- Department of Obstetrics, Chongqing Health Center for Women and Children, Women and Children’s Hospital of Chongqing Medical University, Chongqing, China
Background: Several observational studies have demonstrated that significantly rising circulating adipokine levels are pervasive in preeclampsia or eclampsia disorder (or preeclampsia toxemia (PET)). However, it remains unclear whether this relationship is causal. In this study, we sought to elucidate the causal effects of circulating adipokine levels on PET.
Methods: Summary-level data and independent genetic variants strongly associated with common adipokine molecule (adiponectin, leptin, resistin, sOB-R, and PAI-1) levels were drawn from public genome-wide association study (GWASs). Additionally, the corresponding effects between instrumental variables and PET outcomes were acquired from the FinnGen consortium, including 4,743 cases and 136,325 controls of European ancestry. Subsequently, an inverse-variance weighted (IVW) approach was applied for the principal two-sample Mendelian randomization (MR) and multivariable MR (MVMR) analyses. Various complementary sensitivity analyses were then carried out to determine the robustness of our models.
Results: The results of the IVW method did not reveal any causal relationship shared across genetically predisposed adipokine levels and PET risk (for adiponectin, OR = 0.86, 95% CI: 0.65–1.13, p = 0.274). Additionally, no significant associations were identified after taking into account five circulating adipokines in MVMR research. Complementary sensitivity analysis also supported no significant associations between them. In the reverse MR analysis, genetically predicted PET risk showed a suggestive association with elevating PAI-1 levels by the IVW method (Beta = 0.120, 95% CI: 0.014, 0.227, p = 0.026). Furthermore, there were no strong correlations between genetic liability to PET and other adipokine levels (p > 0.05).
Conclusion: Our MR study did not provide robust evidence supporting the causal role of common circulating adipokine levels in PET, whereas genetically predicted PET may instrumentally affect PAI-1 levels. These findings suggest that PAI-1 may be a useful biomarker for monitoring the diagnosis or therapy of PET rather than a therapeutic target for PET.
Introduction
Preeclampsia or eclampsia, also known as preeclampsia toxemia (PET), is the leading cause of maternal as well as fetal morbidity and mortality among the hypertensive disorders of pregnancy (Rana et al., 2019). Although their incidence varies widely, it is estimated to complicate approximately 4.5% of all pregnancies in the Western world (Abalos et al., 2013). The etiology of PET is probably heterogeneous and not completely clarified, but it is believed that a variety of inflammatory and angiogenic mediators affecting vascular endothelial function and reactivity are attributed to the phenotype of preeclampsia (Bellos et al., 2018). Continued efforts have been made to combat PET; however, there is still a long way to reduce the disease burden of PET. One of the key points is early screening (or diagnosis) based on circulating biomarkers.
It has been well established that adipose-derived adipokines have been assumed to be involved in preeclampsia pathogenesis (Gutaj et al., 2020). As indispensable members of adipokines (a type of immune molecules and inflammatory mediators), common adipokines leptin and resistin have been increasingly studied in the context of risk of PET onset and progression (Masuyama et al., 2010; Daskalakis et al., 2020; Banjac et al., 2021). For example, previous epidemiological studies reported increased serum leptin levels in PET patients and elevated levels of this protein in preeclamptic placentas (Kalinderis et al., 2015; Taylor et al., 2015). Meanwhile, several observational studies indicate that circulating levels of leptin are lower or similar in women with clinical PET than in their normotensive counterparts (Martinez-Abundis et al., 2000; Laml et al., 2001; Doster et al., 2016; Kharb et al., 2017). A recent meta-analysis suggested that patients with PET have a significantly higher leptin level than a group of healthy controls; however, typically only limited research with a small number of patients are available (Shahid et al., 2022).
Conclusive causal relationships remain unestablished on the basis of observational evidence alone due to reverse causation and residual confounding factors, such as incomplete adjustment for confounders, the absence of high-quality evidence, and relatively small sample sizes of trials. Mendelian randomization (MR) is an epidemiological method that uses genetic variants (single nucleotide polymorphisms, SNPs) as instrumental variables (IVs) and could mimic biological effects of clinical biomarkers, and it is less susceptible to the aforementioned shortcomings (Lawlor et al., 2008; Davey Smith and Hemani, 2014). As germline variants in parental genes tend to be randomly distributed to offspring in meiosis during gametogenesis and at conception, MR analysis can eliminate residual confounding from environmental factors and strengthen the causal inference (Burgess et al., 2019). Based on the existing genome-wide association study (GWAS) databank, MR has been widely used to quantitatively assess the effect of circulating adipokine levels on the risk of various diseases, such as cancers, cardiovascular diseases, and auto-inflammatory disorders (Dimou et al., 2021; Harroud et al., 2021; Chen et al., 2022).
However, the potential causal relationship between circulating adipokines and PET has scarcely been explored using the MR approach. Hence, we conducted a bidirectional MR study to test the association of five genetically predicted adipokine biomarkers [adiponectin, leptin, resistin, soluble leptin receptor (sOB-R), and plasminogen activator inhibitor-1 (PAI-1)] with the risk of PET. In addition, we also conducted multivariable MR (MVMR) analysis to determine whether circulating adipokine levels are associated with PET outcomes independently of each other.
Methods
Study design
To allow for an adequate number of SNPs to be included in our MR analysis, we thoroughly relaxed the GWAS p-value threshold of p < 5 × 10–6 for the five adipokine biomarkers. We performed a two-sample MR study using the publicly available summary-level GWAS datasets on adipokines and PET. The validity of MR analysis relies on three critical assumptions: 1) the selected IVs should be closely related to the exposure factor. The IV–exposure strength of genetic instruments was assessed from the F statistic using an approximation (Bowden et al., 2016). If F > 10, there is sufficient strength to avoid a problem of weak instrument bias in the two-sample model (Burgess and Thompson, 2011); 2) each IV must influence the outcome only through exposure factors rather than another pathway; 3) the selected IVs need to be independent of unobserved confounders of the exposure–outcome relationship (Lawlor et al., 2008). Additionally, ethical approval or consent to participate was documented in the original publications. The overview of the study design is presented in Figure 1.
