- 1Department of Orthopedic Surgery, The First Affiliated Hospital of Dalian Medical University, Dalian, China
- 2Dalian Medical University, Dalian, China
- 3College of Integrative Medicine, Dalian Medical University, Dalian, China
- 4Trauma and Tissue Repair Surgery Department, Dalian Municipal Central Hospital, Dalian, China
- 5Pelvic Floor Repair Center, The Affiliated Dalian Maternity Hospital of Dalian Medical University, Dalian, China
- 6Pelvic floor repair center, Dalian Women and Children Medical Center (Group), Dalian, China
Objectives: DFU is a serious chronic disease with high disability and fatality rates, yet there is no completely effective therapy. While ferroptosis is integrated to inflammation and infection, its involvement in DFU is still unclear. The study aimed to identify ferroptosis-related genes in DFU, providing potential therapeutic targets.
Methods: In the GEO database, two DFU microarray datasets (GSE147890 and GSE80178) were collected. WGCNA was conducted to identify the modular genes most involved in DFU. Subsequently, enrichment analysis and PPI analysis were performed. To yield the DFU-associated ferroposis genes, the ferroposis genes were retrieved from the FerrDb database and overlapped with the modular genes. Eventually, an optimal DFU prediction model was created by combining multiple machine learning algorithms (LASSO, SVM-RFE, Boruta, and XGBoost) to detect ferroposis genes most closely associated with DFU. The accuracy of the model was verified by utilizing external datasets (GSE7014) based on ROC curves.
Results: WGCNA yielded seven modules in all, and 1223 DFU-related modular genes were identified. GO analysis revealed that inflammatory response, decidualization, and protein binding were the most highly enriched terms. These module genes were also enriched in the ErbB signaling, IL-17 signaling, MAPK signaling, growth hormone synthesis, secretion and action, and tight junction KEGG pathways. Twenty-five DFU-associated ferroposis genes were obtained by cross-linking with modular genes, which could distinguish DFU patients from controls. Ultimately, the prediction model based on machine learning algorithms was well established, with high AUC values (0.79 of LASSO, 0.80 of SVM, 0.75 of Boruta, 0.70 of XGBoost). MAFG and MAPK3 were identified by the prediction model as the most highly associated ferroposis-genes in DFU. Furthermore, the external dataset (GSE29221) validation revealed that MAPK3 (AUC = 0.81) had superior AUC values than MAFG (AUC = 0.62).
Conclusion: As the most related ferroptosis-genes with DFU, MAFG and MAPK3 may be employed as potential therapeutic targets for DFU patients. Moreover, MAPK3, with higher accuracy, could be the more potential ferroptosis-related biomarker for further experimental validation.
Introduction
Diabetic foot ulcer (DFU), one of the most devastating consequences of diabetes, is a major health concern that places a serious financial, physical, and mental burden on sufferers. Extensive diabetic alterations, such as neuropathy and vascular disease, frequently aggravate the course of DFU (Teena et al., 2020). Even with recent advances in DFU treatment, a considerable proportion of individuals experience chronic trauma as a result of an irreversible process (Chen et al., 2016). Thus, it is critical to investigate the molecular mechanisms of DFU and inhibit the creation of chronic trauma in order to improve the treatment efficiency and prognosis of DFU patients.
Ferroptosis is an iron-dependent programmed cell death that differs from apoptosis, pyroptosis, and necrosis, being characterized by excessive iron accumulation and elevated lipid peroxidation (Stockwell et al., 2017). Induction of ferroptosis in cancer cells has been proven to be a viable alternative treatment for tumor disorders that are resistant to conventional treatments (Hassannia et al., 2019). Since ferroptosis is reported to be correlated with a variety of disorders, it has the potential to play a role in the etiology of DFU. However, the particular regulation mechanism of ferroptosis in DFU is unknown and requires further investigation.
In the current study, we employed WGCNA to identify potential genes in ferroptosis genes linked to specific genes closely associated to DFU. Finally, utilizing multiple machine learning methods, a robust prediction model for identifying DFU patients was established and validated by employing an external DFU dataset. We investigated the genetic connection among ferroptosis with DFU. The DFU-related ferroptosis genes could be employed as biomarkers for disease diagnosis and therapy monitoring, as well as a reference for early therapeutic targets for DFU.
Materials and methods
Microarray data download and data preprocessing
We used the “GEOquery” package of R software (version 4.1.2, http://r-project.org/) to download the DFU sample source from the Gene Expression Omnibus (GEO) (https://www.ncbi.nlm.nih.gov/geo/) database. The DFU-related expression profiles GSE147890, GSE80178, GSE7014, and GSE29221 were all from Homo sapiens (Table 1). The GSE147890 and GSE80178 gene expression matrices were then combined to remove inter-batch differences using the “sva” package and to drop samples where inter-group differences could not be removed between groups (Deng et al., 2020). A flow diagram of the study is shown in Figure 1.
Weighted gene co-expression network analysis.
Firstly, a soft threshold for network construction was selected to construct a gene co-expression network by an R package called “weighted gene co-expression network analysis (WGCNA)” (Wang et al., 2022). The adjacency matrix was constructed by weighting coefficients, and the adjacency matrix was a continuous value between 0 and 1, which conformed to the power-law distribution and was closer to the real biological state. Secondly, a scale-free network was constructed to hierarchically cluster the modules, identify the gene co-expression modules, and assign them to different colors for visualization. Finally, the correlation between sample phenotypes and each module was assessed by Pearson correlation analysis, and the modules with the closest DFU were screened out. The genes most closely associated with DFU were selected according to the appropriate Gene Significance (GS) for DFU and Module Membership (MM) in this module.
DFU-related gene analysis and enrichment analysis.
The “ComplexHeatmap” package was used to heat map DFU-related genes and assess the efficiency of genes by principal component analysis (PCA). The Gene Ontology (GO) analysis, including biological processes (BP), molecular functions (MF), and cellular components (CC), was a widely used functional enrichment method. Kyoto Encyclopedia of Genes and Genomes (KEGG) was a database that stores a large number of biological functions, genomes, chemicals, and drug-related pathways. The Database for Annotation, Visualization and Integrated Discovery (DAVID, https://david.ncifcrf.gov/) (Huang et al., 2009a; Huang et al., 2009b) database was used for GO and KEGG enrichment analysis of DFU-related genes (Zheng et al., 2020), and the results were visualized using the “ggplot2” package.
