (5), 2247–2255. doi:10.1111/bcp.14646
Jiang, Z., Michal, J. J., Wu, X.-L., Pan, Z., and MacNeil, M. D. (2011). The Heparan and Heparin Metabolism Pathway Is Involved in Regulation of Fatty Acid Composition. Int. J. Biol. Sci. 7 (5), 659–663. doi:10.7150/ijbs.7.659
Kanuri, S. H., and Kreutz, R. P. (2019). Pharmacogenomics of Novel Direct Oral Anticoagulants: Newly Identified Genes and Genetic Variants. J. Pers. Med. 9 (1), 7. doi:10.3390/jpm9010007
Kim, J.-M., Noh, J., Park, J.-W., Chung, H., Kim, K.-A., Park, S. B., et al. (2022). Dabigatran Acylglucuronide, the Major Metabolite of Dabigatran, Shows a Weaker Anticoagulant Effect Than Dabigatran. Pharmaceutics 14 (2), 257. doi:10.3390/pharmaceutics14020257
Lähteenmäki, J., Vuorinen, A. L., Pajula, J., Harno, K., Lehto, M., Niemi, M., et al. (2021). Pharmacogenetics of Bleeding and Thromboembolic Events in Direct Oral Anticoagulant Users. Clin. Pharmacol. Ther. 110 (3), 768–776. doi:10.1002/cpt.2316
Li, X., Liu, L., Xu, B., Xiang, Q., Li, Y., Zhang, P., et al. (2020). Bioequivalence and Pharmacodynamics of a Generic Dabigatran Etexilate Capsule in Healthy Chinese Subjects under Fasting and Fed Conditions. Pharmacol. Res. Perspect. 8 (2), e00593. doi:10.1002/prp2.593
Lin, Z.-N., Lin, Y.-C., Zhang, X., Kadlubar, S., Tuo, J., Green, B., et al. (2012). Differential Promoter Activities of Functional Haplotypes in the 5′-flanking Region of humanSulfotransferase 1A1. J. Biochem. Mol. Toxicol. 26 (10), 422–428. doi:10.1002/jbt.21437
Lip, G. Y. H., Banerjee, A., Boriani, G., Chiang, C. e., Fargo, R., Freedman, B., et al. (2018). Antithrombotic Therapy for Atrial Fibrillation: CHEST Guideline and Expert Panel Report. Chest 154 (5), 1121–1201. doi:10.1016/j.chest.2018.07.040
Liu, Z., Xie, Q., Xiang, Q., Zhang, H., Mu, G., Zhao, Z., et al. (2020). Anti-FXa-IIa Activity Test in Asian and its Potential Role for Drug Adherence Evaluation in Patients with Direct Oral Anticoagulants: a Nationwide Multi-center Synchronization Study. Cardiovasc. Diagn. Ther. 10 (5), 1293–1302. doi:10.21037/cdt-20-564
Liu, Y., Yang, C., Qi, W., Pei, Z., Xue, W., Zhu, H., et al. (2021). The Impact of ABCB1 and CES1 Polymorphisms on Dabigatran Pharmacokinetics in Healthy Chinese Subjects. Pharmgenomics Pers. Med. 14, 477–485. doi:10.2147/pgpm.s291723
Ning, B., Nowell, S., Sweeney, C., Ambrosone, C. B., Williams, S., Miao, X., et al. (2005). Common Genetic Polymorphisms in the 5′-flanking Region of the SULT1A1 Gene: Haplotypes and Their Association with Platelet Enzymatic Activity. Pharmacogenet Genomics 15 (7), 465–473. doi:10.1097/01.fpc.0000166823.74378.79
O'Donnell, C. J., Larson, M. G., Feng, D., Sutherland, P. A., Lindpaintner, K., Myers, R. H., et al. (2001). Genetic and Environmental Contributions to Platelet Aggregation: the Framingham Heart Study. Circulation 103 (25), 3051–3056. doi:10.1161/01.cir.103.25.3051
Paré, G., Eriksson, N., Lehr, T., Connolly, S., Eikelboom, J., Ezekowitz, M. D., et al. (2013). Genetic Determinants of Dabigatran Plasma Levels and Their Relation to Bleeding. Circulation 127 (13), 1404–1412. doi:10.1161/CIRCULATIONAHA.112.001233