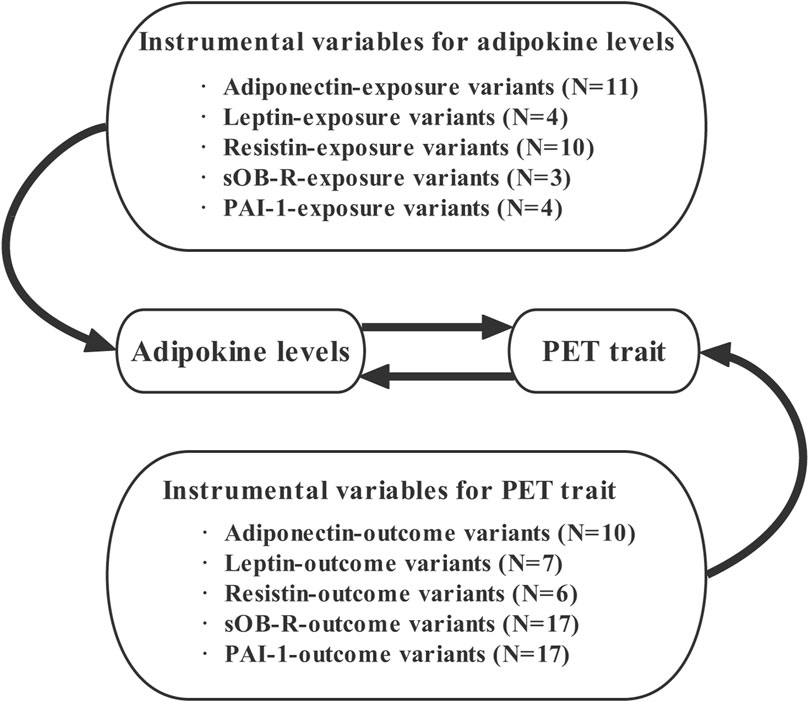
FIGURE 1. Overview of our study design aimed at revealing the bidirectional relationships between circulating adipokines and PET. Abbreviations: N, number of SNPs; PET, preeclampsia or eclampsia.
Data sources
To be more specific, selection of strong adipokine-level estimates of correlations between genetic variants can be obtained from several previously published GWAS datasets of European ancestry (1,338 ≤ N ≤ 39,883) (Dastani et al., 2012; Huang et al., 2012; Kilpelainen et al., 2016; Suhre et al., 2017; Folkersen et al., 2020). A generalized linear mixed model was applied to test for association with genotyped or imputed SNPs that were adjusted for age, sex, and body mass index (BMI). Within each study, circulating adipokine levels were natural logarithm transformed to approximate normal distribution. Further details concerning the relevant genetic information could be acquired from the aforementioned research studies.
Summary data for PET genetic association estimates were extracted from the FinnGen consortium (R6) (https://finngen.gitbook.io/documentation) with 4,743 cases and 136,325 controls of European individuals. Details of population characteristics are available in the original publications and websites. There is no sample overlap between the exposure and outcome datasets. All datasets for the MR analysis are summarized in Table 1. To avoid a possible bias in the estimates of linkage disequilibrium (LD), we attempted to exclude SNPs that met the condition r2 < 0.001. When multiple tagging IVs were in strong LD with each other, only the corresponding IV with the smallest p value was appropriately chosen. Additionally, any specifically requested SNPs that were not available in outcome datasets would be replaced with proxies in LD of r2 > 0.8 or excluded from the MR if no eligible proxies were identified. We also manually searched secondary phenotypes of each selected SNP and its proxy in PhenoScanner V2 (http://www.Phenoscanner.cam.ac.uk/) to rule out the possible influence of pleiotropic effects. The effects of ambiguous SNPs being palindromic with intermediate allele frequencies (where “palindromic SNPs” refer to SNPs with A/T or G/C alleles and “intermediate allele frequencies” refer to 0.01 < allele frequency < 0.30) would be discarded in the subsequent two-sample MR analysis.
Bidirectional Mendelian randomization
To examine the possibility that reverse causality exists in these studies, a bidirectional two-sample MR analysis between genetically predicted PET and circulating adipokines was performed using the same approach as described earlier. Since summary-level data for outcome (sOB-R and PAI-1) were not obtained from the corresponding GWASs, other GWAS summary data for these two adipokines were chosen from the MRC IEU open GWAS platform (https://gwas.mrcieu.ac.uk/); the GWAS IDs corresponding to sOB-R and PAI-1 were “prot-a-1724” and “prot-a-2696,” respectively.
Statistical analysis
The fixed-effects inverse-variance weighted (IVW) meta-analysis based on the Wald ratio method was applied to explore two-sample MR estimates of the associations between the levels of the five adipokines and PET(28). The IVW method, including all valid IVs, would provide the most accurate assessments. We further applied MVMR (Burgess and Thompson, 2015) to dissect the influence of potential adipokine levels on causal estimates. Additionally, a series of complementary sensitivity analyses, such as maximum likelihood, MR-Egger regression, weighted median, and MR-robust adjusted profile score (MR-RAPS) methods, were introduced to produce a consistent causal estimate (Pierce and Burgess, 2013; Bowden et al., 2015; Hartwig et al., 2016; Zhao et al., 2019). Moreover, estimating an intercept with p < 0.05 in MR-Egger regression is akin to allowing for the possibility of additional horizontal pleiotropy. MR-pleiotropy residual sum and outlier (MR-PRESSO) test was applied to detect potential outlying SNPs and provide causal estimates after the removal of outliers (Verbanck et al., 2018). We also applied radial IVW for a better visualization of the regression estimates. The statistical heterogeneity among the estimates of IVs in the IVW method was assessed using Cochran’s Q test, and p values less than 0.05 were considered statistically significant. To address multiple testing, a Bonferroni-corrected p value of 0.005 (0.05/10 adipokines) was considered significant, with a p value < 0.005 or < 0.05 was regarded as suggestive. Furthermore, a “leave-one-out” sensitivity analysis was performed to determine the potential effect of SNPs on the causal estimates. Power calculations were performed based on a web tool available at https://sb452.shinyapps.io/power.
All these analyses were implemented in the R software (version 4.1.0, using the “TwoSampleMR,” “RadialMR,” and “MR-PRESSO” R packages; R Foundation for Statistical Computing, Vienna, Austria).
Results
A detailed description of the selected SNPs, including effect allele (EA), EA frequency, and effect sizes on adipokines and PET, was thoroughly retrieved from the original literatures. All selected SNPs did not have a potent LD calculation (r2 < 0.001). For genetically predicted exposure level, power calculations for our bidirectional two-sample MR analyses were performed. All statistical power calculations for our MR estimates were less than 80%, except for the effect of PAI-1 level on PET (Table 1).
Circulating adipokine levels and PET
Summary information about the selected SNPs for the five adipokine levels is available in Supplementary Table S2. In this study, 6 SNPs (rs601339, rs7133378, rs13081028, rs10773049, rs825453, and rs8182584) for adiponectin level, five SNPs (rs199752470, rs34861192, rs7746716, rs4134826, and rs3745367) for resistin level, and 1 SNP (rs17412403) for sOB-R level were removed due to high LD with other variants or absence from the LD reference panel. Additionally, we eliminated 1 SNP (rs10487505 related to leptin) for being palindromic with intermediate allele frequencies. Thus, the remaining 11 SNPs regarded as IVs for adiponectin level, four SNPs for leptin level, 10 SNPs for resistin level, three SNPs for sOB-R level, and four SNPs for PAI-1 level were included in further MR analysis. The F statistics of all selected SNPs ranged from 18 to 225, demonstrating that there is no bias due to weak instruments. Moreover, the variance explained in circulating adipokine levels by the genetic instruments was 6.04%, 1.69%, 4.67%, 9.06%, and 1.87% for adiponectin, leptin, resistin, sOB-R, and PAI-1 levels, respectively.