Protein-protein interaction creation and identification of hub genes.
Search Tools for the Retrieval of Interacting Genes (STRING, http://string-db.org) (Szklarczyk et al., 2021) was an online tool for predicting the protein-protein interaction (PPI), which was implemented to construct a PPI network with a confidence score of >0.40 and visualize the network model with Cytoscape V3.8.0 software. Nine algorithms (Betweenness, Radiality, MNC, EPC, DMNC, MCC, Degree, Clustering Coefficient, Closeness) in the CytoHubba plugin were used to evaluate the importance of each node and to select the common genes in the top 30 nodes of each algorithm as hub genes (Liu et al., 2020). Subsequently, enrichment analysis of the hub gene including GO, KEGG, and Disease Ontology (DO) was performed by the R package “clusterProfiler” and FDR < 0.05 was considered significant.
Identification and analysis of ferroptosis-related genes.
Ferroptosis-related genes were obtained for further analysis according to the FerrDb database (http://www.zhounan.org/ferrdb/) (Zhou and Bao, 2020) (Table 2), an artificial database collection of ferroptosis-related markers and diseases. Genes in the intersection of ferroptosis-related genes and those in the modules most closely associated with DFU for WGCNA were taken using the online tool Bioinformatics & Evolutionary Genomics (http://bioinformatics.psb.ugent.be/webtools/Venn/), and genes in the intersection were considered to be specifically expressed in DFU patients with ferroptosis-related genes. Subsequently, correlations between ferroptosis-related genes were assessed using the “corrplot” package. Heat maps and PCA were visualized using R software, respectively.
Robust predictive model built using multiple machine learning methods
The R packages “glmnet,” “caret,” “Boruta” and “XGBoost” were used to build a machine learning model (Huang et al., 2022). The least absolute shrinkage and selection operator (LASSO), Support Vector Machine Recursive Feature Elimination (SVM-RFE), Boruta, and extreme gradient boosting (XGBoost) analyses were performed on the entire dataset to screen for key ferroptosis-related genes. In addition, the GSE7014 dataset was used as an external dataset to validate the prediction model, and the prediction efficiency of the model was evaluated by receiver operating characteristic (ROC) curves. Consequently, the intersection genes between genes obtained by model analysis and hub genes were considered the key ferroptosis-related genes associated with DFU. Finally, the “pROC” package was employed to assess the efficiency of key ferroptosis-related genes as therapeutic markers in the GSE29221 external dataset.
Results
Data preprocessing
The two data sets GSE147890 and GSE80178 were combined and normalized to remove the batch differences and remove the abnormal samples GSM2114232, GSM2114233 (Figure 2). In addition, the two external datasets GSE7014 and GSE29221 were normalized with an external validation dataset using the “limma” package (Supplementary Figure S1).
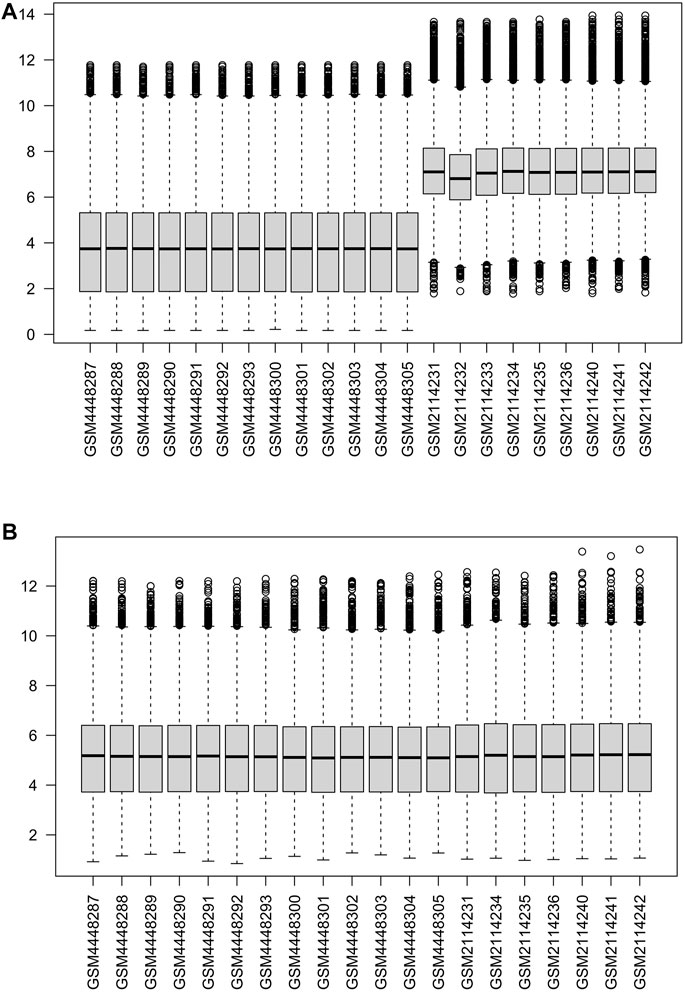
FIGURE 2. Normalization of gene expression data in samples (A). Before normalization (B). After normalization.
Weighted gene co-expression network analysis and identification of core modules.
In order to construct the scale-free network, we chose 20 as a soft threshold (R2 = 0.85) (Figure 3A). Next, the adjacency matrix and the topological overlap matrix were constructed according to the expression matrix. Based on the correlation clustering, the module signature genes that can represent the overall gene expression level of each module were then calculated. A total of seven signature modules were identified and labeled with different colors (Figure 3B). Subsequently, we analyzed the correlation between the modules and the sample phenotypes, and we found the largest correlation among the blue module and DFU (r = 0.79, P = 3e-05) (Figure 3C). The correlation between genes in the blue module and DFU genes was cor = 0.76, p < 1e-200; 146 genes most associated with DFU were selected from this module based on GS = 0.7 and MM = 0.8 (Figure 3D).
