- 1National Center for Cool and Cold Water Aquaculture, Agricultural Research Service, United States Department of Agriculture, Kearneysville, WV, United States
- 2Troutlodge Inc., Sumner, WA, United States
Bacterial cold water disease (BCWD) is an important disease in rainbow trout aquaculture. Previously, we have identified and validated two major QTL (quantitative trait loci) for BCWD resistance, located on chromosomes Omy08 and Omy25, in the odd-year Troutlodge May spawning population. We also demonstrated that marker-assisted selection (MAS) for BCWD resistance using the favorable haplotypes associated with the two major QTL is feasible. However, each favorable haplotype spans a large genomic region of 1.3–1.6 Mb. Recombination events within the haplotype regions will result in new haplotypes associated with BCWD resistance, which will reduce the accuracy of MAS for BCWD resistance over time. The objectives of this study were 1) to identify additional SNPs (single nucleotide polymorphisms) associated with BCWD resistance using whole-genome sequencing (WGS); 2) to validate the SNPs associated with BCWD resistance using family-based association mapping; 3) to refine the haplotypes associated with BCWD resistance; and 4) to evaluate MAS for BCWD resistance using the refined QTL haplotypes. Four consecutive generations of the Troutlodge May spawning population were evaluated for BCWD resistance. Parents and offspring were sequenced as individuals and in pools based on their BCWD phenotypes. Over 12 million SNPs were identified by mapping the sequences from the individuals and pools to the reference genome. SNPs with significantly different allele frequencies between the two BCWD phenotype groups were selected to develop SNP assays for family-based association mapping in three consecutive generations of the Troutlodge May spawning population. Among the 78 SNPs derived from WGS, 77 SNPs were associated with BCWD resistance in at least one of the three consecutive generations. The additional SNPs associated with BCWD resistance allowed us to reduce the physical sizes of haplotypes associated with BCWD resistance to less than 0.5 Mb. We also demonstrated that the refined QTL haplotypes can be used for MAS in the Troutlodge May spawning population. Therefore, the SNPs and haplotypes reported in this study provide additional resources for improvement of BCWD resistance in rainbow trout.
Introduction
The global demand for seafood has roughly doubled since the start of the 21st century, and will likely double again by 2050 (Naylor et al., 2021). Rainbow trout (Oncorhynchus mykiss) is one of the most widely cultured cold freshwater fish, with production on every continent except Antarctica. The global production of rainbow trout was about 917,000 tons in 2019 (FAO, 2021). Outbreaks of infectious disease are one of the major challenges for rainbow trout production and welfare. Bacterial cold water disease (BCWD), caused by Flavobacterium psychrophilum, is a frequent disease in rainbow trout (Nematollahi et al., 2003; Starliper, 2011; Loch and Faisal, 2015). Commercial vaccines for BCWD are not available yet. Use of licensed antibiotics for BCWD treatment increases production cost and antibiotic resistant pathogens may emerge.
Use of genetic resistance is an effective approach to control BCWD in rainbow trout. A rainbow trout line with improved resistance to BCWD has been developed by using family-based selection (Leeds et al., 2010; Wiens et al., 2013a; Wiens et al., 2018). Recently, multiple studies have demonstrated that genomic selection (GS) can substantially improve the accuracy of selection for BCWD resistance in rainbow trout. Vallejo et al. (2017a) reported that GS using a 57K SNP (single nucleotide polymorphism) genotyping array (Palti et al., 2015a) can double the accuracy of selection for BCWD resistance in a commercial breeding population. To reduce the cost of genotyping, the accuracy of GS for BCWD resistance was evaluated with low-density SNP panels. The accuracy of GS remained substantially higher than pedigree-based selection when using 70 SNPs associated with QTLs (quantitative trait locus) for BCWD resistance (Vallejo et al., 2018). To reduce the cost of BCWD phenotyping, it has recently been reported that the accuracy of GS for BCWD resistance without model retraining in the subsequent generation remained higher than pedigree-based selection (Vallejo et al., 2021).
To fully exploit the genetic resistance to BCWD, extensive genetic mapping studies were conducted to identify and validate QTLs for BCWD resistance in rainbow trout. Fraslin et al. (2018) used both immersion and intramuscular injection methods to evaluate double haploids derived from a cross between two rainbow trout isogenic lines, and 15 QTLs for BCWD resistance were identified. Also, two QTLs for BCWD resistance were identified after a natural disease outbreak on a French farm (Fraslin et al., 2019). At the USDA National Center for Cool and Cold Water Aquaculture, we initially used full-sib mapping families to identify and validate QTL for BCWD resistance (Wiens et al., 2013b; Vallejo et al., 2014a; Vallejo et al., 2014b; Palti et al., 2015b; Liu et al., 2015). With the advancement of genomic resources available in rainbow trout such as a SNP genotyping array (Palti et al., 2015a) and a reference genome (Pearse et al., 2019), we performed a genome-wide association study (GWAS) to detect QTL for BCWD resistance (Vallejo et al., 2017b) in the 2013 generation of the Troutlodge May spawning population. Three QTL for BCWD resistance with moderate-large effects, located on chromosomes Omy03, Omy08, and Omy25, were identified. In a follow-up study the three QTLs were validated in the 2015 generation of the Troutlodge May spawning population (Liu et al., 2018). In the same study it was shown that SNP haplotypes associated with the two major QTL on chromosomes Omy08 and Omy25 can be used for marker-assisted selection (MAS) for BCWD resistance. However, the two favorable haplotypes for the two major QTL on chromosomes Omy08 and Omy25 span regions of 1.3 and 1.6 Mb, respectively. Recombination events within the haplotype regions may result in new haplotypes associated with BCWD resistance, which will reduce the accuracy of MAS for BCWD resistance over time. Thus, it is important to identify and validate additional SNPs associated with the two major QTL for BCWD resistance with a goal to reduce the physical size of haplotypes associated with BCWD resistance.
Whole-genome sequencing (WGS) is a powerful tool to discover SNPs and to identify causative genes for traits of interest. With the recent rapid reduction in the cost of next generation sequencing, the use of WGS has become more common in genetic studies of salmonids (Gao et al., 2018; Narum et al., 2018; Thompson et al., 2020; Liu et al., 2021). The objectives of this study were 1) to identify additional SNPs associated with BCWD resistance using WGS; 2) to validate the SNPs associated with BCWD resistance using family-based association mapping; 3) to refine the haplotypes associated with BCWD resistance; and 4) to evaluate MAS for BCWD resistance using the refined QTL haplotypes.
Materials and Methods
Five Consecutive Generations of the Troutlodge May Spawning Strain
Troutlodge, Inc., has four rainbow trout strains (Liu et al., 2017) named by their peak spawning months. All samples used in this study were from the May spawning strain. Five consecutive generations (Table 1) were used in this study. The samples used for WGS were from the 2013 and 2015 generations. Selected families from three consecutive generations, 2015, 2017, and 2019, were used for association analyses of BCWD resistance. Fish of the 2021 generation were used to evaluate MAS for BCWD resistance. Previously, each Troutlodge strain had two populations, odd-year and even-year populations. The odd-year and even-year May spawning populations have been merged into one population since the 2019 generation.

TABLE 1. Summary of samples from five consecutive generations of the Troutlodge May spawning population used in this study.