As shown in Table 2, genetically predicted levels of circulating adipokines were not associated with the risk of PET (adiponectin, OR = 0.86, 95% CI: 0.65–1.13, p = 0.274; leptin, OR = 0.87, 95% CI: 0.41–1.86, p = 0.72; resistin, OR = 1.13, 95% CI: 0.94–1.35, p = 0.199; sOB-R, OR = 1.01, 95% CI: 0.97–1.05, p = 0.599; and PAI-1, OR = 1.36, 95% CI: 0.71–2.62, p = 0.354, Figure 2) using the IVW method. Moreover, such findings were undeviating from several complementary MR analyses (p > 0.05). When five circulating adipokines were included together in the MVMR analysis (Supplementary Table S3), all its estimations with PET hardly surpassed the Bonferroni-corrected threshold (p > 0.05). Cochran’s Q statistics showed evidence of slight heterogeneity based on genetically predicted SNPs of PAI-1 level (p = 0.032), and the associations for PAI-1 were mainly driven by rs6976035 located near the ACHE region in sensitivity analyses. The results of the leave-one-out method demonstrated that the links between other adipokines and PET risk were not driven by SNPs, which indicated that MR results were reliable (Supplementary Figure S1). Moreover, the MR-Egger intercept test detected no evidence of directional pleiotropy (p > 0.05). No outlier SNPs were observed in the MR-PRESSO analysis (data not shown). Additionally, the IVW Radial MR results delineated that no instrumental variables of adipokines that carry large effect sizes on PET could potentially be outliers.
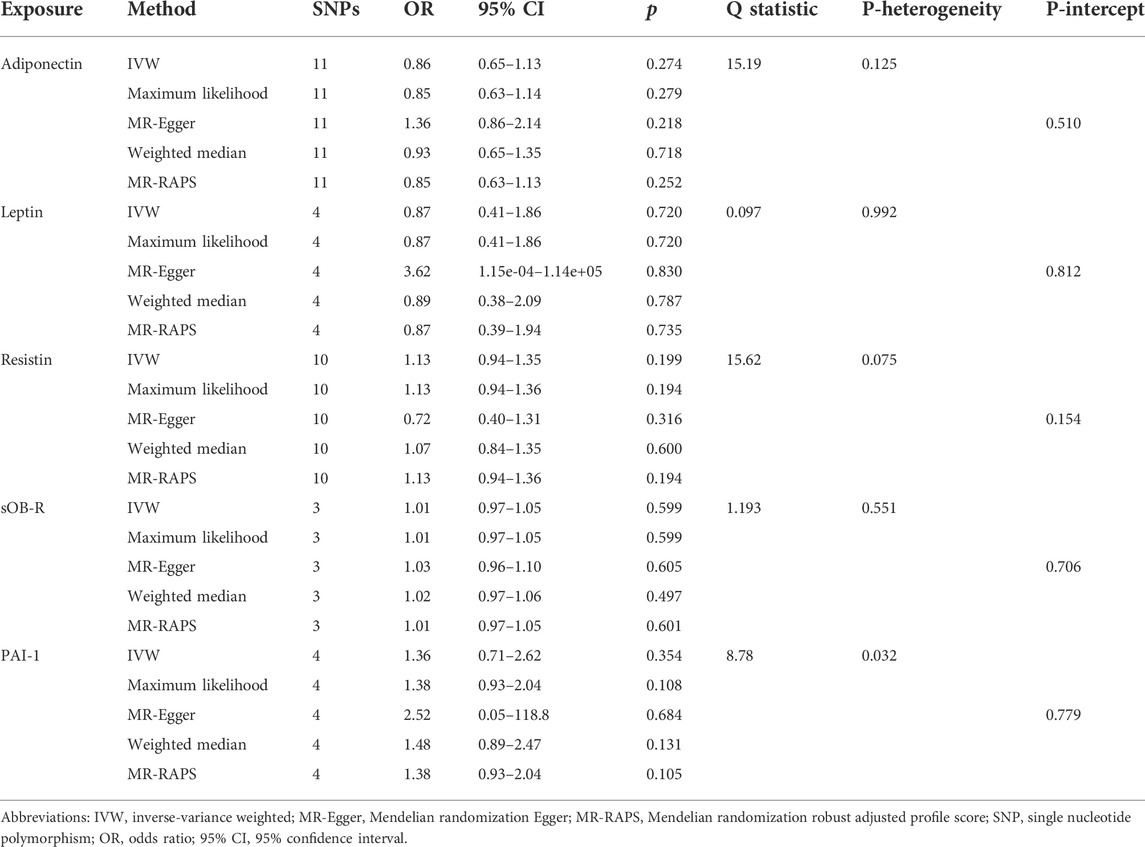
TABLE 2. Two-sample MR estimates of associations between genetically predicted circulating adipokine levels and PET.
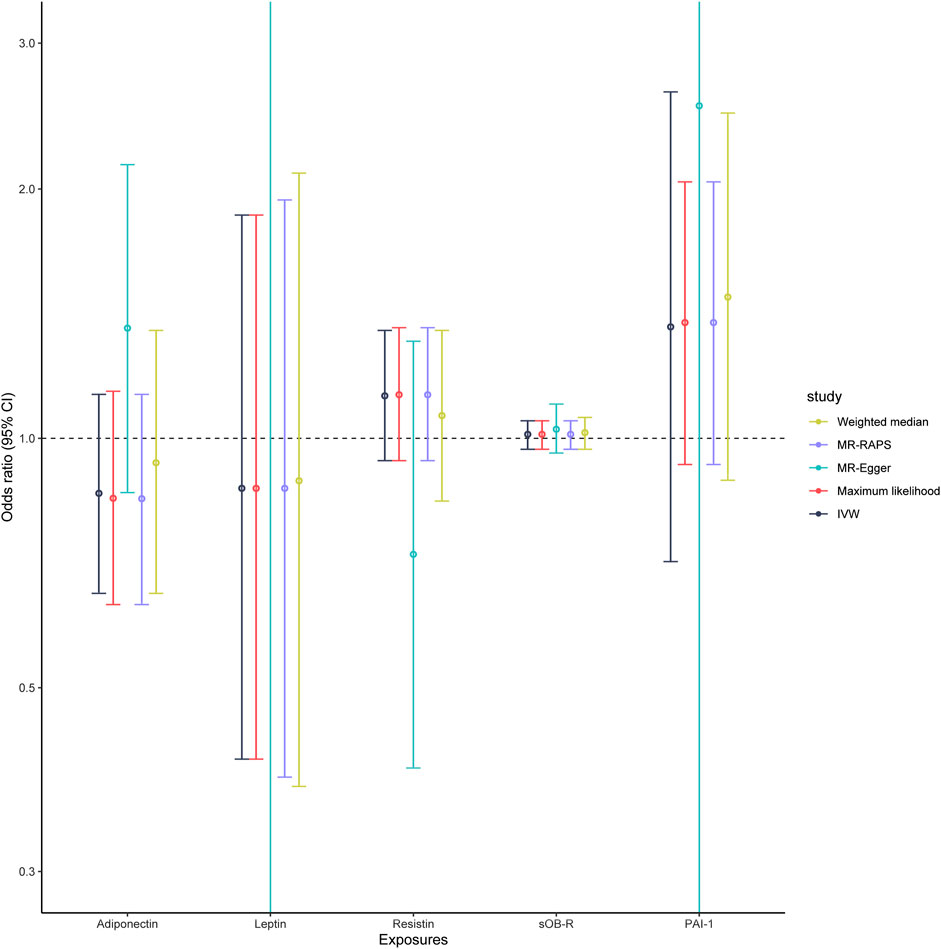
FIGURE 2. Associations of genetically predicted circulating adipokine levels with PET according to different Mendelian randomization methods. Abbreviations: IVW, inverse-variance weighted; MR-Egger, Mendelian randomization Egger; MR-RAPS, Mendelian randomization robust adjusted profile score; OR, odds ratio; 95% CI, 95% confidence interval.