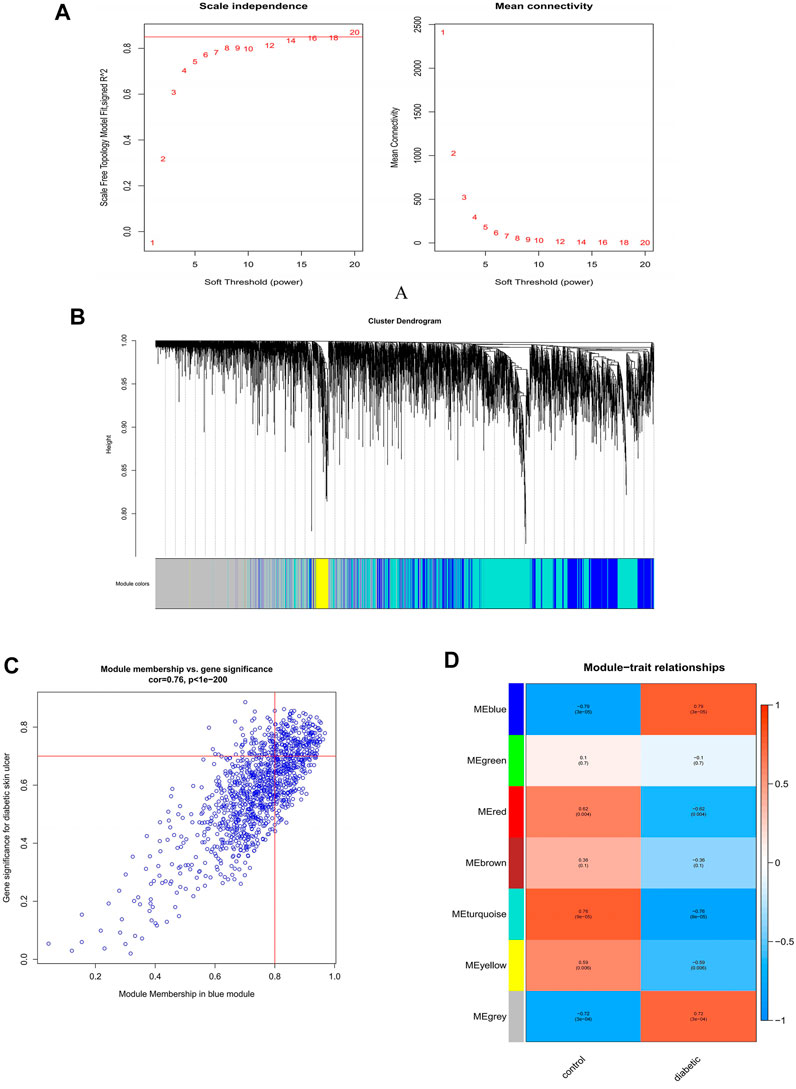
FIGURE 3. Construction and module analysis of weighted gene co-expression network analysis (WGCNA). (A) Network topology analysis under various soft-threshold powers. Left: Analysis of the scale-free index for various soft-threshold powers (β). Right: Analysis of the mean connectivity for various soft-threshold powers. (B) Identification of co-expression gene modules. The branches of the dendrogram cluster into seven modules and each one was labeled in a unique color. (C) A heatmap showing the correlation between each module eigengene and phenotype. (D) The relevance of members in the blue module and DFU.
DFU-related gene analysis and enrichment analysis
The 146 DFU-related genes were presented in a heat map to reveal that they were significantly associated with DFU compared to control samples (Figure 4A). PCA indicated that these genes allowed us to distinguish DFU samples from control samples (Figure 4B). The results of the significant GO enrichment analysis of 146 DFU-related genes, including BP, CC, MF, were illustrated in Figure 4C. The results of GO analysis were mainly related to inflammation, protein binding, and kinase activity, such as inflammatory response, coronification, decidualization, protein binding, protein serine/threonine kinase activity, cytosol (Supplementary Table S1). KEGG enrichment analysis demonstrated that these genes were mainly enriched in the ErbB signaling pathway, IL-17 signaling pathway, MAPK signaling pathway, growth hormone synthesis, secretion and action, and other pathways related to proliferation and differentiation (Figure 4D). Detailed results of the KEGG analysis are shown in Supplementary Table S2. The results of the enrichment analysis of DFU-related genes suggested that these genes were of interest for our study and could be further investigated.
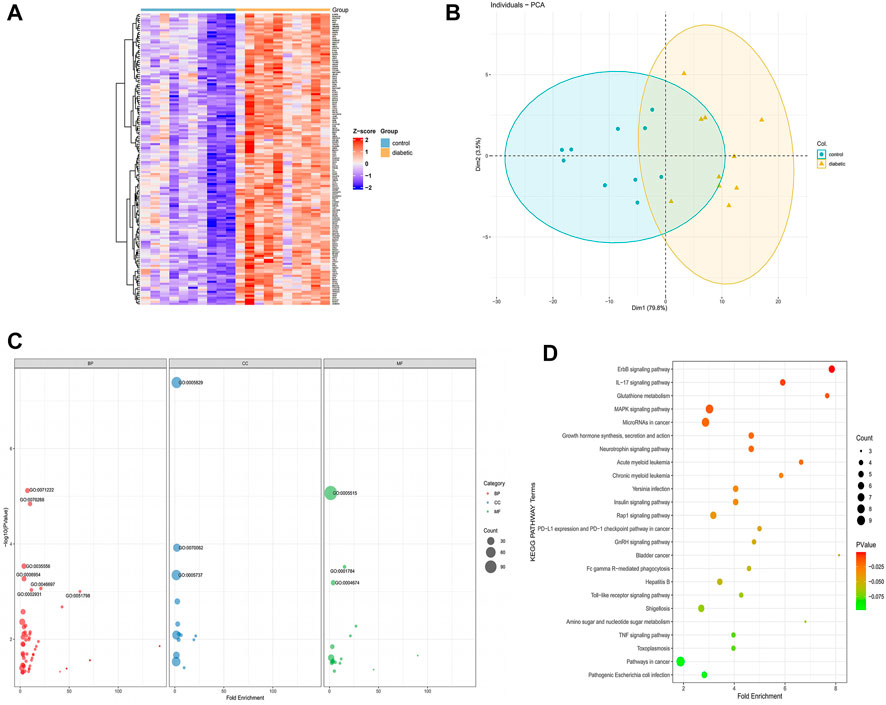
FIGURE 4. DFU-related genes analysis (A). The heat map of the DFU-related gene expression between DFU samples and control samples (B) PCA of DFU-related genes. Principal component 1 (PC1) and principal component 2 (PC2) are used as the X-axis and Y-axis, respectively, to draw the scatter diagram, where each point represents a sample (C). GO analysis divided DFU-related genes into three groups as follows: biological processes (red), cell components (blue), and molecular functions (green). The size of the dot represents the number of gene counts (D) KEGG pathway enrichment analysis of DFU-related genes. The size of the dot represents the number of gene counts, and the color of the dot represents the P-value.