BCWD Challenge Experiments
BCWD challenge experiments were conducted in four consecutive generations, 2015, 2017, 2019, and 2021 (Supplementary Table S1). Fish (80–99 days post-hatch) were challenged by intraperitoneal injection of Flavobacterium psychrophilum strain CSF259-93 using the established protocol described in detail by Hadidi et al. (2008). Mortalities were collected daily for 21 days after intraperitoneal injection. Both survival days (DAYS), the number of days to death after BCWD challenge, and survival status (STATUS), 2 for dead fish and 1 for survivors at day 21, were recorded for each fish. Each family of the 2015 and 2017 generations was evaluated for BCWD resistance using two replicate tanks (3 L tank with a water flow rate of 1 L per minute) with 40 fish per tank, and the details have been reported in our previous publications (Vallejo et al., 2017a; Liu et al., 2018). For the 2019 generation, we increased the number of fish challenged per family to 3 or 4 replicate tanks (40 fish per tank) based on fish availability. The 2021 families were pooled and challenged as described below to evaluate MAS for BCWD resistance.
Sequencing of 40 Parents of the 2015 and 2017 Generations
Based on the BCWD survival rates of the 2015 and 2017 families and parental haplotypes for the two targeted QTL regions, Omy08 and Omy25, we selected 40 (Supplementary Table S2) parents for individual sequencing and pooled sequencing. First, we sorted the families within each generation by BCWD survival rate from high to low. The parents of top 20 families were assigned to a BCWD resistant (R) group, and the parents of bottom 20 families were assigned to a BCWD susceptible (S) group. Then, the QTL haplotypes of these parents were reconstructed for the two QTL regions using the same SNP sets and method reported in Liu et al. (2018). The parents of the 2015 and 2017 generations were selected to target for the Omy08 and Omy25 BCWD QTL, respectively. For each generation, we selected 10 R parents that are fixed for the favorable haplotype for the targeted QTL and have at least one favorable haplotype for the other QTL. We also selected 10 S parents without any favorable haplotype for the targeted QTL. Thus, a total of 40 parents were selected for WGS with a targeted genome coverage of 15x per sample. In addition to sequencing of individuals, we also pooled equal amount of DNA per fish by BCWD groups within each generation for pooled sequencing. The targeted genome coverage per pool was 30x. The raw sequences of the parents were deposited in sequence read archive (SRA) under BioProject PRJNA681179.
Sequencing of Pooled Offspring of the 2015 Generation
The sequencing of parents described above might be biased because both BCWD survival rates and QTL haplotypes were used to select the samples used for sequencing. Thus, we decided to use BCWD phenotype as the only criteria to select samples for additional pooled sequencing. Among the 138 families of the 2015 generation evaluated for BCWD resistance, we selected 60 families with intermediate BCWD survival rates that ranged from 24% to 71% for sequencing. For each of the 60 families, we selected the first four fish that died after day 3 (to avoid fish died from injection injury or stress) and four random survivors. Each of the four dead fish or survivors was randomly assigned to one of the four corresponding BCWD status pools. In total, we had four DNA pools of dead fish (S pools) and four DNA pools of survivors (R pools). Equal amount of DNA per sample was pooled for sequencing with a targeted genome coverage of 45x per DNA pool. The sequences of the pooled offspring were deposited under BioProject PRJNA830380.
Whole-Genome Sequencing and SNP Identification
DNA was extracted from fin clips following the manufacturer’s recommended protocols for AutoGenprep 965 (Autogen, Holliston, MA, United States). Whole-genome DNA sequencing libraries were prepared using the KAPA HyperPrep kit (KAPA Biosystems, Wilmington MA), and were sequenced in paired-end (2 × 150 bp) mode on an Illumina HiSeq X sequencer. The sequence reads were mapped to rainbow trout reference genome GCF_002163495.1 (Pearse et al., 2019) using BWA-MEM algorithm (Li, 2013), and alignments were converted to BAM (Binary sequence Alignment/Map) format using SAMtools v1.11 (Li et al., 2009). PCR duplicates were marked and removed using Picard v2.18.2 (http://broadinstitute.github.io/picard/). Following our previously published SNP calling and filtering pipeline (Gao et al., 2018), SNPs were called using program freebayes v1.3.1 (Garrison and Marth, 2012), and were annotated using program SnpEff v4.3 (Cingolani et al., 2012).
Identification of SNPs in the QTL Regions
For the sequence data of parents for the 2015 and 2017 generations, we used a sliding window approach to identify SNPs in the BCWD QTL regions. We used a window size 10,000 bp and a step size 5,000 bp to calculate the fixation index (Fst) value for each window. For individual sequencing, program VCFtools v0.1.16 (Danecek et al., 2011) was used to calculate Fst value for each sliding window. For pooled sequencing, program PoPoolation2 (Kofler et al., 2011) was used to calculate Fst value for each sliding window. Only windows with at least 15 SNPs were included to identify windows with significantly different allele frequencies (empirical p < 0.0001) between the R and S groups. For individual sequencing, program VCFtools was also used to calculate Fst value for each SNP with MAF (minor allele frequency) greater than 0.05.
To identify SNPs with significantly different allele frequencies between the pools of R and S offspring from the 2015 generation, Fisher’s exact tests were performed using the program PoPoolation2 with the following settings: -min-coverage 40, --max-coverage 400 and--min-count 10. To correct for multiple tests, SNPs with p-values less than 4.05 × 10–9 (Bonferroni-correction for 12,338,978 SNPs) were considered as significant.
SNP Genotyping
Among the SNPs identified by WGS, we selected a set of SNPs in the Omy08 and Omy25 QTL regions for association analyses. The SNPs were selected for assay design because they met one or more of the following criteria: 1) The Fst values between R and S parents were high; 2) The p-values of Fisher’s test for different allele frequencies between R and S pools of the 2015 generation were low; 3) The SNPs had high or moderate effects based on SNP annotation; 4) SNPs are near the six SNPs used in our previous haplotype analysis (Liu et al., 2018). The sequences of the selected SNPs were submitted to Fluidigm (South San Francisco, CA) for assay design. After a preliminary evaluation of assay quality using a subset of mapping samples of the 2015 generation, we assembled a panel of 96 SNPs (Supplementary Table S3) to genotype the mapping samples of three consecutive generations, 2015, 2017, and 2019.
We followed the SNP genotyping protocol described in our previous study (Liu et al., 2016). Briefly, DNA samples were pre-amplified, and the pre-amplified products were diluted and used for genotyping with 96.96 Dynamic Array IFCs (Integrated Fluidic Circuits). The arrays were read using EP1 system, and genotypes were called automatically using Fluidigm SNP genotyping analysis software 4.1 with a confidence threshold of 85. The genotype clusters were examined by eye for each assay, and any wrong calls or no calls were corrected manually. The computer program PedCheck (O'Connell and Weeks, 1998) was used to identify genotypes with Mendelian inheritance errors between parents and offspring. Seven SNPs (Supplementary Table S3) were removed from association analysis due to poor genotype clusters or high rates of genotype discrepancies between parents and offspring.
Family-Based Association Mapping of BCWD Resistance
The program PLINK 1.9 (Chang et al., 2015) was used for family-based association mapping to validate SNPs associated with BCWD resistance (p < 0.01). The procedure QFAM was used to analyze the phenotypic data DAYS, and the PERM option was used to correct the family structure. The procedure TDT (transmission disequilibrium test) was used to analyze the binary phenotype STATUS. The association analyses were performed for each of the three consecutive generations, 2015, 2017, and 2019.