Park, I.-H., Park, J.-W., Chung, H., Kim, J.-M., Lee, S., Kim, K.-A., et al. (2021). Development and Validation of LC-MS/MS Method for Simultaneous Determination of Dabigatran Etexilate and its Active Metabolites in Human Plasma, and its Application in a Pharmacokinetic Study. J. Pharm. Biomed. Anal. 203, 114220. doi:10.1016/j.jpba.2021.114220
Pitera, J. E., Scambler, P. J., and Woolf, A. S. (2008). Fras1, A Basement Membrane-Associated Protein Mutated in Fraser Syndrome, Mediates Both the Initiation of the Mammalian Kidney and the Integrity of Renal Glomeruli. Hum. Mol. Genet. 17 (24), 3953–3964. doi:10.1093/hmg/ddn297
Pruim, R. J., Welch, R. P., Sanna, S., Teslovich, T. M., Chines, P. S., Gliedt, T. P., et al. (2010). LocusZoom: Regional Visualization of Genome-wide Association Scan Results. Bioinformatics 26 (18), 2336–2337. doi:10.1093/bioinformatics/btq419
Raftogianis, R. B., Wood, T. C., Otterness, D. M., Van Loon, J. A., and Weinshilboum, R. M. (1997). Phenol Sulfotransferase Pharmacogenetics in Humans: Association of Common SULT1A1 Alleles with TS PST Phenotype. Biochem. Biophys. Res. Commun. 239 (1), 298–304. doi:10.1006/bbrc.1997.7466
Raymond, J., Imbert, L., Cousin, T., Duflot, T., Varin, R., Wils, J., et al. (2021). Pharmacogenetics of Direct Oral Anticoagulants: A Systematic Review. J. Pers. Med. 11 (1), 37. doi:10.3390/jpm11010037
Reilly, P. A., Lehr, T., Haertter, S., Connolly, S. J., Yusuf, S., Eikelboom, J. W., et al. (2014). The Effect of Dabigatran Plasma Concentrations and Patient Characteristics on the Frequency of Ischemic Stroke and Major Bleeding in Atrial Fibrillation Patients. J. Am. Coll. Cardiol. 63 (4), 321–328. doi:10.1016/j.jacc.2013.07.104
Reyer, H., Oster, M., Wittenburg, D., Murani, E., Ponsuksili, S., and Wimmers, K. (2019). Genetic Contribution to Variation in Blood Calcium, Phosphorus, and Alkaline Phosphatase Activity in Pigs. Front. Genet. 10, 590. doi:10.3389/fgene.2019.00590
Shehab, N., Lovegrove, M. C., Geller, A. I., Rose, K. O., Weidle, N. J., and Budnitz, D. S. (2016). US Emergency Department Visits for Outpatient Adverse Drug Events, 2013-2014. JAMA 316 (20), 2115–2125. doi:10.1001/jama.2016.16201
Shnayder, N. A., Petrova, M. M., Shesternya, P. A., Savinova, A. V., Bochanova, E. N., Zimnitskaya, O. V., et al. (2021). Using Pharmacogenetics of Direct Oral Anticoagulants to Predict Changes in Their Pharmacokinetics and the Risk of Adverse Drug Reactions. Biomedicines 9 (5), 451. doi:10.3390/biomedicines9050451
Stangier, J., and Clemens, A. (2009). Pharmacology, Pharmacokinetics, and Pharmacodynamics of Dabigatran Etexilate, an Oral Direct Thrombin Inhibitor. Clin. Appl. Thromb. Hemost. 15 (Suppl. 1), 9S–16S. doi:10.1177/1076029609343004
Stangier, J., Rathgen, K., Stähle, H., Gansser, D., and Roth, W. (2007). The Pharmacokinetics, Pharmacodynamics and Tolerability of Dabigatran Etexilate, a New Oral Direct Thrombin Inhibitor, in Healthy Male Subjects. Br. J. Clin. Pharmacol. 64 (3), 292–303. doi:10.1111/j.1365-2125.2007.02899.x