Protein-protein interaction analysis and identification of hub genes
The PPI data of 146 DFU-related genes were obtained from the STRING database, then the results were visualized and presented using Cytoscape V3.8.0 (Figure 5A). A total of 14 genes were identified as hub genes based on nine algorithms (Betweenness, Radiality, MNC, EPC, DMNC, MCC, Degree, Clustering Coefficient, Closeness), which were common to the top 30 genes in these algorithms (Figure 5B). The PPI network of 14 hub genes was presented in Figure 5C. Subsequently, we used the “ggcorrplot” package to plot the correlation heat map between hub genes, and the results indicated that the genes were positively correlated with each other (Figure 5D).
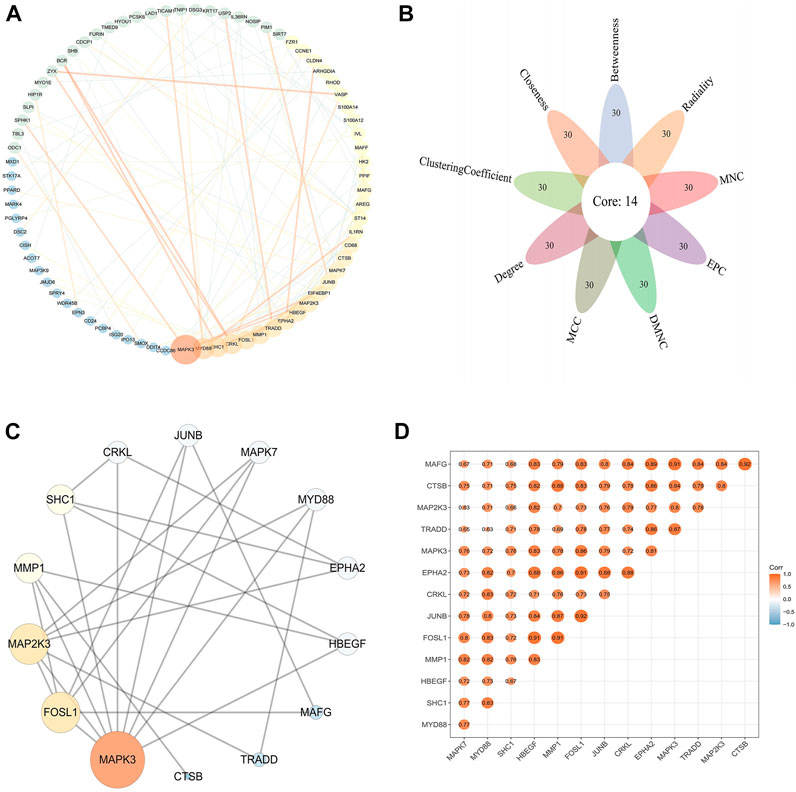
FIGURE 5. PPI network and hub genes in DFU (A) PPI network of DFU-related genes. The size and color of the nodes and edges corresponding to each gene were determined according to the degree and combine-score of interaction, respectively. Color gradients represent the variation of the degrees and combine-score of each gene from high to low (B). Identification of the hub gene. The hub genes were identified by nine algorithms based on CytoHubba (C). PPI network of hub genes. The size and color of the nodes corresponding to each gene were determined according to the degree of interaction. Color gradients represent the variation of the degrees of each gene from high to low (D). Correlation heat map of hub genes. The darker the color, the stronger the correlation.
Enrichment analysis of hub genes
To further analyze the association between hub genes and DFU, the GO, KEGG, and DO enrichment analysis were performed on the hub genes. The results of GO analysis showed that hub genes were mainly related to decidualization, peptidyl-tyrosine phosphorylation, and extrinsic components of the synaptic membrane. Phosphorylated amino acid binding (Figures 6A–C). The DO analysis results were illustrated in Figure 6D. The hub genes were enriched for diseases including bacterial infectious disease, parasitic infectious disease, musculoskeletal system cancer, and other endothelial, infection-related diseases. Moreover, we also submitted the hub genes to KEGG pathway enrichment analysis. As shown in Figures 6E–H, the involved pathways were mainly enriched in the MAPK signaling pathway, the IL-17 signaling pathway, and growth hormone synthesis, secretion and action. The detailed analysis results of GO, DO, and KEGG are shown in Supplementary Table S3.
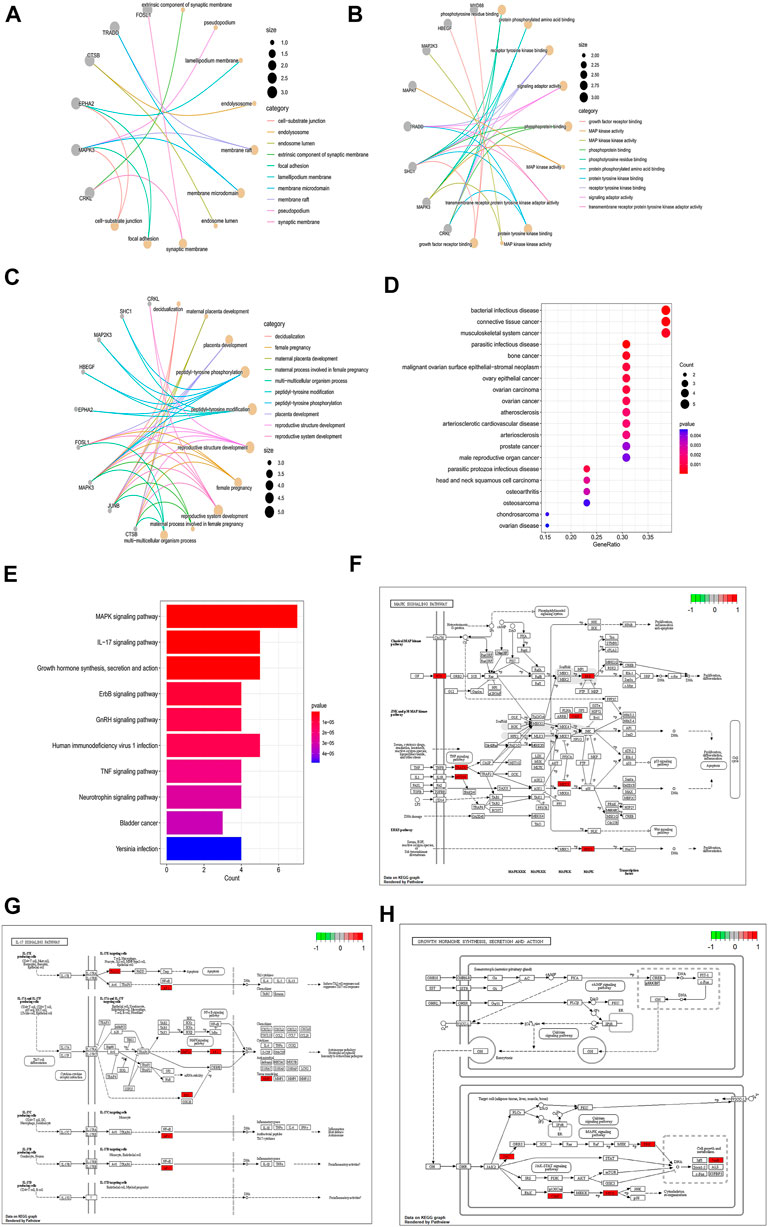
FIGURE 6. Enrichment analysis of hub genes. (A–C) GO enrichment analysis of hub genes (A: BP, (B) CC, (C) MF). The size of the node respondents the number of the gene counts. (D) DO enrichment analysis of hub genes; the size of the dot represents the number of gene counts, and the color of the dot represents the P-value. (E) KEGG enrichment analysis of hub genes; the color of the bar res represents the P-value.(F–H). MAPK signaling pathway; IL-17 signaling pathway; MAPK signaling pathway; Growth hormone synthesis, secretion and action; Red indicates high expression in the pathway, and green indicates low expression in the pathway.