A few genetic variants (rs998584 for adiponectin, rs780093 for leptin, rs3087852 for resistin, rs4655537 for sOB-R, and rs11128603 for PAI-1) were associated with other phenotypes at the threshold of genome-wide significance (p < 5 × 10–8), including different white blood cells, BMI, lipid levels, coronary artery disease, and age at menopause (Supplementary Table S4). Remarkably, all these traits were unlikely to exert any pleiotropic effect on the observed associations between genetically predicted adipokine levels and PET risk.
PET and circulating adipokine levels
To further examine the influence of PET on adipokine concentrations, we conducted another reverse MR design regarding genetical PET trait as exposure and circulating adipokines as outcome. A total of 11 SNPs that were strongly related to PET risk (p < 5 × 10–6) were identified in our MR findings (Supplementary Table S5). The MR results indicated that there was a suggestive positive link between genetic liability to PET and circulating PAI-1 levels using the IVW, maximum likelihood, MR-Egger, and MR-RAPS methods (IVW, Beta = 0.120, 95% CI: 0.014, 0.227, p = 0.026, Figure 3 and Table 3). In addition, null estimates were obtained for the causal effects of PET on other circulating adipokine levels. The leave-one-out analysis also did not demonstrate any evidence that an SNP could drive the overall effect of genetical PET variants on adiponectin levels (Supplementary Figure S2). Similarly, neither indication of a significant intercept for horizontal pleiotropy (intercept = –0.02, p = 0.281) nor strong heterogeneity (Cochran’s Q = 13.32, p = 0.629) was detected in these MR analyses (Table 3).
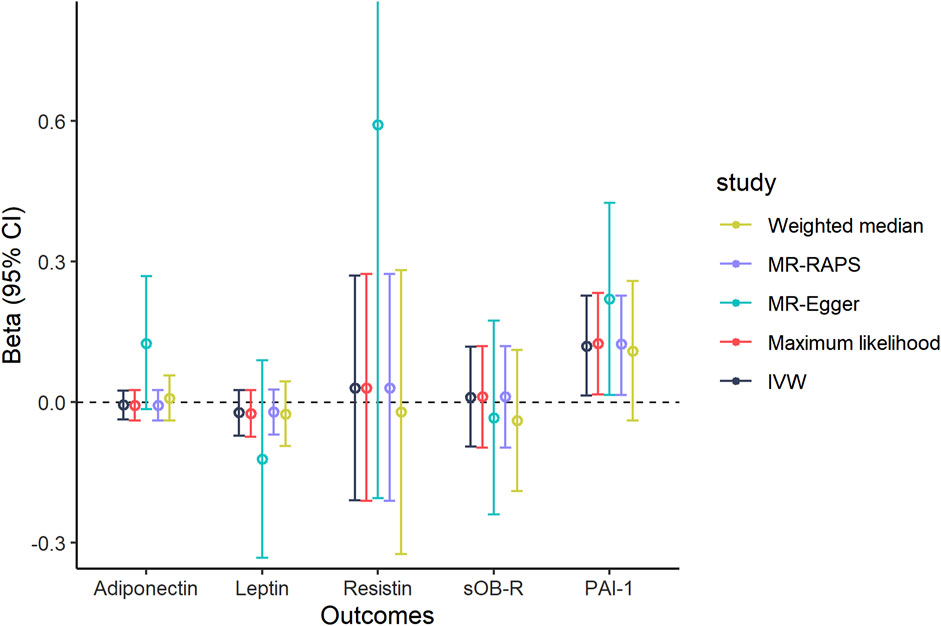
FIGURE 3. Causal association of genetically predicted PET and circulating adipokine levels according to different Mendelian randomization methods. Abbreviations: IVW, inverse-variance weighted; MR-Egger, Mendelian randomization Egger; MR-RAPS, Mendelian randomization robust adjusted profile score; 95% CI, 95% confidence interval.
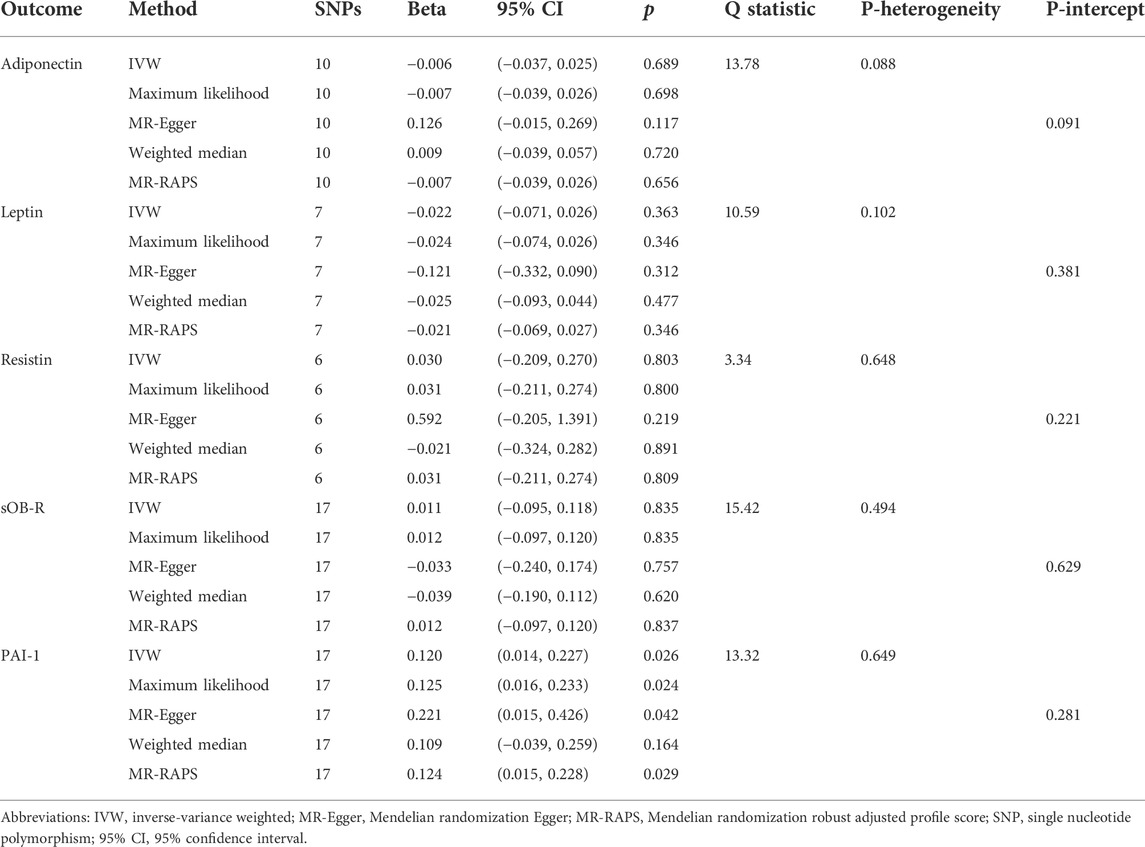
TABLE 3. Two-sample MR estimates of associations between genetically predicted PET and circulating adipokine levels.