Haplotype Association Analysis of BCWD Resistance
We used three criteria to select three SNPs per QTL region for haplotype association analysis. 1) The selected SNPs are highly associated with BCWD resistance based on single SNP association analysis; 2) The MAF for each selected SNP is greater than 0.2; and 3) The three SNPs for each QTL region span a genomic region less than 0.5 Mb according to rainbow trout reference genome GCF_002163495.1 (Pearse et al., 2019). Based these three criteria, three SNPs, P489, P194, and P355, were selected for the Omy08 QTL, and three SNPs, P420, P430, and P212, were selected for the Omy25 QTL. The same families used for single SNP analysis described above were also used for haplotype association analysis. The haplotypes for each fish were constructed using Beagle 5.1 (Browning and Browning, 2007), and haplotypes with frequency larger than 0.1 were retained for haplotype association analysis. Program PLINK1.9 was used for haplotype association analysis following the same method for family-based association analysis of single SNP as described above.
Evaluation of MAS for BCWD Resistance in the 2021 Generation
The parents for the 2021 generation were genotyped with 96 SNPs (Supplementary Table S3), and haplotypes for the Omy08 and Omy25 QTL regions were constructed using the same SNPs and method described above. The 163 families of the 2021 generation were sorted by the total count of favorable haplotypes from high to low. The top 25 families were assigned to the high haplotype group, and the bottom 25 families were assigned to low haplotype group. We pooled 10 fish per family by haplotype groups, and the 250 fish were challenged with BCWD in a 40 L tank with a water flow rate of 2.5 L per minute. There were two replicate tanks for each haplotype group. So, a total of 500 fish were challenged per haplotype group. To test the BCWD survival difference between the high and low favorable haplotype groups, a log-rank test was performed using the survival package (Therneau, 2021) available in R version 4.1.2 (R Core Team, 2021).
Results
Identification of Genomic Regions Associated With BCWD Resistance Using WGS
The 20 parents for the 2015 generation used for sequencing were selected to target the Omy08 QTL for BCWD resistance. For individual sequencing, all windows with significantly different Fst between R and S parents were in a region between 73.2 and 78.2 Mb on chromosome Omy08 (Figure 1A). For pooled sequencing, except two windows on chromosome Omy05 and one window on chromosome Omy13, all the other windows with significantly different Fst between R and S pools were in a region between 73.2 and 78.0 Mb on chromosome Omy08 (Figure 1B). Thus, we selected SNPs in the region from 73.2 to 78.2 Mb to validate SNPs associated with the BCWD QTL on chromosome Omy08.
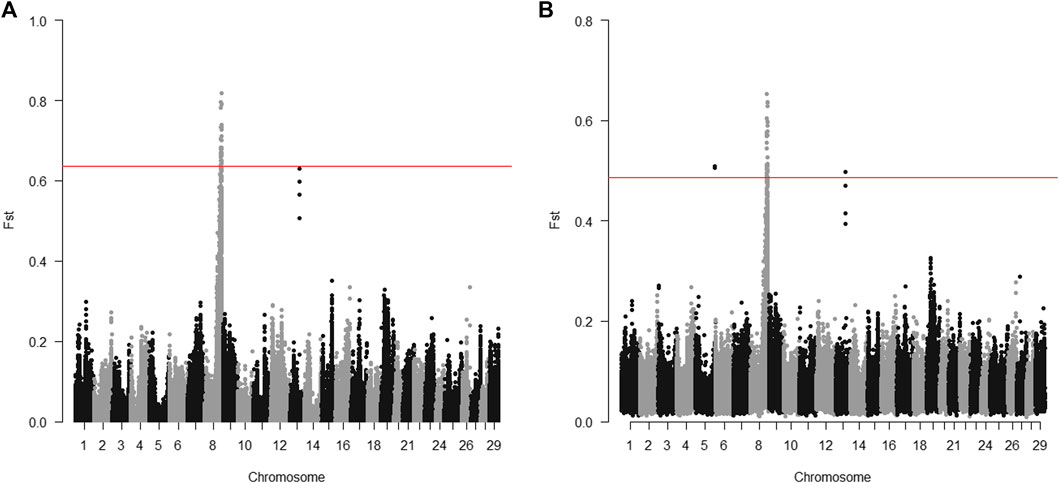
FIGURE 1. Distribution of Fst between R and S groups of parents for the 2015 generation of the Troutlodge May spawning population. (A) sequencing of individuals; (B) sequencing of pooled samples. The red horizontal lines represent the threshold for significantly different Fst (empirical p < 0.0001).
The 20 parents for the 2017 generation used for sequencing were selected to target the Omy25 QTL for BCWD resistance. For individual sequencing, all windows with significantly different Fst between R and S parents were in a region between positions 16.5 and 40.1 Mb on chromosome Omy25 (Figure 2A). For pooled sequencing, except one window on chromosome Omy05 and one window on chromosome Omy12, all the other windows with significantly different Fst between R and S pools were located on chromosome Omy25 in a region between 18.9 and 41.0 Mb (Figure 2B). Thus, we selected SNPs in the region from 16.5 to 41.0 Mb to validate SNPs associated with the BCWD QTL on chromosome Omy25.
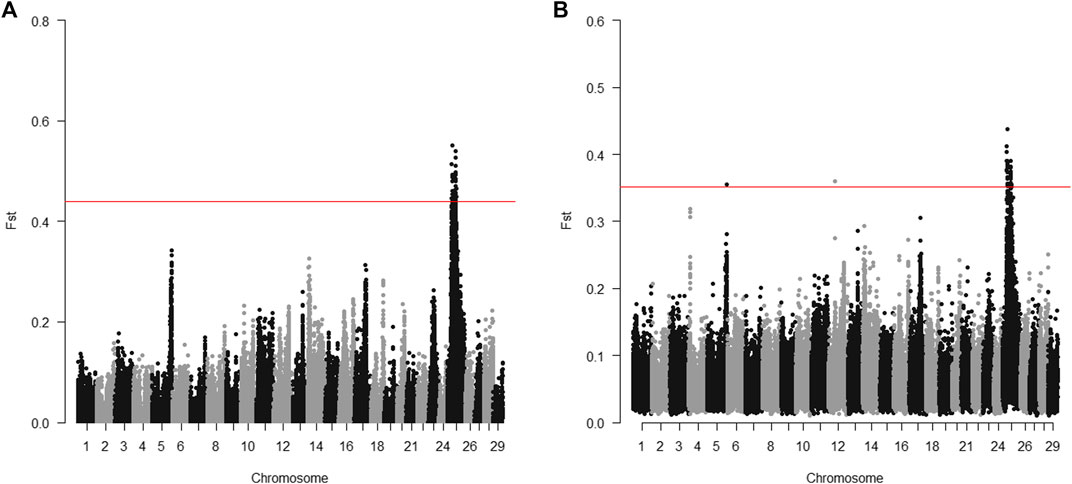
FIGURE 2. Distribution of Fst between R and S groups of parents for the 2017 generation of the Troutlodge May spawning population. (A) sequencing of individuals; (B) sequencing of pooled samples. The red horizontal lines represent the threshold for significantly different Fst (empirical p < 0.0001).
To avoid the potential bias of samples used for sequencing just described above, we also sequenced pooled offspring of the 2015 generation. The samples were selected with BCWD phenotypes alone, and they were pooled by survival status. After mapping the sequence reads to the reference genome, 12.3 million SNPs were identified. Based on Fisher’s test for each SNP, 21 SNPs with significantly different allele frequencies between the R and S pools were identified (Figure 3), and they were located on chromosomes Omy04, Omy05, Omy08, Omy11, Omy16, Omy17, Omy20, and Omy25. Only chromosomes Omy08 and Omy25 had more than three significant SNPs, and these significant SNPs were located in similar QTL regions to those identified by WGS of parents as described above.