Tomita, H., Araki, T., Kadokami, T., Yamada, S., Nakamura, R., Imamura, Y., et al. (2016). Factors Influencing Trough and 90-minute Plasma Dabigatran Etexilate Concentrations Among Patients with Non-valvular Atrial Fibrillation. Thromb. Res. 145, 100–106. doi:10.1016/j.thromres.2016.05.027
Tsume, Y., Mudie, D. M., Langguth, P., Amidon, G. E., and Amidon, G. L. (2014). The Biopharmaceutics Classification System: Subclasses for In Vivo Predictive Dissolution (IPD) Methodology and IVIVC. Eur. J. Pharm. Sci. 57, 152–163. doi:10.1016/j.ejps.2014.01.009
Wieland, E., and Shipkova, M. (2019). Pharmacokinetic and Pharmacodynamic Drug Monitoring of Direct-Acting Oral Anticoagulants: Where Do We Stand? Ther. Drug Monit. 41 (2), 180–191. doi:10.1097/ftd.0000000000000594
Xie, Q., Xiang, Q., Mu, G., Ma, L., Chen, S., Zhou, S., et al. (2018). Effect of ABCB1 Genotypes on the Pharmacokinetics and Clinical Outcomes of New Oral Anticoagulants: A Systematic Review and Meta-Analysis. Curr. Pharm. Des. 24 (30), 3558–3565. doi:10.2174/1381612824666181018153641
Zhan, Q., Huang, R. F., Liang, X. H., Ge, M. X., Jiang, J. W., Lin, H., et al. (2014). FRAS1 Knockdown Reduces A549 Cells Migration and Invasion through Downregulation of FAK Signaling. Int. J. Clin. Exp. Med. 7 (7), 1692–1697.
Zubiaur, P., Saiz-Rodríguez, M., Ochoa, D., Navares-Gómez, M., Mejía, G., Román, M., et al. (2020). Effect of Sex, Use of Pantoprazole and Polymorphisms in SLC22A1, ABCB1, CES1, CYP3A5 and CYP2D6 on the Pharmacokinetics and Safety of Dabigatran. Adv. Ther. 37 (8), 3537–3550. doi:10.1007/s12325-020-01414-x
Keywords: dabigatran, genome-wide association analysis, candidate gene, wholeexome sequencing, pharmacokinetics, pharmacodynamics
Citation: Xie Q, Li Y, Liu Z, Mu G, Zhang H, Zhou S, Wang Z, Wang Z, Jiang J, Li X, Xiang Q and Cui Y (2022) SLC4A4, FRAS1, and SULT1A1 Genetic Variations Associated With Dabigatran Metabolism in a Healthy Chinese Population. Front. Genet. 13:873031. doi: 10.3389/fgene.2022.873031
Received: 10 February 2022; Accepted: 07 April 2022;
Published: 13 May 2022.
Edited by:
Ji-Young Park, Korea University, South KoreaReviewed by:
Guo Chengxian, Central South University, ChinaGeorgia Ragia, Democritus University of Thrace, Greece
Copyright © 2022 Xie, Li, Liu, Mu, Zhang, Zhou, Wang, Wang, Jiang, Li, Xiang and Cui. This is an open-access article distributed under the terms of the Creative Commons Attribution License (CC BY). The use, distribution or reproduction in other forums is permitted, provided the original author(s) and the copyright owner(s) are credited and that the original publication in this journal is cited, in accordance with accepted academic practice. No use, distribution or reproduction is permitted which does not comply with these terms.
*Correspondence: Yimin Cui, Y3VpLnBoYXJtQHBrdWZoLmNvbQ==; Qian Xiang, eGlhbmdxekBwa3VmaC5jb20=; Xin Li, eGluLWxpQGNzc2RzeXkuY29t