DFU-related module genes with ferroptosis-related genes
We overlapped DFU-related genes extracted from the blue module of WGCNA analysis with ferroptosis-related genes extracted from the FerrDb database, and 25 overlapping genes were obtained, namely DFU-related ferroptosis genes, as presented by the Venn diagram (Figure 7A). In addition, PCA revealed that these genes enabled us to effectively distinguish DFU samples from control samples (Figure 7B). Moreover, the heat map drawn could clearly visualize the differences in the expression of these genes among the different samples (Figure 7C). Finally, the correlation network diagram revealed significant inter-correlation between the 25 genes (Figure 7D).
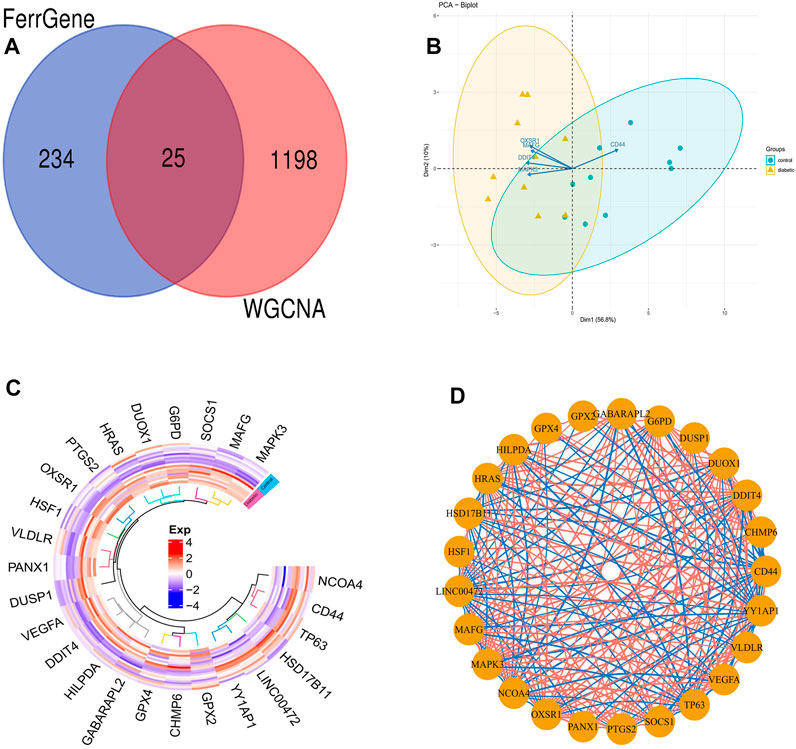
FIGURE 7. DFU-related ferroptosis genes (A). Venn diagram showing the numbers of overlapped genes between DFU-related module genes and ferroptosis-related genes (B). PCA of DFU-related ferroptosis genes showing good differentiation power (C). The heat map of the DFU-related ferroptosis genes expression between DFU samples and control samples (D). The correlation network of DFU-related ferroptosis genes. The darker the color of the edge, the stronger the correlation.
Construction and validation of an optimal ferroptosis-related dfu prediction model
Four validated machine learning algorithms (LASSO, SVM-RFE, Boruta, XGBoost) were applied to identify key genes from 25 ferroptosis genes associated with DFU, yielding 8,12,8,11 genes, respectively (Figures 8A–D). Subsequently, we evaluated the efficiency of the four supervised machine learning algorithms using ROC curves based on the external dataset GSE7014, and the AUC values of all four algorithms were greater than 0.7, considering the prediction model results credible (Figure 8E).