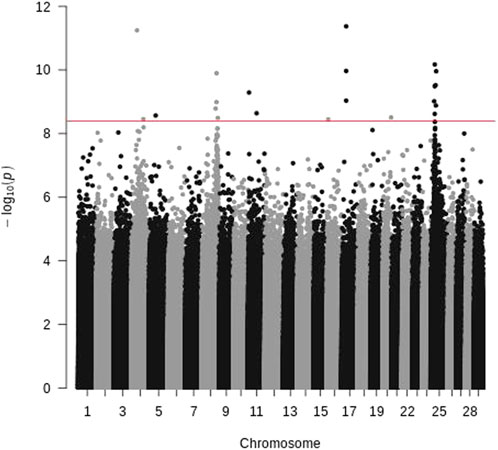
FIGURE 3. Manhattan plot of pooled offspring of the 2015 generation of the Troutlodge May spawning population. The red horizontal line represents the significance threshold (Bonferroni-correction for 12,338,978 SNPs).
Validation of SNPs Associated With BCWD Resistance
Among the 89 SNPs used for association analysis in three consecutive generations, 2015, 2017, and 2019, 85 SNPs were associated with BCWD resistance in at least one of the three generations (Table 2). Also, 77 out of the 85 validated SNPs were identified via WGS reported in this study. Among the 4 SNPs, P161, P176, P316, and P490, that were not associated with BCWD resistance in this study, P490 was the only SNP derived from WGS.
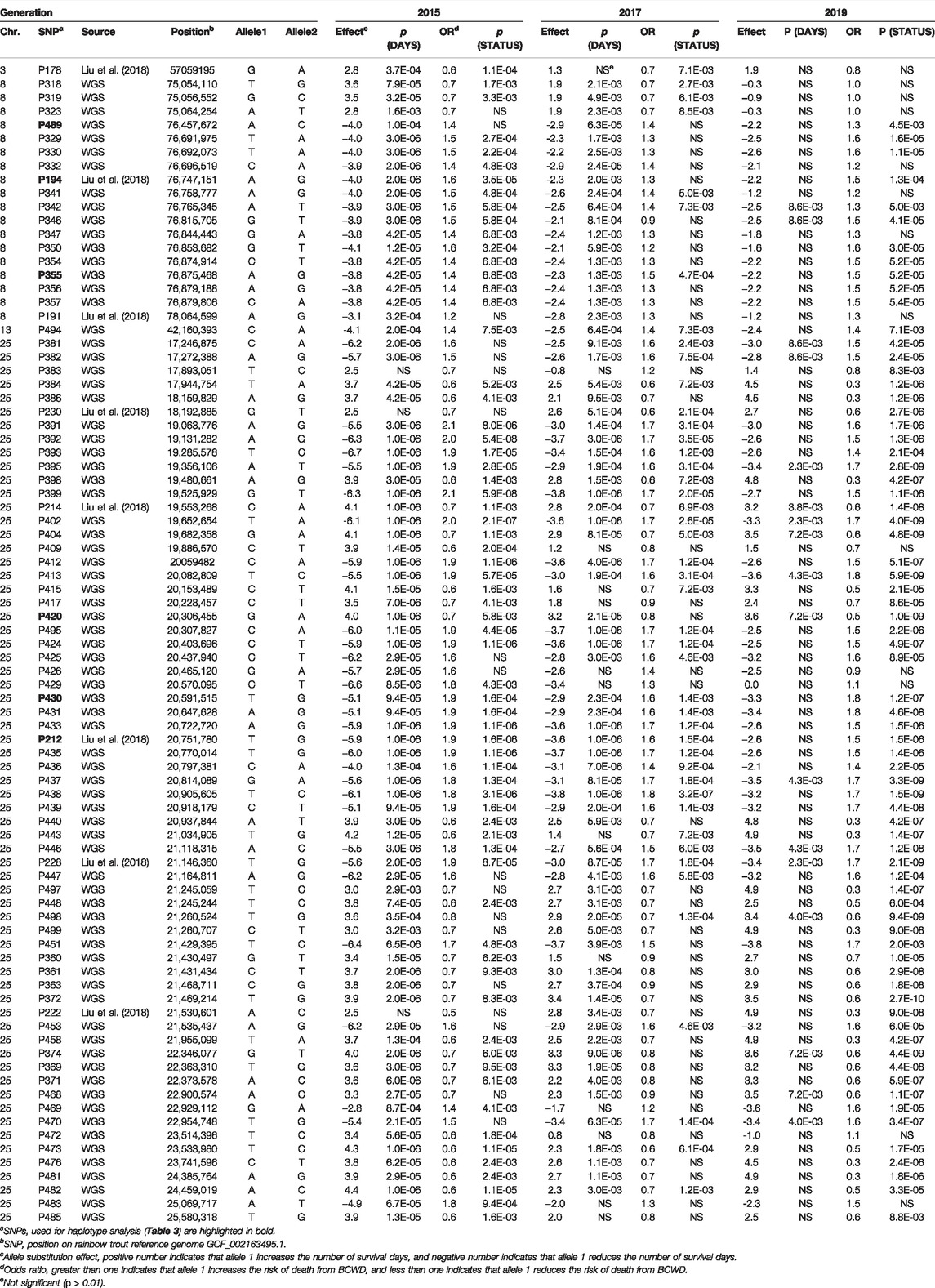
TABLE 2. Validation of SNPs associated with BCWD resistance in three consecutive generations of the Troutlodge May spawning population.
Refined Haplotypes Associated With BCWD Resistance
The 85 SNPs associated with BCWD resistance validated above allowed us to reduce the physical size of the haplotypes associated with BCWD resistance. Using the three criteria described in the method section, we selected SNPs, P489, P194, and P355, for the Omy08 QTL, and SNPs, P420, P430, and P212, for the Omy25 QTL. The three selected SNPs span a region less than 0.5 Mb. Thus, we reduced the sizes of haplotypes by about two-thirds. The results of haplotype association analysis are summarized in Table 3. For the Omy08 QTL, haplotype CGG was associated with BCWD resistance in all three generations, which increases the number of survival days and reduces the risk of death from BCWD. Similarly, haplotype GGG on chromosome Omy25 was also associated with BCWD resistance in all three generations, which increases the number of survival days and reduces the risk of death from BCWD. The other two haplotypes (Table 3) were associated with BCWD susceptibility. Because the goal of selective breeding is to improve BCWD resistance, we will focus on the two haplotypes associated with BCWD resistance and refer to them as favorable haplotypes for BCWD resistance.
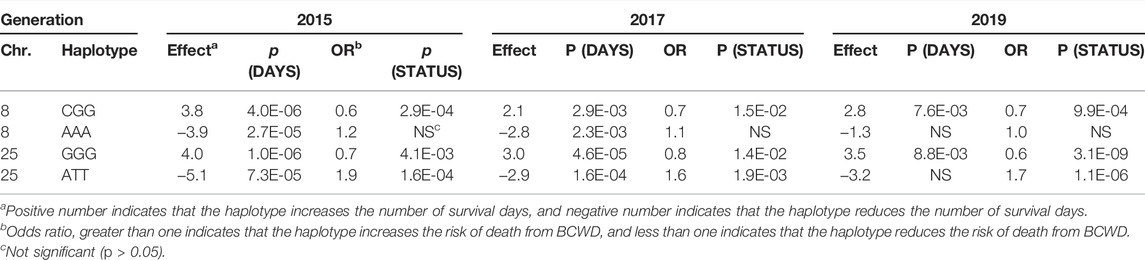
TABLE 3. Haplotype association analysis of BCWD resistance in three consecutive generations of the Troutlodge May spawning population.