Discussion
To the best of our knowledge, this is the first MR analysis evaluating whether five adipokine levels are related to the risk of PET based on the genetic data from large-scale GWAS datasets. The current MR estimates did not support a causal effect of genetically determined circulating adipokines on PET risk. Moreover, reverse MR analyses indicated that genetic predisposition to PET was possibly associated with the level of circulating PAI-1.
Our findings on the role of circulating adipokine levels in the development of PET seem quite inconsistent across observational studies and interventional trials. Compared to healthy controls, several case–control researches have demonstrated elevated levels of PAI-1 in patients with early-onset PET (Chappell et al., 2002; Wikstrom et al., 2009; Bodova et al., 2011; Rampersaud et al., 2020). A positive association has been confirmed between maternal PAI-1 mRNA expression and the severity of PET in the third trimester, and a linear regression model has evaluated that severe PET is remarkably associated with PAI-1 level (Purwosunu et al., 2007; Elzein et al., 2016). In addition, some researchers argued that there was no difference in PAI-1 expressions between PET individuals and healthy pregnant women (Gow et al., 1984; Ho and Yang, 1992). A previous meta-analysis also did not delineate any significant relationship between the occurrence of PE and PAI-1 4G/5G polymorphism (Morgan et al., 2013). Although accumulating evidences show that increased PAI-1 levels play a pivotal role in PET phenotype, information about the function of PAI-1 during pregnancy complicated with PET are still controversial. Our bidirectional MR analysis has shown the shared genetic architecture between PAI-1 levels and PET and found suggestive evidence of causal relationships between them.
Even though we found no indication of potential causality between other genetically predicted adipokine levels and PET risk, such findings are contradictory with previous observational studies. For adiponectin, multiple researchers confirmed that a significant increase was mapped in adiponectin concentration among PET patients compared to controls (Naruse et al., 2005; Takemura et al., 2007). In contrast, several comparative studies have demonstrated significantly lower levels of adiponectin in PET patients (Ouyang et al., 2007; Herse et al., 2009). Some researchers proved that the involvement of adiponectin in PE pathology was attributed to its function in endothelial nitric oxide synthase activation and nitric oxide synthesis, and both could result in the reduction of blood pressure (Sandrim et al., 2008). With regard to resistin, alterations in its concentration level in PET patients are still poorly understood. Some of the studies concerning PET have concluded that maternal resistin concentrations might be elevated (Seol et al., 2010), some observed reduced resistin level (Christiansen et al., 2015), and others also revealed no significant correlation between PET and non-complicated pregnancies (Hendler et al., 2005). The discrepancy between our MR findings and previous observational studies may partly be due to measurement error, small sample size, and residual confounding. Indeed, further research is required to elucidate the internal association of adiponectin, as well as other adipokines with robust null results in our study, with PET and to identify whether there is potential bias or confounding accounted for previous studies.
Several potential mechanisms of increased PAI-1 synthesis in the pathogenesis of PET and their association have been comprehensively discussed. It has been reported that the hypoinvasion and failed conversion of maternal endometrial spiral arteries in the placenta of patients with PET are related to the increased level of PAI-1 (Redman and Sargent, 2005). Additionally, PET has been commonly recognized as an inflammation-mediated disorder, and inflammatory cytokines, including interleukin 1β (IL-1β), IL-6, vascular endothelial growth factor, and epidermal growth factor, could upregulate the level of circulating PAI-1 (Zhai et al., 2022). Moreover, it has been found that the NF-κB pathway activation in the damaged migration of trophoblasts in PET could ultimately promote the mRNA expression of PAI-1 (Ye et al., 2017). Although the fact whether an increased level of PAI-1 is the leading cause of PET or the meaningful consequence of endothelial and placental dysfunction remains undetermined, our reverse MR results partly supported that upregulations of circulating adipokines involved in PAI-1 level appear to be downstream effects of PET.
As far as we know, there are no related MR analyses that have explored the bidirectional association of circulating adipokine levels with the risk of PET. A recent MR research revealed the causal role of resistin in the development of cardiovascular disease, which probably acted through blood pressure (Chen et al., 2022). Another MR study provided evidence for a potential causal link between leptin level and blood pressure among smokers (Shen et al., 2020). In our MR study, we incorporated the largest and most comprehensive datasets to investigate a causal association between genetically determined circulating adipokine concentrations and risks of PET. In addition, we performed various sensitivity analyses and investigated potential associations with secondary phenotypes of interest. Our results were similar and robust, suggesting that pleiotropy did not markedly influence our findings. However, our present findings cannot rule out the possibility of a protective or detrimental role for adipokines in PET etiology, which suggests that the function of adipokines in the causal pathway of this disease is likely to be small.
The present MR design was introduced primarily to avoid the bias of observational studies on the association of genetically predicted adipokine levels with PET risk in large-scale individuals. Several important advantages deserve special emphasis. First, a predominant strength of the bidirectional MR method is benefited to reduce bias from unmeasured residual confounding, reverse causality, and measurement error. Second, the most comprehensive GWAS-identified SNPs were regarded as the IV model. In addition, all selected IVs (F > 10) were robustly associated with the exposure factor, suggesting that there were no evidence of weak estimate. Third, several sensitivity analyses and potential pleiotropic validation imply the decreased probability of bias. Thus, our findings provide strong evidence in support of the genetic assessment between adipokine levels and PET susceptibility.
Notwithstanding the explicit strengths, some limitations in our study should be noted. First, the statistical power might be low for several analyses due to limited case numbers. Thus, the inadequate power might be the reason for the null results. Second, the information for our research was obtained from publicly available GWASs’ summary-level datasets. However, detailed demographic characteristics and clinical manifestations (such as smoking status and PET progress) of subjects were not available to perform risk-stratified and conventional subgroup analysis. Third, our population confinement to European descent could help minimize the impact of ethnic structure bias; however, our findings might not generalize to other populations. Fourth, it is difficult for us to make a distinction between preeclampsia and eclampsia in our MR analyses. Fifth, we cannot completely rule out the relationship of sOB-R level with PET risk, since this might be due to the relatively small sample size. Sixth, our research did not acquire enough SNPs regarding plasma adipokine levels as IVs, resulting in only a limited proportion of variation to be explained and no adequate power to reach a significant threshold. Additionally, we acknowledged that MR methods used in our research cannot address commonly seen pleiotropy and sample structure problems in such causal inference studies. Genome-wide MR methods such as Causal Analysis Using Summary Effect Estimates (Morrison et al., 2020) and constrained maximum likelihood and model averaging (Xue et al., 2021) using weak IVs may relieve the concern for possible false-positive causal findings. Seventh, the effects of PET exposure on five plasma adipokine biomarkers could vary by sex difference, but sufficiently large unbiased female samples for adipokine outcomes are not available to test this possibility. Finally, epigenetic phenomena that were independent of MR design, for example, methylation, acetylation, or potential mechanism of developmental compensation, also could affect the association of adipokine levels with PET risk.