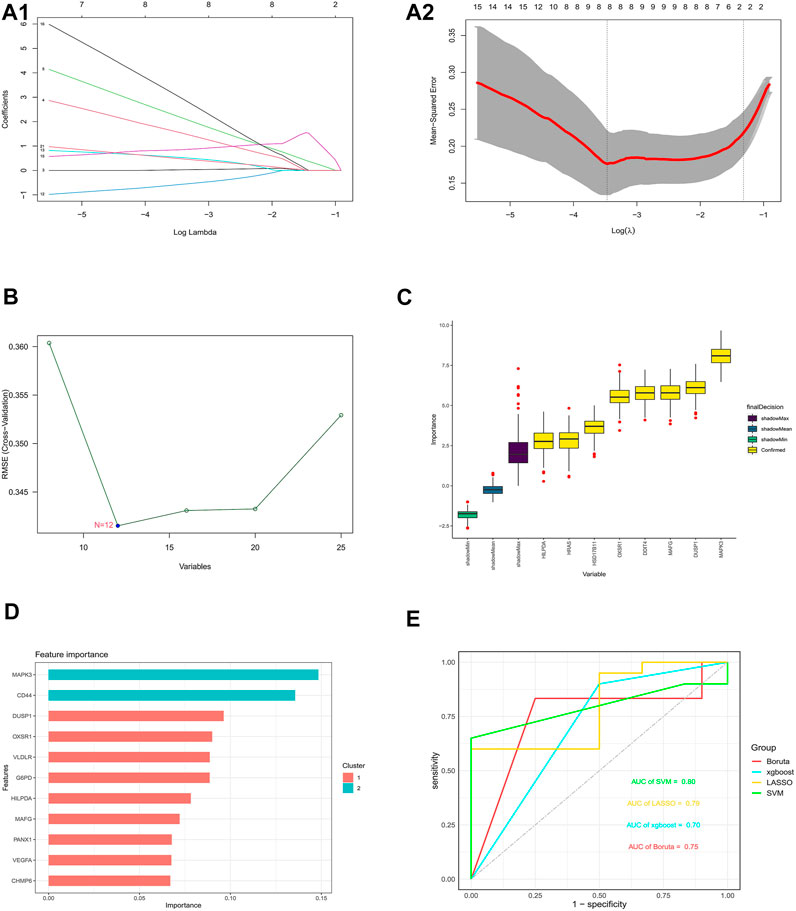
FIGURE 8. Construction and validation of an optimal ferroptosis-related DFU prediction model (A) 8 DFU-related ferroptosis genes obtained using the LASSO algorithm based on the minimum lambda. (B) 12 DFU-related ferroptosis genes obtained using the SVM algorithm. (C) 8 DFU-related ferroptosis genes obtained using the Boruta algorithm. (D) 11 DFU-related ferroptosis genes obtained using the XGBoost algorithm (E). Applying external dataset to validate four predictive models.
Key ferroptosis genes associated with DFU
Genes that commonly belonged to the key ferroptosis genes identified by the four machine learning algorithms and 14 hub genes were considered to be the ferroptosis genes most closely associated with DFU, resulting in two genes (MAFG and MAPK3) (Figure 9A). Furthermore, the box plot results demonstrated that MAPK3 and MAFG were both highly expressed in DFU patients and low in controls (Figure 9B). Finally, ROC curves were plotted based on the external validation set data to verify the potential of these two genes as therapeutic targets for DFU patients. As shown in Figure 9C, the AUC values of both MAPK3 and MAFG exceeded 0.6, with MAPK3 (AUC >0.8) being more effective than MAFG as a DFU therapeutic target.
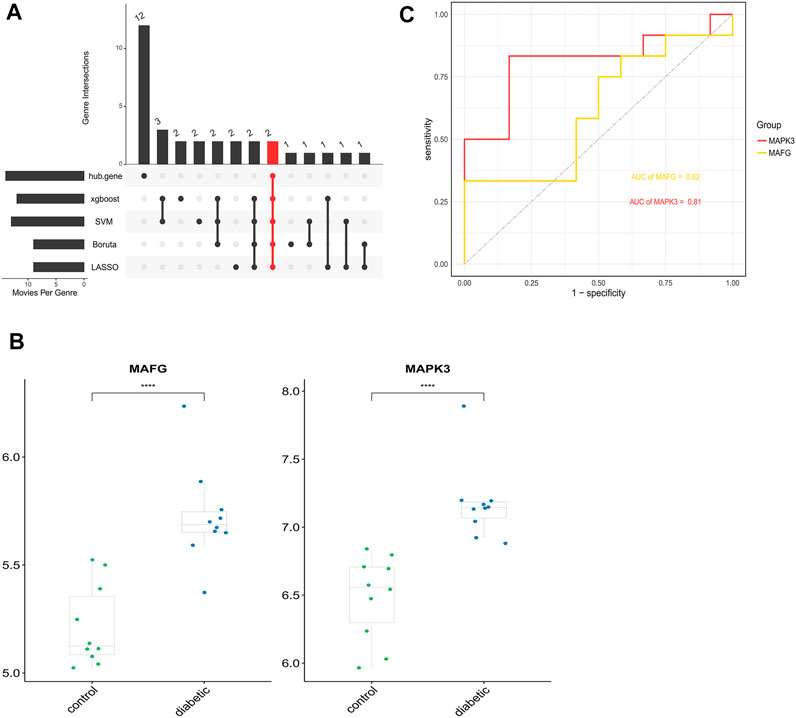
FIGURE 9. Identification and analysis of key DFU-Related ferroptosis genes (A). Two key DFU-related ferroptosis genes were identified by four machine learning algorithms and f hub genes (B). Expression of the 2 key DFU-related ferroptosis genes in DFU samples and control samples; the differences were statistically significant (****: p < 0.0001) (C). Applying external dataset to validate 2 key DFU-related ferroptosis genes.
Discussion
DFU is the leading cause of death in the diabetic population, so an urgent need exists to find molecular therapeutic targets with specificity that can help improve the prognosis of patients and reduce mortality. This study applied WGCNA to identify the most relevant model for DFU. Further follow-up analysis was performed on 146 genes in the model based on the set thresholds. Functional annotation identified the primary involvement of these genes in cell proliferation, differentiation, and multiple classical signaling regulatory pathways, including cornification, protein binding, growth hormone synthesis, secretion, and action, and the MAPK signaling pathway, indicating that the main biological processes involved in the progression of DFU were cell proliferation and differentiation. In addition, we extracted 14 potential hub genes that contribute most to the diagnosis of DFU. Further enrichment analysis revealed that potential hub genes were also mainly enriched in pathways related to cell proliferation and differentiation.
Ferroptosis is a form of iron-dependent cell death driven by intracellular iron overload and lipid peroxidation (Kuang et al., 2020), and its role in physiological and pathogenic processes has been extensively studied. It has been reported that high concentrations of serum iron may be a risk factor for the development of type 2 diabetes, yet the exact mechanism is not clear (Sha et al., 2021). The relationship between ferroptosis and DFU has rarely been reported, but the correlation does exist. Inhibitors related to ferroptosis had been shown to play a protective role in the diabetic foot (He et al., 2022). In addition, studies had shown that paeoniflorin (PF) has the ability to promote DFU wound healing by activating the NRF2 related ferroptosis pathway and the NRF2/HO-1 pathway (Sun et al., 2020). Therefore, exploring the pathogenesis of DFU due to ferroptosis may provide new therapeutic targets for the treatment of DFU and other chronic wounds. Our study obtained 25 ferroptosis genes associated with DFU through the WGCNA and FerrDb databases.