Evaluation of MAS for BCWD Resistance
The 25 families from the 2021 generation with high or low counts of favorable haplotypes for the two major QTL regions had an average of 6.48 and 2.56 favorable haplotypes per family, respectively. The BCWD survival rates for the pooled families with high or low counts of favorable haplotypes were 83.6% and 66.2%, respectively. Survival analysis demonstrated that the two BCWD survival curves were significantly different (p = 7e-10) (Figure 4) for the two groups of families with high or low counts of favorable haplotypes. Thus, the favorable haplotypes can be used to select families with improved BCWD resistance.
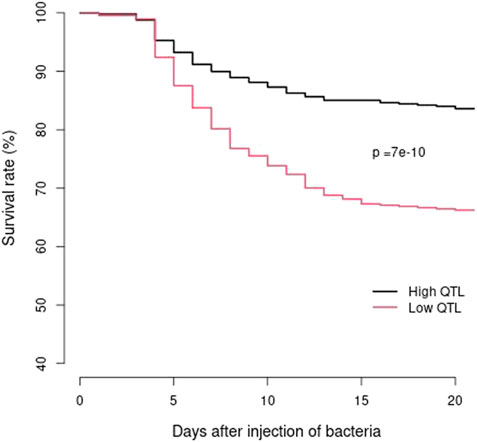
FIGURE 4. BCWD survival curves of the 2021 generation of the Troutlodge May spawning population. The black curve represents the families with high counts of favorable haplotypes for the two major BCWD QTL regions. The red curve represents the families with low counts of favorable haplotypes for the two major BCWD QTL regions.
Discussion
In this study, we used WGS to identify additional SNPs associated with the two major QTL for BCWD resistance, and 77 SNPs identified from WGS were validated by association mapping in three consecutive generations of the Troutlodge May spawning population. The additional SNPs associated with BCWD resistance allowed us to refine the favorable haplotypes associated with BCWD resistance. We demonstrated that the refined favorable QTL haplotypes can be used for MAS for BCWD resistance in the Troutlodge May spawning population.
Identification of SNPs Associated With BCWD Resistance Using WGS
Among the 78 SNPs derived from WGS, only SNP P490 was not associated with BCWD resistance in this study. The high SNP validation rate was largely due to several factors. 1) The samples used for sequencing were selected with two methods, BCWD phenotypes together with QTL haplotypes or BCWD phenotypes alone; 2) We used two sequencing strategies, sequencing of individuals and pooled samples; 3) The SNPs selected for assay design were filtered by multiple criteria as described in the method section; 4) We removed SNPs with poor genotype quality or SNPs were not associated with BCWD resistance based on a preliminary study with a sub-set of mapping samples. Due to the genotyping platform used in this study, only 96 SNPs including both SNPs derived from WGS and SNPs reported in our previous study (Liu et al., 2018) were used for association mapping. However, analysis of WGS revealed many more SNPs that were putatively associated with BCWD resistance. Thus, WGS is a powerful tool to identify SNPs associated with BCWD resistance in rainbow trout.
Sequencing pools of individuals (pool-seq) is cost-effective, and has been successfully applied to a variety of studies (Schlotterer et al., 2014). However, pool-seq also has technical challenges and limitations. Unequal representation of DNA samples in the pools can cause false positive signals. The same parental DNA samples were sequenced individually and by pool-seq in this study. Although the genomic regions with significantly different Fst were similar for both sequencing strategies (Figures 1, 2) for the targeted QTL regions, a few additional genomic regions also showed significantly different allele frequencies for the pooled samples, which was likely due to unequal representation of DNA samples in the pools. Compared to the results of sequencing of parents, more genomic regions showed significantly different allele frequencies between the DNA pools of offspring in the 2015 generation (Figure 3). Most of them are likely false positives due to the technical challenges of pool-seq. In addition to the possibility of unequal representation of each offspring in the pool, unreliable BCWD phenotypes could be a major factor since it is not possible to have replicated BCWD challenges of an individual fish. The offspring were selected for pool-seq on basis of BCWD survival status of individual fish. On the other hand, the family BCWD survival rates are based on a large number of offspring, and hence are much more reliable than the BCWD survival status of an individual fish.
Two Robust QTL for BCWD Resistance in Rainbow Trout
QTL validation is essential for implement of MAS in breeding programs and identification of causative genes. The two major QTL for BCWD resistance, located on chromosomes Omy08 and Omy25, were initially identified in the 2013 generation of Troutlodge May spawning population (Vallejo et al., 2017b), and were validated in the 2015 generation of the same population (Liu et al., 2018). In this study, we used WGS to identify additional SNPs associated with these two major QTL, and 77 additional SNPs associated with BCWD resistance were validated in three consecutive generations, 2015, 2017 and 2019, of the Troutlodge May spawning population. Thus, the two major QTL for BCWD resistance are robust in the Troutlodge May population, and it is worthwhile to evaluate MAS for BCWD resistance and to identify positional candidate genes underlying the QTL.
Robust MAS for BCWD Resistance in Rainbow Trout
We reported previously that the accuracies of retrospective MAS for BCWD resistance using favorable haplotypes associated with the two major BCWD QTL were equal or greater than the accuracies of family-based selection in the same generation of odd-year Troutlodge May spawning population (Liu et al., 2018). In this study, we reduced the physical size of the haplotypes by about two-thirds. We then used the refined haplotypes for MAS for BCWD resistance in the 2021 generation of the Troutlodge May spawning population. Based on the QTL haplotypes of the parents, two groups of families with high or low counts of favorable haplotypes, respectively, were selected for pooled BCWD challenge. The two groups of families had significantly different BCWD survival curves. It is notable that the odd-year and even-year Troutlodge May spawning populations have been combined into one population since the 2019 generation. Together with the results of retrospective MAS reported previously (Liu et al., 2018), we conclude that MAS for BCWD resistance is robust in the Troutlodge May spawning population.
Although we focused on MAS for BCWD in this study, it is important to note that the additional SNPs associated BCWD resistance reported in this study should also be useful to improve the accuracy of GS for BCWD resistance. We reported previously that the accuracy of GS for BCWD resistance using 70 SNPs associated with BCWD resistance was similar to the accuracy of the whole-genome 57K SNP array (Vallejo et al., 2018). Furthermore, it has been documented that functional and causative variants can be used to improve the accuracy of GS (Xiang et al., 2021). Some of the SNPs reported in this study are located within candidate genes for BCWD resistance (see below).
Candidate Genes of QTL for BCWD Resistance in Rainbow Trout
Our long-term goal is to identify causative genes for BCWD resistance in rainbow trout. Although the refined haplotypes are associated with resistance to BCWD, they may or may not span the QTL regions. Thus, we arbitrarily extended 0.5 Mb on each end of the refined favorable haplotypes associated with BCWD resistance, and then examined protein-coding genes in the corresponding regions of rainbow trout Arlee reference genome (Gao et al., 2021), which has a better genome coverage than the previous Swanson reference genome (Pearse et al., 2019). Based on the NCBI rainbow trout gene annotation release 101, a total of 70 annotated protein-coding genes were identified in the two major QTL regions (Supplementary Table S4).