Conclusion
Our bidirectional MR study did not support a critical role for increased circulating adipokine levels in PET but identified a potential causality of PET risk and circulating PAI-1 levels, suggesting that PAI-1 may be a potential biomarker for the diagnosis or therapy of PET. Subsequent studies with updated data from large GWAS datasets are still required to authenticate the mentioned findings.
Data availability statement
The original contributions presented in the study are included in the article/Supplementary Material; further inquiries can be directed to the corresponding author.
Ethics statement
Ethical approval for this study was not required as our analyses were based on summary statistics from published GWAS or the data were publicly accessible and no individual-level data were used.
Author contributions
XC and ZL drafted the manuscript. JC, XC, and JX analyzed the data. XC and WZ designed the study. WZ obtained the funding. All authors contributed to data analysis, drafting or revising the manuscript, gave final approval of the version to be published, and agreed to be accountable for all aspects of the work.
Funding
This work was supported by the High-level Medical Reserved Personnel Training Project of Chongqing and Chongqing Science and Health Joint Medical Research Project (2019GDRC013).
Acknowledgments
The authors thank the contributions of the FinnGen Consortium, United Kingdom Biobank, and the MRC IEU Open GWAS Project in providing high-quality GWAS data for researchers.
Conflict of interest
The authors declare that the research was conducted in the absence of any commercial or financial relationships that could be construed as a potential conflict of interest.
Publisher’s note
All claims expressed in this article are solely those of the authors and do not necessarily represent those of their affiliated organizations, or those of the publisher, the editors, and the reviewers. Any product that may be evaluated in this article, or claim that may be made by its manufacturer, is not guaranteed or endorsed by the publisher.
Supplementary material
The Supplementary Material for this article can be found online at: https://www.frontiersin.org/articles/10.3389/fgene.2022.935757/full#supplementary-material
References
Abalos, E., Cuesta, C., Grosso, A. L., Chou, D., and Say, L. (2013). Global and regional estimates of preeclampsia and eclampsia: A systematic review. Eur. J. Obstet. Gynecol. Reprod. Biol. 170 (1), 1–7. Epub 2013/06/12. doi:10.1016/j.ejogrb.2013.05.005
Banjac, G., Ardalic, D., Mihajlovic, M., Antonic, T., Cabunac, P., Zeljkovic, A., et al. (2021). The role of resistin in early preeclampsia prediction. Scand. J. Clin. Lab. Invest. 81 (6), 432–437. Epub 2021/06/16. doi:10.1080/00365513.2021.1938205
Bellos, I., Karageorgiou, V., Kapnias, D., Karamanli, K. E., and Siristatidis, C. (2018). The role of interleukins in preeclampsia: A comprehensive review. Am. J. Reprod. Immunol. 80 (6), e13055. Epub 2018/09/29. doi:10.1111/aji.13055
Bodova, K. B., Biringer, K., Dokus, K., Ivankova, J., Stasko, J., and Danko, J. (2011). Fibronectin, plasminogen activator inhibitor type 1 (PAI-1) and uterine artery Doppler velocimetry as markers of preeclampsia. Dis. Markers 30 (4), 191–196. Epub 2011/06/23. doi:10.3233/DMA-2011-0772
Bowden, J., Davey Smith, G., and Burgess, S. (2015). Mendelian randomization with invalid instruments: Effect estimation and bias detection through egger regression. Int. J. Epidemiol. 44 (2), 512–525. Epub 2015/06/08. doi:10.1093/ije/dyv080
Bowden, J., Del Greco, M. F., Minelli, C., Davey Smith, G., Sheehan, N. A., and Thompson, J. R. (2016). Assessing the suitability of summary data for two-sample mendelian randomization analyses using MR-egger regression: The role of the I2 statistic. Int. J. Epidemiol. 45 (6), 1961–1974. Epub 2016/09/13. doi:10.1093/ije/dyw220
Burgess, S., Davey Smith, G., Davies, N. M., Dudbridge, F., Gill, D., Glymour, M. M., et al. (2019). Guidelines for performing Mendelian randomization investigations. Wellcome Open Res. 4, 186. Epub 2020/08/11. doi:10.12688/wellcomeopenres.15555.2
Burgess, S., and Thompson, S. G. (2011). Bias in causal estimates from Mendelian randomization studies with weak instruments. Stat. Med. 30 (11), 1312–1323. Epub 2011/03/25. doi:10.1002/sim.4197
Burgess, S., and Thompson, S. G. (2015). Multivariable mendelian randomization: The use of pleiotropic genetic variants to estimate causal effects. Am. J. Epidemiol. 181 (4), 251–260. Epub 2015/01/30. doi:10.1093/aje/kwu283
Chappell, L. C., Seed, P. T., Briley, A., Kelly, F. J., Hunt, B. J., Charnock-Jones, D. S., et al. (2002). A longitudinal study of biochemical variables in women at risk of preeclampsia. Am. J. Obstet. Gynecol. 187 (1), 127–136. Epub 2002/07/13. doi:10.1067/mob.2002.122969
Chen, D., Zhang, Y., Yidilisi, A., Xu, Y., Dong, Q., and Jiang, J. (2022). Causal associations between circulating adipokines and cardiovascular disease: A mendelian randomization study. J. Clin. Endocrinol. Metab. 107, e2572–e2580. Epub 2022/02/09. doi:10.1210/clinem/dgac048
Christiansen, M., Hedley, P. L., Placing, S., Wojdemann, K. R., Carlsen, A. L., Jorgensen, J. M., et al. (2015). Maternal serum resistin is reduced in first trimester preeclampsia pregnancies and is a marker of clinical severity. Hypertens. Pregnancy 34 (4), 422–433. Epub 2015/12/05. doi:10.3109/10641955.2014.913615
Daskalakis, G., Bellos, I., Nikolakea, M., Pergialiotis, V., Papapanagiotou, A., and Loutradis, D. (2020). The role of serum adipokine levels in preeclampsia: A systematic review. Metabolism. 106, 154172. Epub 2020/02/07. doi:10.1016/j.metabol.2020.154172
Dastani, Z., Hivert, M. F., Timpson, N., Perry, J. R., Yuan, X., Scott, R. A., et al. (2012). Novel loci for adiponectin levels and their influence on type 2 diabetes and metabolic traits: A multi-ethnic meta-analysis of 45, 891 individuals. PLoS Genet. 8 (3), e1002607. Epub 2012/04/06. doi:10.1371/journal.pgen.1002607