Machine learning has a wide range of applications in the biomedical field, demonstrating excellent efficiency in clinical diagnosis and optimal treatment (Huang et al., 2022). Subsequently, we applied machine learning algorithms to screen key ferroptosis genes from ferroptosis-related genes associated with DFU, and finally identified two ferroptosis-related genes (MAFG and MAPK3) as potentially effective diagnostic molecules for DFU.
It has been clearly reported that MAF BZIP Transcription Factor G (MAFG) has a promising potential as a potential prognostic biomarker in non-small cell lung cancer. MAFG also can play a role as a molecular biomarker for tumor-targeted therapy to relieve cisplatin resistance of tumor cancer cells, improving therapeutic and prognostic efficiency (Vera-Puente et al., 2018). However, there are few studies related to MAFG in the pathogenesis of DFU progression, and only a few reports suggest that MAFG loss improves glucose metabolism and insulin sensitivity, thus protecting from hyperglycemia (Pradas-Juni et al., 2020). Our results demonstrated that MAFG was highly expressed in DFU samples compared to controls, and we could hypothesize that the high expression of MAFG had a detrimental effect in DFU. Nevertheless, the specific mechanism of MAFG in DFU is unclear and further relevant studies are required to determine whether it can be used as the therapeutic target.
External dataset validation was employed to assess the efficiency of both genes as therapeutic target, suggesting that the accuracy and specificity of MAPK3 was superior to that of MAFG. (Mitogen-Activated Protein Kinase 3) MAPK3, also known as extracellular signal-regulated kinase 1 (ERK1), is a protein-coding gene that encodes a protein belonging to the MAP kinase family (www.ncbi.nlm.nih.gov/gene/), which are involved in a variety of cellular processes such as proliferation, inflammation, and cellular metabolism through phosphorylation of their target proteins (Kassouf and Sumara, 2020). In this study, high expression of MAPK3 played a positive role in variety of the pathways associated with cell proliferation and differentiation, such as MAPK signaling pathway, growth hormone synthesis, secretion and action, ErbB signaling pathway, and GnRH signaling pathway. However, some studies have shown that overactivation of ERK1/2 is associated with deleterious effects during obesity and diabetes. The absence of ERK1/2 in the liver improves systemic insulin and glucose tolerance (Jiao et al., 2013). It has been recently shown that high glucose-activated ERK1/2 increases matrix metalloproteinase 9 (MMP9) expression in the skin and contributes to the delayed healing of DFU wounds (Lang et al., 2021). Additionally, reactive oxygen species (ROS)-triggered activation of ERK1/2 is involved in the NETosis process, and diabetes-induced neutrophil NETosis disrupts wound healing through neutrophil extracellular traps (NETs) (Yang et al., 2019). Accordingly, we deduced that high MAPK3 expression has a delayed wound healing effect on DFU patients. Considering the adverse effects of MAPK3, corresponding new molecular therapeutic strategies should also be developed.
However, there are limitations to this study. Firstly, the machine learning prediction model in the external validation cohort affects the accuracy due to the small sample size, leading to misdiagnosis and missed diagnoses. Thus, the larger DFU sample size can improve the prediction accuracy. Secondly, the ferroptosis-related biomarkers identified in this study that have the potential to be therapeutic targets for DFU require further literature support and basic experimental validation. FerrDb database is constantly being updated, and more ferroptosis-related genes are yet to be discovered.
Data availability statement
The original contributions presented in the study are included in the article/Supplementary Material, further inquiries can be directed to the corresponding authors.
Author contributions
XW, GJ and JZ collected the original data, finished the analysis and drafted the initial version. DL helped revise the manuscript. ML and XQ offered constructive comments on experimental studies. SW, ML and XQ provided the funding. SW designed and conceptualized the study, put forward many constructive comments for the final version and supervised the study. The final version ofthe manuscript was approved by all authors.
Funding
This study was supported by National Natural Science Foundation of China (82074426,82104864,81573734), Natural Science Foundation of Liaoning Province (2021-BS-215), Liaoning Revitalization Talents Program (XLYC1802014), Liaoning Key Research and Development Planning Project (2017226015), Liaoning BaiQianWan Talents Program, Dalian research project of traditional Chinese Medicine (20Z12001), Distinguished Professor Project of Liaoning Province, Dalian outstanding youth science and technology talent project (2015R003).
Acknowledgments
We thank all the participants who supported our study. In particular, thanks to the GEO database and the FerrDb database for the analytical data.
Conflict of interest
The authors declare that the research was conducted in the absence of any commercial or financial relationships that could be construed as a potential conflict of interest.
Publisher’s note
All claims expressed in this article are solely those of the authors and do not necessarily represent those of their affiliated organizations, or those of the publisher, the editors and the reviewers. Any product that may be evaluated in this article, or claim that may be made by its manufacturer, is not guaranteed or endorsed by the publisher.
Supplementary material
The Supplementary Material for this article can be found online at: https://www.frontiersin.org/articles/10.3389/fgene.2022.944425/full#supplementary-material
References
Chen, X., Zhou, W., Zha, K., Liu, G., Yang, S., Ye, S., et al. (2016). Treatment of chronic ulcer in diabetic rats with self assembling nanofiber gel encapsulated-polydeoxyribonucleotide. Am. J. Transl. Res. 8, 3067–3076.