Among the 40 annotated protein-coding genes in the Omy08 QTL region, multiple genes are likely related to immune responses. Both LOC110530755 and LOC110530756 encode NACHT proteins, which are implicated in apoptosis and MHC (major histocompatibility complex) transcription activation (Koonin and Aravind, 2000; Laing et al., 2008), and play important roles in activation of animal innate immune responses to pathogen infection (Jones et al., 2016). Furthermore, NACHT proteins such as Nod like receptors also play an important role in activation of pyroptosis pathway in both mammals and fish (Song et al., 2022). Three other candidate genes, LOC110530758, LOC110530759, and LOC110530764, encode proteins likely belonging to the signaling lymphocytic activation molecule (SLAM) family of receptors, which in mammals are critical elements for both innate and adaptive immune responses (Veillette, 2006; Cannons et al., 2011). Also, these three SLAM genes were modestly upregulated at day 5 post challenge with Flavobacterium psychrophilum in the study reported by Marancik et al. (2015). In addition to the putative functions, the results of association mapping (Table 2) also indicated that these genes are strong candidates for the Omy08 QTL. All 8 SNPs in the candidate gene regions (Supplementary Table S4) are significantly associated with BCWD resistance in three consecutive generations of the Troutlodge May spawning population (Table 2). Thus, these immune-related genes are good candidates for the Omy08 QTL for BCWD resistance.
Among the 30 annotated genes in the Omy25 QTL region, gene LOC100136157, which encodes invariant chain INVX, stands out as a promising candidate gene for this QTL. For simplicity and consistency with rainbow trout literatures, we refer this gene as INVX from now on. Rainbow trout INVX was initially cloned and characterized by Fujiki et al. (2003), and is a homolog of mammalian invariant chain genes, which play important roles in antigen presentation (Schroder, 2016). Transcript level of INVX in rainbow trout cell line culture was significantly increased at 96 and 120 h after immune system activation with PMA (phorbol 12-myristate 13-acetate) (Semple et al., 2019). Also, INVX protein level was significantly reduced at 168 h after PMA stimulation (Semple et al., 2019). In addition to the putative function of INVX, there is also another line of evidence supporting INVX as a candidate gene for the Omy25 QTL. SNP P446, located in the intron of gene INVX, was significantly associated with BCWD resistance in three consecutive generations of the Troutlodge May spawning population (Table 2). Therefore, we will continue to evaluate this candidate gene using other approaches in the future.
In addition to the candidate genes highlighted above, we would like to caution that other genes could also be candidate genes for the BCWD QTL. Although we focused on protein-coding genes, there are also two annotated non-coding RNA genes in the Omy08 QTL region. We should not completely rule out other genes in the QTL regions until we can identify with high confidence the causative genes underlying the two QTL for BCWD resistance in rainbow trout.
Conclusion
WGS is a powerful tool to identify additional SNPs associated with the two major QTL for BCWD resistance in rainbow trout. The additional SNPs allowed us to reduce the physical size of haplotypes associated with BCWD resistance. We also demonstrated that the refined favorable QTL haplotypes can be used for MAS for BCWD resistance in the Troutlodge May spawning population. Thus, the additional SNPs and refined haplotypes associated with BCWD resistance reported in this study are useful for improvement of BCWD resistance and for eventual identification of genes for BCWD resistance in rainbow trout.
Data Availability Statement
The datasets presented in this study can be found in online repositories. The names of the repository/repositories and accession number(s) can be found in the article/Supplementary Material.
Ethics Statement
The animal study was reviewed and approved by Institutional Animal Care and Use Committee, National Center for Cool and Cold Water Aquaculture, Agriculture Research Service, United States Department of Agriculture.
Author Contributions
SL and YP conceived and planned the study; KM participated in the study planning and provided pedigree records, germplasm for disease challenges and tissue or DNA samples from the parents; YP, JE, TL, GW and SL coordinated, supervised and performed the disease challenge experiments; GG mapped the sequences to reference genome and called SNPs; SL designed genotyping plans and RL performed the genotyping experiments; SL analyzed the data and drafted the manuscript. All authors read and approved the final manuscript.
Funding
This study was supported by Agricultural Research Service CRIS projects 8082-32000-007 and 8082-31000-013.
Conflict of Interest
KM was employed by the company Troutlodge Inc.
The remaining authors declare that the research was conducted in the absence of any commercial or financial relationships that could be construed as a potential conflict of interest.
Publisher’s Note
All claims expressed in this article are solely those of the authors and do not necessarily represent those of their affiliated organizations, or those of the publisher, the editors and the reviewers. Any product that may be evaluated in this article, or claim that may be made by its manufacturer, is not guaranteed or endorsed by the publisher.
Acknowledgments
The authors would like to thank Kristy Shewbridge, Ryan Lipscomb, Clayton Birkett, Jenea McGowan, Josh Kretzer, Travis Moreland, Keira Osbourn, Vanessa Panaway, and Joe Beach for fish rearing and help with the disease challenge experiments. Mention of trade names or commercial products in this publication is solely for the purpose of providing specific information and does not imply recommendation or endorsement by the U.S. Department of Agriculture (USDA). USDA is an equal opportunity provider and employer.
Supplementary Material
The Supplementary Material for this article can be found online at: https://www.frontiersin.org/articles/10.3389/fgene.2022.936806/full#supplementary-material
Supplementary Table S1 | Detailed information of BCWD challenge experiments of four consecutive generations of the Troutlodge May spawning population.
Supplementary Table S2 | The 40 parents of the 2015 and 2017 generations of the Troutlodge May spawning population selected for sequencing.
Supplementary Table S3 | The primer sequences of 96 SNPs used for association mapping in three consecutive generations of the Troutlodge May spawning population.
Supplementary Table S4 | Protein-coding genes in the two major BCWD QTL regions based on NCBI rainbow trout gene annotation release 101.
References
Browning, S. R., and Browning, B. L. (2007). Rapid and Accurate Haplotype Phasing and Missing-Data Inference for Whole-Genome Association Studies by Use of Localized Haplotype Clustering. Am. J. Hum. Genet. 81, 1084–1097. doi:10.1086/521987
Cannons, J. L., Tangye, S. G., and Schwartzberg, P. L. (2011). SLAM Family Receptors and SAP Adaptors in Immunity. Annu. Rev. Immunol. 29 (29), 665–705. doi:10.1146/annurev-immunol-030409-101302
Chang, C. C., Chow, C. C., Tellier, L. C., Vattikuti, S., Purcell, S. M., and Lee, J. J. (2015). Second-Generation PLINK: Rising to the Challenge of Larger and Richer Datasets. Gigascience 4, 7. doi:10.1186/s13742-015-0047-8
Cingolani, P., Platts, A., Wang, L. L., Coon, M., Nguyen, T., Wang, L., et al. (2012). A Program for Annotating and Predicting the Effects of Single Nucleotide Polymorphisms, SnpEff: SNPs in the Genome of Drosophila M Strain W1118; Iso-2; Iso-3. Fly 6, 80–92. doi:10.4161/fly.19695
Danecek, P., Auton, A., Abecasis, G., Albers, C. A., Banks, E., Depristo, M. A., et al. (2011). The Variant Call Format and VCFtools. Bioinformatics 27, 2156–2158. doi:10.1093/bioinformatics/btr330
FAO (2021). Fishery and Aquaculture Statistics. Global Aquaculture Production 1950-2019 (FishstatJ). FAO Fisheries Division. Rome. [Online]. Available at: http://www.fao.org/fishery/statistics/software/fishstatj/en (Accessed September 17, 2021).