Davey Smith, G., and Hemani, G. (2014). Mendelian randomization: Genetic anchors for causal inference in epidemiological studies. Hum. Mol. Genet. 23 (R1), R89–R98. Epub 2014/07/30. doi:10.1093/hmg/ddu328
Dimou, N. L., Papadimitriou, N., Mariosa, D., Johansson, M., Brennan, P., Peters, U., et al. (2021). Circulating adipokine concentrations and risk of five obesity-related cancers: A mendelian randomization study. Int. J. Cancer 148 (7), 1625–1636. Epub 2020/10/11. doi:10.1002/ijc.33338
Doster, Y., Cetinkaya Demir, B., Atalay, M. A., Durusoy, E. E., and Kucukkomurcu, S. (2016). The possible role of serum leptin in preeclampsia. Clin. Exp. Obstet. Gynecol. 43 (1), 98–102. Epub 2016/04/07. doi:10.12891/ceog2039.2016
Elzein, H. O., Muddathir, A. R., Rida, M., Rayis, D. A., Elhassan, E. M., and Adam, I. (2016). Fibrinolysis parameters in Sudanese women with severe preeclampsia. Hypertens. Pregnancy 35 (4), 559–564. Epub 2016/11/03. doi:10.1080/10641955.2016.1211676
Folkersen, L., Gustafsson, S., Wang, Q., Hansen, D. H., Hedman, A. K., Schork, A., et al. (2020). Genomic and drug target evaluation of 90 cardiovascular proteins in 30, 931 individuals. Nat. Metab. 2 (10), 1135–1148. Epub 2020/10/18. doi:10.1038/s42255-020-00287-2
Gow, L., Campbell, D. M., and Ogston, D. (1984). The fibrinolytic system in pre-eclampsia. J. Clin. Pathol. 37 (1), 56–58. Epub 1984/01/01. doi:10.1136/jcp.37.1.56
Gutaj, P., Sibiak, R., Jankowski, M., Awdi, K., Bryl, R., Mozdziak, P., et al. (2020). The role of the adipokines in the most common gestational complications. Int. J. Mol. Sci. 21 (24), E9408. Epub 2020/12/17. doi:10.3390/ijms21249408
Harroud, A., Manousaki, D., Butler-Laporte, G., Mitchell, R. E., Davey Smith, G., Richards, J. B., et al. (2021). The relative contributions of obesity, vitamin D, leptin, and adiponectin to multiple sclerosis risk: A mendelian randomization mediation analysis. Mult. Scler. 27 (13), 1994–2000. Epub 2021/02/20. doi:10.1177/1352458521995484
Hartwig, F. P., Davies, N. M., Hemani, G., and Davey Smith, G. (2016). Two-sample mendelian randomization: Avoiding the downsides of a powerful, widely applicable but potentially fallible technique. Int. J. Epidemiol. 45 (6), 1717–1726. Epub 2017/03/25. doi:10.1093/ije/dyx028
Hendler, I., Blackwell, S. C., Mehta, S. H., Whitty, J. E., Russell, E., Sorokin, Y., et al. (2005). The levels of leptin, adiponectin, and resistin in normal weight, overweight, and obese pregnant women with and without preeclampsia. Am. J. Obstet. Gynecol. 193 , 979–983. Epub 2005/09/15. doi:10.1016/j.ajog.2005.06.041
Herse, F., Bai, Y., Staff, A. C., Yong-Meid, J., Dechend, R., and Zhou, R. (2009). Circulating and uteroplacental adipocytokine concentrations in preeclampsia. Reprod. Sci. 16 (6), 584–590. Epub 2009/03/12. doi:10.1177/1933719109332828
Ho, C. H., and Yang, Z. L. (1992). The predictive value of the hemostasis parameters in the development of preeclampsia. Thromb. Haemost. 67 (2), 214–218. Epub 1992/02/03. doi:10.1055/s-0038-1648415
Huang, J., Sabater-Lleal, M., Asselbergs, F. W., Tregouet, D., Shin, S. Y., Ding, J., et al. (2012). Genome-wide association study for circulating levels of PAI-1 provides novel insights into its regulation. Blood 120 (24), 4873–4881. Epub 2012/09/20. doi:10.1182/blood-2012-06-436188
Kalinderis, M., Papanikolaou, A., Kalinderi, K., Vyzantiadis, T. A., Ioakimidou, A., and Tarlatzis, B. C. (2015). Serum levels of leptin and IP-10 in preeclampsia compared to controls. Arch. Gynecol. Obstet. 292 (2), 343–347. Epub 2015/02/20. doi:10.1007/s00404-015-3659-4
Kharb, S., Bala, J., and Nanda, S. (2017). Markers of obesity and growth in preeclamptic and normotensive pregnant women. J. Obstet. Gynaecol. 37 (5), 610–615. Epub 2017/05/04. doi:10.1080/01443615.2017.1286463
Kilpelainen, T. O., Carli, J. F., Skowronski, A. A., Sun, Q., Kriebel, J., Feitosa, M. F., et al. (2016). Genome-wide meta-analysis uncovers novel loci influencing circulating leptin levels. Nat. Commun. 7, 10494. Epub 2016/02/03. doi:10.1038/ncomms10494
Laml, T., Preyer, O., Hartmann, B. W., Ruecklinger, E., Soeregi, G., and Wagenbichler, P. (2001). Decreased maternal serum leptin in pregnancies complicated by preeclampsia. J. Soc. Gynecol. Investig. 8 (2), 89–93. Epub 2001/05/05. doi:10.1016/s1071-5576(01)00090-9
Lawlor, D. A., Harbord, R. M., Sterne, J. A., Timpson, N., and Davey Smith, G. (2008). Mendelian randomization: Using genes as instruments for making causal inferences in epidemiology. Stat. Med. 27 (8), 1133–1163. Epub 2007/09/22. doi:10.1002/sim.3034
Martinez-Abundis, E., Gonzalez-Ortiz, M., and Pascoe-Gonzalez, S. (2000). Serum leptin levels and the severity of preeclampsia. Arch. Gynecol. Obstet. 264 (2), 71–73. Epub 2000/10/25. doi:10.1007/s004040000081
Masuyama, H., Segawa, T., Sumida, Y., Masumoto, A., Inoue, S., Akahori, Y., et al. (2010). Different profiles of circulating angiogenic factors and adipocytokines between early- and late-onset pre-eclampsia. BJOG 117 (3), 314–320. Epub 2009/12/18. doi:10.1111/j.1471-0528.2009.02453.x
Morgan, J. A., Bombell, S., and McGuire, W. (2013). Association of plasminogen activator inhibitor-type 1 (-675 4G/5G) polymorphism with pre-eclampsia: Systematic review. PLoS One 8 (2), e56907. Epub 2013/03/05. doi:10.1371/journal.pone.0056907
Morrison, J., Knoblauch, N., Marcus, J. H., Stephens, M., and He, X. (2020). Mendelian randomization accounting for correlated and uncorrelated pleiotropic effects using genome-wide summary statistics. Nat. Genet. 52 (7), 740–747. Epub 2020/05/27. doi:10.1038/s41588-020-0631-4
Naruse, K., Yamasaki, M., Umekage, H., Sado, T., Sakamoto, Y., and Morikawa, H. (2005). Peripheral blood concentrations of adiponectin, an adipocyte-specific plasma protein, in normal pregnancy and preeclampsia. J. Reprod. Immunol. 65 (1), 65–75. Epub 2005/02/08. doi:10.1016/j.jri.2004.09.004