Deng, Y.-J., Ren, E.-H., Yuan, W.-H., Zhang, G.-Z., Wu, Z.-L., and Xie, Q.-Q. (2020). Grb10 and E2f3 as diagnostic markers of osteoarthritis and their correlation with immune infiltration. Diagnostics 10, 171. doi:10.3390/diagnostics10030171
Hassannia, B., Vandenabeele, P., and Vanden Berghe, T. (2019). Targeting ferroptosis to iron out cancer. Cancer Cell 35, 830–849. doi:10.1016/j.ccell.2019.04.002
He, J., Li, Z., Xia, P., Shi, A., FuChen, X., Zhang, J., et al. (2022). Ferroptosis and ferritinophagy in diabetes complications. Mol. Metab. 60, 101470. doi:10.1016/j.molmet.2022.101470
Huang, D. W., Sherman, B. T., and Lempicki, R. A. (2009a). Bioinformatics enrichment tools: Paths toward the comprehensive functional analysis of large gene lists. Nucleic Acids Res. 37. doi:10.1093/nar/gkn923
Huang, D. W., Sherman, B. T., and Lempicki, R. A. (2009b). Systematic and integrative analysis of large gene lists using david Bioinformatics resources. Nat. Protoc. 4, 44–57. doi:10.1038/nprot.2008.211
Huang, D., Zheng, S., Liu, Z., Zhu, K., Zhi, H., and Ma, G. (2022). Machine learning revealed ferroptosis features and A novel ferroptosis-based classification for diagnosis in acute myocardial infarction. Front. Genet. 13, 813438. doi:10.3389/fgene.2022.813438
Jiao, P., Feng, B., Li, Y., He, Q., and Xu, H. (2013). Hepatic erk activity plays A role in energy metabolism. Mol. And Cell. Endocrinol. 375, 157–166. doi:10.1016/j.mce.2013.05.021
Kassouf, T., and Sumara, G. (2020). Impact of conventional and atypical mapks on the development of metabolic diseases. Biomolecules 10. doi:10.3390/biom10091256
Kuang, F., Liu, J., Tang, D., and Kang, R. (2020). Oxidative damage and antioxidant defense in ferroptosis. Front. Cell Dev. Biol. 8, 586578. doi:10.3389/fcell.2020.586578
Lang, J., Yang, C., Liu, L., Li, L., Wu, L., Liu, Y., et al. (2021). High glucose activates erk1/2 to stabilize Ap1 and increase Mmp9 expression in diabetic foot ulcers. Exp. Cell Res. 403, 112550. doi:10.1016/j.yexcr.2021.112550
Liu, B., Li, A., Wang, H., Wang, J., Zhai, G., Ma, H., et al. (2020). Exploring the key genes and pathways in the formation of corneal scar using Bioinformatics analysis. Biomed. Res. Int. 2020, 6247489. doi:10.1155/2020/6247489
Pradas-Juni, M., Hansmeier, N. R., Link, J. C., Schmidt, E., Larsen, B. D., Klemm, P., et al. (2020). A mafg-lncrna Axis links systemic nutrient abundance to hepatic glucose metabolism. Nat. Commun. 11, 644. doi:10.1038/s41467-020-14323-y
Sha, W., Hu, F., Xi, Y., Chu, Y., and Bu, S. (2021). Mechanism of ferroptosis and its role in type 2 diabetes mellitus. J. Diabetes Res. 2021, 9999612. doi:10.1155/2021/9999612
Stockwell, B. R., Friedmann Angeli, J. P., Bayir, H., Bush, A. I., Conrad, M., Dixon, S. J., et al. (2017). Ferroptosis: A regulated cell death nexus linking metabolism, redox biology, and disease. Cell 171, 273–285. doi:10.1016/j.cell.2017.09.021
Sun, X., Wang, X., Zhao, Z., Chen, J., Li, C., and Zhao, G. (2020). Paeoniflorin accelerates foot wound healing in diabetic rats though activating the Nrf2 pathway. Acta Histochem. 122, 151649. doi:10.1016/j.acthis.2020.151649
Szklarczyk, D., Gable, A. L., Nastou, K. C., Lyon, D., Kirsch, R., Pyysalo, S., et al. (2021). The string database in 2021: Customizable protein-protein networks, and functional characterization of user-uploaded gene/measurement sets. Nucleic Acids Res. 49, D605–D612. doi:10.1093/nar/gkaa1074
Teena, R., Dhamodharan, U., Ali, D., Rajesh, K., and Ramkumar, K. M. (2020). Genetic polymorphism of the Nrf2 promoter region (Rs35652124) is associated with the risk of diabetic foot ulcers. Oxid. Med. Cell Longev. 2020, 9825028. doi:10.1155/2020/9825028
Vera-Puente, O., Rodriguez-Antolin, C., Salgado-Figueroa, A., Michalska, P., Pernia, O., Reid, B. M., et al. (2018). Mafg is A potential therapeutic target to restore chemosensitivity in cisplatin-resistant cancer cells by increasing reactive oxygen species. Transl. Res. J. Laboratory And Clin. Med. 200. doi:10.1016/j.trsl.2018.06.005
Wang, Y., Chen, G., and Shao, W. (2022). Identification of ferroptosis-related genes in alzheimer's disease based on bioinformatic analysis. Front. Neurosci. 16, 823741. doi:10.3389/fnins.2022.823741
Yang, C.-T., Chen, L., Chen, W.-L., Li, N., Chen, M.-J., Li, X., et al. (2019). Hydrogen sulfide primes diabetic wound to close through inhibition of netosis. Mol. And Cell. Endocrinol. 480, 74–82. doi:10.1016/j.mce.2018.10.013
Zheng, L., Dou, X., Song, H., Wang, P., Qu, W., and Zheng, X. (2020). Bioinformatics analysis of key genes and pathways in hashimoto thyroiditis tissues. Biosci. Rep. 40. doi:10.1042/BSR20200759
Keywords: DFU, WGCNA, ferroptosis, machine learning, prediction model
Citation: Wang X, Jiang G, Zong J, Lv D, Lu M, Qu X and Wang S (2022) Revealing the novel ferroptosis-related therapeutic targets for diabetic foot ulcer based on the machine learning. Front. Genet. 13:944425. doi: 10.3389/fgene.2022.944425
Received: 15 May 2022; Accepted: 12 September 2022;
Published: 26 September 2022.
Edited by:
Bruna De Felice, University of Campania Luigi Vanvitelli, ItalyReviewed by:
Nan Zhou, Guangzhou Medical University, ChinaYunbin Xiao, Hunan Children’s Hospital, China
Copyright © 2022 Wang, Jiang, Zong, Lv, Lu, Qu and Wang. This is an open-access article distributed under the terms of the Creative Commons Attribution License (CC BY). The use, distribution or reproduction in other forums is permitted, provided the original author(s) and the copyright owner(s) are credited and that the original publication in this journal is cited, in accordance with accepted academic practice. No use, distribution or reproduction is permitted which does not comply with these terms.
*Correspondence: Ming Lu, dllm@2008.sina.com; Shouyu Wang, wangshouyu666@126.com; Xueling Qu, quxuelingmm@sina.com
†These authors have contributed equally to this work and share first authorship