Fraslin, C., Dechamp, N., Bernard, M., Krieg, F., Hervet, C., Guyomard, R., et al. (2018). Quantitative Trait Loci for Resistance to Flavobacterium Psychrophilum in Rainbow Trout: Effect of the Mode of Infection and Evidence of Epistatic Interactions. Genet. Sel. Evol. 50, 60. doi:10.1186/s12711-018-0431-9
Fraslin, C., Brard‐Fudulea, S., D'ambrosio, J., Bestin, A., Charles, M., Haffray, P., et al. (2019). Rainbow Trout Resistance to Bacterial Cold Water Disease: Two New Quantitative Trait Loci Identified after a Natural Disease Outbreak on a French Farm. Anim. Genet. 50, 293–297. doi:10.1111/age.12777
Fujiki, K., Smith, C. M., Liu, L., Sundick, R. S., and Dixon, B. (2003). Alternate Forms of MHC Class II-Associated Invariant Chain Are Not Produced by Alternative Splicing in Rainbow Trout (Oncorhynchus Mykiss) but Are Encoded by Separate Genes. Dev. Comp. Immunol. 27, 377–391. doi:10.1016/s0145-305x(02)00119-2
Gao, G., Nome, T., Pearse, D. E., Moen, T., Naish, K. A., Thorgaard, G. H., et al. (2018). A New Single Nucleotide Polymorphism Database for Rainbow Trout Generated through Whole Genome Resequencing. Front. Genet. 9, 147. doi:10.3389/fgene.2018.00147
Gao, G. T., Magadan, S., Waldbieser, G. C., Youngblood, R. C., Wheeler, P. A., Scheffler, B. E., et al. (2021). A Long Reads-Based De-Novo Assembly of the Genome of the Arlee Homozygous Line Reveals Chromosomal Rearrangements in Rainbow Trout. G3-Genes Genomes Genet. 11 (4), jkab052. doi:10.1093/g3journal/jkab052
Garrison, E., and Marth, G. (2012). Haplotype-based Variant Detection from Short-Read Sequencing. arXiv:1207.3907 [q-bio.GN].
Hadidi, S., Glenney, G. W., Welch, T. J., Silverstein, J. T., and Wiens, G. D. (2008). Spleen Size Predicts Resistance of Rainbow Trout to Flavobacterium Psychrophilum Challenge. J. Immunol. 180, 4156–4165. doi:10.4049/jimmunol.180.6.4156
Jones, J. D., Vance, R. E., and Dangl, J. L. (2016). Intracellular Innate Immune Surveillance Devices in Plants and Animals. Science 354 (6316), aaf6395. doi:10.1126/science.aaf6395
Kofler, R., Pandey, R. V., and Schlotterer, C. (2011). PoPoolation2: Identifying Differentiation between Populations Using Sequencing of Pooled DNA Samples (Pool-Seq). Bioinformatics 27, 3435–3436. doi:10.1093/bioinformatics/btr589
Koonin, E. V., and Aravind, L. (2000). The NACHT Family - A New Group of Predicted NTPases Implicated in Apoptosis and MHC Transcription Activation. Trends Biochem. Sci. 25, 223–224. doi:10.1016/s0968-0004(00)01577-2
Laing, K. J., Purcell, M. K., Winton, J. R., and Hansen, J. D. (2008). A Genomic View of the NOD-Like Receptor Family in Teleost Fish: Identification of a Novel NLR Subfamily in Zebrafish. BMC Evol. Biol. 8, 42. doi:10.1186/1471-2148-8-42
Leeds, T. D., Silverstein, J. T., Weber, G. M., Vallejo, R. L., Palti, Y., Rexroad, C. E., et al. (2010). Response to Selection for Bacterial Cold Water Disease Resistance in Rainbow Trout. J. Animal Sci. 88, 1936–1946. doi:10.2527/jas.2009-2538
Li, H. (2013). Aligning Sequence Reads, Clone Sequences and Assembly Contigs with BWA-MEM. arXiv:1303.3997v2.
Li, H., Handsaker, B., Wysoker, A., Fennell, T., Ruan, J., Homer, N., et al. (2009). The Sequence Alignment/Map Format and SAMtools. Bioinformatics 25, 2078–2079. doi:10.1093/bioinformatics/btp352
Liu, S., Vallejo, R. L., Palti, Y., Gao, G., Marancik, D. P., Hernandez, A. G., et al. (2015). Identification of Single Nucleotide Polymorphism Markers Associated with Bacterial Cold Water Disease Resistance and Spleen Size in Rainbow Trout. Front. Genet. 6, 298. doi:10.3389/fgene.2015.00298
Liu, S., Gao, G., Layer, R. M., Thorgaard, G. H., Wiens, G. D., Leeds, T. D., et al. (2021). Identification of High-Confidence Structural Variants in Domesticated Rainbow Trout Using Whole-Genome Sequencing. Front. Genet. 12, 639355. doi:10.3389/fgene.2021.639355
Liu, S., Palti, Y., Gao, G., and Rexroad, C. E. (2016). Development and Validation of a SNP Panel for Parentage Assignment in Rainbow Trout. Aquaculture 452, 178–182. doi:10.1016/j.aquaculture.2015.11.001
Liu, S., Palti, Y., Martin, K. E., Parsons, J. E., and Rexroad, C. E. (2017). Assessment of Genetic Differentiation and Genetic Assignment of Commercial Rainbow Trout Strains Using a SNP Panel. Aquaculture 468, 120–125. doi:10.1016/j.aquaculture.2016.10.004
Liu, S., Vallejo, R. L., Evenhuis, J. P., Martin, K. E., Hamilton, A., Gao, G., et al. (2018). Retrospective Evaluation of Marker-Assisted Selection for Resistance to Bacterial Cold Water Disease in Three Generations of a Commercial Rainbow Trout Breeding Population. Front. Genet. 9, 286. doi:10.3389/fgene.2018.00286
Loch, T. P., and Faisal, M. (2015). Emerging Flavobacterial Infections in Fish: A Review. J. Adv. Res. 6, 283–300. doi:10.1016/j.jare.2014.10.009
Marancik, D., Gao, G., Paneru, B., Ma, H., Hernandez, A. G., Salem, M., et al. (2015). Whole-Body Transcriptome of Selectively Bred, Resistant-, Control-, and Susceptible-Line Rainbow Trout Following Experimental Challenge with Flavobacterium Psychrophilum. Front. Genet. 5, 453. doi:10.3389/fgene.2014.00453
Narum, S. R., Di Genova, A., Micheletti, S. J., and Maass, A. (2018). Genomic Variation Underlying Complex Life-History Traits Revealed by Genome Sequencing in Chinook Salmon. Proc. R. Soc. B-Biol. Sci. 285 (1883), 20180935. doi:10.1098/rspb.2018.0935
Naylor, R. L., Kishore, A., Sumaila, U. R., Issifu, I., Hunter, B. P., Belton, B., et al. (2021). Blue Food Demand across Geographic and Temporal Scales. Nat. Commun. 12, 5413. doi:10.1038/s41467-021-25516-4
Nematollahi, A., Decostere, A., Pasmans, F., and Haesebrouck, F. (2003). Flavobacterium Psychrophilum Infections in Salmonid Fish. J. Fish. Dis. 26, 563–574. doi:10.1046/j.1365-2761.2003.00488.x
O'Connell, J. R., and Weeks, D. E. (1998). PedCheck: A Program for Identification of Genotype Incompatibilities in Linkage Analysis. Am. J. Hum. Genet. 63, 259–266. doi:10.1086/301904
Palti, Y., Gao, G., Liu, S., Kent, M. P., Lien, S., Miller, M. R., et al. (2015a). The Development and Characterization of a 57K Single Nucleotide Polymorphism Array for Rainbow Trout. Mol. Ecol. Resour. 15, 662–672. doi:10.1111/1755-0998.12337
Palti, Y., Vallejo, R. L., Gao, G., Liu, S., Hernandez, A. G., Rexroad, C. E., et al. (2015b). Detection and Validation of QTL Affecting Bacterial Cold Water Disease Resistance in Rainbow Trout Using Restriction-Site Associated DNA Sequencing. PLoS One 10, e0138435. doi:10.1371/journal.pone.0138435
Pearse, D. E., Barson, N. J., Nome, T., Gao, G., Campbell, M. A., Abadía-Cardoso, A., et al. (2019). Sex-Dependent Dominance Maintains Migration Supergene in Rainbow Trout. Nat. Ecol. Evol. 3, 1731–1742. doi:10.1038/s41559-019-1044-6
R Core Team (2021). R: A Language and Environment for Statistical Computing [Online]. Vienna, Austria: R Foundation for Statistical Computing. Available: https://www.R-project.org (Accessed Dec. 17, 2021).