Ouyang, Y., Chen, H., and Chen, H. (2007). Reduced plasma adiponectin and elevated leptin in pre-eclampsia. Int. J. Gynaecol. Obstet. 98 (2), 110–114. Epub 2007/06/26. doi:10.1016/j.ijgo.2007.04.021
Pierce, B. L., and Burgess, S. (2013). Efficient design for mendelian randomization studies: Subsample and 2-sample instrumental variable estimators. Am. J. Epidemiol. 178 (7), 1177–1184. Epub 2013/07/19. doi:10.1093/aje/kwt084
Purwosunu, Y., Sekizawa, A., Koide, K., Farina, A., Wibowo, N., Wiknjosastro, G. H., et al. (2007). Cell-free mRNA concentrations of plasminogen activator inhibitor-1 and tissue-type plasminogen activator are increased in the plasma of pregnant women with preeclampsia. Clin. Chem. 53 (3), 399–404. Epub 2007/01/20. doi:10.1373/clinchem.2006.081372
Rampersaud, A. M., Dunk, C. E., Lye, S. J., and Renaud, S. J. (2020). Palmitic acid induces inflammation in placental trophoblasts and impairs their migration toward smooth muscle cells through plasminogen activator inhibitor-1. Mol. Hum. Reprod. 26 (11), 850–865. Epub 2020/09/09. doi:10.1093/molehr/gaaa061
Rana, S., Lemoine, E., Granger, J. P., and Karumanchi, S. A. (2019). Preeclampsia: Pathophysiology, challenges, and perspectives. Circ. Res. 124 (7), 1094–1112. Epub 2019/03/29. doi:10.1161/CIRCRESAHA.118.313276
Redman, C. W., and Sargent, I. L. (2005). Latest advances in understanding preeclampsia. Science 308 (5728), 1592–1594. Epub 2005/06/11. doi:10.1126/science.1111726
Sandrim, V. C., Palei, A. C., Metzger, I. F., Gomes, V. A., Cavalli, R. C., and Tanus-Santos, J. E. (2008). Nitric oxide formation is inversely related to serum levels of antiangiogenic factors soluble fms-like tyrosine kinase-1 and soluble endogline in preeclampsia. Hypertension 52 (2), 402–407. Epub 2008/06/25. doi:10.1161/HYPERTENSIONAHA.108.115006
Seol, H. J., Oh, M. J., Yeo, M. K., Kim, A., Lee, E. S., and Kim, H. J. (2010). Comparison of serum levels and the placental expression of resistin between patients with preeclampsia and normal pregnant women. Hypertens. Pregnancy 29 (3), 310–317. Epub 2010/07/31. doi:10.3109/10641950902849850
Shahid, R., Bari, M. F., and Hussain, M. (2022). Serum biomarkers for the prediction and diagnosis of preeclampsia: A meta-analysis. J. Taibah Univ. Med. Sci. 17 (1), 14–27. Epub 2022/02/11. doi:10.1016/j.jtumed.2021.07.003
Shen, L., Cordero, J. F., Wang, J. S., Shen, Y., Zhang, R., Qi, Y., et al. (2020). The effect of leptin on blood pressure considering smoking status: A mendelian randomization study. Hypertens. Res. 43 (4), 342–349. Epub 2019/12/14. doi:10.1038/s41440-019-0376-6
Suhre, K., Arnold, M., Bhagwat, A. M., Cotton, R. J., Engelke, R., Raffler, J., et al. (2017). Connecting genetic risk to disease end points through the human blood plasma proteome. Nat. Commun. 8, 14357. Epub 2017/02/28. doi:10.1038/ncomms14357
Takemura, Y., Osuga, Y., Koga, K., Tajima, T., Hirota, Y., Hirata, T., et al. (2007). Selective increase in high molecular weight adiponectin concentration in serum of women with preeclampsia. J. Reprod. Immunol. 73 (1), 60–65. Epub 2006/07/25. doi:10.1016/j.jri.2006.05.004
Taylor, B. D., Ness, R. B., Olsen, J., Hougaard, D. M., Skogstrand, K., Roberts, J. M., et al. (2015). Serum leptin measured in early pregnancy is higher in women with preeclampsia compared with normotensive pregnant women. Hypertension 65 (3), 594–599. Epub 2014/12/17. doi:10.1161/HYPERTENSIONAHA.114.03979
Verbanck, M., Chen, C. Y., Neale, B., and Do, R. (2018). Detection of widespread horizontal pleiotropy in causal relationships inferred from Mendelian randomization between complex traits and diseases. Nat. Genet. 50 (5), 693–698. Epub 2018/04/25. doi:10.1038/s41588-018-0099-7
Wikstrom, A. K., Nash, P., Eriksson, U. J., and Olovsson, M. H. (2009). Evidence of increased oxidative stress and a change in the plasminogen activator inhibitor (PAI)-1 to PAI-2 ratio in early-onset but not late-onset preeclampsia. Am. J. Obstet. Gynecol. 201 (6), 597.e1–597.e8. Epub 2009/08/18. doi:10.1016/j.ajog.2009.06.024
Xue, H., Shen, X., and Pan, W. (2021). Constrained maximum likelihood-based Mendelian randomization robust to both correlated and uncorrelated pleiotropic effects. Am. J. Hum. Genet. 108 (7), 1251–1269. Epub 2021/07/03. doi:10.1016/j.ajhg.2021.05.014
Ye, Y., Vattai, A., Zhang, X., Zhu, J., Thaler, C. J., Mahner, S., et al. (2017). Role of plasminogen activator inhibitor type 1 in pathologies of female reproductive diseases. Int. J. Mol. Sci. 18 (8), E1651. Epub 2017/08/02. doi:10.3390/ijms18081651
Zhai, J., Li, Z., Zhou, Y., and Yang, X. (2022). The role of plasminogen activator inhibitor-1 in gynecological and obstetrical diseases: An update review. J. Reprod. Immunol. 150, 103490. Epub 2022/02/06. doi:10.1016/j.jri.2022.103490
Keywords: adipokine, preeclampsia, Mendelian randomization, genetic epidemiology, single nucleotide polymorphism
Citation: Chen X, Liu Z, Cui J, Chen X, Xiong J and Zhou W (2022) Circulating adipokine levels and preeclampsia: A bidirectional Mendelian randomization study. Front. Genet. 13:935757. doi: 10.3389/fgene.2022.935757
Received: 06 May 2022; Accepted: 22 July 2022;
Published: 22 August 2022.
Edited by:
Zhiqiang Li, Qingdao University, ChinaReviewed by:
Jiajun Shi, Vanderbilt University Medical Center, United StatesMasahiro Yoshikawa, Nihon University School of Medicine, Japan
Copyright © 2022 Chen, Liu, Cui, Chen, Xiong and Zhou. This is an open-access article distributed under the terms of the Creative Commons Attribution License (CC BY). The use, distribution or reproduction in other forums is permitted, provided the original author(s) and the copyright owner(s) are credited and that the original publication in this journal is cited, in accordance with accepted academic practice. No use, distribution or reproduction is permitted which does not comply with these terms.
*Correspondence: Wei Zhou, dr.zhouwei@163.com
†These authors have contributed equally to this work