Schlotterer, C., Tobler, R., Kofler, R., and Nolte, V. (2014). Sequencing Pools of Individuals-Mining Genome-Wide Polymorphism Data without Big Funding. Nat. Rev. Genet. 15, 749–763. doi:10.1038/nrg3803
Schröder, B. (2016). The Multifaceted Roles of the Invariant Chain CD74 - More Than Just a Chaperone. Biochim. Biophys. Acta (BBA) - Mol. Cell Res. 1863, 1269–1281. doi:10.1016/j.bbamcr.2016.03.026
Semple, S. L., Heath, G., Christie, D., Braunstein, M., Kales, S. C., and Dixon, B. (2019). Immune Stimulation of Rainbow Trout Reveals Divergent Regulation of MH Class II-Associated Invariant Chain Isoforms. Immunogenetics 71, 407–420. doi:10.1007/s00251-019-01115-y
Song, Z., Zou, J., Wang, M., Chen, Z., and Wang, Q. (2022). A Comparative Review of Pyroptosis in Mammals and Fish. J. Inflamm. Res. 15, 2323–2331. doi:10.2147/jir.s361266
Starliper, C. E. (2011). Bacterial Coldwater Disease of Fishes Caused by Flavobacterium Psychrophilum. J. Adv. Res. 2, 97–108. doi:10.1016/j.jare.2010.04.001
Therneau, T. M. (2021). A Package for Survival Analysis in R. R Package Version 3.2-13. [Online] Available: https://CRAN.R-project.org/package=survival (Accessed Dec. 17, 2021).
Thompson, N. F., Anderson, E. C., Clemento, A. J., Campbell, M. A., Pearse, D. E., Hearsey, J. W., et al. (2020). A Complex Phenotype in Salmon Controlled by a Simple Change in Migratory Timing. Science 370, 609–613. doi:10.1126/science.aba9059
Vallejo, R. L., Leeds, T. D., Gao, G., Parsons, J. E., Martin, K. E., Evenhuis, J. P., et al. (2017a). Genomic Selection Models Double the Accuracy of Predicted Breeding Values for Bacterial Cold Water Disease Resistance Compared to a Traditional Pedigree-Based Model in Rainbow Trout Aquaculture. Genet. Sel. Evol. 49, 17. doi:10.1186/s12711-017-0293-6
Vallejo, R. L., Liu, S., Gao, G., Fragomeni, B. O., Hernandez, A. G., Leeds, T. D., et al. (2017b). Similar Genetic Architecture with Shared and Unique Quantitative Trait Loci for Bacterial Cold Water Disease Resistance in Two Rainbow Trout Breeding Populations. Front. Genet. 8, 156. doi:10.3389/fgene.2017.00156
Vallejo, R. L., Cheng, H., Fragomeni, B. O., Gao, G., Silva, R. M. O., Martin, K. E., et al. (2021). The Accuracy of Genomic Predictions for Bacterial Cold Water Disease Resistance Remains Higher Than the Pedigree-Based Model One Generation after Model Training in a Commercial Rainbow Trout Breeding Population. Aquaculture 545, 737164. doi:10.1016/j.aquaculture.2021.737164
Vallejo, R. L., Palti, Y., Liu, S., Evenhuis, J. P., Gao, G., Rexroad, C. E., et al. (2014b). Detection of QTL in Rainbow Trout Affecting Survival When Challenged with Flavobacterium Psychrophilum. Mar. Biotechnol. 16, 349–360. doi:10.1007/s10126-013-9553-9
Vallejo, R. L., Palti, Y., Liu, S., Marancik, D. P., and Wiens, G. D. (2014a). Validation of Linked QTL for Bacterial Cold Water Disease Resistance and Spleen Size on Rainbow Trout Chromosome Omy19. Aquaculture 432, 139–143. doi:10.1016/j.aquaculture.2014.05.003
Vallejo, R. L., Silva, R. M. O., Evenhuis, J. P., Gao, G., Liu, S., Parsons, J. E., et al. (2018). Accurate Genomic Predictions for BCWD Resistance in Rainbow Trout Are Achieved Using Low‐Density SNP Panels: Evidence that Long‐range LD is a Major Contributing Factor. J. Anim. Breed. Genet. 135, 263–274. doi:10.1111/jbg.12335
Veillette, A. (2006). Immune Regulation by SLAM Family Receptors and SAP-Related Adaptors. Nat. Rev. Immunol. 6, 56–66. doi:10.1038/nri1761
Wiens, G. D., Lapatra, S. E., Welch, T. J., Evenhuis, J. P., Rexroad, C. E., and Leeds, T. D. (2013a). On-Farm Performance of Rainbow Trout (Oncorhynchus Mykiss) Selectively Bred for Resistance to Bacterial Cold Water Disease: Effect of Rearing Environment on Survival Phenotype. Aquaculture 388-391, 128–136. doi:10.1016/j.aquaculture.2013.01.018
Wiens, G. D., Palti, Y., and Leeds, T. D. (2018). Three Generations of Selective Breeding Improved Rainbow Trout (Oncorhynchus Mykiss) Disease Resistance against Natural Challenge with Flavobacterium Psychrophilum during Early Life-Stage Rearing. Aquaculture 497, 414–421. doi:10.1016/j.aquaculture.2018.07.064
Wiens, G. D., Vallejo, R. L., Leeds, T. D., Palti, Y., Hadidi, S., Liu, S., et al. (2013b). Assessment of Genetic Correlation between Bacterial Cold Water Disease Resistance and Spleen Index in a Domesticated Population of Rainbow Trout: Identification of QTL on Chromosome Omy19. PLoS One 8, e75749. doi:10.1371/journal.pone.0075749
Keywords: rainbow trout, bacterial cold water disease, haplotype, SNP, MAS, QTL, whole genome sequencing (WGS)
Citation: Liu S, Martin KE, Gao G, Long R, Evenhuis JP, Leeds TD, Wiens GD and Palti Y (2022) Identification of Haplotypes Associated With Resistance to Bacterial Cold Water Disease in Rainbow Trout Using Whole-Genome Resequencing. Front. Genet. 13:936806. doi: 10.3389/fgene.2022.936806
Received: 31 March 2022; Accepted: 06 June 2022;
Published: 23 June 2022.
Edited by:
Nguyen Hong Nguyen, University of the Sunshine Coast, AustraliaReviewed by:
Antti Kause, Natural Resources Institute Finland (Luke), FinlandYulin Jin, Emory University, United States
Copyright © 2022 Liu, Martin, Gao, Long, Evenhuis, Leeds, Wiens and Palti. This is an open-access article distributed under the terms of the Creative Commons Attribution License (CC BY). The use, distribution or reproduction in other forums is permitted, provided the original author(s) and the copyright owner(s) are credited and that the original publication in this journal is cited, in accordance with accepted academic practice. No use, distribution or reproduction is permitted which does not comply with these terms.
*Correspondence: Sixin Liu, c2l4aW4ubGl1QHVzZGEuZ292